Have a language expert improve your writing
Run a free plagiarism check in 10 minutes, generate accurate citations for free.
- Knowledge Base
Methodology
- Sampling Methods | Types, Techniques & Examples
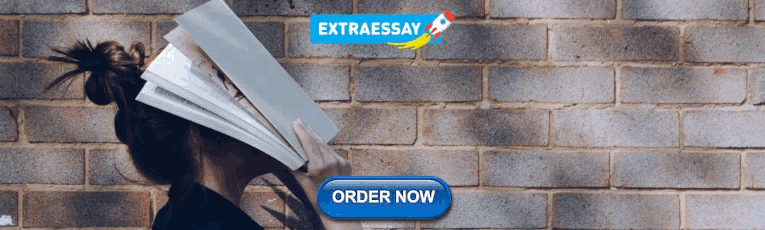
Sampling Methods | Types, Techniques & Examples
Published on September 19, 2019 by Shona McCombes . Revised on June 22, 2023.
When you conduct research about a group of people, it’s rarely possible to collect data from every person in that group. Instead, you select a sample . The sample is the group of individuals who will actually participate in the research.
To draw valid conclusions from your results, you have to carefully decide how you will select a sample that is representative of the group as a whole. This is called a sampling method . There are two primary types of sampling methods that you can use in your research:
- Probability sampling involves random selection, allowing you to make strong statistical inferences about the whole group.
- Non-probability sampling involves non-random selection based on convenience or other criteria, allowing you to easily collect data.
You should clearly explain how you selected your sample in the methodology section of your paper or thesis, as well as how you approached minimizing research bias in your work.
Table of contents
Population vs. sample, probability sampling methods, non-probability sampling methods, other interesting articles, frequently asked questions about sampling.
First, you need to understand the difference between a population and a sample , and identify the target population of your research.
- The population is the entire group that you want to draw conclusions about.
- The sample is the specific group of individuals that you will collect data from.
The population can be defined in terms of geographical location, age, income, or many other characteristics.
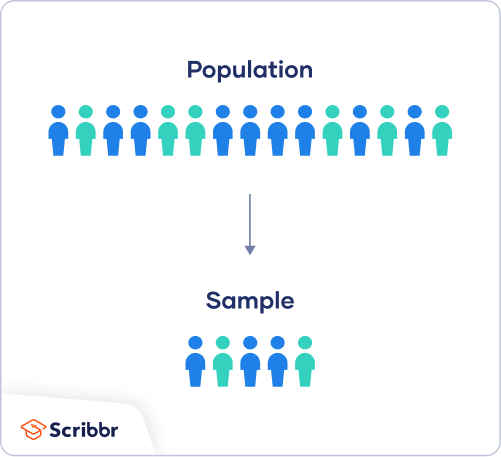
It is important to carefully define your target population according to the purpose and practicalities of your project.
If the population is very large, demographically mixed, and geographically dispersed, it might be difficult to gain access to a representative sample. A lack of a representative sample affects the validity of your results, and can lead to several research biases , particularly sampling bias .
Sampling frame
The sampling frame is the actual list of individuals that the sample will be drawn from. Ideally, it should include the entire target population (and nobody who is not part of that population).
Sample size
The number of individuals you should include in your sample depends on various factors, including the size and variability of the population and your research design. There are different sample size calculators and formulas depending on what you want to achieve with statistical analysis .
Prevent plagiarism. Run a free check.
Probability sampling means that every member of the population has a chance of being selected. It is mainly used in quantitative research . If you want to produce results that are representative of the whole population, probability sampling techniques are the most valid choice.
There are four main types of probability sample.
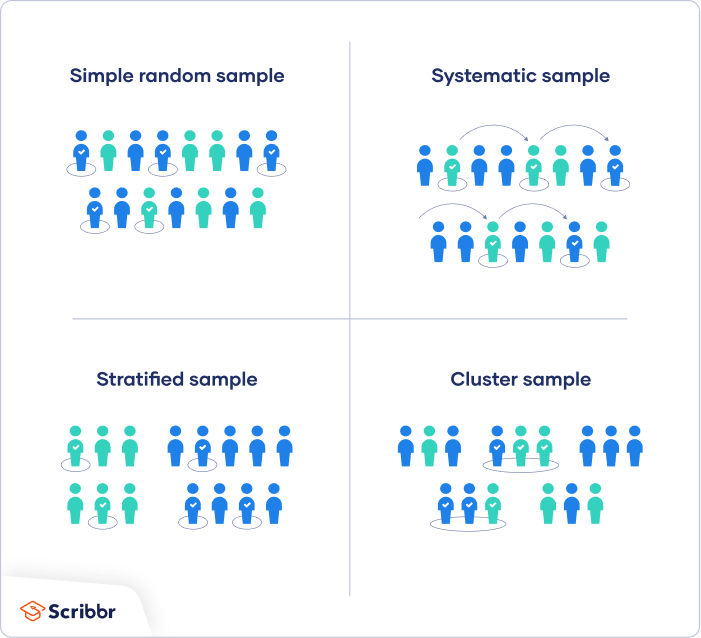
1. Simple random sampling
In a simple random sample, every member of the population has an equal chance of being selected. Your sampling frame should include the whole population.
To conduct this type of sampling, you can use tools like random number generators or other techniques that are based entirely on chance.
2. Systematic sampling
Systematic sampling is similar to simple random sampling, but it is usually slightly easier to conduct. Every member of the population is listed with a number, but instead of randomly generating numbers, individuals are chosen at regular intervals.
If you use this technique, it is important to make sure that there is no hidden pattern in the list that might skew the sample. For example, if the HR database groups employees by team, and team members are listed in order of seniority, there is a risk that your interval might skip over people in junior roles, resulting in a sample that is skewed towards senior employees.
3. Stratified sampling
Stratified sampling involves dividing the population into subpopulations that may differ in important ways. It allows you draw more precise conclusions by ensuring that every subgroup is properly represented in the sample.
To use this sampling method, you divide the population into subgroups (called strata) based on the relevant characteristic (e.g., gender identity, age range, income bracket, job role).
Based on the overall proportions of the population, you calculate how many people should be sampled from each subgroup. Then you use random or systematic sampling to select a sample from each subgroup.
4. Cluster sampling
Cluster sampling also involves dividing the population into subgroups, but each subgroup should have similar characteristics to the whole sample. Instead of sampling individuals from each subgroup, you randomly select entire subgroups.
If it is practically possible, you might include every individual from each sampled cluster. If the clusters themselves are large, you can also sample individuals from within each cluster using one of the techniques above. This is called multistage sampling .
This method is good for dealing with large and dispersed populations, but there is more risk of error in the sample, as there could be substantial differences between clusters. It’s difficult to guarantee that the sampled clusters are really representative of the whole population.
In a non-probability sample, individuals are selected based on non-random criteria, and not every individual has a chance of being included.
This type of sample is easier and cheaper to access, but it has a higher risk of sampling bias . That means the inferences you can make about the population are weaker than with probability samples, and your conclusions may be more limited. If you use a non-probability sample, you should still aim to make it as representative of the population as possible.
Non-probability sampling techniques are often used in exploratory and qualitative research . In these types of research, the aim is not to test a hypothesis about a broad population, but to develop an initial understanding of a small or under-researched population.
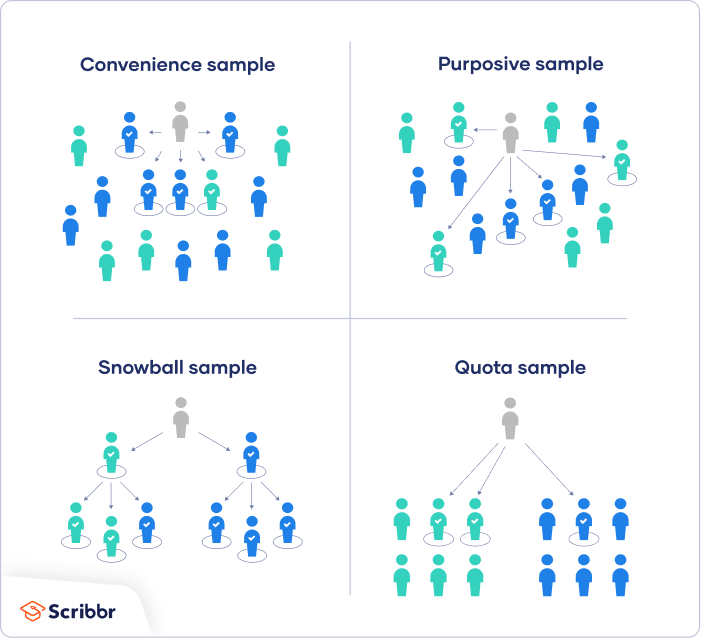
1. Convenience sampling
A convenience sample simply includes the individuals who happen to be most accessible to the researcher.
This is an easy and inexpensive way to gather initial data, but there is no way to tell if the sample is representative of the population, so it can’t produce generalizable results. Convenience samples are at risk for both sampling bias and selection bias .
2. Voluntary response sampling
Similar to a convenience sample, a voluntary response sample is mainly based on ease of access. Instead of the researcher choosing participants and directly contacting them, people volunteer themselves (e.g. by responding to a public online survey).
Voluntary response samples are always at least somewhat biased , as some people will inherently be more likely to volunteer than others, leading to self-selection bias .
3. Purposive sampling
This type of sampling, also known as judgement sampling, involves the researcher using their expertise to select a sample that is most useful to the purposes of the research.
It is often used in qualitative research , where the researcher wants to gain detailed knowledge about a specific phenomenon rather than make statistical inferences, or where the population is very small and specific. An effective purposive sample must have clear criteria and rationale for inclusion. Always make sure to describe your inclusion and exclusion criteria and beware of observer bias affecting your arguments.
4. Snowball sampling
If the population is hard to access, snowball sampling can be used to recruit participants via other participants. The number of people you have access to “snowballs” as you get in contact with more people. The downside here is also representativeness, as you have no way of knowing how representative your sample is due to the reliance on participants recruiting others. This can lead to sampling bias .
5. Quota sampling
Quota sampling relies on the non-random selection of a predetermined number or proportion of units. This is called a quota.
You first divide the population into mutually exclusive subgroups (called strata) and then recruit sample units until you reach your quota. These units share specific characteristics, determined by you prior to forming your strata. The aim of quota sampling is to control what or who makes up your sample.
If you want to know more about statistics , methodology , or research bias , make sure to check out some of our other articles with explanations and examples.
- Student’s t -distribution
- Normal distribution
- Null and Alternative Hypotheses
- Chi square tests
- Confidence interval
- Quartiles & Quantiles
- Cluster sampling
- Stratified sampling
- Data cleansing
- Reproducibility vs Replicability
- Peer review
- Prospective cohort study
Research bias
- Implicit bias
- Cognitive bias
- Placebo effect
- Hawthorne effect
- Hindsight bias
- Affect heuristic
- Social desirability bias
Here's why students love Scribbr's proofreading services
Discover proofreading & editing
A sample is a subset of individuals from a larger population . Sampling means selecting the group that you will actually collect data from in your research. For example, if you are researching the opinions of students in your university, you could survey a sample of 100 students.
In statistics, sampling allows you to test a hypothesis about the characteristics of a population.
Samples are used to make inferences about populations . Samples are easier to collect data from because they are practical, cost-effective, convenient, and manageable.
Probability sampling means that every member of the target population has a known chance of being included in the sample.
Probability sampling methods include simple random sampling , systematic sampling , stratified sampling , and cluster sampling .
In non-probability sampling , the sample is selected based on non-random criteria, and not every member of the population has a chance of being included.
Common non-probability sampling methods include convenience sampling , voluntary response sampling, purposive sampling , snowball sampling, and quota sampling .
In multistage sampling , or multistage cluster sampling, you draw a sample from a population using smaller and smaller groups at each stage.
This method is often used to collect data from a large, geographically spread group of people in national surveys, for example. You take advantage of hierarchical groupings (e.g., from state to city to neighborhood) to create a sample that’s less expensive and time-consuming to collect data from.
Sampling bias occurs when some members of a population are systematically more likely to be selected in a sample than others.
Cite this Scribbr article
If you want to cite this source, you can copy and paste the citation or click the “Cite this Scribbr article” button to automatically add the citation to our free Citation Generator.
McCombes, S. (2023, June 22). Sampling Methods | Types, Techniques & Examples. Scribbr. Retrieved August 26, 2024, from https://www.scribbr.com/methodology/sampling-methods/
Is this article helpful?
Shona McCombes
Other students also liked, population vs. sample | definitions, differences & examples, simple random sampling | definition, steps & examples, sampling bias and how to avoid it | types & examples, what is your plagiarism score.
- Privacy Policy

Home » Sampling Methods – Types, Techniques and Examples
Sampling Methods – Types, Techniques and Examples
Table of Contents

Sampling refers to the process of selecting a subset of data from a larger population or dataset in order to analyze or make inferences about the whole population.
In other words, sampling involves taking a representative sample of data from a larger group or dataset in order to gain insights or draw conclusions about the entire group.
Sampling Methods
Sampling methods refer to the techniques used to select a subset of individuals or units from a larger population for the purpose of conducting statistical analysis or research.
Sampling is an essential part of the Research because it allows researchers to draw conclusions about a population without having to collect data from every member of that population, which can be time-consuming, expensive, or even impossible.
Types of Sampling Methods
Sampling can be broadly categorized into two main categories:
Probability Sampling
This type of sampling is based on the principles of random selection, and it involves selecting samples in a way that every member of the population has an equal chance of being included in the sample.. Probability sampling is commonly used in scientific research and statistical analysis, as it provides a representative sample that can be generalized to the larger population.
Type of Probability Sampling :
- Simple Random Sampling: In this method, every member of the population has an equal chance of being selected for the sample. This can be done using a random number generator or by drawing names out of a hat, for example.
- Systematic Sampling: In this method, the population is first divided into a list or sequence, and then every nth member is selected for the sample. For example, if every 10th person is selected from a list of 100 people, the sample would include 10 people.
- Stratified Sampling: In this method, the population is divided into subgroups or strata based on certain characteristics, and then a random sample is taken from each stratum. This is often used to ensure that the sample is representative of the population as a whole.
- Cluster Sampling: In this method, the population is divided into clusters or groups, and then a random sample of clusters is selected. Then, all members of the selected clusters are included in the sample.
- Multi-Stage Sampling : This method combines two or more sampling techniques. For example, a researcher may use stratified sampling to select clusters, and then use simple random sampling to select members within each cluster.
Non-probability Sampling
This type of sampling does not rely on random selection, and it involves selecting samples in a way that does not give every member of the population an equal chance of being included in the sample. Non-probability sampling is often used in qualitative research, where the aim is not to generalize findings to a larger population, but to gain an in-depth understanding of a particular phenomenon or group. Non-probability sampling methods can be quicker and more cost-effective than probability sampling methods, but they may also be subject to bias and may not be representative of the larger population.
Types of Non-probability Sampling :
- Convenience Sampling: In this method, participants are chosen based on their availability or willingness to participate. This method is easy and convenient but may not be representative of the population.
- Purposive Sampling: In this method, participants are selected based on specific criteria, such as their expertise or knowledge on a particular topic. This method is often used in qualitative research, but may not be representative of the population.
- Snowball Sampling: In this method, participants are recruited through referrals from other participants. This method is often used when the population is hard to reach, but may not be representative of the population.
- Quota Sampling: In this method, a predetermined number of participants are selected based on specific criteria, such as age or gender. This method is often used in market research, but may not be representative of the population.
- Volunteer Sampling: In this method, participants volunteer to participate in the study. This method is often used in research where participants are motivated by personal interest or altruism, but may not be representative of the population.
Applications of Sampling Methods
Applications of Sampling Methods from different fields:
- Psychology : Sampling methods are used in psychology research to study various aspects of human behavior and mental processes. For example, researchers may use stratified sampling to select a sample of participants that is representative of the population based on factors such as age, gender, and ethnicity. Random sampling may also be used to select participants for experimental studies.
- Sociology : Sampling methods are commonly used in sociological research to study social phenomena and relationships between individuals and groups. For example, researchers may use cluster sampling to select a sample of neighborhoods to study the effects of economic inequality on health outcomes. Stratified sampling may also be used to select a sample of participants that is representative of the population based on factors such as income, education, and occupation.
- Social sciences: Sampling methods are commonly used in social sciences to study human behavior and attitudes. For example, researchers may use stratified sampling to select a sample of participants that is representative of the population based on factors such as age, gender, and income.
- Marketing : Sampling methods are used in marketing research to collect data on consumer preferences, behavior, and attitudes. For example, researchers may use random sampling to select a sample of consumers to participate in a survey about a new product.
- Healthcare : Sampling methods are used in healthcare research to study the prevalence of diseases and risk factors, and to evaluate interventions. For example, researchers may use cluster sampling to select a sample of health clinics to participate in a study of the effectiveness of a new treatment.
- Environmental science: Sampling methods are used in environmental science to collect data on environmental variables such as water quality, air pollution, and soil composition. For example, researchers may use systematic sampling to collect soil samples at regular intervals across a field.
- Education : Sampling methods are used in education research to study student learning and achievement. For example, researchers may use stratified sampling to select a sample of schools that is representative of the population based on factors such as demographics and academic performance.
Examples of Sampling Methods
Probability Sampling Methods Examples:
- Simple random sampling Example : A researcher randomly selects participants from the population using a random number generator or drawing names from a hat.
- Stratified random sampling Example : A researcher divides the population into subgroups (strata) based on a characteristic of interest (e.g. age or income) and then randomly selects participants from each subgroup.
- Systematic sampling Example : A researcher selects participants at regular intervals from a list of the population.
Non-probability Sampling Methods Examples:
- Convenience sampling Example: A researcher selects participants who are conveniently available, such as students in a particular class or visitors to a shopping mall.
- Purposive sampling Example : A researcher selects participants who meet specific criteria, such as individuals who have been diagnosed with a particular medical condition.
- Snowball sampling Example : A researcher selects participants who are referred to them by other participants, such as friends or acquaintances.
How to Conduct Sampling Methods
some general steps to conduct sampling methods:
- Define the population: Identify the population of interest and clearly define its boundaries.
- Choose the sampling method: Select an appropriate sampling method based on the research question, characteristics of the population, and available resources.
- Determine the sample size: Determine the desired sample size based on statistical considerations such as margin of error, confidence level, or power analysis.
- Create a sampling frame: Develop a list of all individuals or elements in the population from which the sample will be drawn. The sampling frame should be comprehensive, accurate, and up-to-date.
- Select the sample: Use the chosen sampling method to select the sample from the sampling frame. The sample should be selected randomly, or if using a non-random method, every effort should be made to minimize bias and ensure that the sample is representative of the population.
- Collect data: Once the sample has been selected, collect data from each member of the sample using appropriate research methods (e.g., surveys, interviews, observations).
- Analyze the data: Analyze the data collected from the sample to draw conclusions about the population of interest.
When to use Sampling Methods
Sampling methods are used in research when it is not feasible or practical to study the entire population of interest. Sampling allows researchers to study a smaller group of individuals, known as a sample, and use the findings from the sample to make inferences about the larger population.
Sampling methods are particularly useful when:
- The population of interest is too large to study in its entirety.
- The cost and time required to study the entire population are prohibitive.
- The population is geographically dispersed or difficult to access.
- The research question requires specialized or hard-to-find individuals.
- The data collected is quantitative and statistical analyses are used to draw conclusions.
Purpose of Sampling Methods
The main purpose of sampling methods in research is to obtain a representative sample of individuals or elements from a larger population of interest, in order to make inferences about the population as a whole. By studying a smaller group of individuals, known as a sample, researchers can gather information about the population that would be difficult or impossible to obtain from studying the entire population.
Sampling methods allow researchers to:
- Study a smaller, more manageable group of individuals, which is typically less time-consuming and less expensive than studying the entire population.
- Reduce the potential for data collection errors and improve the accuracy of the results by minimizing sampling bias.
- Make inferences about the larger population with a certain degree of confidence, using statistical analyses of the data collected from the sample.
- Improve the generalizability and external validity of the findings by ensuring that the sample is representative of the population of interest.
Characteristics of Sampling Methods
Here are some characteristics of sampling methods:
- Randomness : Probability sampling methods are based on random selection, meaning that every member of the population has an equal chance of being selected. This helps to minimize bias and ensure that the sample is representative of the population.
- Representativeness : The goal of sampling is to obtain a sample that is representative of the larger population of interest. This means that the sample should reflect the characteristics of the population in terms of key demographic, behavioral, or other relevant variables.
- Size : The size of the sample should be large enough to provide sufficient statistical power for the research question at hand. The sample size should also be appropriate for the chosen sampling method and the level of precision desired.
- Efficiency : Sampling methods should be efficient in terms of time, cost, and resources required. The method chosen should be feasible given the available resources and time constraints.
- Bias : Sampling methods should aim to minimize bias and ensure that the sample is representative of the population of interest. Bias can be introduced through non-random selection or non-response, and can affect the validity and generalizability of the findings.
- Precision : Sampling methods should be precise in terms of providing estimates of the population parameters of interest. Precision is influenced by sample size, sampling method, and level of variability in the population.
- Validity : The validity of the sampling method is important for ensuring that the results obtained from the sample are accurate and can be generalized to the population of interest. Validity can be affected by sampling method, sample size, and the representativeness of the sample.
Advantages of Sampling Methods
Sampling methods have several advantages, including:
- Cost-Effective : Sampling methods are often much cheaper and less time-consuming than studying an entire population. By studying only a small subset of the population, researchers can gather valuable data without incurring the costs associated with studying the entire population.
- Convenience : Sampling methods are often more convenient than studying an entire population. For example, if a researcher wants to study the eating habits of people in a city, it would be very difficult and time-consuming to study every single person in the city. By using sampling methods, the researcher can obtain data from a smaller subset of people, making the study more feasible.
- Accuracy: When done correctly, sampling methods can be very accurate. By using appropriate sampling techniques, researchers can obtain a sample that is representative of the entire population. This allows them to make accurate generalizations about the population as a whole based on the data collected from the sample.
- Time-Saving: Sampling methods can save a lot of time compared to studying the entire population. By studying a smaller sample, researchers can collect data much more quickly than they could if they studied every single person in the population.
- Less Bias : Sampling methods can reduce bias in a study. If a researcher were to study the entire population, it would be very difficult to eliminate all sources of bias. However, by using appropriate sampling techniques, researchers can reduce bias and obtain a sample that is more representative of the entire population.
Limitations of Sampling Methods
- Sampling Error : Sampling error is the difference between the sample statistic and the population parameter. It is the result of selecting a sample rather than the entire population. The larger the sample, the lower the sampling error. However, no matter how large the sample size, there will always be some degree of sampling error.
- Selection Bias: Selection bias occurs when the sample is not representative of the population. This can happen if the sample is not selected randomly or if some groups are underrepresented in the sample. Selection bias can lead to inaccurate conclusions about the population.
- Non-response Bias : Non-response bias occurs when some members of the sample do not respond to the survey or study. This can result in a biased sample if the non-respondents differ from the respondents in important ways.
- Time and Cost : While sampling can be cost-effective, it can still be expensive and time-consuming to select a sample that is representative of the population. Depending on the sampling method used, it may take a long time to obtain a sample that is large enough and representative enough to be useful.
- Limited Information : Sampling can only provide information about the variables that are measured. It may not provide information about other variables that are relevant to the research question but were not measured.
- Generalization : The extent to which the findings from a sample can be generalized to the population depends on the representativeness of the sample. If the sample is not representative of the population, it may not be possible to generalize the findings to the population as a whole.
About the author
Muhammad Hassan
Researcher, Academic Writer, Web developer
You may also like

Purposive Sampling – Methods, Types and Examples

Systematic Sampling – Types, Method and Examples

Quota Sampling – Types, Methods and Examples

Stratified Random Sampling – Definition, Method...

Simple Random Sampling – Types, Method and...

Volunteer Sampling – Definition, Methods and...
Educational resources and simple solutions for your research journey
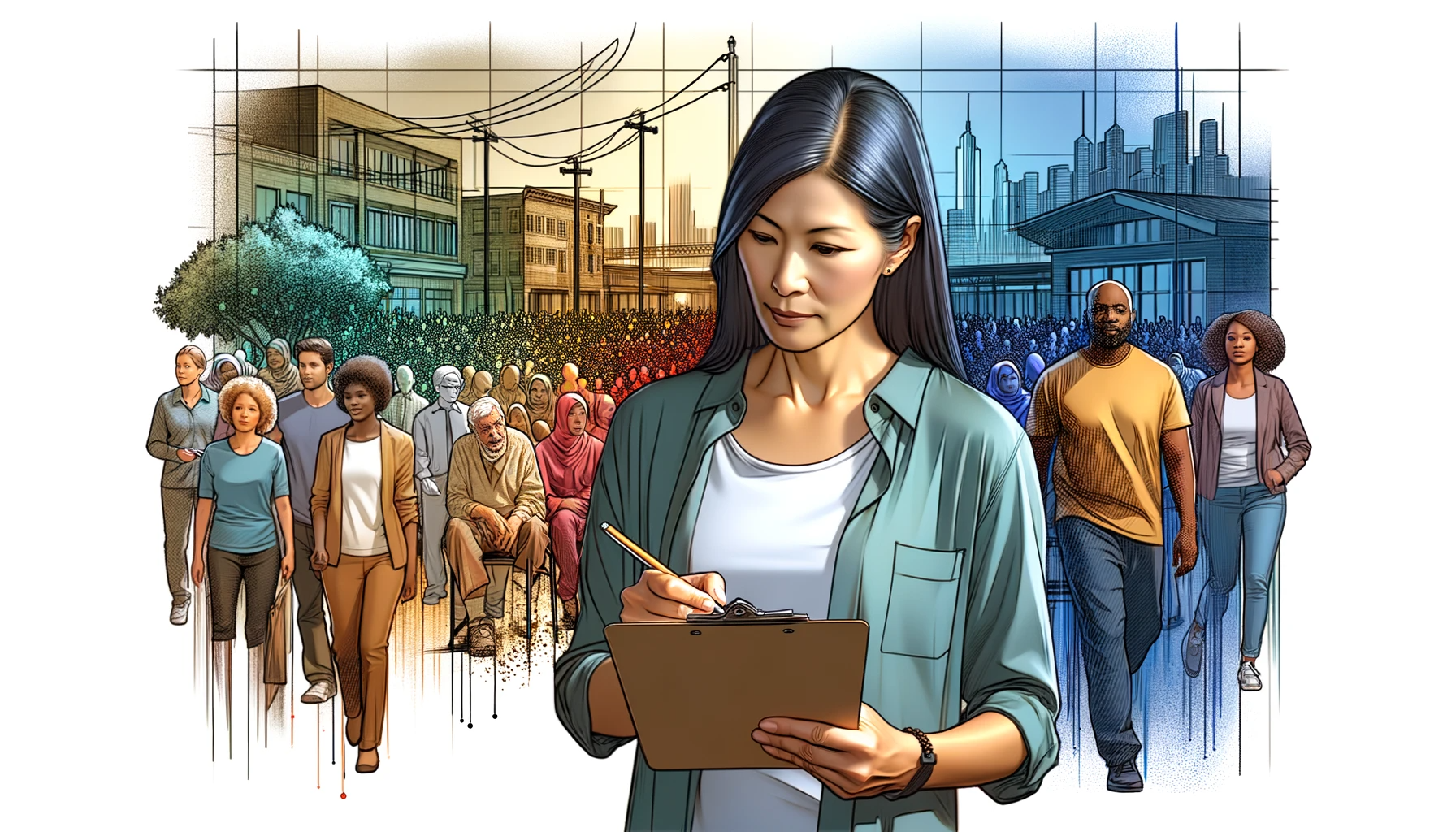
What are Sampling Methods? Techniques, Types, and Examples
Every type of research includes samples from which inferences are drawn. The sample could be biological specimens or a subset of a specific group or population selected for analysis. The goal is often to conclude the entire population based on the characteristics observed in the sample. Now, the question comes to mind: how does one collect the samples? Answer: Using sampling methods. Various sampling strategies are available to researchers to define and collect samples that will form the basis of their research study.
In a study focusing on individuals experiencing anxiety, gathering data from the entire population is practically impossible due to the widespread prevalence of anxiety. Consequently, a sample is carefully selected—a subset of individuals meant to represent (or not in some cases accurately) the demographics of those experiencing anxiety. The study’s outcomes hinge significantly on the chosen sample, emphasizing the critical importance of a thoughtful and precise selection process. The conclusions drawn about the broader population rely heavily on the selected sample’s characteristics and diversity.
Table of Contents
What is sampling?
Sampling involves the strategic selection of individuals or a subset from a population, aiming to derive statistical inferences and predict the characteristics of the entire population. It offers a pragmatic and practical approach to examining the features of the whole population, which would otherwise be difficult to achieve because studying the total population is expensive, time-consuming, and often impossible. Market researchers use various sampling methods to collect samples from a large population to acquire relevant insights. The best sampling strategy for research is determined by criteria such as the purpose of the study, available resources (time and money), and research hypothesis.
For example, if a pet food manufacturer wants to investigate the positive impact of a new cat food on feline growth, studying all the cats in the country is impractical. In such cases, employing an appropriate sampling technique from the extensive dataset allows the researcher to focus on a manageable subset. This enables the researcher to study the growth-promoting effects of the new pet food. This article will delve into the standard sampling methods and explore the situations in which each is most appropriately applied.
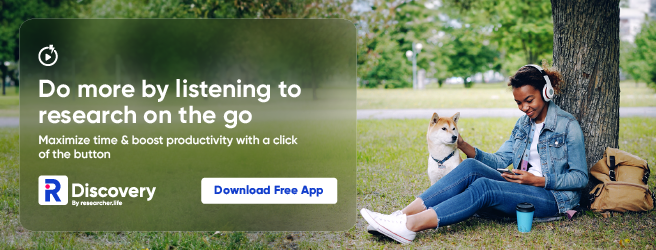
What are sampling methods or sampling techniques?
Sampling methods or sampling techniques in research are statistical methods for selecting a sample representative of the whole population to study the population’s characteristics. Sampling methods serve as invaluable tools for researchers, enabling the collection of meaningful data and facilitating analysis to identify distinctive features of the people. Different sampling strategies can be used based on the characteristics of the population, the study purpose, and the available resources. Now that we understand why sampling methods are essential in research, we review the various sample methods in the following sections.
Types of sampling methods
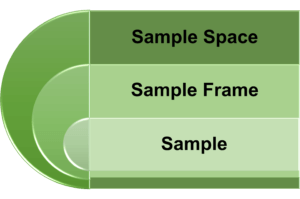
Before we go into the specifics of each sampling method, it’s vital to understand terms like sample, sample frame, and sample space. In probability theory, the sample space comprises all possible outcomes of a random experiment, while the sample frame is the list or source guiding sample selection in statistical research. The sample represents the group of individuals participating in the study, forming the basis for the research findings. Selecting the correct sample is critical to ensuring the validity and reliability of any research; the sample should be representative of the population.
There are two most common sampling methods:
- Probability sampling: A sampling method in which each unit or element in the population has an equal chance of being selected in the final sample. This is called random sampling, emphasizing the random and non-zero probability nature of selecting samples. Such a sampling technique ensures a more representative and unbiased sample, enabling robust inferences about the entire population.
- Non-probability sampling: Another sampling method is non-probability sampling, which involves collecting data conveniently through a non-random selection based on predefined criteria. This offers a straightforward way to gather data, although the resulting sample may or may not accurately represent the entire population.
Irrespective of the research method you opt for, it is essential to explicitly state the chosen sampling technique in the methodology section of your research article. Now, we will explore the different characteristics of both sampling methods, along with various subtypes falling under these categories.
What is probability sampling?
The probability sampling method is based on the probability theory, which means that the sample selection criteria involve some random selection. The probability sampling method provides an equal opportunity for all elements or units within the entire sample space to be chosen. While it can be labor-intensive and expensive, the advantage lies in its ability to offer a more accurate representation of the population, thereby enhancing confidence in the inferences drawn in the research.
Types of probability sampling
Various probability sampling methods exist, such as simple random sampling, systematic sampling, stratified sampling, and clustered sampling. Here, we provide detailed discussions and illustrative examples for each of these sampling methods:
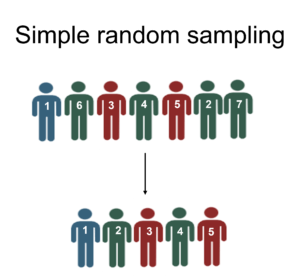
- Simple random sampling: In simple random sampling, each individual has an equal probability of being chosen, and each selection is independent of the others. Because the choice is entirely based on chance, this is also known as the method of chance selection. In the simple random sampling method, the sample frame comprises the entire population.
For example, A fitness sports brand is launching a new protein drink and aims to select 20 individuals from a 200-person fitness center to try it. Employing a simple random sampling approach, each of the 200 people is assigned a unique identifier. Of these, 20 individuals are then chosen by generating random numbers between 1 and 200, either manually or through a computer program. Matching these numbers with the individuals creates a randomly selected group of 20 people. This method minimizes sampling bias and ensures a representative subset of the entire population under study.
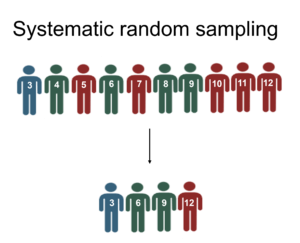
- Systematic sampling: The systematic sampling approach involves selecting units or elements at regular intervals from an ordered list of the population. Because the starting point of this sampling method is chosen at random, it is more convenient than essential random sampling. For a better understanding, consider the following example.
For example, considering the previous model, individuals at the fitness facility are arranged alphabetically. The manufacturer then initiates the process by randomly selecting a starting point from the first ten positions, let’s say 8. Starting from the 8th position, every tenth person on the list is then chosen (e.g., 8, 18, 28, 38, and so forth) until a sample of 20 individuals is obtained.
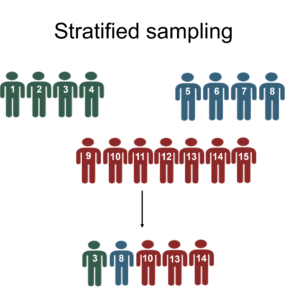
- Stratified sampling: Stratified sampling divides the population into subgroups (strata), and random samples are drawn from each stratum in proportion to its size in the population. Stratified sampling provides improved representation because each subgroup that differs in significant ways is included in the final sample.
For example, Expanding on the previous simple random sampling example, suppose the manufacturer aims for a more comprehensive representation of genders in a sample of 200 people, consisting of 90 males, 80 females, and 30 others. The manufacturer categorizes the population into three gender strata (Male, Female, and Others). Within each group, random sampling is employed to select nine males, eight females, and three individuals from the others category, resulting in a well-rounded and representative sample of 200 individuals.
- Clustered sampling: In this sampling method, the population is divided into clusters, and then a random sample of clusters is included in the final sample. Clustered sampling, distinct from stratified sampling, involves subgroups (clusters) that exhibit characteristics similar to the whole sample. In the case of small clusters, all members can be included in the final sample, whereas for larger clusters, individuals within each cluster may be sampled using the sampling above methods. This approach is referred to as multistage sampling. This sampling method is well-suited for large and widely distributed populations; however, there is a potential risk of sample error because ensuring that the sampled clusters truly represent the entire population can be challenging.
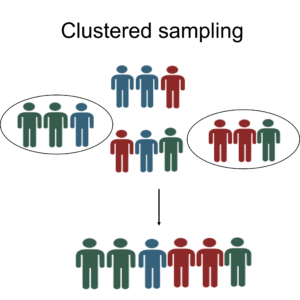
For example, Researchers conducting a nationwide health study can select specific geographic clusters, like cities or regions, instead of trying to survey the entire population individually. Within each chosen cluster, they sample individuals, providing a representative subset without the logistical challenges of attempting a nationwide survey.
Use s of probability sampling
Probability sampling methods find widespread use across diverse research disciplines because of their ability to yield representative and unbiased samples. The advantages of employing probability sampling include the following:
- Representativeness
Probability sampling assures that every element in the population has a non-zero chance of being included in the sample, ensuring representativeness of the entire population and decreasing research bias to minimal to non-existent levels. The researcher can acquire higher-quality data via probability sampling, increasing confidence in the conclusions.
- Statistical inference
Statistical methods, like confidence intervals and hypothesis testing, depend on probability sampling to generalize findings from a sample to the broader population. Probability sampling methods ensure unbiased representation, allowing inferences about the population based on the characteristics of the sample.
- Precision and reliability
The use of probability sampling improves the precision and reliability of study results. Because the probability of selecting any single element/individual is known, the chance variations that may occur in non-probability sampling methods are reduced, resulting in more dependable and precise estimations.
- Generalizability
Probability sampling enables the researcher to generalize study findings to the entire population from which they were derived. The results produced through probability sampling methods are more likely to be applicable to the larger population, laying the foundation for making broad predictions or recommendations.
- Minimization of Selection Bias
By ensuring that each member of the population has an equal chance of being selected in the sample, probability sampling lowers the possibility of selection bias. This reduces the impact of systematic errors that may occur in non-probability sampling methods, where data may be skewed toward a specific demographic due to inadequate representation of each segment of the population.
What is non-probability sampling?
Non-probability sampling methods involve selecting individuals based on non-random criteria, often relying on the researcher’s judgment or predefined criteria. While it is easier and more economical, it tends to introduce sampling bias, resulting in weaker inferences compared to probability sampling techniques in research.
Types of Non-probability Sampling
Non-probability sampling methods are further classified as convenience sampling, consecutive sampling, quota sampling, purposive or judgmental sampling, and snowball sampling. Let’s explore these types of sampling methods in detail.
- Convenience sampling: In convenience sampling, individuals are recruited directly from the population based on the accessibility and proximity to the researcher. It is a simple, inexpensive, and practical method of sample selection, yet convenience sampling suffers from both sampling and selection bias due to a lack of appropriate population representation.
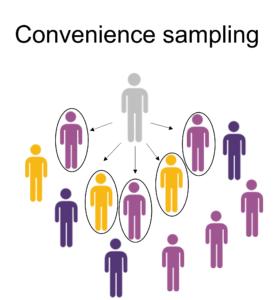
For example, imagine you’re a researcher investigating smartphone usage patterns in your city. The most convenient way to select participants is by approaching people in a shopping mall on a weekday afternoon. However, this convenience sampling method may not be an accurate representation of the city’s overall smartphone usage patterns as the sample is limited to individuals present at the mall during weekdays, excluding those who visit on other days or never visit the mall.
- Consecutive sampling: Participants in consecutive sampling (or sequential sampling) are chosen based on their availability and desire to participate in the study as they become available. This strategy entails sequentially recruiting individuals who fulfill the researcher’s requirements.
For example, In researching the prevalence of stroke in a hospital, instead of randomly selecting patients from the entire population, the researcher can opt to include all eligible patients admitted over three months. Participants are then consecutively recruited upon admission during that timeframe, forming the study sample.
- Quota sampling: The selection of individuals in quota sampling is based on non-random selection criteria in which only participants with certain traits or proportions that are representative of the population are included. Quota sampling involves setting predetermined quotas for specific subgroups based on key demographics or other relevant characteristics. This sampling method employs dividing the population into mutually exclusive subgroups and then selecting sample units until the set quota is reached.
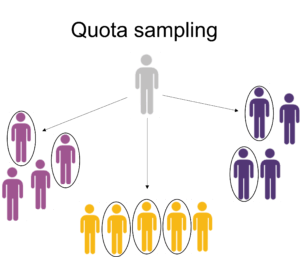
For example, In a survey on a college campus to assess student interest in a new policy, the researcher should establish quotas aligned with the distribution of student majors, ensuring representation from various academic disciplines. If the campus has 20% biology majors, 30% engineering majors, 20% business majors, and 30% liberal arts majors, participants should be recruited to mirror these proportions.
- Purposive or judgmental sampling: In purposive sampling, the researcher leverages expertise to select a sample relevant to the study’s specific questions. This sampling method is commonly applied in qualitative research, mainly when aiming to understand a particular phenomenon, and is suitable for smaller population sizes.
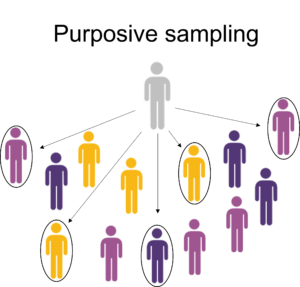
For example, imagine a researcher who wants to study public policy issues for a focus group. The researcher might purposely select participants with expertise in economics, law, and public administration to take advantage of their knowledge and ensure a depth of understanding.
- Snowball sampling: This sampling method is used when accessing the population is challenging. It involves collecting the sample through a chain-referral process, where each recruited candidate aids in finding others. These candidates share common traits, representing the targeted population. This method is often used in qualitative research, particularly when studying phenomena related to stigmatized or hidden populations.

