
- Data Science
Caltech Bootcamp / Blog / /
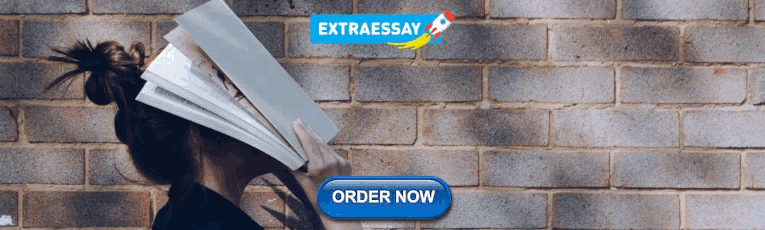
What is Data Visualization, and What is its Role in Data Science?
- Written by Karin Kelley
- Updated on July 29, 2024
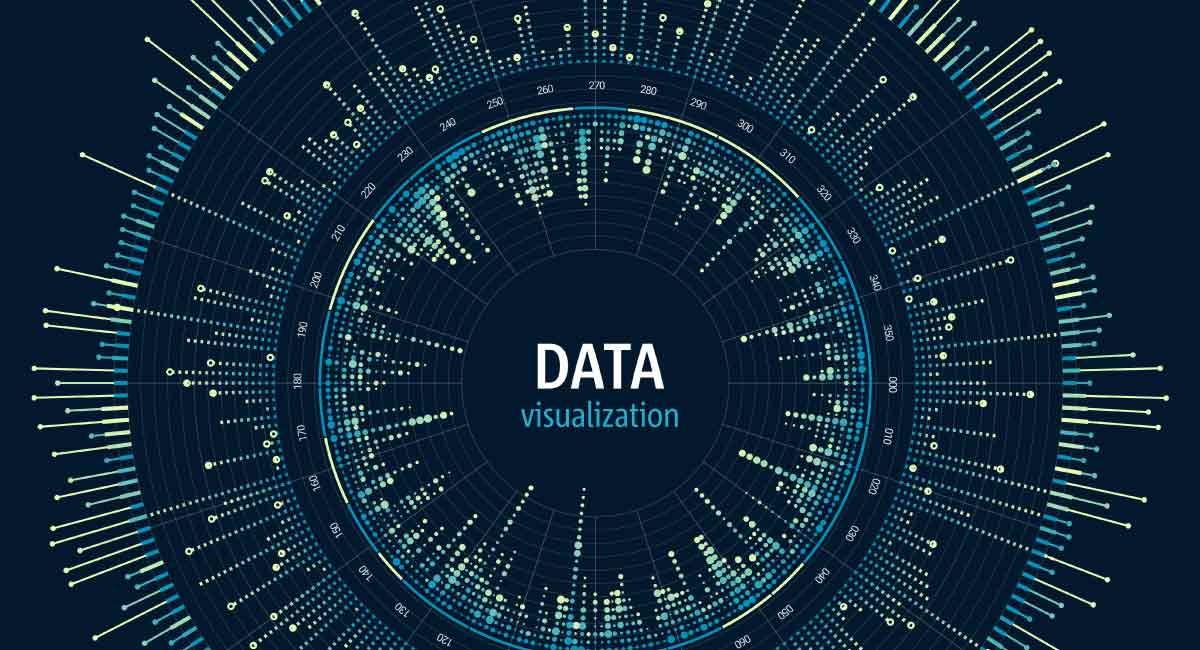
How do you transform endless rows of data into a story that can drive decisions and inspire action? Data visualization is the answer. In our data-driven world, converting complex data sets into intuitive, visual formats is essential for uncovering insights and making informed decisions.
This blog answers the high-level question: “What is data visualization?” and discusses its importance, various categories, techniques, and practical applications. We’ll explore how visualizing data can turn raw numbers into powerful narratives that inform, engage, and persuade. Those looking to master this skill and advance their data science career should consider enrolling in a comprehensive data science bootcamp .
So, What is Data Visualization?
Data visualization is the graphical representation of information and data. Using visual elements like charts, graphs, and maps, data visualization tools provide an accessible way to see and understand trends, outliers, and patterns in data. This visual context helps users understand the data’s insights and make data-driven decisions more effectively. It’s a powerful tool for exploratory data analysis and conveying findings to others.
Data visualization involves transforming raw data into visual formats that reveal patterns, trends, and correlations. This transformation process can range from simple static charts to highly interactive and complex visualizations. The goal is to make the data more understandable, insightful, and actionable. Effective data visualization leverages visual perception principles to present data in a way that is both aesthetically pleasing and informative.
It is not just about creating pretty pictures but about creating meaningful representations that can tell a story or answer a question. This process involves understanding the audience, selecting the appropriate visualization techniques, and presenting the data in a way that aligns with the intended message. For instance, a bar chart might be used to compare sales figures across different regions, while a line chart could illustrate trends in stock prices over time.
Also Read: Technology at Work: Data Science in Finance
Why is Data Visualization Important?
Data visualization is essential because it transforms complex data into clear and actionable insights. Converting raw numbers into visual formats allows for quick comprehension and informed decision-making.
Here are some key reasons why data visualization is so crucial.
- Unlocking the narrative: Data visualization helps uncover the story hidden within data, allowing decision-makers to grasp complex concepts and identify new patterns.
- Effective communication: It plays a vital role in presenting data findings clearly and concisely, ensuring that the message is easily understood.
- Handling big data : Visualization tools enable data scientists and analysts to interpret large data sets quickly and efficiently, driving better business strategies and operations.
- Immediate understanding: Humans are inherently visual creatures, and our brains process visual information more effectively than text or numbers alone. Visual representations allow us to quickly identify trends, spot outliers, and understand relationships within the data.
- Essential for quick decision-making: This immediate comprehension is particularly valuable in fast-paced environments where quick decision-making is crucial.
- Broadening audience reach : Data visualization makes complex data accessible and understandable to a broader audience, including business executives, policymakers, and laypersons.
- Democratizing data: By making data more accessible, visualization empowers more people to engage with and make informed decisions based on data.
What is Data Visualization? Big Data Visualization Categories
Big data visualization involves handling vast and complex data sets, often requiring advanced tools and techniques. It can be categorized into three main types:
Interactive Visualization
This allows users to engage with the data dynamically. Interactive dashboards and reports enable users to manipulate data and uncover insights through various filters and controls. Tools like Tableau and Power BI are commonly used to create interactive visualizations that allow users to drill down into data, explore different perspectives, and gain deeper insights.
Real-time Visualization
This involves visualizing data as it is collected. It’s particularly useful for monitoring live data streams, such as social media feeds, sensor data, or financial market movements. Real-time visualization tools can provide up-to-the-minute insights, enabling quick responses to changing conditions. For example, financial traders use real-time visualization to monitor market movements and make timely investment decisions.
3D Visualization
3D visualization can provide additional depth and clarity for particularly complex data sets. It’s often used in medical imaging, geospatial analysis, and engineering. 3D visualizations can help to understand complex structures and relationships that might be difficult to interpret in two dimensions. For example, 3D visualizations of MRI or CT scans in medical imaging can help doctors diagnose and plan treatments more effectively.
Also Read: The Top Data Science Interview Questions for 2024
Top Data Visualization Techniques
Numerous techniques are available for data visualization, each serving different purposes and data types. Here are some of the top ones.
Bar Charts and Column Charts
These compare different categories or track changes over time. They are simple yet effective for presenting categorical data. Bar charts can be used to compare sales figures across various regions, while column charts can show the change in sales over different quarters.
Line Charts
Line charts connect individual data points to show continuous data, which is ideal for showing trends over time. They are often used to illustrate trends in stock prices, website traffic, or temperature changes over time. Line charts can help to identify patterns and predict future trends.
Pie Charts and Donut Charts
These charts show the proportions of a whole. While popular, they should be used carefully as they can sometimes be misleading. Pie charts are best used for showing simple proportions, such as the market share of different companies. Donut charts are similar but have a central hole, making them visually distinct.
These visualize data through color variations. They effectively show data density and variations across different categories or geographical areas. Heat maps are often used in fields like marketing to show customer activity across various regions or in scientific research to show gene expression levels.
Scatter Plots
These display values for typically two variables for a data set, showing how much one variable is affected by another. Scatter plots help identify correlations and relationships between variables. For example, a scatter plot might show the relationship between advertising spend and sales revenue.
Bubble Charts
Similar to scatter plots, but with an added data dimension represented by the bubble size. Bubble charts can show the relationship between three variables, with the size of the bubble representing the third variable. They are often used in business to show the performance of different products or regions.
Geospatial Maps
Geospatial maps visualize data related to geographical locations and are useful in fields like meteorology, urban planning, and logistics. They can show the distribution of phenomena across different regions, such as the spread of diseases, population density, or delivery routes.
Also Read: Big Data and Analytics: Unlocking the Future
What is Data Visualization? Use Cases and Applications
Data visualization is used across various industries and applications, enhancing the ability to understand and utilize data effectively:
Business Intelligence
Companies use data visualization for performance tracking, market analysis, and strategic planning. Tableau and Power BI are popular for creating interactive dashboards and reports. These tools enable businesses to monitor key performance indicators (KPIs), track sales performance, and analyze real-time market trends. For example, a retail company might use a dashboard to monitor daily sales figures, inventory levels, and customer feedback.
Visualization helps understand patient data, track disease outbreaks, and optimize healthcare operations. For example, heat maps can show the spread of diseases geographically, allowing public health officials to allocate resources effectively. In hospitals, data visualization can be used to monitor patient vitals, track the progress of treatments, and identify potential complications early.
In finance, visualization aids in tracking stock market trends, risk management, and portfolio analysis. Real-time visualization tools are crucial for making timely investment decisions. For example, traders use real-time charts to monitor stock prices, identify trends, and execute trades. Risk managers use visualizations to assess portfolios’ risk exposure and develop mitigation strategies.
Marketers use visualization to analyze consumer behavior, campaign performance, and market trends. Interactive dashboards can show the impact of marketing efforts in real time. For example, a marketing team might use a dashboard to track the performance of different campaigns, analyze customer engagement, and optimize marketing strategies based on data insights.
Data visualization improves teaching methods, tracks student performance, and research trends. Educational institutions use visual tools to analyze and present data on student outcomes. For example, schools use dashboards to monitor student attendance, track academic performance, and identify students who need additional support. Researchers use data visualization to analyze education trends and develop evidence-based policies.
Scientific Research
Scientists use data visualization to interpret complex data sets from experiments and simulations. 3D visualizations can provide in-depth insights into scientific phenomena. For example, climate scientists use visualizations to analyze data from climate models, track changes in temperature and precipitation patterns, and predict future climate scenarios. Biologists use visualizations to analyze gene expression data and understand diseases’ underlying mechanisms.
Government and Public Policy
Governments use data visualization to analyze population data, economic indicators, and public health information. This aids in policy-making and public communication. For example, governments use visualizations to track the spread of COVID-19, monitor economic performance, and allocate resources. Policymakers use data visualization to analyze different policies’ impact and communicate findings to the public.
Also Read: Five Outstanding Data Visualization Examples for Marketing
Building Data Science and Data Visualization Skills
Data visualization is more than just a way to present data; it’s a crucial tool for making sense of complex information and driving informed decision-making across various sectors. Aspiring data scientists and analysts should prioritize gaining data visualization expertise to unlock their data’s full potential. Enrolling in a comprehensive data science program can provide the necessary skills and knowledge to excel in this field.
As data continues to grow in volume and complexity, the importance of data visualization will only increase, making it a vital skill for the future. By harnessing the power of data visualization, individuals and organizations can turn data into actionable insights, drive better decisions, and achieve their goals. Whether you’re working in business, healthcare, finance, education, scientific research, or government, data visualization can help you understand and leverage the power of data.
You might also like to read:
Data Science Bootcamps vs. Traditional Degrees: Which Learning Path to Choose?
Data Scientist vs. Machine Learning Engineer
What is A/B Testing in Data Science?
What is Natural Language Generation in Data Science, and Why Does It Matter?
What is Exploratory Data Analysis? Types, Tools, Importance, etc.
Data Science Bootcamp
- Learning Format:
Online Bootcamp
Leave a comment cancel reply.
Your email address will not be published. Required fields are marked *
Save my name, email, and website in this browser for the next time I comment.
Recommended Articles
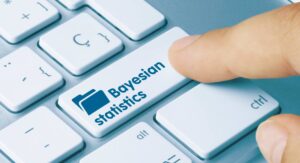
What is Bayesian Statistics, and How Does it Differ from Classical Methods?
What is Bayesian statistics? Learn about this tool used in data science, its fundamentals, uses, and advantages.
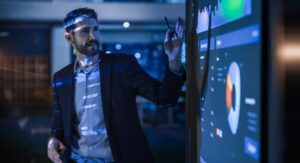
What is Data Imputation, and How Can You Use it to Handle Missing Data?
This article defines data imputation and demonstrates its importance, techniques, and challenges.
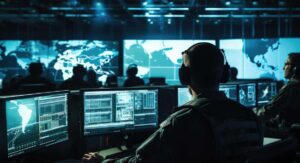
What is Data Governance, How Does it Work, Who Performs it, and Why is it Essential?
What is data governance? This article explores its goals and components, how to implement it, best practices, and more.
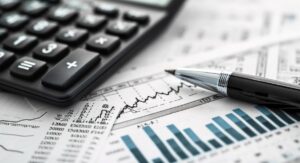
Technology at Work: Data Science in Finance
In today’s data-driven world, industries leverage advanced data analytics and AI-powered tools to improve services and their bottom line. The financial services industry is at the forefront of this innovation. This blog discusses data science in finance, including how companies use it, the skills required to leverage it, and more.
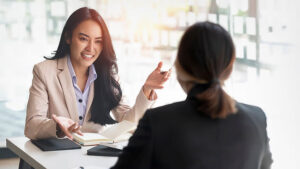
The Top Data Science Interview Questions for 2024
This article covers popular basic and advanced data science interview questions and the difference between data analytics and data science.
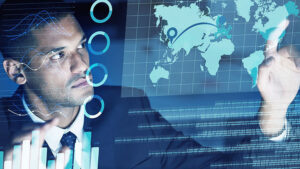
Big Data and Analytics: Unlocking the Future
Unlock the potential and benefits of big data and analytics in your career. Explore essential roles and discover the advantages of data-driven decision-making.
Learning Format
Program Benefits
- 12+ tools covered, 25+ hands-on projects
- Masterclasses by distinguished Caltech CTME instructors
- Caltech CTME Circle Membership
- Industry-specific training from global experts
- Call us on : 1800-212-7688
arXiv's Accessibility Forum starts next month!
Help | Advanced Search
Quantitative Biology > Neurons and Cognition
Title: universal dimensions of visual representation.
Abstract: Do neural network models of vision learn brain-aligned representations because they share architectural constraints and task objectives with biological vision or because they learn universal features of natural image processing? We characterized the universality of hundreds of thousands of representational dimensions from visual neural networks with varied construction. We found that networks with varied architectures and task objectives learn to represent natural images using a shared set of latent dimensions, despite appearing highly distinct at a surface level. Next, by comparing these networks with human brain representations measured with fMRI, we found that the most brain-aligned representations in neural networks are those that are universal and independent of a network's specific characteristics. Remarkably, each network can be reduced to fewer than ten of its most universal dimensions with little impact on its representational similarity to the human brain. These results suggest that the underlying similarities between artificial and biological vision are primarily governed by a core set of universal image representations that are convergently learned by diverse systems.
Subjects: | Neurons and Cognition (q-bio.NC); Computer Vision and Pattern Recognition (cs.CV) |
Cite as: | [q-bio.NC] |
(or [q-bio.NC] for this version) | |
Focus to learn more arXiv-issued DOI via DataCite |
Submission history
Access paper:.
- HTML (experimental)
- Other Formats

References & Citations
- Google Scholar
- Semantic Scholar
BibTeX formatted citation

Bibliographic and Citation Tools
Code, data and media associated with this article, recommenders and search tools.
- Institution
arXivLabs: experimental projects with community collaborators
arXivLabs is a framework that allows collaborators to develop and share new arXiv features directly on our website.
Both individuals and organizations that work with arXivLabs have embraced and accepted our values of openness, community, excellence, and user data privacy. arXiv is committed to these values and only works with partners that adhere to them.
Have an idea for a project that will add value for arXiv's community? Learn more about arXivLabs .
- Neuroscience
Neural dynamics of visual working memory representation during sensory distraction
- Jonas Karolis Degutis author has email address
Simon Weber
John-dylan haynes.
- Bernstein Center for Computational Neuroscience Berlin and Berlin Center for Advanced Neuroimaging, Charité Universitätsmedizin Berlin, corporate member of the Freie Universität Berlin, Humboldt-Universität zu Berlin, and Berlin Institute of Health, Berlin, Germany
- Max Planck School of Cognition, Leipzig, Germany
- Department of Psychology, Humboldt-Universität zu Berlin, Berlin, Germany
- Research Training Group “Extrospection” and Berlin School of Mind and Brain, Humboldt-Universität zu Berlin, Berlin, Germany
- Institute of Psychology, Otto von Guericke University, Mageburg, Germany
- Max Planck Institute for Human Cognitive and Brain Sciences, Leipzig, Germany
- German Center for Neurodegenerative Diseases, Göttingen, Germany
- Research Cluster of Excellence “Science of Intelligence”, Technische Universität Berlin, Berlin, Germany
- Collaborative Research Center “Volition and Cognitive Control”, Technische Universität Dresden, Dresden, Germany
- https://doi.org/ 10.7554/eLife.99290.1
- Open access
- Copyright information
Recent studies have provided evidence for the concurrent encoding of sensory percepts and visual working memory contents (VWM) across visual areas; however, it has remained unclear how these two types of representations are concurrently present. Here, we reanalyzed an open-access fMRI dataset where participants memorized a sensory stimulus while simultaneously being presented with sensory distractors. First, we found that the VWM code in several visual regions did not generalize well between different time points, suggesting a dynamic code. A more detailed analysis revealed that this was due to shifts in coding spaces across time. Second, we collapsed neural signals across time to assess the degree of interference between VWM contents and sensory distractors, specifically by testing the alignment of their encoding spaces. We find that VWM and feature-matching sensory distractors are encoded in separable coding spaces. Together, these results indicate a role of dynamic coding and temporally stable coding spaces in helping multiplex perception and VWM within visual areas.
eLife assessment
This useful study reports a reanalysis of one experiment of a previously published report to characterize the dynamics of neural population codes during visual working memory in the presence of distracting information. The evidence supporting the claims of dynamic codes is incomplete , as only a subset of the original data is analyzed, there is only modest evidence for dynamic coding in the results, and the result might be affected by the signal-to-noise ratio. This research will be of interest to cognitive neuroscientists working on the neural bases of visual perception and memory.
- https://doi.org/ 10.7554/eLife.99290.1.sa2
- Read the peer reviews
- About eLife assessments
Introduction
To successfully achieve behavioral goals, humans rely on the ability to remember, update, and ignore information. Visual working memory (VWM) allows for a brief maintenance of visual stimuli that are no longer present within the environment ( 1 – 3 ). Previous studies have revealed that the contents of VWM are present throughout multiple visual areas, starting from V1 ( 4 – 12 ). These findings raised the question of how areas that are primarily involved in visual perception can also maintain VWM information without interference between the two contents. Recent studies that had participants remember a stimulus while simultaneously being presented with sensory stimuli during the delay period have found supporting evidence that both VWM contents and sensory percepts are multiplexed in occipital and parietal regions ( 8 , 13 , 14 ). However, the mechanism employed in order to segregate bottom-up visual input from VWM contents remains poorly understood.
One proposed mechanism to achieve the separation between sensory and memory representations is dynamic coding ( 15 – 17 ): the change of the population code encoding VWM representations across time. Recent work has shown that the format of VWM might not be as persistent and stable throughout the delay as previously thought ( 18 , 19 ). Frontal regions display dynamic population coding across the delay during the maintenance of category ( 20 ) and spatial contents in the absence of interference ( 21 , 22 ), and also shows dynamic recoding of the memoranda after sensory distraction ( 23 , 24 ). The visual cortex in humans displays dynamic coding of contents during high load trials ( 25 ) and during a spatial VWM task ( 26 ). However, it is not yet clear whether dynamic coding of VWM might help evade sensory distraction in human visual areas.
Another line of evidence suggests that perception could potentially be segregated from VWM representations using stable non-overlapping coding spaces ( 27 ). For example, evidence from neuroanatomy indicates that the sensory bottom-up visual pathway primarily projects to the cytoarchitectonic Layer 4 in V1, while feedback projections culminate in superficial and deep layers of the cortex ( 28 ). Functional results are in line with neuroanatomy by showing that VWM signals preferentially activate the superficial and deep layers in humans ( 29 ) and non-human primates ( 30 ), while perceptual signals are more prevalent in the middle layers ( 31 ). In addition to laminar separation, regional multiplexing of multiple items could potentially rely on rotated representations, as seen in memory and sensory representations orthogonally coded in the auditory cortex ( 32 ) and in the storage of a sequence of multiple spatial locations in the prefrontal cortex (PFC) ( 33 ). Non-overlapping orthogonal representations have also been seen in both humans and trained recurrent neural networks as a way of segregating attended and unattended VWM representations ( 34 – 36 ).
Here we investigated whether the concurrent presence of VWM and sensory information is compatible with predictions offered by dynamic coding or by stable non-aligned coding spaces. For this, we reanalyzed an open-access fMRI dataset by Rademaker et al. ( 8 ) where participants performed a delayed-estimation VWM task with and without sensory distraction. To investigate dynamic coding we employed a temporal cross-decoding analysis that assessed how well the multivariate code encoding VWM generalizes from one time point to another ( 22 , 37 – 39 ), and a temporal neural subspace analysis that examined a sensitive way of looking at alignment of neural populations coding for VWM at different time points. To assess the non-overlapping coding hypothesis, we used neural subspaces ( 21 , 26 , 32 ) to see whether temporally stable representations of the VWM target and the sensory distractor are coded in separable neural populations. Finally, we examined the multivariate VWM code changes during distractor trials when compared to the no-distractor VWM format.
Temporal cross-decoding in distractor and no-distractor trials
In the previously published study ( 8 ) participants completed a VWM task where on a given trial they were asked to remember an orientation of a grating, which they had to then recall at the end of the trial. The delay period was either left blank (no-distractor) or a noise or randomly oriented grating distractor was presented ( Fig. 1a ). To investigate the dynamics of the VWM code, we examined how the multivariate pattern of activity encoding VWM memoranda changed across the duration of the delay period. To do so, we ran a temporal cross-decoding analysis where we trained a decoder (periodic support vector regression, see ( 40 )) on the target orientation, separately for each time point and tested on all time points in turn in a cross-validated fashion. If the information encoding VWM memoranda were to have the same code, the trained decoder would generalize to other time points, indicated by similar decoding accuracies on the diagonal and off-diagonal elements of the matrix. However, if the code exhibited dynamic properties, despite information about the memonda being present (above-chance decoding on the diagonal of the matrix), both off-diagonal elements corresponding to a given on-diagonal element would have lower decoding accuracies ( Fig. 1b ). Such off-diagonal elements are considered an indication of a dynamic code.

Task and temporal cross-decoding.
a) On each trial an oriented grating was presented for the 0.5 s followed by a delay period of 13 s ( 8 ). In a third of the trials a noise distractor was presented for 11 s during the middle of the delay; in another third another orientation grating was presented; one third of trials had no distractor during the delay. b) Illustration of dynamic coding elements. An off-diagonal element had to have a lower decoding accuracy compared to both corresponding diagonal elements (see Methods for details). c) Temporal generalization of the multivariate code encoding VWM representations in three conditions across occipital and parietal regions. Across-participant mean temporal cross-decoding of no-distractor trials. Black outlines: matrix elements showing above-chance decoding (cluster-based permutation test; p < 0.05). Blue outlines with dots: dynamic coding elements; parts of the cross-decoding matrix where the multivariate code fails to generalize (off-diagonal elements having lower decoding accuracy than their corresponding two diagonal elements; conjunction between two cluster-based permutation tests; p < 0.05). d) Same as c), but noise distractor trials. e) Same as c), but orientation distractor trials. f) Dynamicism index; the proportion of dynamic coding elements across time. High values indicate a dynamic non-generalizing code, while low values indicate a generalizing code. Time indicates the time elapsed since the onset of the delay period.
We ran the temporal cross-decoding analysis for the three VWM delay conditions: no-distractor, noise distractor and orientation distractor (feature-matching distractor). First, we examined each element of the cross-decoding matrix to test whether decoding accuracies were above chance. In all three conditions and throughout all ROIs, we found clusters where decoding was above chance ( Fig. 1c-e , black outline; nonparametric cluster-permutation test against null; all clusters p < 0.05) from as early as 4 s after the onset of the delay period. We found that decoding on the diagonal was highest during no-distractor compared to noise and orientation distractor trials in most regions of interest (ROI; Fig. 4a ).
Second, we examined off-diagonal elements to assess whether there was any indication that they reflected a non-generalizing dynamic code (see Methods for full details). Despite a high degree of temporal generalization, we found dynamic coding clusters in all three conditions. Some degree of dynamic coding was observed in all ROIs but LO2 in the noise distractor and no-distractor trials, while it was only present in V1, V2, V3, V4, and IPS in the orientation distractor condition ( Fig. 1c-e , blue outline). The difference between noise and orientation distractor conditions could not be explained by the amount of information present in each ROI, as the decoding accuracy of the diagonal was similar across all ROIs in both the noise and orientation distractor conditions ( Fig. 4a ). We saw a nominally larger number of dynamic coding elements in V1, V2 and V3AB during the noise distractor condition and in V3 during the no-distractor condition ( Fig. 1d ).
To qualitatively compare the amount of dynamic coding in the three conditions across the delay period, we calculated a dynamicism index ( 22 ) ( Fig. 1e ; see Methods), which measured the multivariate code’s uniqueness at each time point; more precisely, the proportion of dynamic elements corresponding to each diagonal element. High values indicate dynamic code and low values indicate a generalizing code. Across all conditions, most dynamic elements occurred between the encoding and early delay periods (4-8 s), and the late delay and retrieval (14.4-16.8 s). Interestingly, during the noise distractor trials in V1 we also saw dynamic coding during the middle of the delay period; the multivariate code not only changed during the onset and offset of the noise stimulus, but also during its presentation and throughout the extent of the delay.
Dynamics of VWM neural subspaces across time
The temporal cross-decoding analysis revealed more dynamic coding in the early visual cortex primarily during the early and late delay phase and a more generalized coding throughout the delay in higher-order regions. In order to understand the nature of these effects in more detail, we conducted a separate series of analyses that directly assessed the neural subspaces in which the orientations were encoded and how these potentially changed across time. Specifically, we followed a previous methodological framework ( 26 ) and applied a principal component analysis (PCA) to the high-dimensional activity patterns at each time point to identify the two axes that explained maximal variance across orientations (see Fig. 2 and Methods).

Assessing the dynamics of neural subspaces in V1-V3AB.
a) Schematic illustration of the neural subspace analysis. A given data matrix (voxels x orientation bins) was subjected to a principal components analysis and the first two dimensions were used to define a neural subspace onto which a left-out test data matrix was projected. This resulted in a matrix of two coordinates for each orientation bin and was visualized (see right). The x and y axes indicate the first two principal components. Each color depicts an angular bin. b) Schematic illustration of the calculation of an above-baseline principal angle (aPA). A principal angle (PA) is the angle between the 2D PCA-based neural subspaces (as in a ) for two different time points t 1 , t 2 . A small angle would indicate alignment of coding spaces; an angle of above-baseline would indicate a shift in the coding space. The above-baseline principle angle (aPA) is the angle for a comparison between two time points (t 1 , t 2 ) minus the angle between cross-validated pairs of the same time points. c) Each row shows a projection that was estimated for one of two time ranges (middle and late delay) and then applied to all time points (using independent, split-half cross-validated data). Opacity increases from early to late time points. For visualization purposes the subspaces were estimated on a participant-aggregated ROI ( 26 ). Fig. S1 depicts the same projections as neural trajectories. d) aPA between all pairwise time point comparisons (nonparametric permutation test against null; FDR-corrected p < 0.05) averaged across 1,000 split-half iterations. Corresponding p -values found in Supplementary Table 1 .
First, we visualized the consistency of the neural subspaces across time. For this, we computed low-dimensional 2D neural subspaces for a given time point and projected left-out data from six time points during the delay onto this subspace ( 26 , 32 ). A projection of data from a single time point resulted in four orientation bin values placed within the subspace ( Fig. 2a , colored circles indicate orientation). Taking into account projected data from all timepoints, if the VWM code were generalizing, we would see a clustering of orientation points in a subspace; however, if orientation points were scattered around the neural subspace, this would show a non-generalizing code.
We examined the projections in a combined ROI spanning V1-V3AB aggregated across participants. We projected left-out data from all six time point bins onto subspaces generated from the early (7.2 s), middle (12 s), and late (16.8 s) time point data for each of the three conditions. Overall, the results showed generalization across time with some exceptions ( Fig. 2c , Fig. S1 ). The clustering of orientation bins in the no-distractor condition was most pronounced ( Fig. 4a ). In contrast, the noise distractor trials showed a resemblance of some degree of dynamic coding, as seen by less variance explained by early time points projected onto the middle subspace and the early and middle time points projected onto late subspace ( Fig. 2c , Fig. S1 ).
To quantify the visualized changes, we measured the alignment between each pair of subspaces by calculating the above-baseline principal angle ( Fig. 2b ) within the combined V1-V3AB ROI. The above-baseline principal angle (aPA) measures the alignment between the 2D subspaces encoding the VWM representations: the higher the angle, the smaller the alignment between two subspaces and an indication of a changed neural coding space. Unlike in the projection of data from time points, the aPA was calculated participant-wise. Using a split-half approach, we measured the aPA between each split-pair of subspaces and subtracted the angles measured within each of the subspaces with the latter acting as a null baseline.
All three conditions showed significant aPAs ( Fig. 2d ; cyan stars; permutation test; p < 0.05, FDR-corrected). Corresponding to the results from the cross-decoding analysis, the early (4.8s) and late (16.8) delay subspaces showed the highest number of significant pairwise aPAs in all conditions, with noise distractor trials having all pairwise aPAs including the early and late subspaces being significant. The three conditions each had two significant aPAs between timepoints in the middle of the delay period.
Alignment between distractor and target subspaces in orientation distractor trials
Next, we assessed any similarity in encoding between the memorized orientation targets and the orientation distractors by focusing on those trials where both occurred. First, we examined whether the encoding of the sensory distractor is stable across its entire presentation duration (1.5 s - 12.5 s after target onset) using the same approach as for the VWM target ( Fig. 1e ). We found stable coding of the distractor in all ROIs with only a few dynamic elements in V2 and V3 ( Fig. 3a , Fig. S2 ). We then assessed whether the sensory distractor had a similar code to the VWM target by examining whether the multivariate code across time generalizes from the target to the distractor and vice versa. When cross-decoded, the sensory distractor ( Fig. 3c ) and target orientation ( Fig. 3b ) had lower decoding accuracies in the early visual cortex compared to when trained and tested on the same label-type, indicative of a non-generalizing code. Such a difference was not seen in higher-order visual regions, as the decoding of the sensory distractor was low to begin with ( Fig. S2 ).

Generalization between target and distractor codes in orientation distractor VWM trials in V1-V3AB.
a) Across-participant mean temporal cross-decoding of the sensory distractor. Black outlines: matrix elements showing above-chance decoding (cluster-based permutation test; p < 0.05). Blue outlines with dots: dynamic coding element (conjunction between two cluster-based permutation tests; p < 0.05). b) Same as a), but the decoder was trained on the target and tested on the sensory distractor in orientation VWM trials. c) Same as a), but trained on the sensory distractor and tested on the target. See Fig. S2 for ROIs from V4-LO2. d) Left: projection of left-out target (green) and sensory distractor (gray) onto an orientation VWM target neural subspace. Right: same as left, but the projections are onto the sensory distractor subspace. e) Principal angle between the sensory distractor and orientation VWM target subspaces ( p = 0.0297, one-tailed permutation test of sample mean). Average across 1,000 split-half iterations. Errorbars indicate ± SEM across participants.
Since we found minimal dynamics in the encoding of the distractor ( Fig. 3a ) and target ( Fig. 1e ), we focused on temporally stable neural subspaces that encoded the target and sensory distractor. We computed stable neural subspaces where we disregarded the temporal variance by averaging across the whole delay period and binned the trials either based on the target orientation ( Fig. 3d , left subpanel) or the distractor orientation ( Fig. 3d , right subpanel). We then projected left-out data binned based on the target ( Fig. 3d , green quadrilateral) or the distractor ( Fig. 3d , gray quadrilateral). This projection provided us with both a baseline (as when training and testing on the same label) and a cross-generalization. Unsurprisingly, the target subspace explained the left-out target data well ( Fig. 3d , left subpanel, green quadrilateral); however, the target subspace explained less variance of the left-out distractor data ( Fig. 3d , left subpanel, gray quadrilateral), as qualitatively seen from the smaller spread of the sensory distractor orientations. A similar but less pronounced dissociation between projections was seen in the distractor subspace ( Fig. 3d , left, quadrilateral in green) with the distractor subspace better explaining the left-out distractor data. We quantified the difference between the target and distractor subspaces and found a significant aPA between them ( p = 0.0297, one-tailed nonparametric permutation test; Fig. 3e ). These results provide evidence for the presence of separable stable neural subspaces that might enable the multiplexing of VWM and perception across the extent of the delay period.
Impact of distractors on VWM multivariate code
To further assess the impact of distractors on the available VWM information, we examined the decoding accuracies of distractor and no-distractor trials across time. Decoding accuracy was higher in the no-distractor trials compared to both orientation and noise distractor trials across all ROIs, but IPS ( Fig. 4a, red and blue lines , p < 0.05, cluster permutation test) across several stages of the delay period. To further assess how distractors affected the delay period information, we increased sensitivity by collapsing across it, because time courses were comparable in all conditions ( Fig. 4a ). To assess to which degree VWM encoding generalized from no-distractor to distractor trials, we trained a decoder on no-distractor trials and tested it on both types of distractor trials ( Fig. 4b noise- and orientation-cross). We expressed the decoding accuracy of each distractor condition as a proportion of the decoding accuracy in the no-distractor condition. Values close to one indicate comparable information, while values below one mean the decoder does not generalize well. We found that the cross-decoding accuracies were significantly lower than the no-distractor in all ROIs but V4 (in both noise and orientation) and LO2 (only noise). Thus, in most areas the decoder did not generalize well from the no-distractor to distractor conditions. However, the total amount of information in distractor trials was generally slightly lower ( Fig. 4a ). Thus, we also compared the generalization to a decoder trained and tested on the same distractor condition ( Fig. 4b noise- and orientation-within), which might thus be able to extract more information. We found that indeed information recovered in areas V2 and V3AB in the noise distractor condition ( Fig. 4b , pairwise permutation test). Thus, there was more information in the noise distractor condition, but it was not accessible to a decoder trained only on no-distractor trials. Additionally, a temporal cross-decoding analysis where all training time points were no-distractor trials had less dynamic coding in early visual regions ( Fig. S3 ) when compared to the temporal cross-decoding matrix when trained and tested on noise distractor trials ( Fig. 1d ). These results indicate a change in the VWM format between the noise distractor and no-distractor trials.

Cross-decoding between distractor and no-distractor conditions.
a) Decoding accuracy (feature continuous accuracy; FCA) across time for train and test on no-distractor trials (purple), train and test on noise distractor trials (dark green) and train and test on orientation distractor trials (light green). Horizontal lines indicate clusters where there is a difference between two time courses (all clusters p < 0.05; nonparametric cluster permutation test, see color code on the right). b) Decoding accuracy as a proportion of no-distractor decoding estimated on the averaged delay period (4-16.8s). Nonparametric permutation tests compared the decoding accuracy of each analysis to the no-distractor decoding baseline (indicated as a dashed line) and between a decoder trained and tested on distractor trials (noise- or orientation-within) and a decoder trained on no-distractor trials and tested on distractor trials (noise or orientation-cross). FDR-corrected across ROIs. * p < 0.05, *** p < 0.001. Corresponding p -values found in Supplementary Table 2 .
We examined the dynamics of visual working memory (VWM) with and without distractors and explored the impact of sensory distractors on the coding spaces of VWM contents in visual areas by reanalyzing previously published data ( 8 ). Participants completed a task during which they had to maintain an orientation stimulus in VWM. During the delay period either no distractor, an orientation distractor, or a noise distractor were presented. We assessed two potential mechanisms that could help concurrently maintain the superimposed sensory and memory representations. First, we examined whether changes were observable in the multivariate code for memory contents across time, which we term dynamic coding. For this we used two different analyses: temporal cross-classification and a direct assessment of angles between coding spaces. We found evidence for dynamic coding in all conditions, but there were differences in these dynamics between conditions and regions. Dynamic coding was most pronounced during the noise distractor trials in early visual regions. Second, we assessed the complementary question of temporally stable coding spaces. We computed the stable neural subspaces by averaging across the delay period. We saw that coding of the VWM target and concurrent sensory distractors occurred in different stable neural subspaces. Finally, we observed that the format of the multivariate VWM code during the noise distraction differs from the VWM code when distractors were not present.
Dynamic encoding of VWM contents has been previously repeatedly examined. Temporal cross-decoding analyses have been used in a number of non-human primate electrophysiology and human fMRI studies ( 15 , 22 , 23 , 25 , 26 , 38 , 41 ). Spaak et al. ( 22 ) found dynamic coding in the non-human primate PFC during a spatial VWM task. They observed a change in the multivariate code between different stages; specifically a first shift between the encoding and maintenance periods, and also a second shift between the maintenance and retrieval periods. The initial transformation between the encoding and maintenance periods might recode the percept of the target into a stable VWM representation, whereas the second might transform the stable memoranda into a representation suited for initiation of motor output. A similar dynamic coding pattern was also observed in human visual regions using neuroimaging ( 26 ). In this study, in all three conditions we find a comparable pattern of results, where the multivariate code changes between the early delay and middle delay, and middle delay and late delay periods.
When noise distractors are added to the delay period we find evidence of additional coding shifts in V1 during the middle of the delay. Previous research in non-human primates has shown that the presentation of a distractor induces a change in multivariate encoding for VWM in lateral PFC (lPFC) ( 23 ). More precisely, a lack of generalization was observed between the population code encoding VWM before the presentation of a distractor (first half of the delay) and after its presentation (the second half). Additionally, continuous shifts in encoding have been observed in the extrastriate cortex throughout the extent of the delay period when decoding multiple remembered items at high VWM load ( 25 ). The dynamic code has been interpreted to enable multiplexing of representations when the visual cortex is overloaded by the maintenance of multiple stimuli at once. Future research could examine how properties of the distractor and of the target stimulus could interact to lead to dynamic coding. One intriguing hypothesis is that distractors that perturb the activity of feature channels that are used to encode VWM representations induce changes in its coding space over time. It is important to note that in this experiment, the activation of the encoded target features was highest for the noise stimulus. Thus the shared spatial frequencies between noise distractor and the VWM contents potentially contribute to a more pronounced dynamic coding effect.
In a complementary analysis we directly assessed subspaces in which orientations were encoded in VWM. We defined the subspaces for three different time windows, early, middle and late. We find no evidence that the identity of orientations is confusable across time, e.g. we do not observe 45° at one given time point being recoded as 90° from a different time point. Such dynamics have been previously observed in the rotation of projected angles within a fixed neural subspace ( 32 , 34 ). Rather, we find a decreased generalization between neural subspaces at different time points, as previously observed in a spatial VWM task ( 26 ). These results suggest that the temporal dynamics across the VWM trial periods are driven by changes in the coding subspace of VWM. We do observe a preservation of the topology of the projected angles, as more similar angles remained closer together (e.g. the bin containing 45° was always closer to the bin containing 0° and 90°). Such a topology has been seen in V4 during a color perception task ( 42 ).
We also find evidence that the VWM contents are encoded in a different way depending on whether a noise distractor is presented or not. The decoder trained on no-distractor trials does not generalize well, presumably because it fails to fully access all the information present in noise distractor trials. If the decoders are trained directly on the distractor conditions the VWM related information is much higher. Additionally, we see that the code generalizes better across time when training on no-distractor trial time points and testing on noise distractor trials. This may imply that by training our decoder on the no-distractor trials we are able to uncover an underlying stable population code encoding VWM in noise distractor trials. Consistent with this finding, Murray et al. ( 21 ) demonstrated that subspaces derived on the delay period could still generalize to the more dynamic encoding and retrieval periods, albeit not perfectly.
Interestingly, we found limited dynamic coding in the orientation distractor condition; primarily a change in the code between the early delay and middle delay periods was observed. Nonetheless, we find distinct temporally stable coding spaces in which sensory distractors and memory targets are encoded. These results correspond to prior research demonstrating a rotated format between perception and memory representations ( 32 ), attended and unattended VWM representations in both humans and recurrent-neural networks trained on a 2-back VWM ( 34 ) and serial retro-cueing tasks ( 35 , 43 ). Additionally, similar rotation dynamics have been observed between multiple spatial VWM locations stored in the non-human primate lPFC ( 33 ). Considering the consistency of these results across different paradigms, we speculate that separate coding spaces might be a general mechanism of how feature-matching items can be concurrently multiplexed within visual regions. With growing evidence of the relationship between VWM capacity and neural resources available within the visual cortex ( 44 – 46 ), further research could examine the number of feature-matching items that can be stored in non-aligned coding spaces.
It remains to be seen whether the degree of change or rotation between subspaces correlates with behavior. In this experiment, we do not observe a behavioral deficit in the feature-matching orientation distractor trials ( 8 ). Yet there is evidence from behavioral and neural studies that show interactions between perception and VWM: feature-matching distractors behaviorally bias retrieved VWM contents ( 47 , 48 ); VWM representations influence perception ( 49 – 52 ); neural visual VWM representations in the early visual cortices are biased towards distractors ( 53 ); and the fidelity of VWM neural representations within the visual cortex negatively correlates with behavioral errors when recalling VWM during a sensory distraction task ( 54 ). In cases where a distractor does induce a drop in recall accuracy or biases the recalled VWM target, VWM and the sensory distractor neural subspaces might overlap more.
To our surprise, we did not observe a significant difference in the coding format of VWM between orientation distractor and no-distractor trials. Our initial expectation was that the VWM coding might undergo changes due to the target representation avoiding the distractor stimulus. However, the presence of a generalizing code between no-distractor and orientation distractor trials, along with the non-aligned coding spaces between the target and distractor in the orientation trials, suggests an alternative explanation. We suggest that the sensory distractor stimulus occupies a distinct coding space throughout its presentation during the delay, while the coding space of the target remains the same in both orientation and no-distractor trials. Layer-specific coding differences in perception and VWM might explain these findings ( 29 , 31 , 55 ). Specifically, the sensory distractor neural subspace might predominantly reside in the bottom-up middle layers of early visual cortices, while the neural subspace encoding VWM might primarily occupy the superficial and deep layers.
We provide evidence for two types of mechanisms found in visual areas during the presence of both VWM and sensory distractors. First, our findings show dynamic coding of VWM within the human visual cortex during sensory distraction and indicate that such activity is not only present within the lPFC. Second, we find that VWM and feature-matching sensory distractors are encoded in shifted coding spaces. Taking into account previous findings, we posit that different coding spaces within the same region might be a more general mechanism of segregating feature-matching stimuli. In sum, these results provide possible mechanisms of how VWM and perception are concurrently present within visual areas.
Participants, stimuli, procedure, and preprocessing
The following section is a brief explanation of parts of the methods covered in Rademaker et al. ( 8 ). Readers may refer to that paper for details. We reanalyzed data from Experiment 1.
Six participants performed two tasks while in the scanner: a VWM task and a perceptual localizer task. In the perceptual localizer task, either a donut-shaped or a circle-shaped grating was presented in 9 second blocks. The participants had to respond whenever the grating dimmed. There were a total of 20 donut-shaped and 20 circle-shaped gratings in one run. Participants completed a total of 15-17 runs.
The visual VWM task began with the presentation of a colored 100% valid cue which indicated the type of trial: no-distractor, orientation distractor, or noise distractor. Following the cue, the target orientation grating was presented centrally for 500 ms, followed by a 13 s delay period. In the trials with the distractor, a stimulus of the same shape and size as the target grating was presented centrally for 11 s in the middle of the delay period ( Fig. 1a ). The orientation and noise distractors reversed contrast at 4 Hz. At the end of the delay, a probe stimulus bar appeared at a random orientation. The participants had to align the bar to the target orientation and had to respond in 3 s.
The orientations for the VWM sample were pseudo-randomly chosen from six orientation bins each consisting of 30 orientations. The orientation distractor and sample were counterbalanced in order not to have the same orientation presented as a distractor. Each run consisted of four trials of each condition. Across three sessions participants completed 27 runs of the task resulting in a total of 108 trials per condition.
The data were acquired using a simultaneous multi-slice EPI sequence with a TR of 800 ms, TE of 35 ms, flip angle of 52°, and isotropic voxels of 2 mm. The data were preprocessed using FreeSurfer and FSL and time-series were z-scored across time for each voxel.
Voxel selection
We used the same regions of interest (ROI) as in Rademaker et al. ( 8 ), which were derived using retinotopic mapping. In contrast to the original study, we reduced the size of our ROIs by selecting voxels that reliably responded to both the donut-shaped orientation perception task and the no-distractor VWM task. In order to select reliably activating voxels, we calculated four tuning functions for each voxel: two from the perceptual localizer and two from the no-distractor VWM task. The tuning functions spanned the continuous feature space in bins of 30°. Thus, to calculate the tuning functions, we ran a split-half analysis using stratified sampling where we binned all trials into six bins (of 30°). For both halves, tuning functions were estimated using a GLM that included six orientation regressors (one for each bin) and assumed an additive noise component independent and identically distributed across trials. We calculated Pearson correlations between the no-distractor memory and the perception tuning functions across the six parameter estimates extracted from the GLM, thus generating one memory-memory and one perception-perception correlation coefficient for each voxel.
The same analysis was additionally performed 1,000 times on randomly permuted orientation labels to generate a null distribution for each participant and each ROI. These distributions were used to check for the reliability of voxel activation to perception and no-distractor VWM. After performing Fisher z-transformation on the correlations, we selected voxels that had a value above the 75th percentile of the null distributions in both the memory-memory and perception-perception correlations. This population of voxels was then used for all subsequent analyses. IPS included reliable voxels from retinotopically derived IPS0, IPS1, and IPS2.
Periodic support vector regression
We used periodic support vector regression (pSVR) to predict the target orientation from the multivariate BOLD activity ( 40 ). PSVR uses a regression approach to estimate the sine and cosine components of a given orientation independently and therefore accounts for the circular nature of stimuli. In order to have a proper periodic function, orientation labels from the range [0°, 180°) were projected into the range [0, 2π).
We used the support vector regression algorithm using a non-linear radial basis function (RBF) kernel implemented in LIBSVM ( 56 ) for orientation decoding. Specifically, sine and cosine components of the presented orientations were predicted based on multivariate fMRI signals from a set of voxels at specific time points within a trial (see Temporal Generalization ). In each cross-validation fold, we rescaled the training data voxel activation into the range [0, 1] and applied the training data parameters to rescale the test data. For each participant we had a total of three iterations in our cross-validation, where we trained on two thirds (i.e. two sessions) and tested on one third of the data (i.e. the left-out session). We selected three iterations in order to mitigate training and test data leakage (see Temporal Generalization ).
After pSVR-based analysis, reconstructed orientations were obtained by plugging the predicted sine and cosine components into the four-quadrant inverse tangent:
where x p and y p are pSVR outputs in the test set. Prediction accuracy was measured as the trial-wise absolute angular deviation between predicted orientation and actual orientation:
where θ is the labeled orientation and θ p is the predicted orientation. This measure was then transformed into a trial-wise feature continuous accuracy (FCA) ( 57 ) as follows:
The final across-trial accuracy was the mean of the trial-wise FCAs. Mean FCA was calculated across predicted orientations from all test sets after cross-validation was complete. The FCA is an equivalent measurement to standard accuracy measured in decoding analyses falling into the range between 0 and 100%, but extended to the continuous domain. In the case of random guessing, the expected angular deviation is π/2, resulting in chance-level FCA at 50%.
Temporal cross-decoding
To determine the underlying stability of the VWM code, we ran a temporal cross-decoding analysis using pSVR ( Fig. 1 ). We trained on data from a given time point and then predicted orientations for all time points, using the presented targets as labels. We trained on two-thirds of the trials per iteration and tested on the left-out third. Training and test data were never taken from the same trials, both when testing on the same and different time points.
We used a cluster-based approach to test for significance for above-chance decoding clusters ( 58 ). To determine whether the size of the cluster of the above-chace values was significantly larger than chance, we calculated a summed t-value for each cluster. We then generated a null distribution by randomly permuting the sign of the estimated above-chance accuracy (each FCA value was subtracted by 50%, such that 0 corresponds to chance level) of all components within the temporal cross-decoding matrix. We calculated the summed t-value for the largest randomly occurring above-chance cluster. This procedure was repeated 1000 times to estimate a null distribution. The empirical summed t-value of each cluster was then compared to the null distribution to determine significance ( p < 0.05; without control of multiple cluster comparisons).
Dynamic coding clusters were defined as elements within the temporal cross-decoding matrix where the multivariate code at a given time point did not fully generalize to another time point; in other words, an off-diagonal element was significantly smaller in accuracy compared to its two corresponding on-diagonal elements ( a ij < a ii and a ij < a jj , Fig. 1b ). In order to test for significance of these clusters, we ran two cluster-permutation tests as done in previous studies to define dynamic clusters ( 22 , 26 ). In each test, we subtracted one or the other corresponding diagonal elements from the off-diagonal elements ( a ij – a ii and a ij – a jj ). We then ran the same sign permutation test as for the above-chance decoding cluster for both comparisons. An off-diagonal element was deemed dynamic, if both tests were significant ( p < 0.05) and it was part of the above-chance decoding cluster.
Following ( 22 ), we also computed the dynamicism index as a proportion of elements across time that were dynamic. Specifically, we calculated the proportion of (off-diagonal) dynamic elements corresponding to a diagonal time point in both columns (corresponding to the test time points) and rows (corresponding to the train time points) of the temporal cross-decoding matrix.
Neural subspaces
We adapted the method from ( 26 ) to calculate two-dimensional neural subspaces encoding VWM information at a given time point. To do so, we used principal component analysis (PCA). To maximize power, we binned trial-wise fMRI activations into four equidistant bins of 45 degrees and averaged the signal across all trials within a bin ( Fig. 2a ). The data matrix X was defined as a p×ν matrix where p = 4 was the four orientation bins, and ν was the number of voxels. We mean-centered the columns (i.e. each voxel) of the data matrix.
This analysis focused on the time points from 4 s to 17.6 s after delay onset. The first TRs were not used since the temporal cross-decoding results showed no above-chance decoding. We averaged across every three TRs leading to six non-overlapping temporal bins resulting in six X matrices. We calculated the principal components (PCs) using eigendecomposition of the covariance matrix for each X and defined the matrix V using the two largest eigenvalues as a ν × 2 matrix, resulting in six neural subspaces, one for each non-overlapping temporal bin.
Neural subspaces across time
For visualization purposes, we used three out of the total of six neural subspaces from the following time points: early (7.2 s), middle (12 s), and late (16.8 s). Following the aforementioned procedure, these subspaces were calculated on half of the trials, as we projected the left-out data onto the subspaces. The left-out data were binned into six temporal bins between 4 s and 17.6 s after target onset with no overlap just like in the calculation of the six subspaces. The projection resulted in a p × 2 matrix P for each projected time bin (resulting in a total of six P matrices). We use distinct colors to plot the temporal trajectories of each orientation bin across time in a 2D subspace flattened ( Fig. 2c ) and not flattened ( Fig. S1 ) across the time dimension. Importantly, the visualization analysis was done on a combined participant-aggregated V1-V3AB region, which included all reliable voxels across the four regions and all six participants (see Voxel Selection ).
To measure the alignment between coding spaces at different times, we calculated an above-baseline principal angle (aPA) between all subspaces ( Fig. 2c ). We used the MATLAB function subspace for an implementation of the method proposed by ( 59 ) to measure the angle between two V matrices. This provided us with a possible principal angle between 0-90°; the higher the angle, the larger the difference between the two subspaces. In order to avoid overfitting and as in the visualization analysis, we used a split-half approach to compute the aPA between subspaces. Half of the binned trials were used to calculate V i,A and V j,A and half for V i,B V j,B , where A and B refer to the two halves of the split and and i and j refer to the two time bins compared. For significance testing, the within-subspace angle (the angle between two splits of the data within a given temporal bin (i.e. V i,A and V i,B )) was subtracted from the between-subspace PA (the angle between two different temporal bins (e.g. V i,A and V j,B )). Unlike the visualization analysis, the PA was calculated per participant 1,000 times using different splits of the data on a combined V1-V3AB region that included the reliable voxels across the four regions (see Voxel Selection ). The final aPA value was an average across all iterations for each participant.
Sensory distractor and orientation VWM target neural subspaces
For the orientation VWM target and sensory distractor neural subspace, we followed the aforementioned subspace analysis, but instead of calculating subspaces on six temporal bins, we averaged across the 4-17.6 s delay period and calculated a single subspace. As in the previous analysis, we split the orientation VWM trials in half. We then binned the trials either based on the target orientation or the sensory distractor. For visualization purposes, we projected the left-out data averaged based on the sensory distractor and the target onto subspaces derived from both the sensory distractor and target subspaces. As in the previous visualization, the analysis was run on a participant-aggregated V1-V3AB region.
To calculate the aPA we had the following subspaces: V Target,A , V Dist,A , V Target,B and V Dist,B , where the subspaces were calculated on trials binned either based on the target orientation or the sensory distractor. The aPA was calculated by subtracting the within-subspace angle ( V Target,A and V Target,B , V Dist,A and V Dist,B ) from the sensory distractor and working memory angle ( V Target,A and V Dist,B , V Target,B and V Dist,A ). The split-half aPA analysis was performed 1,000 times and the final value was an average across these iterations for each participant.
Data availability
The preprocessed data are shared open-access https://osf.io/dkx6y/ . The analysis scripts and results are shared https://osf.io/jq3ma/?view_only=8fcef0ef33a047e693c4102e3794319f (the link will become public (e.g. not view only) when the manuscript is published).
Acknowledgements
J.K.D. was funded by the Max Planck Society and BMBF (as part of the Max Planck School of Cognition). J.D.H. was supported by the Deutsche Forschungsgemeinschaft (DFG, Exzellenzcluster Science of Intelligence); SFB 940 “Volition and Cognitive Control”; and SFB-TRR 295 “Retuning dynamic motor network disorders using neuromodulation”. S.W. was supported by Deutsche Forschungsgemeinschaft (DFG) Research Training Group 2386 451 and EXC 2002/1 “Science of Intelligence.” Open access funding provided by Max Planck Society. We thank Rosanne Rademaker, Chaipat Chunharas, and John Serences for collecting and sharing their data open access, without which this reanalysis would not have been possible. We also thank Rosanne Rademaker, Michael Wolff, Amir Rawal, and Maria Servetnik for extensive discussions of the results. We also thank Vivien Chopurian and Thomas Christophel for their feedback on the manuscript.
Author contributions
Conceptualization, J.K.D.; Methodology, J.K.D, S.W., J.S., J.-D.H.; Formal Analysis, J.K.D.; Software, J.K.D, S.W., J.S.; Visualization, J.K.D.; Funding Acquisition, J.K.D., J.-D.H.; Writing - Original Draft Preparation, J.K.D.; Writing – Review & Editing, J.K.D., S.W., J.S., J.-D.H. Supervision, J.-D.H.
Declaration of interests
The authors declare no competing interests.

Neural trajectories across time.
Same as Figure 2c ), but the time dimension is on the z-axis.

Extension of Figure 3 for V4-LO2.

Temporal cross-decoding generalization between distractor and no-distractor VWM trials.
a) Across-participant mean temporal cross-decoding of noise distractor trials when trained on no-distractor trials. b) Same as a), but orientation distractor trials trained on no-distractor trials.

FDR-corrected p -values corresponding to Figure 2d .

FDR-corrected p -values corresponding to Figure 4b .
- D’Esposito M
- Goldman-Rakic PS
- Alexander GE
- Harrison SA
- Christophel TB
- Serences JT
- Rademaker RL
- Chunharas C
- Roelfsema PR
- Iamshchinina P
- Bettencourt KC
- Muhle-Karbe PS
- Lundqvist M
- Sreenivasan KK
- Freedman DJ
- Bernacchia A
- Constantinidis C
- Funahashi S
- Parthasarathy A
- Herikstad R
- Libedinsky C
- Lewis-Peacock JA
- Felleman DJ
- Van Essen DC
- Lawrence SJD
- van Mourik T
- Koopmans PJ
- de Lange FP
- van Kerkoerle T
- Buschman TJ
- Menendez JA
- Van Loon AM
- Olmos-Solis K
- Fahrenfort JJ
- Christophel T
- Cavanagh SE
- Kennerley SW
- Summerfield C
- Franconeri SL
- Mummaneni A
- Van Der Stigchel S
- Guggenmos M
- Vandenbroucke ARE
- Hallenbeck GE
- Oostenveld R
Article and author information
Jonas karolis degutis, for correspondence:, version history.
- Preprint posted : May 23, 2024
- Sent for peer review : May 23, 2024
- Reviewed Preprint version 1 : August 27, 2024
© 2024, Degutis et al.
This article is distributed under the terms of the Creative Commons Attribution License , which permits unrestricted use and redistribution provided that the original author and source are credited.
Views, downloads and citations are aggregated across all versions of this paper published by eLife.
Be the first to read new articles from eLife

Data visualization is the representation of data through use of common graphics, such as charts, plots, infographics and even animations. These visual displays of information communicate complex data relationships and data-driven insights in a way that is easy to understand.
Data visualization can be utilized for a variety of purposes, and it’s important to note that is not only reserved for use by data teams. Management also leverages it to convey organizational structure and hierarchy while data analysts and data scientists use it to discover and explain patterns and trends. Harvard Business Review (link resides outside ibm.com) categorizes data visualization into four key purposes: idea generation, idea illustration, visual discovery, and everyday dataviz. We’ll delve deeper into these below:
Idea generation
Data visualization is commonly used to spur idea generation across teams. They are frequently leveraged during brainstorming or Design Thinking sessions at the start of a project by supporting the collection of different perspectives and highlighting the common concerns of the collective. While these visualizations are usually unpolished and unrefined, they help set the foundation within the project to ensure that the team is aligned on the problem that they’re looking to address for key stakeholders.
Idea illustration
Data visualization for idea illustration assists in conveying an idea, such as a tactic or process. It is commonly used in learning settings, such as tutorials, certification courses, centers of excellence, but it can also be used to represent organization structures or processes, facilitating communication between the right individuals for specific tasks. Project managers frequently use Gantt charts and waterfall charts to illustrate workflows . Data modeling also uses abstraction to represent and better understand data flow within an enterprise’s information system, making it easier for developers, business analysts, data architects, and others to understand the relationships in a database or data warehouse.
Visual discovery
Visual discovery and every day data viz are more closely aligned with data teams. While visual discovery helps data analysts, data scientists, and other data professionals identify patterns and trends within a dataset, every day data viz supports the subsequent storytelling after a new insight has been found.
Data visualization
Data visualization is a critical step in the data science process, helping teams and individuals convey data more effectively to colleagues and decision makers. Teams that manage reporting systems typically leverage defined template views to monitor performance. However, data visualization isn’t limited to performance dashboards. For example, while text mining an analyst may use a word cloud to to capture key concepts, trends, and hidden relationships within this unstructured data. Alternatively, they may utilize a graph structure to illustrate relationships between entities in a knowledge graph. There are a number of ways to represent different types of data, and it’s important to remember that it is a skillset that should extend beyond your core analytics team.
Use this model selection framework to choose the most appropriate model while balancing your performance requirements with cost, risks and deployment needs.
Register for the ebook on generative AI
The earliest form of data visualization can be traced back the Egyptians in the pre-17th century, largely used to assist in navigation. As time progressed, people leveraged data visualizations for broader applications, such as in economic, social, health disciplines. Perhaps most notably, Edward Tufte published The Visual Display of Quantitative Information (link resides outside ibm.com), which illustrated that individuals could utilize data visualization to present data in a more effective manner. His book continues to stand the test of time, especially as companies turn to dashboards to report their performance metrics in real-time. Dashboards are effective data visualization tools for tracking and visualizing data from multiple data sources, providing visibility into the effects of specific behaviors by a team or an adjacent one on performance. Dashboards include common visualization techniques, such as:
- Tables: This consists of rows and columns used to compare variables. Tables can show a great deal of information in a structured way, but they can also overwhelm users that are simply looking for high-level trends.
- Pie charts and stacked bar charts: These graphs are divided into sections that represent parts of a whole. They provide a simple way to organize data and compare the size of each component to one other.
- Line charts and area charts: These visuals show change in one or more quantities by plotting a series of data points over time and are frequently used within predictive analytics. Line graphs utilize lines to demonstrate these changes while area charts connect data points with line segments, stacking variables on top of one another and using color to distinguish between variables.
- Histograms: This graph plots a distribution of numbers using a bar chart (with no spaces between the bars), representing the quantity of data that falls within a particular range. This visual makes it easy for an end user to identify outliers within a given dataset.
- Scatter plots: These visuals are beneficial in reveling the relationship between two variables, and they are commonly used within regression data analysis. However, these can sometimes be confused with bubble charts, which are used to visualize three variables via the x-axis, the y-axis, and the size of the bubble.
- Heat maps: These graphical representation displays are helpful in visualizing behavioral data by location. This can be a location on a map, or even a webpage.
- Tree maps, which display hierarchical data as a set of nested shapes, typically rectangles. Treemaps are great for comparing the proportions between categories via their area size.
Access to data visualization tools has never been easier. Open source libraries, such as D3.js, provide a way for analysts to present data in an interactive way, allowing them to engage a broader audience with new data. Some of the most popular open source visualization libraries include:
- D3.js: It is a front-end JavaScript library for producing dynamic, interactive data visualizations in web browsers. D3.js (link resides outside ibm.com) uses HTML, CSS, and SVG to create visual representations of data that can be viewed on any browser. It also provides features for interactions and animations.
- ECharts: A powerful charting and visualization library that offers an easy way to add intuitive, interactive, and highly customizable charts to products, research papers, presentations, etc. Echarts (link resides outside ibm.com) is based in JavaScript and ZRender, a lightweight canvas library.
- Vega: Vega (link resides outside ibm.com) defines itself as “visualization grammar,” providing support to customize visualizations across large datasets which are accessible from the web.
- deck.gl: It is part of Uber's open source visualization framework suite. deck.gl (link resides outside ibm.com) is a framework, which is used for exploratory data analysis on big data. It helps build high-performance GPU-powered visualization on the web.
With so many data visualization tools readily available, there has also been a rise in ineffective information visualization. Visual communication should be simple and deliberate to ensure that your data visualization helps your target audience arrive at your intended insight or conclusion. The following best practices can help ensure your data visualization is useful and clear:
Set the context: It’s important to provide general background information to ground the audience around why this particular data point is important. For example, if e-mail open rates were underperforming, we may want to illustrate how a company’s open rate compares to the overall industry, demonstrating that the company has a problem within this marketing channel. To drive an action, the audience needs to understand how current performance compares to something tangible, like a goal, benchmark, or other key performance indicators (KPIs).
Know your audience(s): Think about who your visualization is designed for and then make sure your data visualization fits their needs. What is that person trying to accomplish? What kind of questions do they care about? Does your visualization address their concerns? You’ll want the data that you provide to motivate people to act within their scope of their role. If you’re unsure if the visualization is clear, present it to one or two people within your target audience to get feedback, allowing you to make additional edits prior to a large presentation.
Choose an effective visual: Specific visuals are designed for specific types of datasets. For instance, scatter plots display the relationship between two variables well, while line graphs display time series data well. Ensure that the visual actually assists the audience in understanding your main takeaway. Misalignment of charts and data can result in the opposite, confusing your audience further versus providing clarity.
Keep it simple: Data visualization tools can make it easy to add all sorts of information to your visual. However, just because you can, it doesn’t mean that you should! In data visualization, you want to be very deliberate about the additional information that you add to focus user attention. For example, do you need data labels on every bar in your bar chart? Perhaps you only need one or two to help illustrate your point. Do you need a variety of colors to communicate your idea? Are you using colors that are accessible to a wide range of audiences (e.g. accounting for color blind audiences)? Design your data visualization for maximum impact by eliminating information that may distract your target audience.
An AI-infused integrated planning solution that helps you transcend the limits of manual planning.
Build, run and manage AI models. Prepare data and build models on any cloud using open source code or visual modeling. Predict and optimize your outcomes.
Unlock the value of enterprise data and build an insight-driven organization that delivers business advantage with IBM Consulting.
Your trusted Watson co-pilot for smarter analytics and confident decisions.
Use features within IBM Watson® Studio that help you visualize and gain insights into your data, then cleanse and transform your data to build high-quality predictive models.
Data Refinery makes it easy to explore, prepare, and deliver data that people across your organization can trust.
Learn how to use Apache Superset (a modern, enterprise-ready business intelligence web application) with Netezza database to uncover the story behind the data.
Predict outcomes with flexible AI-infused forecasting and analyze what-if scenarios in real-time. IBM Planning Analytics is an integrated business planning solution that turns raw data into actionable insights. Deploy as you need, on premises or on cloud.

What Is Data Visualization and Why Is It Important?
The sheer amount of data generated today means we need new ways to understand what’s happening in order to take action faster. Every click, transaction, subscription, loyalty card swipe, and social media interaction contributes to a digital footprint that continues to grow exponentially. The result? A massive explosion of data that is revolutionizing the way we live and work. Data visualization, in particular, plays a critical role in presenting data in a meaningful and understandable format. By using a visual representation of data , it’s much easier to identify patterns, trends, and relationships that may not be immediately apparent when sifting through large data sets.
Here’s what we’ll cover in this guide to data visualization:
- Data Visualization Definition
Benefits of Data Visualization
Why data visualization is important .
- Types of Data Visualization and Examples
- Evaluating Data Visualization Tools
- Take the Next Step and Start Analyzing With Data Visualization
Data Visualization Definition
Data visualization is the process of transforming raw data into visual formats, such as charts, graphs, or maps, to help identify patterns, trends, and insights that might not be apparent from numerical data alone.
Additionally, it enables data to be more accessible, understandable, and impactful, especially when communicating with stakeholders, investors, or team members who may not be familiar with the data.
For example, data visualization could help:
- In retail, gaining insights into customer behavior, purchase patterns, and product performance.
- In finance, monitoring market trends, tracking portfolio performance, and conducting risk analysis.
- In public health, showing the geographical distribution of outbreaks and helping track the spread of infectious diseases.
- In supply chain industries, tracking inventory levels, monitoring logistics operations, and optimizing resource allocation.
- In sports, evaluating player performance, game strategies, and match statistics.
- In education, tracking student performance, analyzing learning outcomes, and identifying areas for improvement.
Data visualization has several benefits for businesses including: the ability to process information faster, identify trends at scale, and make data more digestible. Companies regularly use data to make decisions, and through data visualization, can find insights quickly and move to action. Data visualization specifically helps with the following:
- Visualizing patterns and relationships
- Storytelling, including specifically data storytelling
- Accessibility to information
Exploration
Let’s take a look at each of these benefits in detail.
Visualize patterns and relationships
Data visualization constitutes an excellent method for the discernment of interconnections and patterns amidst vast collections of information. For example, a scatter plot can be used to display the relationship between two variables, such as the correlation between temperature and sales. This enables users to understand the relationship and identify trends and outliers more quickly and easily.
Read a guide of Sigma’s visual library.

Storytelling
Your audience, whether it's coworkers or clients, want to hear a coherent story from your data. Storytelling with data cannot be done successfully without visualizations. Colorful charting and dynamic pivots are just as important as characters and plots are in a traditional story, so using them to communicate information makes data that much more engaging and memorable for audiences. Data can be complex and convoluted for some audiences, so data storytelling is an approach to convey important information effectively through a captivating narrative. Good visualizations are a vital part of that narrative.
For example, if an analyst is investigating the performance of e-commerce sales for their retail company over time, they may leverage several data sources such as spreadsheets, calculations, code, etc. to do so. However, when they report these new insights to their stakeholders, the analyst will need to summarize and communicate their findings in a digestible way.
An easy way the analyst could do this is by using the data to create a map of the U.S. with a color gradient overlaying every state that is lighter or darker based on its total sales volume. This visual story tells the least and most successful retail locations at a glance.

Accessibility / Easily Share Information
Data visualization serves as an invaluable mechanism for the facilitation of accessibility, allowing for the communication of information amongst individuals, even for those who may not usually engage with data , which broadens the audience.
Visualizations help simplify complex information by leveraging people’s ability to naturally recognize patterns. A viewer typically does not have to be taught that bigger means more and that smaller means less. In a case where an analyst wants to highlight the difference in scale between one product’s profitability vs. another, a bar chart can clearly show the user which product is more profitable and by how much, making it easy for even non-technical team members to understand and compare the performance of different products.
Exploration is a key component of successful data visualization. The more flexible charting and dashboarding is, the more follow-up questions end users can ask directly of their data. For example, an interactive dashboard can be used to explore retail sales data over time, enabling users to filter and drill down into the data to identify trends and patterns.
Data visualization exploration is often associated with the concept of “drill downs.” Drill downs in data visualization refer to the process of starting with an overview of data and then narrowing the focus to more specific aspects of it. As an example, one might start with a visualization of global climate data and drill down to data about a specific country, a specific state, a specific city, or even a specific neighborhood within that city. Each drill down reveals more precise, detailed, and nuanced information.
The main goal of data visualization is that it helps unify and bring teams onto the same page. The human mind is wired to grasp visual information more effortlessly than raw data in spreadsheets or detailed reports. Thus, graphical representation of voluminous and intricate data is more user-friendly. Data visualization offers a swift and straightforward method to communicate ideas in a universally understood format, with the added benefit of enabling scenario testing through minor modifications.
By translating information into visual form, it ensures everyone, irrespective of the complexity of the data or the depth of the analysis, can share a unified understanding. Any industry can benefit from using data visualization, because pretty much every industry relies on data to power it. That includes finance, marketing, consumer goods, education, government, sports, history, and many more. Another thing to keep in mind is that data visualization can be a double-edged sword. For example, charts can be manipulated and skewed to force a desired outcome. Ungoverned, static, desktop tools can become the wild west in suggesting an inaccurate outcome “proven by data.” Even in the cases where the visualization builder is acting in good faith, there are still pitfalls to watch out for. Always be considerate of:
- Individual outliers having an outsized impact, skewing the visual direction of a chart
- The need for for business users to see the underlying data
- Allowing for transparency down to row-level detail in data sets

Types of Data Visualizations & Examples
There is a long list of types of data visualization techniques and methods that can be used to represent data. While no type of data visualization is perfect, we’ll walk through different examples and when to apply each one.
We’ll be looking at:
- Line charts and area charts
- Scatter plots
- Pivot tables
- Box-and-whisker plots
- Sankey charts
Tables, although more commonly thought of as a data source, can also be considered a type of data visualization. Especially when conditional formatting is applied to the table’s rows and columns, the data within the table becomes more visually engaging and informative. With conditional formatting, important insights and patterns can be highlighted, making it easier for viewers to identify trends and outliers at a glance. Additionally, tables offer a structured and organized way to present information, allowing for a comprehensive comparison of data points, which further enhances data understanding and analysis. For example, Sigma’s UI is based on a spreadsheet-like interface, which means almost everything in Sigma begins in a table format. That said, you can also create visual tables that display a smaller amount of data in order to tell a clearer story. In data visualization, tables are a simplified way of representing this interface.
When to use tables:
- For detailed numeric comparisons, or when precision of data is key
- For displaying multidimensional data; tables can handle this complexity quite well
When to avoid tables:
- When patterns, trends, or relationships need to be highlighted at a glance
- When dealing with large amounts of data

Pie charts —similar to stacked bar charts—are useful for displaying categorical data, such as market share or customer demographics. Pie charts are often used to display data that can be divided into categories or subgroups, and to show how each category or subgroup contributes to the whole. For example, a pie chart could be used to show the proportion of sales for different product categories in a given period of time, or the percent of a company's revenue broken down by various regions.
When to use pie charts:
- You want to display a proportion or percentage of a whole
- You’re visualizing only seven categories or less
When to avoid pie charts:
- You’re visualizing more than seven categories
- You want to compare something with more details, rather than just proportion
- You want to display and pinpoint exact values
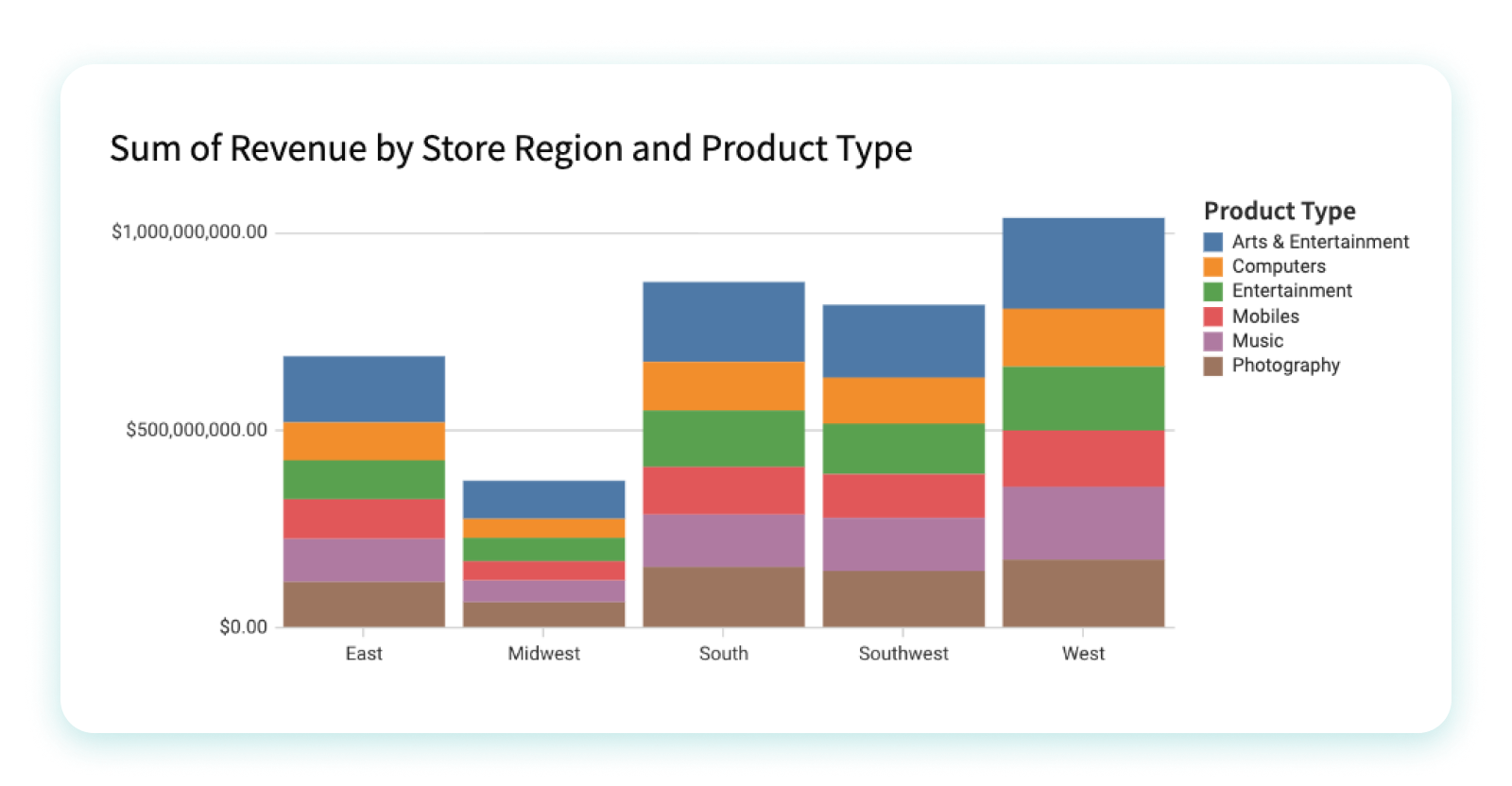
A bar chart, or bar graph, constitutes a variety of graphs that employ rectangular bars to depict data. These bars can be oriented either horizontally or vertically, with their extent being directly proportional to the numerical values they are intended to embody. Predominantly utilized for juxtaposing data across disparate categories or illustrating shifts in data over temporal progressions, bar charts offer a straightforward, yet potent means of conveying information visually. They frequently function as the initial tool in the exploratory process of data investigation.
When to use bar charts:
- Emphasizing and contrasting different sets of data, making the disparities or similarities between categories clear
- To display a subset of a larger dataset
When to avoid bar charts:
- When a particular field encompasses an overwhelming variety of data types
- When the differences between fields are too subtle, or when these differences exist on different scales, as it could lead to confusion or misinterpretation
Line Charts & Area Charts

Line charts and area charts are two types of charts that are commonly used to visualize data trends over time. A line chart, also called a line graph, is a distinct type of graphical representation that exhibits information in the form of a multitude of data points, which are interconnected by unbroken lines. These line charts are typically employed to demonstrate transformations in data over a certain duration, where the horizontal axis symbolizes time, and the vertical axis signifies the values under scrutiny. Furthermore, they can serve to juxtapose several series of data within the same chart, or to graphically illustrate predicted time periods.
For example, a line chart can be used to visualize a company's stock prices over the course of a year. Similarly, an area chart can be used to visualize the temperature changes over a day.
When to use line charts:
- When you’re displaying time-based continuous data
- When you have multiple series or larger datasets
When to avoid line charts:
- When you have smaller datasets, bar charts are likely a better way to present the information
- Avoid when you need to compare multiple categories at once

When to use area charts:
- When you want to display the volume of the data you have
- When comparing data across more than one time period
When to avoid area charts:
- Avoid if you need to compare multiple categories, as well as when you need to examine the specific data value
Scatter Plots
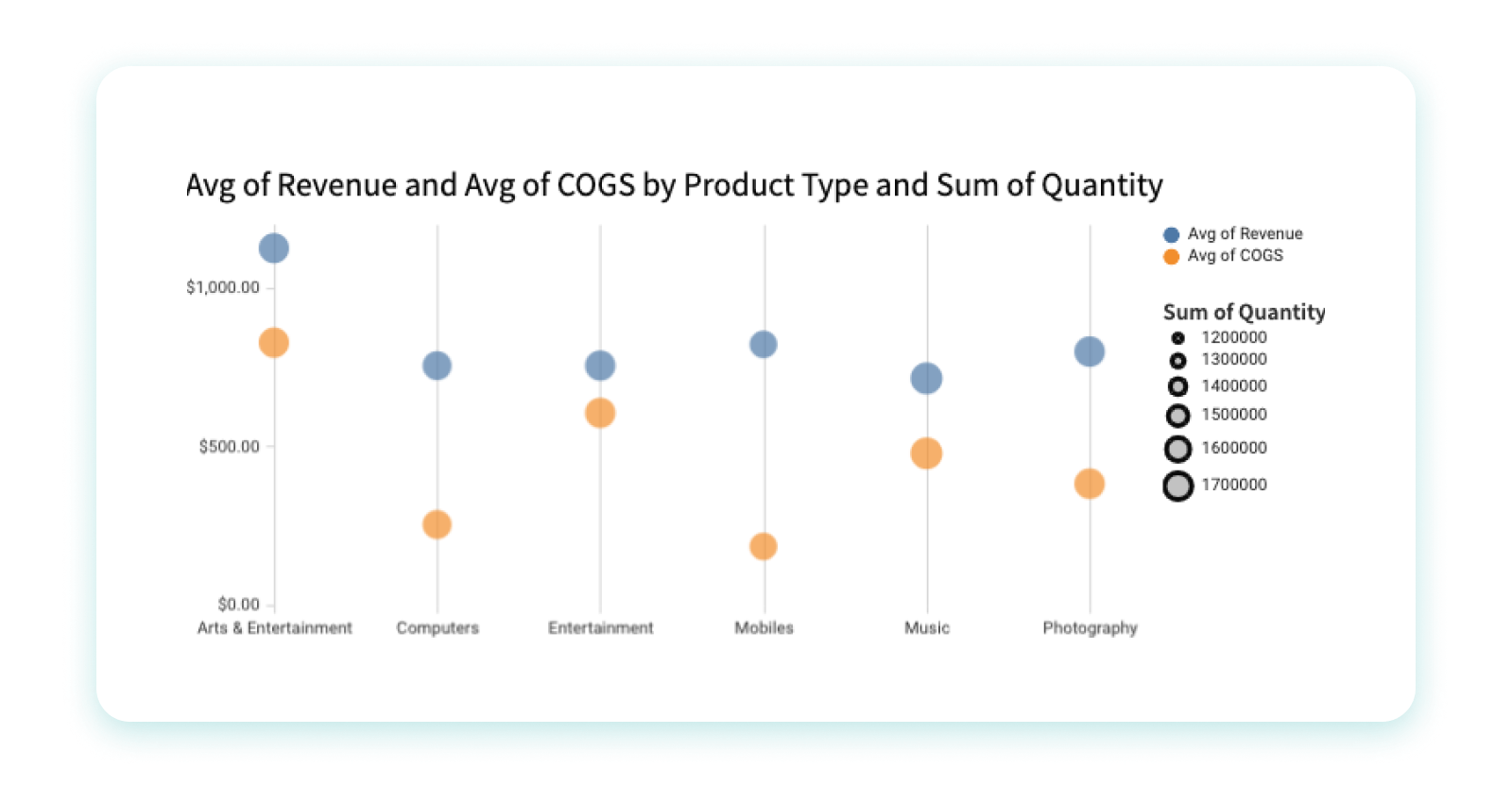
A scatter plot , also called a scatter chart or scatter graph, is a specialized form of chart that demonstrates the correlation between two distinct variables by mapping them as a succession of individual data points. Each data point denotes a combined value of the two variables, with its specific placement within the chart dictated by these values.
Scatter charts prove instrumental in discerning patterns and trends within data, and they also help us understand how strong and in what direction the relationship is between two variables. They also serve as effective tools for identifying outliers, or those data points that deviate significantly from anticipated values based on the pattern displayed by other data points. These charts find widespread use across a range of fields including, but not limited to, statistics, engineering, and social sciences, for the purpose of analyzing and visualizing intricate data sets. In the realm of business, they are frequently utilized to identify correlations between different variables, for instance, examining the relationship between marketing outlays and resultant sales revenue. For example, a scatter plot might be used to visualize the relationship between the age and income of a group of people. Another example would be to plot the correlation between the amount of rainfall and the crop yield for a particular region.
When to use scatter plots:
- Highlight correlations within your data
- They are useful tools for statistical investigations
- Consider scatter plots to reveal underlying patterns or trends
When to avoid scatter plots:
- For smaller datasets, scatter plots may not be optimal
- Avoid scatter plots for excessively large datasets to prevent unintelligible data clustering
- If your data lacks correlations, scatter plots may not be the best choice
Pivot Tables
While pivot tables may not be what first comes to mind for data visualization, they can give important context with hard numbers and provide strong visual indicators through formatting. Pivot tables can also be enhanced with conditional formatting to provide color scales that make performance trends more visible. Data bars can also be added to cells to run either red or green for positive and negative values.
When to Use Pivot Tables:
- Cohort analysis performance trends or portfolio analysis with a mix of positive and negative values
What Not to Use Pivot Tables:
- When your dataset is too large to get a good understanding of the whole
- When data can easily be summarized with a bar chart instead

An example of a pivot table, where colors are used to show positive or negative progress on a company’s portfolio. The user can pivot the table to show multiple categories in different ways.
A heat map is a type of chart that uses color to represent data values. It is often used to visualize data that is organized in a matrix or table format. The color of each cell in the matrix is determined by the value of the corresponding data point. Heat maps are best used when analyzing data that is organized in a two-dimensional grid or matrix.
For example, a heat map can be used to visualize a company's website traffic, where the rows represent different pages on the website, and the columns represent different periods of time.
When to use heat maps:
- When you need to visualize the density or intensity of variables
- When you want to display patterns or trends over time or space
When to avoid heat maps:
- When precise values are needed; heat maps are better at showing relative differences rather than precise values
- When working with small data sets
A tree map is a type of chart that is used to visualize hierarchical data. It consists of a series of nested rectangles, where the size and color of each rectangle represent a different variable. Tree maps are best used when analyzing data that has a hierarchical structure.
For example, a tree map can be used to visualize the market share of different companies in an industry. The largest rectangle would represent the entire industry, with smaller rectangles representing the market share of each individual company.
When to use tree maps:
- When you want to visualize hierarchical data
- When you need to illustrate the proportion of different categories within a whole
When to avoid tree maps:
- When exact values are important
- When there are too many categories
Box-and-Whisker Plots