For example, In a study focusing on understanding the experiences and challenges of individuals in hidden or stigmatized communities (e.g., LGBTQ+ individuals in specific cultural contexts), the snowball sampling technique can be employed. The researcher initiates contact with one community member, who then assists in identifying additional candidates until the desired sample size is achieved.
Uses of non-probability sampling
Non-probability sampling approaches are employed in qualitative or exploratory research where the goal is to investigate underlying population traits rather than generalizability. Non-probability sampling methods are also helpful for the following purposes:
- Generating a hypothesis
In the initial stages of exploratory research, non-probability methods such as purposive or convenience allow researchers to quickly gather information and generate hypothesis that helps build a future research plan.
- Qualitative research
Qualitative research is usually focused on understanding the depth and complexity of human experiences, behaviors, and perspectives. Non-probability methods like purposive or snowball sampling are commonly used to select participants with specific traits that are relevant to the research question.
- Convenience and pragmatism
Non-probability sampling methods are valuable when resource and time are limited or when preliminary data is required to test the pilot study. For example, conducting a survey at a local shopping mall to gather opinions on a consumer product due to the ease of access to potential participants.
Probability vs Non-probability Sampling Methods
Selection of participants | Random selection of participants from the population using randomization methods | Non-random selection of participants from the population based on convenience or criteria |
Representativeness | Likely to yield a representative sample of the whole population allowing for generalizations | May not yield a representative sample of the whole population; poor generalizability |
Precision and accuracy | Provides more precise and accurate estimates of population characteristics | May have less precision and accuracy due to non-random selection |
Bias | Minimizes selection bias | May introduce selection bias if criteria are subjective and not well-defined |
Statistical inference | Suited for statistical inference and hypothesis testing and for making generalization to the population | Less suited for statistical inference and hypothesis testing on the population |
Application | Useful for quantitative research where generalizability is crucial | Commonly used in qualitative and exploratory research where in-depth insights are the goal |
Frequently asked questions
- What is multistage sampling ? Multistage sampling is a form of probability sampling approach that involves the progressive selection of samples in stages, going from larger clusters to a small number of participants, making it suited for large-scale research with enormous population lists.
- What are the methods of probability sampling? Probability sampling methods are simple random sampling, stratified random sampling, systematic sampling, cluster sampling, and multistage sampling.
- How to decide which type of sampling method to use? Choose a sampling method based on the goals, population, and resources. Probability for statistics and non-probability for efficiency or qualitative insights can be considered . Also, consider the population characteristics, size, and alignment with study objectives.
- What are the methods of non-probability sampling? Non-probability sampling methods are convenience sampling, consecutive sampling, purposive sampling, snowball sampling, and quota sampling.
- Why are sampling methods used in research? Sampling methods in research are employed to efficiently gather representative data from a subset of a larger population, enabling valid conclusions and generalizations while minimizing costs and time.
R Discovery is a literature search and research reading platform that accelerates your research discovery journey by keeping you updated on the latest, most relevant scholarly content. With 250M+ research articles sourced from trusted aggregators like CrossRef, Unpaywall, PubMed, PubMed Central, Open Alex and top publishing houses like Springer Nature, JAMA, IOP, Taylor & Francis, NEJM, BMJ, Karger, SAGE, Emerald Publishing and more, R Discovery puts a world of research at your fingertips.
Try R Discovery Prime FREE for 1 week or upgrade at just US$72 a year to access premium features that let you listen to research on the go, read in your language, collaborate with peers, auto sync with reference managers, and much more. Choose a simpler, smarter way to find and read research – Download the app and start your free 7-day trial today !
Related Posts

How Does R Discovery’s Interplatform Capability Enhance Research Accessibility

What is Convenience Sampling: Definition, Method, and Examples

Sampling Methods & Strategies 101
Everything you need to know (including examples)
By: Derek Jansen (MBA) | Expert Reviewed By: Kerryn Warren (PhD) | January 2023
If you’re new to research, sooner or later you’re bound to wander into the intimidating world of sampling methods and strategies. If you find yourself on this page, chances are you’re feeling a little overwhelmed or confused. Fear not – in this post we’ll unpack sampling in straightforward language , along with loads of examples .
Overview: Sampling Methods & Strategies
- What is sampling in a research context?
- The two overarching approaches
Simple random sampling
Stratified random sampling, cluster sampling, systematic sampling, purposive sampling, convenience sampling, snowball sampling.
- How to choose the right sampling method
What (exactly) is sampling?
At the simplest level, sampling (within a research context) is the process of selecting a subset of participants from a larger group . For example, if your research involved assessing US consumers’ perceptions about a particular brand of laundry detergent, you wouldn’t be able to collect data from every single person that uses laundry detergent (good luck with that!) – but you could potentially collect data from a smaller subset of this group.
In technical terms, the larger group is referred to as the population , and the subset (the group you’ll actually engage with in your research) is called the sample . Put another way, you can look at the population as a full cake and the sample as a single slice of that cake. In an ideal world, you’d want your sample to be perfectly representative of the population, as that would allow you to generalise your findings to the entire population. In other words, you’d want to cut a perfect cross-sectional slice of cake, such that the slice reflects every layer of the cake in perfect proportion.
Achieving a truly representative sample is, unfortunately, a little trickier than slicing a cake, as there are many practical challenges and obstacles to achieving this in a real-world setting. Thankfully though, you don’t always need to have a perfectly representative sample – it all depends on the specific research aims of each study – so don’t stress yourself out about that just yet!
With the concept of sampling broadly defined, let’s look at the different approaches to sampling to get a better understanding of what it all looks like in practice.
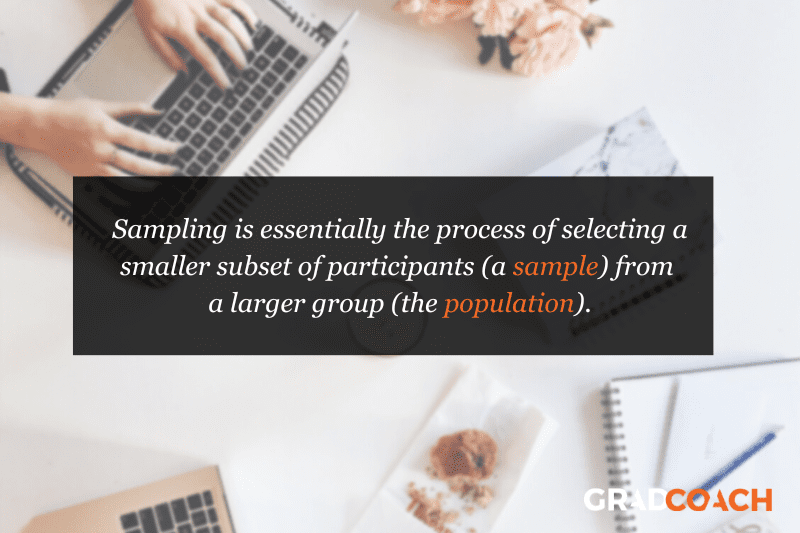
The two overarching sampling approaches
At the highest level, there are two approaches to sampling: probability sampling and non-probability sampling . Within each of these, there are a variety of sampling methods , which we’ll explore a little later.
Probability sampling involves selecting participants (or any unit of interest) on a statistically random basis , which is why it’s also called “random sampling”. In other words, the selection of each individual participant is based on a pre-determined process (not the discretion of the researcher). As a result, this approach achieves a random sample.
Probability-based sampling methods are most commonly used in quantitative research , especially when it’s important to achieve a representative sample that allows the researcher to generalise their findings.
Non-probability sampling , on the other hand, refers to sampling methods in which the selection of participants is not statistically random . In other words, the selection of individual participants is based on the discretion and judgment of the researcher, rather than on a pre-determined process.
Non-probability sampling methods are commonly used in qualitative research , where the richness and depth of the data are more important than the generalisability of the findings.
If that all sounds a little too conceptual and fluffy, don’t worry. Let’s take a look at some actual sampling methods to make it more tangible.
Need a helping hand?
Probability-based sampling methods
First, we’ll look at four common probability-based (random) sampling methods:
Importantly, this is not a comprehensive list of all the probability sampling methods – these are just four of the most common ones. So, if you’re interested in adopting a probability-based sampling approach, be sure to explore all the options.
Simple random sampling involves selecting participants in a completely random fashion , where each participant has an equal chance of being selected. Basically, this sampling method is the equivalent of pulling names out of a hat , except that you can do it digitally. For example, if you had a list of 500 people, you could use a random number generator to draw a list of 50 numbers (each number, reflecting a participant) and then use that dataset as your sample.
Thanks to its simplicity, simple random sampling is easy to implement , and as a consequence, is typically quite cheap and efficient . Given that the selection process is completely random, the results can be generalised fairly reliably. However, this also means it can hide the impact of large subgroups within the data, which can result in minority subgroups having little representation in the results – if any at all. To address this, one needs to take a slightly different approach, which we’ll look at next.
Stratified random sampling is similar to simple random sampling, but it kicks things up a notch. As the name suggests, stratified sampling involves selecting participants randomly , but from within certain pre-defined subgroups (i.e., strata) that share a common trait . For example, you might divide the population into strata based on gender, ethnicity, age range or level of education, and then select randomly from each group.
The benefit of this sampling method is that it gives you more control over the impact of large subgroups (strata) within the population. For example, if a population comprises 80% males and 20% females, you may want to “balance” this skew out by selecting a random sample from an equal number of males and females. This would, of course, reduce the representativeness of the sample, but it would allow you to identify differences between subgroups. So, depending on your research aims, the stratified approach could work well.
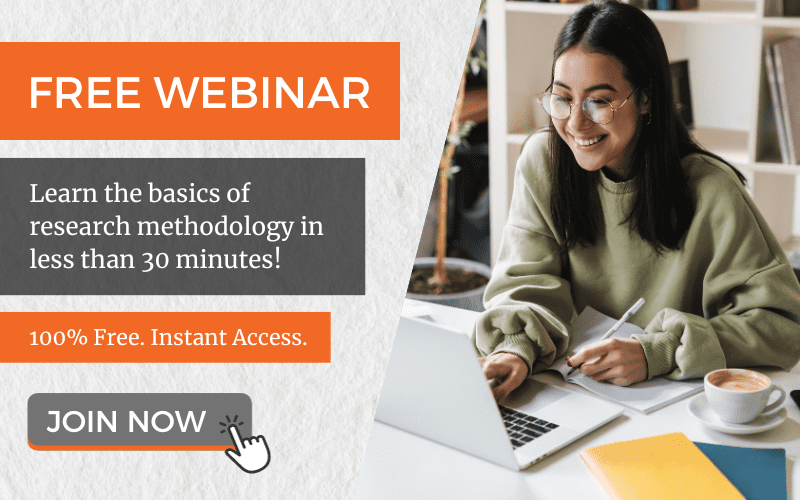
Next on the list is cluster sampling. As the name suggests, this sampling method involves sampling from naturally occurring, mutually exclusive clusters within a population – for example, area codes within a city or cities within a country. Once the clusters are defined, a set of clusters are randomly selected and then a set of participants are randomly selected from each cluster.
Now, you’re probably wondering, “how is cluster sampling different from stratified random sampling?”. Well, let’s look at the previous example where each cluster reflects an area code in a given city.
With cluster sampling, you would collect data from clusters of participants in a handful of area codes (let’s say 5 neighbourhoods). Conversely, with stratified random sampling, you would need to collect data from all over the city (i.e., many more neighbourhoods). You’d still achieve the same sample size either way (let’s say 200 people, for example), but with stratified sampling, you’d need to do a lot more running around, as participants would be scattered across a vast geographic area. As a result, cluster sampling is often the more practical and economical option.
If that all sounds a little mind-bending, you can use the following general rule of thumb. If a population is relatively homogeneous , cluster sampling will often be adequate. Conversely, if a population is quite heterogeneous (i.e., diverse), stratified sampling will generally be more appropriate.
The last probability sampling method we’ll look at is systematic sampling. This method simply involves selecting participants at a set interval , starting from a random point .
For example, if you have a list of students that reflects the population of a university, you could systematically sample that population by selecting participants at an interval of 8 . In other words, you would randomly select a starting point – let’s say student number 40 – followed by student 48, 56, 64, etc.
What’s important with systematic sampling is that the population list you select from needs to be randomly ordered . If there are underlying patterns in the list (for example, if the list is ordered by gender, IQ, age, etc.), this will result in a non-random sample, which would defeat the purpose of adopting this sampling method. Of course, you could safeguard against this by “shuffling” your population list using a random number generator or similar tool.
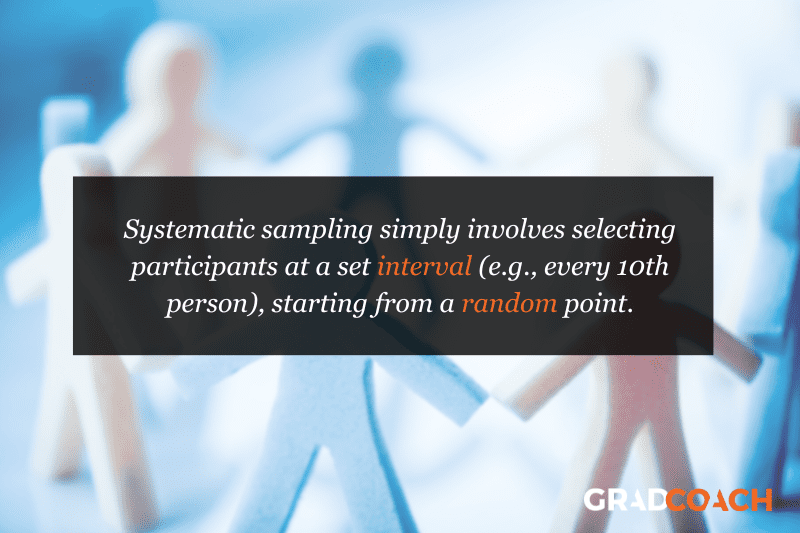
Non-probability-based sampling methods
Right, now that we’ve looked at a few probability-based sampling methods, let’s look at three non-probability methods :
Again, this is not an exhaustive list of all possible sampling methods, so be sure to explore further if you’re interested in adopting a non-probability sampling approach.
First up, we’ve got purposive sampling – also known as judgment , selective or subjective sampling. Again, the name provides some clues, as this method involves the researcher selecting participants using his or her own judgement , based on the purpose of the study (i.e., the research aims).
For example, suppose your research aims were to understand the perceptions of hyper-loyal customers of a particular retail store. In that case, you could use your judgement to engage with frequent shoppers, as well as rare or occasional shoppers, to understand what judgements drive the two behavioural extremes .
Purposive sampling is often used in studies where the aim is to gather information from a small population (especially rare or hard-to-find populations), as it allows the researcher to target specific individuals who have unique knowledge or experience . Naturally, this sampling method is quite prone to researcher bias and judgement error, and it’s unlikely to produce generalisable results, so it’s best suited to studies where the aim is to go deep rather than broad .
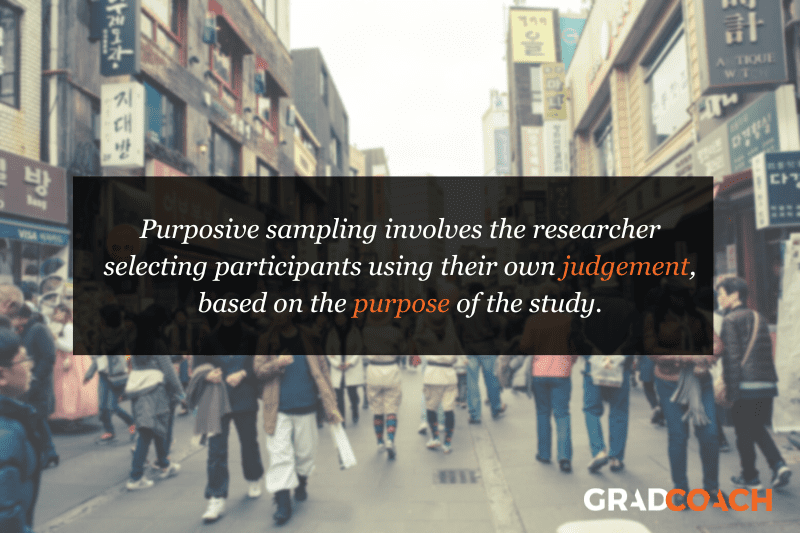
Next up, we have convenience sampling. As the name suggests, with this method, participants are selected based on their availability or accessibility . In other words, the sample is selected based on how convenient it is for the researcher to access it, as opposed to using a defined and objective process.
Naturally, convenience sampling provides a quick and easy way to gather data, as the sample is selected based on the individuals who are readily available or willing to participate. This makes it an attractive option if you’re particularly tight on resources and/or time. However, as you’d expect, this sampling method is unlikely to produce a representative sample and will of course be vulnerable to researcher bias , so it’s important to approach it with caution.
Last but not least, we have the snowball sampling method. This method relies on referrals from initial participants to recruit additional participants. In other words, the initial subjects form the first (small) snowball and each additional subject recruited through referral is added to the snowball, making it larger as it rolls along .
Snowball sampling is often used in research contexts where it’s difficult to identify and access a particular population. For example, people with a rare medical condition or members of an exclusive group. It can also be useful in cases where the research topic is sensitive or taboo and people are unlikely to open up unless they’re referred by someone they trust.
Simply put, snowball sampling is ideal for research that involves reaching hard-to-access populations . But, keep in mind that, once again, it’s a sampling method that’s highly prone to researcher bias and is unlikely to produce a representative sample. So, make sure that it aligns with your research aims and questions before adopting this method.
How to choose a sampling method
Now that we’ve looked at a few popular sampling methods (both probability and non-probability based), the obvious question is, “ how do I choose the right sampling method for my study?”. When selecting a sampling method for your research project, you’ll need to consider two important factors: your research aims and your resources .
As with all research design and methodology choices, your sampling approach needs to be guided by and aligned with your research aims, objectives and research questions – in other words, your golden thread. Specifically, you need to consider whether your research aims are primarily concerned with producing generalisable findings (in which case, you’ll likely opt for a probability-based sampling method) or with achieving rich , deep insights (in which case, a non-probability-based approach could be more practical). Typically, quantitative studies lean toward the former, while qualitative studies aim for the latter, so be sure to consider your broader methodology as well.
The second factor you need to consider is your resources and, more generally, the practical constraints at play. If, for example, you have easy, free access to a large sample at your workplace or university and a healthy budget to help you attract participants, that will open up multiple options in terms of sampling methods. Conversely, if you’re cash-strapped, short on time and don’t have unfettered access to your population of interest, you may be restricted to convenience or referral-based methods.
In short, be ready for trade-offs – you won’t always be able to utilise the “perfect” sampling method for your study, and that’s okay. Much like all the other methodological choices you’ll make as part of your study, you’ll often need to compromise and accept practical trade-offs when it comes to sampling. Don’t let this get you down though – as long as your sampling choice is well explained and justified, and the limitations of your approach are clearly articulated, you’ll be on the right track.
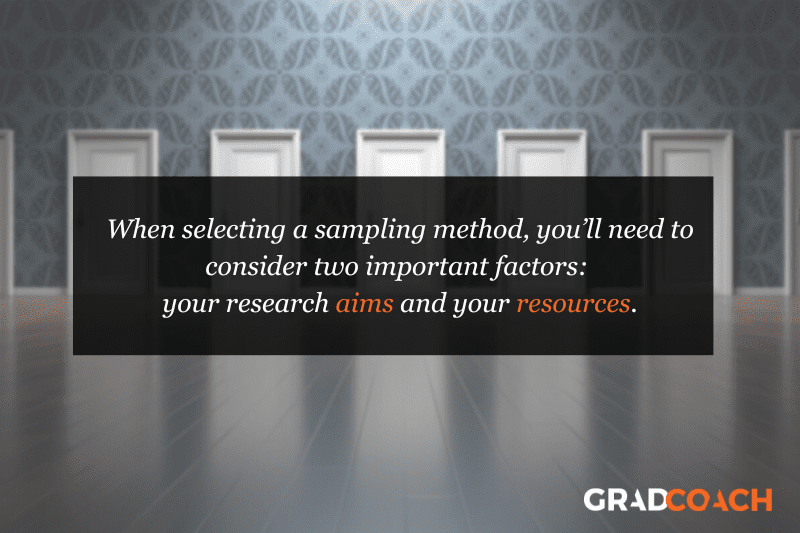
Let’s recap…
In this post, we’ve covered the basics of sampling within the context of a typical research project.
- Sampling refers to the process of defining a subgroup (sample) from the larger group of interest (population).
- The two overarching approaches to sampling are probability sampling (random) and non-probability sampling .
- Common probability-based sampling methods include simple random sampling, stratified random sampling, cluster sampling and systematic sampling.
- Common non-probability-based sampling methods include purposive sampling, convenience sampling and snowball sampling.
- When choosing a sampling method, you need to consider your research aims , objectives and questions, as well as your resources and other practical constraints .
If you’d like to see an example of a sampling strategy in action, be sure to check out our research methodology chapter sample .
Last but not least, if you need hands-on help with your sampling (or any other aspect of your research), take a look at our 1-on-1 coaching service , where we guide you through each step of the research process, at your own pace.
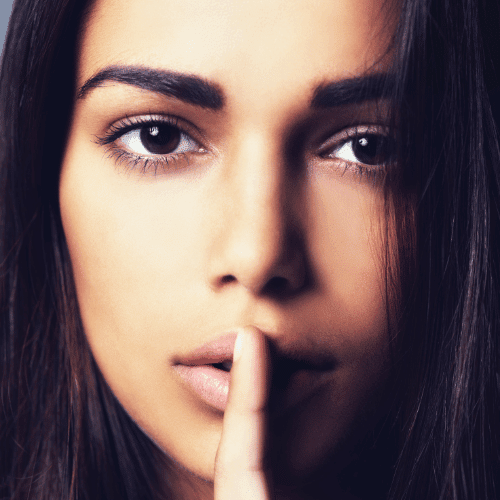
Psst... there’s more!
This post was based on one of our popular Research Bootcamps . If you're working on a research project, you'll definitely want to check this out ...
Excellent and helpful. Best site to get a full understanding of Research methodology. I’m nolonger as “clueless “..😉
Excellent and helpful for junior researcher!
Grad Coach tutorials are excellent – I recommend them to everyone doing research. I will be working with a sample of imprisoned women and now have a much clearer idea concerning sampling. Thank you to all at Grad Coach for generously sharing your expertise with students.
Submit a Comment Cancel reply
Your email address will not be published. Required fields are marked *
Save my name, email, and website in this browser for the next time I comment.
- Print Friendly
Sampling Methods In Reseach: Types, Techniques, & Examples
Saul McLeod, PhD
Editor-in-Chief for Simply Psychology
BSc (Hons) Psychology, MRes, PhD, University of Manchester
Saul McLeod, PhD., is a qualified psychology teacher with over 18 years of experience in further and higher education. He has been published in peer-reviewed journals, including the Journal of Clinical Psychology.
Learn about our Editorial Process
Olivia Guy-Evans, MSc
Associate Editor for Simply Psychology
BSc (Hons) Psychology, MSc Psychology of Education
Olivia Guy-Evans is a writer and associate editor for Simply Psychology. She has previously worked in healthcare and educational sectors.
On This Page:
Sampling methods in psychology refer to strategies used to select a subset of individuals (a sample) from a larger population, to study and draw inferences about the entire population. Common methods include random sampling, stratified sampling, cluster sampling, and convenience sampling. Proper sampling ensures representative, generalizable, and valid research results.
- Sampling : the process of selecting a representative group from the population under study.
- Target population : the total group of individuals from which the sample might be drawn.
- Sample: a subset of individuals selected from a larger population for study or investigation. Those included in the sample are termed “participants.”
- Generalizability : the ability to apply research findings from a sample to the broader target population, contingent on the sample being representative of that population.
For instance, if the advert for volunteers is published in the New York Times, this limits how much the study’s findings can be generalized to the whole population, because NYT readers may not represent the entire population in certain respects (e.g., politically, socio-economically).
The Purpose of Sampling
We are interested in learning about large groups of people with something in common in psychological research. We call the group interested in studying our “target population.”
In some types of research, the target population might be as broad as all humans. Still, in other types of research, the target population might be a smaller group, such as teenagers, preschool children, or people who misuse drugs.
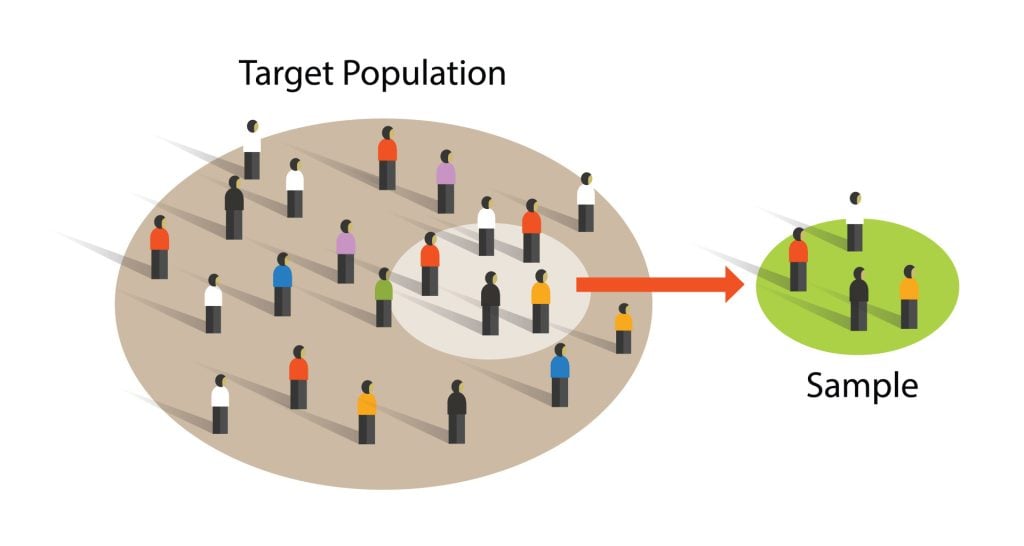
Studying every person in a target population is more or less impossible. Hence, psychologists select a sample or sub-group of the population that is likely to be representative of the target population we are interested in.
This is important because we want to generalize from the sample to the target population. The more representative the sample, the more confident the researcher can be that the results can be generalized to the target population.
One of the problems that can occur when selecting a sample from a target population is sampling bias. Sampling bias refers to situations where the sample does not reflect the characteristics of the target population.
Many psychology studies have a biased sample because they have used an opportunity sample that comprises university students as their participants (e.g., Asch ).
OK, so you’ve thought up this brilliant psychological study and designed it perfectly. But who will you try it out on, and how will you select your participants?
There are various sampling methods. The one chosen will depend on a number of factors (such as time, money, etc.).
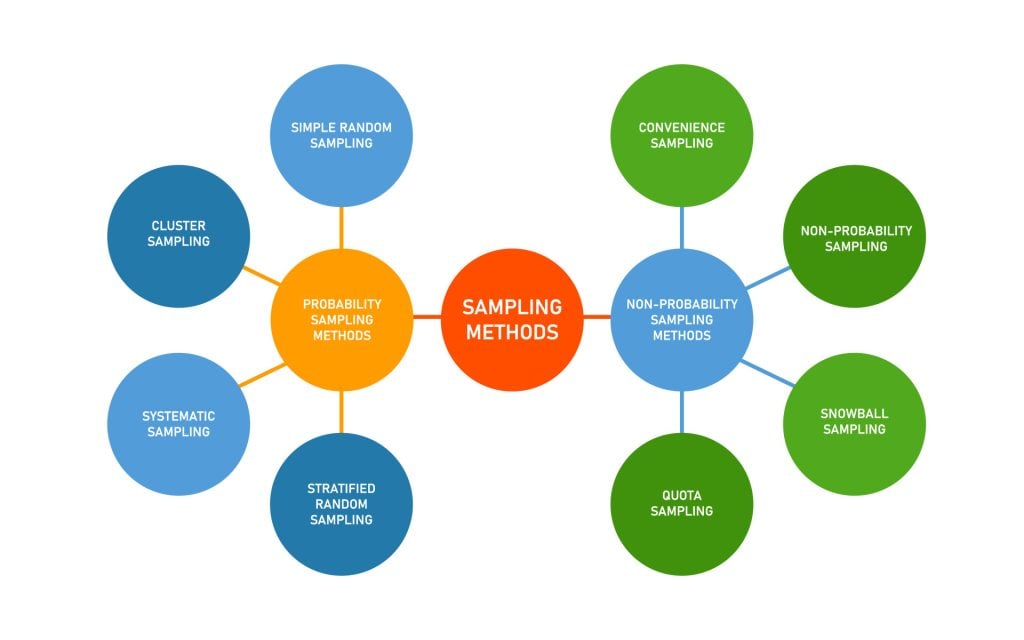
Random Sampling
Random sampling is a type of probability sampling where everyone in the entire target population has an equal chance of being selected.
This is similar to the national lottery. If the “population” is everyone who bought a lottery ticket, then everyone has an equal chance of winning the lottery (assuming they all have one ticket each).
Random samples require naming or numbering the target population and then using some raffle method to choose those to make up the sample. Random samples are the best method of selecting your sample from the population of interest.
- The advantages are that your sample should represent the target population and eliminate sampling bias.
- The disadvantage is that it is very difficult to achieve (i.e., time, effort, and money).
Stratified Sampling
During stratified sampling , the researcher identifies the different types of people that make up the target population and works out the proportions needed for the sample to be representative.
A list is made of each variable (e.g., IQ, gender, etc.) that might have an effect on the research. For example, if we are interested in the money spent on books by undergraduates, then the main subject studied may be an important variable.
For example, students studying English Literature may spend more money on books than engineering students, so if we use a large percentage of English students or engineering students, our results will not be accurate.
We have to determine the relative percentage of each group at a university, e.g., Engineering 10%, Social Sciences 15%, English 20%, Sciences 25%, Languages 10%, Law 5%, and Medicine 15%. The sample must then contain all these groups in the same proportion as the target population (university students).
- The disadvantage of stratified sampling is that gathering such a sample would be extremely time-consuming and difficult to do. This method is rarely used in Psychology.
- However, the advantage is that the sample should be highly representative of the target population, and therefore we can generalize from the results obtained.
Opportunity Sampling
Opportunity sampling is a method in which participants are chosen based on their ease of availability and proximity to the researcher, rather than using random or systematic criteria. It’s a type of convenience sampling .
An opportunity sample is obtained by asking members of the population of interest if they would participate in your research. An example would be selecting a sample of students from those coming out of the library.
- This is a quick and easy way of choosing participants (advantage)
- It may not provide a representative sample and could be biased (disadvantage).
Systematic Sampling
Systematic sampling is a method where every nth individual is selected from a list or sequence to form a sample, ensuring even and regular intervals between chosen subjects.
Participants are systematically selected (i.e., orderly/logical) from the target population, like every nth participant on a list of names.
To take a systematic sample, you list all the population members and then decide upon a sample you would like. By dividing the number of people in the population by the number of people you want in your sample, you get a number we will call n.
If you take every nth name, you will get a systematic sample of the correct size. If, for example, you wanted to sample 150 children from a school of 1,500, you would take every 10th name.
- The advantage of this method is that it should provide a representative sample.
Sample size
The sample size is a critical factor in determining the reliability and validity of a study’s findings. While increasing the sample size can enhance the generalizability of results, it’s also essential to balance practical considerations, such as resource constraints and diminishing returns from ever-larger samples.
Reliability and Validity
Reliability refers to the consistency and reproducibility of research findings across different occasions, researchers, or instruments. A small sample size may lead to inconsistent results due to increased susceptibility to random error or the influence of outliers. In contrast, a larger sample minimizes these errors, promoting more reliable results.
Validity pertains to the accuracy and truthfulness of research findings. For a study to be valid, it should accurately measure what it intends to do. A small, unrepresentative sample can compromise external validity, meaning the results don’t generalize well to the larger population. A larger sample captures more variability, ensuring that specific subgroups or anomalies don’t overly influence results.
Practical Considerations
Resource Constraints : Larger samples demand more time, money, and resources. Data collection becomes more extensive, data analysis more complex, and logistics more challenging.
Diminishing Returns : While increasing the sample size generally leads to improved accuracy and precision, there’s a point where adding more participants yields only marginal benefits. For instance, going from 50 to 500 participants might significantly boost a study’s robustness, but jumping from 10,000 to 10,500 might not offer a comparable advantage, especially considering the added costs.

- En español – ExME
- Em português – EME
What are sampling methods and how do you choose the best one?
Posted on 18th November 2020 by Mohamed Khalifa
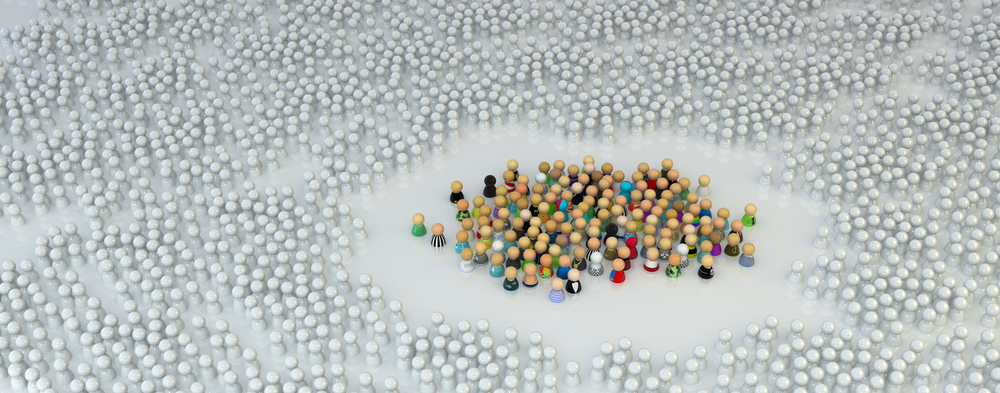
This tutorial will introduce sampling methods and potential sampling errors to avoid when conducting medical research.
Introduction to sampling methods
Examples of different sampling methods, choosing the best sampling method.
It is important to understand why we sample the population; for example, studies are built to investigate the relationships between risk factors and disease. In other words, we want to find out if this is a true association, while still aiming for the minimum risk for errors such as: chance, bias or confounding .
However, it would not be feasible to experiment on the whole population, we would need to take a good sample and aim to reduce the risk of having errors by proper sampling technique.
What is a sampling frame?
A sampling frame is a record of the target population containing all participants of interest. In other words, it is a list from which we can extract a sample.
What makes a good sample?
A good sample should be a representative subset of the population we are interested in studying, therefore, with each participant having equal chance of being randomly selected into the study.
We could choose a sampling method based on whether we want to account for sampling bias; a random sampling method is often preferred over a non-random method for this reason. Random sampling examples include: simple, systematic, stratified, and cluster sampling. Non-random sampling methods are liable to bias, and common examples include: convenience, purposive, snowballing, and quota sampling. For the purposes of this blog we will be focusing on random sampling methods .
Example: We want to conduct an experimental trial in a small population such as: employees in a company, or students in a college. We include everyone in a list and use a random number generator to select the participants
Advantages: Generalisable results possible, random sampling, the sampling frame is the whole population, every participant has an equal probability of being selected
Disadvantages: Less precise than stratified method, less representative than the systematic method
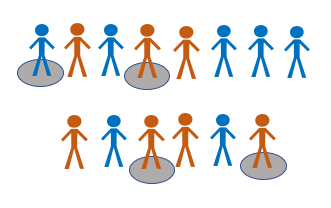
Example: Every nth patient entering the out-patient clinic is selected and included in our sample
Advantages: More feasible than simple or stratified methods, sampling frame is not always required
Disadvantages: Generalisability may decrease if baseline characteristics repeat across every nth participant
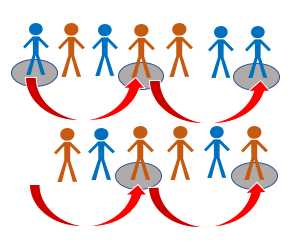
Example: We have a big population (a city) and we want to ensure representativeness of all groups with a pre-determined characteristic such as: age groups, ethnic origin, and gender
Advantages: Inclusive of strata (subgroups), reliable and generalisable results
Disadvantages: Does not work well with multiple variables
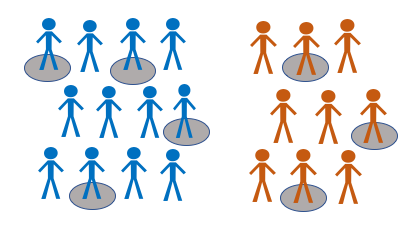
Example: 10 schools have the same number of students across the county. We can randomly select 3 out of 10 schools as our clusters
Advantages: Readily doable with most budgets, does not require a sampling frame
Disadvantages: Results may not be reliable nor generalisable
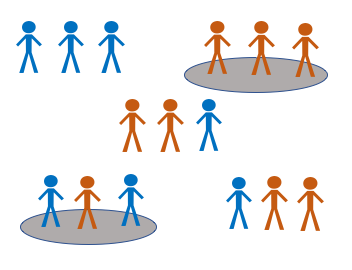
How can you identify sampling errors?
Non-random selection increases the probability of sampling (selection) bias if the sample does not represent the population we want to study. We could avoid this by random sampling and ensuring representativeness of our sample with regards to sample size.
An inadequate sample size decreases the confidence in our results as we may think there is no significant difference when actually there is. This type two error results from having a small sample size, or from participants dropping out of the sample.
In medical research of disease, if we select people with certain diseases while strictly excluding participants with other co-morbidities, we run the risk of diagnostic purity bias where important sub-groups of the population are not represented.
Furthermore, measurement bias may occur during re-collection of risk factors by participants (recall bias) or assessment of outcome where people who live longer are associated with treatment success, when in fact people who died were not included in the sample or data analysis (survivors bias).
By following the steps below we could choose the best sampling method for our study in an orderly fashion.
Research objectiveness
Firstly, a refined research question and goal would help us define our population of interest. If our calculated sample size is small then it would be easier to get a random sample. If, however, the sample size is large, then we should check if our budget and resources can handle a random sampling method.
Sampling frame availability
Secondly, we need to check for availability of a sampling frame (Simple), if not, could we make a list of our own (Stratified). If neither option is possible, we could still use other random sampling methods, for instance, systematic or cluster sampling.
Study design
Moreover, we could consider the prevalence of the topic (exposure or outcome) in the population, and what would be the suitable study design. In addition, checking if our target population is widely varied in its baseline characteristics. For example, a population with large ethnic subgroups could best be studied using a stratified sampling method.
Random sampling
Finally, the best sampling method is always the one that could best answer our research question while also allowing for others to make use of our results (generalisability of results). When we cannot afford a random sampling method, we can always choose from the non-random sampling methods.
To sum up, we now understand that choosing between random or non-random sampling methods is multifactorial. We might often be tempted to choose a convenience sample from the start, but that would not only decrease precision of our results, and would make us miss out on producing research that is more robust and reliable.
References (pdf)
Mohamed Khalifa
Leave a reply cancel reply.
Your email address will not be published. Required fields are marked *
Save my name, email, and website in this browser for the next time I comment.
No Comments on What are sampling methods and how do you choose the best one?
Thank you for this overview. A concise approach for research.
really helps! am an ecology student preparing to write my lab report for sampling.
I learned a lot to the given presentation.. It’s very comprehensive… Thanks for sharing…
Very informative and useful for my study. Thank you
Oversimplified info on sampling methods. Probabilistic of the sampling and sampling of samples by chance does rest solely on the random methods. Factors such as the random visits or presentation of the potential participants at clinics or sites could be sufficiently random in nature and should be used for the sake of efficiency and feasibility. Nevertheless, this approach has to be taken only after careful thoughts. Representativeness of the study samples have to be checked at the end or during reporting by comparing it to the published larger studies or register of some kind in/from the local population.
Thank you so much Mr.mohamed very useful and informative article
Subscribe to our newsletter
You will receive our monthly newsletter and free access to Trip Premium.
Related Articles
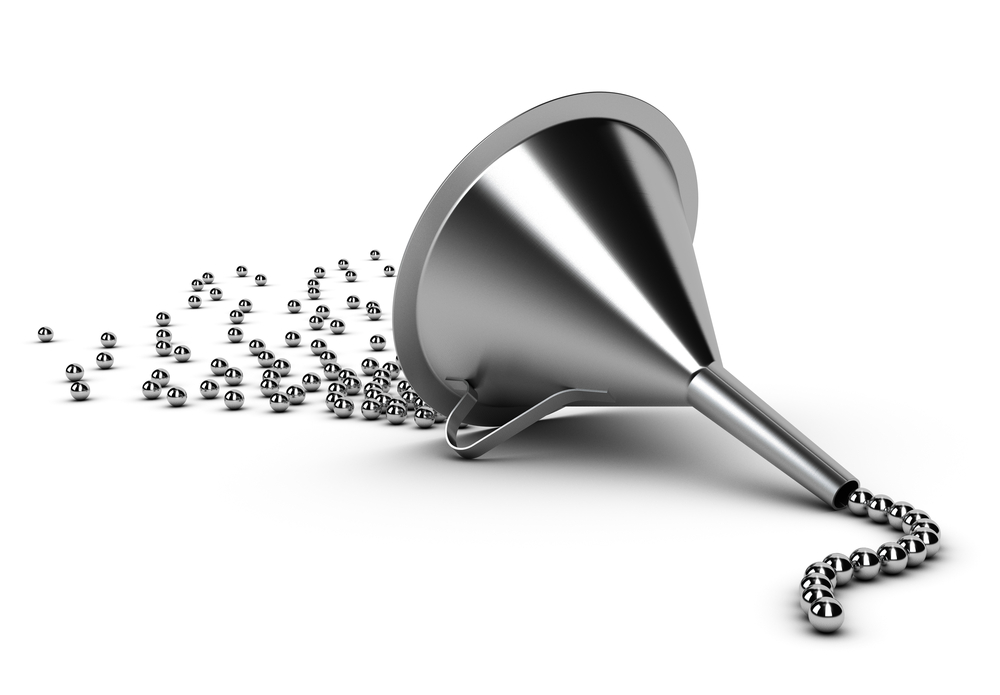
How to read a funnel plot
This blog introduces you to funnel plots, guiding you through how to read them and what may cause them to look asymmetrical.
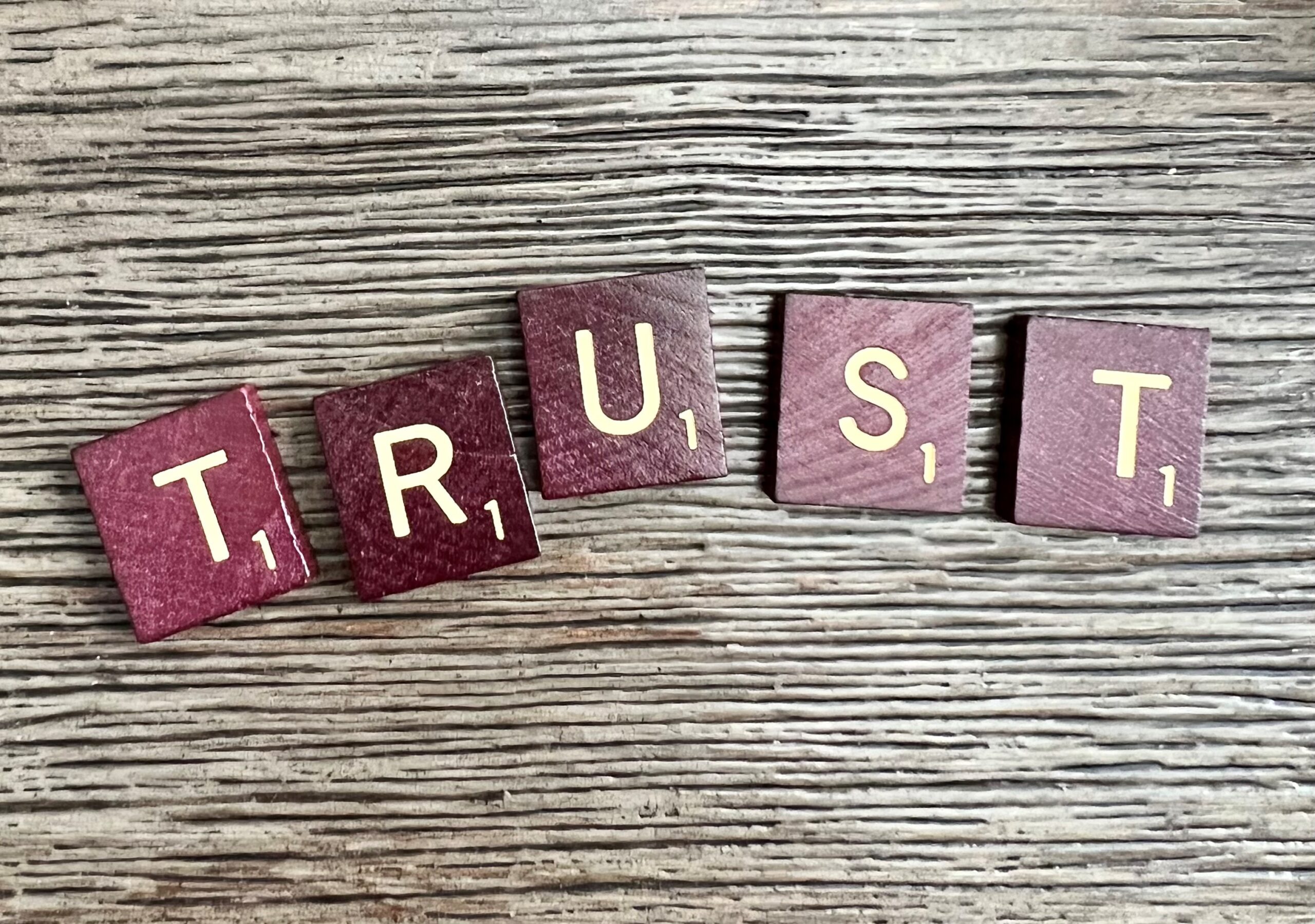
Internal and external validity: what are they and how do they differ?
Is this study valid? Can I trust this study’s methods and design? Can I apply the results of this study to other contexts? Learn more about internal and external validity in research to help you answer these questions when you next look at a paper.
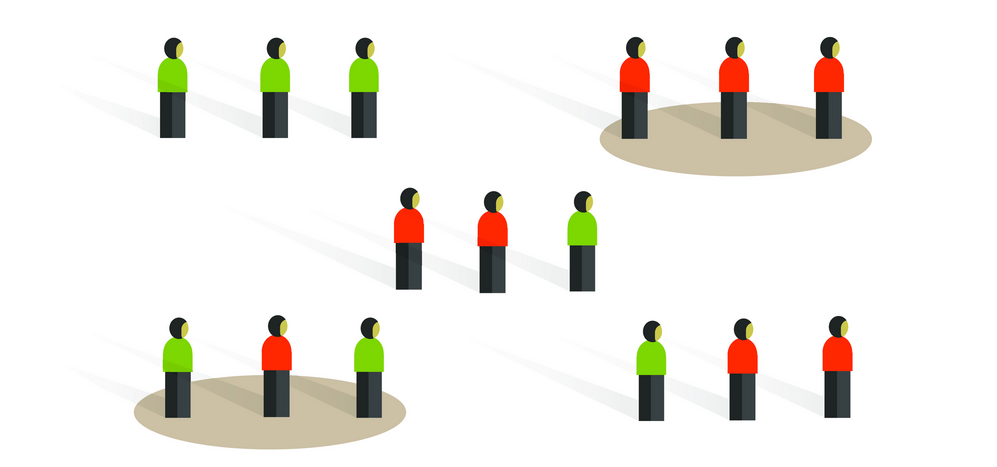
Cluster Randomized Trials: Concepts
This blog summarizes the concepts of cluster randomization, and the logistical and statistical considerations while designing a cluster randomized controlled trial.
Root out friction in every digital experience, super-charge conversion rates, and optimize digital self-service
Uncover insights from any interaction, deliver AI-powered agent coaching, and reduce cost to serve
Increase revenue and loyalty with real-time insights and recommendations delivered to teams on the ground
Know how your people feel and empower managers to improve employee engagement, productivity, and retention
Take action in the moments that matter most along the employee journey and drive bottom line growth
Whatever they’re are saying, wherever they’re saying it, know exactly what’s going on with your people
Get faster, richer insights with qual and quant tools that make powerful market research available to everyone
Run concept tests, pricing studies, prototyping + more with fast, powerful studies designed by UX research experts
Track your brand performance 24/7 and act quickly to respond to opportunities and challenges in your market
Explore the platform powering Experience Management
- Free Account
- Product Demos
- For Digital
- For Customer Care
- For Human Resources
- For Researchers
- Financial Services
- All Industries
Popular Use Cases
- Customer Experience
- Employee Experience
- Net Promoter Score
- Voice of Customer
- Customer Success Hub
- Product Documentation
- Training & Certification
- XM Institute
- Popular Resources
- Customer Stories
- Artificial Intelligence
- Market Research
- Partnerships
- Marketplace
The annual gathering of the experience leaders at the world’s iconic brands building breakthrough business results, live in Salt Lake City.
- English/AU & NZ
- Español/Europa
- Español/América Latina
- Português Brasileiro
- REQUEST DEMO
- Experience Management
- Sampling Methods
Try Qualtrics for free
Sampling methods, types & techniques.
15 min read Your comprehensive guide to the different sampling methods available to researchers – and how to know which is right for your research.
Author: Will Webster
What is sampling?
In survey research, sampling is the process of using a subset of a population to represent the whole population. To help illustrate this further, let’s look at data sampling methods with examples below.
Let’s say you wanted to do some research on everyone in North America. To ask every person would be almost impossible. Even if everyone said “yes”, carrying out a survey across different states, in different languages and timezones, and then collecting and processing all the results , would take a long time and be very costly.
Sampling allows large-scale research to be carried out with a more realistic cost and time-frame because it uses a smaller number of individuals in the population with representative characteristics to stand in for the whole.
However, when you decide to sample, you take on a new task. You have to decide who is part of your sample list and how to choose the people who will best represent the whole population. How you go about that is what the practice of sampling is all about.
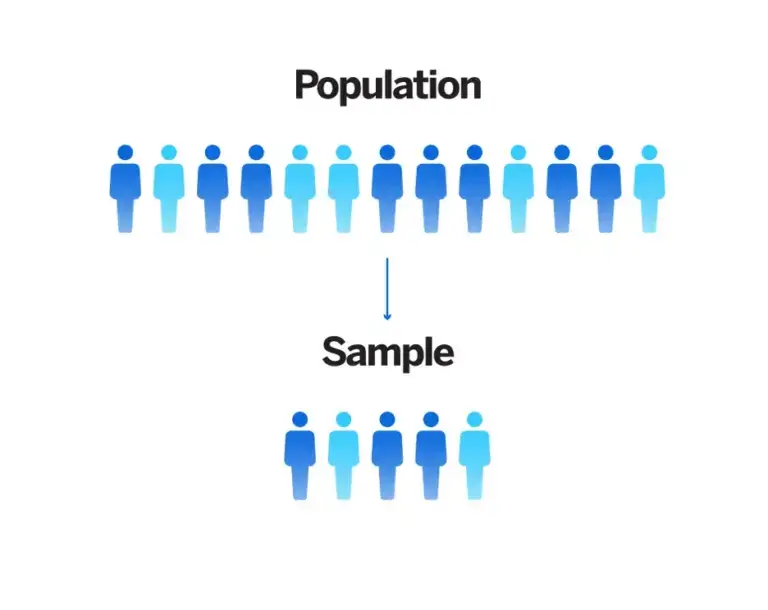
Sampling definitions
- Population: The total number of people or things you are interested in
- Sample: A smaller number within your population that will represent the whole
- Sampling: The process and method of selecting your sample
Free eBook: 2024 Market Research Trends
Why is sampling important?
Although the idea of sampling is easiest to understand when you think about a very large population, it makes sense to use sampling methods in research studies of all types and sizes. After all, if you can reduce the effort and cost of doing a study, why wouldn’t you? And because sampling allows you to research larger target populations using the same resources as you would smaller ones, it dramatically opens up the possibilities for research.
Sampling is a little like having gears on a car or bicycle. Instead of always turning a set of wheels of a specific size and being constrained by their physical properties, it allows you to translate your effort to the wheels via the different gears, so you’re effectively choosing bigger or smaller wheels depending on the terrain you’re on and how much work you’re able to do.
Sampling allows you to “gear” your research so you’re less limited by the constraints of cost, time, and complexity that come with different population sizes.
It allows us to do things like carrying out exit polls during elections, map the spread and effects rates of epidemics across geographical areas, and carry out nationwide census research that provides a snapshot of society and culture.
Types of sampling
Sampling strategies in research vary widely across different disciplines and research areas, and from study to study.
There are two major types of sampling methods: probability and non-probability sampling.
- Probability sampling , also known as random sampling , is a kind of sample selection where randomization is used instead of deliberate choice. Each member of the population has a known, non-zero chance of being selected.
- Non-probability sampling techniques are where the researcher deliberately picks items or individuals for the sample based on non-random factors such as convenience, geographic availability, or costs.
As we delve into these categories, it’s essential to understand the nuances and applications of each method to ensure that the chosen sampling strategy aligns with the research goals.
Probability sampling methods
There’s a wide range of probability sampling methods to explore and consider. Here are some of the best-known options.
1. Simple random sampling
With simple random sampling , every element in the population has an equal chance of being selected as part of the sample. It’s something like picking a name out of a hat. Simple random sampling can be done by anonymizing the population – e.g. by assigning each item or person in the population a number and then picking numbers at random.
Pros: Simple random sampling is easy to do and cheap. Designed to ensure that every member of the population has an equal chance of being selected, it reduces the risk of bias compared to non-random sampling.
Cons: It offers no control for the researcher and may lead to unrepresentative groupings being picked by chance.
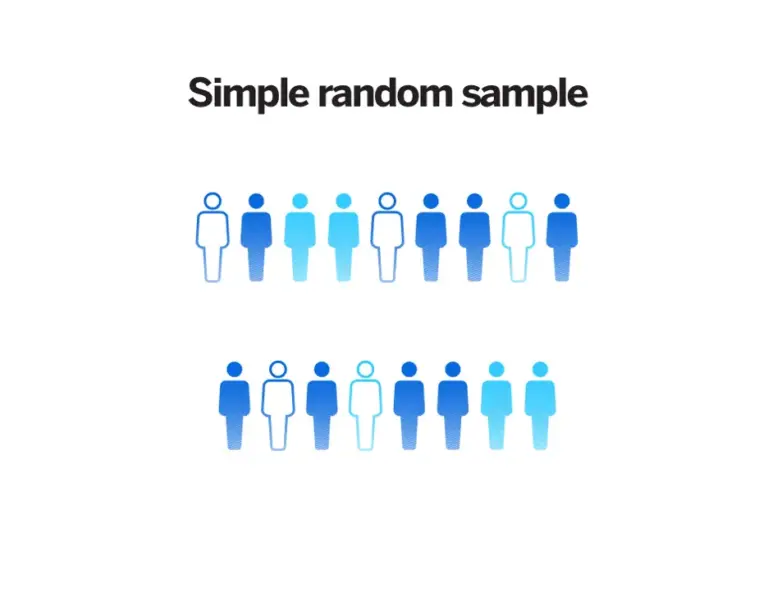
2. Systematic sampling
With systematic sampling the random selection only applies to the first item chosen. A rule then applies so that every nth item or person after that is picked.
Best practice is to sort your list in a random way to ensure that selections won’t be accidentally clustered together. This is commonly achieved using a random number generator. If that’s not available you might order your list alphabetically by first name and then pick every fifth name to eliminate bias, for example.
Next, you need to decide your sampling interval – for example, if your sample will be 10% of your full list, your sampling interval is one in 10 – and pick a random start between one and 10 – for example three. This means you would start with person number three on your list and pick every tenth person.
Pros: Systematic sampling is efficient and straightforward, especially when dealing with populations that have a clear order. It ensures a uniform selection across the population.
Cons: There’s a potential risk of introducing bias if there’s an unrecognized pattern in the population that aligns with the sampling interval.
3. Stratified sampling
Stratified sampling involves random selection within predefined groups. It’s a useful method for researchers wanting to determine what aspects of a sample are highly correlated with what’s being measured. They can then decide how to subdivide (stratify) it in a way that makes sense for the research.
For example, you want to measure the height of students at a college where 80% of students are female and 20% are male. We know that gender is highly correlated with height, and if we took a simple random sample of 200 students (out of the 2,000 who attend the college), we could by chance get 200 females and not one male. This would bias our results and we would underestimate the height of students overall. Instead, we could stratify by gender and make sure that 20% of our sample (40 students) are male and 80% (160 students) are female.
Pros: Stratified sampling enhances the representation of all identified subgroups within a population, leading to more accurate results in heterogeneous populations.
Cons: This method requires accurate knowledge about the population’s stratification, and its design and execution can be more intricate than other methods.
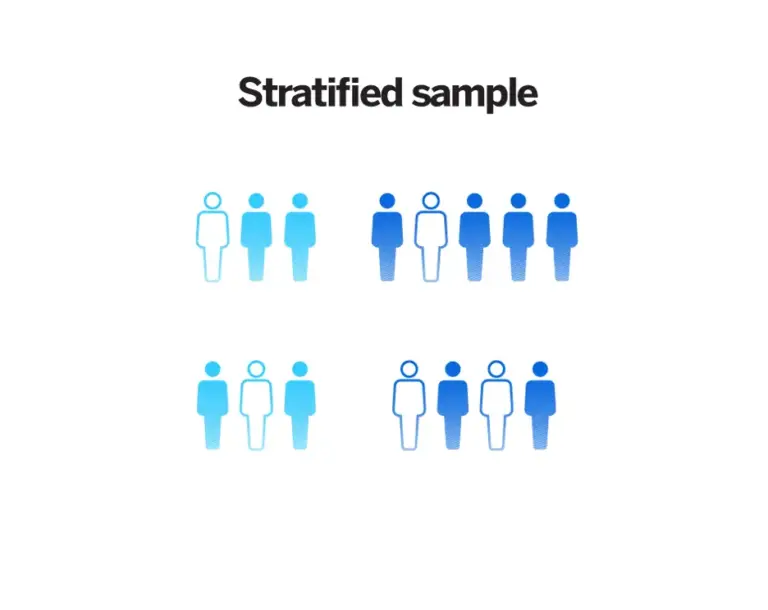
4. Cluster sampling
With cluster sampling, groups rather than individual units of the target population are selected at random for the sample. These might be pre-existing groups, such as people in certain zip codes or students belonging to an academic year.
Cluster sampling can be done by selecting the entire cluster, or in the case of two-stage cluster sampling, by randomly selecting the cluster itself, then selecting at random again within the cluster.
Pros: Cluster sampling is economically beneficial and logistically easier when dealing with vast and geographically dispersed populations.
Cons: Due to potential similarities within clusters, this method can introduce a greater sampling error compared to other methods.
Non-probability sampling methods
The non-probability sampling methodology doesn’t offer the same bias-removal benefits as probability sampling, but there are times when these types of sampling are chosen for expediency or simplicity. Here are some forms of non-probability sampling and how they work.
1. Convenience sampling
People or elements in a sample are selected on the basis of their accessibility and availability. If you are doing a research survey and you work at a university, for example, a convenience sample might consist of students or co-workers who happen to be on campus with open schedules who are willing to take your questionnaire .
This kind of sample can have value, especially if it’s done as an early or preliminary step, but significant bias will be introduced.
Pros: Convenience sampling is the most straightforward method, requiring minimal planning, making it quick to implement.
Cons: Due to its non-random nature, the method is highly susceptible to biases, and the results are often lacking in their application to the real world.
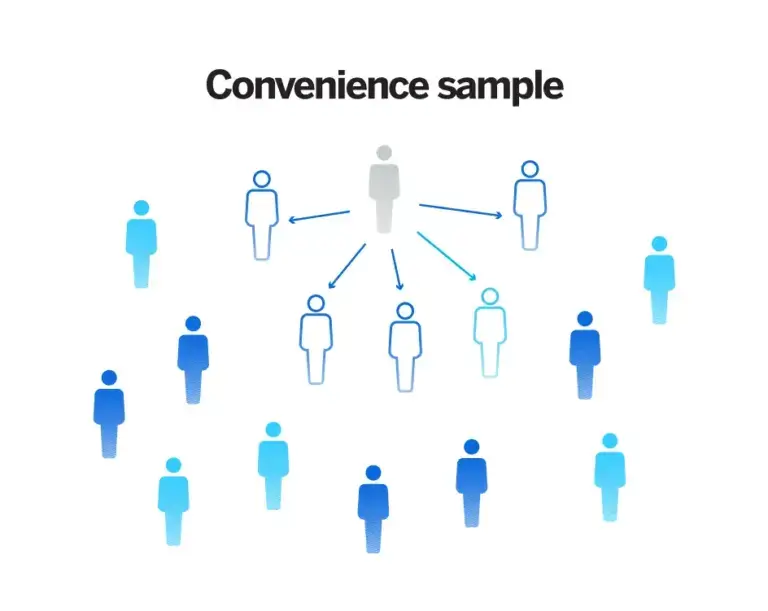
2. Quota sampling
Like the probability-based stratified sampling method, this approach aims to achieve a spread across the target population by specifying who should be recruited for a survey according to certain groups or criteria.
For example, your quota might include a certain number of males and a certain number of females. Alternatively, you might want your samples to be at a specific income level or in certain age brackets or ethnic groups.
Pros: Quota sampling ensures certain subgroups are adequately represented, making it great for when random sampling isn’t feasible but representation is necessary.
Cons: The selection within each quota is non-random and researchers’ discretion can influence the representation, which both strongly increase the risk of bias.
3. Purposive sampling
Participants for the sample are chosen consciously by researchers based on their knowledge and understanding of the research question at hand or their goals.
Also known as judgment sampling, this technique is unlikely to result in a representative sample , but it is a quick and fairly easy way to get a range of results or responses.
Pros: Purposive sampling targets specific criteria or characteristics, making it ideal for studies that require specialized participants or specific conditions.
Cons: It’s highly subjective and based on researchers’ judgment, which can introduce biases and limit the study’s real-world application.
4. Snowball or referral sampling
With this approach, people recruited to be part of a sample are asked to invite those they know to take part, who are then asked to invite their friends and family and so on. The participation radiates through a community of connected individuals like a snowball rolling downhill.
Pros: Especially useful for hard-to-reach or secretive populations, snowball sampling is effective for certain niche studies.
Cons: The method can introduce bias due to the reliance on participant referrals, and the choice of initial seeds can significantly influence the final sample.
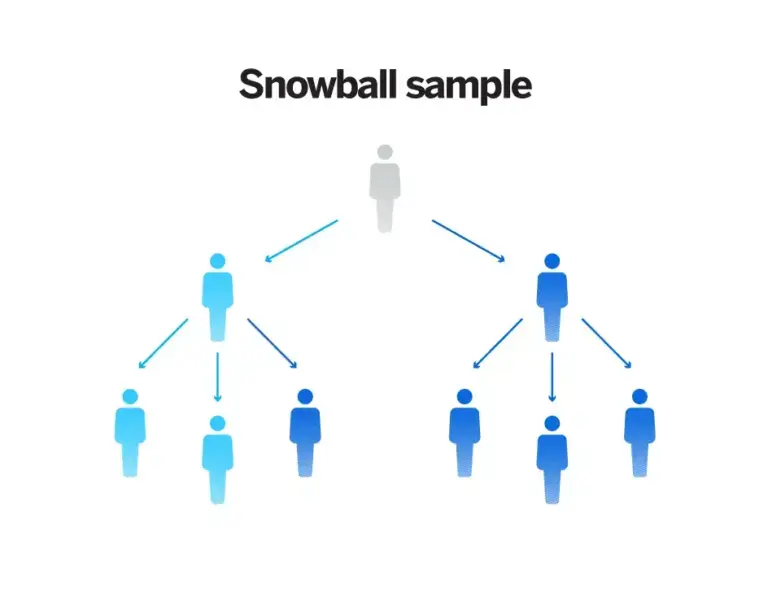
What type of sampling should I use?
Choosing the right sampling method is a pivotal aspect of any research process, but it can be a stumbling block for many.
Here’s a structured approach to guide your decision.
1) Define your research goals
If you aim to get a general sense of a larger group, simple random or stratified sampling could be your best bet. For focused insights or studying unique communities, snowball or purposive sampling might be more suitable.
2) Assess the nature of your population
The nature of the group you’re studying can guide your method. For a diverse group with different categories, stratified sampling can ensure all segments are covered. If they’re widely spread geographically , cluster sampling becomes useful. If they’re arranged in a certain sequence or order, systematic sampling might be effective.
3) Consider your constraints
Your available time, budget and ease of accessing participants matter. Convenience or quota sampling can be practical for quicker studies, but they come with some trade-offs. If reaching everyone in your desired group is challenging, snowball or purposive sampling can be more feasible.
4) Determine the reach of your findings
Decide if you want your findings to represent a much broader group. For a wider representation, methods that include everyone fairly (like probability sampling ) are a good option. For specialized insights into specific groups, non-probability sampling methods can be more suitable.
5) Get feedback
Before fully committing, discuss your chosen method with others in your field and consider a test run.
Avoid or reduce sampling errors and bias
Using a sample is a kind of short-cut. If you could ask every single person in a population to take part in your study and have each of them reply, you’d have a highly accurate (and very labor-intensive) project on your hands.
But since that’s not realistic, sampling offers a “good-enough” solution that sacrifices some accuracy for the sake of practicality and ease. How much accuracy you lose out on depends on how well you control for sampling error, non-sampling error, and bias in your survey design . Our blog post helps you to steer clear of some of these issues.
How to choose the correct sample size
Finding the best sample size for your target population is something you’ll need to do again and again, as it’s different for every study.
To make life easier, we’ve provided a sample size calculator . To use it, you need to know your:
- Population size
- Confidence level
- Margin of error (confidence interval)
If any of those terms are unfamiliar, have a look at our blog post on determining sample size for details of what they mean and how to find them.
Unlock the insights of yesterday to shape tomorrow
In the ever-evolving business landscape, relying on the most recent market research is paramount. Reflecting on 2022, brands and businesses can harness crucial insights to outmaneuver challenges and seize opportunities.
Equip yourself with this knowledge by exploring Qualtrics’ ‘2022 Market Research Global Trends’ report.
Delve into this comprehensive study to unearth:
- How businesses made sense of tricky situations in 2022
- Tips that really helped improve research results
- Steps to take your findings and put them into action
Related resources
How to determine sample size 12 min read, selection bias 11 min read, systematic random sampling 15 min read, convenience sampling 18 min read, probability sampling 8 min read, non-probability sampling 17 min read, stratified random sampling 12 min read, request demo.
Ready to learn more about Qualtrics?
- Skip to secondary menu
- Skip to main content
- Skip to primary sidebar
Statistics By Jim
Making statistics intuitive
Sampling Methods: Different Types in Research
By Jim Frost 3 Comments
What Are Sampling Methods?
Sampling methods are the processes by which you draw a sample from a population . When performing research, you’re typically interested in the results for an entire population. Unfortunately, they are almost always too large to study fully. Consequently, researchers use samples to draw conclusions about a population—the process of making statistical inferences.