Box plots are useful for quickly summarizing the distribution of a dataset, particularly its central tendency and variability. For example, a box-and-whisker plot can be used to visualize the test scores of a group of students.
Colloquially recognized as a box-and-whisker plot, a box plot is a distinct form of chart that showcases the distribution of a collection of numerical data through its quartile divisions. Box plots serve as efficient tools for rapidly encapsulating the distribution of a dataset, specifically its central propensity and variability.
A box-and-whisker plot consists of a rectangle (the "box") and a pair of "whiskers" that extend from it. The box embodies the middle 50% of the data, with the lower boundary of the box signaling the first quartile (25th percentile) and the upper boundary of the box indicating the third quartile (75th percentile). The line situated within the box signifies the median value of the data. The whiskers project from the box to the minimum and maximum values of the data, or to a designated distance from the box referred to as the "fences." Any data points that reside outside the whiskers or fences are categorized as outliers and are plotted as individual points. When to use box plot charts:
- When you want to display data spread and skewness
- When showcasing the distribution of data, including the range, quartiles, and potential outliers
- When comparing multiple groups or categories side-by-side; they allow for easy comparison of different distributions.
When to avoid box plot charts:
- If you need to show more detail, since box plots focus on a high-level summary
- When individual data points are important to the story you’re telling
- When your audience isn’t familiar with them, since they can sometimes be less intuitive than other types of visualizations
A histogram is a type of chart that displays the distribution of a dataset. It consists of a series of vertical bars, where the height of each bar represents the number of observations in a particular range. Histograms are best used when analyzing continuous data. It’s used the most when you want to understand the frequency distribution of a numerical variable, like height, weight, or age. For example, a histogram can be used to visualize the distribution of heights in a population. Read more about building histograms in Sigma here.
When to Use a Histogram:
- When understanding the shape of a distribution; for example, whether it’s symmetric, skewed to the left or right, or bimodal
- When identifying outliers, like which data points are significantly different from the rest of the data
- When comparing distribution of a variable across different groups, such as males and females, or different age groups.
- To set boundaries for data ranges; for example, you might use a histogram to determine what constitutes a "normal" or "abnormal" value for a particular variable
When to Avoid a Histogram:
- When you need to look at multiple dimensions at the same time
- If your data isn’t all on the same scale
Sankey Charts
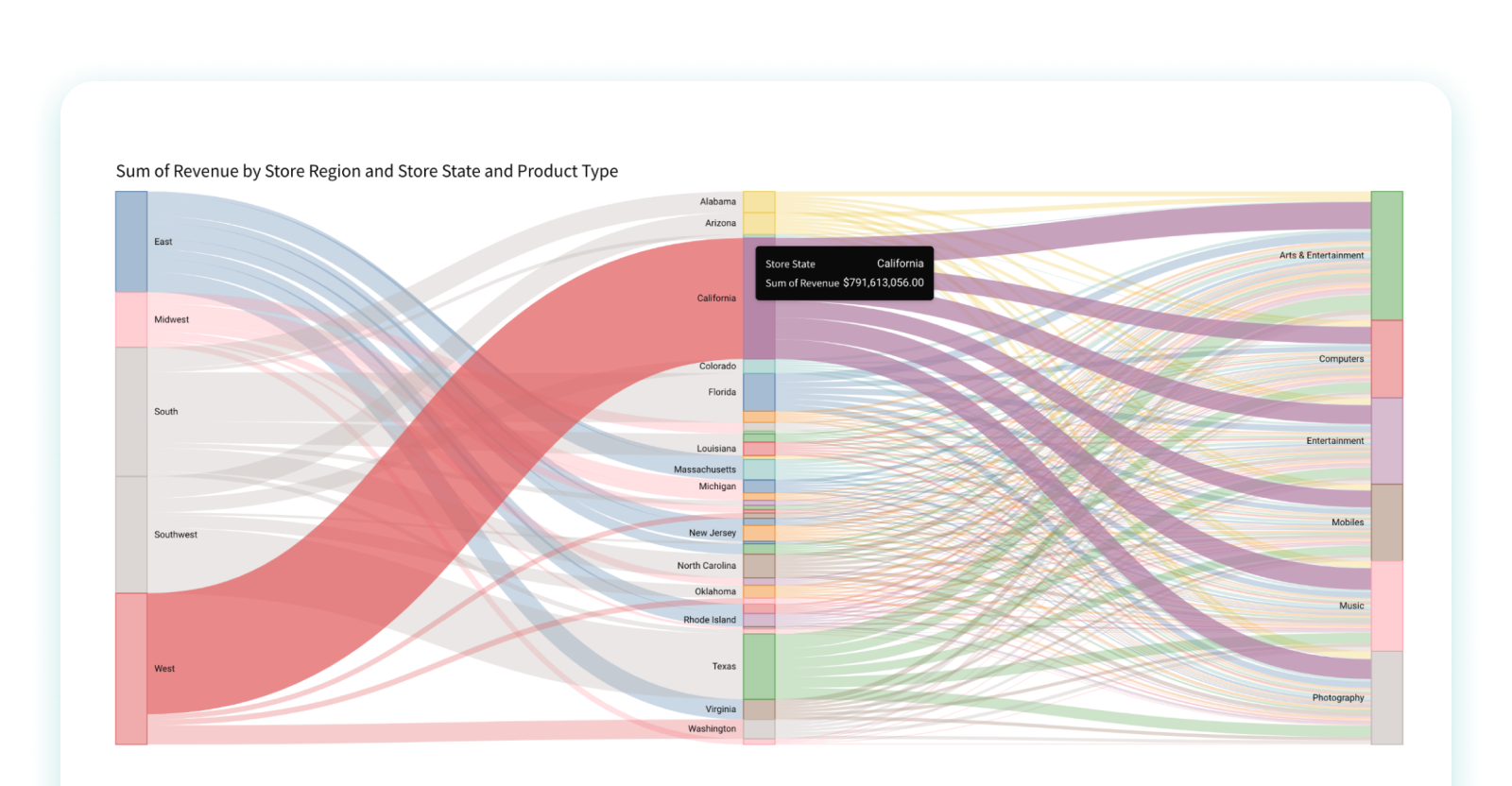
We end our guide with the controversial Sankey chart. A Sankey chart is a type of diagram that illustrates the movement or transfer of data, resources, or quantities through various stages of a system or process. Common applications of Sankey charts include visualizing complex sequences like energy usage, material distribution, or even a website's user journey. The structure of the chart includes nodes and links—with nodes representing the starting points, endpoints, or intermediate steps, and links depicting the transition of quantities or data between these nodes.
The thickness of the links in a Sankey chart directly corresponds to the volume of data or resources being moved, offering an intuitive comparison of the relative sizes of these transfers. They can be invaluable for recognizing inefficiencies, bottlenecks, or potential areas for enhancement in a system or process. These charts serve as a powerful tool for communicating complex information in a straightforward and comprehensible way. However, if there are too many nodes or links, Sankey charts can become cluttered and challenging to interpret, hence their use should be considerate and targeted.
When to use Sankey charts:
- When you want to show the data as part of a process
When to avoid Sankey charts:
- When it starts to feel too confusing, which can quickly happen when there are too many nodes or links
- When you need to see exact values, it might not be the most intuitive option.
Evaluating Data Visualization Tools
Data visualization tools have become increasingly popular in recent years, with a wide variety of options available to choose from. However, determining which tool best suits your needs can be challenging with so many options. When evaluating data visualization tools, there are several key questions to consider:
- What are your goals and needs? It's crucial to clearly understand your goals and needs before selecting a data visualization tool. Are you looking to explore your data, communicate a specific message, or both? Understanding your objectives will help you choose the right tool for your project.
- What features do you require? Different data visualization tools come with different features. Before selecting a tool, you should consider what features you need to achieve your goals. For example, do you require interactive capabilities or the ability to create custom visualizations?
- Where will your data come from? The source of your data is another critical factor to consider when selecting a data visualization tool. Some tools are better suited for specific types of data, such as structured or unstructured data, while others may require specific file formats or data storage solutions.
- Where will you need to see your data? Different data visualization tools may be more suitable for specific platforms or devices. For example, some tools may be optimized for mobile devices, while others are designed for desktop computers or specific web browsers. You may also be interested in embedding visualizations elsewhere , such as internal applications or external portals.
- Where would you like to publish your visualization? Finally, consider where you would like to publish your visualization. Some tools may provide built-in publishing capabilities, while others may require you to export your visualization to a separate platform. Selecting a tool that supports your publishing needs is important to ensure your visualization reaches your intended audience.
By considering these key questions, you can evaluate different data visualization tools and select the one that best meets your needs.
Read a side-by-side comparison of Sigma against similar BI tools.
Take the Next Step & Start Analyzing With Data Visualization
Data visualization is a powerful tool for understanding and communicating complex data. While there are many data visualization tools on the market, Sigma offers an intuitive and familiar spreadsheet interface that allows users to easily explore, analyze, and collaborate on their data.
Explore Sigma’s capabilities and start transforming your data today via a free trial of Sigma .
Related resources
.webp)
This is the default text value
Watch on-DEMAND DEMOS
ATTEND A LIVE LAB
Get a free trial
SEE WORKBOOK EXAMPLES
JOIN THE COMMUNITY
SCHEDULE A CALL
JOIN THE SIGMA COMMUNITY
We use essential cookies to make Venngage work. By clicking “Accept All Cookies”, you agree to the storing of cookies on your device to enhance site navigation, analyze site usage, and assist in our marketing efforts.
Manage Cookies
Cookies and similar technologies collect certain information about how you’re using our website. Some of them are essential, and without them you wouldn’t be able to use Venngage. But others are optional, and you get to choose whether we use them or not.
Strictly Necessary Cookies
These cookies are always on, as they’re essential for making Venngage work, and making it safe. Without these cookies, services you’ve asked for can’t be provided.
Show cookie providers
- Google Login
Functionality Cookies
These cookies help us provide enhanced functionality and personalisation, and remember your settings. They may be set by us or by third party providers.
Performance Cookies
These cookies help us analyze how many people are using Venngage, where they come from and how they're using it. If you opt out of these cookies, we can’t get feedback to make Venngage better for you and all our users.
- Google Analytics
Targeting Cookies
These cookies are set by our advertising partners to track your activity and show you relevant Venngage ads on other sites as you browse the internet.
- Google Tag Manager
Infographics
- Daily Infographics
- Popular Templates
- Accessibility
- Graphic Design
- Graphs and Charts
- Data Visualization
- Human Resources
- Beginner Guides
Blog Graphic Design What is Data Visualization? (Definition, Examples, Best Practices)
What is Data Visualization? (Definition, Examples, Best Practices)
Written by: Midori Nediger Jun 05, 2020
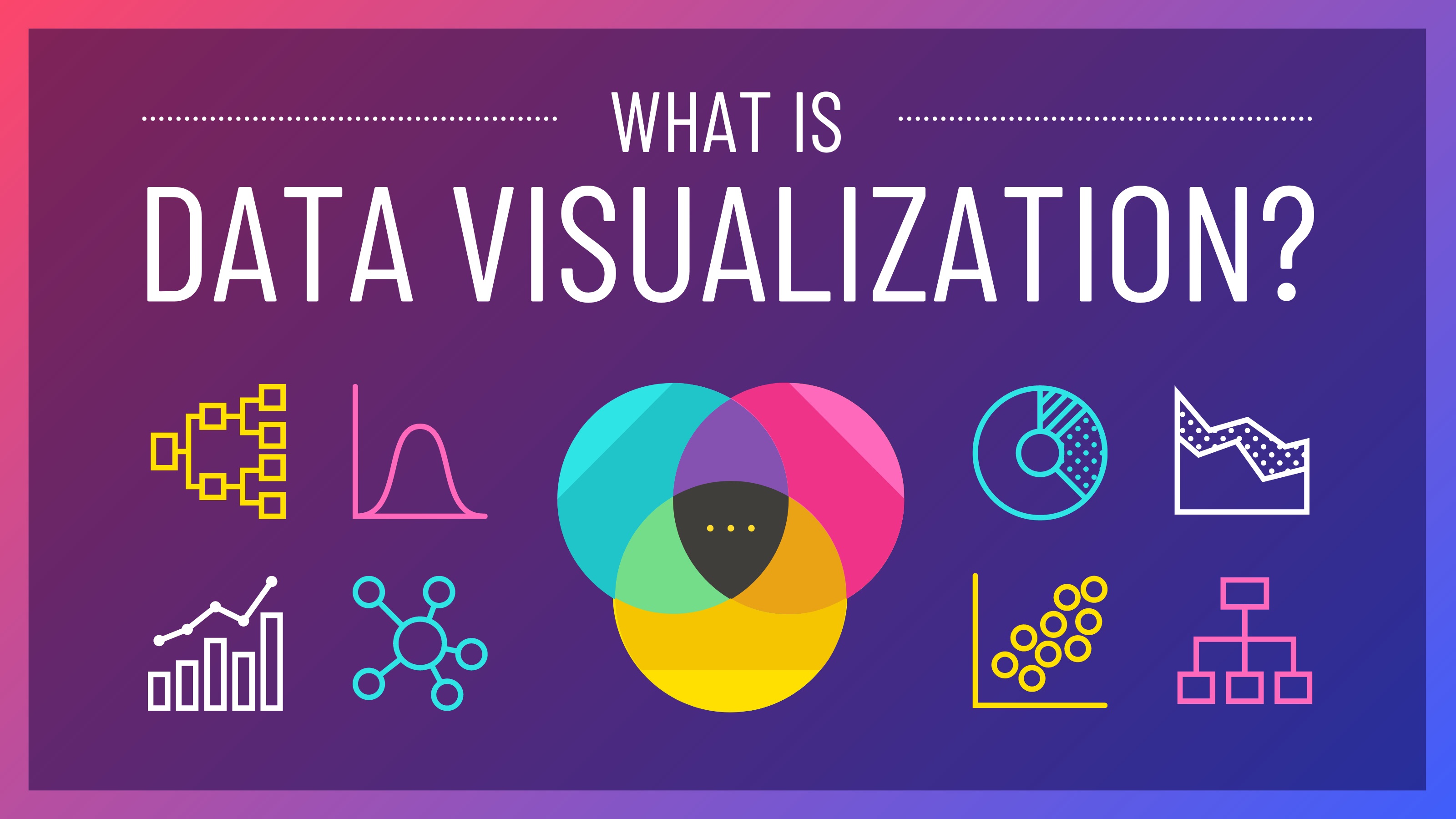
Words don’t always paint the clearest picture. Raw data doesn’t always tell the most compelling story.
The human mind is very receptive to visual information. That’s why data visualization is a powerful tool for communication.
But if “data visualization” sounds tricky and technical don’t worry—it doesn’t have to be.
This guide will explain the fundamentals of data visualization in a way that anyone can understand. Included are a ton of examples of different types of data visualizations and when to use them for your reports, presentations, marketing, and more.
Table of Contents
- What is data visualization?
What is data visualization used for?
Types of data visualizations.
- How to present data visually (for businesses, marketers, nonprofits, and education)
- Data visualization examples
Data visualization is used everywhere.
Businesses use data visualization for reporting, forecasting, and marketing.
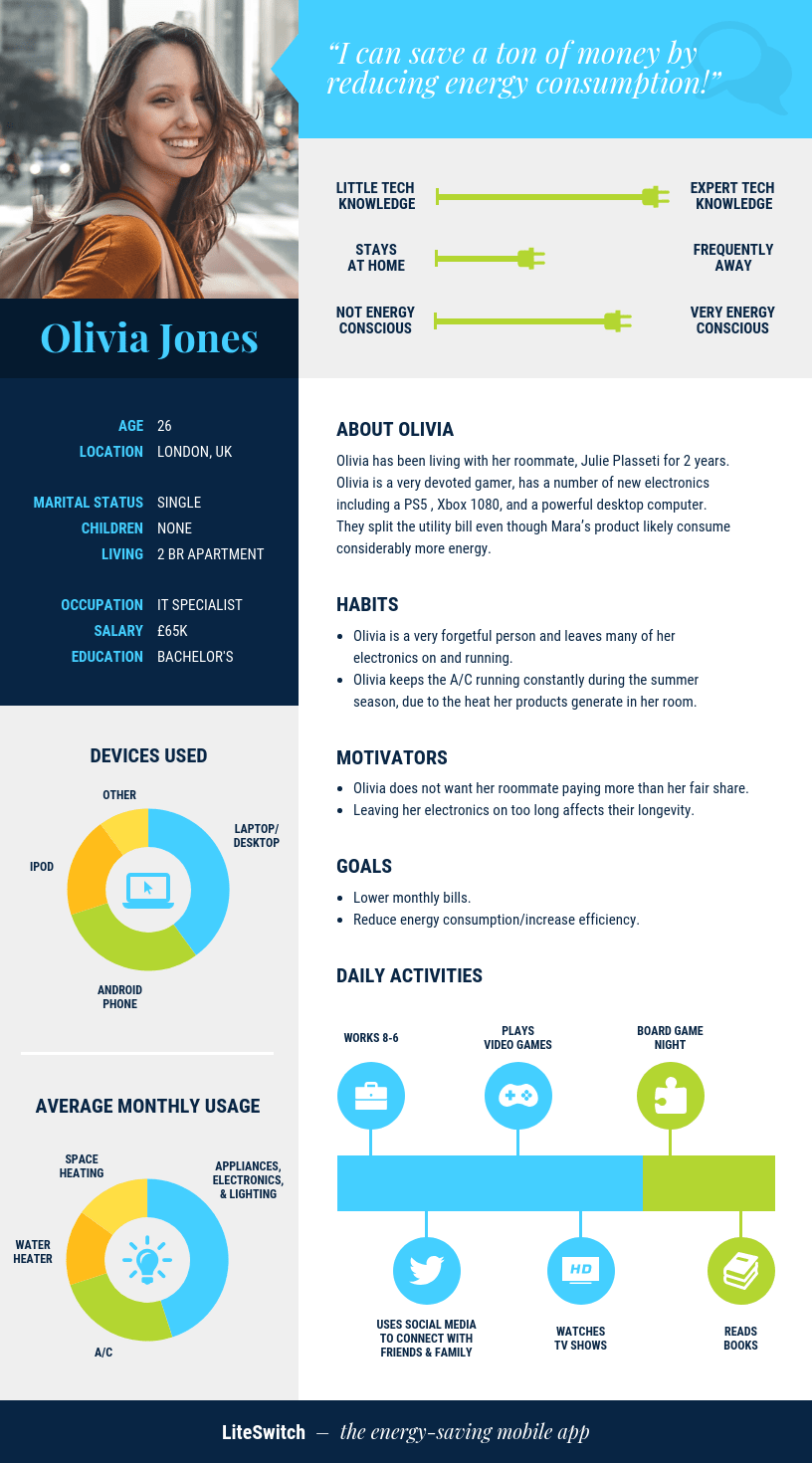
CREATE THIS REPORT TEMPLATE
Nonprofits use data visualizations to put stories and faces to numbers.
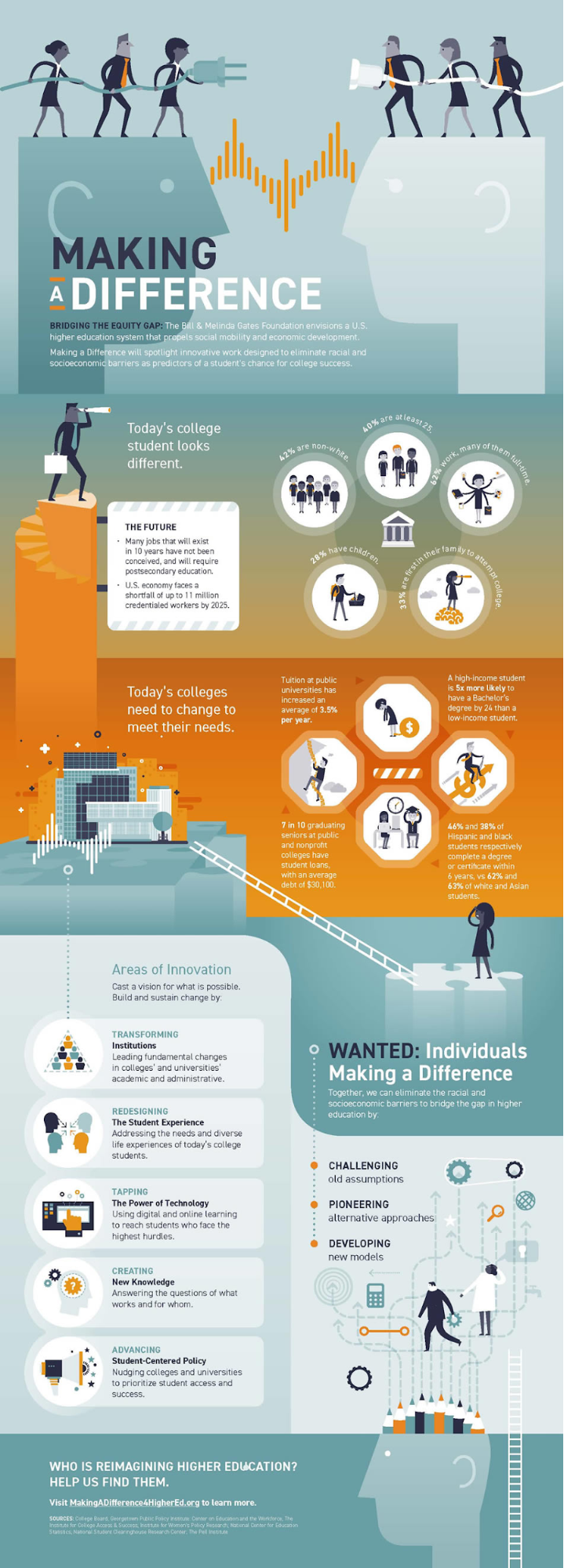
Source: Bill and Melinda Gates Foundation
Scholars and scientists use data visualization to illustrate concepts and reinforce their arguments.
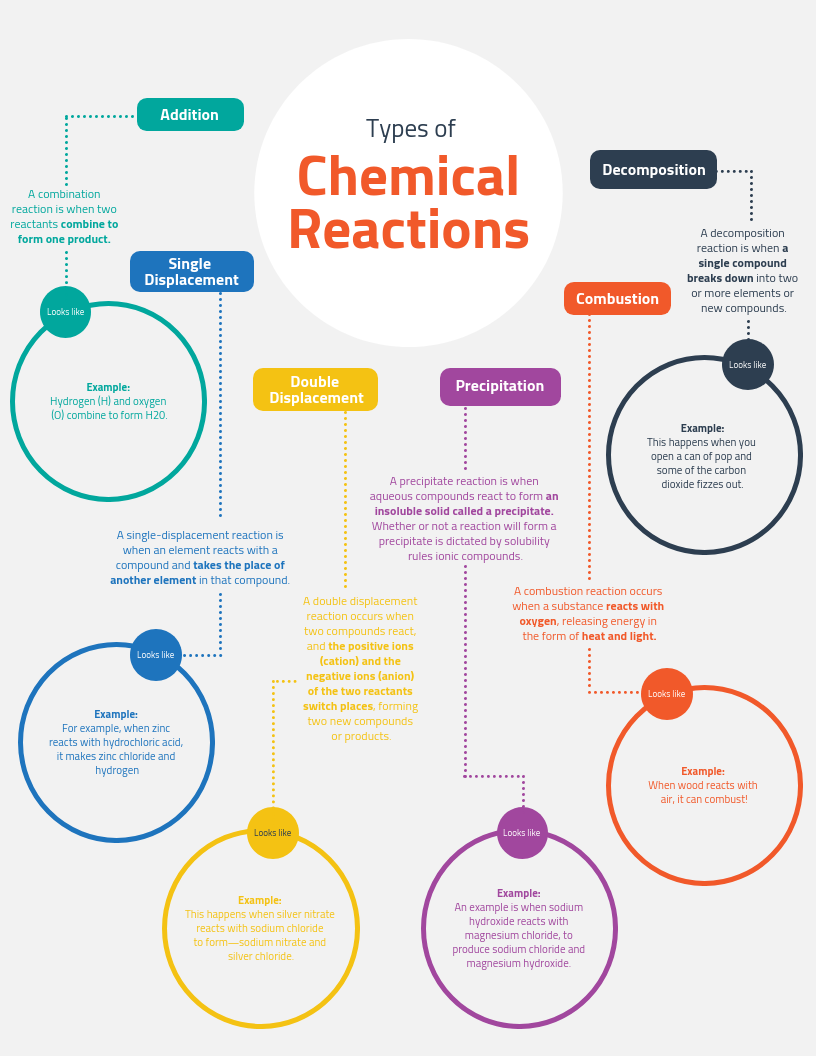
CREATE THIS MIND MAP TEMPLATE
Reporters use data visualization to show trends and contextualize stories.
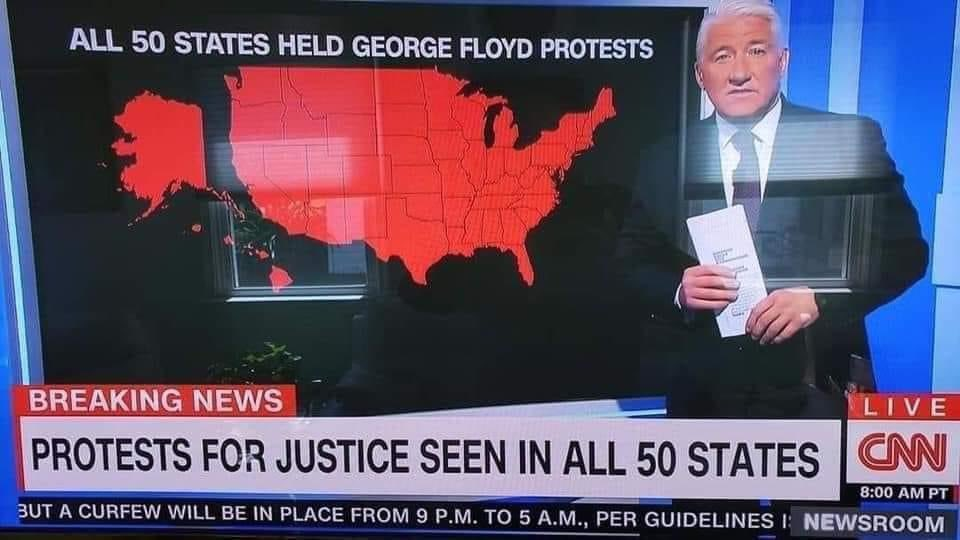
While data visualizations can make your work more professional, they can also be a lot of fun.
What is data visualization? A simple definition of data visualization:
Data visualization is the visual presentation of data or information. The goal of data visualization is to communicate data or information clearly and effectively to readers. Typically, data is visualized in the form of a chart , infographic , diagram or map.
The field of data visualization combines both art and data science. While a data visualization can be creative and pleasing to look at, it should also be functional in its visual communication of the data.
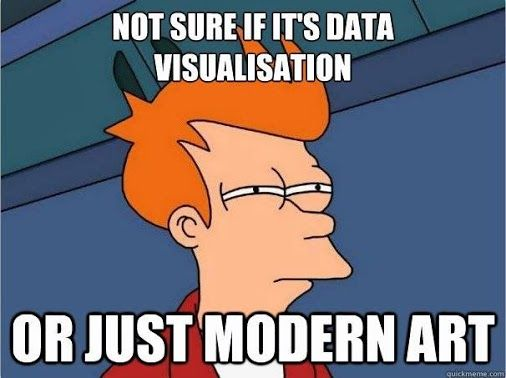
Data, especially a lot of data, can be difficult to wrap your head around. Data visualization can help both you and your audience interpret and understand data.
Data visualizations often use elements of visual storytelling to communicate a message supported by the data.
There are many situations where you would want to present data visually.
Data visualization can be used for:
- Making data engaging and easily digestible
- Identifying trends and outliers within a set of data
- Telling a story found within the data
- Reinforcing an argument or opinion
- Highlighting the important parts of a set of data
Let’s look at some examples for each use case.
1. Make data digestible and easy to understand
Often, a large set of numbers can make us go cross-eyed. It can be difficult to find the significance behind rows of data.
Data visualization allows us to frame the data differently by using illustrations, charts, descriptive text, and engaging design. Visualization also allows us to group and organize data based on categories and themes, which can make it easier to break down into understandable chunks.
Related : How to Use Data Visualization in Your Infographics
For example, this infographic breaks down the concept of neuroplasticity in an approachable way:
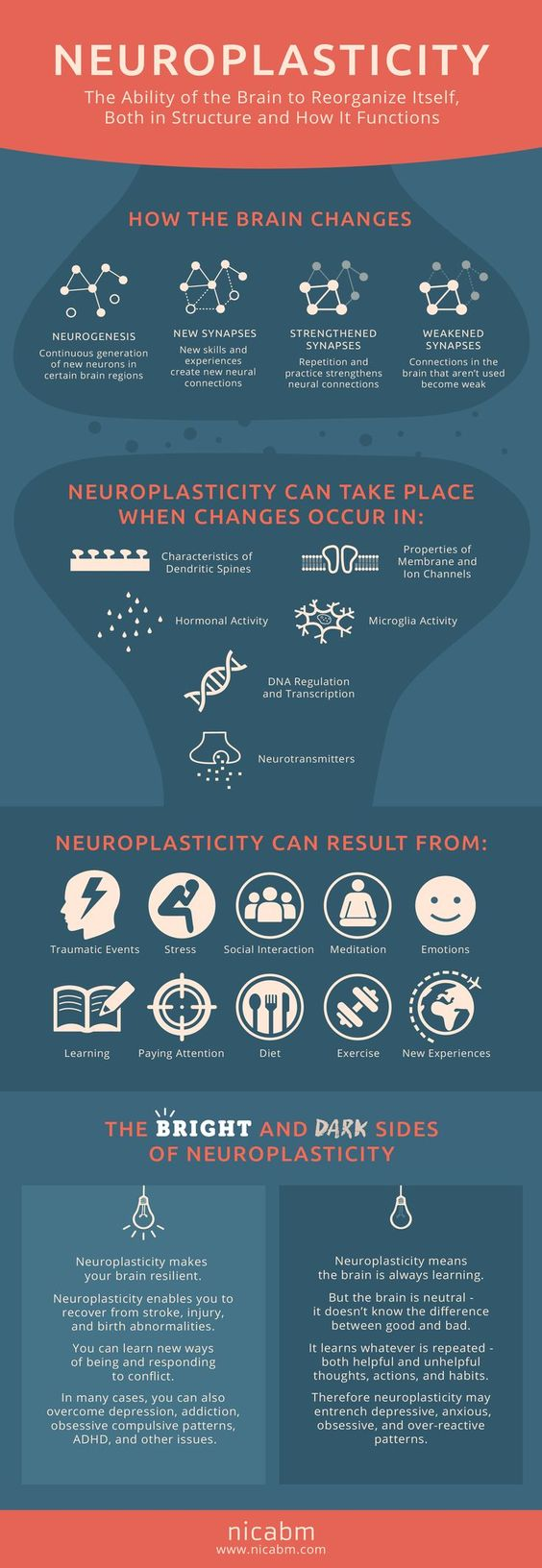
Source: NICABM
The same goes for complex, specialized concepts. It can often be difficult to break down the information in a way that non-specialists will understand. But an infographic that organizes the information, with visuals, can demystify concepts for novice readers.
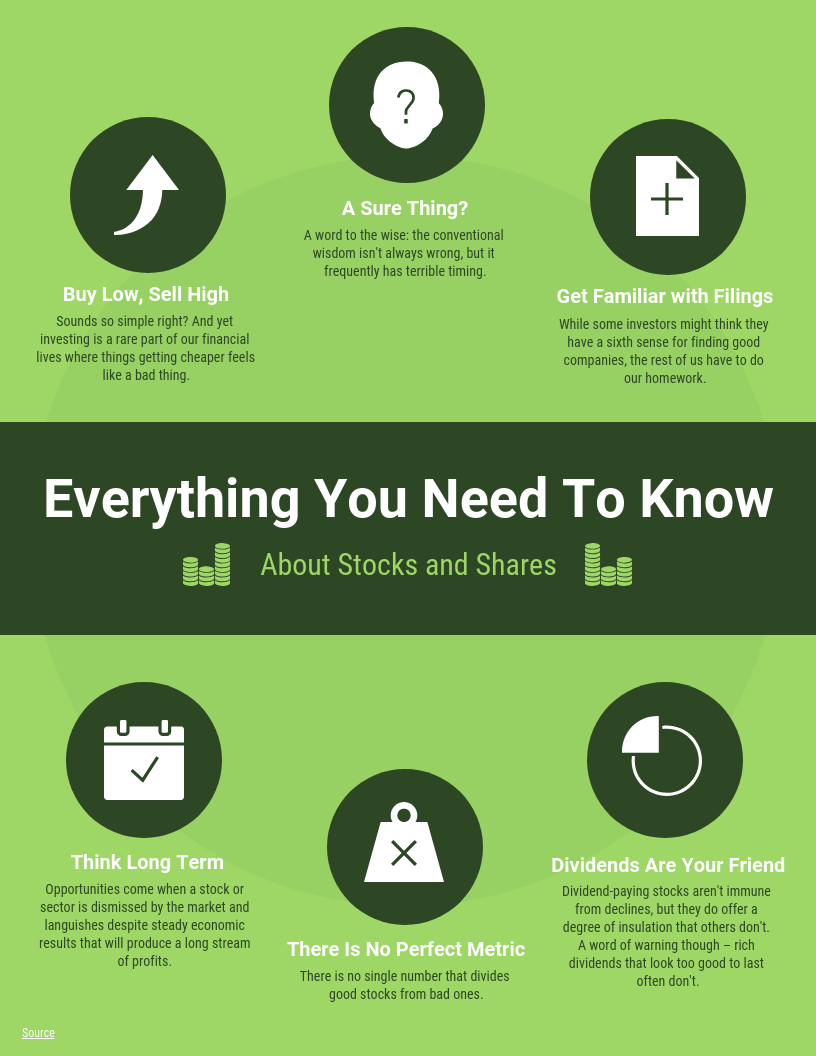
CREATE THIS INFOGRAPHIC TEMPLATE
NEW! Introducing: Marketing Statistics Report 2022
It’s 2022 already. Marketers, are you still using data from pre-COVID times?
Don’t make decisions based on outdated data that no longer applies. It’s time you keep yourself informed of the latest marketing statistics and trends during the past two years, and learn how COVID-19 has affected marketing efforts in different industries — with this FREE marketing statistics report put together by Venngage and HubSpot .
The report uses data gathered from over 100,000 customers of HubSpot CRM. In addition to that, you’ll also know about the trends in using visuals in content marketing and the impacts of the pandemic on visual content, from 200+ marketers all over the world interviewed by Venngage.
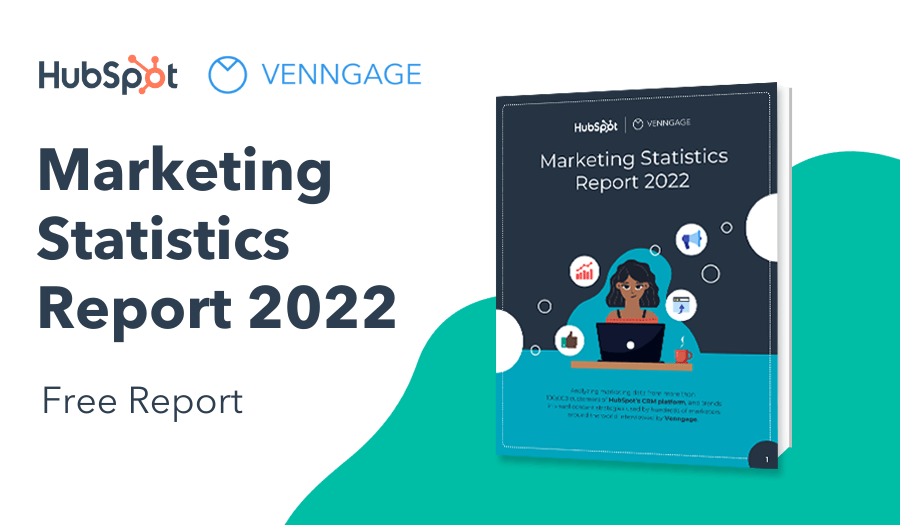
GET YOUR FREE COPY
2. Identify trends and outliers
If you were to sift through raw data manually, it could take ages to notice patterns, trends or outlying data. But by using data visualization tools like charts, you can sort through a lot of data quickly.
Even better, charts enable you to pick up on trends a lot quicker than you would sifting through numbers.
For example, here’s a simple chart generated by Google Search Console that shows the change in Google searches for “toilet paper”. As you can see, in March 2020 there was a huge increase in searches for toilet paper:
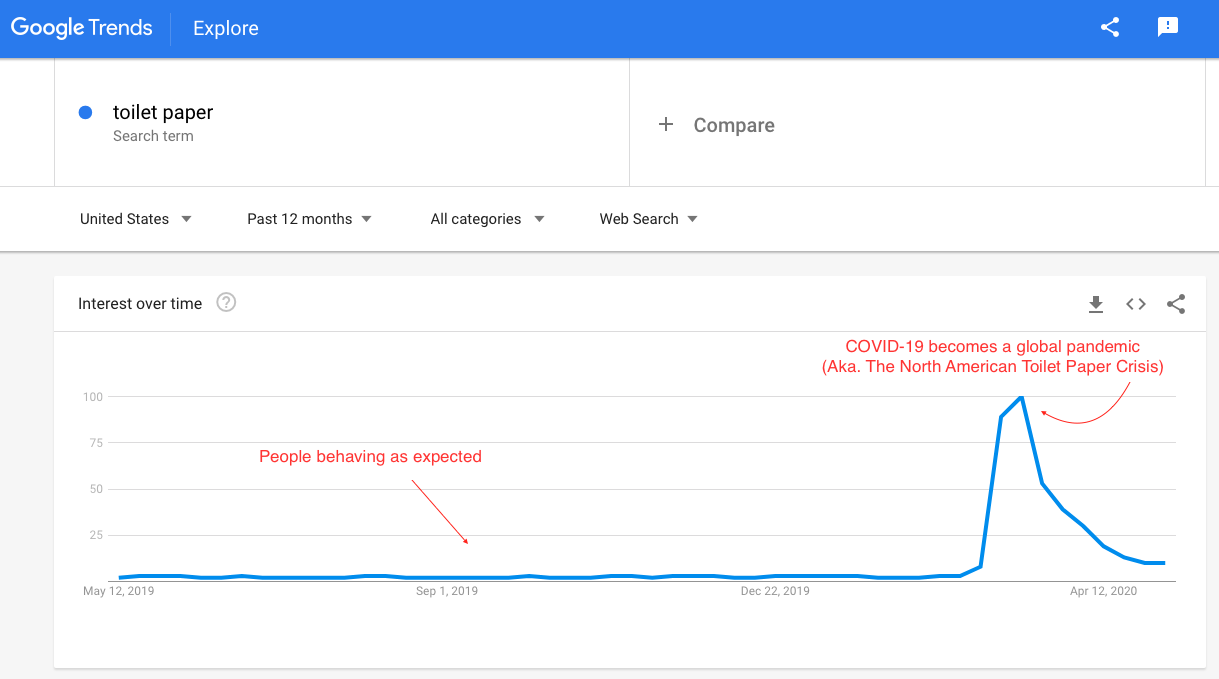
Source: How to Use SEO Data to Fuel Your Content Marketing Strategy in 2020
This chart shows an outlier in the general trend for toilet paper-related Google searches. The reason for the outlier? The outbreak of COVID-19 in North America. With a simple data visualization, we’ve been able to highlight an outlier and hint at a story behind the data.
Uploading your data into charts, to create these kinds of visuals is easy. While working on your design in the editor, select a chart from the left panel. Open the chart and find the green IMPORT button under the DATA tab. Then upload the CSV file and your chart automatically visualizes the information.
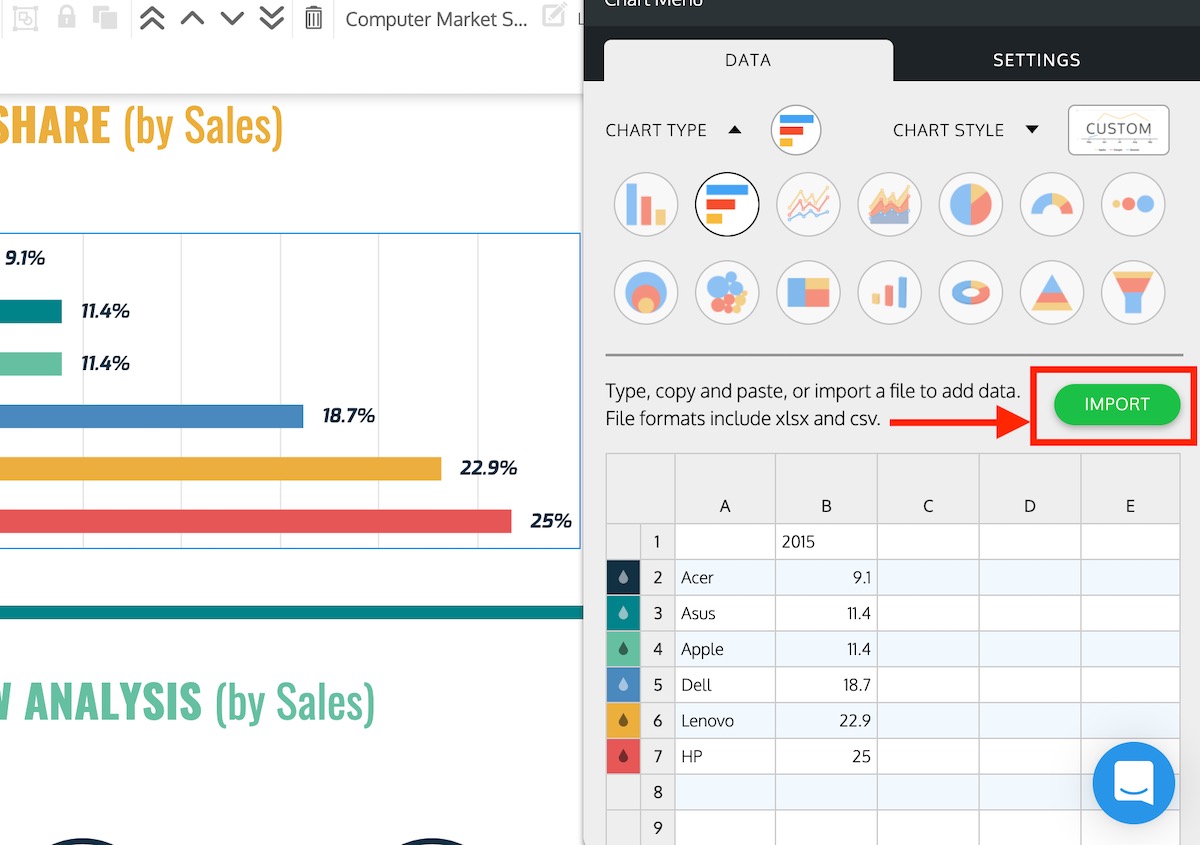
3. Tell a story within the data
Numbers on their own don’t tend to evoke an emotional response. But data visualization can tell a story that gives significance to the data.
Designers use techniques like color theory , illustrations, design style and visual cues to appeal to the emotions of readers, put faces to numbers, and introduce a narrative to the data.
Related : How to Tell a Story With Data (A Guide for Beginners)
For example, here’s an infographic created by World Vision. In the infographics, numbers are visualized using illustrations of cups. While comparing numbers might impress readers, reinforcing those numbers with illustrations helps to make an even greater impact.
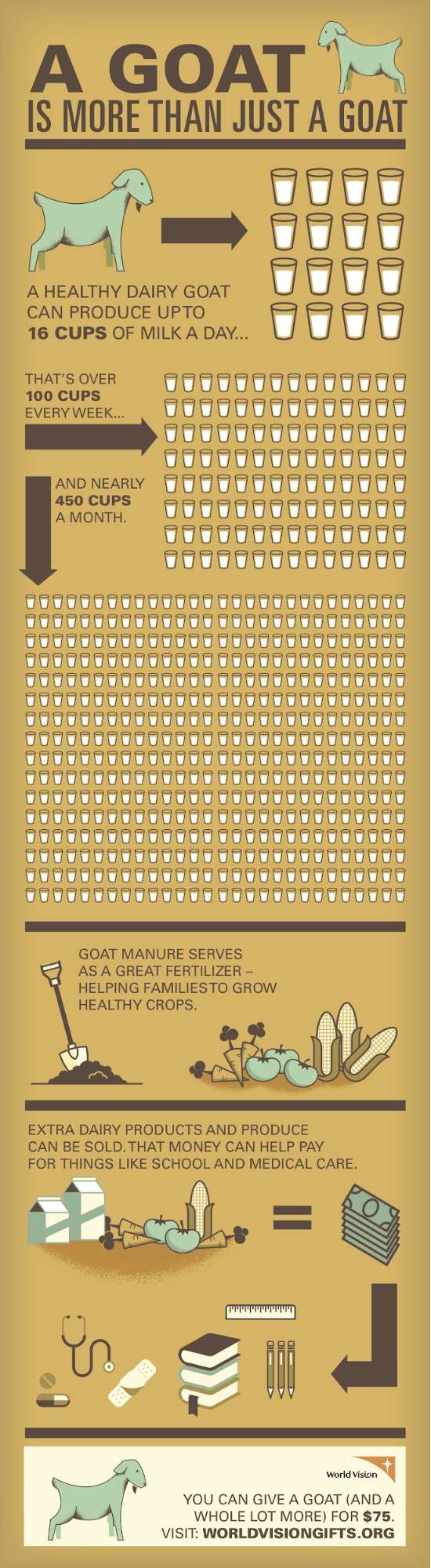
Source: World Vision
Meanwhile, this infographic uses data to draw attention to an often overlooked issue:

Read More: The Coronavirus Pandemic and the Refugee Crisis
4. Reinforce an argument or opinion
When it comes to convincing people your opinion is right, they often have to see it to believe it. An effective infographic or chart can make your argument more robust and reinforce your creativity.
For example, you can use a comparison infographic to compare sides of an argument, different theories, product/service options, pros and cons, and more. Especially if you’re blending data types.
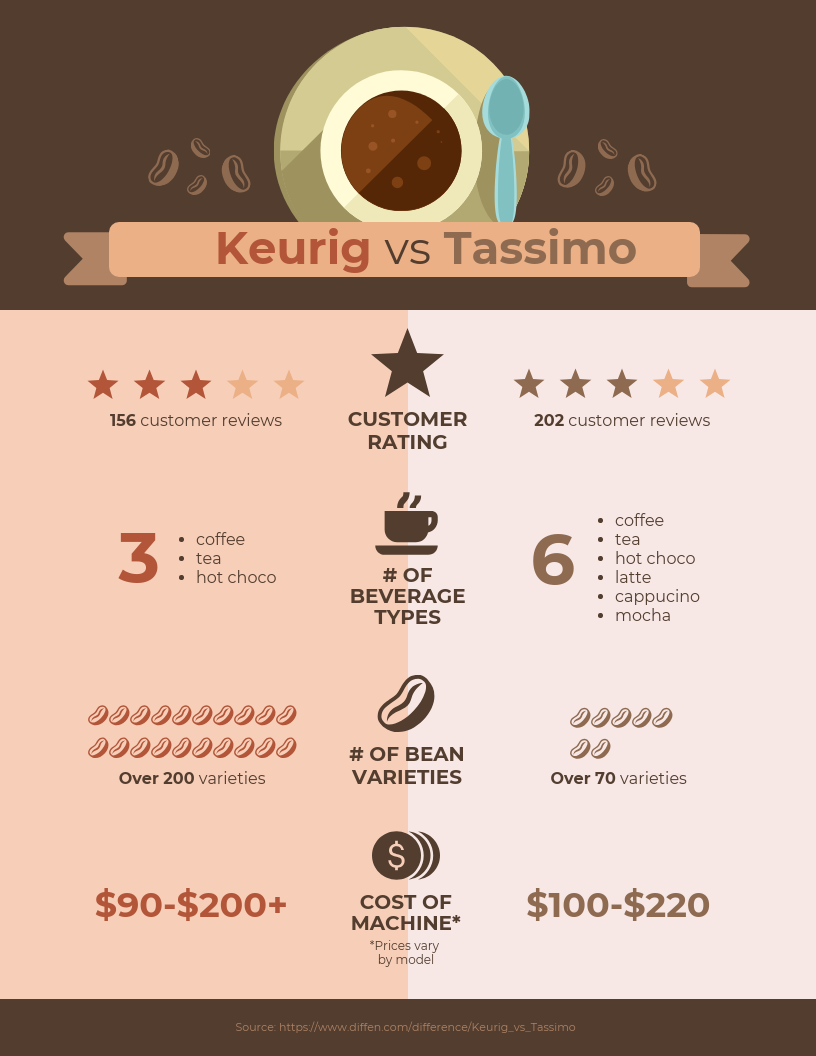
5. Highlight an important point in a set of data
Sometimes we use data visualizations to make it easier for readers to explore the data and come to their own conclusions. But often, we use data visualizations to tell a story, make a particular argument, or encourage readers to come to a specific conclusion.
Designers use visual cues to direct the eye to different places on a page. Visual cues are shapes, symbols, and colors that point to a specific part of the data visualization, or that make a specific part stand out.
For example, in this data visualization, contrasting colors are used to emphasize the difference in the amount of waste sent to landfills versus recycled waste:
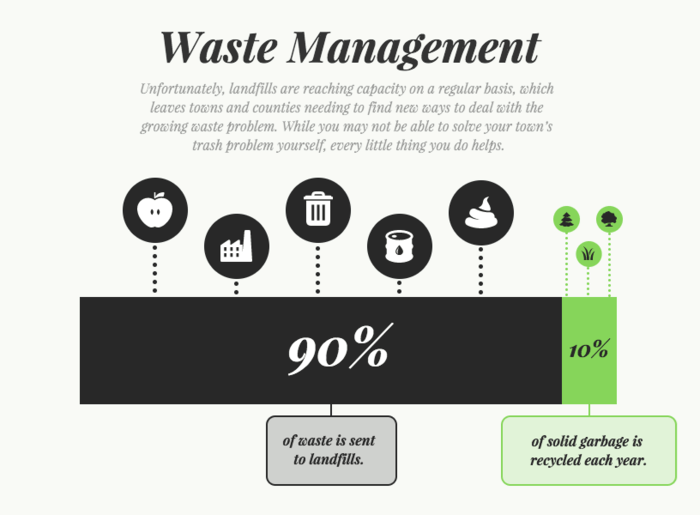
Here’s another example. This time, a red circle and an arrow are used to highlight points on the chart where the numbers show a drop:
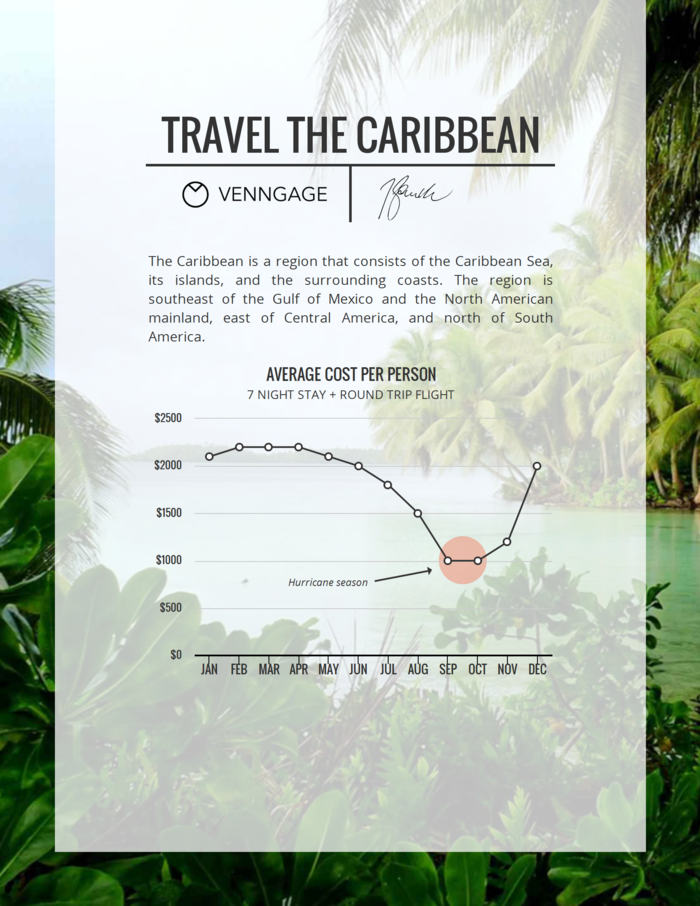
Highlighting specific data points helps your data visualization tell a compelling story.
6. Make books, blog posts, reports and videos more engaging
At Venngage, we use data visualization to make our blog posts more engaging for readers. When we write a blog post or share a post on social media, we like to summarize key points from our content using infographics.
The added benefit of creating engaging visuals like infographics is that it has enabled our site to be featured in publications like The Wall Street Journal , Mashable , Business Insider , The Huffington Post and more.
That’s because data visualizations are different from a lot of other types of content people consume on a daily basis. They make your brain work. They combine concrete facts and numbers with impactful visual elements. They make complex concepts easier to grasp.
Here’s an example of an infographic we made that got a lot of media buzz:
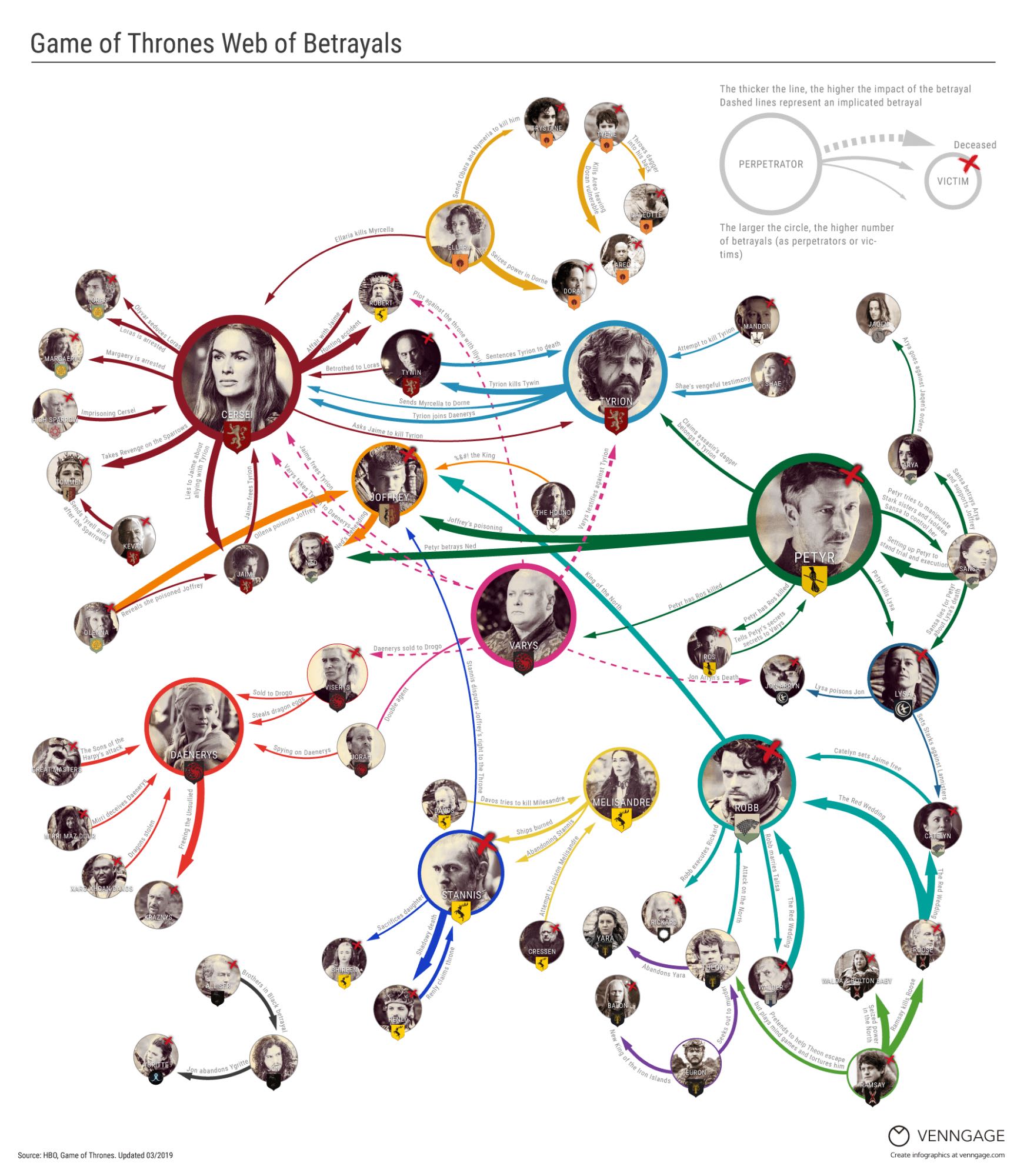
Read the Blog Post: Every Betrayal Ever in Game of Thrones
We created this infographic because a bunch of people on our team are big Game of Thrones fans and we wanted to create a visual that would help other fans follow the show. Because we approached a topic that a lot of people cared about in an original way, the infographic got picked up by a bunch of media sites.
Whether you’re a website looking to promote your content, a journalist looking for an original angle, or a creative building your portfolio, data visualizations can be an effective way to get people’s attention.
Data visualizations can come in many different forms. People are always coming up with new and creative ways to present data visually.
Generally speaking, data visualizations usually fall under these main categories:
An infographic is a collection of imagery, charts, and minimal text that gives an easy-to-understand overview of a topic.
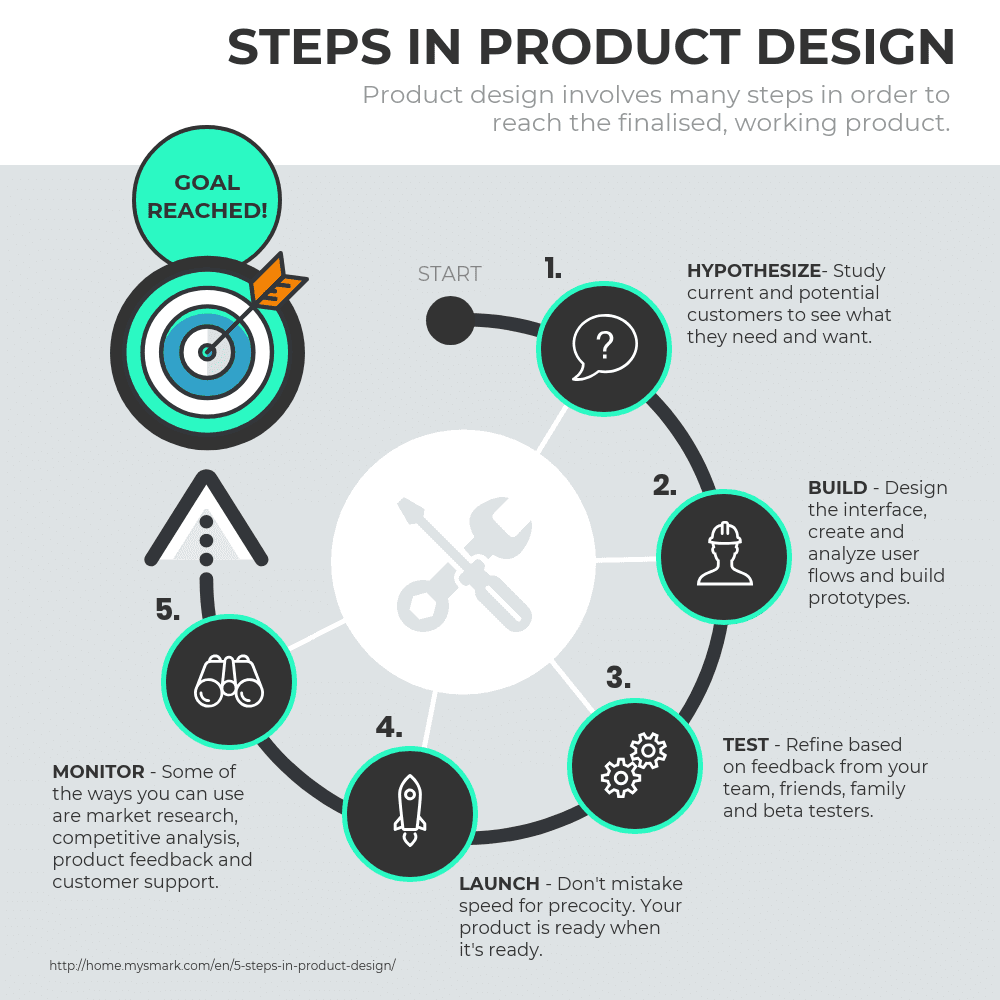
While infographics can take many forms, they can typically be categorized by these infographic types:
- Statistical infographics
- Informational infographics
- Timeline infographics
- Process infographics
- Geographic infographics
- Comparison infographics
- Hierarchical infographics
- List infographics
- Resume infographics
Read More: What is an Infographic? Examples, Templates & Design Tips
Charts
In the simplest terms, a chart is a graphical representation of data. Charts use visual symbols like line, bars, dots, slices, and icons to represent data points.
Some of the most common types of charts are:
- Bar graphs /charts
- Line charts
- Bubble charts
- Stacked bar charts
- Word clouds
- Pictographs
- Area charts
- Scatter plot charts
- Multi-series charts
The question that inevitably follows is: what type of chart should I use to visualize my data? Does it matter?
Short answer: yes, it matters. Choosing a type of chart that doesn’t work with your data can end up misrepresenting and skewing your data.
For example: if you’ve been in the data viz biz for a while, then you may have heard some of the controversy surrounding pie charts. A rookie mistake that people often make is using a pie chart when a bar chart would work better.
Pie charts display portions of a whole. A pie chart works when you want to compare proportions that are substantially different. Like this:
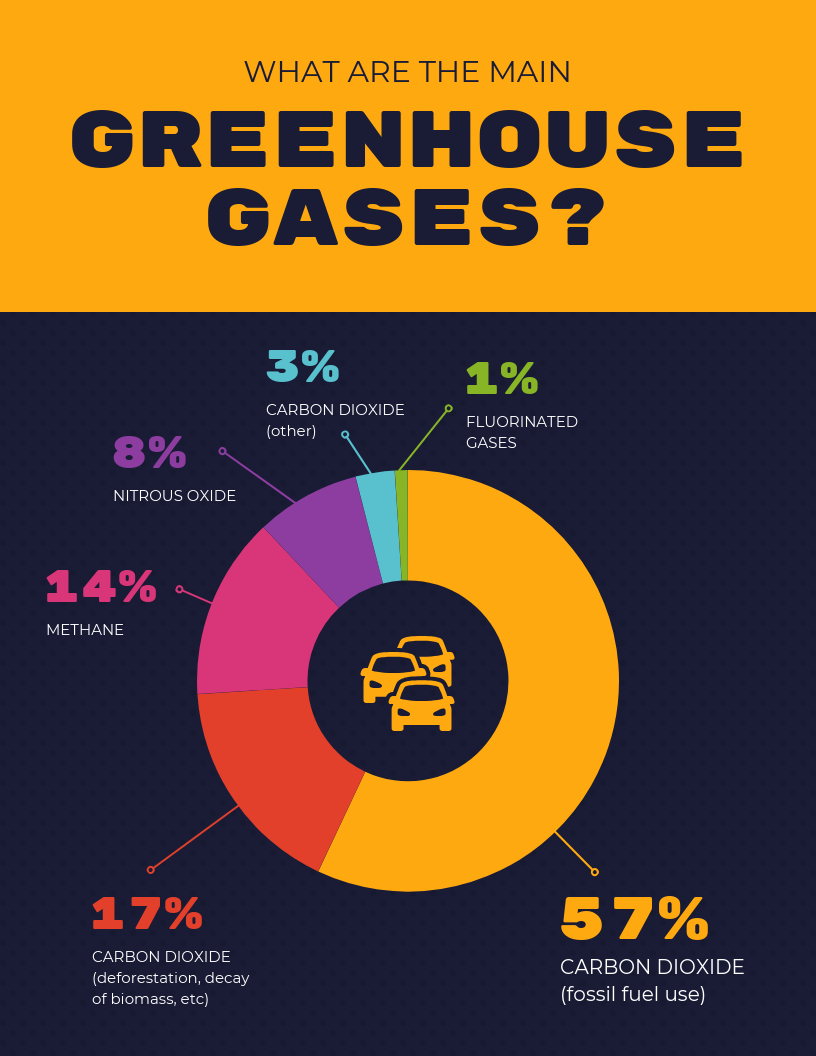
CREATE THIS CHART TEMPLATE
But when your proportions are similar, a pie chart can make it difficult to tell which slice is bigger than the other. That’s why, in most other cases, a bar chart is a safer bet.
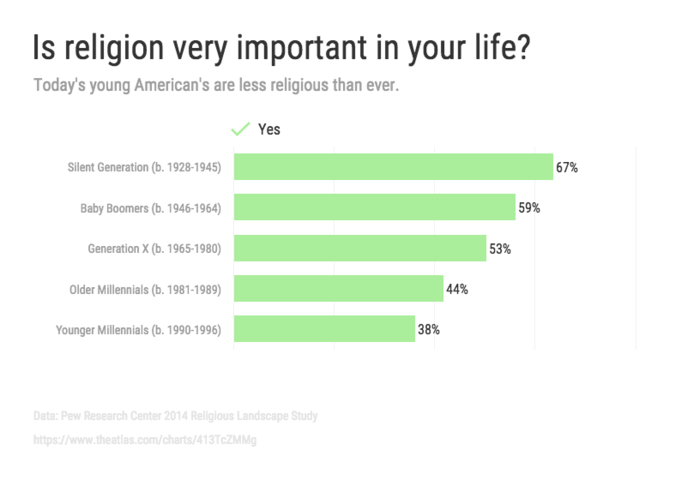
Here is a cheat sheet to help you pick the right type of chart for your data:
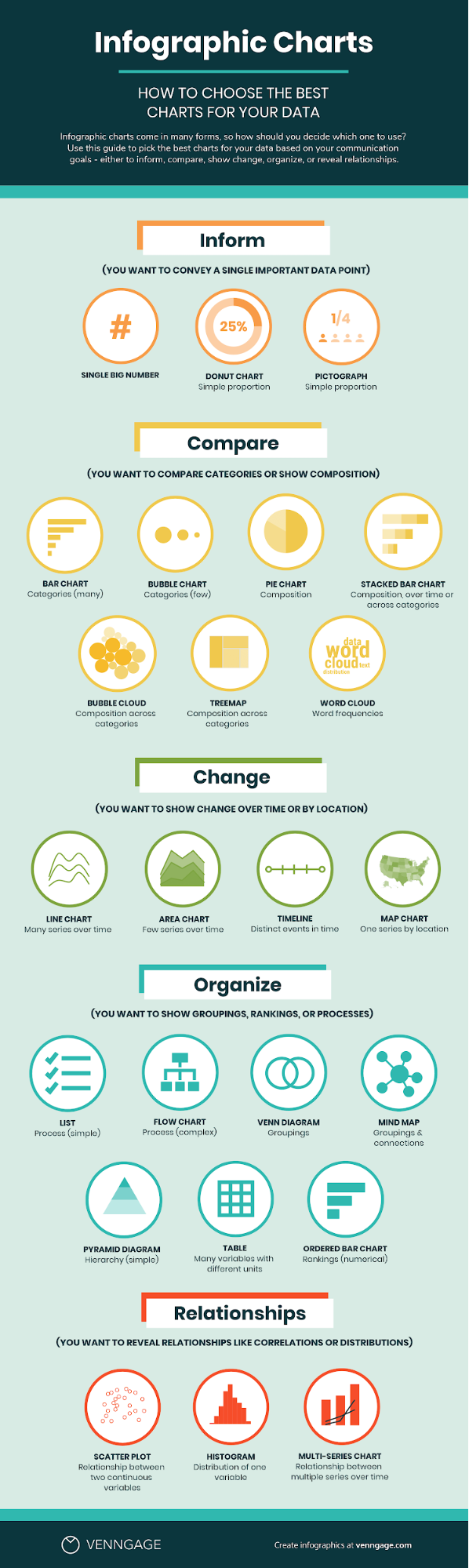
Want to make better charts? Make engaging charts with Venngage’s Chart Maker .
Related : How to Choose the Best Types of Charts For Your Data
Similar to a chart, a diagram is a visual representation of information. Diagrams can be both two-dimensional and three-dimensional.
Some of the most common types of diagrams are:
- Venn diagrams
- Tree diagrams
- SWOT analysis
- Fishbone diagrams
- Use case diagrams
Diagrams are used for mapping out processes, helping with decision making, identifying root causes, connecting ideas, and planning out projects.
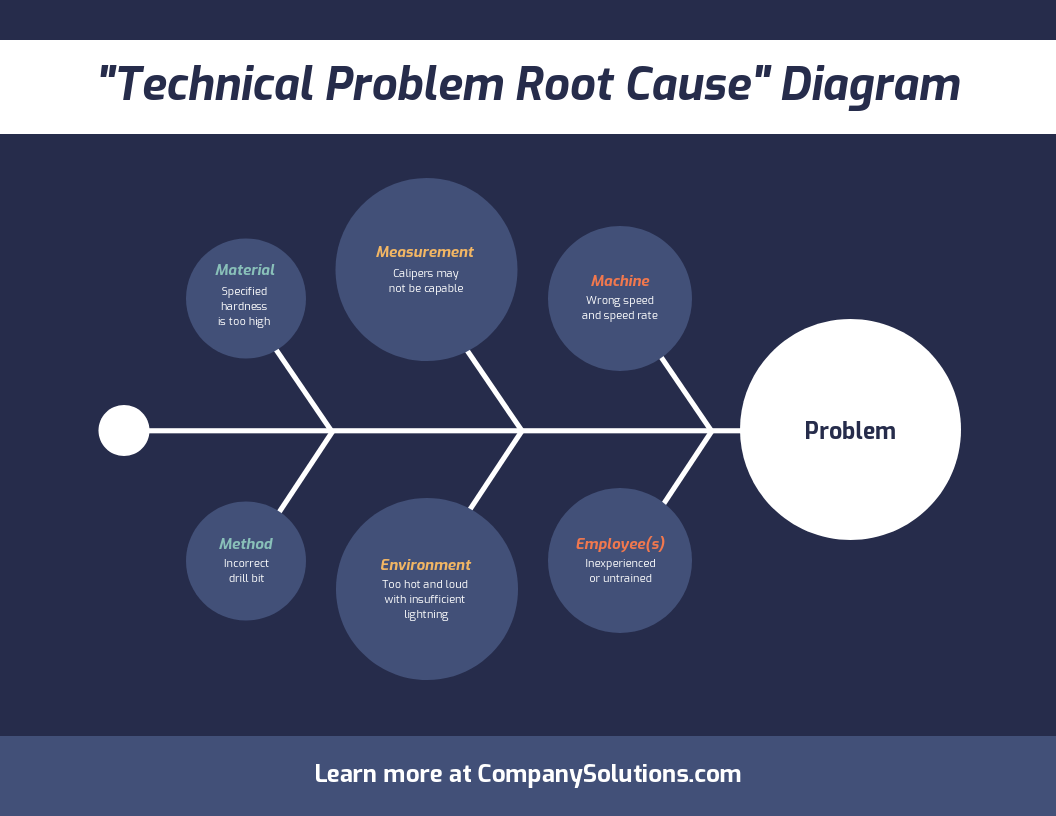
CREATE THIS DIAGRAM TEMPLATE
Want to make a diagram ? Create a Venn diagram and other visuals using our free Venn Diagram Maker .
A map is a visual representation of an area of land. Maps show physical features of land like regions, landscapes, cities, roads, and bodies of water.
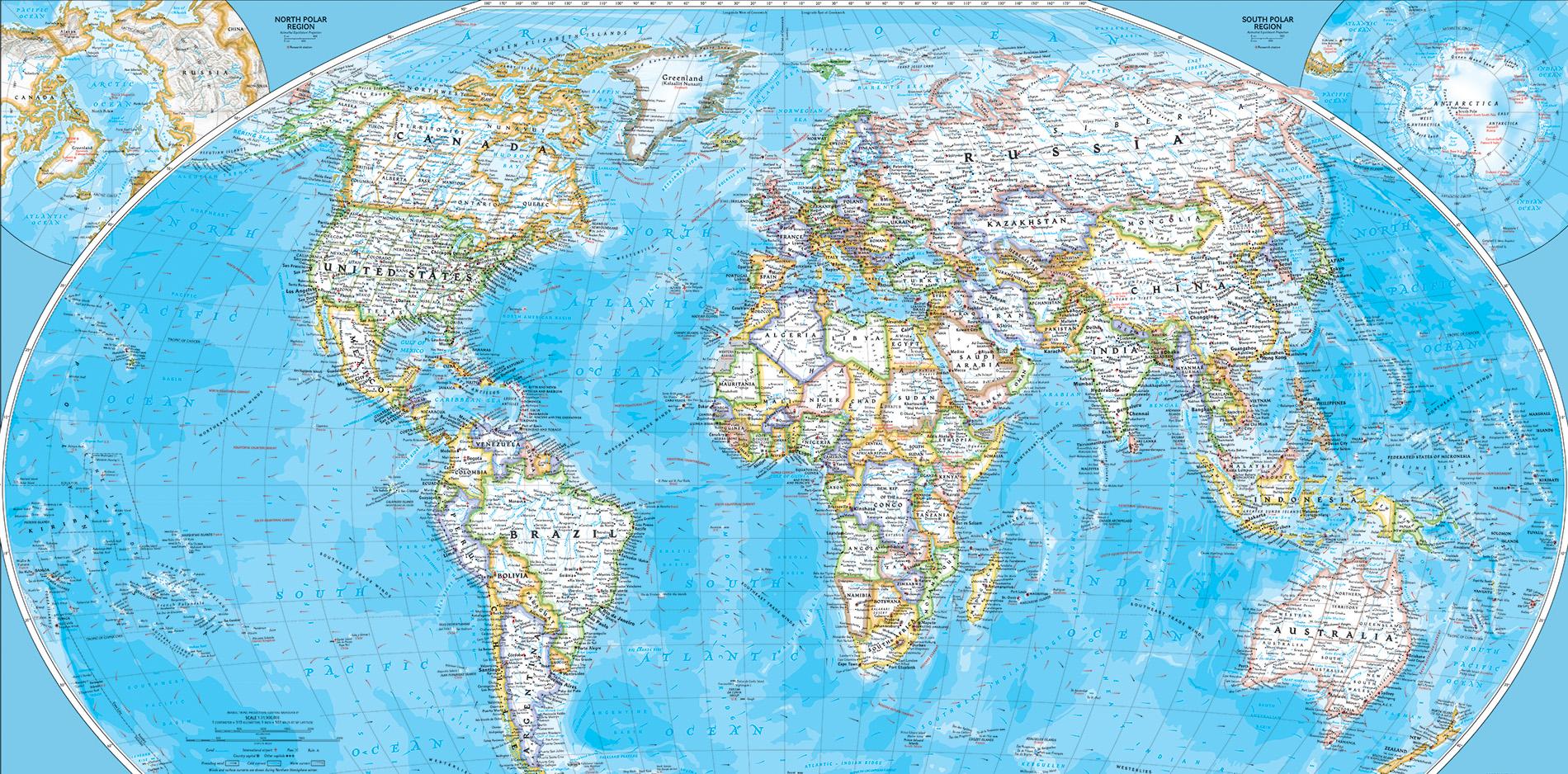
Source: National Geographic
A common type of map you have probably come across in your travels is a choropleth map . Choropleth maps use different shades and colors to indicate average quantities.
For example, a population density map uses varying shades to show the difference in population numbers from region to region:
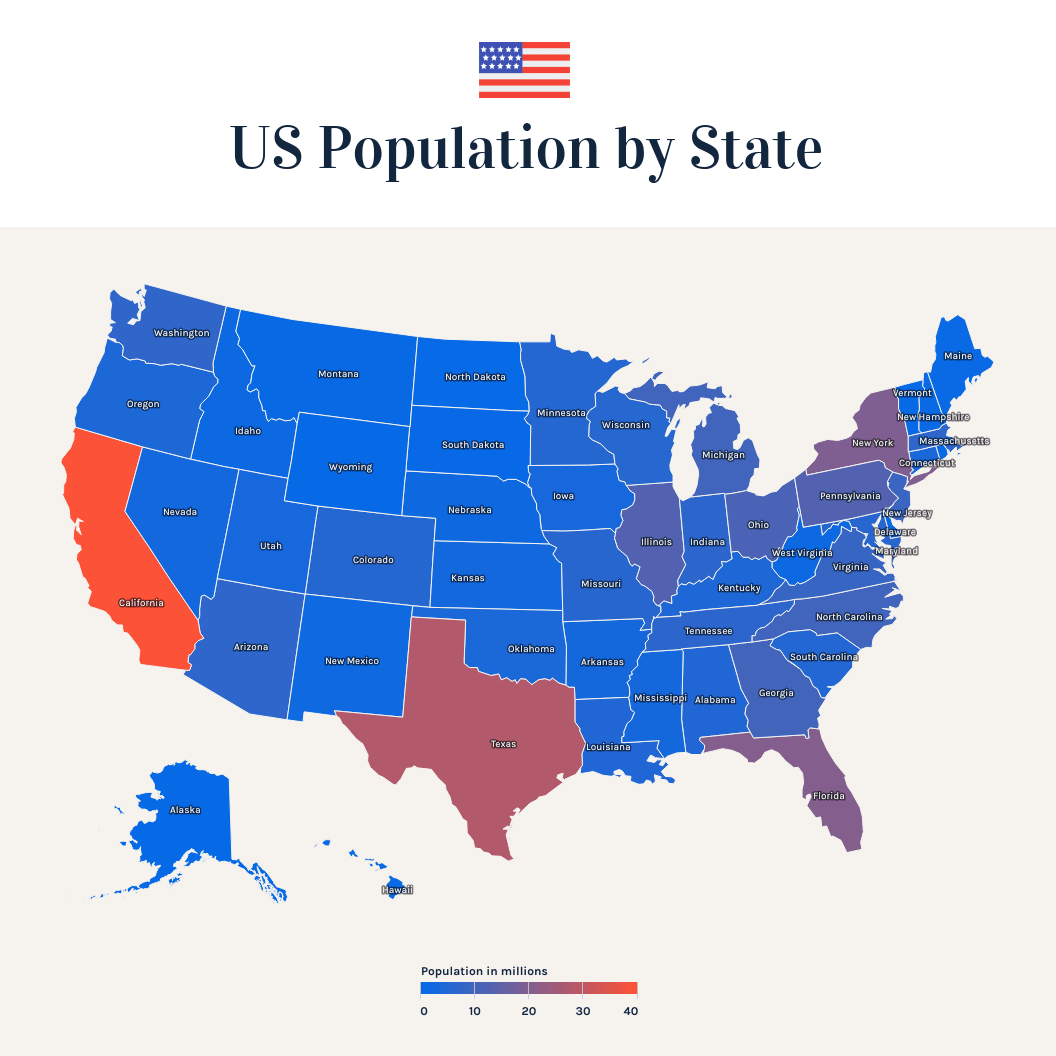
Create your own map for free with Venngage’s Map Maker .
How to present data visually (data visualization best practices)
While good data visualization will communicate data or information clearly and effectively, bad data visualization will do the opposite. Here are some practical tips for how businesses and organizations can use data visualization to communicate information more effectively.
Not a designer? No problem. Venngage’s Graph Maker will help you create better graphs in minutes.
1. Avoid distorting the data
This may be the most important point in this whole blog post. While data visualizations are an opportunity to show off your creative design chops, function should never be sacrificed for fashion.
The chart styles, colors, shapes, and sizing you use all play a role in how the data is interpreted. If you want to present your data accurately and ethically, then you need to take care to ensure that your data visualization does not present the data falsely.
There are a number of different ways data can be distorted in a chart. Some common ways data can be distorted are:
- Making the baselines something other than 0 to make numbers seem bigger or smaller than they are – this is called “truncating” a graph
- Compressing or expanding the scale of the Y-axis to make a line or bar seem bigger or smaller than it should be
- Cherry picking data so that only the data points you want to include are on a graph (i.e. only telling part of the story)
- Using the wrong type of chart, graph or diagram for your data
- Going against standard, expected data visualization conventions
Because people use data visualizations to reinforce their opinions, you should always read data visualizations with a critical eye. Often enough, writers may be using data visualization to skew the data in a way that supports their opinions, but that may not be entirely truthful.

Read More: 5 Ways Writers Use Graphs To Mislead You
Want to create an engaging line graph? Use Venngage’s Line Graph Maker to create your own in minutes.
2. Avoid cluttering up your design with “chartjunk”
When it comes to best practices for data visualization, we should turn to one of the grandfather’s of data visualization: Edward Tufte. He coined the term “ chartjunk ”, which refers to the use of unnecessary or confusing design elements that skews or obscures the data in a chart.
Here’s an example of a data visualization that suffers from chartjunk:
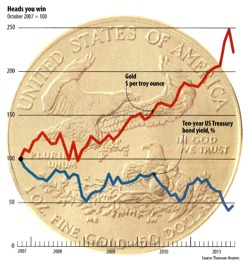
Source: ExcelUser
In this example, the image of the coin is distracting for readers trying to interpret the data. Note how the fonts are tiny – almost unreadable. Mistakes like this are common when a designers tries to put style before function.
Read More : The Worst Infographics of 2020 (With Lessons for 2021)
3. Tell a story with your data
Data visualizations like infographics give you the space to combine data and narrative structure in one page. Visuals like icons and bold fonts let you highlight important statistics and facts.
For example, you could customize this data visualization infographic template to show the benefit of using your product or service (and post it on social media):
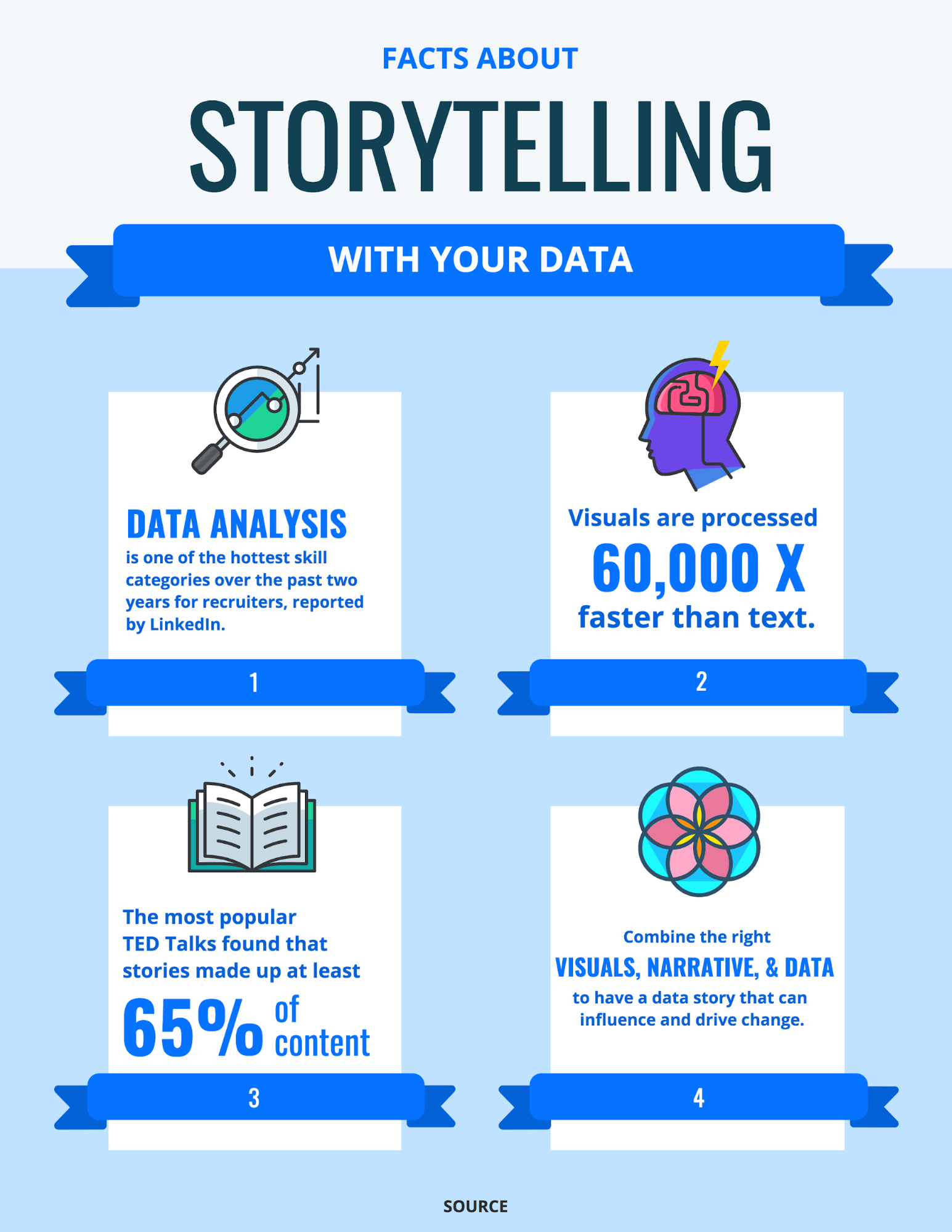
USE THIS TEMPLATE
This data visualization relies heavily on text and icons to tell the story of its data:
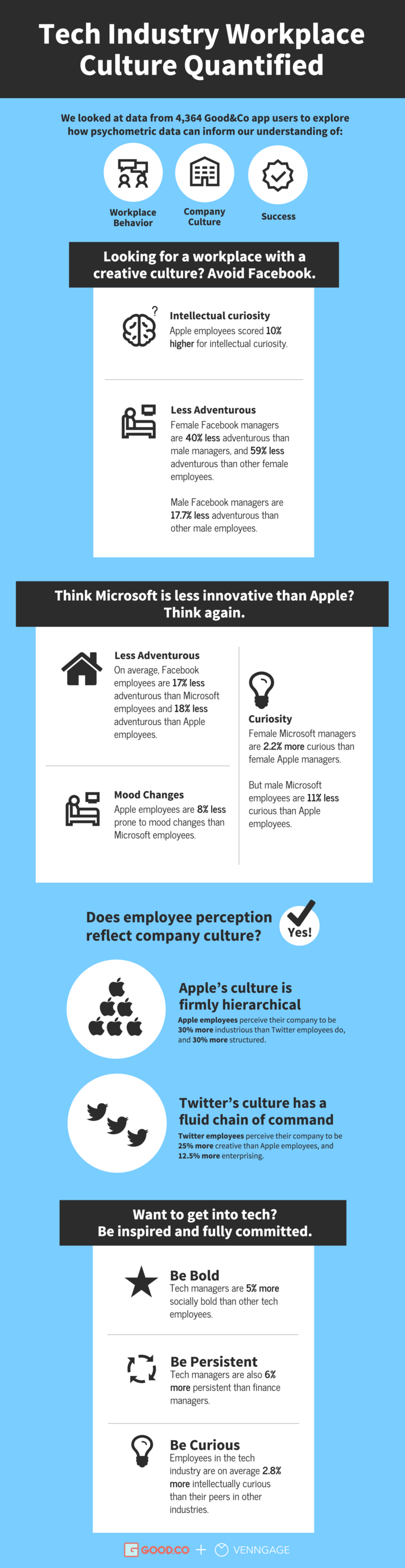
This type of infographic is perfect for those who aren’t as comfortable with charts and graphs. It’s also a great way to showcase original research, get social shares and build brand awareness.
4. Combine different types of data visualizations
While you may choose to keep your data visualization simple, combining multiple types of charts and diagrams can help tell a more rounded story.
Don’t be afraid to combine charts, pictograms and diagrams into one infographic. The result will be a data visualization infographic that is engaging and rich in visual data.
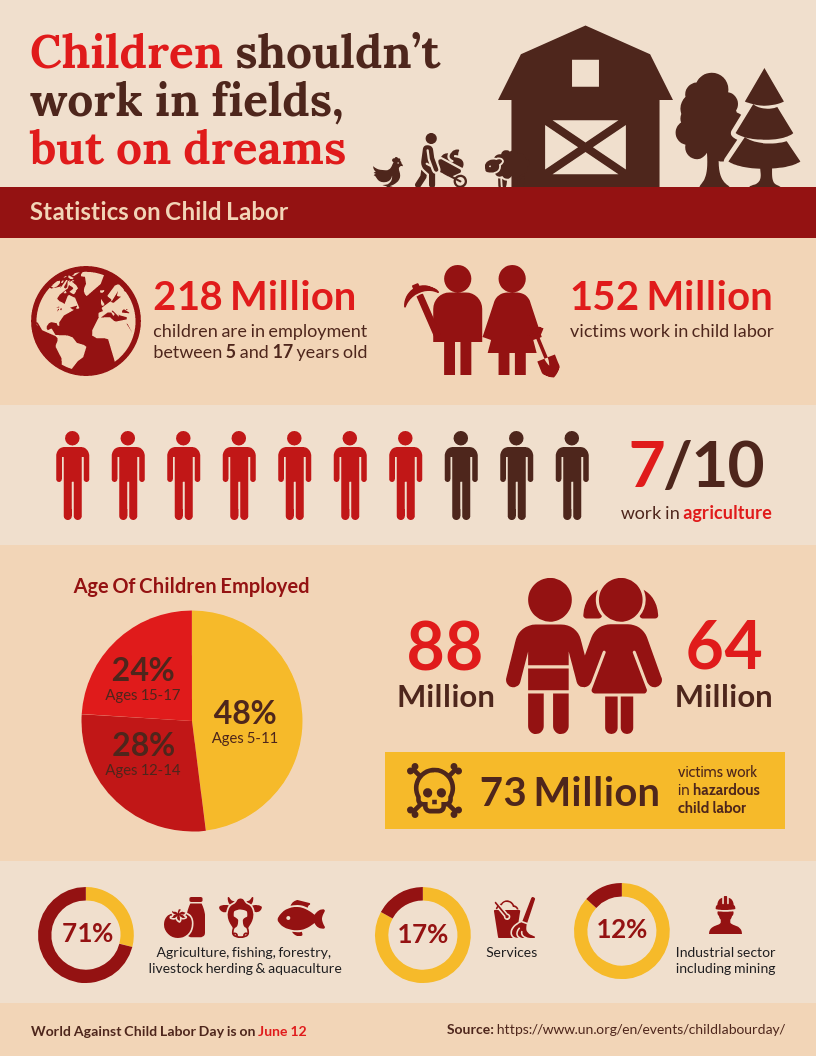
Design Tip: This data visualization infographic would be perfect for nonprofits to customize and include in an email newsletter to increase awareness (and donations).
Or take this data visualization that also combines multiple types of charts, pictograms, and images to engage readers. It could work well in a presentation or report on customer research, customer service scores, quarterly performance and much more:
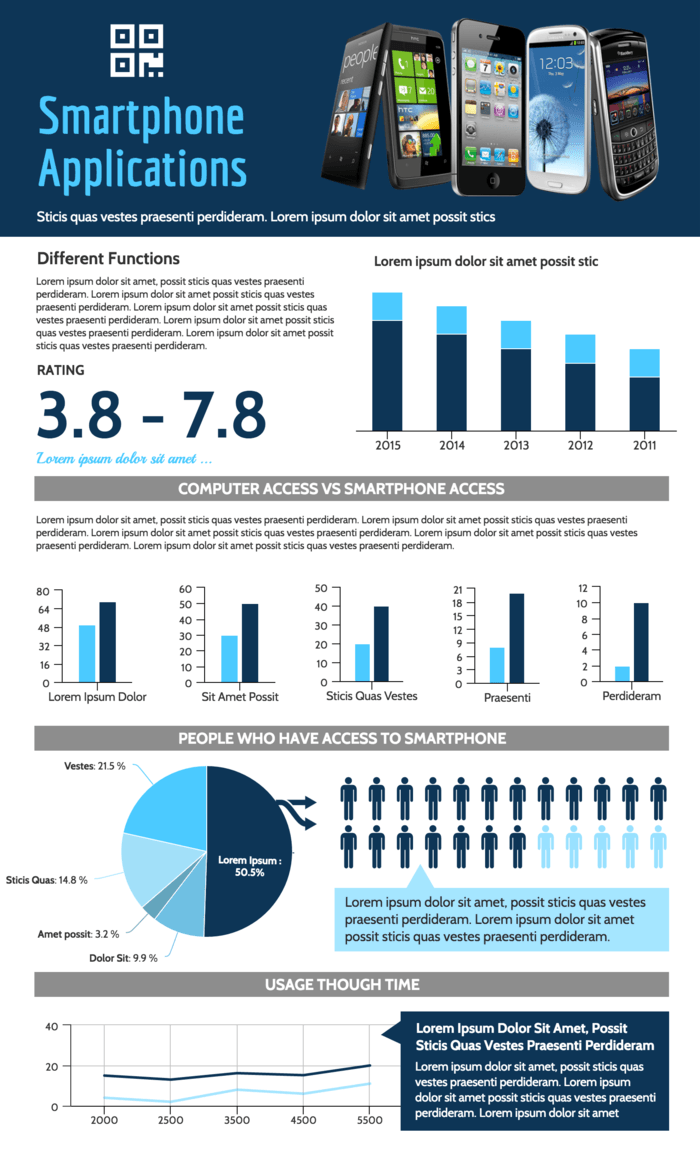
Design Tip: This infographic could work well in a presentation or report on customer research, customer service scores, quarterly performance and much more.
Make your own bar graph in minutes with our free Bar Graph Maker .
5. Use icons to emphasize important points
Icons are perfect for attracting the eye when scanning a page. (Remember: use visual cues!)
If there are specific data points that you want readers to pay attention to, placing an icon beside it will make it more noticeable:

Design Tip: This infographic template would work well on social media to encourage shares and brand awareness.
You can also pair icons with headers to indicate the beginning of a new section.
Meanwhile, this infographic uses icons like bullet points to emphasize and illustrate important points.
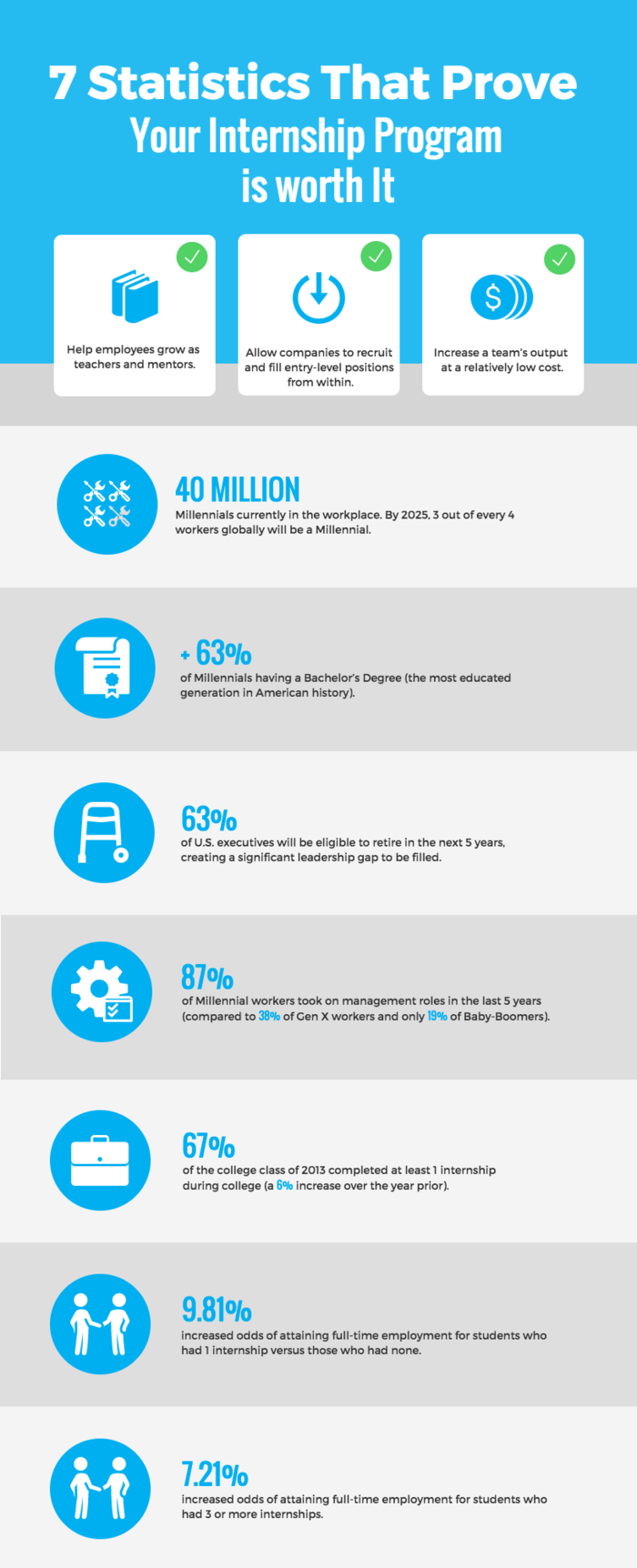
Design Tip: This infographic would make a great sales piece to promote your course or other service.
6. Use bold fonts to make text information engaging
A challenge people often face when setting out to visualize information is knowing how much text to include. After all, the point of data visualization is that it presents information visually, rather than a page of text.
Even if you have a lot of text information, you can still create present data visually. Use bold, interesting fonts to make your data exciting. Just make sure that, above all else, your text is still easy to read.
This data visualization uses different fonts for the headers and body text that are bold but clear. This helps integrate the text into the design and emphasizes particular points:
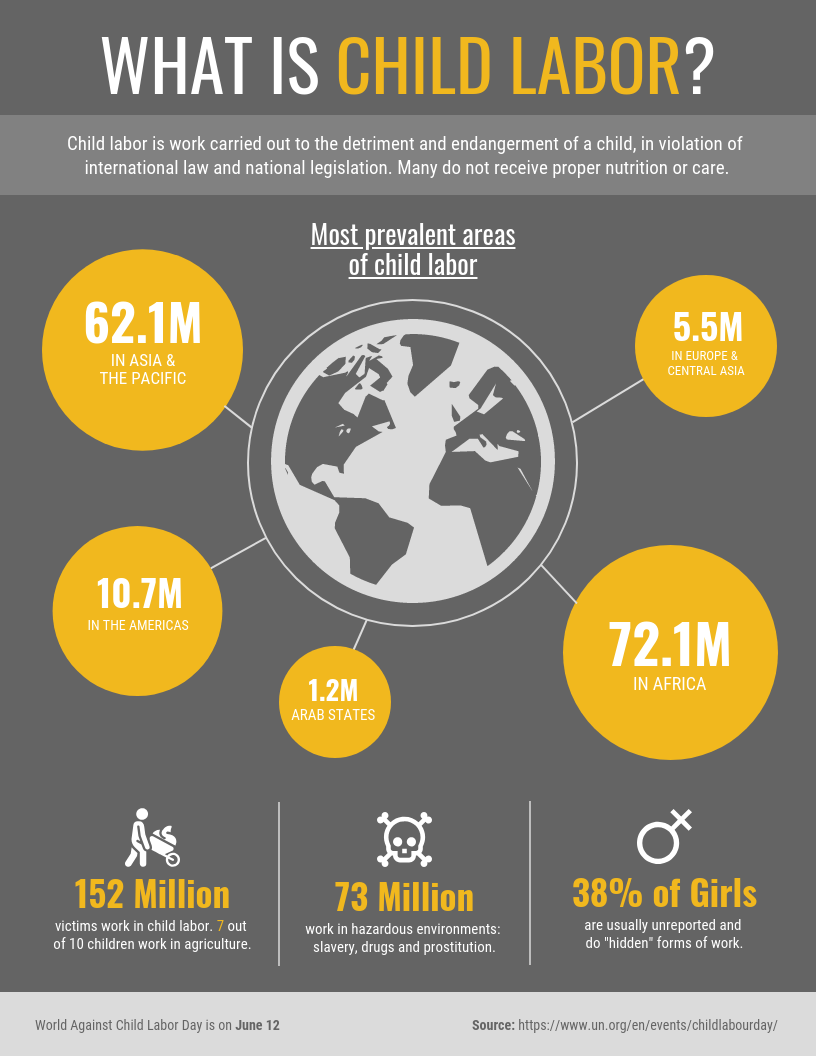
Design Tip: Nonprofits could use this data visualization infographic in a newsletter or on social media to build awareness, but any business could use it to explain the need for their product or service.
As a general rule of thumb, stick to no more than three different font types in one infographic.
This infographic uses one font for headers, another font for body text, and a third font for accent text.
Read More: How to Choose Fonts For Your Designs (With Examples)
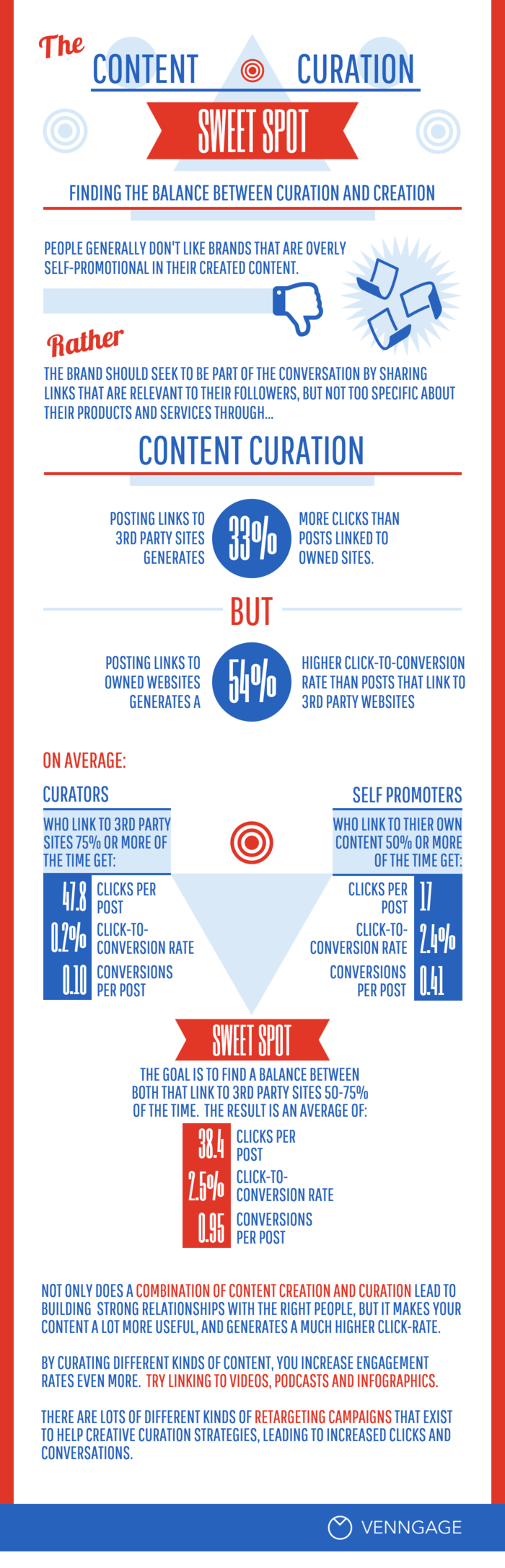
Design Tip: Venngage has a library of fonts to choose from. If you can’t find the icon you’re looking for , you can always request they be added. Our online editor has a chat box with 24/7 customer support.
7. Use colors strategically in your design
In design, colors are as functional as they are fashionable. You can use colors to emphasize points, categorize information, show movement or progression, and more.
For example, this chart uses color to categorize data:
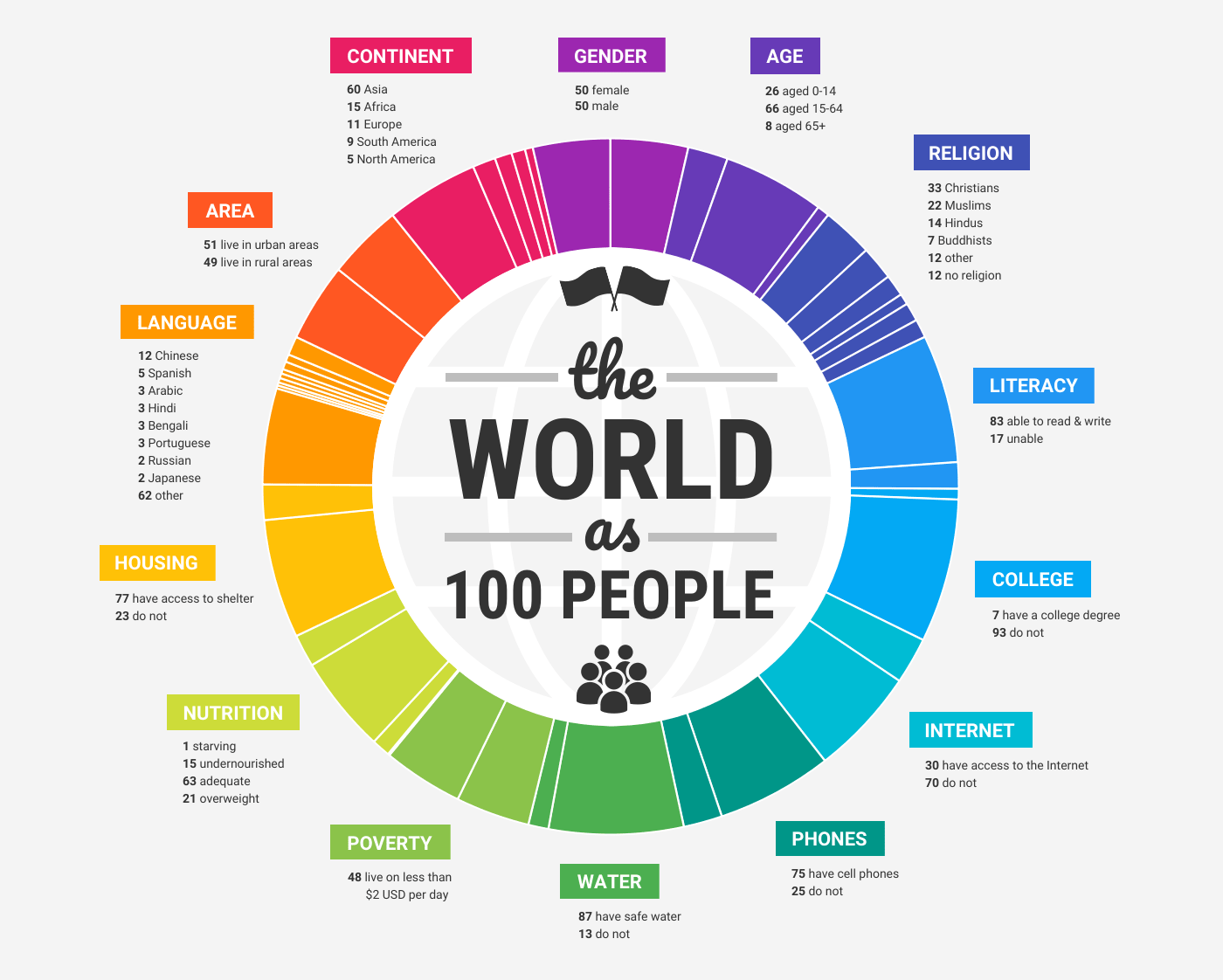
Design Tip : This pie chart can actually be customized in many ways. Human resources could provide a monthly update of people hired by department, nonprofits could show a breakdown of how they spent donations and real estate agents could show the average price of homes sold by neighbourhood.
You can also use light colored text and icons on dark backgrounds to make them stand out. Consider the mood that you want to convey with your infographic and pick colors that will reflect that mood. You can also use contrasting colors from your brand color palette.
This infographic template uses a bold combination of pinks and purples to give the data impact:
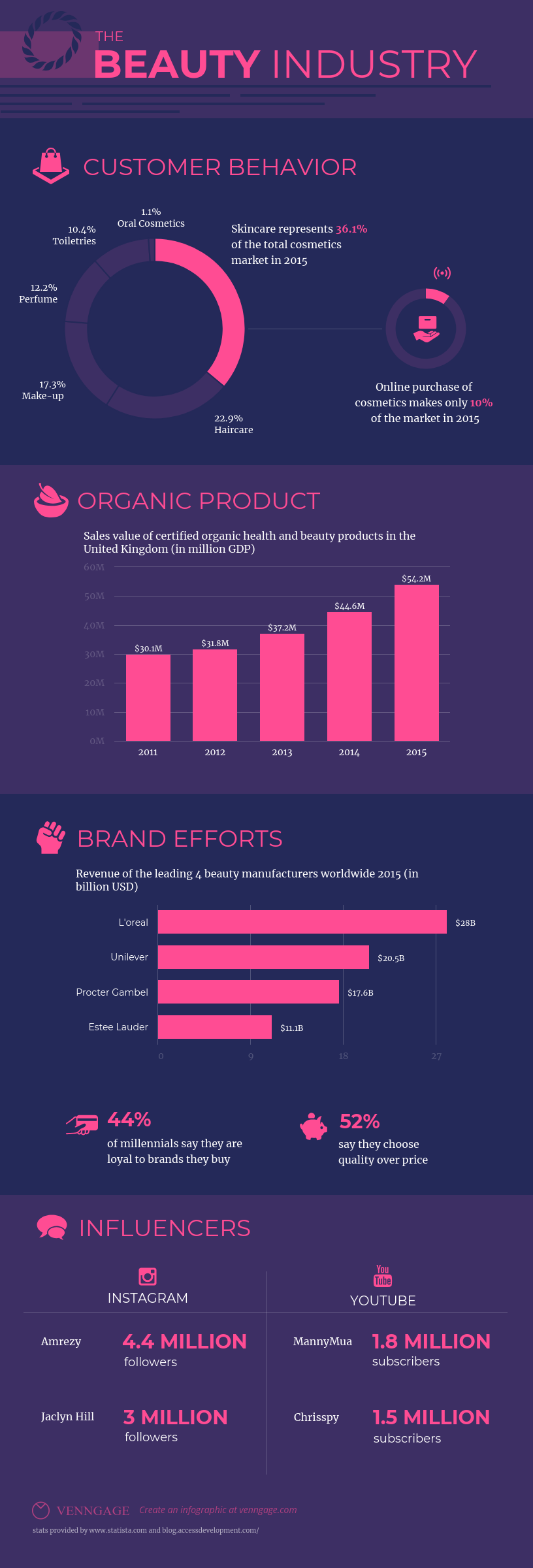
Read More: How to Pick Colors to Captivate Readers and Communicate Effectively
8. Show how parts make up a whole
It can be difficult to break a big topic down into smaller parts. Data visualization can make it a lot easier for people to conceptualize how parts make up a whole.
Using one focus visual, diagram or chart can convey parts of a whole more effectively than a text list can. Look at how this infographic neatly visualizes how marketers use blogging as part of their strategy:
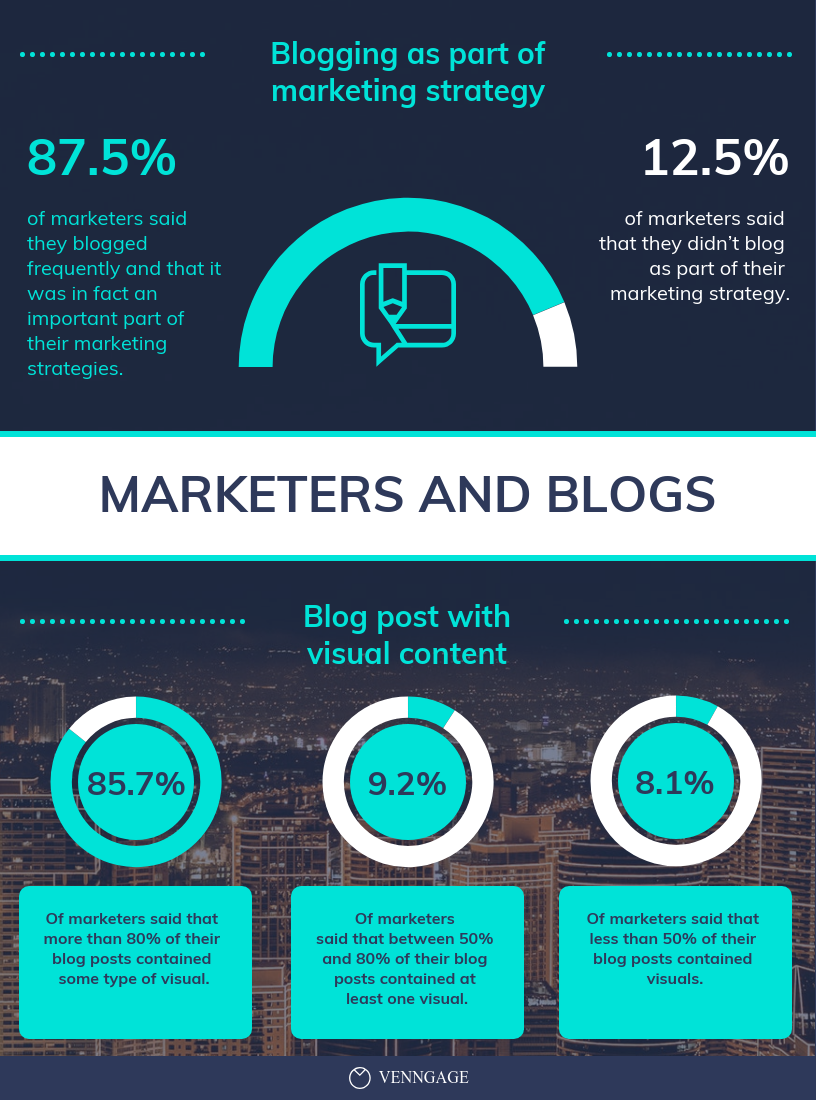
Design Tip: Human resources could use this graphic to show the results of a company survey. Or consultants could promote their services by showing their success rates.
Or look at how this infographic template uses one focus visual to illustrate the nutritional makeup of a banana:
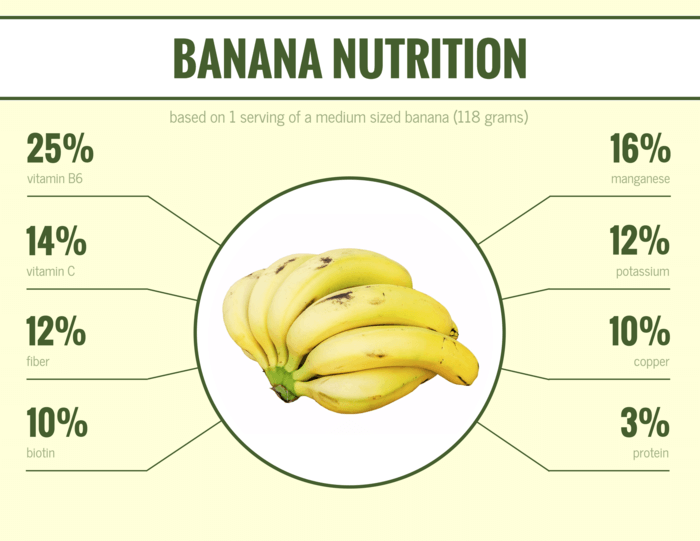
CREATE THIS FLYER TEMPLATE
9. Focus on one amazing statistic
If you are preparing a presentation, it’s best not to try and cram too many visuals into one slide. Instead, focus on one awe-inspiring statistic and make that the focus of your slide.
Use one focus visual to give the statistic even more impact. Smaller visuals like this are ideal for sharing on social media, like in this example:
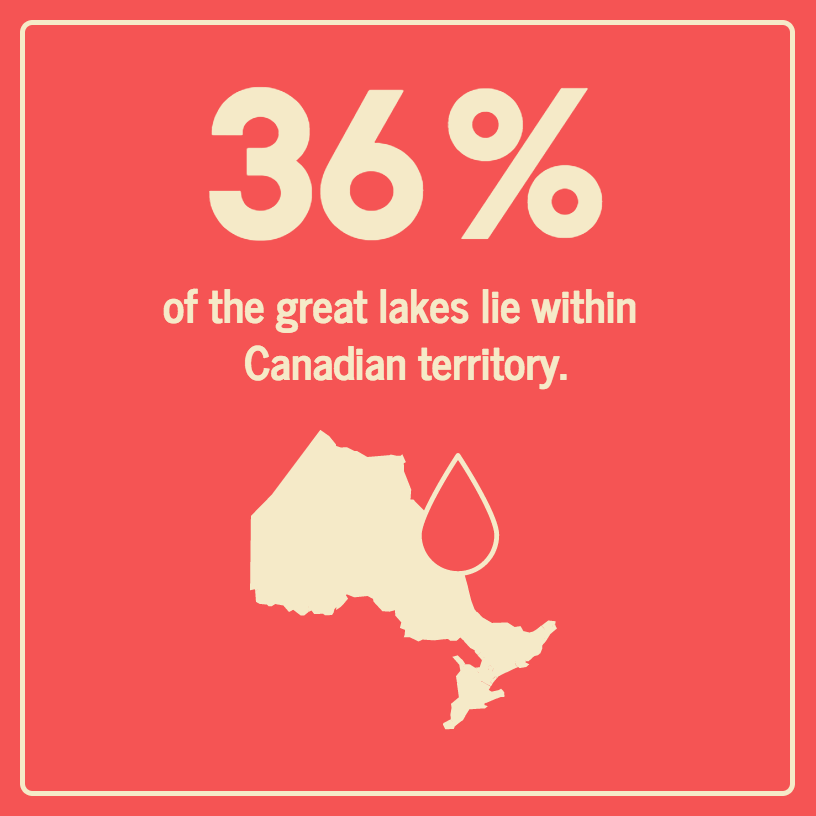
Design Tip: You can easily swap out the icon above (of Ontario, Canada) using Venngage’s drag-and-drop online editor and its in-editor library of icons. Click on the template above to get started.
This template also focuses on one key statistic and offers some supporting information in the bar on the side:
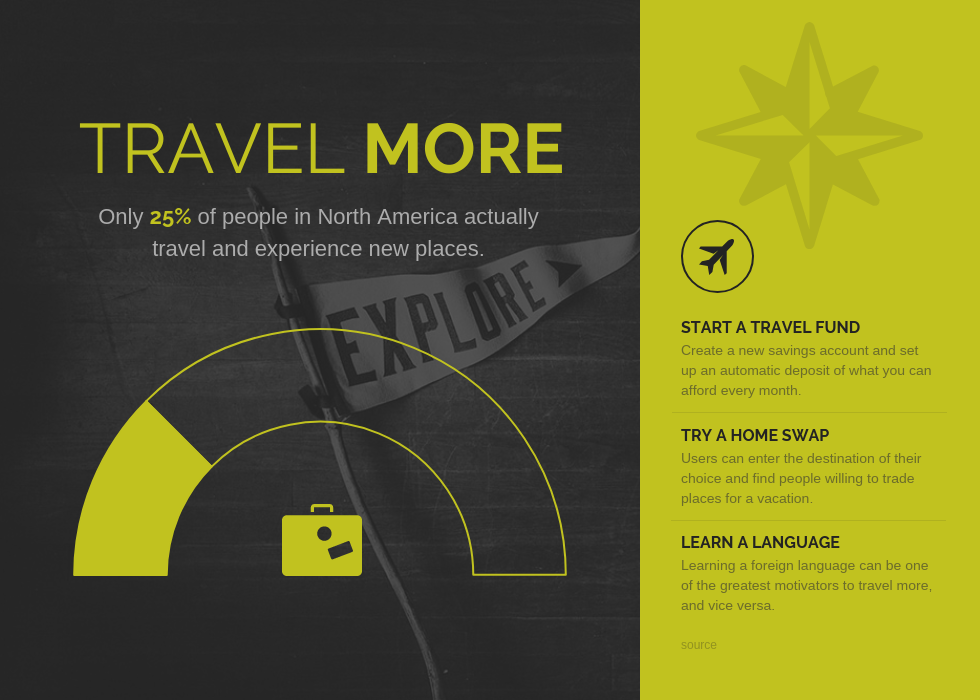
10. Optimize your data visualization for mobile
Complex, information-packed infographics are great for spicing up reports, blog posts, handouts, and more. But they’re not always the best for mobile viewing.
To optimize your data visualization for mobile viewing, use one focus chart or icon and big, legible font. You can create a series of mobile-optimized infographics to share multiple data points in a super original and attention-grabbing way.
For example, this infographic uses concise text and one chart to cut to the core message behind the data:
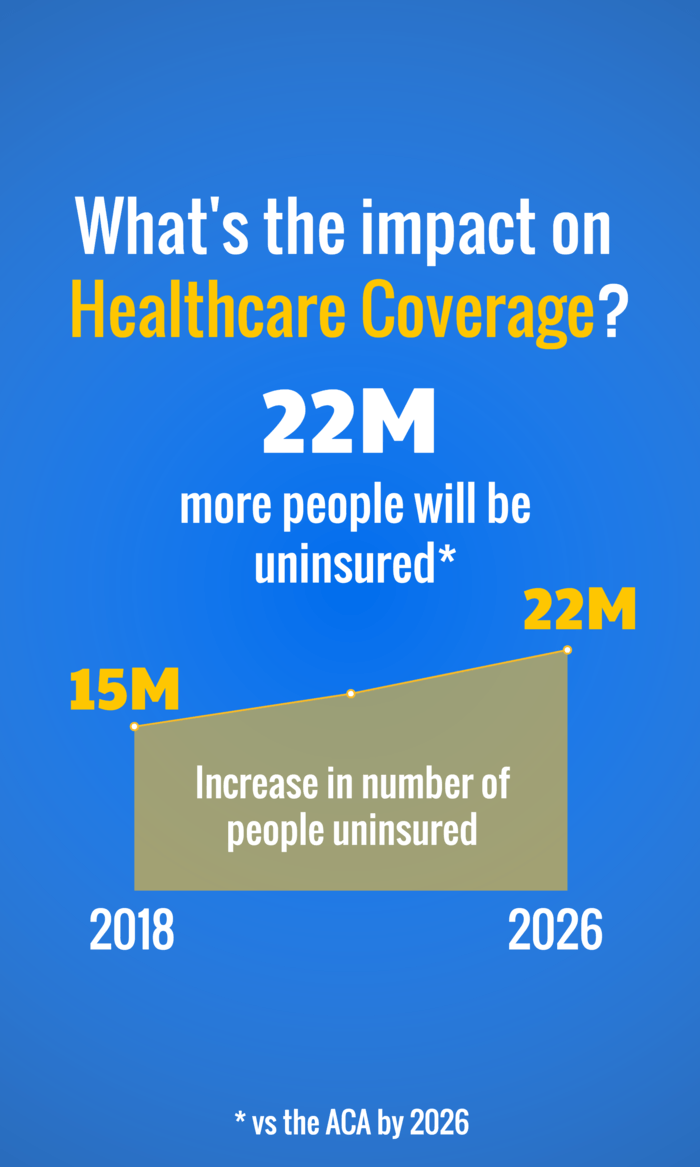
CREATE THIS SOCIAL MEDIA TEMPLATE
Some amazing data visualization examples
Here are some of the best data visualization examples I’ve come across in my years writing about data viz.
Evolution of Marketing Infographic

Graphic Design Trends Infographic
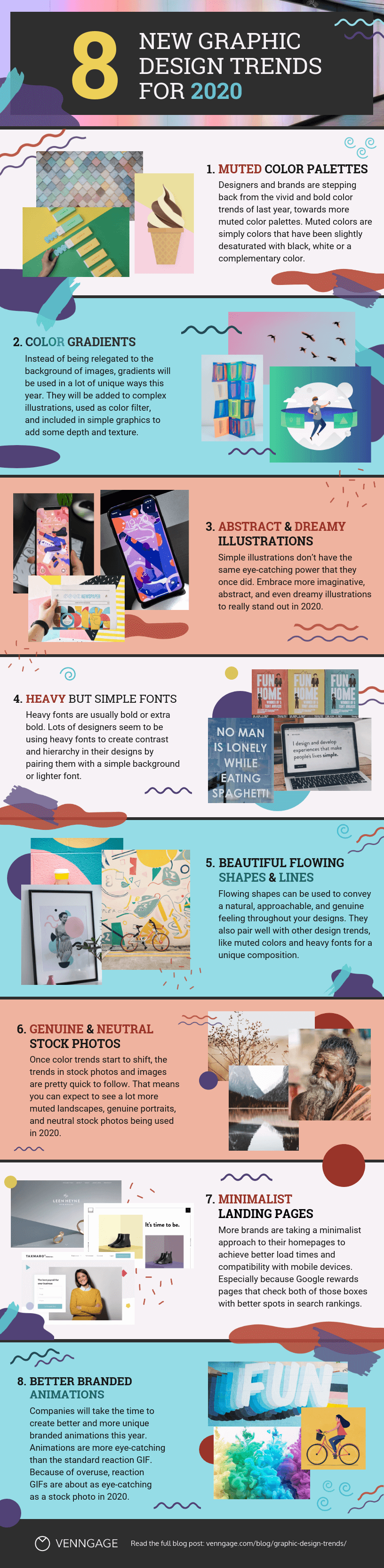
Stop Shark Finning Nonprofit Infographic
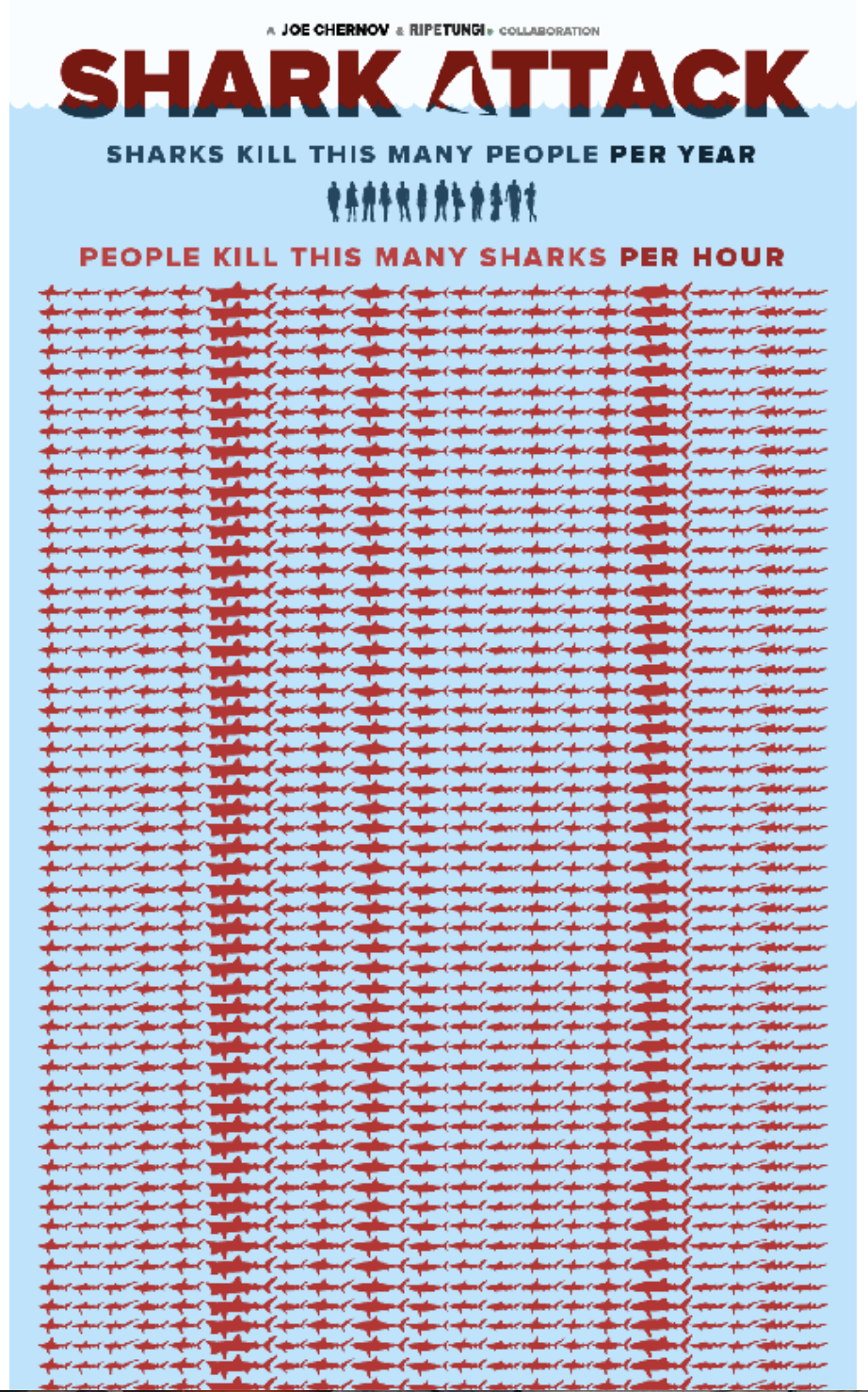
Source: Ripetungi
Coronavirus Impact on Environment Data Visualization

What Disney Characters Tell Us About Color Theory
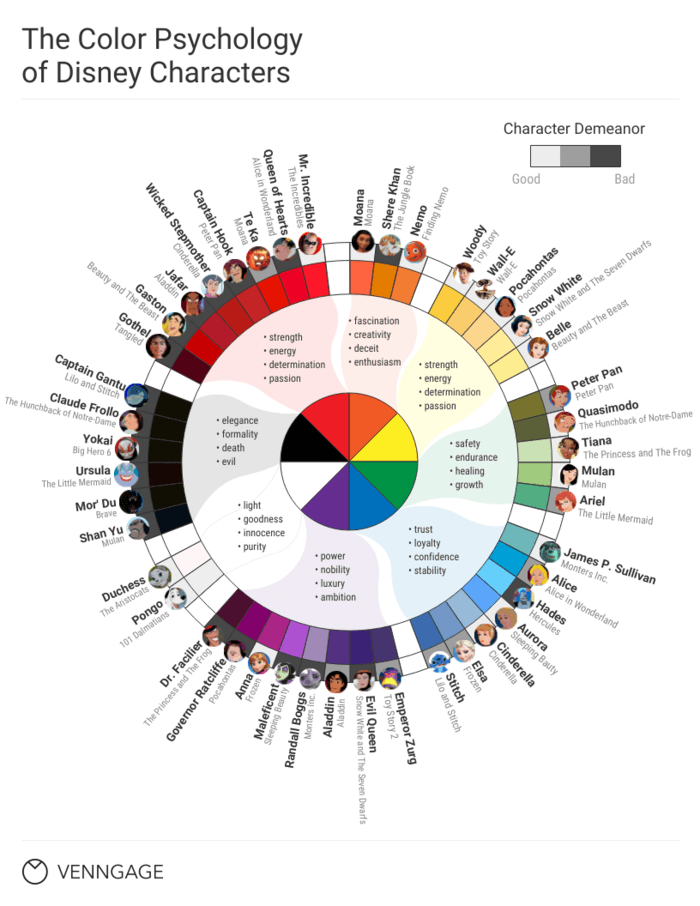
World’s Deadliest Animal Infographic
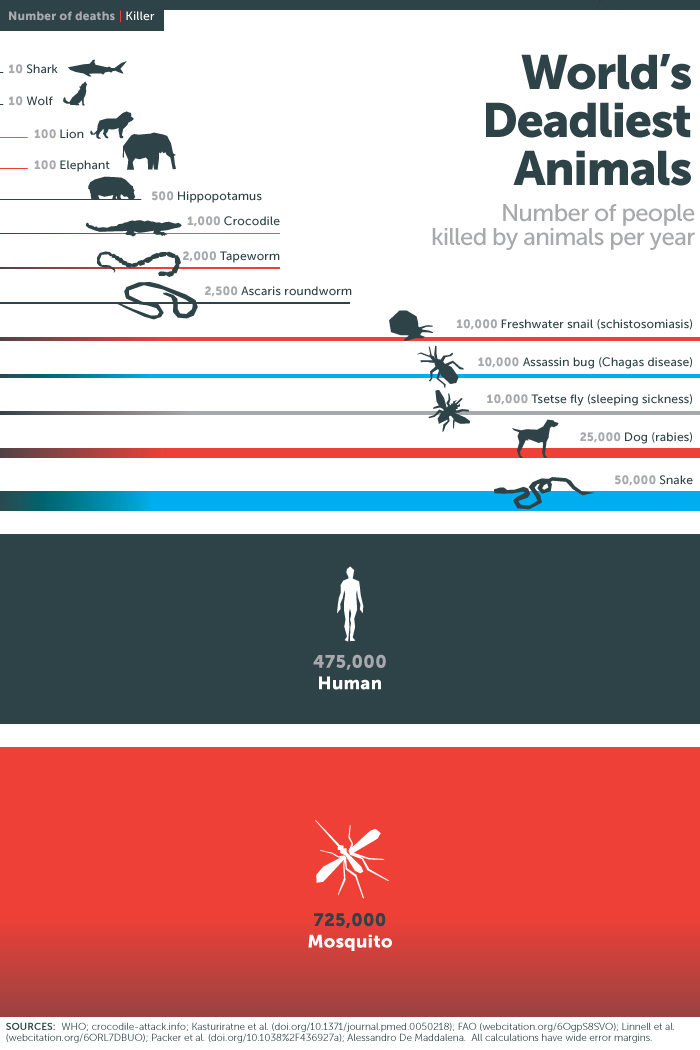
Source: Bill and Melinda Gates Foundation
The Secret Recipe For a Viral Creepypasta