A population is the complete set of individuals that you’re studying. A sample is the subset of the population that you actually measure, test, or evaluate and base your results. Sampling methods are how you obtain your sample.
Before beginning your study, carefully define the population because your results apply to the target population. You can define your population as narrowly as necessary to meet the needs of your study—for example, adult Swedish women who are otherwise healthy but have osteoporosis. Then choose your sampling method.
Learn more about populations and samples , inferential vs. descriptive statistics and populations and parameters .
In research and inferential statistics , sampling methods are a vital issue. How you draw your sample affects how much you can trust the results! If your sample doesn’t reflect the population, your results might not be valid. It’s a crucial part of experimental design .
In this post, learn more about sampling methods, which ones produce representative samples, and the pros and cons of each procedure.
Probability vs Non-Probability Sampling Methods
Sampling methods have the following two broad categories:
- Probability sampling : Entails random selection and typically, but not always, requires a list of the entire population.
- Non-probability sampling : Does not use random selection but some other process, such as convenience. Usually does not sample from the whole population.
Probability sampling is typically more difficult and costly to implement, but, in exchange, these processes tend to increase validity by producing representative samples. In short, you can make valid conclusions about the population. A statistical inference is when you use a sample to learn about a population. Learn more about Making Statistical Inferences .
On the other hand, non-probability sampling methods are often easier and less expensive, but the trade-off is that the validity of your conclusions is questionable. You might not be able to trust the results. Sampling bias is more likely to occur.
Learn more about Validity in Research and Psychology: Types & Examples and Internal and External Validity .
Probability Sampling Methods
Given the benefits of using representative samples, you’ll typically want to use a probability sampling method whenever possible. Let’s go over the standard methods. They each have pros and cons. Click the links to learn more about each sampling method and see examples. Learn more about representative samples .
To use a probability method, you’ll first need to develop a sampling frame, which lists all members of your target population. Then you can use one of the following methods.
Learn more about Sampling Frames: Definition, Examples & Uses .
Simple Random Sampling (SRS)
In simple random sampling (SRS), researchers take a complete list of the population and randomly select participants from it. All population members have an equal likelihood of being selected. Out of all sampling methods, statisticians consider this one to be the gold standard for producing representative samples. It’s entirely random, leaving little room for accidentally biasing the results.
However, this sampling method has some drawbacks.
First and foremost, this method can be pretty unwieldy and require abundant resources. For one thing, it requires a list of all population members, which can be a tremendous hurdle by itself. Attempting to perform SRS with an incomplete population list causes undercoverage bias and a nonrepresentative sample.
Furthermore, while random selection is beneficial, it also ensures that the subjects are maximally dispersed, making them harder to contact.
SRS can exclude smaller but crucial subpopulations purely by chance. Additionally, this approach produces less precise estimates for subgroups and the differences between subgroups than some other probability sampling methods.
Learn more about Simple Random Sampling and Undercoverage Bias: Definition & Examples
Systematic Sampling
Systematic sampling is similar to SRS but attempts to ease some of the difficulties for researchers. There are several versions of this method.
One form uses a complete list of the population. The researchers randomly select the first subject and then move down the list choosing every X th subject rather than using a randomized technique.
The other form does not use a complete list of the population. This sampling method is suitable for populations that are tough to document, such as the homeless, because a comprehensive list won’t exist. The essential requirement for this sampling method is knowing how to locate them. While it’s not perfect, it’s a feasible option when you can’t obtain the full list.
Suppose you want to survey theater patrons but lack a complete list. Instead, you can use systematic sampling and recruit every 20th person who exits the theater. This approach works because they leave randomly.
This sampling method has some disadvantages. The form that uses a complete list of the population can closely mirror the results of simple random sampling. However, the non-randomness increases the potential for manipulation, even if accidentally. Additionally, patterns in the list can unintentionally create a non-representative sample.
The form that doesn’t use a list has more potential problems. Namely, it increases the potential for missing subgroups and acquiring a non-representative sample. This sampling method increases the knowledge you must have about the population and their habits. Without that knowledge, you won’t be able to find subjects that reflect the whole population.
Learn more about Systematic Sampling .
Stratified Sampling
In stratified sampling, researchers divide a population into similar subpopulations (strata). Then they randomly sample from the strata.
This sampling method can guarantee the presence of small but vital subpopulations in the sample. Relative to SRS, this method can increase the precision of subgroup estimates and the differences between subgroups. In short, it helps researchers gain a better understanding of the subgroups. Dividing the whole population into smaller, more similar subsets can also reduce costs and simplify data collection.
The drawbacks are that this sampling method requires additional upfront knowledge and planning. The researchers must know enough about the subgroups to devise an effective strata scheme. Then they must have sufficient information about all population members to assign them to the correct strata.
Learn more about Stratified Sampling .
Cluster Sampling
Like stratified sampling, the cluster sampling method divides the whole population into smaller groups. However, unlike strata, each cluster mirrors the full diversity present in the population. Then the researchers randomly sample from some of these clusters.
The primary benefit of this sampling method is that it reduces the costs of studying large, geographically dispersed populations. Using this method, researchers don’t need to sample the entire geographic region but only certain areas because they know individual clusters are similar to the population. Additionally, they don’t need to develop a list of potential subjects for clusters from which they’re not sampling. These considerations can significantly reduce planning, administrative, and travel costs.
When researchers can’t create a list of the entire population, cluster sampling can be an excellent choice.
On the downside, cluster sampling increases the design complexity. Researchers must understand how well each cluster approximates the whole population. If the clusters don’t fully represent the population, results can be biased. In real-world studies, clusters tend to be naturally occurring groups that don’t mirror the population, which reduces the ability to draw valid conclusions.
Learn more about Cluster Sampling .
Non-Probability Sampling Methods
Non-probability sampling methods don’t use random selection, and they typically don’t use a complete population list. While these methods are simpler and less expensive, your results are more likely to be biased, reducing your ability to make sound conclusions.
Researchers often use non-probability sampling methods for exploratory research, pilot studies, and qualitative research . These sampling methods provide quick and rough assessments, help work kinks out of measurement instruments and procedures, and help refine the design for a more rigorous study in the future.
Below are several standard non-probability sampling methods:
- Convenience sampling : The main criteria for recruiting subjects are those who are easy to contact and willing to participate. There are no inclusion requirements. Online polls are a type of convenience sampling. Learn more about Convenience Sampling .
- Quota Sampling : Non-random selection of subjects from population subgroups that the researchers define. Learn more about Quota Sampling .
- Purposive sampling : Investigators use subject-area knowledge to handpick a sample they think will help their study. Learn more about Purposive Sampling .
- Snowball sampling : Researchers use subjects to find and recruit other subjects. This method is helpful when a population is hard to contact. When recruits help you find more recruits, and those help find even more, and so on, the total number snowballs. Learn more about Snowball Sampling .
As you can see, there are many sampling methods. Each one has benefits and disadvantages. When designing a study, evaluate the nature of your target population, your research goals, and the available time and resources to choose your sampling method. After deciding between the sampling methods, calculate your sample size using a power analysis .
Sampling in Developmental Science: Situations, Shortcomings, Solutions, and Standards (nih.gov)
Share this:

Reader Interactions
July 24, 2024 at 8:56 am
Hello Mr. Frost,
I would like to know whether people with mild Parkinson’s Disease symptoms are less likely to have kidney stones. Do PwP (People with Parkinson’s) have significantly less incidences of kidney stones than in the general population (~ 10%). So far, I have asked 12 people I know who has been diagnosed with Parkinson’s and 0% had kidney stones. I would like to increase my sampling size by randomly sampling members of a forum for PwP I belong to. Should I get a list of all forum subscribers and randomly select around 40 forum members to pose the question, “If you have been officially diagnosed with Parkinson’s, have also had a kidney stone?”. What would you suggest? I had posed the question in the forum before, but only PwP folks that had a Kidney stone responded.
Thanks, Mike
May 17, 2022 at 12:38 am
I think stratified sampling will work __ mke two groups as stratas _ then use SRS to obtain a complete sample .
May 15, 2022 at 7:37 pm
hi.what sampling technique will i use if my respondents are 1st yr college students awardees vs non awardees of different courses?
Comments and Questions Cancel reply
An overview of sampling methods
Last updated
27 February 2023
Reviewed by
Cathy Heath
When researching perceptions or attributes of a product, service, or people, you have two options:
Survey every person in your chosen group (the target market, or population), collate your responses, and reach your conclusions.
Select a smaller group from within your target market and use their answers to represent everyone. This option is sampling .
Sampling saves you time and money. When you use the sampling method, the whole population being studied is called the sampling frame .
The sample you choose should represent your target market, or the sampling frame, well enough to do one of the following:
Generalize your findings across the sampling frame and use them as though you had surveyed everyone
Use the findings to decide on your next step, which might involve more in-depth sampling
Make research less tedious
Dovetail streamlines research to help you uncover and share actionable insights
How was sampling developed?
Valery Glivenko and Francesco Cantelli, two mathematicians studying probability theory in the early 1900s, devised the sampling method. Their research showed that a properly chosen sample of people would reflect the larger group’s status, opinions, decisions, and decision-making steps.
They proved you don't need to survey the entire target market, thereby saving the rest of us a lot of time and money.
- Why is sampling important?
We’ve already touched on the fact that sampling saves you time and money. When you get reliable results quickly, you can act on them sooner. And the money you save can pay for something else.
It’s often easier to survey a sample than a whole population. Sample inferences can be more reliable than those you get from a very large group because you can choose your samples carefully and scientifically.
Sampling is also useful because it is often impossible to survey the entire population. You probably have no choice but to collect only a sample in the first place.
Because you’re working with fewer people, you can collect richer data, which makes your research more accurate. You can:
Ask more questions
Go into more detail
Seek opinions instead of just collecting facts
Observe user behaviors
Double-check your findings if you need to
In short, sampling works! Let's take a look at the most common sampling methods.
- Types of sampling methods
There are two main sampling methods: probability sampling and non-probability sampling. These can be further refined, which we'll cover shortly. You can then decide which approach best suits your research project.
Probability sampling method
Probability sampling is used in quantitative research , so it provides data on the survey topic in terms of numbers. Probability relates to mathematics, hence the name ‘quantitative research’. Subjects are asked questions like:
How many boxes of candy do you buy at one time?
How often do you shop for candy?
How much would you pay for a box of candy?
This method is also called random sampling because everyone in the target market has an equal chance of being chosen for the survey. It is designed to reduce sampling error for the most important variables. You should, therefore, get results that fairly reflect the larger population.
Non-probability sampling method
In this method, not everyone has an equal chance of being part of the sample. It's usually easier (and cheaper) to select people for the sample group. You choose people who are more likely to be involved in or know more about the topic you’re researching.
Non-probability sampling is used for qualitative research. Qualitative data is generated by questions like:
Where do you usually shop for candy (supermarket, gas station, etc.?)
Which candy brand do you usually buy?
Why do you like that brand?
- Probability sampling methods
Here are five ways of doing probability sampling:
Simple random sampling (basic probability sampling)
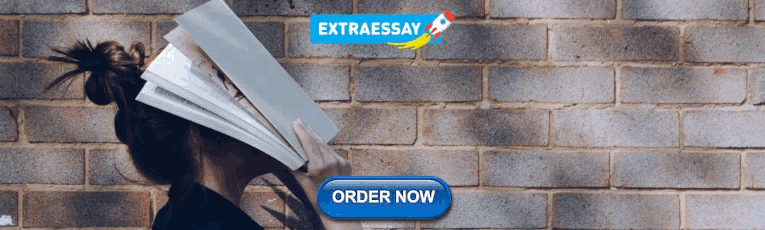
Systematic sampling
Stratified sampling.
Cluster sampling
Multi-stage sampling
Simple random sampling.
There are three basic steps to simple random sampling:
Choose your sampling frame.
Decide on your sample size. Make sure it is large enough to give you reliable data.
Randomly choose your sample participants.
You could put all their names in a hat, shake the hat to mix the names, and pull out however many names you want in your sample (without looking!)
You could be more scientific by giving each participant a number and then using a random number generator program to choose the numbers.
Instead of choosing names or numbers, you decide beforehand on a selection method. For example, collect all the names in your sampling frame and start at, for example, the fifth person on the list, then choose every fourth name or every tenth name. Alternatively, you could choose everyone whose last name begins with randomly-selected initials, such as A, G, or W.
Choose your system of selecting names, and away you go.
This is a more sophisticated way to choose your sample. You break the sampling frame down into important subgroups or strata . Then, decide how many you want in your sample, and choose an equal number (or a proportionate number) from each subgroup.
For example, you want to survey how many people in a geographic area buy candy, so you compile a list of everyone in that area. You then break that list down into, for example, males and females, then into pre-teens, teenagers, young adults, senior citizens, etc. who are male or female.
So, if there are 1,000 young male adults and 2,000 young female adults in the whole sampling frame, you may want to choose 100 males and 200 females to keep the proportions balanced. You then choose the individual survey participants through the systematic sampling method.
Clustered sampling
This method is used when you want to subdivide a sample into smaller groups or clusters that are geographically or organizationally related.
Let’s say you’re doing quantitative research into candy sales. You could choose your sample participants from urban, suburban, or rural populations. This would give you three geographic clusters from which to select your participants.
This is a more refined way of doing cluster sampling. Let’s say you have your urban cluster, which is your primary sampling unit. You can subdivide this into a secondary sampling unit, say, participants who typically buy their candy in supermarkets. You could then further subdivide this group into your ultimate sampling unit. Finally, you select the actual survey participants from this unit.
- Uses of probability sampling
Probability sampling has three main advantages:
It helps minimizes the likelihood of sampling bias. How you choose your sample determines the quality of your results. Probability sampling gives you an unbiased, randomly selected sample of your target market.
It allows you to create representative samples and subgroups within a sample out of a large or diverse target market.
It lets you use sophisticated statistical methods to select as close to perfect samples as possible.
- Non-probability sampling methods
To recap, with non-probability sampling, you choose people for your sample in a non-random way, so not everyone in your sampling frame has an equal chance of being chosen. Your research findings, therefore, may not be as representative overall as probability sampling, but you may not want them to be.
Sampling bias is not a concern if all potential survey participants share similar traits. For example, you may want to specifically focus on young male adults who spend more than others on candy. In addition, it is usually a cheaper and quicker method because you don't have to work out a complex selection system that represents the entire population in that community.
Researchers do need to be mindful of carefully considering the strengths and limitations of each method before selecting a sampling technique.
Non-probability sampling is best for exploratory research , such as at the beginning of a research project.
There are five main types of non-probability sampling methods:
Convenience sampling
Purposive sampling, voluntary response sampling, snowball sampling, quota sampling.
The strategy of convenience sampling is to choose your sample quickly and efficiently, using the least effort, usually to save money.
Let's say you want to survey the opinions of 100 millennials about a particular topic. You could send out a questionnaire over the social media platforms millennials use. Ask respondents to confirm their birth year at the top of their response sheet and, when you have your 100 responses, begin your analysis. Or you could visit restaurants and bars where millennials spend their evenings and sign people up.
A drawback of convenience sampling is that it may not yield results that apply to a broader population.
This method relies on your judgment to choose the most likely sample to deliver the most useful results. You must know enough about the survey goals and the sampling frame to choose the most appropriate sample respondents.
Your knowledge and experience save you time because you know your ideal sample candidates, so you should get high-quality results.
This method is similar to convenience sampling, but it is based on potential sample members volunteering rather than you looking for people.
You make it known you want to do a survey on a particular topic for a particular reason and wait until enough people volunteer. Then you give them the questionnaire or arrange interviews to ask your questions directly.
Snowball sampling involves asking selected participants to refer others who may qualify for the survey. This method is best used when there is no sampling frame available. It is also useful when the researcher doesn’t know much about the target population.
Let's say you want to research a niche topic that involves people who may be difficult to locate. For our candy example, this could be young males who buy a lot of candy, go rock climbing during the day, and watch adventure movies at night. You ask each participant to name others they know who do the same things, so you can contact them. As you make contact with more people, your sample 'snowballs' until you have all the names you need.
This sampling method involves collecting the specific number of units (quotas) from your predetermined subpopulations. Quota sampling is a way of ensuring that your sample accurately represents the sampling frame.
- Uses of non-probability sampling
You can use non-probability sampling when you:
Want to do a quick test to see if a more detailed and sophisticated survey may be worthwhile
Want to explore an idea to see if it 'has legs'
Launch a pilot study
Do some initial qualitative research
Have little time or money available (half a loaf is better than no bread at all)
Want to see if the initial results will help you justify a longer, more detailed, and more expensive research project
- The main types of sampling bias, and how to avoid them
Sampling bias can fog or limit your research results. This will have an impact when you generalize your results across the whole target market. The two main causes of sampling bias are faulty research design and poor data collection or recording. They can affect probability and non-probability sampling.
Faulty research
If a surveyor chooses participants inappropriately, the results will not reflect the population as a whole.
A famous example is the 1948 presidential race. A telephone survey was conducted to see which candidate had more support. The problem with the research design was that, in 1948, most people with telephones were wealthy, and their opinions were very different from voters as a whole. The research implied Dewey would win, but it was Truman who became president.
Poor data collection or recording
This problem speaks for itself. The survey may be well structured, the sample groups appropriate, the questions clear and easy to understand, and the cluster sizes appropriate. But if surveyors check the wrong boxes when they get an answer or if the entire subgroup results are lost, the survey results will be biased.
How do you minimize bias in sampling?
To get results you can rely on, you must:
Know enough about your target market
Choose one or more sample surveys to cover the whole target market properly
Choose enough people in each sample so your results mirror your target market
Have content validity . This means the content of your questions must be direct and efficiently worded. If it isn’t, the viability of your survey could be questioned. That would also be a waste of time and money, so make the wording of your questions your top focus.
If using probability sampling, make sure your sampling frame includes everyone it should and that your random sampling selection process includes the right proportion of the subgroups
If using non-probability sampling, focus on fairness, equality, and completeness in identifying your samples and subgroups. Then balance those criteria against simple convenience or other relevant factors.
What are the five types of sampling bias?
Self-selection bias. If you mass-mail questionnaires to everyone in the sample, you’re more likely to get results from people with extrovert or activist personalities and not from introverts or pragmatists. So if your convenience sampling focuses on getting your quota responses quickly, it may be skewed.
Non-response bias. Unhappy customers, stressed-out employees, or other sub-groups may not want to cooperate or they may pull out early.
Undercoverage bias. If your survey is done, say, via email or social media platforms, it will miss people without internet access, such as those living in rural areas, the elderly, or lower-income groups.
Survivorship bias. Unsuccessful people are less likely to take part. Another example may be a researcher excluding results that don’t support the overall goal. If the CEO wants to tell the shareholders about a successful product or project at the AGM, some less positive survey results may go “missing” (to take an extreme example.) The result is that your data will reflect an overly optimistic representation of the truth.
Pre-screening bias. If the researcher, whose experience and knowledge are being used to pre-select respondents in a judgmental sampling, focuses more on convenience than judgment, the results may be compromised.
How do you minimize sampling bias?
Focus on the bullet points in the next section and:
Make survey questionnaires as direct, easy, short, and available as possible, so participants are more likely to complete them accurately and send them back
Follow up with the people who have been selected but have not returned their responses
Ignore any pressure that may produce bias
- How do you decide on the type of sampling to use?
Use the ideas you've gleaned from this article to give yourself a platform, then choose the best method to meet your goals while staying within your time and cost limits.
If it isn't obvious which method you should choose, use this strategy:
Clarify your research goals
Clarify how accurate your research results must be to reach your goals
Evaluate your goals against time and budget
List the two or three most obvious sampling methods that will work for you
Confirm the availability of your resources (researchers, computer time, etc.)
Compare each of the possible methods with your goals, accuracy, precision, resource, time, and cost constraints
Make your decision
- The takeaway
Effective market research is the basis of successful marketing, advertising, and future productivity. By selecting the most appropriate sampling methods, you will collect the most useful market data and make the most effective decisions.
Should you be using a customer insights hub?
Do you want to discover previous research faster?
Do you share your research findings with others?
Do you analyze research data?
Start for free today, add your research, and get to key insights faster
Editor’s picks
Last updated: 18 April 2023
Last updated: 27 February 2023
Last updated: 22 August 2024
Last updated: 5 February 2023
Last updated: 16 August 2024
Last updated: 9 March 2023
Last updated: 30 April 2024
Last updated: 12 December 2023
Last updated: 11 March 2024
Last updated: 4 July 2024
Last updated: 6 March 2024
Last updated: 5 March 2024
Last updated: 13 May 2024
Latest articles
Related topics, .css-je19u9{-webkit-align-items:flex-end;-webkit-box-align:flex-end;-ms-flex-align:flex-end;align-items:flex-end;display:-webkit-box;display:-webkit-flex;display:-ms-flexbox;display:flex;-webkit-flex-direction:row;-ms-flex-direction:row;flex-direction:row;-webkit-box-flex-wrap:wrap;-webkit-flex-wrap:wrap;-ms-flex-wrap:wrap;flex-wrap:wrap;-webkit-box-pack:center;-ms-flex-pack:center;-webkit-justify-content:center;justify-content:center;row-gap:0;text-align:center;max-width:671px;}@media (max-width: 1079px){.css-je19u9{max-width:400px;}.css-je19u9>span{white-space:pre;}}@media (max-width: 799px){.css-je19u9{max-width:400px;}.css-je19u9>span{white-space:pre;}} decide what to .css-1kiodld{max-height:56px;display:-webkit-box;display:-webkit-flex;display:-ms-flexbox;display:flex;-webkit-align-items:center;-webkit-box-align:center;-ms-flex-align:center;align-items:center;}@media (max-width: 1079px){.css-1kiodld{display:none;}} build next, decide what to build next, log in or sign up.
Get started for free

An official website of the United States government
The .gov means it’s official. Federal government websites often end in .gov or .mil. Before sharing sensitive information, make sure you’re on a federal government site.
The site is secure. The https:// ensures that you are connecting to the official website and that any information you provide is encrypted and transmitted securely.
- Publications
- Account settings
- My Bibliography
- Collections
- Citation manager
Save citation to file
Email citation, add to collections.
- Create a new collection
- Add to an existing collection
Add to My Bibliography
Your saved search, create a file for external citation management software, your rss feed.
- Search in PubMed
- Search in NLM Catalog
- Add to Search
Sampling Methods: A guide for researchers
Affiliation.
- 1 Arizona School of Dentistry & Oral Health A.T. Still University, Mesa, AZ, USA [email protected].
- PMID: 37553279
Sampling is a critical element of research design. Different methods can be used for sample selection to ensure that members of the study population reflect both the source and target populations, including probability and non-probability sampling. Power and sample size are used to determine the number of subjects needed to answer the research question. Characteristics of individuals included in the sample population should be clearly defined to determine eligibility for study participation and improve power. Sample selection methods differ based on study design. The purpose of this short report is to review common sampling considerations and related errors.
Keywords: research design; sample size; sampling.
Copyright © 2023 The American Dental Hygienists’ Association.
PubMed Disclaimer
Similar articles
- Common Sampling Errors in Research Studies. Spolarich AE. Spolarich AE. J Dent Hyg. 2023 Dec;97(6):50-53. J Dent Hyg. 2023. PMID: 38061808
- Folic acid supplementation and malaria susceptibility and severity among people taking antifolate antimalarial drugs in endemic areas. Crider K, Williams J, Qi YP, Gutman J, Yeung L, Mai C, Finkelstain J, Mehta S, Pons-Duran C, Menéndez C, Moraleda C, Rogers L, Daniels K, Green P. Crider K, et al. Cochrane Database Syst Rev. 2022 Feb 1;2(2022):CD014217. doi: 10.1002/14651858.CD014217. Cochrane Database Syst Rev. 2022. PMID: 36321557 Free PMC article.
- [A comparison of convenience sampling and purposive sampling]. Suen LJ, Huang HM, Lee HH. Suen LJ, et al. Hu Li Za Zhi. 2014 Jun;61(3):105-11. doi: 10.6224/JN.61.3.105. Hu Li Za Zhi. 2014. PMID: 24899564 Chinese.
- Power and sample size. Case LD, Ambrosius WT. Case LD, et al. Methods Mol Biol. 2007;404:377-408. doi: 10.1007/978-1-59745-530-5_19. Methods Mol Biol. 2007. PMID: 18450060 Review.
- Concepts in sample size determination. Rao UK. Rao UK. Indian J Dent Res. 2012 Sep-Oct;23(5):660-4. doi: 10.4103/0970-9290.107385. Indian J Dent Res. 2012. PMID: 23422614 Review.
- Search in MeSH
Related information
- Citation Manager
NCBI Literature Resources
MeSH PMC Bookshelf Disclaimer
The PubMed wordmark and PubMed logo are registered trademarks of the U.S. Department of Health and Human Services (HHS). Unauthorized use of these marks is strictly prohibited.
- Technical Support
- Technical Papers
- Knowledge Base
- Question Library
Call our friendly, no-pressure support team.
Sampling Methods in Survey Research: Definition, Types, Methods, and More
Table of Contents
What Is Sampling? Why Is Sampling Important?
Sampling is the backbone for primary data collection and analysis in marketing research and the social sciences. It involves selecting a subset of individuals from a larger population to participate in a study, with the aim of making valid inferences about the entire population without needing to survey every individual within that population. Sampling makes research both practical (cost and time effective) and feasible.
The key to effective sampling lies in the concept of a representative sample —a subset that fairly mirrors the diverse characteristics of the population it is drawn from. Achieving this ensures the data collection and data analysis phases yield accurate and meaningful insights, minimizing the risk of biased (systematically wrong) or irrelevant findings.
Get Started with Your Survey Research Today!
Ready for your next research study? Get access to our free survey research tool. In just a few minutes, you can create powerful surveys with our easy-to-use interface.
Start Survey Research for Free or Request a Product Tour
“Dewey Beats Truman” USA Presidential Election 1948 Example
The classic example showing how poor sampling procedures can lead to erroneous conclusions about the population is had in the oft-cited “Dewey Beats Truman” false headline newspaper article on November 3, 1948, the day after Truman had actually beaten Dewey to win the presidency of the United States of America.
How did the newspaper get it wrong? Conventional wisdom, based on various polls with large sample sizes, predicted that Dewey would win. Facing the printing deadline and anxious to be able to report the winner of the election the next morning, they decided to print the expected results prior to the election results being finalized. “Dewey Defeats Truman” the headline trumpeted; but they had gotten it wrong.
(President Harry Truman gleefully showing the erroneous headline) Image Credit: Byron Rollins, The Washington Post
There were multiple reasons that the polls didn’t get it right. Prominent among those was that telephone polls in 1948 led to a biased sample of respondents who tended to favor Dewey, because telephones were something more commonly found in well-to-do households. Well-to-do households were more likely to vote for Dewey. The sample used to project that Dewey would beat Truman, though substantial, was nonetheless biased.
Key Sampling Definitions: Population vs. Sample
Understanding the distinction between a population and sample is crucial in sampling:
- Sampling : The process of selecting a sample from a population.
- Population : The entire group of individuals or entities relevant to a particular research study, from which a sample is drawn.
- Sample : A subset of the population selected for participation in the research study.
- Sample Frame : A list or database from which the sample is drawn, ideally encompassing the entire population.
These foundational concepts lay the groundwork for understanding the various sampling methods and their applications in research, guiding the choice of method based on the research objectives and constraints.
Types of Sampling Methods in Research
Sampling methods are broadly categorized into probability and non-probability sampling , each with distinct approaches and implications for research accuracy and validity.
Probability Sampling Method
Probability sampling , the first of two primary sampling methods, ensures every member of the population has a known and non-zero chance of being selected. This method fosters objectivity and minimizes sampling bias, enhancing the representativeness of the sample.
1. Simple Random Sampling (SRS) Method
Simple random sampling (SRS) epitomizes equal chance selection, where each population member has an identical probability of being chosen. Its advantages include simplicity and reduced bias, while disadvantages may involve logistical challenges in large populations. Theory-based classroom examples involve drawing balls of different colors out of a cylinder where the balls have been thoroughly mixed. A real-world application is selecting respondents for a customer satisfaction survey from a database of all customers.
2. Systematic Sampling Method
Systemic sampling selection is made at regular intervals from an ordered list, combining efficiency with a reduced risk of selection bias. However, its systematic nature can introduce bias if the list has an underlying pattern. An application example is selecting every 100th theme park visitor coming through the turnstiles to provide feedback.
3. Stratified Sampling Method
Stratified sampling involves dividing the population into subgroups based on identifiable characteristics and randomly sampling from each. It enhances representation but is more complex to implement. A practical use case is conducting a health survey across different age groups to ensure all are adequately represented.
Stratified sampling reduces the likelihood of obtaining an unlucky sample that is not representative. For example, if we sampled 30 respondents, it’s possible you’ll obtain only a very few male respondents. If we divided the sample frame into males and females and drew 15 females and 15 males, we could ensure good representation with respect to gender. In practice, stratified sampling typically involves consideration of more than one demographic (or otherwise known) characteristic of the sample elements.
4. Cluster Sampling Method
Cluster sampling selection occurs by dividing the population into clusters and randomly selecting entire clusters. This approach is cost-effective, especially for geographically dispersed populations, but can increase sampling error. It's sometimes used in field surveys where researchers select specific neighborhoods or schools to study.
Non-Probability Sampling Method
Non-probability sampling, the second primary sampling method, does not provide every individual with a known and non-zero chance of selection, often used when probability sampling is impractical or unnecessary.
1. Convenience Sampling Method
Convenience sampling selection is based on ease of access, favoring quick and easy data collection despite inherent bias risks. Examples related to survey research involve placing a link to take a survey on social media, or using Mechanical Turk (Amazon’s crowdsourcing marketplace) where people go to do a variety of tasks, such as completing surveys, to make money.
Although we would like to think otherwise, many sample sources we rely on for marketing and social survey research purposes, including online panel samples, are in reality convenience samples.
2. Quota Sampling Method
Quota sampling involves selecting individuals to fill a "quota" such as 500 smokers in a non-random manner, such as through convenience sampling approaches.
3. Judgement Sampling Method
In judgement sampling, researchers select participants based on specific criteria and judgment, useful for targeted studies but susceptible to subjective bias. For example, selecting experts in a field for in-depth interviews.
4. Snowball Sampling Method
For hard-to-reach populations, snowball sampling relies on referrals from initial subjects to recruit further participants. While it can be effective for niche studies, it can lead to bias. A case in point is researching a rare medical condition where patients might know and might be able to refer other patients. (Snowball sampling is rarely used in practice.)
Each sampling method has its context of application, influenced by the study's objectives, the population's nature, and logistical considerations. Choosing the appropriate method is important for research validity and reliability.
Get Started with Market Research Today!
Ready for your next market research study? Get access to our free survey research tool. In just a few minutes, you can create powerful surveys with our easy-to-use interface.
Start Market Research for Free or Request a Product Tour
Differences Between Probability and Non-Probability Sampling Methods
Each sampling method has its own set of advantages and limitations, influencing the accuracy, applicability, and generalizability of research findings. Here's a summary exploration of the differences:
|
|
|
| Random selection, every member has a known and non-zero chance of being selected. | Non-random selection, not all members have a known and non-zero chance of being selected. |
| Simple random sampling, systematic sampling, stratified sampling, cluster sampling. | Convenience sampling, quota sampling, judgement sampling, snowball sampling. |
| Generally results in a representative sample. | Usually does not produce a representative sample. |
| Appropriate for inferential statistics and studies requiring generalization. | Used in exploratory research, qualitative studies, or specific subgroup focuses. |
| Reduces sampling bias, allows calculation of sampling error, more reliable. | Easier and less expensive to implement, useful for inaccessible populations. |
| More time-consuming and costly, requires comprehensive population list. | May introduce bias, limits generalization of findings to the entire population. |
Choosing the Right Sampling Method in Research
Selecting the appropriate sampling method can significantly affect the validity of your research findings. This choice should be guided by a structured decision-making process, taking into account several key considerations:
- Research Goals : Begin by clarifying whether your study seeks to gain general insights applicable to the broader population or if it focuses on specific segments or behaviors. This determination will influence whether a probability or non-probability sampling method is more appropriate.
- Nature of the Population : Consider the diversity, geographic distribution, and accessibility of your target population. Probability sampling methods are preferable for a diverse and widespread population to ensure representativeness, while non-probability methods might suffice for more homogeneous or accessible groups.
- Constraints : Practical constraints such as time, budget, and resources available for your study can significantly affect your sampling strategy. Non-probability methods often require less time and resources, making them suitable for studies with tight constraints.
- Reach of Findings : Decide on the importance of the study’s findings being representative of the broader population. Studies aiming for broad applicability should lean towards probability methods to ensure a representative sample.
- Feedback : Engaging with peers or experts in your field can provide valuable insights and feedback on your chosen sampling method. Pilot tests or preliminary studies can also help validate your approach before full-scale implementation.
When interviewing human subjects for market or social survey research, we can do our best to try to follow sound sampling procedures, but non-response bias (when the people who don’t complete the survey systematically differ from those that do) can still be a significant problem, leading to incorrect inferences about the population.
Sampling Methods Theory vs. Practice
This article mainly focuses on the theory of sampling. The theory and science of sampling leads to well-established formulas that lead to probability-based inferences we can make about the population (such as a mean with its accompanying confidence interval). But, in the practice of marketing research and social sciences, we’re dealing with humans, rather than colored balls being drawn from buckets or samples of widgets being pulled off the line at a factory.
Humans self-select themselves out of the sample, by refusing to complete surveys or by having filters on their email servers that block our emailed invitations to complete a survey. The formulas we rely on typically assume simple random sampling (SRS), whereas we rarely ever achieve SRS when conducting survey research with human respondents. To the degree that our samples are biased, the measurements and confidence intervals we obtain in surveys are also systematically incorrect. No amount of sample size (short of interviewing every human in the population) can erase the negative effects of biased sampling procedures.
Avoiding Sampling Errors and Bias
The integrity of research findings hinges on the ability to control and minimize sampling errors and biases . Sampling error refers to the natural variation that occurs by chance because a sample, rather than the entire population, is surveyed. Non-sampling error, on the other hand, encompasses all other errors in the research process, from data collection to analysis. Bias, a systematic error, can significantly alter the results (e.g., too high, too low, not variable enough, or too variable), leading to inaccurate conclusions.
Sampling Error and Bias Mitigation Strategies
- Careful Design and Planning : Developing a robust sampling plan that considers the objectives and scope of the study can help in selecting the most suitable sampling method to minimize errors and bias.
- Randomization : Employing random selection methods where possible to ensure each population member has an equal chance of being included, thereby reducing selection bias.
- Stratification : Using stratified sampling to ensure that important subgroups within the population are adequately represented can minimize sampling bias related to specific characteristics.
By proactively addressing these areas, researchers can enhance the reliability and validity of their study outcomes, ensuring that conclusions drawn are reflective of the true population characteristics.
A Note on Online Panel Providers
Whereas phone and snail mail surveys were popular in the 1940s through the 1980s for sampling from the general population, online panel providers are the most commonly used approach today. Although panel providers may report that their samples are balanced to represent the demographic characteristics of the population as a whole, the samples are not unbiased. The kinds of people who participate in panels and self-select to take surveys may be systematically different on the characteristics that are the subject of your study than those who do not participate in your survey.
Need Sample for Your Research?
Let us connect you with your ideal audience! Reach out to us to request sample for your survey research.
Request Sample
Figuring Out Sample Size
Figuring out (determining) the appropriate sample size is crucial for ensuring that your study results are statistically significant and representative of the population. An inadequately small sample size may lead to unreliable results, while an unnecessarily large sample can waste resources and time.
Factors to consider when determining sample size include:
- Population Size : Larger populations may require a larger sample to accurately reflect the population's characteristics, although the relationship is not linear.
- Confidence Level : This reflects how sure you can be that the population’s true mean falls within a certain range. 95% confidence is a common threshold. A higher confidence level requires a larger sample size.
- Margin of Error : Also known as the confidence interval, it indicates the range within which the true value is likely to lie (given a specified degree of confidence, such as 95%). A smaller margin of error requires a larger sample size.
- Power: The ability to detect true differences or relationships in statistics/variables if they in actuality exist.
Combining the elements of confidence level and margin of error, it’s common to read that a poll found that 30% of respondents said they would vote for candidate A with a margin of error of +/- 3% at the 95% confidence level. (If it isn’t explicitly stated, it is generally assumed that the research organization has assumed a 95% confidence level when calculating the margin of error.)
Several sample size calculators and formulas are available to assist researchers in selecting the sample size for determining margins of error at given confidence levels. Consulting with statistical experts or utilizing specialized software can provide additional accuracy and confidence in these calculations, especially regarding power .
False Positives (Type 1 error) vs. False Negatives (Type 2 error)
Most common sample size calculators used for survey research focus on achieving reasonably good precision, meaning tight confidence intervals (such as the common +/-3% confidence interval for proportions at 95% confidence).
Most researchers applying tests for significance (of effects or parameters) want a likelihood of 5% or lower of signaling that a difference or impact (effect) is non-zero when in reality it is not. This is called a False Positive, or a Type 1 error, and 5% “alpha” threshold for Type 1 errors is typical.
Most researchers don’t think about the danger of False Negatives (Type 2 error) when calculating needed sample size. Setting the likelihood of Type 1 errors at 5% means that you are only 50% likely to find a significant effect that actually exists for hypothesis testing purposes. To increase the power that you will be able to detect significant effects if they exist requires additional sample size. Our consultants at Sawtooth Analytics can help you with decisions regarding precision and power related to selecting an appropriate sample size.
Understanding and correctly applying sampling methods are pivotal for the success of market and survey research. The choice of sampling method directly impacts the accuracy, reliability, and applicability of the research findings. By carefully considering the research objectives, population characteristics, practical constraints, and by diligently working to avoid errors and biases, researchers can select the most appropriate sampling strategy for their studies.
Furthermore, determining the right sample size is essential for achieving statistically significant results without overextending resources. Through thoughtful planning and execution, researchers can ensure their studies contribute valuable insights that lead to good policy, decision-making, and inferences about the population.
Sawtooth Software
3210 N Canyon Rd Ste 202
Provo UT 84604-6508
United States of America
Support: [email protected]
Consulting: [email protected]
Sales: [email protected]
Products & Services
Support & Resources
- Sign Up Now
- -- Navigate To -- CR Dashboard Connect for Researchers Connect for Participants
- Log In Log Out Log In
- Recent Press
- Papers Citing Connect
- Connect for Participants
- Connect for Researchers
- Connect AI Training
- Managed Research
- Prime Panels
- MTurk Toolkit
- Health & Medicine
- Enterprise Accounts
- Conferences
- Knowledge Base
- The Online Researcher’s Guide To Sampling
What Is the Purpose of Sampling in Research?
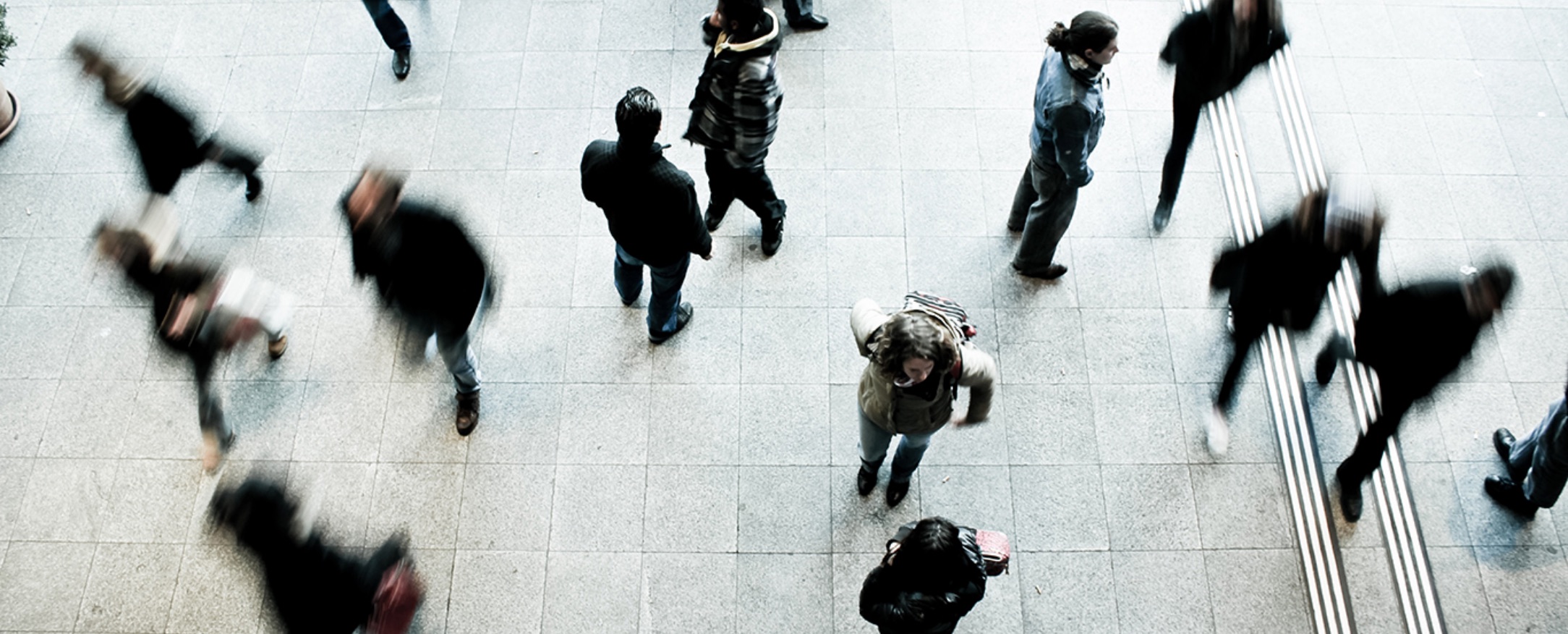
Quick Navigation:
Defining random vs. non-random sampling.
- Why is Sampling Important for Researchers?
Collect Richer Data
The importance of knowing where to sample.
- Different Use Cases for Online Sampling
Academic Research
Market research, public polling, user testing.
By Aaron Moss, PhD, Cheskie Rosenzweig, PhD, & Leib Litman, PhD
Online Researcher’s Sampling Guide, Part 1: What Is the Purpose of Sampling in Research?
Every ten years, the U.S. government conducts a census—a count of every person living in the country—as required by the constitution. It’s a massive undertaking.
The Census Bureau sends a letter or a worker to every U.S. household and tries to gather data that will allow each person to be counted. After the data are gathered, they have to be processed, tabulated and reported. The entire operation takes years of planning and billions of dollars, which begs the question: Is there a better way?
As it turns out, there is.
Instead of contacting every person in the population, researchers can answer most questions by sampling people. In fact, sampling is what the Census Bureau does in order to gather detailed information about the population such as the average household income, the level of education people have, and the kind of work people do for a living. But what, exactly, is sampling, and how does it work?
At its core, a research sample is like any other sample: It’s a small piece or part of something that represents a larger whole.
So, just like the sample of glazed salmon you eat at Costco or the double chocolate brownie ice cream you taste at the ice cream shop, behavioral scientists often gather data from a small group (a sample) as a way to understand a larger whole (a population). Even when the population being studied is as large as the U.S.—about 330 million people—researchers often need to sample just a few thousand people in order to understand everyone.
Now, you may be asking yourself how that works. How can researchers accurately understand hundreds of millions of people by gathering data from just a few thousand of them? Your answer comes from Valery Ivanovich Glivenko and Francesco Paolo Cantelli.
Glivenko and Cantelli were mathematicians who studied probability. At some point during the early 1900s, they discovered that several observations randomly drawn from a population will naturally take on the shape of the population distribution. What this means in plain English is that, as long as researchers randomly sample from a population and obtain a sufficiently sized sample, then the sample will contain characteristics that roughly mirror those of the population.
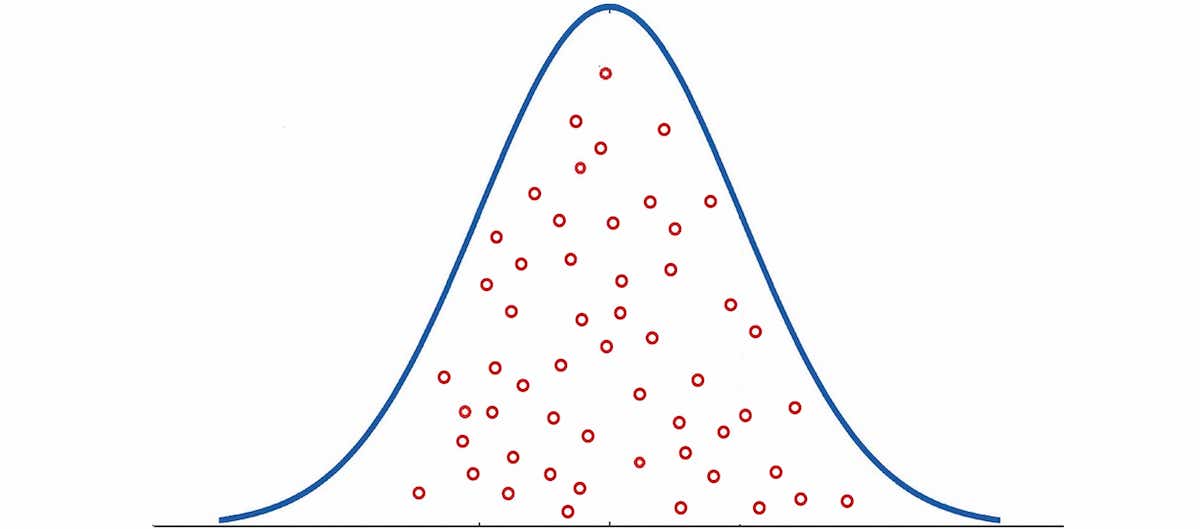
“Ok. That’s great,” you say. But what does it mean to randomly sample people, and how does a researcher do that?
Random sampling occurs when a researcher ensures every member of the population being studied has an equal chance of being selected to participate in the study. Importantly, ‘the population being studied’ is not necessarily all the inhabitants of a country or a region. Instead, a population can refer to people who share a common quality or characteristic. So, everyone who has purchased a Ford in the last five years can be a population and so can registered voters within a state or college students at a city university. A population is the group that researchers want to understand.
In order to understand a population using random sampling, researchers begin by identifying a sampling frame —a list of all the people in the population the researchers want to study. For example, a database of all landline and cell phone numbers in the U.S. is a sampling frame. Once the researcher has a sampling frame, he or she can randomly select people from the list to participate in the study.
However, as you might imagine, it is not always practical or even possible to gather a sampling frame. There is not, for example, a master list of all the people who use the internet, purchase coffee at Dunkin’, have grieved the death of a parent in the last year, or consider themselves fans of the New York Yankees. Nevertheless, there are very good reasons why researchers may want to study people in each of these groups.
When it isn’t possible or practical to gather a random sample, researchers often gather a non-random sample. A non-random sample is one in which every member of the population being studied does not have an equal chance of being selected into the study.
Because non-random samples do not select participants based on probability, it is often difficult to know how well the sample represents the population of interest. Despite this limitation, a wide range of behavioral science studies conducted within academia, industry and government rely on non-random samples. When researchers use non-random samples, it is common to control for any known sources of sampling bias during data collection. By controlling for possible sources of bias, researchers can maximize the usefulness and generalizability of their data.
Why Is Sampling Important for Researchers?
Everyone who has ever worked on a research project knows that resources are limited; time, money and people never come in an unlimited supply. For that reason, most research projects aim to gather data from a sample of people, rather than from the entire population (the census being one of the few exceptions). This is because sampling allows researchers to:
Contacting everyone in a population takes time. And, invariably, some people will not respond to the first effort at contacting them, meaning researchers have to invest more time for follow-up. Random sampling is much faster than surveying everyone in a population, and obtaining a non-random sample is almost always faster than random sampling. Thus, sampling saves researchers lots of time.
The number of people a researcher contacts is directly related to the cost of a study. Sampling saves money by allowing researchers to gather the same answers from a sample that they would receive from the population.
Non-random sampling is significantly cheaper than random sampling, because it lowers the cost associated with finding people and collecting data from them. Because all research is conducted on a budget, saving money is important.
Sometimes, the goal of research is to collect a little bit of data from a lot of people (e.g., an opinion poll). At other times, the goal is to collect a lot of information from just a few people (e.g., a user study or ethnographic interview). Either way, sampling allows researchers to ask participants more questions and to gather richer data than does contacting everyone in a population.
Efficient sampling has a number of benefits for researchers. But just as important as knowing how to sample is knowing where to sample . Some research participants are better suited for the purposes of a project than others. Finding participants that are fit for the purpose of a project is crucial, because it allows researchers to gather high-quality data.
For example, consider an online research project. A team of researchers who decides to conduct a study online has several different sources of participants to choose from. Some sources provide a random sample, and many more provide a non-random sample. When selecting a non-random sample, researchers have several options to consider. Some studies are especially well-suited to an online panel that offers access to millions of different participants worldwide. Other studies, meanwhile, are better suited to a crowdsourced site that generally has fewer participants overall but more flexibility for fostering participant engagement.
To make these options more tangible, let’s look at examples of when researchers might use different kinds of online samples.
Different Use Cases of Online Sampling
Academic researchers gather all kinds of samples online. Some projects require random samples based on probability sampling methods. Most other projects rely on non-random samples. In these non-random samples, researchers may sample a general audience from crowdsourcing websites or selectively target members of specific groups using online panels . The variety of research projects conducted within academia lends itself to many different types of online samples.
Market researchers often want to understand the thoughts, feelings and purchasing decisions of customers or potential customers. For that reason, most online market research is conducted in online panels that provide access to tens of millions of people and allow for complex demographic targeting. For some projects, crowdsourcing sites, such as Amazon Mechanical Turk, allow researchers to get more participant engagement than is typically available in online panels, because they allow researchers to select participants based on experience and to award bonuses.
Public polling is most accurate when it is conducted on a random sample of the population. Hence, lots of public polling is conducted with nationally representative samples. There are, however, an increasing number of opinion polls conducted with non-random samples. When researchers poll people using non-random methods, it is common to adjust for known sources of bias after the data are gathered.
User testing requires people to engage with a website or product. For this reason, user testing is best done on platforms that allow researchers to get participants to engage deeply with their study. Crowdsourcing platforms are ideal for user testing studies, because researchers can often control participant compensation and reward people who are willing to make the effort in a longer study.
Online research is big business. There are hundreds of companies that provide researchers with access to online participants, but only a few facilitate research across different types of online panels or direct you to the right panel for your project. At CloudResearch, we are behavioral and computer science experts with the knowledge to connect you with the right participants for your study and provide expert advice to ensure your project’s successful conclusion. Learn more by contacting us today.
Continue Reading: The Online Researcher’s Guide to Sampling
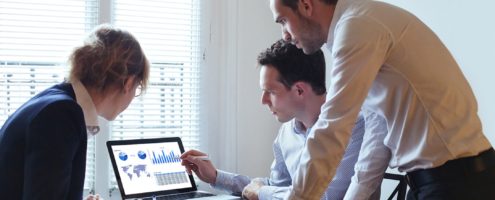
Part 2: How to Reduce Sampling Bias in Research
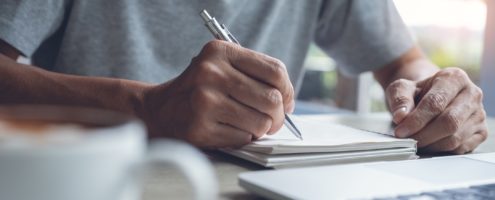
Part 3: How to Build a Sampling Process for Marketing Research
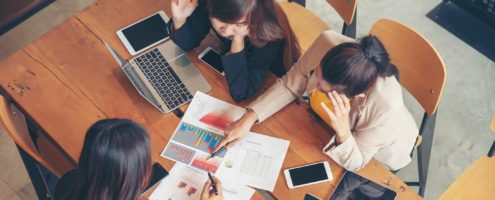
Part 4: Pros and Cons of Different Sampling Methods
Related articles, what is data quality and why is it important.
If you were a researcher studying human behavior 30 years ago, your options for identifying participants for your studies were limited. If you worked at a university, you might be...
How to Identify and Handle Invalid Responses to Online Surveys
As a researcher, you are aware that planning studies, designing materials and collecting data each take a lot of work. So when you get your hands on a new dataset,...
SUBSCRIBE TO RECEIVE UPDATES
2024 grant application form, personal and institutional information.
- Full Name * First Last
- Position/Title *
- Affiliated Academic Institution or Research Organization *
Detailed Research Proposal Questions
- Project Title *
- Research Category * - Antisemitism Islamophobia Both
- Objectives *
- Methodology (including who the targeted participants are) *
- Expected Outcomes *
- Significance of the Study *
Budget and Grant Tier Request
- Requested Grant Tier * - $200 $500 $1000 Applicants requesting larger grants may still be eligible for smaller awards if the full amount requested is not granted.
- Budget Justification *
Research Timeline
- Projected Start Date * MM slash DD slash YYYY Preference will be given to projects that can commence soon, preferably before September 2024.
- Estimated Completion Date * MM slash DD slash YYYY Preference will be given to projects that aim to complete within a year.
- Project Timeline *
- Comments This field is for validation purposes and should be left unchanged.
- Name * First Name Last Name
- I would like to request a demo of the Sentry platform
- Name This field is for validation purposes and should be left unchanged.
- Name * First name Last name
- Name * First Last
- Name * First and Last
- Please select the best time to discuss your project goals/details to claim your free Sentry pilot for the next 60 days or to receive 10% off your first Managed Research study with Sentry.
- Phone This field is for validation purposes and should be left unchanged.
- Email * Enter Email Confirm Email
- Organization
- Job Title *
- Email This field is for validation purposes and should be left unchanged.
- Write My Statistics Paper
- Jamovi Assignment Help
- Business Statistics Assignment Help
- RapidMiner Assignment Help
- Econometric Software Assignment Help
- Econometrics Assignment Help
- Game Theory Assignment Help
- Take My Statistics Exam
- Statistics Assignment Helper
- Statistics Research Paper Assignment Help
- Do My Statistics Assignment
- Pay Someone to Do My Statistics Assignment
- Professional Statistics Assignment Writers
- Need Help with My Statistics Assignment
- Hire Someone to Do My Statistics Assignment
- Statistics Assignment Experts
- Statistics Midterm Assignment Help
- Statistics Capstone Project Help
- Urgent Statistics Assignment Help
- Take My Statistics Quiz
- Professional Statistics Assignment Help
- Statistics Assignment Writers
- Best Statistics Assignment Help
- Affordable Statistics Assignment Help
- Final Year Statistics Project Help
- Statistics Coursework Help
- Reliable Statistics Assignment Help
- Take My Statistics Test
- Custom Statistics Assignment Help
- Doctorate Statistics Assignment Help
- Undergraduate Statistics Assignment Help
- Graduate Statistics Assignment Help
- College Statistics Assignment Help
- ANCOVA Assignment Help
- SPSS Assignment Help
- STATA Assignment Help
- SAS Assignment Help
- Excel Assignment Help
- Statistical Hypothesis Formulation Assignment Help
- LabVIEW Assignment Help
- LISREL Assignment Help
- Minitab Assignment Help
- Analytica Software Assignment Help
- Statistica Assignment Help
- Design Expert Assignment Help
- Orange Assignment Help
- KNIME Assignment Help
- WinBUGS Assignment Help
- Statistix Assignment Help
- Calculus Assignment Help
- JASP Assignment Help
- JMP Assignment Help
- Alteryx Assignment Help
- Statistical Software Assignment Help
- GRETL Assignment Help
- Apache Hadoop Assignment Help
- XLSTAT Assignment Help
- Linear Algebra Assignment Help
- Data Analysis Assignment Help
- Finance Assignment Help
- ANOVA Assignment Help
- Black Scholes Assignment Help
- Experimental Design Assignment Help
- CNN (Convolutional Neural Network) Assignment Help
- Statistical Tests and Measures Assignment Help
- Multiple Linear Regression Assignment Help
- Correlation Analysis Assignment Help
- Data Classification Assignment Help
- Decision Making Assignment Help
- 2D Geometry Assignment Help
- Distribution Theory Assignment Help
- Decision Theory Assignment Help
- Data Manipulation Assignment Help
- Binomial Distributions Assignment Help
- Linear Regression Assignment Help
- Statistical Inference Assignment Help
- Structural Equation Modeling (SEM) Assignment Help
- Numerical Methods Assignment Help
- Markov Processes Assignment Help
- Non-Parametric Tests Assignment Help
- Multivariate Statistics Assignment Help
- Data Flow Diagram Assignment Help
- Bivariate Normal Distribution Assignment Help
- Matrix Operations Assignment Help
- CFA Assignment Help
- Mathematical Methods Assignment Help
- Probability Assignment Help
- Kalman Filter Assignment Help
- Kruskal-Wallis Test Assignment Help
- Stochastic Processes Assignment Help
- Chi-Square Test Assignment Help
- Six Sigma Assignment Help
- Hypothesis Testing Assignment Help
- GAUSS Assignment Help
- GARCH Models Assignment Help
- Simple Random Sampling Assignment Help
- GEE Models Assignment Help
- Principal Component Analysis Assignment Help
- Multicollinearity Assignment Help
- Linear Discriminant Assignment Help
- Logistic Regression Assignment Help
- Survival Analysis Assignment Help
- Nonparametric Statistics Assignment Help
- Poisson Process Assignment Help
- Cluster Analysis Assignment Help
- ARIMA Models Assignment Help
- Measures of central tendency
- Time Series Analysis Assignment Help
- Factor Analysis Assignment Help
- Regression Analysis Assignment Help
- Survey Methodology Assignment Help
- Statistical Modeling Assignment Help
- Survey Design Assignment Help
- Linear Programming Assignment Help
- Confidence Interval Assignment Help
- Quantitative Methods Assignment Help
Core Concepts in Sampling and Estimation for Statistical Analysis
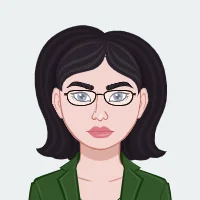
Submit Your Statistical Analysis Assignment
Get FREE Quote
Claim Your Offer
Unlock a fantastic deal at www.statisticsassignmenthelp.com with our latest offer. Get an incredible 20% off on your second statistics assignment, ensuring quality help at a cheap price. Our expert team is ready to assist you, making your academic journey smoother and more affordable. Don't miss out on this opportunity to enhance your skills and save on your studies. Take advantage of our offer now and secure top-notch help for your statistics assignments.

- Statistical Analysis: Sampling Techniques and Estimation Explained
Sampling Methods: With Replacement vs. Without Replacement
Sample mean vs. population mean: understanding the difference, parameter of interest: what it is and why it matters, sampling variability: definition and example, point estimate: what it is and its importance, the sampling frame: a critical component of sampling.
In the field of statistics, the concepts of sampling and estimation are foundational for conducting research and making inferences about populations. Whether you're working on a simple survey or a complex study, understanding these concepts is crucial for ensuring the accuracy and reliability of your findings. This knowledge is essential for students who aim to solve their statistics assignment effectively. This blog will delve into several key ideas, including sampling methods, the distinction between sample and population means, parameters of interest, sampling variability, point estimates, and the importance of a well-defined sampling frame. By grasping these concepts, you'll be better equipped to tackle assignments and real-world problems that require statistical analysis .
Sampling is a process by which a subset of individuals or observations is selected from a larger population for the purpose of making inferences about the population. There are two primary methods of sampling: with replacement and without replacement.
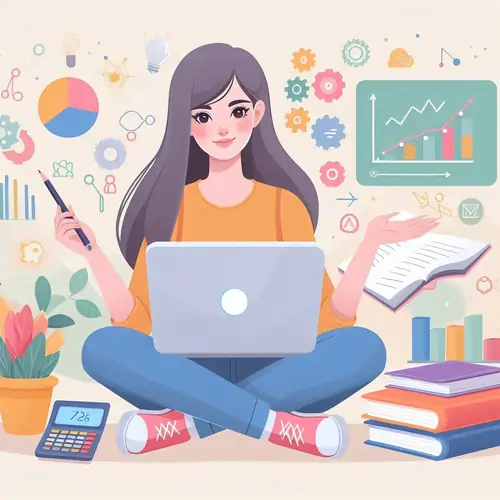
- Sampling With Replacement: In this method, after an individual or observation is selected from the population, it is returned to the population before the next selection is made. This means that the same individual or observation can be selected more than once. Sampling with replacement is often used in simulations and theoretical models because it simplifies the mathematics and allows for independent selections. However, it may not always reflect real-world scenarios where repeated selection of the same individual is unlikely.
- Sampling Without Replacement: In contrast, when sampling without replacement, once an individual or observation is selected, it is not returned to the population. This method ensures that each individual or observation can only be selected once. Sampling without replacement is more commonly used in practical research because it mirrors real-world situations where, for example, a person cannot be surveyed twice in the same study. This method tends to reduce sampling variability and often provides more precise estimates of population parameters.
Understanding the differences between these two methods is essential for choosing the appropriate sampling strategy for your research and ensuring that your results are both accurate and applicable to the population of interest.
One of the fundamental distinctions in statistics is between the sample mean and the population mean. These two measures are used to summarize data, but they serve different purposes and have different implications.
- Population Mean: The population mean (often denoted as μ) is the average of all the values in a population. It is a fixed value that represents the true central tendency of the entire population. Since the population mean includes every member of the population, it is often unknown and can be challenging to calculate, especially when dealing with large populations.
- Sample Mean: The sample mean (denoted as xˉ\bar{x}xˉ) is the average of the values in a sample, which is a subset of the population. The sample mean is used as an estimate of the population mean. Because it is based on a subset of the population, the sample mean can vary from one sample to another. This variability is known as sampling variability, and it highlights the importance of selecting a representative sample to ensure that the sample mean is a good approximation of the population mean.
The key difference between the sample mean and the population mean lies in their variability. While the population mean is a constant value, the sample mean can fluctuate depending on the sample chosen. Understanding this difference is crucial for interpreting statistical results and making inferences about the broader population.
In statistical research, the parameter of interest is a specific numerical characteristic of a population that a researcher aims to estimate or test. This parameter could be the population mean, proportion, variance, or any other measurable attribute that defines the population's characteristics.
Identifying the parameter of interest is a critical step in any statistical study because it determines the focus of the analysis. For example, in a study aiming to understand the average height of a population, the population mean height is the parameter of interest. In another study focusing on the proportion of individuals with a particular trait, the population proportion is the parameter of interest.
The parameter of interest guides the choice of statistical methods, sampling strategies, and data analysis techniques. It also influences the formulation of hypotheses and the interpretation of results. By clearly defining the parameter of interest, researchers can ensure that their study is well-structured and that the conclusions drawn are relevant to the research question.
Sampling variability refers to the natural fluctuation in sample statistics that occurs when different samples are drawn from the same population. This variability is an inherent aspect of the sampling process and is a key consideration when making inferences about a population based on sample data.
For example, imagine you are estimating the average income of residents in a city. If you take multiple random samples from the city's population, the sample means you calculate for each sample will likely differ slightly. This variation in sample means is due to sampling variability. The extent of sampling variability depends on factors such as the sample size, the sampling method, and the inherent variability within the population.
Sampling variability is important because it affects the precision of your estimates. A high level of sampling variability can lead to less precise estimates, making it more challenging to draw accurate conclusions about the population. To mitigate sampling variability, researchers often increase the sample size or use more sophisticated sampling techniques. Understanding and accounting for sampling variability is crucial for interpreting statistical results and assessing the reliability of your conclusions.
A point estimate is a single value derived from sample data that serves as a best guess for a population parameter. For instance, the sample mean is a point estimate of the population means, and the sample proportion is a point estimate of the population proportion.
Point estimates are widely used in statistics because they provide a straightforward and concise summary of what the data suggests about the population. However, point estimates are subject to sampling variability, meaning that different samples from the same population may yield different point estimates.
While point estimates are useful, they do not provide information about the precision of the estimate or the uncertainty associated with it. For this reason, point estimates are often accompanied by confidence intervals, which offer a range of values within which the true population parameter is likely to fall. By combining point estimates with confidence intervals, researchers can present their findings more accurately and provide a clearer picture of the uncertainty inherent in their estimates.
A sampling frame is a list or database that includes all the elements or individuals from which a sample will be drawn. The sampling frame is a critical component of the sampling process because it defines the population from which the sample is selected and ensures that the sample is representative of the entire population.
For example, if you are conducting a survey of university students, your sampling frame might be a list of all enrolled students. The quality of the sampling frame directly affects the accuracy of your sample. If the sampling frame is incomplete or biased (e.g., it excludes certain groups of students), the sample may not accurately reflect the population, leading to biased or misleading results.
Choosing an appropriate and comprehensive sampling frame is essential for minimizing sampling bias and ensuring that the sample is truly representative of the population. Researchers must carefully consider the sampling frame during the design phase of a study to avoid errors that could compromise the validity of their findings.
Understanding the concepts of sampling and estimation is vital for conducting reliable and valid statistical research. Whether you're dealing with sampling methods, distinguishing between sample and population means, identifying parameters of interest, or understanding sampling variability, these concepts form the backbone of statistical analysis.
By mastering these topics, you'll be better equipped to tackle assignments and real-world problems that require statistical reasoning. Remember that the choice of sampling method, the clarity in defining your parameter of interest, and the careful selection of a sampling frame are all crucial steps in ensuring the accuracy and reliability of your statistical conclusions.
As you apply these concepts to your assignments, consider how each element—whether it's a point estimate or the variability introduced by sampling—affects your overall findings. By doing so, you'll not only improve your statistical skills but also enhance the quality of your research, leading to more meaningful and trustworthy results.
You Might Also Like
Our popular services.
- Search Search Please fill out this field.
- Stratified Random Sampling
- Disadvantages
- Stratified Random Sampling FAQs
The Bottom Line
- Corporate Finance
- Financial Analysis
Stratified Random Sampling: Advantages and Disadvantages
Pros and Cons of Stratified Random Sampling
:max_bytes(150000):strip_icc():format(webp)/me_jpeg__chris_murphy-5bfc262746e0fb0051bcea2f.jpg)
Getty Images / sutiwat jutiamornloes
When researching a group of people, it is often impossible to measure every individual data point. However, statistical methods allow for inferences about a population by analyzing the results of a smaller sample extracted from that population.
Stratified random sampling is one way of doing this. This technique enables researchers to obtain a sample population that best represents the entire population being studied by making sure that each subgroup of interest is represented. However, it is not without its disadvantages.
Key Takeaways
- Stratified random sampling allows researchers to obtain a sample population that best represents the entire population being studied by dividing it into subgroups called strata.
- This method of statistical sampling, however, cannot be used in every study design or with every data set.
- Stratified random sampling differs from simple random sampling, which involves the random selection of data from an entire population so each possible sample is equally likely to occur.
Stratified Random Sampling: An Overview
Stratified random sampling involves dividing a population into subpopulations and then applying random sampling methods to each subpopulation to form a test group.
Stratified random sampling is different from simple random sampling , which involves the random selection of data from the entire population so that each possible sample is equally likely to occur. In contrast, stratified random sampling divides the population into smaller groups, or strata, based on shared characteristics. A random sample is then taken from each stratum in direct proportion to the size of the stratum compared to the population.
Stratified Random Sampling Example
Researchers are performing a study designed to evaluate the political leanings of economics students at a major university. The researchers want to ensure the random sample best approximates the student population, including gender, undergraduates, and graduate students. The total population in the study is 1,000 students and, from there, subgroups are created as shown below.
Total population = 1,000
Researchers would assign every economics student at the university to one of four subpopulations: male undergraduate, female undergraduate, male graduate, and female graduate. Researchers would next count how many students from each subgroup make up the total population of 1,000 students. From there, researchers calculate each subgroup's percentage representation of the total population.
- Male undergraduates = 450 students or 45% of the population
- Female undergraduates = 200 students or 20%
- Male graduate students = 200 students or 20%
- Female graduate students = 150 students or 15%
Random sampling of each subpopulation is done based on its representation within the population as a whole. Since male undergraduates are 45% of the population, 45 male undergraduates are randomly chosen out of that subgroup. Moreover, because male graduates make up only 20% of the population, 20 are selected for the sample, and so on.
This method has several conditions, including the need to classify every member of the population into a subgroup, so it can't be used in every study.
Advantages of Stratified Random Sampling
Stratified random sampling has advantages when compared to simple random sampling.
It reflects the population being studied because researchers are stratifying the entire population before applying random sampling methods. In short, it ensures each subgroup within the population receives proper representation within the sample. As a result, stratified random sampling provides better coverage of the population since the researchers have control over the subgroups to ensure all of them are represented in the sampling.
With simple random sampling , there isn't any guarantee that any particular subgroup or type of person is chosen.
In our earlier example of the university students, using simple random sampling to procure a sample of 100 from the population might result in the selection of only 25 male undergraduates or only 25% of the total population. Also, 35 female graduate students might be selected (35% of the population) resulting in under-representation of male undergraduates and over-representation of female graduate students. Any errors in the representation of the population have the potential to diminish the accuracy of the study.
Disadvantages of Stratified Random Sampling
Stratified random sampling also presents researchers with a disadvantage.
Unfortunately, this method of research cannot be used in every study. The method's disadvantage is that several conditions must be met for it to be used properly. Researchers must identify every member of a population being studied and classify each of them into one, and only one, subpopulation.
As a result, stratified random sampling is disadvantageous when researchers can't confidently classify every member of the population into a subgroup. Also, finding an exhaustive and definitive list of an entire population can be challenging.
Overlapping can be an issue if there are subjects that fall into multiple subgroups. When simple random sampling is performed, those who are in multiple subgroups are more likely to be chosen. The result could be a misrepresentation or inaccurate reflection of the population.
In our example, undergraduate, graduate, male, and female are clearly defined groups. In other situations, however, it might be far more difficult. Imagine incorporating characteristics such as race, ethnicity, or religion. The sorting process becomes more difficult, rendering stratified random sampling an ineffective and less-than-ideal method.
What Are the Five Main Types of Sampling?
There are various sampling techniques. The main ones are simple random sampling, systematic sampling, stratified sampling, and cluster sampling.
Why Is It Called Stratified Random Sampling?
It is called stratified random sampling because the population being analyzed is arranged into subgroups called strata. The data is stratified.
What Is the Difference Between Simple Random Sampling and Stratified Sampling?
Simple random sampling randomly selects data from the population assuming all areas will be covered. Stratified sampling, on the other hand, goes a step further by dividing the population into subpopulations based on shared characteristics to ensure each group within the population is properly represented. In theory, stratified sampling offers a fairer representation of the population. However, this approach is also more time-consuming and not always easy to apply.
When Is it Better to Use Simple Random Sampling Over Stratified Sampling?
Simple random sampling would be used if there is very little information available about the population or if the population is too diverse to divide into subsets or similar and difficult to distinguish. Sometimes grouping people into categories can be helpful. Other times, it is not necessary.
Stratified random sampling, a method of sampling that involves dividing a population into smaller subgroups known as strata, is sometimes preferred over simple random sampling because it ensures each subgroup within the population receives proper representation. However, making this extra effort is not always necessary and guaranteed to make the research more reliable and reflective of the population. Stratified random sampling is not always easy or possible to apply either.
:max_bytes(150000):strip_icc():format(webp)/GettyImages-1351963017-ac636379d60f433aa6a6181b0f7c8540.jpg)
- Terms of Service
- Editorial Policy
- Privacy Policy

An official website of the United States government
The .gov means it’s official. Federal government websites often end in .gov or .mil. Before sharing sensitive information, make sure you’re on a federal government site.
The site is secure. The https:// ensures that you are connecting to the official website and that any information you provide is encrypted and transmitted securely.
- Publications
- Account settings
Preview improvements coming to the PMC website in October 2024. Learn More or Try it out now .
- Advanced Search
- Journal List
- An Bras Dermatol
- v.91(3); May-Jun 2016
Sampling: how to select participants in my research study? *
Jeovany martínez-mesa.
1 Faculdade Meridional (IMED) - Passo Fundo (RS), Brazil.
David Alejandro González-Chica
2 University of Adelaide - Adelaide, Australia.
Rodrigo Pereira Duquia
3 Universidade Federal de Ciências da Saúde de Porto Alegre (UFCSPA) - Porto Alegre (RS), Brazil.
Renan Rangel Bonamigo
João luiz bastos.
4 Universidade Federal de Santa Catarina (UFSC) - Florianópolis (RS), Brazil.
In this paper, the basic elements related to the selection of participants for a health research are discussed. Sample representativeness, sample frame, types of sampling, as well as the impact that non-respondents may have on results of a study are described. The whole discussion is supported by practical examples to facilitate the reader's understanding.
To introduce readers to issues related to sampling.
INTRODUCTION
The essential topics related to the selection of participants for a health research are: 1) whether to work with samples or include the whole reference population in the study (census); 2) the sample basis; 3) the sampling process and 4) the potential effects nonrespondents might have on study results. We will refer to each of these aspects with theoretical and practical examples for better understanding in the sections that follow.
TO SAMPLE OR NOT TO SAMPLE
In a previous paper, we discussed the necessary parameters on which to estimate the sample size. 1 We define sample as a finite part or subset of participants drawn from the target population. In turn, the target population corresponds to the entire set of subjects whose characteristics are of interest to the research team. Based on results obtained from a sample, researchers may draw their conclusions about the target population with a certain level of confidence, following a process called statistical inference. When the sample contains fewer individuals than the minimum necessary, but the representativeness is preserved, statistical inference may be compromised in terms of precision (prevalence studies) and/or statistical power to detect the associations of interest. 1 On the other hand, samples without representativeness may not be a reliable source to draw conclusions about the reference population (i.e., statistical inference is not deemed possible), even if the sample size reaches the required number of participants. Lack of representativeness can occur as a result of flawed selection procedures (sampling bias) or when the probability of refusal/non-participation in the study is related to the object of research (nonresponse bias). 1 , 2
Although most studies are performed using samples, whether or not they represent any target population, census-based estimates should be preferred whenever possible. 3 , 4 For instance, if all cases of melanoma are available on a national or regional database, and information on the potential risk factors are also available, it would be preferable to conduct a census instead of investigating a sample.
However, there are several theoretical and practical reasons that prevent us from carrying out census-based surveys, including:
- Ethical issues: it is unethical to include a greater number of individuals than that effectively required;
- Budgetary limitations: the high costs of a census survey often limits its use as a strategy to select participants for a study;
- Logistics: censuses often impose great challenges in terms of required staff, equipment, etc. to conduct the study;
- Time restrictions: the amount of time needed to plan and conduct a census-based survey may be excessive; and,
- Unknown target population size: if the study objective is to investigate the presence of premalignant skin lesions in illicit drugs users, lack of information on all existing users makes it impossible to conduct a census-based study.
All these reasons explain why samples are more frequently used. However, researchers must be aware that sample results can be affected by the random error (or sampling error). 3 To exemplify this concept, we will consider a research study aiming to estimate the prevalence of premalignant skin lesions (outcome) among individuals >18 years residing in a specific city (target population). The city has a total population of 4,000 adults, but the investigator decided to collect data on a representative sample of 400 participants, detecting an 8% prevalence of premalignant skin lesions. A week later, the researcher selects another sample of 400 participants from the same target population to confirm the results, but this time observes a 12% prevalence of premalignant skin lesions. Based on these findings, is it possible to assume that the prevalence of lesions increased from the first to the second week? The answer is probably not. Each time we select a new sample, it is very likely to obtain a different result. These fluctuations are attributed to the "random error." They occur because individuals composing different samples are not the same, even though they were selected from the same target population. Therefore, the parameters of interest may vary randomly from one sample to another. Despite this fluctuation, if it were possible to obtain 100 different samples of the same population, approximately 95 of them would provide prevalence estimates very close to the real estimate in the target population - the value that we would observe if we investigated all the 4,000 adults residing in the city. Thus, during the sample size estimation the investigator must specify in advance the highest or maximum acceptable random error value in the study. Most population-based studies use a random error ranging from 2 to 5 percentage points. Nevertheless, the researcher should be aware that the smaller the random error considered in the study, the larger the required sample size. 1
SAMPLE FRAME
The sample frame is the group of individuals that can be selected from the target population given the sampling process used in the study. For example, to identify cases of cutaneous melanoma the researcher may consider to utilize as sample frame the national cancer registry system or the anatomopathological records of skin biopsies. Given that the sample may represent only a portion of the target population, the researcher needs to examine carefully whether the selected sample frame fits the study objectives or hypotheses, and especially if there are strategies to overcome the sample frame limitations (see Chart 1 for examples and possible limitations).
Examples of sample frames and potential limitations as regards representativeness
Sample frames | Limitations |
---|---|
Population census | • If the census was not conducted in recent years, areas with high migration might be outdated |
• Homeless or itinerant people cannot be represented | |
Hospital or Health Services records | • Usually include only data of affected people (this is a limitation, depending on the study objectives) |
• Depending on the service, data may be incomplete and/or outdated | |
• If the lists are from public units, results may differ from those who seek private services | |
School lists | • School lists are currently available only in the public sector |
• Children/ teenagers not attending school will not be represented | |
• Lists are quickly outdated | |
• There will be problems in areas with high percentage of school absenteeism | |
List of phone numbers | • Several population groups are not represented: individuals with no phone line at home (low-income families, young people who use only cell phones), those who spend less time at home, etc. |
Mailing lists | • Individuals with multiple email addresses, which increase the chance of selection compared to individuals with only one address |
• Individuals without an email address may be different from those who have it, according to age, education, etc. |
Sampling can be defined as the process through which individuals or sampling units are selected from the sample frame. The sampling strategy needs to be specified in advance, given that the sampling method may affect the sample size estimation. 1 , 5 Without a rigorous sampling plan the estimates derived from the study may be biased (selection bias). 3
TYPES OF SAMPLING
In figure 1 , we depict a summary of the main sampling types. There are two major sampling types: probabilistic and nonprobabilistic.