Read More: Creepypasta Study: The Secret Recipe For a Viral Horror Story
The Hero’s Journey Infographic
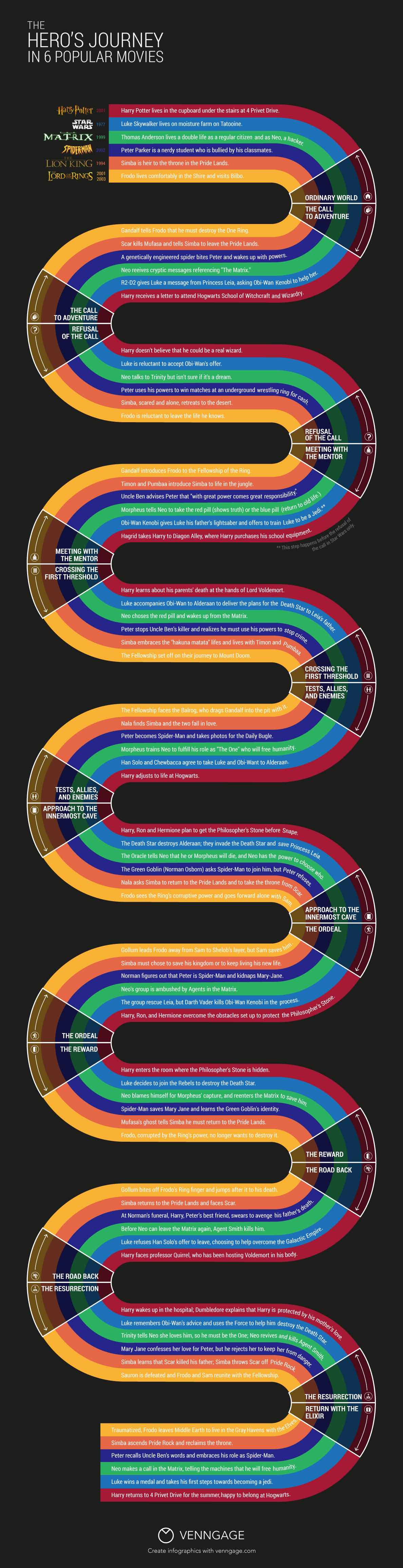
Read More: What Your 6 Favorite Movies Have in Common
Emotional Self Care Guide Infographic
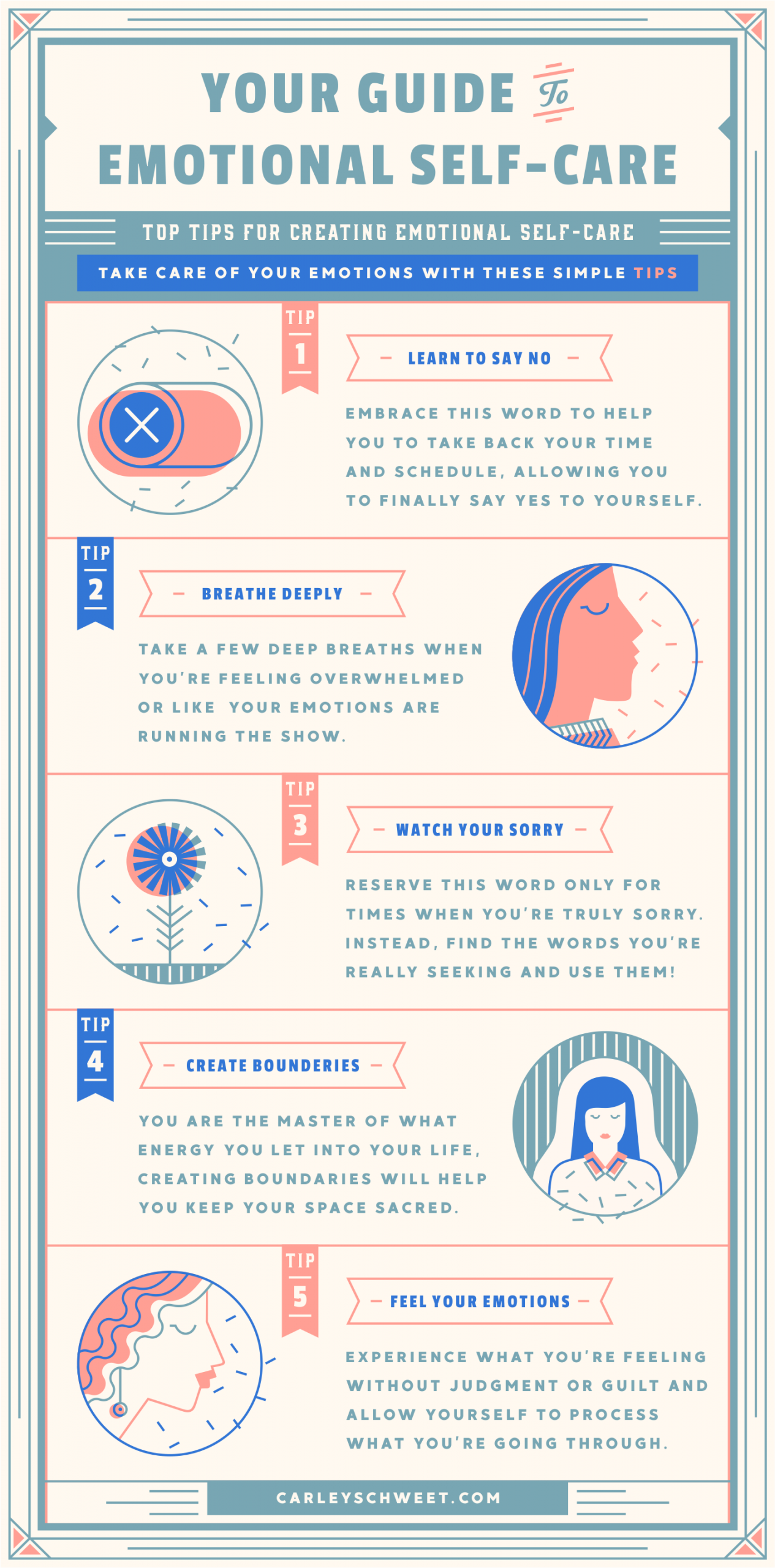
Source: Carley Schweet
Want to look at more amazing data visualization? Read More: 50+ Infographic Ideas, Examples & Templates for 2020 (For Marketers, Nonprofits, Schools, Healthcare Workers, and more)
Discover popular designs
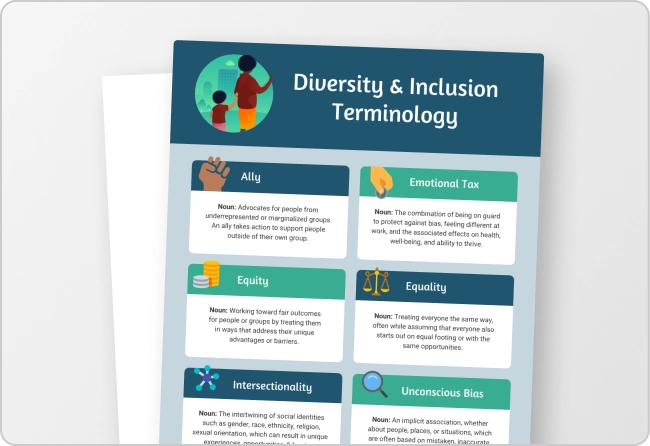
Infographic maker
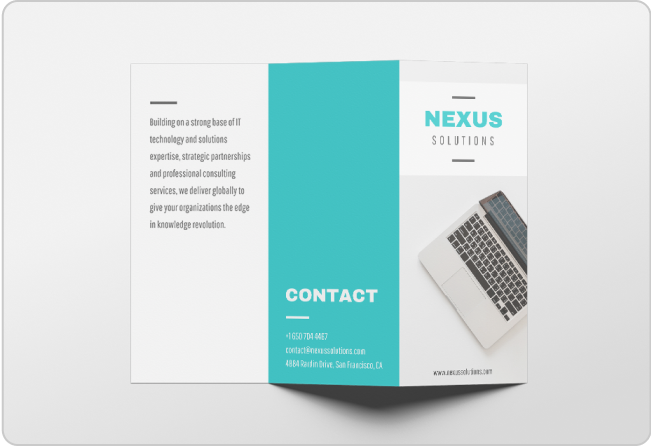
Brochure maker
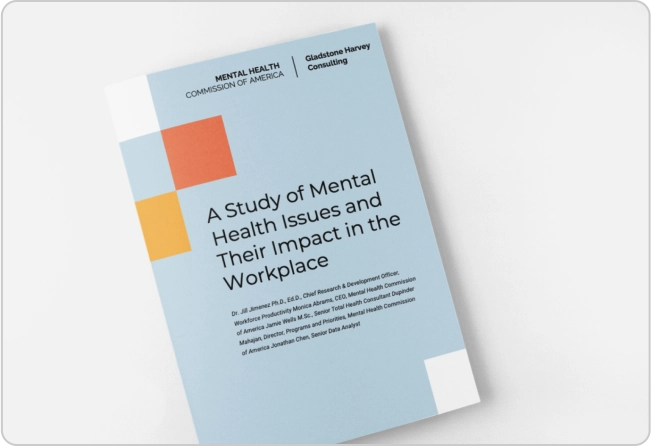
White paper online
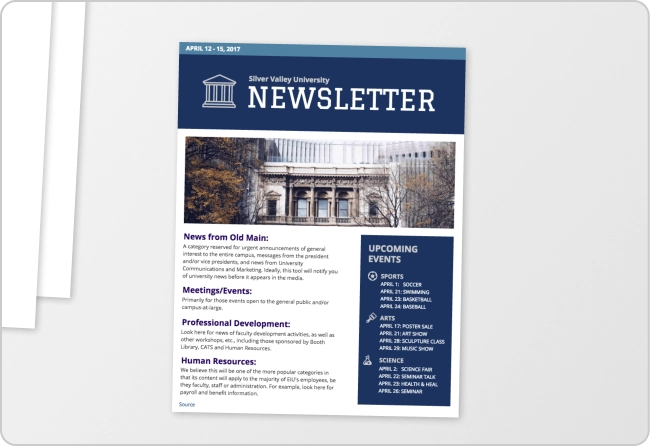
Newsletter creator
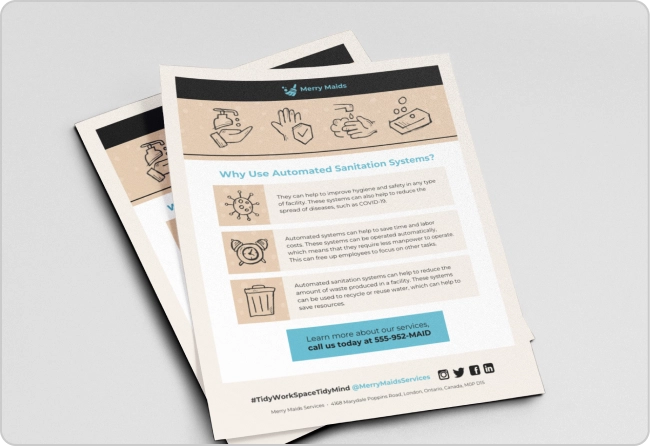
Flyer maker
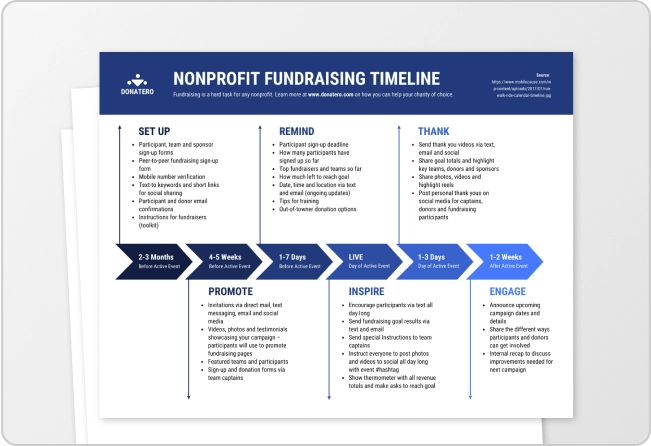
Timeline maker
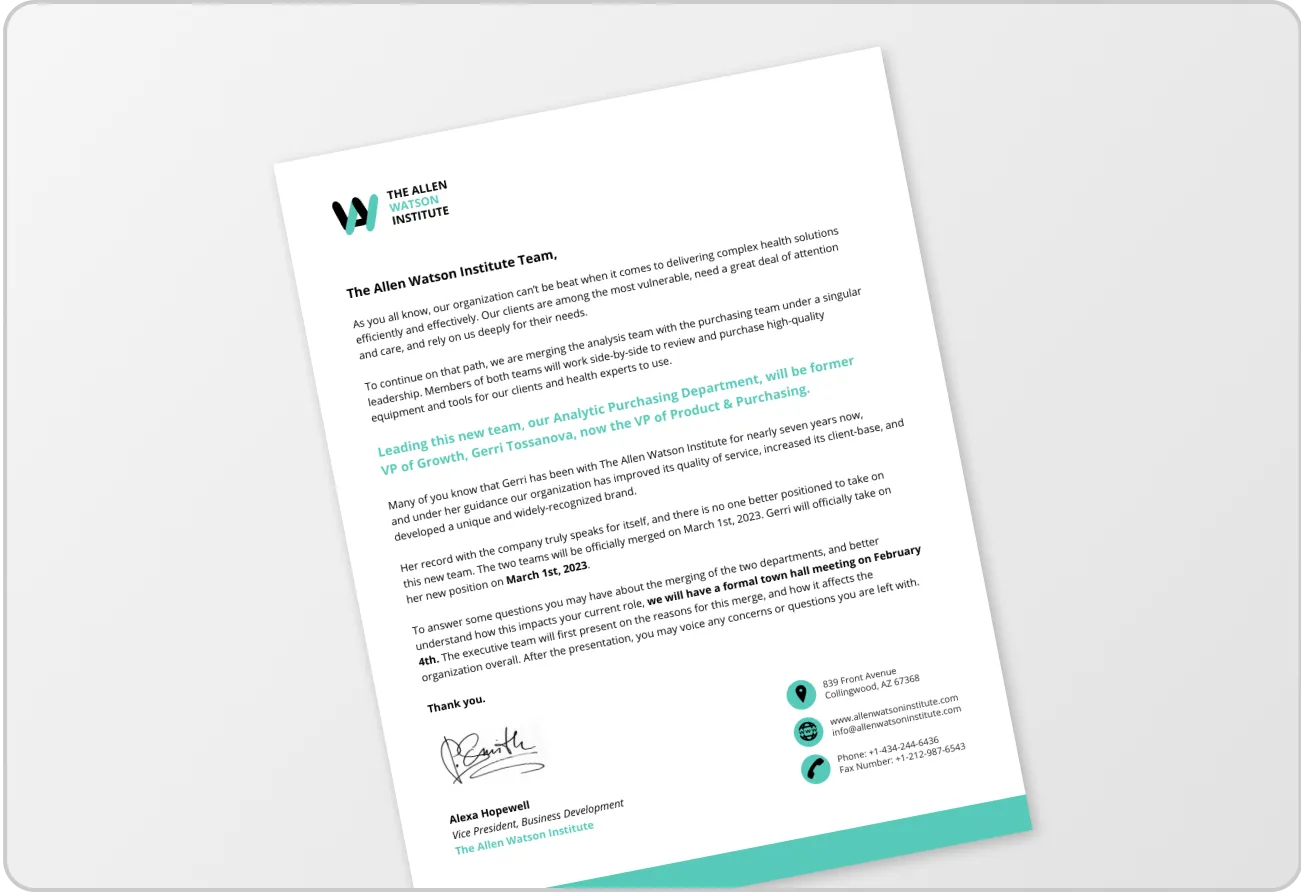
Letterhead maker
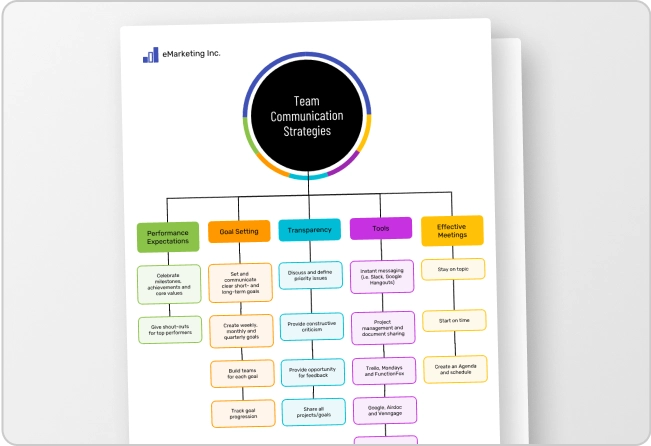
Mind map maker
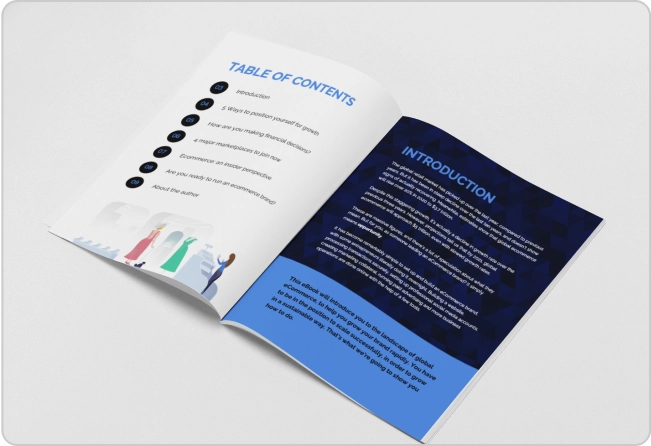
Ebook maker
What Is Data Visualization: Brief Theory, Useful Tips and Awesome Examples
- Share on Facebook
- Share on Twitter
By Al Boicheva
in Insights , Inspiration
3 years ago
Viewed 11,789 times
Spread the word about this article:
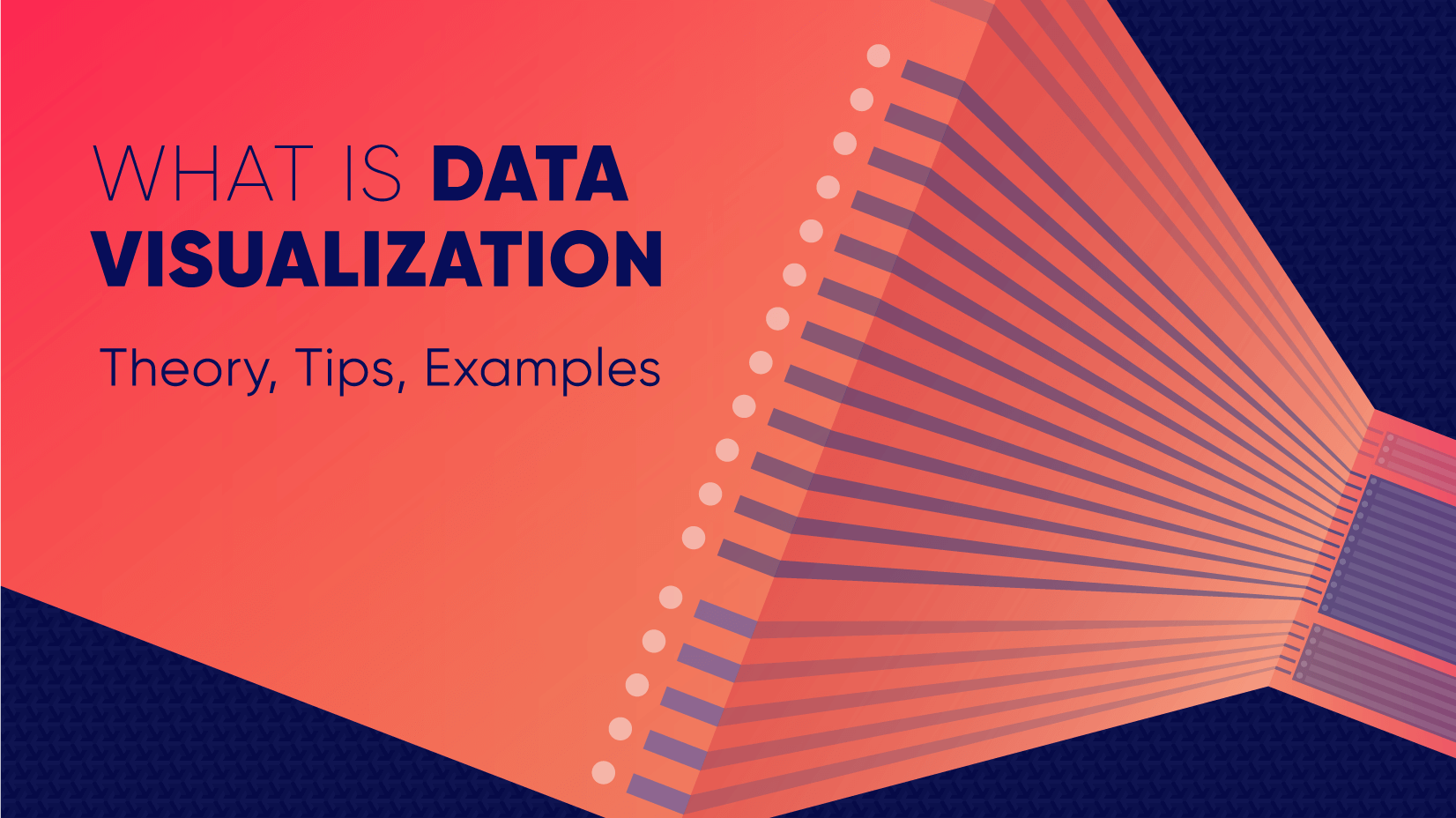
Updated: June 23, 2022
To create data visualization in order to present your data is no longer just a nice to have skill. Now, the skill to effectively sort and communicate your data through charts is a must-have for any business in any field that deals with data. Data visualization helps businesses quickly make sense of complex data and start making decisions based on that data. This is why today we’ll talk about what is data visualization. We’ll discuss how and why does it work, what type of charts to choose in what cases, how to create effective charts, and, of course, end with beautiful examples.
So let’s jump right in. As usual, don’t hesitate to fast-travel to a particular section of your interest.
Article overview: 1. What Does Data Visualization Mean? 2. How Does it Work? 3. When to Use it? 4. Why Use it? 5. Types of Data Visualization 6. Data Visualization VS Infographics: 5 Main Differences 7. How to Create Effective Data Visualization?: 5 Useful Tips 8. Examples of Data Visualization
1. What is Data Visualization?
Data Visualization is a graphic representation of data that aims to communicate numerous heavy data in an efficient way that is easier to grasp and understand . In a way, data visualization is the mapping between the original data and graphic elements that determine how the attributes of these elements vary. The visualization is usually made by the use of charts, lines, or points, bars, and maps.
- Data Viz is a branch of Descriptive statistics but it requires both design, computer, and statistical skills.
- Aesthetics and functionality go hand in hand to communicate complex statistics in an intuitive way.
- Data Viz tools and technologies are essential for making data-driven decisions.
- It’s a fine balance between form and functionality.
- Every STEM field benefits from understanding data.
2. How Does it Work?
If we can see it, our brains can internalize and reflect on it. This is why it’s much easier and more effective to make sense of a chart and see trends than to read a massive document that would take a lot of time and focus to rationalize. We wouldn’t want to repeat the cliche that humans are visual creatures, but it’s a fact that visualization is much more effective and comprehensive.
In a way, we can say that data Viz is a form of storytelling with the purpose to help us make decisions based on data. Such data might include:
- Tracking sales
- Identifying trends
- Identifying changes
- Monitoring goals
- Monitoring results
- Combining data
3. When to Use it?
Data visualization is useful for companies that deal with lots of data on a daily basis. It’s essential to have your data and trends instantly visible. Better than scrolling through colossal spreadsheets. When the trends stand out instantly this also helps your clients or viewers to understand them instead of getting lost in the clutter of numbers.
With that being said, Data Viz is suitable for:
- Annual reports
- Presentations
- Social media micronarratives
- Informational brochures
- Trend-trafficking
- Candlestick chart for financial analysis
- Determining routes
Common cases when data visualization sees use are in sales, marketing, healthcare, science, finances, politics, and logistics.
4. Why Use it?
Short answer: decision making. Data Visualization comes with the undeniable benefits of quickly recognizing patterns and interpret data. More specifically, it is an invaluable tool to determine the following cases.
- Identifying correlations between the relationship of variables.
- Getting market insights about audience behavior.
- Determining value vs risk metrics.
- Monitoring trends over time.
- Examining rates and potential through frequency.
- Ability to react to changes.
5. Types of Data Visualization
As you probably already guessed, Data Viz is much more than simple pie charts and graphs styled in a visually appealing way. The methods that this branch uses to visualize statistics include a series of effective types.
Map visualization is a great method to analyze and display geographically related information and present it accurately via maps. This intuitive way aims to distribute data by region. Since maps can be 2D or 3D, static or dynamic, there are numerous combinations one can use in order to create a Data Viz map.
COVID-19 Spending Data Visualization POGO by George Railean
The most common ones, however, are:
- Regional Maps: Classic maps that display countries, cities, or districts. They often represent data in different colors for different characteristics in each region.
- Line Maps: They usually contain space and time and are ideal for routing, especially for driving or taxi routes in the area due to their analysis of specific scenes.
- Point Maps: These maps distribute data of geographic information. They are ideal for businesses to pinpoint the exact locations of their buildings in a region.
- Heat Maps: They indicate the weight of a geographical area based on a specific property. For example, a heat map may distribute the saturation of infected people by area.
Charts present data in the form of graphs, diagrams, and tables. They are often confused with graphs since graphs are indeed a subcategory of charts. However, there is a small difference: graphs show the mathematical relationship between groups of data and is only one of the chart methods to represent data.
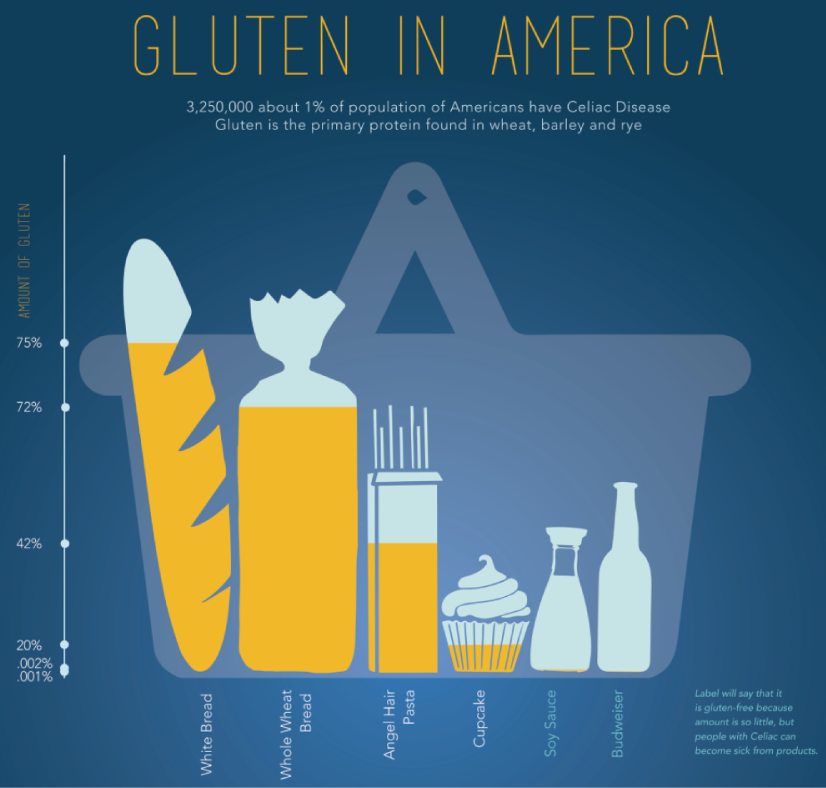
Infographic Data Visualization by Madeline VanRemmen
With that out of the way, let’s talk about the most basic types of charts in data visualization.
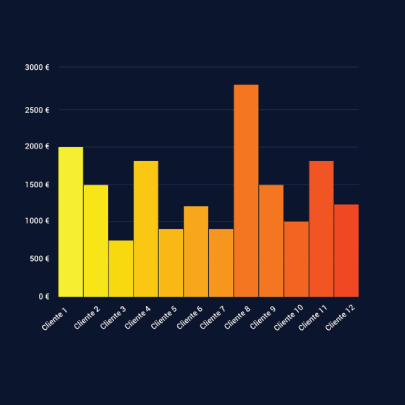
They use a series of bars that illustrate data development. They are ideal for lighter data and follow trends of no more than three variables or else, the bars become cluttered and hard to comprehend. Ideal for year-on-year comparisons and monthly breakdowns.
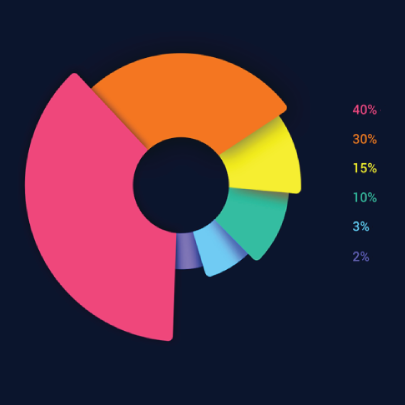
These familiar circular graphs divide data into portions. The bigger the slice, the bigger the portion. They are ideal for depicting sections of a whole and their sum must always be 100%. Avoid pie charts when you need to show data development over time or lack a value for any of the portions. Doughnut charts have the same use as pie charts.
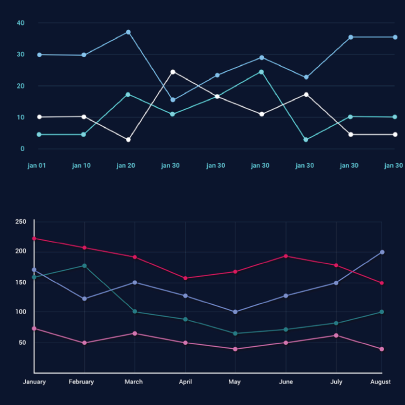
They use a line or more than one lines that show development over time. It allows tracking multiple variables at the same time. A great example is tracking product sales by a brand over the years. Area charts have the same use as line charts.
Scatter Plot
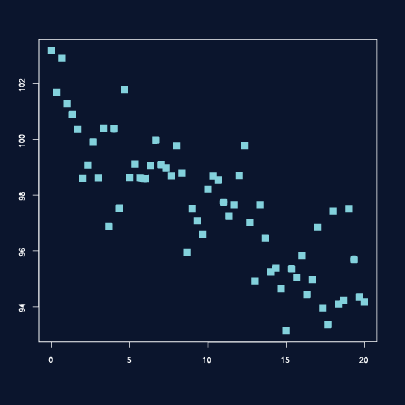
These charts allow you to see patterns through data visualization. They have an x-axis and a y-axis for two different values. For example, if your x-axis contains information about car prices while the y-axis is about salaries, the positive or negative relationship will tell you about what a person’s car tells about their salary.
Unlike the charts we just discussed, tables show data in almost a raw format. They are ideal when your data is hard to present visually and aim to show specific numerical data that one is supposed to read rather than visualize.
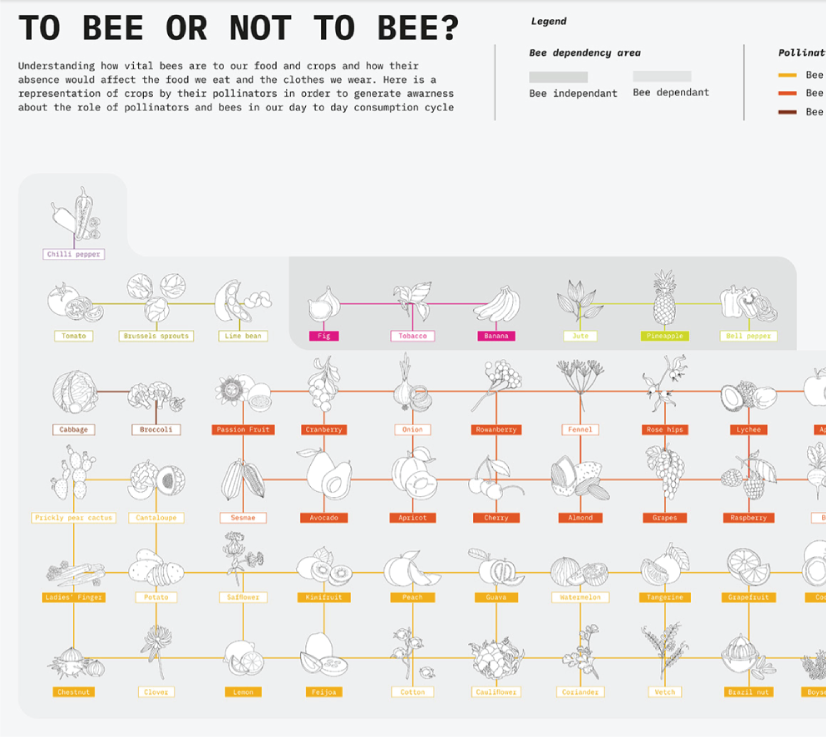
Data Visualisation | To bee or not to bee by Aishwarya Anand Singh
For example, charts are perfect to display data about a particular illness over a time period in a particular area, but a table comes to better use when you also need to understand specifics such as causes, outcomes, relapses, a period of treatment, and so on.
6. Data Visualization VS Infographics
5 main differences.
They are not that different as both visually represent data. It is often you search for infographics and find images titled Data Visualization and the other way around. In many cases, however, these titles aren’t misleading. Why is that?
- Data visualization is made of just one element. It could be a map, a chart, or a table. Infographics , on the other hand, often include multiple Data Viz elements.
- Unlike data visualizations that can be simple or extremely complex and heavy, infographics are simple and target wider audiences. The latter is usually comprehensible even to people outside of the field of research the infographic represents.
- Interestingly enough, data Viz doesn’t offer narratives and conclusions, it’s a tool and basis for reaching those. While infographics, in most cases offer a story and a narrative. For example, a data visualization map may have the title “Air pollution saturation by region”, while an infographic with the same data would go “Areas A and B are the most polluted in Country C”.
- Data visualizations can be made in Excel or use other tools that automatically generate the design unless they are set for presentation or publishing. The aesthetics of infographics , however, are of great importance and the designs must be appealing to wider audiences.
- In terms of interaction, data visualizations often offer interactive charts, especially in an online form. Infographics, on the other hand, rarely have interaction and are usually static images.
While on topic, you could also be interested to check out these 50 engaging infographic examples that make complex data look great.
7. Tips to Create Effective Data Visualization
The process is naturally similar to creating Infographics and it revolves around understanding your data and audience. To be more precise, these are the main steps and best practices when it comes to preparing an effective visualization of data for your viewers to instantly understand.
1. Do Your Homework
Preparation is half the work already done. Before you even start visualizing data, you have to be sure you understand that data to the last detail.
Knowing your audience is undeniable another important part of the homework, as different audiences process information differently. Who are the people you’re visualizing data for? How do they process visual data? Is it enough to hand them a single pie chart or you’ll need a more in-depth visual report?
The third part of preparing is to determine exactly what you want to communicate to the audience. What kind of information you’re visualizing and does it reflect your goal?
And last, think about how much data you’ll be working with and take it into account.
2. Choose the Right Type of Chart
In a previous section, we listed the basic chart types that find use in data visualization. To determine best which one suits your work, there are a few things to consider.
- How many variables will you have in a chart?
- How many items will you place for each of your variables?
- What will be the relation between the values (time period, comparison, distributions, etc.)
With that being said, a pie chart would be ideal if you need to present what portions of a whole takes each item. For example, you can use it to showcase what percent of the market share takes a particular product. Pie charts, however, are unsuitable for distributions, comparisons, and following trends through time periods. Bar graphs, scatter plots,s and line graphs are much more effective in those cases.
Another example is how to use time in your charts. It’s way more accurate to use a horizontal axis because time should run left to right. It’s way more visually intuitive.
3. Sort your Data
Start with removing every piece of data that does not add value and is basically excess for the chart. Sometimes, you have to work with a huge amount of data which will inevitably make your chart pretty complex and hard to read. Don’t hesitate to split your information into two or more charts. If that won’t work for you, you could use highlights or change the entire type of chart with something that would fit better.
Tip: When you use bar charts and columns for comparison, sort the information in an ascending or a descending way by value instead of alphabetical order.
4. Use Colors to Your Advantage
In every form of visualization, colors are your best friend and the most powerful tool. They create contrasts, accents, and emphasis and lead the eye intuitively. Even here, color theory is important.
When you design your chart, make sure you don’t use more than 5 or 6 colors. Anything more than that will make your graph overwhelming and hard to read for your viewers. However, color intensity is a different thing that you can use to your advantage. For example, when you compare the same concept in different periods of time, you could sort your data from the lightest shade of your chosen color to its darker one. It creates a strong visual progression, proper to your timeline.
Things to consider when you choose colors:
- Different colors for different categories.
- A consistent color palette for all charts in a series that you will later compare.
- It’s appropriate to use color blind-friendly palettes.
5. Get Inspired
Always put your inspiration to work when you want to be at the top of your game. Look through examples, infographics, and other people’s work and see what works best for each type of data you need to implement.
This Twitter account Data Visualization Society is a great way to start. In the meantime, we’ll also handpick some amazing examples that will get you in the mood to start creating the visuals for your data.
8. Examples for Data Visualization
As another art form, Data Viz is a fertile ground for some amazing well-designed graphs that prove that data is beautiful. Now let’s check out some.
Dark Souls III Experience Data
We start with Meng Hsiao Wei’s personal project presenting his experience with playing Dark Souls 3. It’s a perfect example that infographics and data visualization are tools for personal designs as well. The research is pretty massive yet very professionally sorted into different types of charts for the different concepts. All data visualizations are made with the same color palette and look great in infographics.
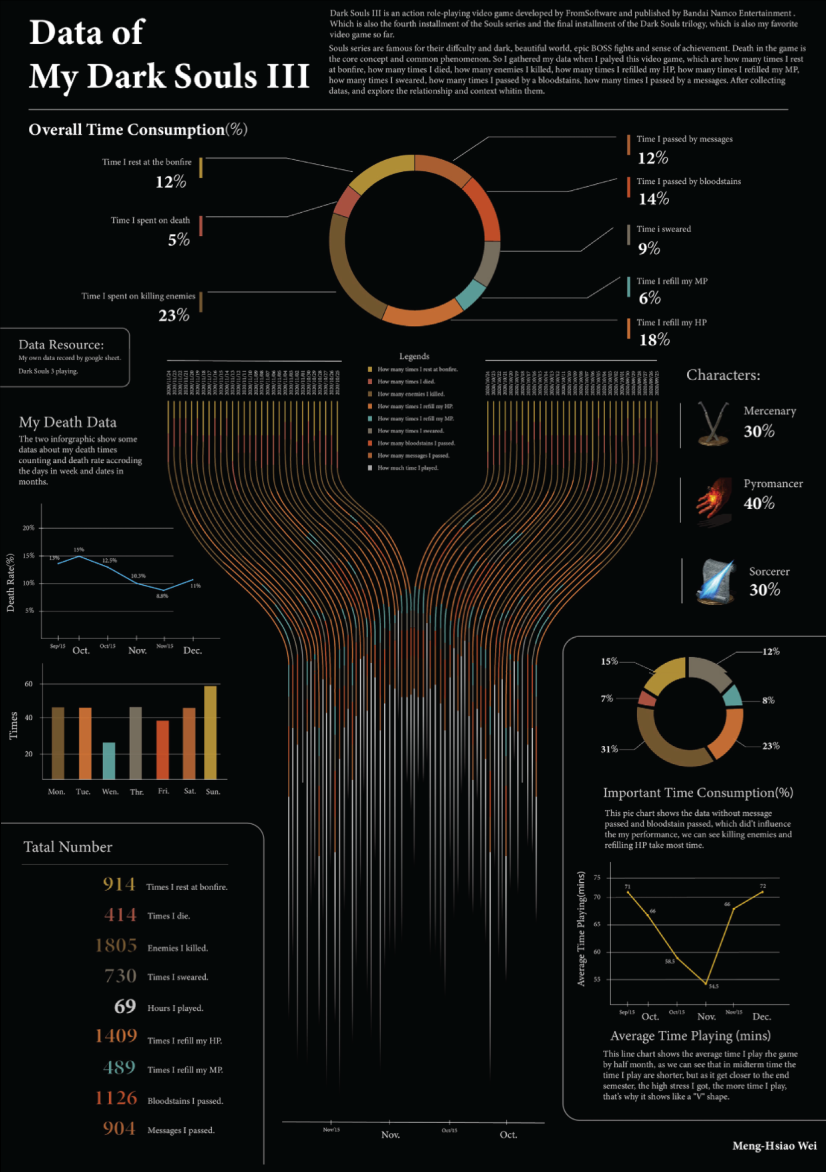
My dark souls 3 playing data by Meng Hsiao Wei
Greatest Movies of all Time
Katie Silver has compiled a list of the 100 greatest movies of all time based on critics and crowd reviews. The visualization shows key data points for every movie such as year of release, oscar nominations and wins, budget, gross, IMDB score, genre, filming location, setting of the film, and production studio. All movies are ordered by the release date.
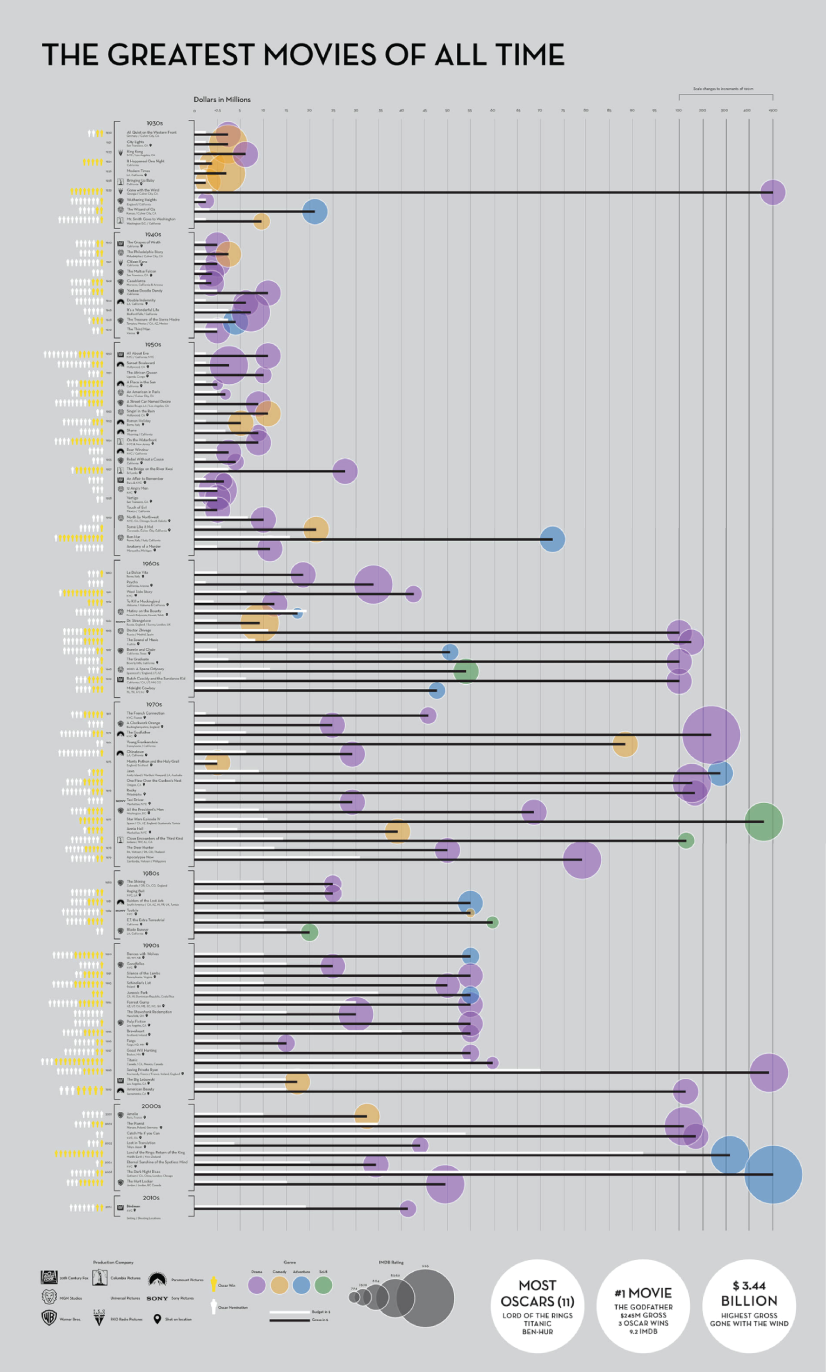
100 Greatest Movies Data Visualization by Katie Silver
The Most Violent Cities
Federica Fragapane shows data for the 50 most violent cities in the world in 2017. The items are arranged on a vertical axis based on population and ordered along the horizontal axis according to the homicide rate.
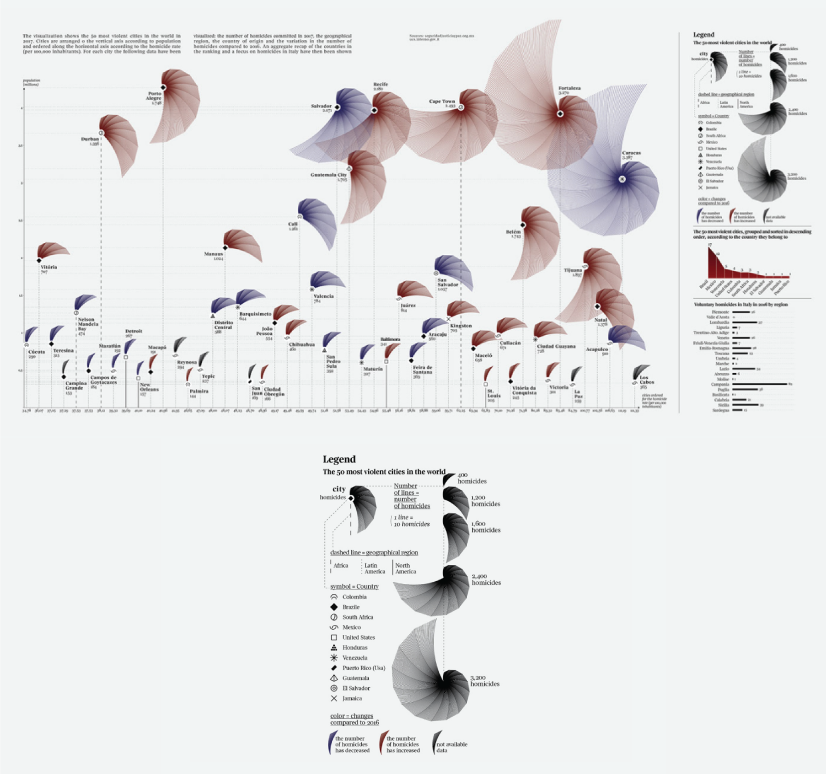
The Most Violent Cities by Federica Fragapane
Family Businesses as Data
These data visualizations and illustrations were made by Valerio Pellegrini for Perspectives Magazine. They show a pie chart with sector breakdown as well as a scatter plot for contribution for employment.
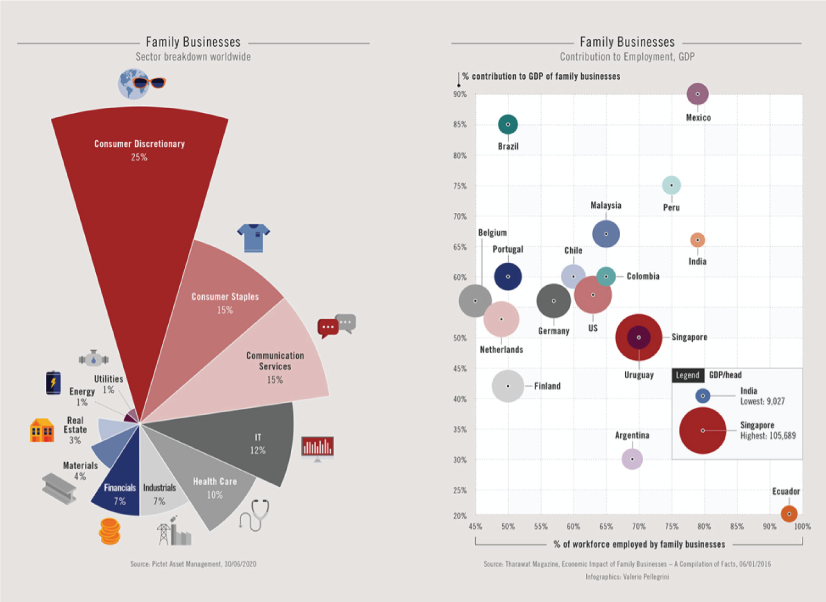
PERSPECTIVES MAGAZINE – Family Businesses by Valerio Pellegrini
Orbit Map of the Solar System
The map shows data on the orbits of more than 18000 asteroids in the solar system. Each asteroid is shown at its position on New Years’ Eve 1999, colored by type of asteroid.
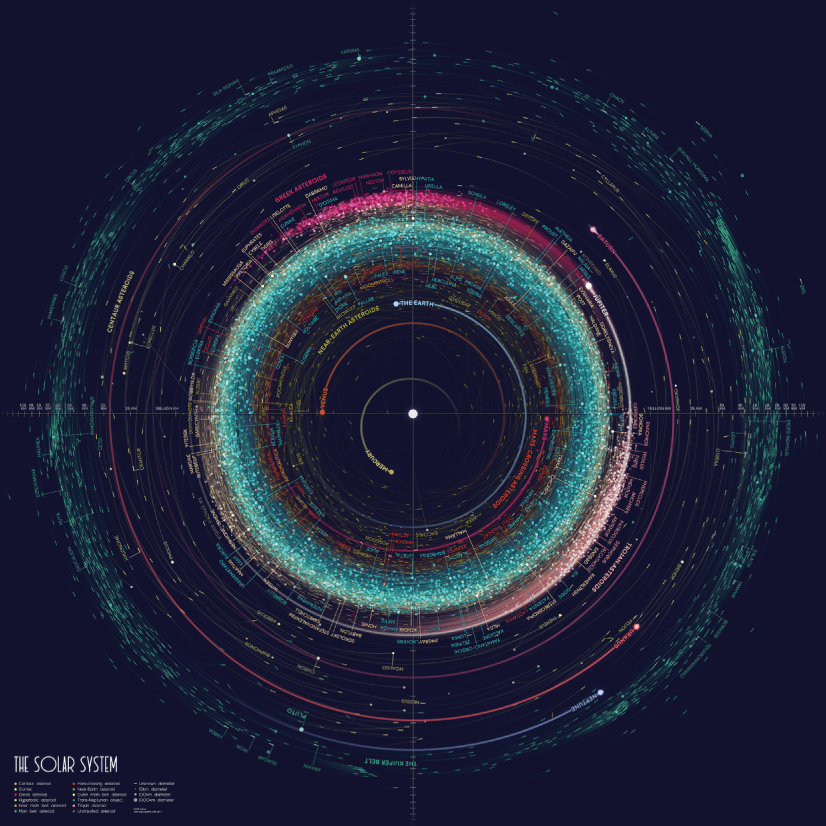
An Orbit Map of the Solar System by Eleanor Lutz
The Semantics Of Headlines
Katja Flükiger has a take on how headlines tell the story. The data visualization aims to communicate how much is the selling influencing the telling. The project was completed at Maryland Institute College of Art to visualize references to immigration and color-coding the value judgments implied by word choice and context.
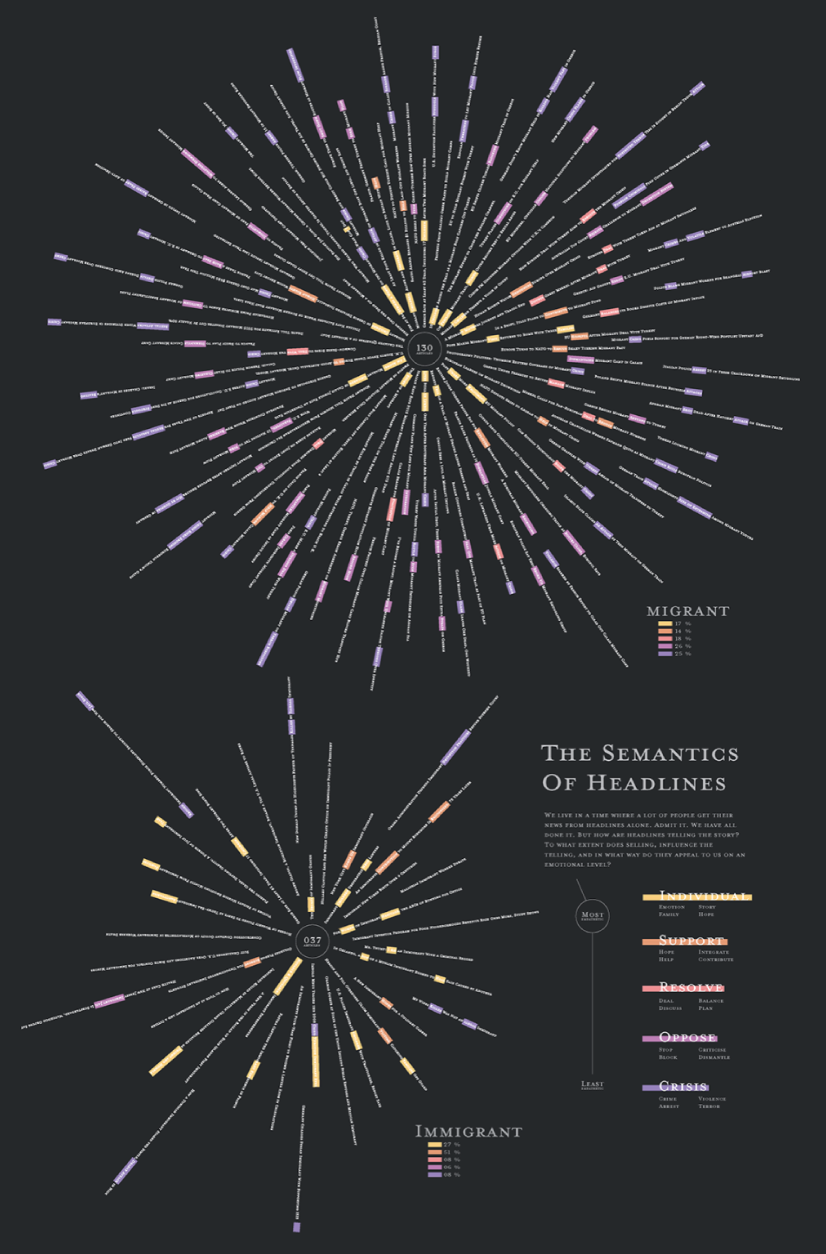
The Semantics of Headlines by Katja Flükiger
Moon and Earthquakes
This data visualization works on answering whether the moon is responsible for earthquakes. The chart features the time and intensity of earthquakes in response to the phase and orbit location of the moon.
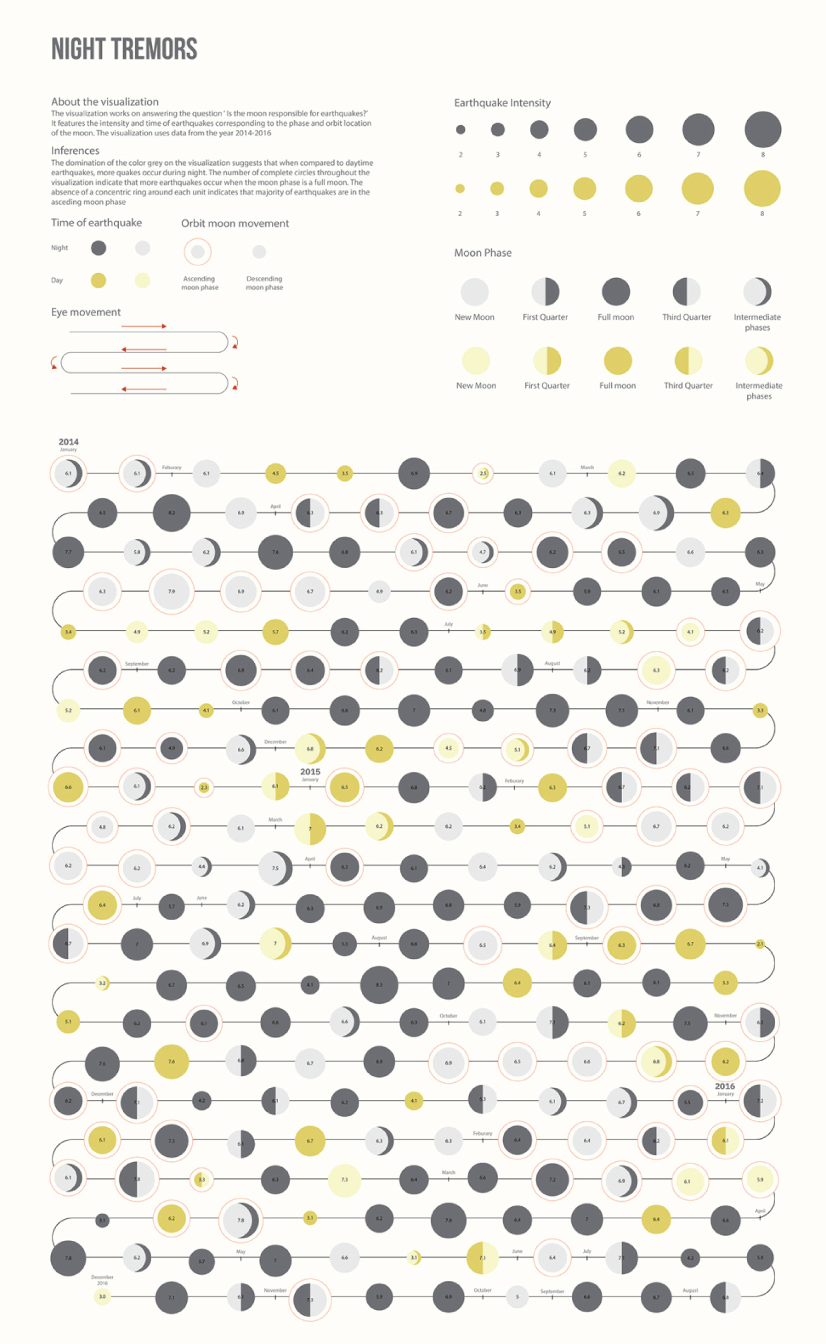
Moon and Earthquakes by Aishwarya Anand Singh
Dawn of the Nanosats
The visualization shows the satellites launched from 2003 to 2015. The graph represents the type of institutions focused on projects as well as the nations that financed them. On the left, it is shown the number of launches per year and satellite applications.
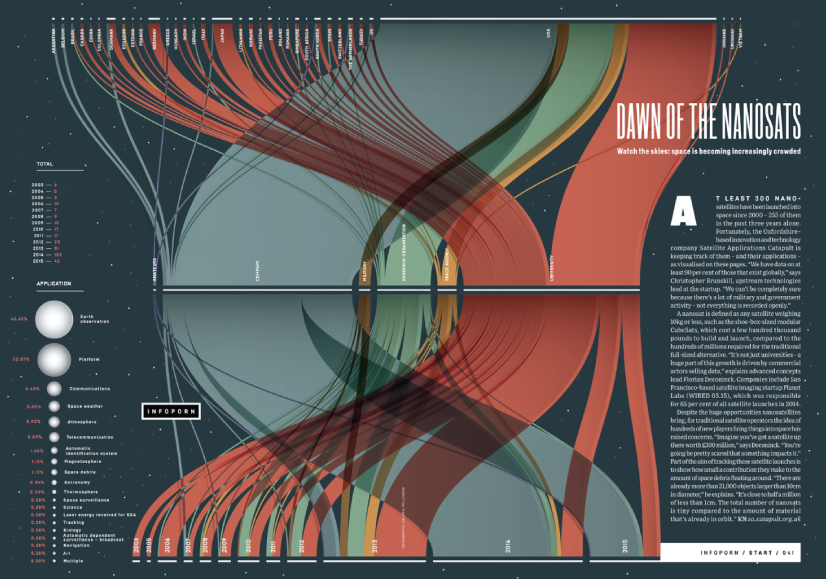
WIRED UK – Dawn of the by Nanosats by Valerio Pellegrini
Final Words
Data visualization is not only a form of science but also a form of art. Its purpose is to help businesses in any field quickly make sense of complex data and start making decisions based on that data. To make your graphs efficient and easy to read, it’s all about knowing your data and audience. This way you’ll be able to choose the right type of chart and use visual techniques to your advantage.
You may also be interested in some of these related articles:
- Infographics for Marketing: How to Grab and Hold the Attention
- 12 Animated Infographics That Will Engage Your Mind from Start to Finish
- 50 Engaging Infographic Examples That Make Complex Ideas Look Great
- Good Color Combinations That Go Beyond Trends: Inspirational Examples and Ideas
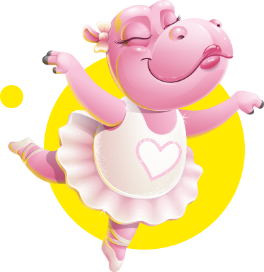
Add some character to your visuals
Cartoon Characters, Design Bundles, Illustrations, Backgrounds and more...
Like us on Facebook
Subscribe to our newsletter
Be the first to know what’s new in the world of graphic design and illustrations.
- [email protected]
Browse High Quality Vector Graphics
E.g.: businessman, lion, girl…
Related Articles
Creative modern menu designs that boost the appetite, 27 visual content marketing statistics for a game-changing 2017, 25 engaging visual content marketing examples feat. illustrations, the best free animation software options on the market right now, 30 inspiring ux design examples for your next vision in 2022, check out our infographics bundle with 500+ infographic templates:, enjoyed this article.
Don’t forget to share!
- Comments (2)
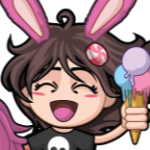
Al Boicheva
Al is an illustrator at GraphicMama with out-of-the-box thinking and a passion for anything creative. In her free time, you will see her drooling over tattoo art, Manga, and horror movies.
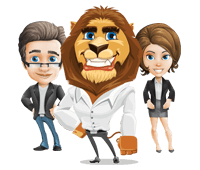
Thousands of vector graphics for your projects.
Hey! You made it all the way to the bottom!
Here are some other articles we think you may like:
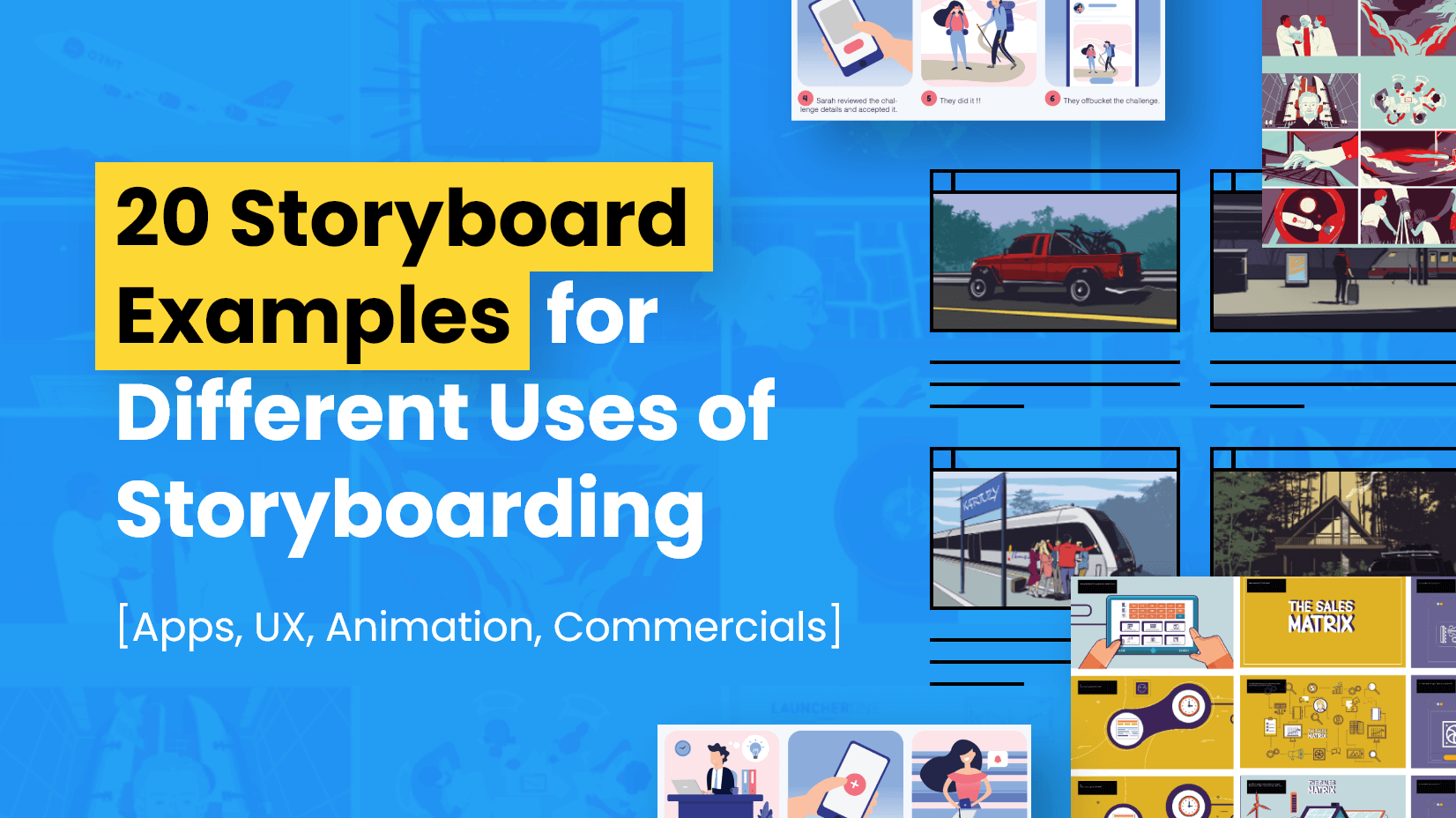
20 Storyboard Examples For Different Uses of Storyboarding [Apps, UX, Animation, Commercials]
by Al Boicheva
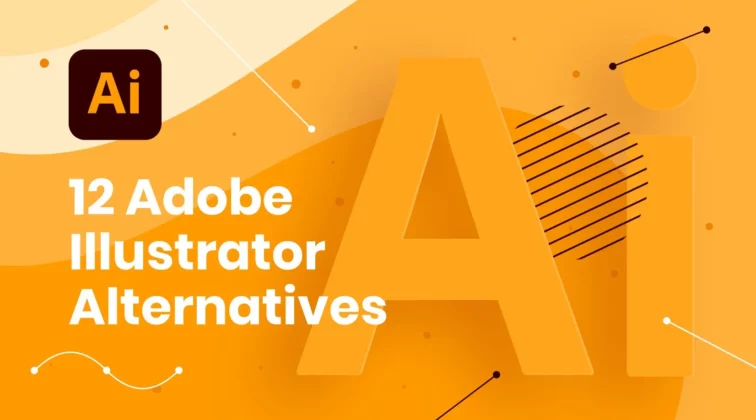
12 of the Best Adobe Illustrator Alternatives [Free & Paid]
by Nikolay Kaloyanov
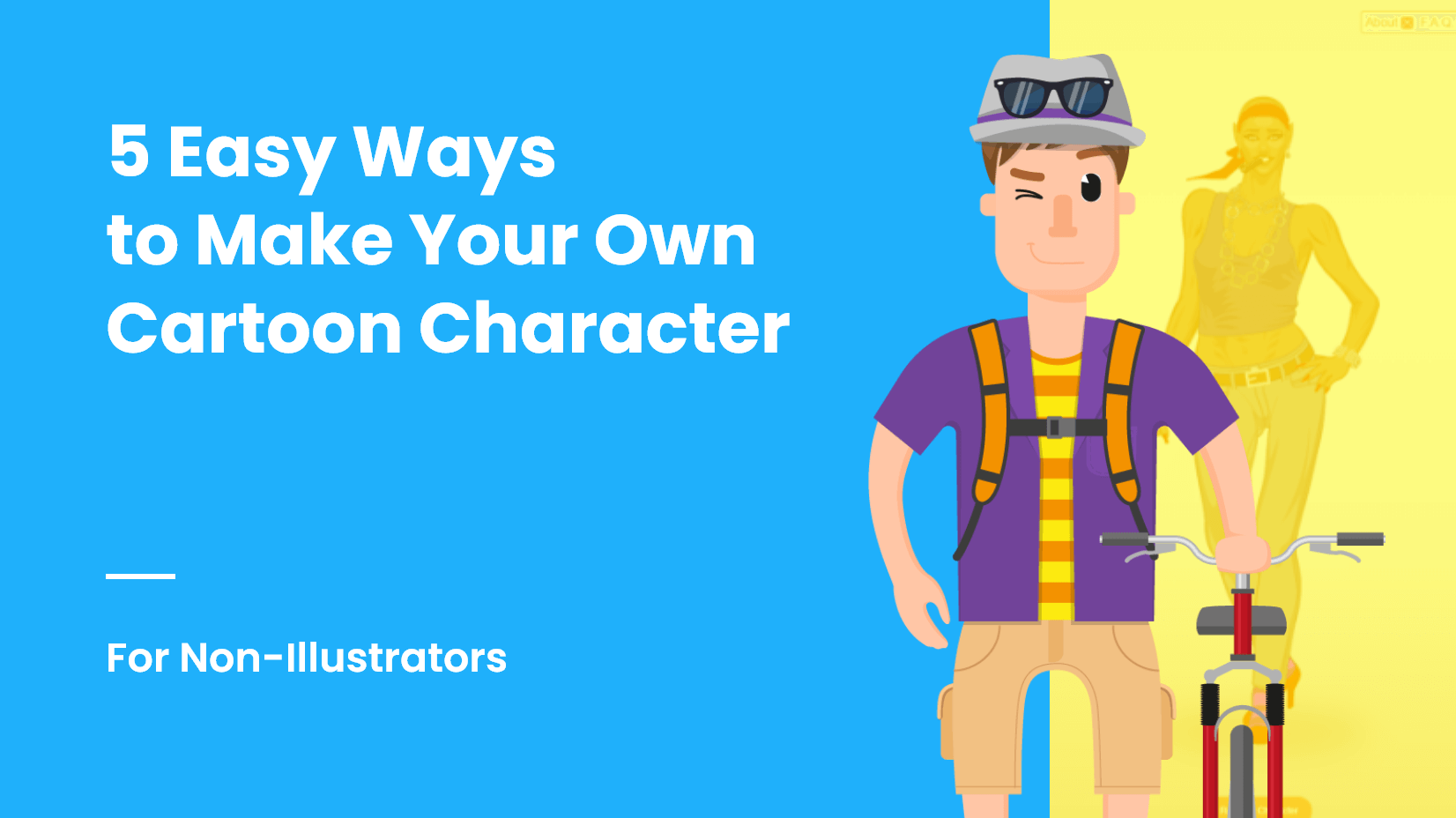
5 Easy Ways to Make Your Own Cartoon Character [For Non-Illustrators]
by Lyudmil Enchev
Looking for Design Bundles or Cartoon Characters?
A source of high-quality vector graphics offering a huge variety of premade character designs, graphic design bundles, Adobe Character Animator puppets, and more.