Sampling types used in scientific studies
NONPROBABILISTIC SAMPLING
In the context of nonprobabilistic sampling, the likelihood of selecting some individuals from the target population is null. This type of sampling does not render a representative sample; therefore, the observed results are usually not generalizable to the target population. Still, unrepresentative samples may be useful for some specific research objectives, and may help answer particular research questions, as well as contribute to the generation of new hypotheses. 4 The different types of nonprobabilistic sampling are detailed below.
Convenience sampling : the participants are consecutively selected in order of apperance according to their convenient accessibility (also known as consecutive sampling). The sampling process comes to an end when the total amount of participants (sample saturation) and/or the time limit (time saturation) are reached. Randomized clinical trials are usually based on convenience sampling. After sampling, participants are usually randomly allocated to the intervention or control group (randomization). 3 Although randomization is a probabilistic process to obtain two comparable groups (treatment and control), the samples used in these studies are generally not representative of the target population.
Purposive sampling: this is used when a diverse sample is necessary or the opinion of experts in a particular field is the topic of interest. This technique was used in the study by Roubille et al, in which recommendations for the treatment of comorbidities in patients with rheumatoid arthritis, psoriasis, and psoriatic arthritis were made based on the opinion of a group of experts. 6
Quota sampling: according to this sampling technique, the population is first classified by characteristics such as gender, age, etc. Subsequently, sampling units are selected to complete each quota. For example, in the study by Larkin et al., the combination of vemurafenib and cobimetinib versus placebo was tested in patients with locally-advanced melanoma, stage IIIC or IV, with BRAF mutation. 7 The study recruited 495 patients from 135 health centers located in several countries. In this type of study, each center has a "quota" of patients.
"Snowball" sampling : in this case, the researcher selects an initial group of individuals. Then, these participants indicate other potential members with similar characteristics to take part in the study. This is frequently used in studies investigating special populations, for example, those including illicit drugs users, as was the case of the study by Gonçalves et al, which assessed 27 users of cocaine and crack in combination with marijuana. 8
PROBABILISTIC SAMPLING
In the context of probabilistic sampling, all units of the target population have a nonzero probability to take part in the study. If all participants are equally likely to be selected in the study, equiprobabilistic sampling is being used, and the odds of being selected by the research team may be expressed by the formula: P=1/N, where P equals the probability of taking part in the study and N corresponds to the size of the target population. The main types of probabilistic sampling are described below.
Simple random sampling: in this case, we have a full list of sample units or participants (sample basis), and we randomly select individuals using a table of random numbers. An example is the study by Pimenta et al, in which the authors obtained a listing from the Health Department of all elderly enrolled in the Family Health Strategy and, by simple random sampling, selected a sample of 449 participants. 9
Systematic random sampling: in this case, participants are selected from fixed intervals previously defined from a ranked list of participants. For example, in the study of Kelbore et al, children who were assisted at the Pediatric Dermatology Service were selected to evaluate factors associated with atopic dermatitis, selecting always the second child by consulting order. 10
Stratified sampling: in this type of sampling, the target population is first divided into separate strata. Then, samples are selected within each stratum, either through simple or systematic sampling. The total number of individuals to be selected in each stratum can be fixed or proportional to the size of each stratum. Each individual may be equally likely to be selected to participate in the study. However, the fixed method usually involves the use of sampling weights in the statistical analysis (inverse of the probability of selection or 1/P). An example is the study conducted in South Australia to investigate factors associated with vitamin D deficiency in preschool children. Using the national census as the sample frame, households were randomly selected in each stratum and all children in the age group of interest identified in the selected houses were investigated. 11
Cluster sampling: in this type of probabilistic sampling, groups such as health facilities, schools, etc., are sampled. In the above-mentioned study, the selection of households is an example of cluster sampling. 11
Complex or multi-stage sampling: This probabilistic sampling method combines different strategies in the selection of the sample units. An example is the study of Duquia et al. to assess the prevalence and factors associated with the use of sunscreen in adults. The sampling process included two stages. 12 Using the 2000 Brazilian demographic census as sampling frame, all 404 census tracts from Pelotas (Southern Brazil) were listed in ascending order of family income. A sample of 120 tracts were systematically selected (first sampling stage units). In the second stage, 12 households in each of these census tract (second sampling stage units) were systematically drawn. All adult residents in these households were included in the study (third sampling stage units). All these stages have to be considered in the statistical analysis to provide correct estimates.
NONRESPONDENTS
Frequently, sample sizes are increased by 10% to compensate for potential nonresponses (refusals/losses). 1 Let us imagine that in a study to assess the prevalence of premalignant skin lesions there is a higher percentage of nonrespondents among men (10%) than among women (1%). If the highest percentage of nonresponse occurs because these men are not at home during the scheduled visits, and these participants are more likely to be exposed to the sun, the number of skin lesions will be underestimated. For this reason, it is strongly recommended to collect and describe some basic characteristics of nonrespondents (sex, age, etc.) so they can be compared to the respondents to evaluate whether the results may have been affected by this systematic error.
Often, in study protocols, refusal to participate or sign the informed consent is considered an "exclusion criteria". However, this is not correct, as these individuals are eligible for the study and need to be reported as "nonrespondents".
SAMPLING METHOD ACCORDING TO THE TYPE OF STUDY
In general, clinical trials aim to obtain a homogeneous sample which is not necessarily representative of any target population. Clinical trials often recruit those participants who are most likely to benefit from the intervention. 3 Thus, the more strict criteria for inclusion and exclusion of subjects in clinical trials often make it difficult to locate participants: after verification of the eligibility criteria, just one out of ten possible candidates will enter the study. Therefore, clinical trials usually show limitations to generalize the results to the entire population of patients with the disease, but only to those with similar characteristics to the sample included in the study. These peculiarities in clinical trials justify the necessity of conducting a multicenter and/or global studiesto accelerate the recruitment rate and to reach, in a shorter time, the number of patients required for the study. 13
In turn, in observational studies to build a solid sampling plan is important because of the great heterogeneity usually observed in the target population. Therefore, this heterogeneity has to be also reflected in the sample. A cross-sectional population-based study aiming to assess disease estimates or identify risk factors often uses complex probabilistic sampling, because the sample representativeness is crucial. However, in a case-control study, we face the challenge of selecting two different samples for the same study. One sample is formed by the cases, which are identified based on the diagnosis of the disease of interest. The other consists of controls, which need to be representative of the population that originated the cases. Improper selection of control individuals may introduce selection bias in the results. Thus, the concern with representativeness in this type of study is established based on the relationship between cases and controls (comparability).
In cohort studies, individuals are recruited based on the exposure (exposed and unexposed subjects), and they are followed over time to evaluate the occurrence of the outcome of interest. At baseline, the sample can be selected from a representative sample (population-based cohort studies) or a non-representative sample. However, in the successive follow-ups of the cohort member, study participants must be a representative sample of those included in the baseline. 14 , 15 In this type of study, losses over time may cause follow-up bias.
Researchers need to decide during the planning stage of the study if they will work with the entire target population or a sample. Working with a sample involves different steps, including sample size estimation, identification of the sample frame, and selection of the sampling method to be adopted.
Financial Support: None.
* Study performed at Faculdade Meridional - Escola de Medicina (IMED) - Passo Fundo (RS), Brazil.
This paper is in the following e-collection/theme issue:
Published on 8.4.2024 in Vol 8 (2024)
Mobile Technology Use in Clinical Research Examining Challenges and Implications for Health Promotion in South Africa: Mixed Methods Study
Authors of this article:

Original Paper
- Khuthala Mabetha 1 , PhD ;
- Larske M Soepnel 1, 2 , PhD ;
- Gugulethu Mabena 1 , MA ;
- Molebogeng Motlhatlhedi 1 , BSc ;
- Lukhanyo Nyati 1 , PhD ;
- Shane A Norris 1, 3 , PhD ;
- Catherine E Draper 1 , PhD
1 South African Medical Research Council/Wits Developmental Pathways for Health Research Unit, Department of Paediatrics, Faculty of Health Sciences, School of Clinical Medicine, University of the Witwatersrand, Johannesburg, South Africa
2 Julius Center for Health Sciences and Primary Care, University Medical Center, Utrecht University, Utrecht, Netherlands
3 School of Human Development and Health, University of Southampton, Southampton, United Kingdom
Corresponding Author:
Khuthala Mabetha, PhD
South African Medical Research Council/Wits Developmental Pathways for Health Research Unit, Department of Paediatrics, Faculty of Health Sciences, School of Clinical Medicine
University of the Witwatersrand
7 York Rd, Parktown
Johannesburg, 2193
South Africa
Phone: 27 011 717 2382
Email: [email protected]
Background: The use of mobile technologies in fostering health promotion and healthy behaviors is becoming an increasingly common phenomenon in global health programs. Although mobile technologies have been effective in health promotion initiatives and follow-up research in higher-income countries and concerns have been raised within clinical practice and research in low- and middle-income settings, there is a lack of literature that has qualitatively explored the challenges that participants experience in terms of being contactable through mobile technologies.
Objective: This study aims to explore the challenges that participants experience in terms of being contactable through mobile technologies in a trial conducted in Soweto, South Africa.
Methods: A convergent parallel mixed methods research design was used. In the quantitative phase, 363 young women in the age cohorts 18 to 28 years were contacted telephonically between August 2019 and January 2022 to have a session delivered to them or to be booked for a session. Call attempts initiated by the study team were restricted to only 1 call attempt, and participants who were reached at the first call attempt were classified as contactable (189/363, 52.1%), whereas those whom the study team failed to contact were classified as hard to reach (174/363, 47.9%). Two outcomes of interest in the quantitative phase were “contactability of the participants” and “participants’ mobile number changes,” and these outcomes were analyzed at a univariate and bivariate level using descriptive statistics and a 2-way contingency table. In the qualitative phase, a subsample of young women (20 who were part of the trial for ≥12 months) participated in in-depth interviews and were recruited using a convenience sampling method. A reflexive thematic analysis approach was used to analyze the data using MAXQDA software (version 20; VERBI GmbH).
Results: Of the 363 trial participants, 174 (47.9%) were hard to reach telephonically, whereas approximately 189 (52.1%) were easy to reach telephonically. Most participants (133/243, 54.7%) who were contactable did not change their mobile number. The highest percentage of mobile number changes was observed among participants who were hard to reach, with three-quarters of the participants (12/16, 75%) being reported to have changed their mobile number ≥2 times. Eight themes were generated following the analysis of the transcripts, which provided an in-depth account of the reasons why some participants were hard to reach. These included mobile technical issues, coverage issues, lack of ownership of personal cell phones, and unregistered number.
Conclusions: Remote data collection remains an important tool in public health research. It could, thus, serve as a hugely beneficial mechanism in connecting with participants while actively leveraging the established relationships with participants or community-based organizations to deliver health promotion and practice.
Introduction
The use of mobile technologies in engaging people and communities to choose healthy behaviors and to improve their health is becoming an increasingly common phenomenon in global health programs [ 1 - 4 ]. Mobile technologies have increasingly become a common mode of delivering telemedicine in sub-Saharan Africa and other low- and middle-income countries (LMICs) given improvements in coverage [ 5 ], with the prospects of improving access to information pertaining to health care services in areas where health care systems lag in meeting minimum health standards [ 6 , 7 ]. Mobile phone technology use in clinical studies is a potential avenue for both the follow-up of participants in research [ 8 - 10 ] and the provision of targeted and tailored messaging to facilitate behavior change support. For instance, patients now progressively receive reminders regarding clinic appointments [ 11 ], medication uptake [ 12 ], health promotion [ 13 , 14 ], medical treatment or diagnosis [ 15 ], and accessing information on disease outbreaks and other health-related data [ 16 ] through SMS text messaging systems and telephone calls; all these methods have collectively contributed to better health [ 1 , 17 ]. These digital interventions are reinforced by the high level of mobile phone penetration in many LMICs.
Mobile technologies typically exist in the form of internet-enabled devices such as smartphones, tablets, and watches [ 18 ]. Mobile phones constitute the most commonly used mobile technologies for cellular communication in LMICs [ 19 , 20 ] and are used as a cellular communication system for making and receiving phone calls, video calls, text and instant messaging, surfing the internet, GPS navigation, and playing games [ 21 ]. For the purposes of this study, the focus was particularly restricted to mobile phones, with the form of cellular communication being restricted only to phone calls.
Research conducted in the sub-Saharan African region has shown that individuals who participate in research studies are intermittently reached through the phone, which is the most commonly used method in the region [ 22 ]. Among African countries, South Africa has the third highest number of people who are active mobile phone users, providing a robust platform for telemedicine prospects [ 22 ]. For example, 97% of households in South Africa have access to a mobile phone [ 23 ]. According to the Independent Communications Authority of South Africa 2019 report on the state of information and communications technology, an estimated 82% of the country’s total population have a smartphone [ 24 ], with 88% of the population reported to have prepaid mobile cellular subscriptions and 12% of the population reported to have contract subscriptions [ 24 ]. Although mobile technologies have shown some success in their effectiveness in health promotion initiatives and in improving health knowledge, the use of mobile phones in delivering these interventions within clinical practice and research raises some concerns [ 25 ]. For example, although a large proportion of people own mobile phones, there are low retention rates in clinical research, and this has been largely attributed to challenges of participant reachability and accessibility by phone [ 22 ]. For instance, in a clinical trial conducted in Togo, out of an approximate proportion of 13,726 respondents who had provided their mobile number, 80% could not be reached on that number [ 26 ].
In addition, research conducted in Côte d’Ivoire among patients who were receiving antiretroviral treatment showed that out of the 7000 patients who were traced telephonically after initially actively participating in the research but were lost at the point of follow-up in the study, only 40% of the patients could be reached on the telephone number that was provided [ 27 ]. A randomized controlled trial conducted in South Africa among 400 HIV-positive patients found that only 60.3% of the patients could be reached telephonically after being contacted a maximum of 3 times via telephone on different days and at different times for every phone number that the participant had provided [ 22 ]. In addition, another South African study indicated that reachability by phone is a common challenge in the country. This is caused, for instance, by theft and loss of phones, which results in connectivity loss with respondents and loss to follow-up [ 28 ]. Although several scholars have investigated the effectiveness of mobile phones in follow-up studies [ 1 , 6 - 8 , 13 , 24 , 27 - 29 ], there is a dearth of literature in South Africa that has qualitatively explored the challenges that participants experience in terms of being contactable through mobile technologies. Gaining insight into these challenges will help in developing targeted strategies to facilitate ways for research staff and researchers to remain in contact with participants over time and for follow-up research. The results could also potentially inform the use of mobile technologies for reaching patients in a clinical setting.
The trial is being conducted in Soweto, South Africa. It is the Bukhali preconception health trial, which is nested in the Healthy Life Trajectories Initiative [ 30 ]. The aim of the trial is to optimize the physical and mental health of young women, fostering positive dynamic changes in their health for themselves, and where relevant, for future cohorts. In this trial, young women received intervention materials and resources delivered by community health workers, referred to as “Health Helpers.” These materials include health literacy materials, multimicronutrient supplements, health screening and referral conducted in person, nutritional risk and support, and monthly sessions that help with modifying or transforming health behaviors. The monthly sessions were conducted telephonically by the Health Helpers with on-site visits scheduled every 6 months [ 30 - 32 ]. However, there have been challenges in contacting participants for booking and delivering monthly trial sessions because the participants were unreachable on the contact numbers registered for the study. Therefore, this study aimed to explore the challenges that participants experienced with regard to being contactable through mobile technologies, using both quantitative and qualitative data.
Study Design
A convergent parallel mixed methods research design was applied. It is a research design that involves the collection and analysis of quantitative and qualitative data separately, which are then compared simultaneously to better understand the phenomenon under study [ 33 ]. The main presumption of the research design was that both quantitative and qualitative data offer varying information, with the qualitative data providing narrative accounts of the participants and the quantitative data providing scores on selected variables [ 33 ]. This design provides a broader insight into the issue being studied by comparing the findings and results to discern whether they confirm or disconfirm each other. Combining both qualitative and quantitative methods as complementary methods has the potential to contribute to broader applicability of the findings in other settings or with other wider populations [ 33 - 36 ]. This method is applicable in this study given that the quantitative data provided insight into data on many Bukhali trial participants, whereas the qualitative data provided an in-depth narrative account of the mobile phone challenges faced by participants and other reasons for being hard to reach.
Participants and Recruitment
The study population consisted of young women aged 18 to 28 years who participated in the trial within the precinct of the Chris Hani Baragwanath Academic Hospital, located in Soweto, Johannesburg, South Africa, and were recruited into the intervention arm of the Bukhali trial [ 30 ]. Soweto is a largely overpopulated and multilingual area that lies on the outskirts of the greater Johannesburg city. It is characterized by several socioeconomic issues, namely, joblessness, gender-based violence, and food insecurity, as well as factors hindering healthy behaviors [ 37 ], which have the potential to influence the mobile phone use patterns of young women.
Overall, the study team contacted participants telephonically to (1) deliver monthly sessions to the participants, (2) book subsequent monthly sessions for the participants, (3) arrange to have supplements delivered to the participants’ homes, or (4) follow-up on the participants who have experienced an adverse event. The mobile phone information of the participants was captured by the study team to enable the team to reach the participants. For the quantitative component of this study, we included all young women who were contacted telephonically between August 2019 and January 2022. These women were contacted to either have a session delivered to them or to be booked for a session (n=363) out of the wider group of Healthy Life Trajectories Initiative participants. This was done to identify young women who were reachable and those who were unreachable. Participants who had (1) in-person contact with the study team (either off-site to deliver supplements or on-site to deliver a session), (2) contact via SMS, or (3) contacted through other means of communication (eg, home visits or instant messaging) were excluded from this study to focus on the extent to which participants could be contacted by phone.
The standard process used by the intervention team to facilitate the intervention involved making several call attempts to reach the participants. All call attempts and contact statuses of the participants were captured on a contact log. At most, 3 call attempts were made and if the participants were still unreachable, the study team generated tracing lists that prompted them to trace these participants by conducting further fieldwork to update the contact details of the participants. For the purposes of this study, we restricted the call attempts to only 1 call attempt given that the study only aimed to provide a cross-sectional picture and not an analysis that focuses on more longitudinal repeated attempts, which was beyond the scope of this study. Thus, participants who were reached at the first call attempt were classified as “reachable.” Participants whom the study team failed to make contact with at the first call attempt were classified as “hard to reach” or “failed to make contact.” In the same period, some participants changed their mobile number once, some did it several times, and others did not change their number. A flowchart indicating the stages of participant recruitment is shown in Figure 1 .

For the qualitative component, a subsample of participants (n=20) who were part of the Bukhali trial for ≥12 months were recruited telephonically for those who were easily contactable, and recruitment was performed by a fieldwork team for those who were hard to reach. This sample of participants was purposively selected using a convenience sampling technique. A group of 20 participants was selected by drawing them from the same group of young women who participated in the trial between August 2019 and January 2022. The final group of women who participated in the trial would be booked to receive a monthly session either in person, telephonically, or through SMS or to have supplements delivered to them. We then first restricted this group to only young women whom we failed to make contact with, those who had a session delivered to them, and those who were booked for a session. Furthermore, participants who could not be reached after repeated call attempts were excluded, and we then further restricted the sample to those who only had 1 call attempt. This resulted in a group of those who were contactable and those who were hard to reach, and this group was included in the quantitative analysis. Thereafter, 20 young women who were included in the qualitative analysis were selected from the 363 young women who were included in the quantitative analysis. This was done by selecting the record IDs of young women extracted from REDCap (Research Electronic Data Capture; Vanderbilt University), a secure database that stores data for research studies [ 38 ]. Qualitative data were collected only for this group of young women given that the trial aimed to deliver an intervention that fosters behavior change. Thus, the lack of contactability or loss to follow-up of this group of participants poses a substantial threat to the effective delivery of the intervention and could potentially have adverse biases on the conclusiveness of the results of the trial, which could have significant effects on the credibility and reliability of the trial.
Data Collection
Quantitative data collection.
Quantitative data collection was conducted, and the data were recorded and stored on REDCap [ 38 ]. The data included a set of interview-led administered questionnaires that collected baseline data on the demographic characteristics of the participants. The variables that were used in this study to show a descriptive profile of the sociodemographic characteristics of the participants who were contactable and those who were hard to reach included (1) age, (2) family size, (3) highest level of education, (4) vocational activity, (5) type of dwelling unit, (6) times in the past 12 months when family went hungry, (7) phone ownership, (8) contract type, and (9) type of cell phone.
In this component of the study, the first outcome of interest was the “contactability” of the participants. Two groups of young women were of interest in this phase of analysis. These were (1) young women who were contactable and (2) young women who were hard to reach at the first call attempt. The second outcome was the “mobile number changes of participants,” which were captured on REDCap. These number changes were tracked from randomization to the trial. The number changes were categorized as (1) no number change, (2) 1 number change, or (3) >2 number changes.
Qualitative Data Collection
Overall, 20 participants were interviewed individually using an in-depth interview guide by 2 female interviewers who were fluent in both English and other South African vernacular languages. The interview schedule was developed by the study team to capture the participants’ reasons for changing their mobile numbers and to discern why staying in contact with participants was challenging. Interview questions focused on young women’s demographic characteristics, questions around ownership of a mobile phone or mobile phones, mobile phone use behavior and patterns, and receipt of health information. The interviews were conducted face-to-face with the participants within the precinct of the Chris Hani Baragwanath Academic Hospital in February and March 2022. The interviews lasted between 30 and 40 minutes and were audio recorded. Interview notes were captured during each scheduled interview session to record the participants’ main accounts and nonverbal cues. Before analysis was conducted, the recordings were transcribed to their original form and translated into English, where necessary.
Data Analysis
Quantitative data analysis.
To statistically analyze the differences between the 2 groups of participants (failed to make contact and contactable), the data were analyzed descriptively at univariate and bivariate levels. At the univariate level, to visually illustrate the differences between the contact status of participants by mobile number change, a graph was generated to compare the proportion or rate of mobile number change of each group in relation to another. Similarly, another graph was generated to identify the most cited and least cited outcomes of the attempted call for participants we failed to make contact with. At the bivariate level, a contingency table was generated to depict the differences in the results of the tabulation of observations at each level of a variable for both groups, that is, cross-tabulation of the 2 groups to show the relationship or differences in the proportions and frequencies of the sociodemographic characteristics of the 2 groups. This table included the Pearson chi-square test of association to statistically identify the independence or association between the 2 groups or categorical variables and accompanying sociodemographic covariates. All data were analyzed using STATA (version 17; StataCorp) [ 39 ].
Qualitative Data Analysis
A reflexive thematic analysis approach was used for analysis using MAXQDA software (version 20; VERBI GmbH) [ 36 ], which helped in the recording, coding, and interpretation of the transcripts. Reflexive thematic analysis is an interpretive method that enables researchers to establish the outcome of the work rather than following a specific theory [ 35 ]. Six steps were used in the analysis. In the first step, data familiarization was conducted by checking the quality of the transcripts against the recordings to understand the data thoroughly, which would make it possible to search for patterns and meanings. This involved reading and rereading transcripts and taking notes. In the second step, initial codes were generated by labeling and organizing participants’ narratives to form a complete meaning.
In the third phase, initial themes were generated by sorting initial codes that had been generated into themes and identifying meanings and relationships between the initial codes. In the fourth phase, the themes were reviewed by identifying coherent patterns and ensuring that the generation of themes was supported by sufficient data. This also included collapsing overlapping themes and regenerating and improving the codes and themes. This was done as a collective effort among KM, LMS, and CED. In the fifth phase, themes were defined and named by linking each narrative to an appropriate theme. Finally, in the sixth phase, the narratives of the participants were presented under each generated theme in a concise manner.
Ethical Considerations
Ethics approval was granted by the Human Research Ethics Committee (Medical) located at the University of the Witwatersrand (M190449). All participants provided written informed consent to participate and consented to have the interviews audio recorded.
Although the information provided by the participants was captured on an audio recorder, the data were protected by securely storing them in a password-protected computer in a secure locked cabinet. The identities of the participants were protected through the deidentification of all their personal information and characteristics. The transcripts contained ID numbers that represented each participant’s response.
Quantitative Results
The sociodemographic characteristics of the participants (N=363) are presented in Table 1 . Of the 363 participants, 174 (47.9%) were hard to reach telephonically, whereas 189 (52.1%) were easy to reach telephonically. Most participants (133/243, 54.7%) who were contactable did not change their mobile number. The highest percentage of mobile number changes was observed among participants who were difficult to reach, with three-quarters of the participants (12/16, 75%) being reported to have changed their mobile number ≥2 times ( Multimedia Appendix 1 ). Although 58% (101/174) of the participants could not be reached as the attempted call went to voicemail, 29.3% (51/174) of the participants were reported to not have taken the call when the attempted call was placed, and only 2.2% (4/174) of the participants took the call but ended it ( Multimedia Appendix 2 ).
Table 2 shows the mobile phone information of both hard-to-reach participants and contactable participants. There was a significant difference in mobile phone ownership among participants who were hard to reach and those who were contactable, as 96.8% (183/189; P =.03) of those who were contactable owned a personal cell phone compared with 91.4% (159/174) of those who were hard to reach. Most of the young women (both contactable and hard to reach) had a smartphone (297/342; >80% each; P =.02) and did not have a phone contract (324/342; >90% each). Results indicated no statistically significant differences in these variables among participants who were hard to reach and participants who were contactable.
Characteristics | Failed to make contact (n=174), n (%) | Contactable (n=189), n (%) | Total (N=363), n (%) | value | ||||||||
.19 | ||||||||||||
18-20 | 70 (40.2) | 59 (31.2) | 129 (35.5) | |||||||||
21-23 | 48 (27.6) | 64 (33.9) | 112 (30.8) | |||||||||
24-26 | 38 (21.8) | 51 (27) | 89 (24.5) | |||||||||
27-28 | 18 (10.3) | 15 (7.9) | 33 (9.1) | |||||||||
.78 | ||||||||||||
1 | 8 (4.6) | 13 (6.9) | 21 (5.8) | |||||||||
2 | 13 (7.5) | 17 (9) | 30 (8.3) | |||||||||
3 | 25 (14.4) | 23 (12.2) | 48 (13.2) | |||||||||
4-7 | 95 (54.6) | 105 (55.6) | 200 (55.1) | |||||||||
>7 | 33 (19) | 31 (16.4) | 64 (17.6) | |||||||||
.08 | ||||||||||||
Primary | 57 (32.8) | 42 (22.3) | 99 (27.3) | |||||||||
Secondary | 91 (52.3) | 118 (62.8) | 209 (57.7) | |||||||||
Tertiary | 8 (4.6) | 13 (6.9) | 21 (5.8) | |||||||||
Other | 18 (10.3) | 15 (8) | 33 (9.1) | |||||||||
Missing | 0 (0) | 1 (0.5) | 1 (0.5) | |||||||||
.07 | ||||||||||||
Currently enrolled in higher education institution, employed, or currently looking for a job | 9 (5.2) | 19 (10) | 28 (7.7) | |||||||||
Not enrolled in higher education institution, not employed, or not currently looking for a job | 165 (94.8) | 170 (89.9) | 334 (92.3) | |||||||||
.43 | ||||||||||||
House of brick or concrete block structure on a separate stand or yard or on a farm | 122 (70.1) | 128 (67.7) | 250 (68.9) | |||||||||
House, flat, or room on your homestead | 24 (13.8) | 35 (18.5) | 59 (16.2) | |||||||||
Informal dwelling or shack in backyard | 15 (8.6) | 14 (7.4) | 29 (8) | |||||||||
Informal dwelling or shack not in backyard | 7 (4) | 3 (1.6) | 10 (2.7) | |||||||||
Other | 6 (3.4) | 9 (4.8) | 15 (4.1) | |||||||||
.12 | ||||||||||||
Yes | 68 (39.1) | 59 (31.2) | 127 (35) | |||||||||
No | 106 (60.9) | 130 (68.8) | 236 (65) |
a Missing refers to missing data or missing values that have not been stored for the education level of some participants (could be attributed to incomplete data entry or no information provided by participants).
Failed to make contact, n (%) | Contactable, n (%) | Total, n (%) | value | ||||||
Yes | 159 (91.4) | 183 (96.8) | 342 (94.2) | ||||||
No | 25 (8.6) | 6 (3.2) | 21 (5.8) | ||||||
Total | 174 (100) | 189 (100) | 363 (100) | ||||||
.20 | |||||||||
Yes | 11 (6.9) | 7 (3.8) | 18 (5.3) | ||||||
No | 148 (93.1) | 176 (96.2) | 324 (94.7) | ||||||
Total | 159 (100) | 183 (100) | 342 (100) | ||||||
.32 | |||||||||
Yes | 135 (84.9) | 162 (88.5) | 297 (86.8) | ||||||
No | 24 (15.1) | 21 (11.5) | 45 (13.2) | ||||||
Total | 159 (100) | 183 (100) | 342 (100) |
a P <.05 (indicates significant association between participants’ ownership of a mobile phone and contact status).
Qualitative Results
Table 3 shows the sociodemographic profile of the interview participants. The mean age of the participants who were interviewed was 22 (SD 2.94) years. In addition, 50% (10/20) of the participants had a secondary school qualification, 40% (8/20) had not completed secondary education, and only 10% (2/20) had a tertiary qualification at the time of the assessment. Almost all the participants (19/20, 95%) lived with a parent or relative, whereas only 5% (1/20) of the participants lived with her partner and 65% (13/20) of the participants were not in a relationship at the time of assessment.
Textbox 1 presents an overview of the superordinate themes and subthemes generated from the qualitative analysis. As presented in Textbox 1 , this section is sorted into the narrative findings, with illustrative quotations provided for each superordinate theme and subtheme.
Respondent number | Current age (years) | Highest level of education attained | Lives with | Relationship status |
Participant 1 | 25 | Secondary school qualification | Siblings and child | Single |
Participant 2 | 22 | Tertiary qualification | Parent | Single |
Participant 3 | 21 | Secondary school qualification | Parents, child, and sibling | Single |
Participant 4 | 22 | Grade 11 | Parent, siblings, and child | Single |
Participant 5 | 28 | Grade 11 | Parent, sibling, children, and relatives | Single |
Participant 6 | 20 | Grade 11 | Parent and siblings | In a relationship |
Participant 7 | 21 | Secondary school qualification | Parent | Single |
Participant 8 | 26 | Grade 11 | Partner | In a relationship |
Participant 9 | 20 | Secondary school qualification | Parent, siblings, and relatives | Single |
Participant 10 | 24 | Grade 10 | Grandparent, siblings, and children | In a relationship |
Participant 11 | 22 | Grade 11 | Grandparent and relatives | Single |
Participant 12 | 24 | Grade 11 | Parent, siblings, and relative | In a relationship |
Participant 13 | 20 | Secondary school qualification | Parent, sibling, and child | Single |
Participant 14 | 21 | Currently doing matric | Sibling, parent, and child | In a relationship |
Participant 15 | 25 | Secondary school qualification | Relatives | Single |
Participant 16 | 20 | Secondary school qualification | Parent, siblings, and child | In a relationship |
Participant 17 | 20 | Secondary school qualification | Siblings | Single |
Participant 18 | 22 | Secondary school qualification | Parents | Single |
Participant 19 | 22 | Tertiary qualification | Grandparents and relatives | Single |
Participant 20 | 24 | Secondary school qualification | Sibling, relatives, and child | In a relationship |
Superordinate theme and subthemes
- Mobile phone technical issues
- Electrical unreliability
- Network connectivity issues
- Lack of ownership of personal cell phone
- Unregistered number
- Cell phone theft in the community
- Stalking behavior
- Inconsistent SIM use
- Availability of data and airtime
- Lack of interest in possessing a mobile phone
Mobile Phone Technical Issues
Technical barriers were reported by most participants as a major challenge that affected the efficiency, functioning, and usability of their mobile phones. Key challenges reported by the participants that resulted in inherent limitations in operating their mobile phones included poor battery life, faulty charging system, mobile phone and app crashes, and an inadequate touch screen response. Such challenges resulted in the participants not being easily contactable or contactable at all. Some participants resorted to changing their mobile phones or using alternative communication methods such as using the phones of individuals within their social networks:
I’ve experienced battery challenges and the charging system, ja that’s the only thing that’s been wrong with my phone. I had to charge my phone a certain way and I couldn’t even use it until I finished charging and the battery will finish fast sometimes when I’m trying to do something. [Participant 2, 25 years old, single]
The phone I was using, it lived on life support. I had to use it while it was in a charger at home. The charger also was faulty so sometimes I would think it’s on and it’s off. [Participant 9, 20 years old, single]
It sometimes jams. The battery finishes quickly while you are chatting. So those are the challenges that I face with my phone. [Participant 16, 20 years old, in a relationship]
My phone has problems. Like it just switches off, because it fell once, and now every time when it hits hard or something, it just switches off, and it is going to take time for it to switch on, so I wait for it to switch on. [Participant 1, 25 years old, single]
The touch screen doesn’t work properly now because it fell sometime last year. I don’t know if it’s a space issue or just the phone itself because sometimes when I get incoming calls the phone rings but then I can’t touch it for me to be able to pick up the call because of the touch screen problems with the phone. [Participant 13, 20 years old, single]
It has a tendency to freeze if it fell, maybe if I’m receiving a call, I can’t answer it because the phone is frozen, you know. [Participant 15, 25 years old, single]
Coverage Issues
Coverage issues such as network and electricity made it difficult for some participants to be contactable. Participants indicated that they could not receive calls or messages or had to change their mobile SIMs because of challenges concerning the connectivity, reliability, and quality of the mobile network. This included signal weakness and network availability issues with some mobile service providers being cited as having general network unreliability or the network being offline in some instances. Participants reported experiencing unreliability of the electric supply owing to frequent power cuts. This greatly affected their ability to use their mobile phones, thus being unable to be in touch with people:
My network becomes very slow, sometimes I don’t even get calls I just get messages that I have a missed call but then it didn’t even ring if you don’t have electricity. It’s been hectic hey, we didn’t have electricity on Sunday. [Participant 2, 22 years old, single]
It has been a while since we have been without electricity but right now, we don’t have it because they said that the substation was on fire. [Participant 7, 21 years old, single]
I first bought the Telkom sim card but then I don’t know what happened with the coverage in Orlando, but it was giving me problems, so I couldn’t get network, so I used my MTN sim card. I think I got it like two years ago. I was not using it as frequently as the Telkom number, so I had to use the MTN number. [Participant 9, 20 years old, single]
Telkom network is problematic, sometimes you want to make a call, it will tell you that there is no service, things like that. Sometimes the sim card does not connect. I will find myself taking it out and putting it back on but still, so I decided to change MTN, then, MTN gave me a problem of taking my airtime every time when I recharged. [Participant 1, 25 years old, single]
Lack of Ownership of Personal Cell Phone
Some participants indicated that they were not easily contactable because they do not currently own their own personal cell phone or previously did not own one. The reasons attributed to the lack of ownership ranged from the phone being reported to be broken, stolen, lost, or not having one at all. Despite this, participants used alternative means of communication to try and be contactable. Participants reported using their social networks to try and solve the problem of not having a mobile phone by sharing a phone with their siblings, peers, or partners. However, sharing a phone limited their ability to stay connected with others and to be reachable because their time with the device was limited. In some cases, this was exacerbated by the participants not living close to the people they shared the phone with. In other instances, participants’ social networks would not relay the message back to them. These narratives are reflected in the following excerpts:
I didn’t have a phone for two months, so I spent quite a while without a phone. I used parents’ phones. It was for when people couldn’t get hold of me, they would call my parents for emergencies. [Participant 18, 22 years old, single]
I didn’t have a phone for four months. I was not reachable. I used my boyfriend’s phone. The first number that I applied with is my grandmothers, that was the first one. I did not have a phone at that time, and so I applied with her phone, and they tried to call her, but it did not get through to her. Because her phone, she is a person that does business, it would switch off and she would not find the charger, and then it would be charged after a while, and then I gave them the number of my partner, and then they said that they can’t get me with that one as well. [Participant 10, 24 years old, in a relationship]
I stayed for a long time without a phone. I think it had been two years without having a phone. I used to borrow my friend’s phone if for instance there is someone who had asked for my number, then I would give them my friend’s number, and my friend would tell me that so and so had phoned, they called me because you don’t have a phone. So, when people wanted my number, I would give them my friend’s number. It was not easy for them to get hold of me because sometimes I am at my home, and she is at her home. [Participant 1, 25 years old, single]
Other participants further highlighted that they were not contactable because their mobile phone was stolen or had gone missing:
I lost my phone and then I took time to do the sim swap and by the time I decided to do it, they had already given the number to someone else. [Participant 9, 20 years old, single]
I started by using said provider, yes I was using said provider first, and now I am on another provider because my phones got lost. [Participant 11, 22 years old, single]
I had a phone, it was last year, I had another phone and they mugged me in March. [Participant 20, 24 years old, in a relationship]
One participant lost her mobile phone due to theft, but her mobile SIM was still in her possession. As an alternative means of communication, she would insert her SIM card in other people’s phones and reach out to people given that she did not have the financial means to buy a new phone:
I was going to the mall and then I was mugged but then I have a sim card which I did a sim swap, yes so every time I need a phone, I can contact people I wasn’t able to contact. [Participant 12, 24 years old, in a relationship]
Unregistered Number
Another factor contributing to the difficulty in reaching participants is the unregistered status of their mobile numbers. Some participants reported that they had lost their phones with the mobile SIM card. Two participants reported that they bought new phones and SIM cards and went to a mobile phone store to get a SIM swap, but the mobile phone store could not perform the SIM swap. One reason for this was because their previous mobile numbers were no longer registered under the participants’ names and had been reassigned to new owners. Another reason was the inability to recall the last 5 numbers called on the SIM, which is a requirement for performing a SIM swap. Consequently, participants had to purchase new SIM cards with new mobile numbers, different from those recorded by the trial:
I had to go this year January to Telkom to do a sim swap because I had applied for jobs with that number. Then when I got to Telkom, they told me that that number was not registered under my name, you see these sim cards that you get on the streets. So, I had to start another sim and so I started afresh with that number. [Participant 17, 20 years old, single]
They took my number in January and then I got this one in February. [Participant 11, 22 years old, single]
I lost the phone and then when I went to do the sim swap, they told me I couldn’t do it because the numbers were wrong, and that I wanted the last five numbers that I last called, and so I was not able to continue with the sim swap. [Participant 7, 21 years old, single]
Use Patterns
Although many participants owned a personal cell phone, an inconsistent pattern of mobile phone use was observed in the narratives of the participants, which impacted the ability to contact them. Some participants reported that they did not carry their mobile phones outdoors and only used the device at home. However, the reasons for this behavior were not reported by the participants:
I don’t use it when I am on the road, even when I am going to town or to the mall, I usually leave it behind. [Participant 3, 21 years old, single]
When I go outside, I don’t take it with me, I leave it at home. I use it when I know that I am not going anywhere. [Participant 11, 22 years old, single]
Sometimes I’m at school, and sometimes I’m busy and I left it at home and went to the shops, ja I’m not on my phone every time, I just use my phone only when I do important things, ja. [Participant 14, 21 years old, in a relationship]
I couldn’t go out with it, like I had to use it at home. When I go out, I leave it at home so I had to tell the person that I am meeting that we are meeting at a specific time and place, and I just had to hope they will be there. [Participant 9, 20 years old, single]
Conversely, some participants reported that their communities were not safe and there was a high rate of mobile phone theft in their area. This prompted them to not use their mobile phones in public. This has contributed to participants not being easily contactable given that they use their mobile phones inconsistently:
I don’t carry my phone in the streets. It’s not safe because most of the time there’s people taking other people’s phones, most of the time, like maybe in a week we’ll hear about 2 people, 3, ja so it’s not safe. [Participant 2, 22 years old, single]
I use when I am at home alone, outside there are a lot of thieves, when you are outside and you are using your phone, they take it from you. [Participant 10, 24 years old, in a relationship]
Conversely, another participant indicated that she was no longer comfortable using her phone or taking calls as she had experienced continuous threatening harassment over the phone from an unknown individual. This thus resulted in other people finding it difficult to reach her:
There were people stalking me, and I didn’t know where they were getting my number from. They would tell me where I was, meanwhile, I don’t know them and I didn’t see them, then I decided to change my number. [Participant 3, 21 years old, single]
Inconsistent SIM Use
For other participants, their inconsistent mobile phone behavior was largely because of the participants owning dual SIM cards. Participants reported that they often used one mobile number more than the other, whereas another participant indicated that she had to change her SIM cards frequently given some technical issues she was experiencing with the card. Similarly, another participant reported changing her SIM cards frequently because she would lose 1 SIM because of repeatedly changing mobile devices and thereafter had to replace it with another SIM card. Another participant’s inconsistent use of her mobile phone was largely because of her younger sibling who did not own his own personal cell phone. Given this circumstance, she shared her phone with her younger sibling as he frequently took out the participant’s SIM card and put his own for a certain period. This greatly affected the participant’s ability to receive calls or messages, thus making her seemingly unreachable. Other participants indicated that the procedures for performing a SIM swap are often offline every time they went to do one. This has led to the participants’ losing interest in doing a SIM swap and has prompted them to change their mobile number. All these factors have thus contributed greatly to the participants being unreachable:
The sim card used to get lost because I kept changing the phones. I was using the other network’s * number. The 060. So now I am using another network. I don’t know the other network’s number, but this one. [Participant 10, 24 years old, in a relationship]
My younger brother likes using it a lot because he likes logging in on Facebook and chat with his friends and then bring it back. He takes out my starter pack and puts in his own. Maybe they call me, and it goes to voicemail, that is because my sim card is out. [Participant 1, 25 years old, single]
I have two numbers and there is one that I always use and the other one that I use sometimes, because I have two sim cards, and both are in the phone. So sometimes I give people the number that I don’t use every day. [Participant 16, 20 years old, in a relationship]
I used to use two starter packs because I wanted to use Telkom and MTN, and at the time I was using Cell C as well. I had a lot of starter packs, and I changed the numbers a lot. Telkom was giving me problems, and then MTN used to take airtime every time when I recharged. I didn’t know where my airtime was going every time when I recharged the number, so yeah, I used to change the number a lot. [Participant 1, 25 years old, in a relationship]
Because when I do a sim swap, they ask a lot of questions, so I sometimes find that it is better if I change the number. Yes because this time I had to do a sim swap, and they said I should go to Shoprite with my ID and my proof of address, when I got to Shoprite, they said that their machines were offline, and then that is where I gave up, and then I said it is better that I change my numbers, because the numbers of most of the people that I know, I have them, I have written them in my book. [Participant 11, 22 years old, single]
You know what, I was tired of changing number so I decided that you know what, let me stick to this number because a lot of people have this number, and they call me on it, when I give out my CVs, this is the number that I use, so I don’t take it out, this is the one that I am using. [Participant 17, 20 years old, single]
Interestingly, 1 contrary finding from 1 participant’s narrative showed that the participant had the same number for >10 years and decided not to change her number to ensure that she remained contactable:
I’ve had this number for more than 10 years I think, the reason why it was more it made sense to do a sim swap instead of changing numbers it was for convenience and with this phone number I had registered it on a lot of platforms, you know when you apply online when they ask you to leave your details you leave your number, so the first thing that came to mind is that for people to be able to get hold of me it’d better to have the same number and also because had lost contacts as well for me to get back my contacts I had to have the number I was using. [Participant 15, 25 years old, single]
Availability of Data and Airtime
Other participants reported that a major challenge that they face, which has resulted in them not being able to stay in contact with people or being difficult to reach, is that airtime and data costs are very steep, and they lack the financial ability to afford data and airtime and thus cannot purchase it regularly. As a result, there are times when they do not have any airtime or data on their phone and thus cannot make or return any calls or messages received:
When I don’t have money where I am, or I don’t have money at that time. It depends on whether I have data at the time. If in a week my data finishes on Wednesday, then I will buy it on Sunday. There are days when I don’t have data. Maybe for a short while, for maybe two or one week. [Participant 16, 20 years old, in a relationship]
I was supposed to attend the interview today and I was unable to go, because I did not have data and I saw the message on a Friday. [Participant 3, 21 years old, single]
Before I bought a new phone, I used Vodacom, but I just didn’t like it because their data and airtime are expensive. So, the other phone came with an MTN sim, and I carried on with that one. [Participant 18, 22 years old, single]
Lack of Interest in Possessing a Mobile Phone
Some participants reported no interest in possessing a mobile device to stay in contact with their social networks or being contactable on the phone. One of the common reasons for this was that the participants were often in the vicinity of their social networks and could communicate in person as opposed to communicating via mobile phone. Others mentioned that they do not have friends with whom to communicate, whereas others hardly used their phones and often misplaced it given the lack of importance they give to owning a phone:
Not that important, it’s not that important in fact. Because I don’t spend a lot of time on the phone, so I wouldn’t say that it is important. Eh, they can contact me during the say but sometimes I don’t answer the phone. I think I don’t like it that much to have it all the time, I feel like I can stay without a phone. [Participant 16, 20 years old, in a relationship]
It’s not that important. It’s just to keep boredom off. I don’t care much about the phone. [Participant 10, 24 years old, in a relationship]
I don’t spend a lot of time on the phone, so I wouldn’t say that it is important. I think I don’t like it that much to have it all the time, I feel like I can stay without a phone. [Participant 16, 20 years old, in a relationship]
I am very reckless with my phone. I don’t use my phone often so I just leave it on the table or the cupboard or wherever and then I leave the house and people will call me and not reach me until I started to realise that okay, I need to send my CV somewhere and a phone is necessary to have on you. [Participant 5, 28 years old, single]
One participant indicated that she had previously lost her phone before purchasing a new one months later. The amount of time she spent without a phone resulted in her losing interest in possessing a phone as she had grown accustomed to being a nonmobile user:
I didn’t see the importance of having a phone. I stayed a long time, like I didn’t care about the phone. I stayed for a long time without it, and I didn’t feel like I didn’t have a phone. So, I didn’t see the importance of having a phone, even though I saw other people with phones, but for me, I never had that feeling of not having a phone. I had already gotten used to not having a phone. [Participant 12, 24 years old, in a relationship]
In contrast to these findings, other participants indicated that possessing a mobile phone was important to them and helped them to stay in touch with their social networks, to keep abreast of any job opportunities that may arise, to apply to university or college, and to access emails and the internet. Participants indicated using their phones all the time thus suggested that they were easily contactable and reachable. These findings are reflected in the following excerpts:
It’s very important because they need to find me every time I’m needed and most things I do on the phone, so I view it most times. I use my cell phone a lot, like every day. [Participant 2, 22 years old, single]
I use my phone all the time like 10 hours. When I wake up, before I go to bed, and I always provide the alternative number in case my phone is maybe low, or I have lost it. I always put in an alternative number. [Participant 9, 20 years old, single]
It’s important because even the study get hold of me on that phone. When people are looking for me, they get hold of me on that phone. Even now the study helped me because if I didn’t have a phone, I would not be reachable because it has been a while since I have been here. [Participant 19, 22 years old, single]
Principal Findings
This study aimed to explore the challenges that participants experience in terms of being contactable through mobile phones in a trial conducted in Soweto, South Africa. This study highlighted that although most participants who are difficult to contact do own a personal cell phone, which is a smartphone, most of them face a myriad of interrelated mobile phone challenges, particularly technical and coverage issues, mobile theft in their communities, and high costs of data and airtime. Consequently, these challenges contribute to participants’ need to change their mobile numbers, which subsequently has the potential to affect their reachability. The novelty of this study was the use of a mixed methods research design. It was important to conduct a study of this nature within the context of Soweto, a resource-constrained setting, which is characterized by a short supply of free public Wi-Fi, unequal mobile coverage, and comparably high data costs [ 40 ], among other factors.
This study was descriptive in nature. Therefore, caution should be exercised when interpreting the results of this study as no significant associations were found in the quantitative analysis. The quantitative results of this study showed that a large proportion of individuals owned a mobile phone. This result is in line with previous literature, which has shown that mobile connectivity in South Africa has grown rapidly with a mobile penetration rate of >95%, with 91% of all phones being smartphones [ 1 , 17 , 24 ]. Although this was also corroborated by the qualitative findings, a key finding was that some participants were mobile phone sharers, whereas others had no phones at all and thus relied on their social networks who had phones and often depended on using these phones for a short while to be in touch with other individuals. Previous literature has shown that the use of mobile phones is an inherent part of people’s lives, which exists in socioeconomic practices and realities [ 15 ]. Although South Africa has seen an exponential increase in mobile phone ownership and access, the high cost of mobile phones still represents a formidable barrier that limits mobile phone use [ 40 ]. In fact, the lack of income has been reported as the primary factor in the lack of phone ownership in many LMICs [ 41 , 42 ]. This largely surpasses other probable barriers such as the perceived importance of using a mobile phone and technical literacy [ 41 , 42 ].
Given that this study aimed to explore the challenges that participants experience in terms of being contactable through mobile phones, overall, the quantitative and qualitative findings in this study suggest that the socioeconomic context of the participants is a major factor that has contributed to their mobile use behavior and difficulty in reaching them. Thus, the findings of this study uniquely contribute to the existing body of knowledge in various ways. First, although phone ownership has increased rapidly in South Africa, there are some important use gaps that remain pronounced, and these are evident in an LMIC such as Soweto. To illustrate this, although phone ownership may be predominantly high among both groups included in this study, their socioeconomic backgrounds are complex and create different realities, which subsequently leads to variability in mobile phone use and usability. Thus, daily realities such as unemployment, poverty, and infrastructural barriers have the potential to prevent them from fully using their devices in a sustainable manner. In addition, the challenges that were explored in this study in terms of participants being contactable through the mobile phone show that the experiences of youth are vastly different, and complexities around socioeconomic backgrounds and environmental settings create different realities pertaining to phone possession and use. Although the participants may reside in one central area, the circumstances of young people (phone sharers, phone owners, and non–phone owners) differ quite substantially, not only depending on their age, level of education, or vocational activity but also on factors such as socioeconomic circumstances, family structure, household resources, and livelihood patterns. This aligns with the evidence from the World Bank, which has shown that 50% of South African inhabitants who reside in urban settings live in low-income settings, accounting for approximately 40% of the individuals who constitute the working population and catering to approximately 60% of nonworking citizens who live in cramped family settings [ 29 ].
These previous findings align with the quantitative results of this study, which demonstrated that most participants were individuals who were still in early young adulthood (18-25 years), with >90% of them Not in Education, Employment, or Training and only 5% had a mobile phone contract. Previous studies have also indicated a digital divide along gender lines [ 43 ]. The study indicated that women are more at risk of poor mobile phone use compared with men and attributed it to economic inequality, namely, women’s lower educational attainment and poor income levels [ 43 ]. These findings illuminate the way mobile phone use is interwoven with the daily realities and experiences of the participants, which has the potential to prevent the participants from fully using their devices in a sustainable manner.
Existing research has further shown that individuals who earn a low income have limited connectivity, which is inhibited by disparities in wealth, resources, and other opportunities, presenting a challenge in terms of using mobile technologies [ 44 ]. This finding is strongly congruent with the qualitative findings, which revealed that participants faced a myriad of mobile phone challenges, leading to them being hard to reach, which is in line with what the study aimed to explore (challenges experienced in terms of being contactable over the mobile phone). Such structural inequalities that make it hard for participants to be easy to reach include coverage issues, high costs of data, and airtime and safety in their communities. Furthermore, the quantitative results of this study showed that in addition to the fact that most participants only have a secondary school qualification and are Not in Education, Employment, or Training, approximately one-third of these participants have been reported to live in households that are food insecure. Household constraints serve as important factors for people’s access to mobile technology [ 45 ]. Another study showed that ownership of a mobile phone necessitates the need to have a phone that is in good working condition (available data and airtime, functional battery, and network signal) and the financial freedom to sustain this without giving up other necessities [ 46 ]. All these factors are unevenly distributed, with those living in resource-constrained settings being the most disadvantaged [ 46 ]. These findings thus suggest that the mobile phone challenges experienced by the participants are interwoven with their socioeconomic backgrounds, thus making it difficult to maintain mobile technology use.
This study further found that the proportion of those who own phones decreased with each successive age category for both participants who were hard to reach and those who were contactable. These findings are supported by the previous literature, which showed that age is a social determinant of digital inequality [ 22 ]. A curvilinear relationship was found between age and digital use in LMICs [ 22 ]. For instance, 1 study conducted in India showed that phone ownership was >66% for participants aged 15 to 24 years but only 55% for those aged 25 to 44 years [ 41 ]. In addition, a study conducted in Tanzania found that women aged ≤30 years were 17% less likely to remain phone owners (Roessler, P, unpublished data, December 2018).
Mobile technologies have the potential to improve health behaviors among individuals participating in clinical research [ 38 ]. For instance, the COVID-19 pandemic has led to an increased need to use mobile technologies for health promotion because of social distancing and nationwide lockdowns [ 47 ]. This reliance on mobile technologies means that challenges in reaching these participants could disrupt the continuity of health promotion for both research and clinical practice. Participants could, for example, seek health advice outside of their health care system or not attend their regular health sessions [ 39 ] owing to unresolved concerns via telephone. This may thus lead to clinical interventions being highly inefficient. Thus, using mobile phones in any clinical study can inform how these devices could be used in future interventional research. Although this study found that the mobile challenges that participants face are mostly influenced by structural inequalities, it is pivotal to note that addressing and alleviating these structural inequalities is beyond the scope of this study.
However, several feasible solutions exist for the problems encountered in this study. To maximize engagement in mobile health studies, health promotion initiatives can be delivered via in-person consultations or sessions with the participants. These in-person consultations would afford participants the opportunity to have increased personal contact with the study team and to obtain study support and personalized study feedback. This strategy has proved to be successful in a previous clinical trial where personalized care, including allowing participants to share their personal problems and enabling participants to increase contact with the study team or investigators, helped in maximizing engagement [ 48 ]. This could include fieldwork to complement the use of mobile technologies and thus ensure participant retention. Furthermore, advisory groups could be created by bringing together the participants and giving them an opportunity to engage in discussions around crafting possible solutions to the mobile technology barriers that were identified in this study. In addition, automated health promotion messages could be relayed to the participants’ phones, particularly in instances where calls do go through but are not answered by participants.
Strengths and Limitations
The strength of this study was the use of a convergent parallel mixed methods approach, which assisted in exploring the quantitative findings by relating the findings obtained from the qualitative and quantitative analyses. The use of this method thus helped in providing comprehensive insight into the mobile phone behaviors of young women and the factors that contributed to this behavior. A limitation of this study was that the quantitative analysis was only restricted to the first instance (ie, the first call attempt in which some young women were hard to reach and some were easily contactable). Therefore, repeat instances or call attempts for subsequent monthly sessions were excluded. This could have provided a broader longitudinal picture of the time from randomization to infancy of all call attempts and whether any demographic characteristics were associated with these changes. However, this was beyond the scope of this study as the focus was only on providing a descriptive profile of women who were hard to reach while comparing their demographic profile with those who were easily contactable.
Conclusions
Despite the availability of mobile technology and network accessibility, there are substantial economic barriers that prevent young adults from fully benefitting from technology. These barriers consequently affect health promotion and behavioral changes. For individuals to remain digitally connected, multiple strategies should be used. For research, remote data collection remains an important tool in public health research and could thus serve as a hugely beneficial mechanism in connecting with participants while actively using established relationships with participants or community-based organizations to deliver health promotion and practice. With increasing phone ownership in LMICs including South Africa, greater accessibility to less expensive or free data networks or digital platforms is needed universally across South Africa to ensure more equitable access to such technologies.
Acknowledgments
This study was supported by the South African Medical Research Council and the Canadian Institutes of Health Research. SAN was supported by the Department of Science and Innovation-National Research Foundation Centre of Excellence in Human Development.
Data Availability
The data sets generated and analyzed during this study are not publicly available because the trial is still ongoing but are available from the corresponding author on reasonable request.
Conflicts of Interest
None declared.
Contact status of the trial participants by mobile number change.
Outcome of the call attempted for participants who were unreachable.
- Soepnel LM, McKinley MC, Klingberg S, Draper CE, Prioreschi A, Norris SA, et al. Evaluation of a text messaging intervention to promote preconception micronutrient supplement use: feasibility study nested in the healthy life trajectories initiative study in South Africa. JMIR Form Res. Aug 18, 2022;6(8):e37309. [ FREE Full text ] [ CrossRef ] [ Medline ]
- Mechael PN. A look back to look forward: a multidisciplinary evaluation of an mHealth service in Malawi. Afr Popul Stud. Jun 04, 2015;29(1):1729-1733. [ FREE Full text ] [ CrossRef ]
- Njoroge M, Zurovac D, Ogara EA, Chuma J, Kirigia D. Assessing the feasibility of eHealth and mHealth: a systematic review and analysis of initiatives implemented in Kenya. BMC Res Notes. Feb 10, 2017;10(1):90. [ FREE Full text ] [ CrossRef ] [ Medline ]
- Khan NU, Rasheed S, Sharmin T, Siddique AK, Dibley M, Alam A. How can mobile phones be used to improve nutrition service delivery in rural Bangladesh? BMC Health Serv Res. Jul 09, 2018;18(1):530. [ FREE Full text ] [ CrossRef ] [ Medline ]
- Aker JC, Mbiti IM. Mobile phones and economic development in Africa. J Econ Perspect. Aug 2010;24(3):207-232. [ CrossRef ]
- Anstey Watkins JO, Goudge J, Gómez-Olivé FX, Griffiths F. Mobile phone use among patients and health workers to enhance primary healthcare: a qualitative study in rural South Africa. Soc Sci Med. Feb 2018;198:139-147. [ FREE Full text ] [ CrossRef ] [ Medline ]
- Till S, Mkhize M, Farao J, Shandu LD, Muthelo L, Coleman TL, et al. CoMaCH Network. Digital health technologies for maternal and child health in Africa and other low- and middle-income countries: cross-disciplinary scoping review with stakeholder consultation. J Med Internet Res. Apr 07, 2023;25:e42161. [ FREE Full text ] [ CrossRef ] [ Medline ]
- Mistiaen P, Poot E. Telephone follow-up, initiated by a hospital-based health professional, for postdischarge problems in patients discharged from hospital to home. Cochrane Database Syst Rev. Oct 18, 2006;2006(4):CD004510. [ FREE Full text ] [ CrossRef ] [ Medline ]
- Dudas V, Bookwalter T, Kerr KM, Pantilat SZ. The impact of follow-up telephone calls to patients after hospitalization. Am J Med. Dec 21, 2001;111(9B):26S-30S. [ FREE Full text ] [ CrossRef ] [ Medline ]
- Chi BH, Stringer JS. Mobile phones to improve HIV treatment adherence. Lancet. Nov 27, 2010;376(9755):1807-1808. [ CrossRef ] [ Medline ]
- Hasvold PE, Wootton R. Use of telephone and SMS reminders to improve attendance at hospital appointments: a systematic review. J Telemed Telecare. Sep 20, 2011;17(7):358-364. [ FREE Full text ] [ CrossRef ] [ Medline ]
- Mahmud N, Rodriguez J, Nesbit J. A text message-based intervention to bridge the healthcare communication gap in the rural developing world. Technol Health Care. 2010;18(2):137-144. [ CrossRef ] [ Medline ]
- Gold J, Lim MS, Hellard ME, Hocking JS, Keogh L. What's in a message? Delivering sexual health promotion to young people in Australia via text messaging. BMC Public Health. Dec 29, 2010;10:792. [ FREE Full text ] [ CrossRef ] [ Medline ]
- Gisore P, Shipala E, Otieno K, Rono B, Marete I, Tenge C, et al. Community based weighing of newborns and use of mobile phones by village elders in rural settings in Kenya: a decentralised approach to health care provision. BMC Pregnancy and Childbirth. Mar 19, 2012;12:15. [ FREE Full text ] [ CrossRef ] [ Medline ]
- Gilbert JW. Telemedicine by cell phone technology. Pediatr Neurosurg. Mar 18, 2011;46(5):408. [ CrossRef ] [ Medline ]
- Thinyane H, Hansen S, Foster G, Wilson L. Using mobile phones for rapid reporting of zoonotic diseases in rural South Africa. Stud Health Technol Inform. 2010;161:179-189. [ CrossRef ] [ Medline ]
- Noordam AC, Kuepper BM, Stekelenburg J, Milen A. Improvement of maternal health services through the use of mobile phones. Trop Med Int Health. May 2011;16(5):622-626. [ FREE Full text ] [ CrossRef ] [ Medline ]
- Haleem A, Javaid M, Qadri MA, Suman R. Understanding the role of digital technologies in education: a review. Sustain Oper Comput. May 23, 2022;3:275-285. [ FREE Full text ] [ CrossRef ]
- World Bank Group, International Telecommunication Union. The little data book on information and communication technology. World Bank. Washington, DC. World Bank Publications; 2018. URL: https://www.itu.int/en/ITU-D/Statistics/Documents/publications/ldb/LDB_ICT_2018.pdf [accessed 2024-03-16]
- Hightow-Weidman LB, Muessig KE, Bauermeister J, Zhang C, LeGrand S. Youth, technology, and HIV: recent advances and future directions. Curr HIV/AIDS Rep. Dec 2015;12(4):500-515. [ FREE Full text ] [ CrossRef ] [ Medline ]
- Smale WT, Hutcheson R, Russo CJ. Cell phones, student rights, and school safety: finding the right balance. Can J Educ Adm Policy. Mar 11, 2021;(195):49-64. [ CrossRef ]
- Draaijer M, Lalla-Edward ST, Venter WD, Vos A. Phone calls to retain research participants and determinants of reachability in an African setting: observational study. JMIR Form Res. Sep 30, 2020;4(9):e19138. [ FREE Full text ] [ CrossRef ] [ Medline ]
- Mapham W. Mobile phones: changing health care one SMS at a time: opinion. South Afr J HIV Med. 2008;9(4):11-16. [ FREE Full text ]
- Shava H. The relationship between service quality and customer satisfaction in the South African mobile network telecommunications industry. J Int Stud. 2021;14(2):70-83. [ CrossRef ]
- Achampong EK, Keney G, Attah Sr NO. The effects of mobile phone use in clinical practice in cape coast teaching hospital. Online J Public Health Inform. Sep 21, 2018;10(2):e210. [ FREE Full text ] [ CrossRef ] [ Medline ]
- Saka B, Landoh DE, Patassi A, d'Almeida S, Singo A, Gessner BD, et al. Loss of HIV-infected patients on potent antiretroviral therapy programs in Togo: risk factors and the fate of these patients. Pan Afr Med J. May 26, 2013;15:35. [ FREE Full text ] [ CrossRef ] [ Medline ]
- Kouassi Kan V, Coly A, N'Guessan J, Dobe S, Agbo S, Zimin T, et al. Research report summary: factors associated with loss to follow-up status among ART patients in Cote d’Ivoire. University Research Co. Jan 2014. URL: https://pdf.usaid.gov/pdf_docs/PA00M6CM.pdf [accessed 2024-03-23]
- Crankshaw T, Corless IB, Giddy J, Nicholas PK, Eichbaum Q, Butler LM. Exploring the patterns of use and the feasibility of using cellular phones for clinic appointment reminders and adherence messages in an antiretroviral treatment clinic, Durban, South Africa. AIDS Patient Care STDS. Nov 10, 2010;24(11):729-734. [ CrossRef ] [ Medline ]
- Teague S, Youssef GJ, Macdonald JA, Sciberras E, Shatte A, Fuller-Tyszkiewicz M, et al. SEED Lifecourse Sciences Theme. Retention strategies in longitudinal cohort studies: a systematic review and meta-analysis. BMC Med Res Methodol. Nov 26, 2018;18(1):151. [ FREE Full text ] [ CrossRef ] [ Medline ]
- Norris SA, Draper CE, Prioreschi A, Smuts CM, Ware LJ, Dennis C, et al. Building knowledge, optimising physical and mental health and setting up healthier life trajectories in South African women (Bukhali): a preconception randomised control trial part of the Healthy Life Trajectories Initiative (HeLTI). BMJ Open. Apr 21, 2022;12(4):e059914. [ FREE Full text ] [ CrossRef ] [ Medline ]
- Draper CE, Mabena G, Motlhatlhedi M, Thwala N, Lawrence W, Weller S, et al. Implementation of Healthy conversation skills to support behaviour change in the Bukhali trial in Soweto, South Africa: a process evaluation. SSM Mental Health. Dec 2022;2:100132. [ FREE Full text ] [ CrossRef ]
- Draper CE, Thwala N, Slemming W, Lye SJ, Norris SA. Development, implementation, and process evaluation of Bukhali: an intervention from preconception to early childhood. Glob Implement Res Appl. Mar 11, 2023;3(1):31-43. [ FREE Full text ] [ CrossRef ] [ Medline ]
- Creswell JW, Creswell JD. Research Design: Qualitative, Quantitative, and Mixed Methods Approaches. Thousand Oaks, CA. Sage publications; 2017.
- Creswell JW, Plano Clark VL. Designing and Conducting Mixed Methods Research. California City, CA. Sage Publications; 2007.
- Tashakkori A, Teddlie C. Sage Handbook of Mixed Methods in Social & Behavioral Research. Thousand Oaks, CA. SAGE publications; 2021.
- Support vector machine (SVM). MAXQDA by VERBI Software. URL: https://www.maxqda.com/about [accessed 2024-03-16]
- Battersby J, McLachlan M. Urban food insecurity: a neglected public health challenge. S Afr Med J. Sep 04, 2013;103(10):716-717. [ CrossRef ] [ Medline ]
- Patridge EF, Bardyn TP. Research electronic data capture (REDCap). J Med Libr Assoc. Jan 02, 2018;106(1):142-144. [ CrossRef ]
- Pinzon E. Differences-in-differences in Stata 17. In: Proceedings of the 2021 London Stata Conference. 2021. Presented at: LSC '21; September 9-10, 2021; Virtual Event. URL: https://ideas.repec.org/p/boc/usug21/21.html
- Chauke H. Understanding the impact of free public WiFi hotspots using the choice framework. School of Economic and Business Sciences, University of the Witwatersrand. 2019. URL: https://wiredspace.wits.ac.za/server/api/core/bitstreams/4320356c-7da5-4e99-bb7c-5781f275ae9d/content [accessed 2024-03-16]
- Hilbert M. Digital gender divide or technologically empowered women in developing countries? A typical case of lies, damned lies, and statistics. Women's Stud Int Forum. Nov 2011;34(6):479-489. [ CrossRef ]
- Faith B. Maintenance affordances and structural inequalities: mobile phone use by low-income women in the United Kingdom. Inf Technol Int Dev. 2018.:66-80. [ FREE Full text ]
- Barboni G, Field E, Pande R, Rigol N, Schaner S, Moore CT. A tough call: understanding barriers to and impacts of women’s mobile phone adoption in India. Evidence for Policy Design, Harvard Kennedy School. 2018. URL: https://epod.cid.harvard.edu/sites/default/files/2018-10/A_Tough_Call.pdf [accessed 2024-03-16]
- Hampshire K, Porter G, Owusu SA, Mariwah S, Abane A, Robson E, et al. Informal m-health: how are young people using mobile phones to bridge healthcare gaps in Sub-Saharan Africa? Soc Sci Med. Oct 2015;142:90-99. [ FREE Full text ] [ CrossRef ] [ Medline ]
- Roessler P. The mobile phone revolution and digital inequality: scope, determinants and consequences. Pathways for Prosperity Commission. 2018. URL: https://pathwayscommission.bsg.ox.ac.uk/sites/default/files/2019-09/the_mobile_phone_revolution_and_digital_inequality.pdf [accessed 2024-03-16]
- Howard J. When kids get their first cell phones around the world. CNN Health. Dec 11, 2017. URL: https://edition.cnn.com/2017/12/11/health/cell-phones-for-kids-parenting-without-borders-explainer-intl/index.html [accessed 2024-03-16]
- Rahul P, Chander KR, Murugesan M, Anjappa AA, Parthasarathy R, Manjunatha N, et al. Accredited social health activist (ASHA) and her role in district mental health program: learnings from the COVID 19 pandemic. Community Ment Health J. Apr 2021;57(3):442-445. [ FREE Full text ] [ CrossRef ] [ Medline ]
- Poongothai S, Anjana RM, Aarthy R, Unnikrishnan R, Venkat Narayan KM, Ali MK, et al. Strategies for participant retention in long term clinical trials: a participant -centric approaches. Perspect Clin Res. Jan 2023;14(1):3-9. [ FREE Full text ] [ CrossRef ] [ Medline ]
Abbreviations
low- and middle-income country |
Research Electronic Data Capture |
Edited by A Mavragani; submitted 13.04.23; peer-reviewed by C Weerth, A Pandya, S Jalil; comments to author 10.10.23; revised version received 21.10.23; accepted 23.10.23; published 08.04.24.
©Khuthala Mabetha, Larske M Soepnel, Gugulethu Mabena, Molebogeng Motlhatlhedi, Lukhanyo Nyati, Shane A Norris, Catherine E Draper. Originally published in JMIR Formative Research (https://formative.jmir.org), 08.04.2024.
This is an open-access article distributed under the terms of the Creative Commons Attribution License (https://creativecommons.org/licenses/by/4.0/), which permits unrestricted use, distribution, and reproduction in any medium, provided the original work, first published in JMIR Formative Research, is properly cited. The complete bibliographic information, a link to the original publication on https://formative.jmir.org, as well as this copyright and license information must be included.

Epidemiological Study and Analysis of Factors Related to Skin Lesions Caused by Medical Disinfectants, and Personal Protective Equipment among Epidemic-Prevention Workers During the Severe Acute Respiratory Syndrome Coronavirus 2 Pandemic Lockdown Period
- Find this author on Google Scholar
- Find this author on PubMed
- Search for this author on this site
- ORCID record for Jing-Yi Hu
- For correspondence: [email protected]
- Info/History
- Preview PDF
Objective The aim of this study was to investigate the prevalence of and potential risk factors associated with skin lesions resulting from the use of medical disinfectants and personal protective equipment (PPE) among epidemic prevention workers (including healthcare professionals, temporary sampling site workers, community members and volunteers) during the Severe Acute Respiratory Syndrome Coronavirus 2 (SARS-CoV-2) pandemic lockdown period in China.
Methods We conducted a survey to investigate the prevalence and factors associated with skin lesions during SARS-CoV-2 pandemic lockdown period among epidemic prevention workers in Gaojing town of Baoshan distract, Shanghai, China.
Results A total of 1033 questionnaires were reviewed, with 995 deemed valid. Among the 995 respondents, 209 (21.01%) reported comorbidities, while 786 (78.99%) were considered as controls. Autoimmune diseases, family history of dermatitis, cardiovascular diseases (CVDs), palmar and plantar hyperhidrosis, allergic diseases and the total time spent on skin cleansing and antisepsis procedures were identified as independent risk factors for these s kin lesions.
Conclusion During the SARS-CoV-2 pandemic lockdown period, skin lesions among epidemic prevention workers was prevalent, which was primarily attributed to the use of medical disinfectants and PPE. These skin lesions frequently manifested as a combination of various subtypes across different areas of the body. Several individual factors, along with the total time spent on skin cleansing and skin antisepsis procedures, were identified as significant risk factors for the development of these skin lesions.
Competing Interest Statement
The authors have declared no competing interest.
Funding Statement
The author(s) received no specific funding for this work.
Author Declarations
I confirm all relevant ethical guidelines have been followed, and any necessary IRB and/or ethics committee approvals have been obtained.
The details of the IRB/oversight body that provided approval or exemption for the research described are given below:
Informed consent was obtained from all subjects involved in the study. This study was reviewed and approved by the Research Ethics Committee of Wusong Hospital, Zhongshan Hospital, Fudan University (IRB No.2022-SYY-15)
I confirm that all necessary patient/participant consent has been obtained and the appropriate institutional forms have been archived, and that any patient/participant/sample identifiers included were not known to anyone (e.g., hospital staff, patients or participants themselves) outside the research group so cannot be used to identify individuals.
I understand that all clinical trials and any other prospective interventional studies must be registered with an ICMJE-approved registry, such as ClinicalTrials.gov. I confirm that any such study reported in the manuscript has been registered and the trial registration ID is provided (note: if posting a prospective study registered retrospectively, please provide a statement in the trial ID field explaining why the study was not registered in advance).
I have followed all appropriate research reporting guidelines, such as any relevant EQUATOR Network research reporting checklist(s) and other pertinent material, if applicable.
Data Availability
All relevant data are within the manuscript and its Supporting Information files.
Abbreviations
View the discussion thread.
Thank you for your interest in spreading the word about medRxiv.
NOTE: Your email address is requested solely to identify you as the sender of this article.