The Ultimate Guide to Data Visualization
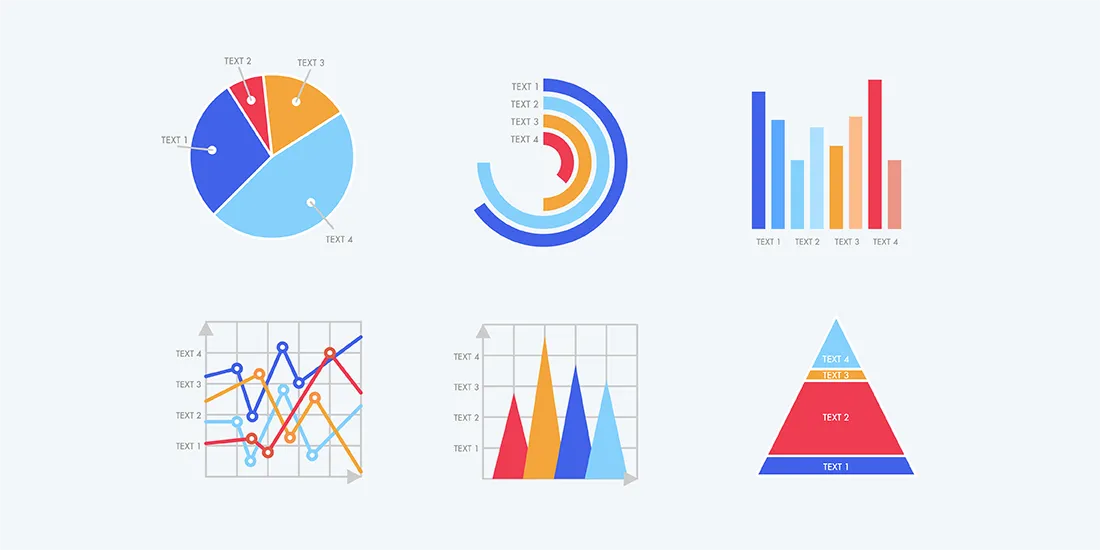
Data visualization is important because it breaks down complex data and extracts meaningful insights in a more digestible way. Displaying the data in a more engaging way helps audiences make sense of the information with a higher chance of retention. But with a variety of charts and graphs, how can you tell which is best for your specific content and audience?
Consider this your ultimate guide to data visualization. We’re breaking down popular charts and graphs and explaining the differences between each so that you can choose the best slide for your story.
Charts vs. graphs
We know that numbers don’t lie and are a strong way to back up your story, but that doesn’t always mean they’re easy to understand. By packaging up complex numbers and metrics in visually appealing graphics you’re telling your audience exactly what they need to know without having to rack their brain to comprehend it. Graphs and charts are important in your presentation because they take your supporting statistics, and story, and make them more relatable.
Charts present data or complex information through tables, infographics , and diagrams, while graphs show a connection between two or more sets of data.
A histogram is a visual representation of the distribution of data. The graph itself consists of a set of rectangles— each rectangle represents a range of values (called a "bin"), while the height corresponds to the numbers of the data that fall within that range.
Histograms are oftentimes used to visualize the frequency distribution of continuous data. Things such as measurements of height, weight, or time can all be organized in the graph. They can also be used to display the distribution of discrete data, like the number of shoes sold in a shoe department during any given period of time.
Histograms are a useful tool for analyzing data, as they allow you to quickly see the shape of the data distribution, the location of the central tendency (the mean or median), and the full spread of the data. They’re a great chart that can also reveal any changes in the data, making it easier to digest.
Need to add a little visual interest to your business presentation? A bar graph slide can display your data easily and effectively. Whether you use a vertical bar graph or horizontal bar graph, a bar graph gives you options to help simplify and present complex data, ensuring you get your point across.
Use it to track long-term changes.
Vertical bar graphs are great for comparing different groups that change over a long period of time. Small or short-term changes may not be as obvious in bar graph form.
Don’t be afraid to play with design .
You can use one bar graph template slide to display a lot of information, as long as you differentiate between data sets. Use colors, spacing, and labels to make the differences obvious.
Use a horizontal graph when necessary.
If your data labels are long, a horizontal bar graph may be easier to read and organize than a vertical bar graph.
Don’t use a horizontal graph to track time.
A vertical bar graph makes more sense when graphing data over time, since the x-axis is usually read from left to right.
Histograms vs. bar graphs
While a histogram is similar to a bar graph, it groups numbers into ranges and displays data in a different way.
Bar graphs are used to represent categorical data, where each bar represents a different category with a height or length proportional to the associated value. The categories of a bar graph don’t overlap, and the bars are usually separated by a gap to differentiate from one another. Bar graphs are ideal when you need to compare the data of different categories.
On the other hand, histograms divide data into a set of intervals or "bins". The bars of a histogram are typically adjacent to each other, with no gaps, as the bins are continuous and can overlap. Histograms are used to visualize the shape, center, and spread of a distribution of numerical data.
A pie chart is a circular graph (hence the name ‘pie’) that’s used to show or compare different segments — or ‘slices’ — of data. Each slice represents a proportion that relates to the whole. When added up, each slice should equal the total. Pie charts are best used for showcasing part-to-whole relationships. In other words, if you have different parts or percentages of a whole, using a pie chart is likely the way to go. Just make sure the total sum equals 100%, or the chart won’t make a lot of sense or convey the message you want it to. Essentially, any type of content or data that can be broken down into comparative categories is suitable to use. Revenue, demographics, market shares, survey results — these are just a few examples of the type of content to use in a pie chart. However, you don’t want to display more than six categories of data or the pie chart can be difficult to read and compare the relative size of slices.
Donut Charts
A donut chart is almost identical to a pie chart, but the center is cut out (hence the name ‘donut’). Donut charts are also used to show proportions of categories that make up the whole, but the center can also be used to display data. Like pie charts, donut charts can be used to display different data points that total 100%. These are also best used to compare a handful of categories at-a-glance and how they relate to the whole. The same type of content you’d use for a pie chart can also work for a donut chart. However, with donut charts, you have room for fewer categories than pie charts — anywhere from 2 to 5. That’s because you want your audience to be able to quickly tell the difference between arc lengths, which can help tell a more compelling story and get your point across more efficiently.
Pie charts vs. donut charts
You may notice that a donut chart and a pie chart look almost identical . While a donut chart is essentially the same as a pie chart in function, with its center cut out, the “slices” in a donut chart are sometimes more clearly defined than in a pie chart.
When deciding between a pie chart or a donut chart for your presentation, make sure the data you’re using is for comparison analysis only. Pie and donut charts are usually limited to just that — comparing the differences between categories. The easiest way to decide which one to use?
The number of categories you’re comparing. If you have more than 4 or 5 categories, go with a pie chart. If you have between 2 and 4 categories, go with a donut chart. Another way to choose? If you have an extra data point to convey (e.g. all of your categories equal an increase in total revenue), use a donut chart so you can take advantage of the space in the middle.
Comparison charts
As its name implies, a comparison chart or comparison graph draws a comparison between two or more items across different parameters. You might use a comparison chart to look at similarities and differences between items, weigh multiple products or services in order to choose one, or present a lot of data in an easy-to-read format.
For a visually interesting twist on a plain bar chart, add a data comparison slide to your presentation. Our data comparison template is similar to a bar graph, using bars of varying lengths to display measured data. The data comparison template, however, displays percentages instead of exact numbers. One of the best things about using Beautiful.ai’s data comparison slide? You can customize it for your presentation. Create a horizontal or vertical slide, remove or add grid lines, play with its design, and more.
Gantt charts
A Gantt chart , named after its early 20th century inventor Henry Gantt, is a birds-eye view of a project. It visually organizes tasks displayed over time. Gantt charts are incredibly useful tools that work for projects and groups of all sizes.
It’s a type of bar chart that you would use to show the start and finish dates of several elements of a project such as what the project tasks are, who is working on each task, how long each task will take, and how tasks group together, overlap, and link with each other. The left side of a Gantt chart lists each task in a project by name. Running along the top of the chart from left to right is a timeline. Depending on the demands and details of your project, the timeline may be broken down by quarter, month, week, or even day.
Project management can be complex, so it’s important to keep your chart simple by using a color scheme with cool colors like blues or greens. You can color code items thematically or by department or person, or even highlight a single task with a contrasting color to call attention to it. You can also choose to highlight important tasks using icons or use images for other annotations. This will make your chart easier to read and more visually appealing.
Additional tips for creating an effective Gantt chart slide .
Use different colors
How many colors you use and how you assign them is up to you. You might choose one color to represent a specific team or department so that you can see who is responsible for which tasks on your chart, for example.
Set milestones
Don’t forget to set milestones where they make sense: deadlines required by clients or customers, when a new department takes over the next phase of the project, or when a long list of tasks is completed.
Label your tasks
When used with a deliberate color scheme, labeling your tasks with its project owner will prevent confusion and make roles clear to everyone.

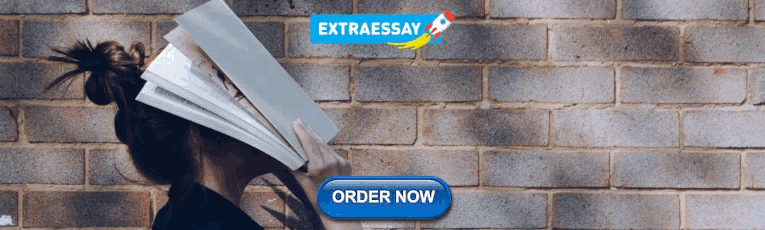
Jordan Turner
Jordan is a Bay Area writer, social media manager, and content strategist.
Recommended Articles
How to visualize your data using an infographic, 5 ways to use a horizontal bar chart, when and how to use the venn diagram, tell your story through hub and spoke imagery.
- Business Essentials
- Leadership & Management
- Credential of Leadership, Impact, and Management in Business (CLIMB)
- Entrepreneurship & Innovation
- Digital Transformation
- Finance & Accounting
- Business in Society
- For Organizations
- Support Portal
- Media Coverage
- Founding Donors
- Leadership Team
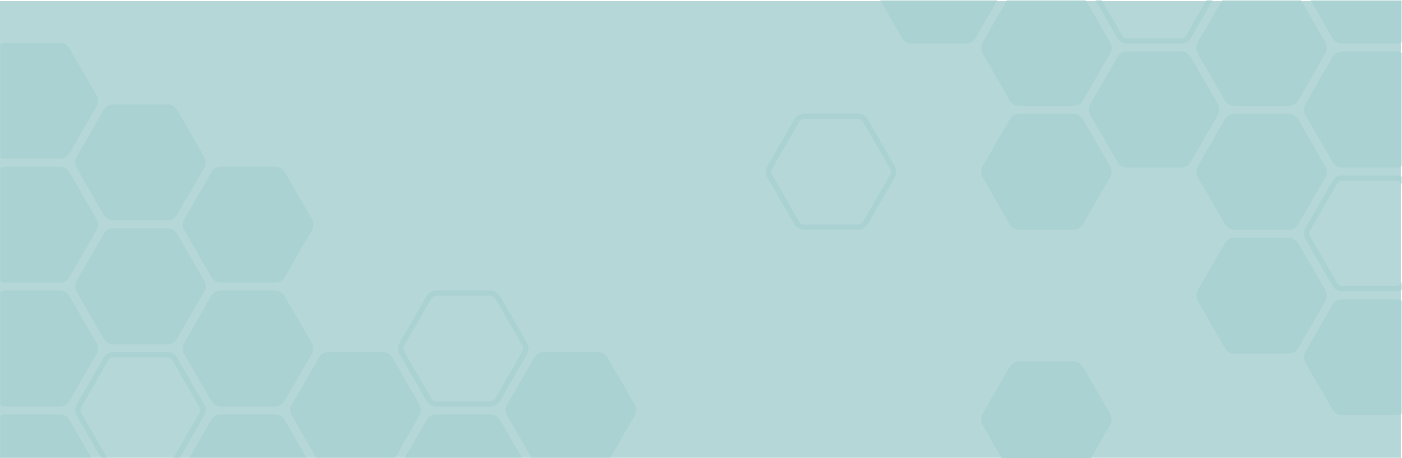
- Harvard Business School →
- HBS Online →
- Business Insights →
Business Insights
Harvard Business School Online's Business Insights Blog provides the career insights you need to achieve your goals and gain confidence in your business skills.
- Career Development
- Communication
- Decision-Making
- Earning Your MBA
- Negotiation
- News & Events
- Productivity
- Staff Spotlight
- Student Profiles
- Work-Life Balance
- AI Essentials for Business
- Alternative Investments
- Business Analytics
- Business Strategy
- Business and Climate Change
- Creating Brand Value
- Design Thinking and Innovation
- Digital Marketing Strategy
- Disruptive Strategy
- Economics for Managers
- Entrepreneurship Essentials
- Financial Accounting
- Global Business
- Launching Tech Ventures
- Leadership Principles
- Leadership, Ethics, and Corporate Accountability
- Leading Change and Organizational Renewal
- Leading with Finance
- Management Essentials
- Negotiation Mastery
- Organizational Leadership
- Power and Influence for Positive Impact
- Strategy Execution
- Sustainable Business Strategy
- Sustainable Investing
- Winning with Digital Platforms
6 Data Visualization Examples To Inspire Your Own
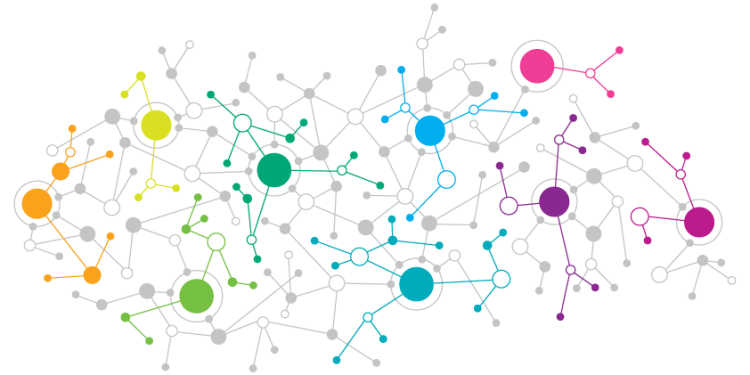
- 12 Jan 2017
Data informs virtually every business decision an organization makes. Because of this, it’s become increasingly important for professionals of all backgrounds to be adept at working with data.
While data can provide immense value, it’s important that professionals are able to effectively communicate the significance of the data to stakeholders. This is where data visualization comes into play. By transforming raw data into engaging visuals using various data visualization tools , it’s much easier to communicate insights gleaned from it.
Here are six real-world examples of data visualization that you can use to inspire your own.
What Is Data Visualization?
Data visualization is the process of turning raw data into graphical representations.
Visualizations make it easy to communicate trends in data and draw conclusions. When presented with a graph or chart, stakeholders can easily visualize the story the data is telling, rather than try to glean insights from raw data.
There are countless data visualization techniques , including:
- Scatter plots
The technique you use will vary based on the type of data you’re handling and what you’re trying to communicate.
6 Real-World Data Visualization Examples
1. the most common jobs by state.

Source: NPR
National Public Radio (NPR) produced a color-coded, interactive display of the most common jobs in each state in each year from 1978 to 2014. By dragging the scroll bar at the bottom of the map, you’re able to visualize occupational changes over time.
If you’re trying to represent geographical data, a map is the best way to go.
2. COVID-19 Hospitalization Rates

Source: CDC
Throughout the COVID-19 pandemic, the Centers for Disease Control and Prevention (CDC) has been transforming raw data into easily digestible visuals. This line graph represents COVID-19 hospitalization rates from March through November 2020.
The CDC tactfully incorporated color to place further emphasis on the stark increase in hospitalization rates, using a darker shade for lower values and a lighter shade for higher values.
3. Forecasted Revenue of Amazon.com

Source: Statista
Data visualizations aren’t limited to historical data. This bar chart created by Statista visualizes the forecasted gross revenue of Amazon.com from 2018 to 2025.
This visualization uses a creative title to summarize the main message that the data is conveying, as well as a darker orange color to spike out the most important data point.
4. Web-Related Statistics

Source: Internet Live Stats
Internet Live Stats has tracked web-related statistics and pioneered methods for visualizing data to show how different digital properties have ebbed and flowed over time.
Simple infographics like this one are particularly effective when your goal is to communicate key statistics rather than visualizing trends or forecasts.
5. Most Popular Food Delivery Items

Source: Eater
Eater, Vox’s food and dining brand, has created this fun take on a “pie” chart, which shows the most common foods ordered for delivery in each of the United States.
To visualize this data, Eater used a specific type of pie chart known as a spie chart. Spie charts are essentially pie charts in which you can vary the height of each segment to further visualize differences in data.
6. Netflix Viewing Patterns

Source: Vox
Vox created this interesting visualization depicting the viewing patterns of Netflix users over time by device type. This Sankey diagram visualizes the tendency of users to switch to streaming via larger device types.