Citation Manager Formats
- EndNote (tagged)
- EndNote 8 (xml)
- RefWorks Tagged
- Ref Manager
- Tweet Widget
- Facebook Like
- Google Plus One
Subject Area
- Dermatology
- Addiction Medicine (343)
- Allergy and Immunology (666)
- Anesthesia (180)
- Cardiovascular Medicine (2630)
- Dentistry and Oral Medicine (314)
- Dermatology (222)
- Emergency Medicine (397)
- Endocrinology (including Diabetes Mellitus and Metabolic Disease) (932)
- Epidemiology (12181)
- Forensic Medicine (10)
- Gastroenterology (756)
- Genetic and Genomic Medicine (4070)
- Geriatric Medicine (387)
- Health Economics (678)
- Health Informatics (2627)
- Health Policy (998)
- Health Systems and Quality Improvement (981)
- Hematology (361)
- HIV/AIDS (845)
- Infectious Diseases (except HIV/AIDS) (13662)
- Intensive Care and Critical Care Medicine (792)
- Medical Education (399)
- Medical Ethics (109)
- Nephrology (431)
- Neurology (3840)
- Nursing (209)
- Nutrition (571)
- Obstetrics and Gynecology (735)
- Occupational and Environmental Health (691)
- Oncology (2011)
- Ophthalmology (582)
- Orthopedics (239)
- Otolaryngology (304)
- Pain Medicine (250)
- Palliative Medicine (74)
- Pathology (471)
- Pediatrics (1109)
- Pharmacology and Therapeutics (460)
- Primary Care Research (448)
- Psychiatry and Clinical Psychology (3405)
- Public and Global Health (6504)
- Radiology and Imaging (1390)
- Rehabilitation Medicine and Physical Therapy (808)
- Respiratory Medicine (869)
- Rheumatology (401)
- Sexual and Reproductive Health (407)
- Sports Medicine (341)
- Surgery (441)
- Toxicology (52)
- Transplantation (185)
- Urology (165)
- Mathematical Sciences
- Sampling Methods
Sampling Methods in Research Methodology; How to Choose a Sampling Technique for Research
- January 2016
- SSRN Electronic Journal 5(2):18-27

- University Canada West
Abstract and Figures

Discover the world's research
- 25+ million members
- 160+ million publication pages
- 2.3+ billion citations
- Nonophela Buhle Mvakwendlu

- Samuel Agyapong
- Nicholas Oppong Mensah

- Frank Osei Tutu
- Scoffy N. Wangang

- T Hapuarachchi
- S Gunathilake
- Phil Johnson

- J.E. Barlett
- J. W. Kotrlik
- C. C. Higgins

- N.W.M. Ogink

- ORGAN RES METHODS
- J A Maxwell
- Recruit researchers
- Join for free
- Login Email Tip: Most researchers use their institutional email address as their ResearchGate login Password Forgot password? Keep me logged in Log in or Continue with Google Welcome back! Please log in. Email · Hint Tip: Most researchers use their institutional email address as their ResearchGate login Password Forgot password? Keep me logged in Log in or Continue with Google No account? Sign up

An official website of the United States government
Here's how you know
Official websites use .gov A .gov website belongs to an official government organization in the United States.
Secure .gov websites use HTTPS A lock ( ) or https:// means you’ve safely connected to the .gov website. Share sensitive information only on official, secure websites.
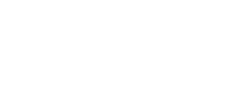
- Digg
Latest Earthquakes | Chat Share Social Media
Simulation modeling to assess line transect distance sampling under a range of translocation scenarios
The accuracy of posttranslocation population monitoring methods is critical to assessing long-term success in translocation programs. Translocation can produce unique challenges to monitoring efforts; therefore, it is important to understand the flexibility and robustness of commonly used monitoring methods. In Florida, USA, thousands of gopher tortoises Gopherus polyphemus have been, and continue to be, translocated from development sites to permitted recipient sites. These recipient sites create a broad range of potential monitoring scenarios due to variability in soft-release strategies, habitat conditions, and population demographics. Line transect distance sampling is an effective method for monitoring natural tortoise populations, but it is currently untested for translocated populations. We therefore produced 3,024 individual-based, spatially explicit scenarios of translocated tortoise populations that differed in recipient site and tortoise population properties, based on real-world examples, literature review, and expert opinion. We virtually sampled simulated tortoise populations by using line transect distance sampling methods and built a Bayesian hierarchical model to estimate the population density for each simulation, which incorporated individual-level covariates (i.e., burrow width and burrow occupancy). Line transect distance sampling was largely appropriate for the conditions that typify gopher tortoise recipient sites, particularly when detection probability on the transect lines was greater than or equal to 0.85. Designing the layout of transects relative to the orientation of soft-release pens, to avoid possible sampling biases that lead to extreme outliers in estimates of tortoise densities, resulted in more accurate population estimates. We also suggest that use of individual-level covariates, applied using a Bayesian framework as demonstrated in our study, may improve the applicability of line transect distance sampling surveys in a variety of contexts and that simulation can be a powerful tool for assessing survey design in complex sampling situations.
Citation Information
Publication Year | 2023 |
---|---|
Title | Simulation modeling to assess line transect distance sampling under a range of translocation scenarios |
DOI | |
Authors | Max D. Jones, Lora L. Smith, Katherine Gentry Richardson, J. Nicole DeSha, Traci Castellón, Dan Hipes, Alex Kalfin, Neal T. Halstead, Elizabeth Ann Hunter |
Publication Type | Article |
Publication Subtype | Journal Article |
Series Title | Journal of Fish and Wildlife Management |
Index ID | |
Record Source | |
USGS Organization | Coop Res Unit Leetown |
Related Content
Elizabeth a. hunter, phd, research ecologist.
Information
- Author Services
Initiatives
You are accessing a machine-readable page. In order to be human-readable, please install an RSS reader.
All articles published by MDPI are made immediately available worldwide under an open access license. No special permission is required to reuse all or part of the article published by MDPI, including figures and tables. For articles published under an open access Creative Common CC BY license, any part of the article may be reused without permission provided that the original article is clearly cited. For more information, please refer to https://www.mdpi.com/openaccess .
Feature papers represent the most advanced research with significant potential for high impact in the field. A Feature Paper should be a substantial original Article that involves several techniques or approaches, provides an outlook for future research directions and describes possible research applications.
Feature papers are submitted upon individual invitation or recommendation by the scientific editors and must receive positive feedback from the reviewers.
Editor’s Choice articles are based on recommendations by the scientific editors of MDPI journals from around the world. Editors select a small number of articles recently published in the journal that they believe will be particularly interesting to readers, or important in the respective research area. The aim is to provide a snapshot of some of the most exciting work published in the various research areas of the journal.
Original Submission Date Received: .
- Active Journals
- Find a Journal
- Proceedings Series
- For Authors
- For Reviewers
- For Editors
- For Librarians
- For Publishers
- For Societies
- For Conference Organizers
- Open Access Policy
- Institutional Open Access Program
- Special Issues Guidelines
- Editorial Process
- Research and Publication Ethics
- Article Processing Charges
- Testimonials
- Preprints.org
- SciProfiles
- Encyclopedia
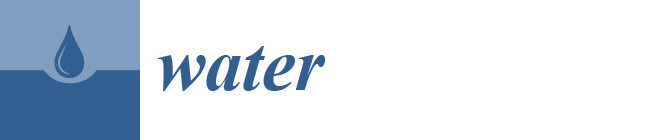
Article Menu
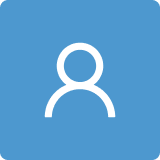
- Subscribe SciFeed
- Recommended Articles
- Google Scholar
- on Google Scholar
- Table of Contents
Find support for a specific problem in the support section of our website.
Please let us know what you think of our products and services.
Visit our dedicated information section to learn more about MDPI.
JSmol Viewer
Combining multiple machine learning methods based on cars algorithm to implement runoff simulation.
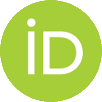
1. Introduction
2. methodology, 2.1. runoff forecasting models, 2.2. feature selection algorithm based on cars, 2.3. machine learning models, 2.3.1. gradient boosting decision tree (gbdt) model, 2.3.2. neural network models, 2.3.3. shallow machine learning models, 3. study area and data description, 3.1. study area, 3.2. data description, 4. data-driven runoff forecasting model based on cars, 4.1. identification of model order, 4.2. settings of model parameter, 4.3. performance evaluation metrics, 5. results and analysis, 5.1. overall forecasting performance evaluation, 5.2. effectiveness of flood forecasting for different events, 5.3. percentage analysis of duration curves for low, medium, and high flows, 5.4. analysis of model generalization ability, 6. conclusions, author contributions, data availability statement, conflicts of interest.
- Kan, G.; Hong, Y.; Liang, K. Flood Forecasting Research Based on Coupled Machine Learning Models. China Rural Water Hydrol. 2018 , 10 , 165–169, 176. (In Chinese) [ Google Scholar ]
- Kan, G. Study on Application and Comparative of Data-Driven Model and Semi-Data-Driven Model for Rainfall-Runoff Simulation. Acta Geod. Cartogr. Sin. 2017 , 46 , 265. (In Chinese) [ Google Scholar ]
- Liang, K.; Kan, G.; Li, Z. Application of A New Coupled Data-driven Model in Rainfall-Runoff Simulation. J. China Hydrol. 2016 , 36 , 1–7. (In Chinese) [ Google Scholar ]
- Beven, K.J.; Kirkby, M.J. A physically based, variable contributing area model of basin hydrology/Un modèle à base physique de zone d’appel variable de l’hydrologie du bassin versant. Hydrol. Sci. J. 1979 , 24 , 43–69. [ Google Scholar ] [ CrossRef ]
- Du, T.; Guo, M.; Zhang, J.; Tian, S. Hydrological simulation and application of VIC model in the Xijiang River Basin. Res. Soil Water Conserv. 2021 , 28 , 121–127. (In Chinese) [ Google Scholar ] [ CrossRef ]
- Zhao, A.Z.; Liu, X.F.; Zhu, X.F.; Pan, Y.Z.; Li, Y.Z. Spatial and temporal distribution of drought in the Weihe River Basin based on SWAT model. Prog. Geogr. 2015 , 34 , 1156–1166. (In Chinese) [ Google Scholar ]
- Baker, T.J.; Miller, S.N. Using the Soil and Water Assessment Tool (SWAT) to assess land use impact on water resources in an East African watershed. J. Hydrol. 2013 , 486 , 100–111. [ Google Scholar ] [ CrossRef ]
- Assaf, M.N.; Manenti, S.; Creaco, E.; Giudicianni, C.; Tamellini, L.; Todeschini, S. New optimization strategies for SWMM modeling of stormwater quality applications in urban area. J. Environ. Manag. 2024 , 361 , 121244. [ Google Scholar ] [ CrossRef ]
- Zhang, L.; Wang, H.; Guo, N.; Xu, Y.; Li, L.; Xie, J. Ensemble modeling of non-stationary runoff series based on time series decomposition and machine learning. Prog. Water Sci. 2023 , 34 , 42–52. (In Chinese) [ Google Scholar ]
- Li, B.; Tian, F.; Li, Y.; Ni, G. Deep learning hydrological model integrating spatial-temporal characteristics of meteorological elements. Prog. Water Sci. 2022 , 33 , 904–913. (In Chinese) [ Google Scholar ]
- Valipour, M. Long-term runoff study using SARIMA and ARIMA models in the United States. Meteorol. Appl. 2015 , 22 , 592–598. [ Google Scholar ] [ CrossRef ]
- Duan, Y.; Ren, L. Research on daily runoff prediction of the middle reaches of the Yellow River based on BP neural network. People’s Yellow River 2020 , 42 , 5–8. (In Chinese) [ Google Scholar ]
- Kratzert, F.; Klotz, D.; Brenner, C.; Schulz, K.; Herrnegger, M. Rainfall-runoff modelling using long short-term memory (LSTM) networks. Hydrol. Earth Syst. Sci. 2018 , 22 , 6005–6022. [ Google Scholar ] [ CrossRef ]
- Chiang, Y.-M.; Chang, F.-J. Integrating hydrometeorological information for rainfall-runoff modelling by artificial neural networks. Hydrol. Process. Int. J. 2009 , 23 , 1650–1659. [ Google Scholar ] [ CrossRef ]
- Kan, G.; Yao, C.; Li, Q.; Li, Z.; Yu, Z.; Liu, Z.; Ding, L.; He, X.; Liang, K. Improving event-based rainfall-runoff simulation using an ensemble artificial neural network based hybrid data-driven model. Stoch. Environ. Res. Risk Assess. 2015 , 29 , 1345–1370. [ Google Scholar ] [ CrossRef ]
- Guo, T.; Song, S.; Zhang, T.; Wang, H. A new step-by-step decomposition integrated runoff prediction model based on two-stage particle swarm optimization algorithm. J. Hydraul. Eng. 2022 , 53 , 1456–1466. (In Chinese) [ Google Scholar ]
- Xiong, Y.; Zhou, J.; Sun, N.; Zhang, J.; Zhu, S. Monthly runoff forecast based on adaptive variational mode decomposition and long short-term memory network. J. Hydraul. Eng. 2023 , 54 , 172–183, 198. (In Chinese) [ Google Scholar ]
- Li, C.; Jiao, Y.; Kan, G.; Fu, X.; Chai, F.; Yu, H.; Liang, K. Comparisons of Different Machine Learning-Based Rainfall–Runoff Simulations under Changing Environments. Water 2024 , 16 , 302. [ Google Scholar ] [ CrossRef ]
- Kan, G.; Li, J.; Zhang, X.; Ding, L.; He, X.; Liang, K.; Jiang, X.; Ren, M.; Li, H.; Wang, F.; et al. A new hybrid data-driven model for event-based rainfall–runoff simulation. Neural Comput. Appl. 2017 , 28 , 2519–2534. [ Google Scholar ] [ CrossRef ]
- Ding, Q.; Wang, Y.; Zhang, J.; Jia, K.; Huang, H. Joint estimation of soil moisture and organic matter content in saline-alkali farmland using the CARS algorithm. Chin. J. Appl. Ecol. 2024 , 35 , 1321–1330. [ Google Scholar ] [ CrossRef ]
- Wang, Y.; Ding, Q.; Zhang, J.; Chen, R.; Jia, K.; Li, X. Retrieval of soil water and salt information based on UAV hyperspectral remote sensing and machine learning. Appl. Ecol. J. 2023 , 34 , 3045–3052. [ Google Scholar ]
- Shang, T.; Chen, R.; Zhang, J.; Wang, Y. Estimation of soil organic matter content in Yinchuan Plain based on fractional differential combined spectral index. J. Appl. Ecol. 2023 , 34 , 717–725. [ Google Scholar ]
- Szczepanek, R. Daily Streamflow Forecasting in Mountainous Catchment Using XGBoost, LightGBM and CatBoost. Hydrology 2022 , 9 , 226. [ Google Scholar ] [ CrossRef ]
- Hao, R.; Bai, Z. Comparative Study for Daily Streamflow Simulation with Different Machine Learning Methods. Water 2023 , 15 , 1179. [ Google Scholar ] [ CrossRef ]
- Hochreiter, S.; Schmidhuber, J. Long short-term memory. Neural Comput. 1997 , 9 , 1735–1780. [ Google Scholar ] [ CrossRef ] [ PubMed ]
- Rumelhart, D.E.; Hinton, G.E.; Williams, R.J. Learning representations by back-propagating errors. Nature 1986 , 323 , 533–536. [ Google Scholar ] [ CrossRef ]
- Chang, C.-C.; Lin, C.-J. LIBSVM: A Library for Support Vector Machines. ACM Trans. Intell. Syst. Technol. 2011 , 2 , 27. [ Google Scholar ] [ CrossRef ]
- Shi, J.; Jin, S.; Hu, C. Analysis of peak flow characteristics change in the Bahe River Basin. Shaanxi Water Resour. 2023 , 58–61. [ Google Scholar ] [ CrossRef ]
- Ke, X.; Wang, N. Comparative study on runoff variation laws in typical north and south Qinling basins. J. Xi’an Univ. Technol. 2019 , 35 , 452–458. [ Google Scholar ] [ CrossRef ]
- Kotthoff, L.; Thornton, C.; Hoos, H.H.; Hutter, F.; Vanschoren, J. Automated Machine Learning: Methods, Systems, Challenges ; Springer: Cham, Switzerland, 2019. [ Google Scholar ]
- Pelikan, M.; Pelikan, M. Bayesian optimization algorithm. In Hierarchical Bayesian Optimization Algorithm: Toward a New Generation of Evolutionary Algorithms ; Springer: Berlin/Heidelberg, Germany, 2005; pp. 31–48. [ Google Scholar ]
- Gupta, H.V.; Kling, H.; Yilmaz, K.K.; Martinez, G.F. Decomposition of the mean squared error and NSE performance criteria: Implications for improving hydrological modelling. J. Hydrol. 2009 , 377 , 80–91. [ Google Scholar ] [ CrossRef ]
- Yilmaz, K.K.; Gupta, H.V.; Wagener, T. A process-based diagnostic approach to model evaluation. Application to the NWS distributed hydrologic model. Water Resour. Res. 2008 , 44 . [ Google Scholar ] [ CrossRef ]
- Fu, X.; Kan, G.; Liu, R.; Liang, K.; He, X.; Ding, L. Research on Rain Pattern Classification Based on Machine Learning: A Case Study in Pi River Basin. Water 2023 , 15 , 1570. [ Google Scholar ] [ CrossRef ]
Click here to enlarge figure
Model Name | Hyperparameter Name | Range | Optimal Value |
---|---|---|---|
CatBoost | Iterations | [50, 300] | 184 |
Learning rate | [0, 1] | 0.15 | |
Depth | [2, 15] | 8 | |
XGBoost | Iterations | [50, 300] | 280 |
Learning rate | [0, 1] | 0.15 | |
Depth | [2, 15] | 10 | |
LightGBM | Iterations | [50, 300] | 190 |
Learning rate | [0, 1] | 0.01 | |
Depth | [2, 15] | 10 | |
BP neural network | Number of neurons in the hidden layer 1 | [50, 300] | 96 |
Number of neurons in the hidden layer 2 | [50, 300] | 50 | |
Learning rate | [0, 1] | 0.0001 | |
Epochs | [50, 300] | 126 | |
LSTM neural network | Number of neurons in unit1 | [50, 300] | 32 |
Number of neurons in unit2 | [50, 300] | 59 | |
Learning rate | [0, 1] | 0.006 | |
Epochs | [50, 300] | 254 | |
SVR | C | [0.1, 100] | 10 |
kernel | [‘linear’, ‘rbf’, ‘poly’] | rbf | |
epsilon | [0.01, 0.2] | 0.01 | |
KNN | K | [1, 9] | 6 |
Forecast Period | Model Name | NSE | R | RMSE | %BiasFHV | %BiasFMS | %BiasFLV |
---|---|---|---|---|---|---|---|
T = 1 h | CatBoost | 0.9703 | 0.9391 | 19.55 | −0.75% | −2.36% | −44.75% |
XGBoost | 0.9796 | 0.9741 | 16.19 | −0.07% | 1.05% | −44.38% | |
LightGBM | 0.9661 | 0.9415 | 20.89 | −1.11% | −0.81% | −48.00% | |
LSTM | 0.9205 | 0.8853 | 32.01 | −18.63% | −25.80% | −43.43% | |
BP | 0.9689 | 0.9624 | 19.99 | −7.33% | −8.82% | −85.77% | |
KNN | 0.9680 | 0.9139 | 20.28 | −2.51% | −1.32% | 0.91% | |
SVR | 0.9965 | 0.9730 | 6.72 | −1.58% | 2.31% | −7.25% | |
T = 2 h | CatBoost | 0.9311 | 0.9092 | 29.77 | −0.75% | −2.55% | −53.05% |
XGBoost | 0.9361 | 0.9374 | 28.66 | 0.16% | 1.54% | −52.73% | |
LightGBM | 0.9407 | 0.8969 | 27.61 | −2.07% | 0.69% | −54.48% | |
LSTM | 0.9140 | 0.8802 | 33.27 | −14.17% | −24.35% | −59.03% | |
BP | 0.9466 | 0.9422 | 26.22 | −6.78% | −8.97% | −65.09% | |
KNN | 0.9419 | 0.8879 | 27.33 | −2.91% | −2.31% | 0.17% | |
SVR | 0.9874 | 0.9212 | 12.72 | −3.01% | 17.35% | 45.23% | |
T = 3 h | CatBoost | 0.8789 | 0.8921 | 39.47 | −0.75% | −2.30% | −55.05% |
XGBoost | 0.8832 | 0.9033 | 38.75 | −0.27% | 0.49% | −54.69% | |
LightGBM | 0.9011 | 0.8725 | 35.66 | −2.91% | −1.45% | −65.27% | |
LSTM | 0.8941 | 0.8780 | 36.93 | −11.86% | −17.70% | −68.20% | |
BP | 0.9019 | 0.9177 | 35.53 | −6.82% | −7.44% | −58.66% | |
KNN | 0.8996 | 0.8641 | 35.93 | −3.14% | −3.07% | −0.53% | |
SVR | 0.9707 | 0.8826 | 19.44 | −4.13% | 11.00% | 24.25% | |
T = 4 h | CatBoost | 0.8185 | 0.8836 | 48.32 | −0.75% | −3.53% | −44.77% |
XGBoost | 0.8162 | 0.8646 | 48.63 | −0.17% | 1.27% | −44.19% | |
LightGBM | 0.8507 | 0.8588 | 43.83 | −3.59% | −0.09% | −65.57% | |
LSTM | 0.8597 | 0.8715 | 42.55 | −9.85% | −6.85% | −83.26% | |
BP | 0.8659 | 0.8954 | 41.55 | −8.13% | −9.90% | −48.85% | |
KNN | 0.8469 | 0.8456 | 44.38 | −3.47% | −3.64% | −1.09% | |
SVR | 0.9474 | 0.8486 | 26.04 | −7.61% | 9.28% | 32.45% | |
T = 5 h | CatBoost | 0.7563 | 0.8766 | 55.99 | −0.79% | −3.79% | −61.98% |
XGBoost | 0.7522 | 0.8369 | 56.45 | −0.44% | 0.51% | −61.01% | |
LightGBM | 0.7954 | 0.8467 | 51.30 | −4.06% | −3.66% | −65.08% | |
LSTM | 0.8209 | 0.8586 | 48.13 | −6.92% | 14.62% | 13.90% | |
BP | 0.8221 | 0.8809 | 47.86 | −7.81% | −9.38% | −65.79% | |
KNN | 0.7881 | 0.8249 | 52.22 | −3.49% | −2.49% | −1.32% | |
SVR | 0.9004 | 0.8411 | 35.82 | −8.92% | 11.78% | 19.15% | |
T = 6 h | CatBoost | 0.6912 | 0.8718 | 63.03 | −0.77% | −3.17% | −56.11% |
XGBoost | 0.6874 | 0.8319 | 63.41 | −0.65% | 0.68% | −54.42% | |
LightGBM | 0.7373 | 0.8293 | 58.13 | −3.85% | −1.38% | −60.46% | |
LSTM | 0.7983 | 0.8471 | 51.04 | −7.38% | 4.83% | −28.25% | |
BP | 0.7654 | 0.8645 | 54.94 | −8.07% | −9.59% | −64.61% | |
KNN | 0.7274 | 0.8019 | 59.22 | −3.54% | −2.69% | −1.31% | |
SVR | 0.8496 | 0.8315 | 44.01 | −10.92% | 12.81% | 21.85% |
Flood Event Number | Model Name | 1 h | 3 h | 6 h |
---|---|---|---|---|
20090828 | svr | 0.30% | 1.96% | 2.65% |
knn | −4.60% | −2.14% | 7.98% | |
bp | 0.57% | 3.26% | 5.23% | |
lightGBM | −4.21% | −1.12% | 7.64% | |
lstm | 0.70% | 2.67% | 16.43% | |
Xgboost | −1.87% | −1.97% | 4.76% | |
catboost | −0.20% | 0.30% | 10.50% | |
20100820 | svr | −0.10% | 1.73% | 3.08% |
knn | 0.11% | 2.52% | 8.23% | |
bp | −1.75% | 2.65% | 3.21% | |
lightGBM | −2.32% | 0.50% | 3.36% | |
lstm | −1.35% | 3.60% | 23.36% | |
Xgboost | −0.90% | 3.25% | 4.90% | |
catboost | −3.30% | −3.05% | 11.50% |
Forecast Period | Model | Training | Validation | Testing |
---|---|---|---|---|
T = 1 h | CatBoost | 0.2341 | 0.4302 | 0.4021 |
XGBoost | 0.2366 | 0.2555 | 0.2099 | |
LightGBM | 0.3009 | 0.2862 | 0.3153 | |
LSTM | 0.4323 | 0.6051 | 0.5346 | |
BP | 0.2699 | 0.4682 | 0.2746 | |
KNN | 0.1983 | 0.3595 | 0.5575 | |
SVR | 0.0963 | 0.1182 | 0.0908 | |
T = 2 h | CatBoost | 0.4084 | 0.5407 | 0.4819 |
XGBoost | 0.4097 | 0.5568 | 0.3567 | |
LightGBM | 0.3904 | 0.4405 | 0.4245 | |
LSTM | 0.4385 | 0.7263 | 0.5439 | |
BP | 0.3597 | 0.5863 | 0.3523 | |
KNN | 0.3213 | 0.5329 | 0.6115 | |
SVR | 0.1816 | 0.2340 | 0.1689 | |
T = 3 h | CatBoost | 0.5589 | 0.7006 | 0.5647 |
XGBoost | 0.5598 | 0.6944 | 0.4878 | |
LightGBM | 0.4942 | 0.6297 | 0.5639 | |
LSTM | 0.4827 | 0.8591 | 0.5859 | |
BP | 0.4887 | 0.8054 | 0.4612 | |
KNN | 0.4554 | 0.7313 | 0.6878 | |
SVR | 0.2733 | 0.3907 | 0.2584 | |
T = 4 h | CatBoost | 0.6908 | 0.8687 | 0.6487 |
XGBoost | 0.6922 | 0.8745 | 0.6687 | |
LightGBM | 0.5990 | 0.8277 | 0.7015 | |
LSTM | 0.5552 | 1.0233 | 0.6548 | |
BP | 0.5767 | 0.9180 | 0.5296 | |
KNN | 0.5790 | 0.9202 | 0.7797 | |
SVR | 0.3651 | 0.5269 | 0.3487 | |
T = 5 h | CatBoost | 0.8029 | 1.0217 | 0.7268 |
XGBoost | 0.8045 | 0.9835 | 0.7907 | |
LightGBM | 0.6978 | 0.9894 | 0.8246 | |
LSTM | 0.6286 | 1.1786 | 0.7220 | |
BP | 0.6655 | 1.0571 | 0.6036 | |
KNN | 0.6865 | 1.1190 | 0.8743 | |
SVR | 0.4993 | 0.7531 | 0.4748 | |
T = 6 h | CatBoost | 0.9025 | 1.1709 | 0.8119 |
XGBoost | 0.9048 | 1.0853 | 0.8936 | |
LightGBM | 0.7976 | 1.1246 | 0.9009 | |
LSTM | 0.6660 | 1.2568 | 0.7629 | |
BP | 0.7662 | 1.2017 | 0.6904 | |
KNN | 0.7811 | 1.2928 | 0.9665 | |
SVR | 0.6132 | 0.9273 | 0.5832 |
The statements, opinions and data contained in all publications are solely those of the individual author(s) and contributor(s) and not of MDPI and/or the editor(s). MDPI and/or the editor(s) disclaim responsibility for any injury to people or property resulting from any ideas, methods, instructions or products referred to in the content. |
Share and Cite
Fan, Y.; Fu, X.; Kan, G.; Liang, K.; Yu, H. Combining Multiple Machine Learning Methods Based on CARS Algorithm to Implement Runoff Simulation. Water 2024 , 16 , 2397. https://doi.org/10.3390/w16172397
Fan Y, Fu X, Kan G, Liang K, Yu H. Combining Multiple Machine Learning Methods Based on CARS Algorithm to Implement Runoff Simulation. Water . 2024; 16(17):2397. https://doi.org/10.3390/w16172397
Fan, Yuyan, Xiaodi Fu, Guangyuan Kan, Ke Liang, and Haijun Yu. 2024. "Combining Multiple Machine Learning Methods Based on CARS Algorithm to Implement Runoff Simulation" Water 16, no. 17: 2397. https://doi.org/10.3390/w16172397
Article Metrics
Article access statistics, further information, mdpi initiatives, follow mdpi.
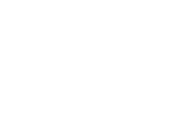
Subscribe to receive issue release notifications and newsletters from MDPI journals
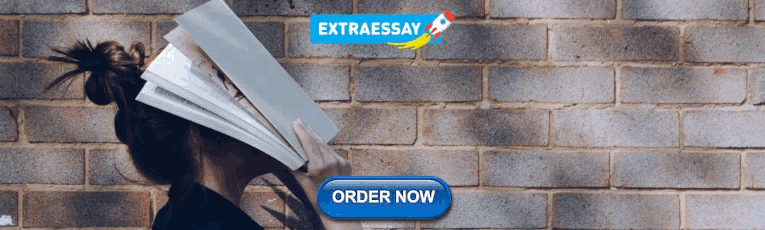
COMMENTS
2. Systematic sampling. Systematic sampling is similar to simple random sampling, but it is usually slightly easier to conduct. Every member of the population is listed with a number, but instead of randomly generating numbers, individuals are chosen at regular intervals. Example: Systematic sampling.
Psychology: Sampling methods are used in psychology research to study various aspects of human behavior and mental processes. For example, researchers may use stratified sampling to select a sample of participants that is representative of the population based on factors such as age, gender, and ethnicity.
Sampling methods or sampling techniques in research are statistical methods for selecting a sample representative of the whole population to study the population's characteristics. Sampling methods serve as invaluable tools for researchers, enabling the collection of meaningful data and facilitating analysis to identify distinctive features ...
Simple random sampling. Simple random sampling involves selecting participants in a completely random fashion, where each participant has an equal chance of being selected.Basically, this sampling method is the equivalent of pulling names out of a hat, except that you can do it digitally.For example, if you had a list of 500 people, you could use a random number generator to draw a list of 50 ...
Sampling methods in psychology refer to strategies used to select a subset of individuals (a sample) from a larger population, to study and draw inferences about the entire population. Common methods include random sampling, stratified sampling, cluster sampling, and convenience sampling. Proper sampling ensures representative, generalizable, and valid research results.
We could choose a sampling method based on whether we want to account for sampling bias; a random sampling method is often preferred over a non-random method for this reason. Random sampling examples include: simple, systematic, stratified, and cluster sampling. Non-random sampling methods are liable to bias, and common examples include ...
Abstract. Knowledge of sampling methods is essential to design quality research. Critical questions are provided to help researchers choose a sampling method. This article reviews probability and non-probability sampling methods, lists and defines specific sampling techniques, and provides pros and cons for consideration.
Sampling: The process and method of selecting your sample; Free eBook: 2024 Market Research Trends. Why is sampling important? Although the idea of sampling is easiest to understand when you think about a very large population, it makes sense to use sampling methods in research studies of all types and sizes.
Researchers often use non-probability sampling methods for exploratory research, pilot studies, and qualitative research. These sampling methods provide quick and rough assessments, help work kinks out of measurement instruments and procedures, and help refine the design for a more rigorous study in the future. Below are several standard non ...
When you use the sampling method, the whole population being studied is called the sampling frame. The sample you choose should represent your target market, or the sampling frame, well enough to do one of the following: ... Launch a pilot study. Do some initial qualitative research. Have little time or money available (half a loaf is better ...
The purpose of this section is to discuss various sampling methods used in research. After finalizing the research question and the research design, it is important to select the appropriate sample for the study. The method by which the researcher selects the sample is the "Sampling Method" [Figure 1].
Sampling is one of the most important factors which determines the accuracy of a study. This article review the sampling techniques used in research including Probability sampling techniques ...
Stratified random sample. Definition: Split a population into groups. Randomly select some members from each group to be in the sample. Example: Split up all students in a school according to their grade - freshman, sophomores, juniors, and seniors. Ask 50 students from each grade to complete a survey about the school lunches.
Sampling types. There are two major categories of sampling methods ( figure 1 ): 1; probability sampling methods where all subjects in the target population have equal chances to be selected in the sample [ 1, 2] and 2; non-probability sampling methods where the sample population is selected in a non-systematic process that does not guarantee ...
Sampling is a critical element of research design. Different methods can be used for sample selection to ensure that members of the study population reflect both the source and target populations, including probability and non-probability sampling. Power and sample size are used to determine the number of subjects needed to answer the research ...
In quantitative studies, the sampling plan, including sample size, is determined in detail in beforehand but qualitative research projects start with a broadly defined sampling plan. This plan enables you to include a variety of settings and situations and a variety of participants, including negative cases or extreme cases to obtain rich data.
Also, in the case of a small. sample set, a representation of the entire population is more likely to be compromised ( Bhardwaj, 2019; Sharma, 2017 ). 3.2. Systematic Sampling. Systematic sampling ...
Discover the different types of sampling methods in research: including probability and non-probability sampling methods. Learn about various sampling techniques, their applications, advantages, and limitations to enhance your study's accuracy and reliability. Perfect for market research professionals and data analysts.
Sometimes, the goal of research is to collect a little bit of data from a lot of people (e.g., an opinion poll). At other times, the goal is to collect a lot of information from just a few people (e.g., a user study or ethnographic interview). Either way, sampling allows researchers to ask participants more questions and to gather richer data ...
The main purpose of sampling in research is to make the research process doable. The research sample helps to reduce bias, accurately present the population and is cost-effective.
Field sampling of this study was conducted in 2022 in 18 plots in the Tuntang Estuary, located in six research sites that were determined by purposive random method sampling, based on various ...
Sampling without replacement is more commonly used in practical research because it mirrors real-world situations where, for example, a person cannot be surveyed twice in the same study. This method tends to reduce sampling variability and often provides more precise estimates of population parameters.
It reflects the population being studied because researchers are stratifying the entire population before applying random sampling methods. In short, it ensures each subgroup within the population ...
The essential topics related to the selection of participants for a health research are: 1) whether to work with samples or include the whole reference population in the study (census); 2) the sample basis; 3) the sampling process and 4) the potential effects nonrespondents might have on study results. We will refer to each of these aspects ...
A new study at CHR is testing an alternative to the Pap test for cervical cancer screening. CHR Investigator Amanda Petrik's new study, Self-Testing for Cervical Cancer in Priority Populations—the STEP-2 Trial, funded by the National Cancer Institute, seeks to increase cervical cancer screening rates in safety net clinics. Nearly 30 million people in the U.S. get their health care in ...
Background: The use of mobile technologies in fostering health promotion and healthy behaviors is becoming an increasingly common phenomenon in global health programs. Although mobile technologies have been effective in health promotion initiatives and follow-up research in higher-income countries and concerns have been raised within clinical practice and research in low- and middle-income ...
Objective: The aim of this study was to investigate the prevalence of and potential risk factors associated with skin lesions resulting from the use of medical disinfectants and personal protective equipment (PPE) among epidemic prevention workers (including healthcare professionals, temporary sampling site workers, community members and volunteers) during the Severe Acute Respiratory Syndrome ...
collect data from all cases. Thus, there is a need to select a sample. The entire set of cases from. which researcher sample is drawn in called the population. Since, researchers neither have time ...
The accuracy of posttranslocation population monitoring methods is critical to assessing long-term success in translocation programs. Translocation can produce unique challenges to monitoring efforts; therefore, it is important to understand the flexibility and robustness of commonly used monitoring methods. In Florida, USA, thousands of gopher tortoises Gopherus polyphemus have been, and continue
Runoff forecasting is crucial for water resource management and flood safety and remains a central research topic in hydrology. Recent advancements in machine learning provide novel approaches for predicting runoff. This study employs the Competitive Adaptive Reweighted Sampling (CARS) algorithm to integrate various machine learning models into a data-driven rainfall-runoff simulation model.