Visualizing Data to Make Business Decisions
The insights and conclusions drawn from data visualizations can guide the decision-making and strategic planning processes for your organization.
To ensure your visualizations are relevant, accurate, and ethical, familiarize yourself with basic data science concepts . With a foundational knowledge in data science, you can maintain confidence in your data and better understand its significance. An online analytics course can help you get started.
Are you interested in improving your data science and analytical skills? Download our Beginner’s Guide to Data & Analytics to learn how you can leverage the power of data for professional and organizational success.
This post was updated on February 26, 2021. It was originally published on January 12, 2017.
What Is Data Visualization and Why Is It Important? A Complete Introduction
They say a picture is worth a thousand words, and this is especially true for data analytics.
Data visualization is all about presenting data in a visual format, using charts, graphs, and maps to tell a meaningful story. It’s a crucial step in the data analysis process—and a technique (or art form!) that all areas of business can benefit from.
In this guide, we’ll tell you everything you need to know about data visualization (also known as data viz). We’ll explain what it is, why it matters, some of the most common types, as well as the tools you can use to create them.
This guide is ideal for anyone who wants to present, communicate, and share data-driven insights.
If you’d like to learn more data analytics skills, try this free data short course .
- What is data visualization?
- Why is data visualization important?
- When should you visualize your data?
- Different types of data visualization and when to use them
- Top data visualization tools
- Best practices and principles for effective data visualization
- Getting started with data visualization
So: What is data visualization? Let’s start with a definition.
1. What is data visualization? A definition
Data visualization is the graphical or visual representation of data. It helps to highlight the most useful insights from a dataset, making it easier to spot trends, patterns, outliers, and correlations.
Imagine you’re presented with a spreadsheet containing rows and rows of data. You probably won’t be able to decipher the data without delving into it, and it’s unlikely that you’ll be able to spot trends and patterns at first glance.
Now imagine seeing the same data presented as a bar chart, or on a color-coded map. It’s much easier to see what the data is telling you, right?
That’s the whole point of data visualization. It makes insights visible to the naked eye, so that virtually anyone can see and understand what’s going on. When done well, data visualization tells a story.
This storytelling aspect is crucial as it makes your data actionable. There’s a huge difference between simply having lots of data versus actually understanding how to use it to drive actions and decisions—and data visualization bridges that gap.
There are two broad categories of data visualization: exploration and explanation. Let’s take a look at those now.
What are the two main types of data visualization? Exploration vs. explanation
We’ll look at specific types of data visualization later on, but for now, it’s important to distinguish between exploratory and explanatory data visualization.
In a nutshell, exploratory data visualization helps you figure out what’s in your data, while explanatory visualization helps you to communicate what you’ve found. Exploration takes place while you’re still analyzing the data, while explanation comes towards the end of the process when you’re ready to share your findings.
Exploration
When faced with a new dataset, one of the first things you’ll do is carry out an exploratory data analysis . This is where you investigate the dataset and identify some of its main features, laying the foundation for more thorough analysis.
At this stage, visualizations can make it easier to get a sense of what’s in your dataset and to spot any noteworthy trends or anomalies. Ultimately, you’re getting an initial lay of the land and finding clues as to what the data might be trying to tell you.
Explanation
Once you’ve conducted your analysis and have figured out what the data is telling you, you’ll want to share these insights with others.
These could be key business stakeholders who can take action based on the data, for example, or public audiences who have an interest in your topic area.
Explanatory data visualizations help you tell this story, and it’s up to you to determine which visualizations will help you to do so most effectively. We’ll introduce some of the most common types of data visualization (and when to use them) in section four.
Want to learn more about data visualization, and try your hand at creating visualizations of your own? Give this free introductory tutorial a go. We’ll show you, step by step, how to create bar charts, line graphs, and more for a real dataset in Google Sheets.
2. Why is data visualization important?
The importance of effective data visualization is rooted in the importance of data analytics in general.
We’re living in an increasingly data-rich world; at the start of 2020, the digital universe comprised approximately 44 zettabytes of data . For perspective, one zettabyte is roughly equal to a trillion gigabytes. By 2025, it’s estimated that around 463 exabytes of data will be created every 24 hours across the globe. An exabyte is equivalent to one billion gigabytes. Basically, we’re producing tons and tons of data all the time.
Data analytics allows us to make sense of (at least some of) that data. From a business perspective, it enables companies to learn from the past and plan ahead for the future. In fields like healthcare, it can help to improve patient care and treatment. In finance and insurance, it can help to assess risk and combat fraudulent activity. Essentially, we need data analytics in order to make smart decisions—and data visualization is a crucial part of that.
Data visualization helps us to understand what certain data is telling us, presenting it in a way that’s accessible to a range of audiences—not just data experts. It’s how you bridge the gap between your expertise as a data analyst or data scientist, and those people who can use or act upon the insights you discover.
A line graph and a bar chart taken from the Fitbit app.
The advantages and benefits of effective data visualization at a glance
Data visualization allows you to:
- Get an initial understanding of your data by making trends, patterns, and outliers easily visible to the naked eye
- Comprehend large volumes of data quickly and efficiently
- Communicate insights and findings to non-data experts, making your data accessible and actionable
- Tell a meaningful and impactful story, highlighting only the most relevant information for a given context
Now we know what data visualization is and why it matters, let’s take a look at when and why you might need to visualize your data.
3. When should you visualize your data?
Aside from exploratory data visualization which takes place in the early stages, data visualization usually comprises the final step in the data analysis process . To recap, the data analysis process can be set out as follows:
- Define the question: What problem are you trying to solve?
- Collect the data: Determine what kind of data you need and where you’ll find it.
- Clean the data: Remove errors, duplicates, outliers, and unwanted data points—anything that might skew how your data is interpreted. You can learn more about data cleaning (and how to do it) in this guide .
- Analyze the data: Determine the type of data analysis you need to carry out in order to find the insights you’re looking for.
- Visualize the data and share your findings: Translate your key insights into visual format (e.g. graphs, charts, or heatmaps) and present them to the relevant audience(s).
Essentially, you visualize your data any time you want to summarize and highlight key findings and share them with others. With that in mind, let’s consider what kinds of insights you can convey with data visualizations.
What is data visualization used for?
Within the broader goal of conveying key insights, different visualizations can be used to tell different stories. Data visualizations can be used to:
- Convey changes over time: For example, a line graph could be used to present how the value of Bitcoin changed over a certain time period.
- Determine the frequency of events: You could use a histogram to visualize the frequency distribution of a single event over a certain time period (e.g. number of internet users per year from 2007 to 2021). Learn how to create a histogram in this guide .
- Highlight interesting relationships or correlations between variables: If you wanted to highlight the relationship between two variables (e.g. marketing spend and revenue, or hours of weekly exercise vs. cardiovascular fitness), you could use a scatter plot to see, at a glance, if one increases as the other decreases (or vice versa).
- Examine a network: If you want to understand what’s going on within a certain network (for example, your entire customer base), network visualizations can help you to identify (and depict) meaningful connections and clusters within your network of interest.
- Analyze value and risk: If you want to weigh up value versus risk in order to figure out which opportunities or strategies are worth pursuing, data visualizations—such as a color-coded system—could help you to categorize and identify, at a glance, which items are feasible.
So far, we’ve taken a rather broad, high-level look at data visualization. Now let’s drill down to some specific types of data visualization and when to use them.
An example of data visualization, as seen in the Fitbit app.
4. How to visualize your data: Different types of data visualization (and when to use them)
There are many different options when it comes to visualizing your data. The visualization you choose depends on the type of data you’re working with and what you want to convey or highlight. It’s also important to consider the complexity of your data and how many different variables are involved. Not all types of data visualization lend themselves to elaborate or complex depictions, so it’s important to choose a suitable technique.
Before we explore some of the most common types of data visualization, let’s first introduce five main data visualization categories.
Five data visualization categories
When considering the different types of data viz, it helps to be aware of the different categories that these visualizations may fall into:
- Temporal data visualizations are linear and one-dimensional. Examples include scatterplots, timelines, and line graphs.
- Hierarchical visualizations organize groups within larger groups, and are often used to display clusters of information. Examples include tree diagrams, ring charts, and sunburst diagrams.
- Network visualizations show the relationships and connections between multiple datasets. Examples include matrix charts, word clouds, and node-link diagrams.
- Multidimensional or 3D visualizations are used to depict two or more variables. Examples include pie charts, Venn diagrams, stacked bar graphs, and histograms.
- Geospatial visualizations convey various data points in relation to physical, real-world locations (for example, voting patterns across a certain country). Examples include heat maps, cartograms, and density maps.
With those categories in mind, let’s explore some of the most common types of data visualization.
Five common types of data visualization (and when to use them)
In this section, we’ll introduce some useful types of data visualization. We’ll also point you to our more comprehensive guide where you can learn about additional data visualization methods and how to use them.
1. Scatterplots
Scatterplots (or scatter graphs) visualize the relationship between two variables. One variable is shown on the x-axis, and the other on the y-axis, with each data point depicted as a single “dot” or item on the graph. This creates a “scatter” effect, hence the name.
Source: displayr.com
Scatterplots are best used for large datasets when there’s no temporal element. For example, if you wanted to visualize the relationship between a person’s height and weight, or between how many carats a diamond measures and its monetary value, you could easily visualize this using a scatterplot.
It’s important to bear in mind that scatterplots simply describe the correlation between two variables; they don’t infer any kind of cause-and-effect relationship.
2. Bar charts
Bar charts are used to plot categorical data against discrete values.
Categorical data refers to data that is not numeric, and it’s often used to describe certain traits or characteristics. Some examples of categorical data include things like education level (e.g. high school, undergrad, or post-grad) and age group (e.g. under 30, under 40, under 50, or 50 and over).
Discrete values are those which can only take on certain values—there are no “half measures” or “gray areas.” For example, the number of people attending an event would be a discrete variable, as would the number of sales made in a certain time period (think about it: you can’t make “half a sale” or have “half an event attendee.”)
Source: chartio.com
So, with a bar chart, you have your categorical data on the x-axis plotted against your discrete values on the y-axis.
The height of the bars is directly proportional to the values they represent, making it easy to compare your data at a glance.
3. Pie charts
Just like bar charts, pie charts are used to visualize categorical data.
However, while bar charts represent multiple categories of data, pie charts are used to visualize just one single variable broken down into percentages or proportions. A pie chart is essentially a circle divided into different “slices,” with each slice representing the percentage it contributes to the whole.
Thus, the size of each pie slice is proportional to how much it contributes to the whole “pie.”
Imagine you have a class of thirty students and you want to divide them up based on what color t-shirt they’re wearing on a given day.
The possible “slices” are red, green, blue, and yellow, with each color representing 40%, 30%, 25%, and 5% of the class total respectively. You could easily visualize this using a pie chart—and the yellow slice (5%) would be considerably thinner than the red slice (40%)! Pie charts are best suited for data that can be split into a maximum of five or six categories.
4. Network graphs
Not all data is simple enough to be summarized in a bar or pie chart. For those more complex datasets, there are a range of more elaborate data visualizations at your disposal—network graphs being one of them.
Network graphs show how different elements or entities within a network relate to one another, with each element represented by an individual node. These nodes are connected to other, related nodes via lines.
Source: networkofthrones.wordpress.com
Network graphs are great for spotting and representing clusters within a large network of data.
Let’s imagine you have a huge database filled with customers, and you want to segment them into meaningful clusters for marketing purposes. You could use a network graph to draw connections and parallels between all your customers or customer groups.
With any luck, certain clusters and patterns would emerge, giving you a logical means by which to group your audience.
5. Geographical maps
Geo maps are used to visualize the distribution of data in relation to a physical, geographical area.
For example, you could use a color-coded map to see how natural oil reserves are distributed across the world, or to visualize how different states voted in a political election. Maps are an extremely versatile form of data visualization, and are an excellent way of communicating all kinds of location-related data.
Some other types of maps used in data visualization include dot distribution maps (think scatterplots combined with a map), and cartograms which distort the size of geographical areas to proportionally represent a given variable (population density, for example).
Source: pmfias.com
Here, we’ve introduced just a handful of data visualization types. If you want to learn more, check out our complete guide to different types of data visualization and when to use them .
5. Top data visualization tools
When it comes to creating informative, eye-catching visualizations, there are plenty of tools at your disposal.
When choosing a tool, it’s important to consider your needs in terms of the kinds of visualizations you want to create, as well as your own technical expertise; some tools will require coding knowledge, while others are more suited to non-technical users.
In this section, we’ll briefly introduce some of the most popular data visualization tools. If you’re on the market for a data viz tool and want a more thorough comparison, this guide to the seven best data visualization tools will help you. For now, here are our top three data viz tools to get familiar with:
- Plotly: Open-source software built on Python. Plotly is ideal if you’ve got some coding knowledge and want to create highly customizable visualizations.
- D3.js: A free, open-source data viz library built using JavaScript. As with Plotly, you’ll need some programming knowledge in order to use this data viz tool.
- Tableau: Perhaps one of the most popular data analytics tools , Tableau is known for its user-friendliness—you don’t need any coding knowledge to create beautiful visualizations in Tableau. And, unlike some other BI tools, it’s good at handling large volumes of data.
Before deciding on a tool, it’s worth trying out a few options. The good news is that there are plenty of data viz tools on the market— as well as a number of free tools —allowing you to create beautiful and informative visualizations—even if you’re a newcomer to the field.
What are data dashboards?
Dashboards are another useful tool for data tracking and visualization. A data dashboard essentially allows you to keep track of multiple data sources, visualizing them in one single location for easy viewing.
A common example is the Google Analytics dashboard , which displays a whole host of visualizations on one page—a geo map showing where your website visitors are located, for example, or a pie chart showing what percentage of your users access your website using specific devices.
If you want multiple stakeholders to be able to access and view certain data insights, a dashboard can help you to create a single hub with easy-to-understand visualizations.
A snapshot of a data dashboard, taken from Google Analytics.
6. What are some data visualization best practices?
Data visualization truly is an art form—but the goal is always, first and foremost, to provide valuable information and insights.
If you can do this by way of beautiful visualizations, you’re onto a winner. So, when creating data visualizations, it’s important to adhere to certain best practices.
These will help you strike the right balance, keeping your audience engaged and informed. Here’s how to excel at data visualization.
1. Define a clear purpose
Like any data analytics project, it’s important to define a clear purpose for your data visualizations.
What are the priorities in terms of what you want to convey and communicate? What should your audience take away from your visualization? It’s essential to have this defined from the outset; that way, you can ensure that you’re only presenting the most valuable information—and giving your audience something they can use and act upon.
2. Know your audience
The purpose of data visualization is to communicate insights to a specific audience, so you’ll want to give some thought to who your audience is and how familiar they are with the information you’re presenting.
What kind of context can you provide around your visualizations in order to help your audience understand them? What types of visualization are likely to be most accessible to this particular group of people? Keep your audience in mind at all times.
3. Keep it simple
When creating visualizations, it’s often the case that less is more.
Ultimately, you want your visualizations to be as digestible as possible, and that means trimming away any unnecessary information while presenting key insights clearly and succinctly. The goal is to keep cognitive load to a minimum—that is, the amount of “brainpower” or mental effort it takes to process information.
Even if the data is complex, your visualizations don’t have to be, so strive for simplicity at all times.
4. Avoid distorting the data
You should strive to present your findings as accurately as possible, so avoid any kind of visual “tricks” that could bias how your data is perceived and interpreted.
Think about the labels you use, as well as how you scale your visualizations. For example, things like “blowing up” certain data segments to make them appear more significant, or starting your graph axis on a number other than zero are both bad practices which could mislead your audience. Prioritize integrity and accuracy!
5. Ensure your visualizations are inclusive
Last but by no means least, make sure that your visualizations are accessible and inclusive.
Think about how colors, contrasts, font sizes, and the use of white space affect the readability of your visualization. Is it easy for your users to distinguish between the data and see what’s going on, regardless of whether they have twenty-twenty vision or a visual impairment?
Inclusivity and accessibility are central to good data visualization, so don’t overlook this step.
7. Getting started with data visualization
By now, you hopefully have a good understanding of what data visualization is and why it matters.
Of course, the best way to get to grips with it is to see it in action. Check out our round-up of some of the most beautiful and informative data visualization examples from around the web.
Keen to give it a go yourself? Why not download a free dataset and see what you can do! If you’d like to learn it more, then check out this list of data visualization courses out there to try.
Data visualization is an excellent skill to have, whether you’re forging a career in the data industry or just want to share valuable insights with your colleagues. If you are pursuing a career as a data analyst or data scientist, be sure to include data visualizations in your data portfolio —it’s something that employers will be looking out for.
CareerFoundry’s Data Visualizations with Python course is designed to ease you into this vital area of data analytics. You can take it as a standalone course as well as a specialization within our full Data Analytics Program, you’ll learn and apply the principles of data viz in a real-world project, as well as getting to grips with various data visualization libraries.
Want to learn more? Try your hand at this free, introductory data analytics short course , and check out the following guides:
- What is data quality and why is it important?
- What is web scraping? A beginner’s guide
- An introduction to multivariate analysis
- Reviews / Why join our community?
- For companies
- Frequently asked questions
Information Visualization
What is information visualization.
Information visualization is the process of representing data in a visual and meaningful way so that a user can better understand it. Dashboards and scatter plots are common examples of information visualization. Via its depicting an overview and showing relevant connections, information visualization allows users to draw insights from abstract data in an efficient and effective manner.
Information visualization plays an important role in making data digestible and turning raw information into actionable insights. It draws from the fields of human-computer interaction, visual design, computer science, and cognitive science, among others. Examples include world map-style representations, line graphs, and 3-D virtual building or town plan designs.
The process of creating information visualization typically starts with understanding the information needs of the target user group. Qualitative research (e.g., user interviews) can reveal how, when, and where the visualization will be used. Taking these insights, a designer can determine which form of data organization is needed for achieving the users’ goals. Once information is organized in a way that helps users understand it better—and helps them apply it so as to reach their goals—visualization techniques are the next tools a designer brings out to use. Visual elements (e.g., maps and graphs) are created, along with appropriate labels, and visual parameters such as color, contrast, distance, and size are used to create an appropriate visual hierarchy and a visual path through the information.
Information visualization is becoming increasingly interactive, especially when used in a website or application. Being interactive allows for manipulation of the visualization by users, making it highly effective in catering to their needs. With interactive information visualization, users are able to view topics from different perspectives, and manipulate their visualizations of these until they reach the desired insights. This is especially useful if users require an explorative experience.
Questions related to Information Visualization
There are many types of information visualization . And different types cater to diverse needs. The most common forms include charts, graphs, diagrams, and maps. Charts, like bar graphs, succinctly display data trends. Diagrams, such as flowcharts, convey processes. Maps visually represent spatial information, enhancing geographical insights.
Each type serves a unique purpose, offering a comprehensive toolkit for effective information representation.
Information visualization and data visualization share a connection but diverge in scope. Data visualization centers on graphically representing raw data using charts or graphs. Information visualization extends beyond raw data, embracing a comprehensive array of contextual details and intricate datasets. It strives for a complete presentation, often employing interactivity to convey insights.
Data visualization concentrates on visually representing data points. Conversely, information visualization adopts a holistic approach. It considers the context for deeper comprehension and decision-making.
This video illustrates this concept using a routine example. It highlights the creative process and the importance of capturing and structuring ideas for effective communication.
- Transcript loading…
Information visualization and infographics play unique roles. Human memory is visual, often remembering images and patterns more than raw data. Information visualization capitalizes on this aspect. It simplifies complex data through graphics for better understanding.
This article gives valuable insights into the properties of human memory and their significance for information visualization .
Infographics portray information in engaging formats, often for storytelling or marketing. Both use visuals, but information visualization prioritizes clarity for users and turning data into usable insights. However, the latter focuses on effective communication and engagement.
No, Information Design and data visualization are distinctive in their objectives and applications. Information Design is a broader concept. It helps organize and present information to improve communication in the bigger picture. It considers the text, images, and layout to convey information effectively.
On the other hand, data visualization translates raw data into graphical representations. It extracts meaningful insights and patterns. The approach focuses on visual elements to simplify the analysis of complex datasets.
Information visualization is a process that transforms complex data into easy-to-understand visuals. The seven stages include:
Data collection: Gathering relevant data from diverse sources to form the basis for visualization.
Data analysis: Examining and processing the collected data to identify patterns, trends, and insights.
Data pre-processing: Cleaning and organizing the data to make it suitable for visualization.
Visual representation: Choosing appropriate visualization techniques to represent data accurately and effectively.
Interaction design: Developing user-friendly interfaces that allow meaningful interaction with the visualized data.
Interpretation: Enabling users to interpret and derive insights from the visualized information.
Evaluation: Assessing the effectiveness of the visualization in conveying information and meeting objectives.
This article provides a comprehensive overview of the data analysis process and explores key techniques for analysis.
Information visualization helps people understand data and make decisions. It turns complicated data into easy-to-understand visuals. This makes it easier to see patterns and get a good overall picture. It also helps people communicate by showing information in a visually exciting way. Visualizations empower individuals to interact with data, enhancing engagement and enabling deeper exploration. Additionally, visual representations facilitate easier retention and recall of information.
Data visualization has advantages and disadvantages. One big challenge is misinterpretation. The visualization of data can be misleading if presented inappropriately. It can also lead to false conclusions, especially for those who do not understand the information.
Another major problem is too much information, as this article explains: Information Overload, Why it Matters, and How to Combat It . A crowded or complex visualization can overwhelm users and make communicating difficult.
Also, making good visualizations takes time and skill. This can sometimes be challenging for newbies.
Data visualization is a powerful tool. Creating valuable and impactful visualizations requires a combination of skills. You must understand the data, choose suitable visualization methods, and tell a compelling story . All this requires a good understanding of data and design, as explained in this video.
Interpreting complex data and choosing compelling visualizations can be challenging for beginners. However, leveraging available resources and enhancing skills can simplify data visualization despite the occasional difficulty.
Check out this course to learn more about Information Visualization . The course also explains the connection between the eye and the brain in creating images. It looks at the history of information visualization, how it has evolved, and common mistakes that you must avoid in visual perception.
It will teach you how to design compelling information visualizations and use various techniques for your projects.
Literature on Information Visualization
Here’s the entire UX literature on Information Visualization by the Interaction Design Foundation, collated in one place:
Learn more about Information Visualization
Take a deep dive into Information Visualization with our course Information Visualization .
Information visualization skills are in high demand, partly thanks to the rise in big data. Tech research giant Gartner Inc. observed that digital transformation has put data at the center of every organization. With the ever-increasing amount of information being gathered and analyzed, there’s an increasing need to present data in meaningful and understandable ways.
In fact, even if you are not involved in big data, information visualization will be able to help in your work processes as a designer. This is because many design processes—including conducting user interviews and analyzing user flows and sales funnels—involve the collation and presentation of information. Information visualization turns raw data into meaningful patterns, which will help you find actionable insights. From designing meaningful interfaces, to processing your own UX research, information visualization is an indispensable tool in your UX design kit.
This course is presented by Alan Dix, a former professor at Lancaster University in the UK. A world-renowned authority in the field of human-computer interaction, Alan is the author of the university-level textbook Human-Computer Interaction . “Information Visualization” is full of simple but practical lessons to guide your development in information visualization. We start with the basics of what information visualization is, including its history and necessity, and then walk you through the initial steps in creating your own information visualizations. While there’s plenty of theory here, we’ve got plenty of practice for you, too.
All open-source articles on Information Visualization
Information overload, why it matters and how to combat it.
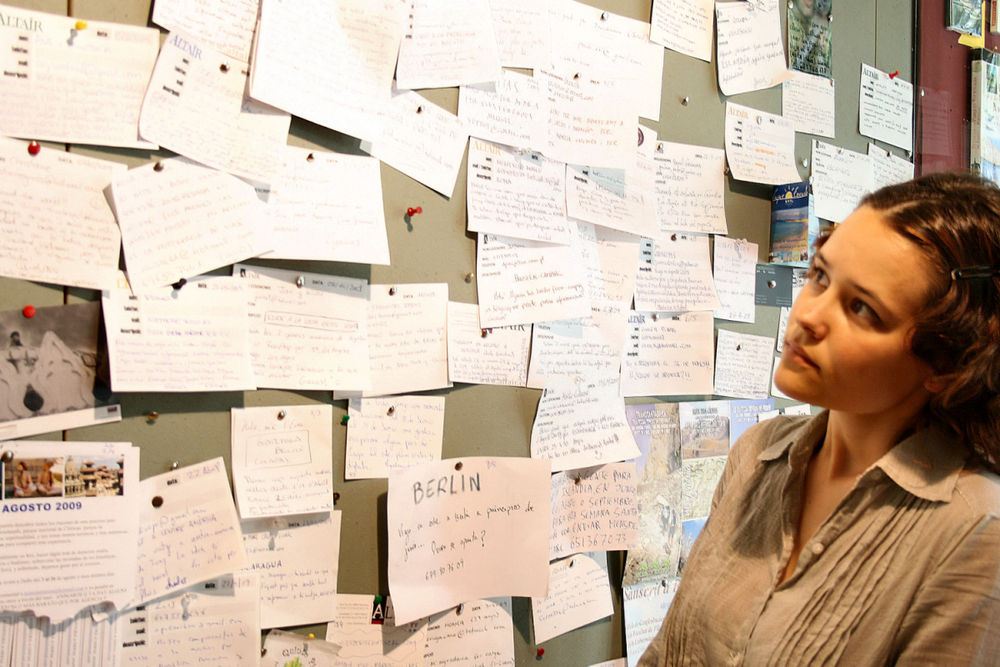
- 1.1k shares
- 4 years ago
Visual Representation
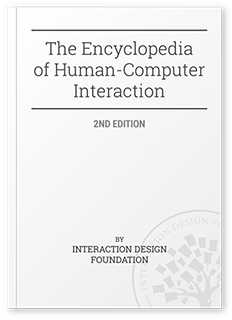
How to Design an Information Visualization
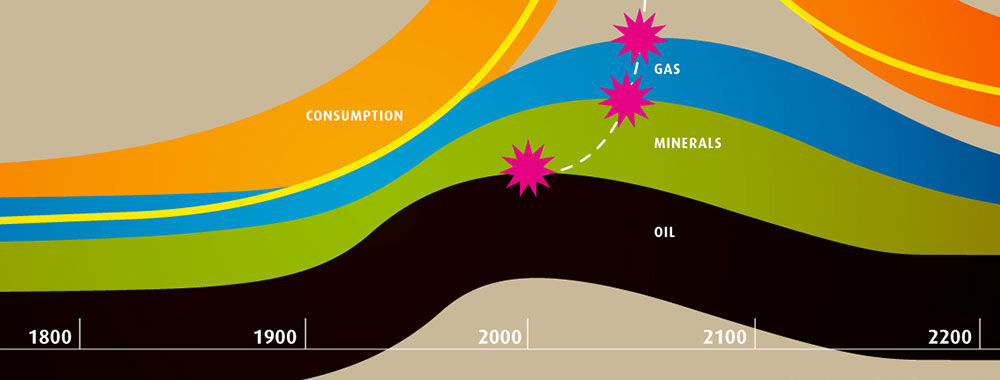
How to Visualize Your Qualitative User Research Results for Maximum Impact
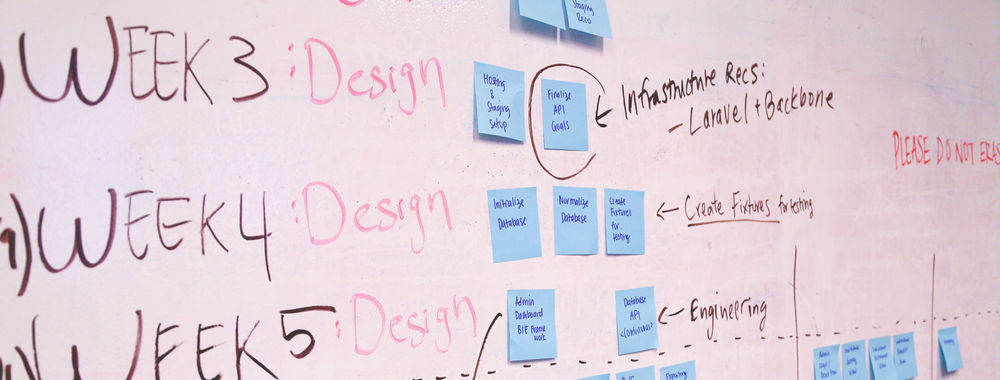
- 3 years ago
Preattentive Visual Properties and How to Use Them in Information Visualization
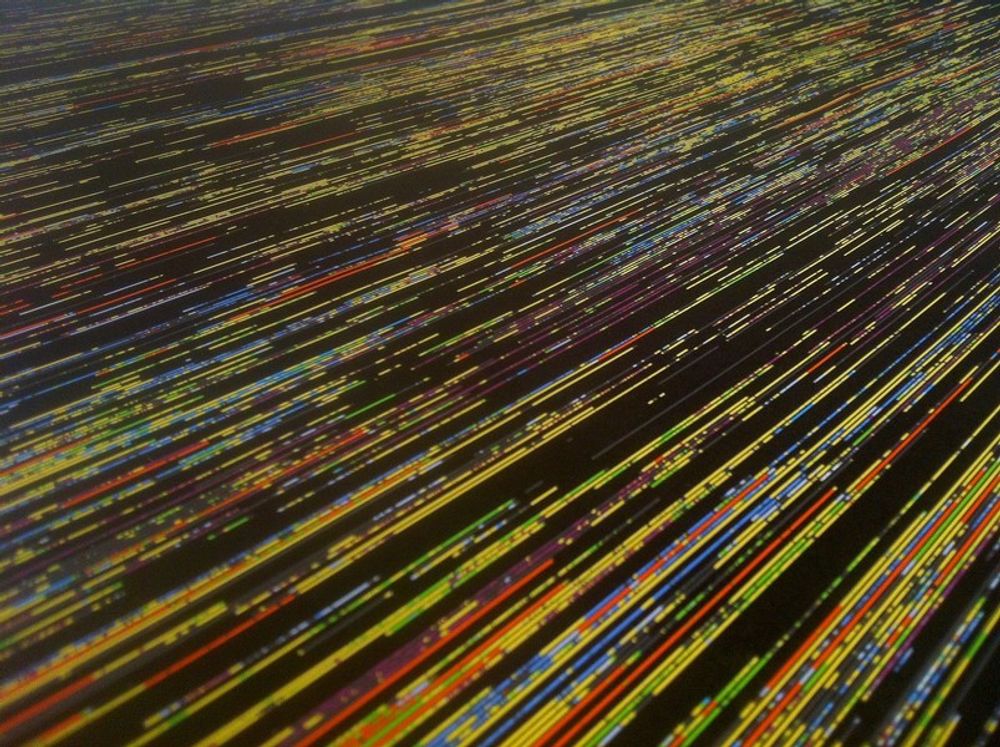
- 5 years ago
How to Conduct Focus Groups
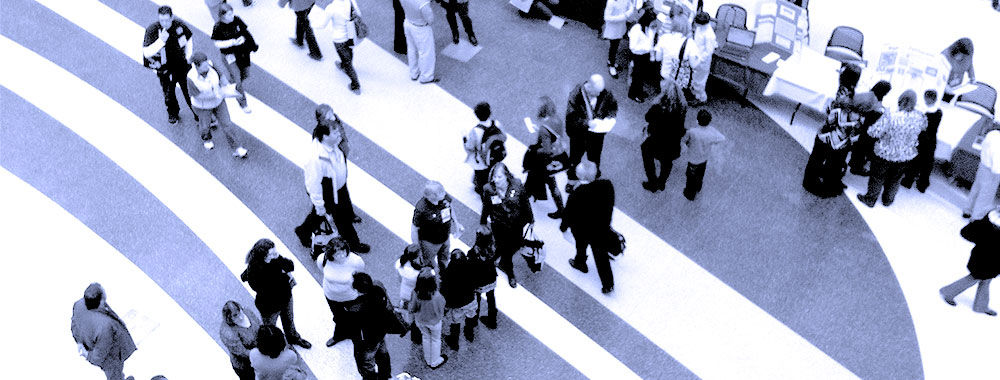
The Properties of Human Memory and Their Importance for Information Visualization
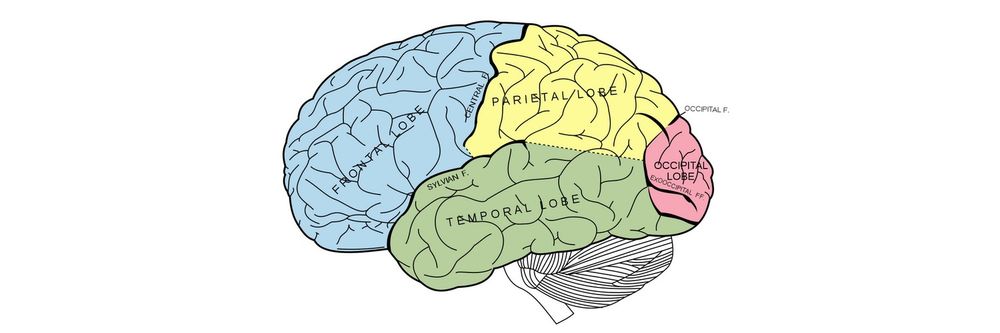
- 7 years ago
Information Visualization – A Brief Introduction
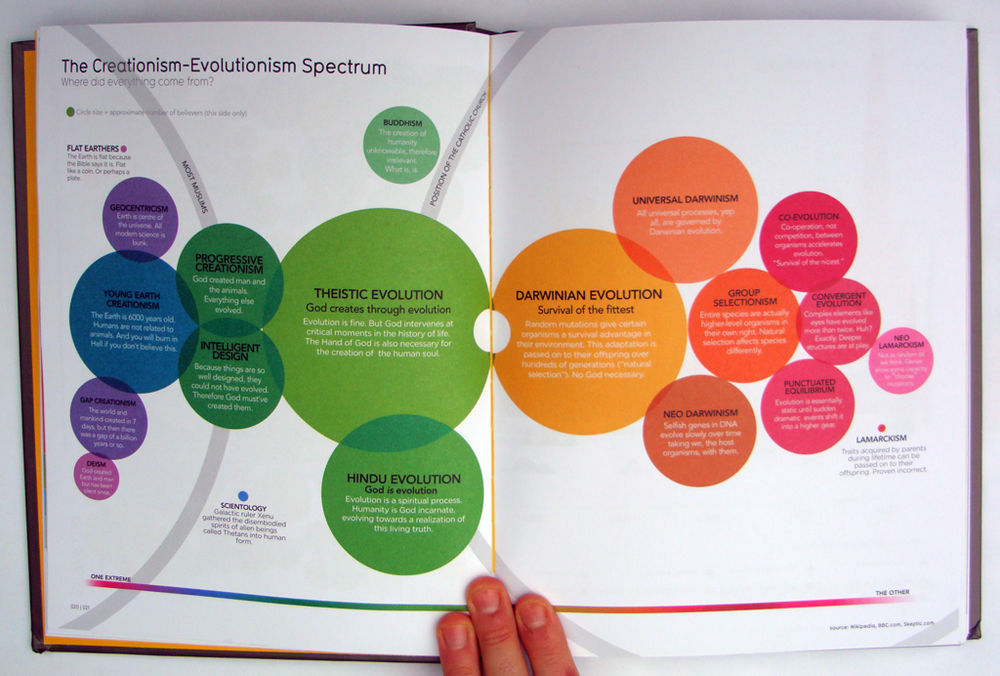
Visual Mapping – The Elements of Information Visualization
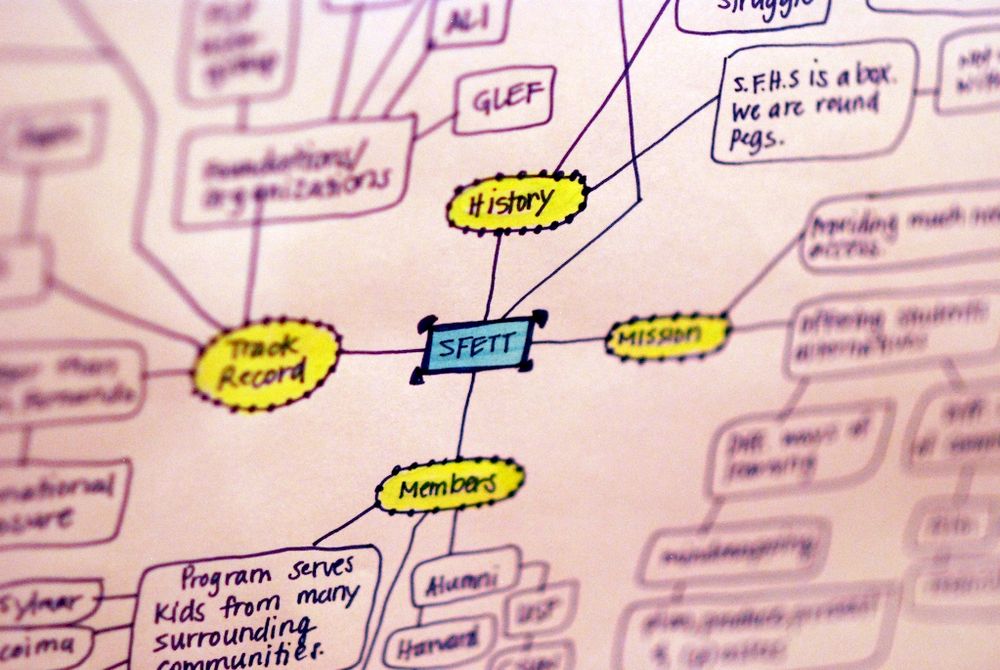
Guidelines for Good Visual Information Representations
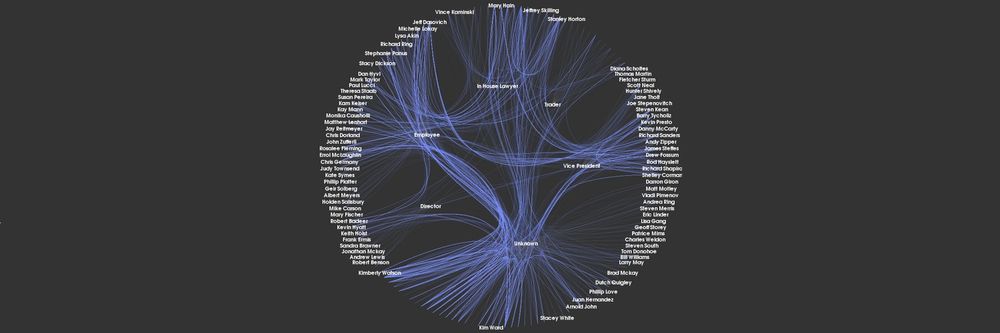
How to Show Hierarchical Data with Information Visualization
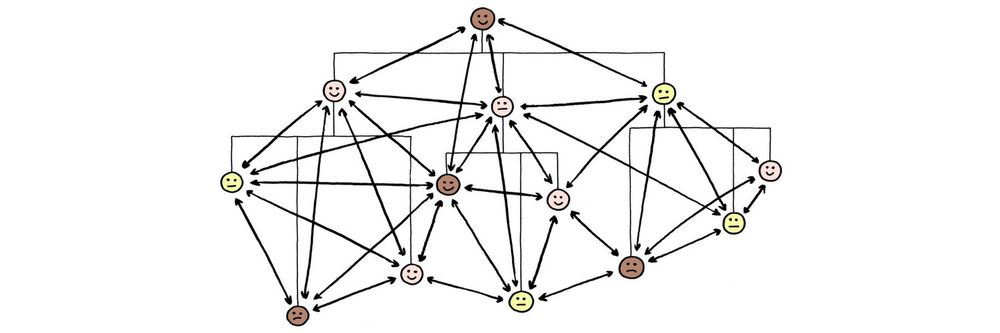
Information Visualization – An Introduction to Multivariate Analysis
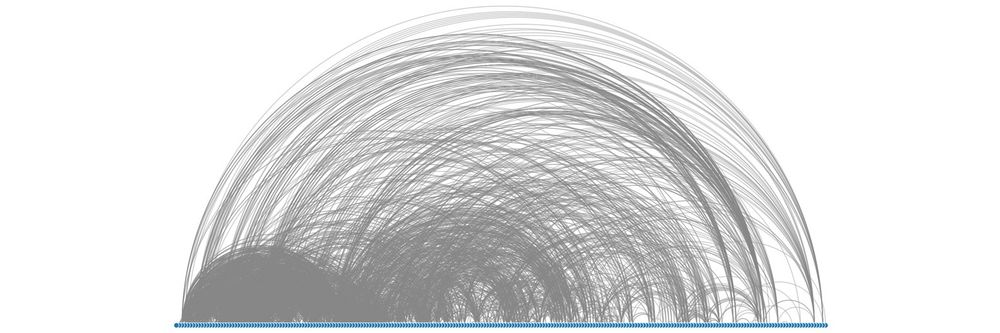
- 8 years ago
How to Display Complex Network Data with Information Visualization
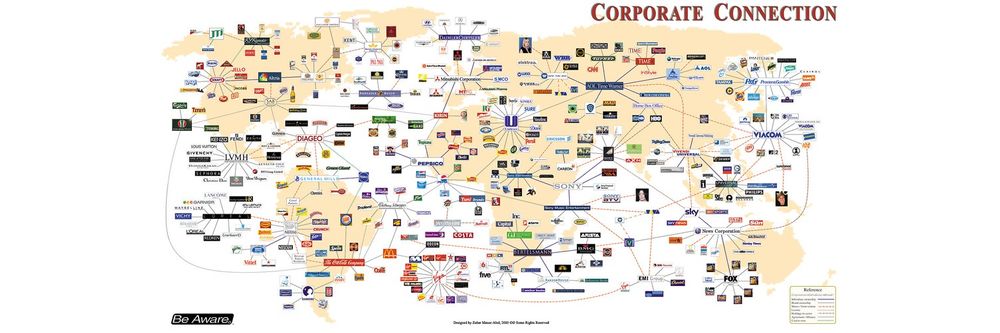
Information Visualization – Who Needs It?
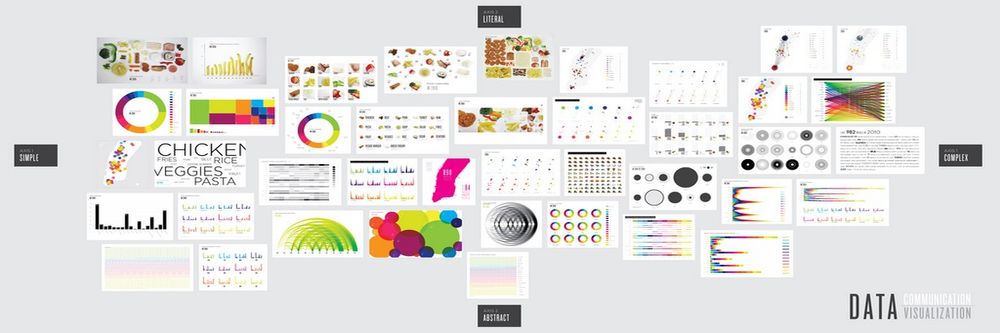
Vision and Visual Perception Challenges
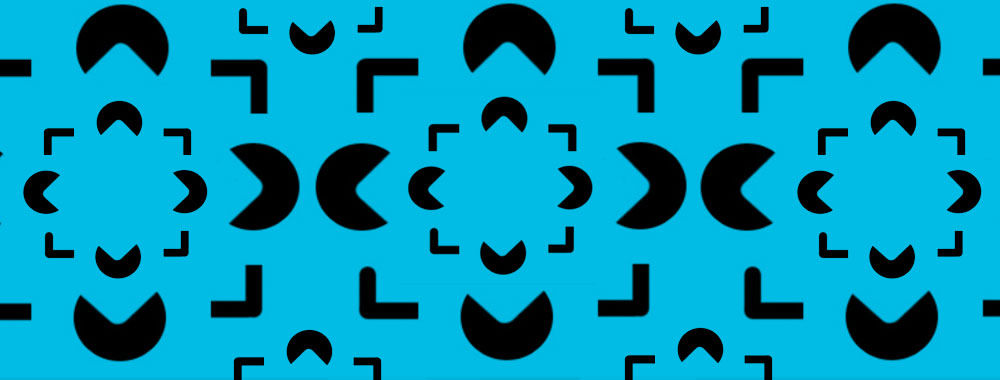
Information Visualization an Introduction to Transformable Information Representations
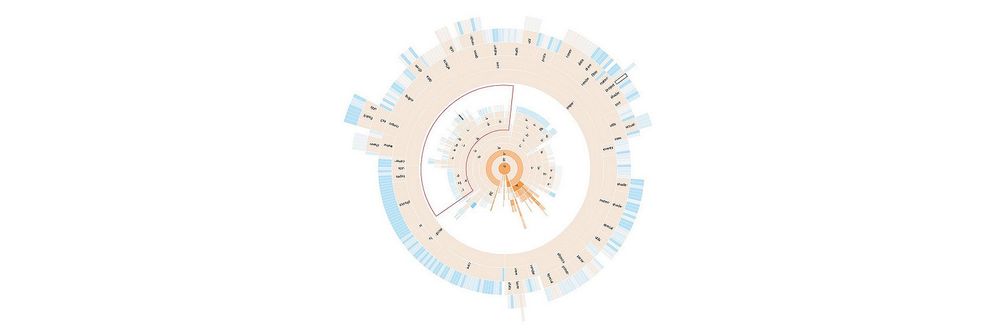
The Principles of Information Visualization for Basic Network Data
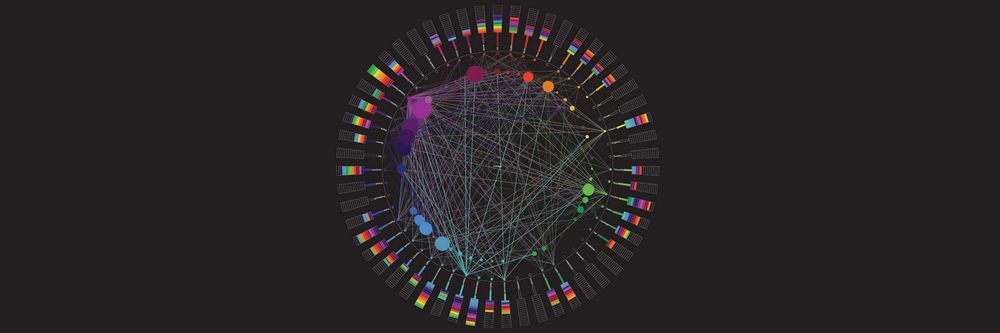
The Continuum of Understanding and Information Visualization
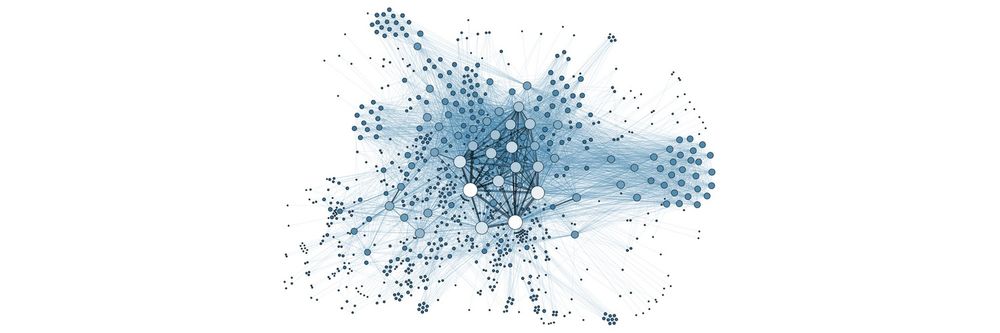
- 6 years ago
Information Visualization – A Brief Pre-20th Century History
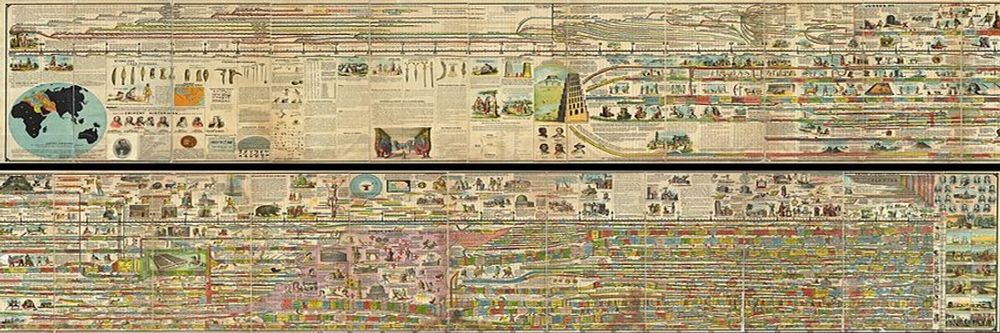
Information Visualization an Introduction to Manipulable Information Representations
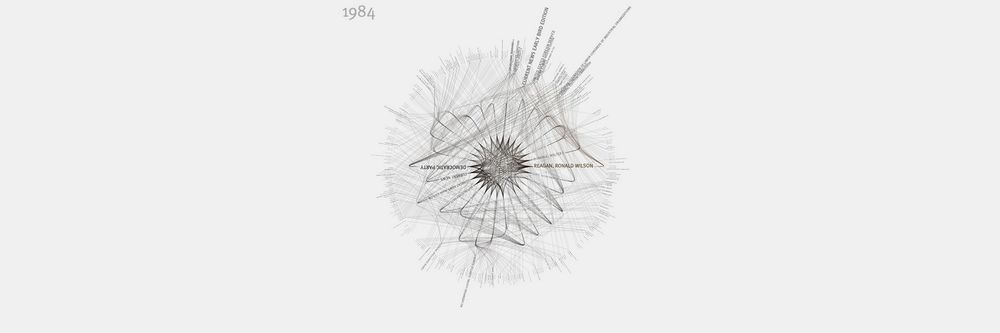
Open Access—Link to us!
We believe in Open Access and the democratization of knowledge . Unfortunately, world-class educational materials such as this page are normally hidden behind paywalls or in expensive textbooks.
If you want this to change , cite this page , link to us, or join us to help us democratize design knowledge !
Privacy Settings
Our digital services use necessary tracking technologies, including third-party cookies, for security, functionality, and to uphold user rights. Optional cookies offer enhanced features, and analytics.
Experience the full potential of our site that remembers your preferences and supports secure sign-in.
Governs the storage of data necessary for maintaining website security, user authentication, and fraud prevention mechanisms.
Enhanced Functionality
Saves your settings and preferences, like your location, for a more personalized experience.
Referral Program
We use cookies to enable our referral program, giving you and your friends discounts.
Error Reporting
We share user ID with Bugsnag and NewRelic to help us track errors and fix issues.
Optimize your experience by allowing us to monitor site usage. You’ll enjoy a smoother, more personalized journey without compromising your privacy.
Analytics Storage
Collects anonymous data on how you navigate and interact, helping us make informed improvements.
Differentiates real visitors from automated bots, ensuring accurate usage data and improving your website experience.
Lets us tailor your digital ads to match your interests, making them more relevant and useful to you.
Advertising Storage
Stores information for better-targeted advertising, enhancing your online ad experience.
Personalization Storage
Permits storing data to personalize content and ads across Google services based on user behavior, enhancing overall user experience.
Advertising Personalization
Allows for content and ad personalization across Google services based on user behavior. This consent enhances user experiences.
Enables personalizing ads based on user data and interactions, allowing for more relevant advertising experiences across Google services.
Receive more relevant advertisements by sharing your interests and behavior with our trusted advertising partners.
Enables better ad targeting and measurement on Meta platforms, making ads you see more relevant.
Allows for improved ad effectiveness and measurement through Meta’s Conversions API, ensuring privacy-compliant data sharing.
LinkedIn Insights
Tracks conversions, retargeting, and web analytics for LinkedIn ad campaigns, enhancing ad relevance and performance.
LinkedIn CAPI
Enhances LinkedIn advertising through server-side event tracking, offering more accurate measurement and personalization.
Google Ads Tag
Tracks ad performance and user engagement, helping deliver ads that are most useful to you.
Share Knowledge, Get Respect!
or copy link
Cite according to academic standards
Simply copy and paste the text below into your bibliographic reference list, onto your blog, or anywhere else. You can also just hyperlink to this page.
New to UX Design? We’re Giving You a Free ebook!
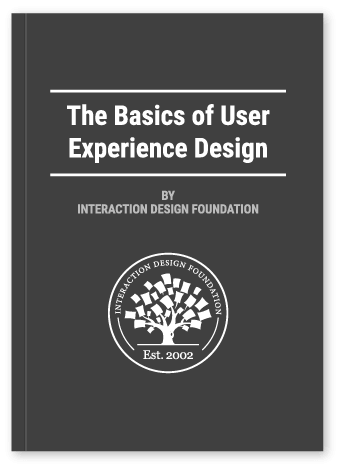
Download our free ebook The Basics of User Experience Design to learn about core concepts of UX design.
In 9 chapters, we’ll cover: conducting user interviews, design thinking, interaction design, mobile UX design, usability, UX research, and many more!

An official website of the United States government
The .gov means it’s official. Federal government websites often end in .gov or .mil. Before sharing sensitive information, make sure you’re on a federal government site.
The site is secure. The https:// ensures that you are connecting to the official website and that any information you provide is encrypted and transmitted securely.
- Publications
- Account settings
Preview improvements coming to the PMC website in October 2024. Learn More or Try it out now .
- Advanced Search
- Journal List
- Patterns (N Y)
- v.1(9); 2020 Dec 11

Principles of Effective Data Visualization
Stephen r. midway.
1 Department of Oceanography and Coastal Sciences, Louisiana State University, Baton Rouge, LA 70803, USA
We live in a contemporary society surrounded by visuals, which, along with software options and electronic distribution, has created an increased importance on effective scientific visuals. Unfortunately, across scientific disciplines, many figures incorrectly present information or, when not incorrect, still use suboptimal data visualization practices. Presented here are ten principles that serve as guidance for authors who seek to improve their visual message. Some principles are less technical, such as determining the message before starting the visual, while other principles are more technical, such as how different color combinations imply different information. Because figure making is often not formally taught and figure standards are not readily enforced in science, it is incumbent upon scientists to be aware of best practices in order to most effectively tell the story of their data.
The Bigger Picture
Visuals are an increasingly important form of science communication, yet many scientists are not well trained in design principles for effective messaging. Despite challenges, many visuals can be improved by taking some simple steps before, during, and after their creation. This article presents some sequential principles that are designed to improve visual messages created by scientists.
Many scientific visuals are not as effective as they could be because scientists often lack basic design principles. This article reviews the importance of effective data visualization and presents ten principles that scientists can use as guidance in developing effective visual messages.
Introduction
Visual learning is one of the primary forms of interpreting information, which has historically combined images such as charts and graphs (see Box 1 ) with reading text. 1 However, developments on learning styles have suggested splitting up the visual learning modality in order to recognize the distinction between text and images. 2 Technology has also enhanced visual presentation, in terms of the ability to quickly create complex visual information while also cheaply distributing it via digital means (compared with paper, ink, and physical distribution). Visual information has also increased in scientific literature. In addition to the fact that figures are commonplace in scientific publications, many journals now require graphical abstracts 3 or might tweet figures to advertise an article. Dating back to the 1970s when computer-generated graphics began, 4 papers represented by an image on the journal cover have been cited more frequently than papers without a cover image. 5
Regarding terminology, the terms graph , plot , chart , image , figure , and data visual(ization) are often used interchangeably, although they may have different meanings in different instances. Graph , plot , and chart often refer to the display of data, data summaries, and models, while image suggests a picture. Figure is a general term but is commonly used to refer to visual elements, such as plots, in a scientific work. A visual , or data visualization , is a newer and ostensibly more inclusive term to describe everything from figures to infographics. Here, I adopt common terminology, such as bar plot, while also attempting to use the terms figure and data visualization for general reference.
There are numerous advantages to quickly and effectively conveying scientific information; however, scientists often lack the design principles or technical skills to generate effective visuals. Going back several decades, Cleveland 6 found that 30% of graphs in the journal Science had at least one type of error. Several other studies have documented widespread errors or inefficiencies in scientific figures. 7 , 8 , 9 In fact, the increasing menu of visualization options can sometimes lead to poor fits between information and its presentation. These poor fits can even have the unintended consequence of confusing the readers and setting them back in their understanding of the material. While objective errors in graphs are hopefully in the minority of scientific works, what might be more common is suboptimal figure design, which takes place when a design element may not be objectively wrong but is ineffective to the point of limiting information transfer.
Effective figures suggest an understanding and interpretation of data; ineffective figures suggest the opposite. Although the field of data visualization has grown in recent years, the process of displaying information cannot—and perhaps should not—be fully mechanized. Much like statistical analyses often require expert opinions on top of best practices, figures also require choice despite well-documented recommendations. In other words, there may not be a singular best version of a given figure. Rather, there may be multiple effective versions of displaying a single piece of information, and it is the figure maker's job to weigh the advantages and disadvantages of each. Fortunately, there are numerous principles from which decisions can be made, and ultimately design is choice. 7
The data visualization literature includes many great resources. While several resources are targeted at developing design proficiency, such as the series of columns run by Nature Communications , 10 Wilkinson's The Grammar of Graphics 11 presents a unique technical interpretation of the structure of graphics. Wilkinson breaks down the notion of a graphic into its constituent parts—e.g., the data, scales, coordinates, geometries, aesthetics—much like conventional grammar breaks down a sentence into nouns, verbs, punctuation, and other elements of writing. The popularity and utility of this approach has been implemented in a number of software packages, including the popular ggplot2 package 12 currently available in R. 13 (Although the grammar of graphics approach is not explicitly adopted here, the term geometry is used consistently with Wilkinson to refer to different geometrical representations, whereas the term aesthetics is not used consistently with the grammar of graphics and is used simply to describe something that is visually appealing and effective.) By understanding basic visual design principles and their implementation, many figure authors may find new ways to emphasize and convey their information.
The Ten Principles
Principle #1 diagram first.
The first principle is perhaps the least technical but very important: before you make a visual, prioritize the information you want to share, envision it, and design it. Although this seems obvious, the larger point here is to focus on the information and message first, before you engage with software that in some way starts to limit or bias your visual tools. In other words, don't necessarily think of the geometries (dots, lines) you will eventually use, but think about the core information that needs to be conveyed and what about that information is going to make your point(s). Is your visual objective to show a comparison? A ranking? A composition? This step can be done mentally, or with a pen and paper for maximum freedom of thought. In parallel to this approach, it can be a good idea to save figures you come across in scientific literature that you identify as particularly effective. These are not just inspiration and evidence of what is possible, but will help you develop an eye for detail and technical skills that can be applied to your own figures.
Principle #2 Use the Right Software
Effective visuals typically require good command of one or more software. In other words, it might be unrealistic to expect complex, technical, and effective figures if you are using a simple spreadsheet program or some other software that is not designed to make complex, technical, and effective figures. Recognize that you might need to learn a new software—or expand your knowledge of a software you already know. While highly effective and aesthetically pleasing figures can be made quickly and simply, this may still represent a challenge to some. However, figure making is a method like anything else, and in order to do it, new methodologies may need to be learned. You would not expect to improve a field or lab method without changing something or learning something new. Data visualization is the same, with the added benefit that most software is readily available, inexpensive, or free, and many come with large online help resources. This article does not promote any specific software, and readers are encouraged to reference other work 14 for an overview of software resources.
Principle #3 Use an Effective Geometry and Show Data
Geometries are the shapes and features that are often synonymous with a type of figure; for example, the bar geometry creates a bar plot. While geometries might be the defining visual element of a figure, it can be tempting to jump directly from a dataset to pairing it with one of a small number of well-known geometries. Some of this thinking is likely to naturally happen. However, geometries are representations of the data in different forms, and often there may be more than one geometry to consider. Underlying all your decisions about geometries should be the data-ink ratio, 7 which is the ratio of ink used on data compared with overall ink used in a figure. High data-ink ratios are the best, and you might be surprised to find how much non-data-ink you use and how much of that can be removed.
Most geometries fall into categories: amounts (or comparisons), compositions (or proportions), distributions , or relationships . Although seemingly straightforward, one geometry may work in more than one category, in addition to the fact that one dataset may be visualized with more than one geometry (sometimes even in the same figure). Excellent resources exist on detailed approaches to selecting your geometry, 15 and this article only highlights some of the more common geometries and their applications.
Amounts or comparisons are often displayed with a bar plot ( Figure 1 A), although numerous other options exist, including Cleveland dot plots and even heatmaps ( Figure 1 F). Bar plots are among the most common geometry, along with lines, 9 although bar plots are noted for their very low data density 16 (i.e., low data-ink ratio). Geometries for amounts should only be used when the data do not have distributional information or uncertainty associated with them. A good use of a bar plot might be to show counts of something, while poor use of a bar plot might be to show group means. Numerous studies have discussed inappropriate uses of bar plots, 9 , 17 noting that “because the bars always start at zero, they can be misleading: for example, part of the range covered by the bar might have never been observed in the sample.” 17 Despite the numerous reports on incorrect usage, bar plots remain one of the most common problems in data visualization.

Examples of Visual Designs
(A) Clustered bar plots are effective at showing units within a group (A–C) when the data are amounts.
(B) Histograms are effective at showing the distribution of data, which in this case is a random draw of values from a Poisson distribution and which use a sequential color scheme that emphasizes the mean as red and values farther from the mean as yellow.
(C) Scatterplot where the black circles represent the data.
(D) Logistic regression where the blue line represents the fitted model, the gray shaded region represents the confidence interval for the fitted model, and the dark-gray dots represent the jittered data.
(E) Box plot showing (simulated) ages of respondents grouped by their answer to a question, with gray dots representing the raw data used in the box plot. The divergent colors emphasize the differences in values. For each box plot, the box represents the interquartile range (IQR), the thick black line represents the median value, and the whiskers extend to 1.5 times the IQR. Outliers are represented by the data.
(F) Heatmap of simulated visibility readings in four lakes over 5 months. The green colors represent lower visibility and the blue colors represent greater visibility. The white numbers in the cells are the average visibility measures (in meters).
(G) Density plot of simulated temperatures by season, where each season is presented as a small multiple within the larger figure.
For all figures the data were simulated, and any examples are fictitious.
Compositions or proportions may take a wide range of geometries. Although the traditional pie chart is one option, the pie geometry has fallen out of favor among some 18 due to the inherent difficulties in making visual comparisons. Although there may be some applications for a pie chart, stacked or clustered bar plots ( Figure 1 A), stacked density plots, mosaic plots, and treemaps offer alternatives.
Geometries for distributions are an often underused class of visuals that demonstrate high data density. The most common geometry for distributional information is the box plot 19 ( Figure 1 E), which shows five types of information in one object. Although more common in exploratory analyses than in final reports, the histogram ( Figure 1 B) is another robust geometry that can reveal information about data. Violin plots and density plots ( Figure 1 G) are other common distributional geometries, although many less-common options exist.
Relationships are the final category of visuals covered here, and they are often the workhorse of geometries because they include the popular scatterplot ( Figures 1 C and 1D) and other presentations of x - and y -coordinate data. The basic scatterplot remains very effective, and layering information by modifying point symbols, size, and color are good ways to highlight additional messages without taking away from the scatterplot. It is worth mentioning here that scatterplots often develop into line geometries ( Figure 1 D), and while this can be a good thing, presenting raw data and inferential statistical models are two different messages that need to be distinguished (see Data and Models Are Different Things ).
Finally, it is almost always recommended to show the data. 7 Even if a geometry might be the focus of the figure, data can usually be added and displayed in a way that does not detract from the geometry but instead provides the context for the geometry (e.g., Figures 1 D and 1E). The data are often at the core of the message, yet in figures the data are often ignored on account of their simplicity.
Principle #4 Colors Always Mean Something
The use of color in visualization can be incredibly powerful, and there is rarely a reason not to use color. Even if authors do not wish to pay for color figures in print, most journals still permit free color figures in digital formats. In a large study 20 of what makes visualizations memorable, colorful visualizations were reported as having a higher memorability score, and that seven or more colors are best. Although some of the visuals in this study were photographs, other studies 21 also document the effectiveness of colors.
In today's digital environment, color is cheap. This is overwhelmingly a good thing, but also comes with the risk of colors being applied without intention. Black-and-white visuals were more accepted decades ago when hard copies of papers were more common and color printing represented a large cost. Now, however, the vast majority of readers view scientific papers on an electronic screen where color is free. For those who still print documents, color printing can be done relatively cheaply in comparison with some years ago.
Color represents information, whether in a direct and obvious way, or in an indirect and subtle way. A direct example of using color may be in maps where water is blue and land is green or brown. However, the vast majority of (non-mapping) visualizations use color in one of three schemes: sequential , diverging , or qualitative . Sequential color schemes are those that range from light to dark typically in one or two (related) hues and are often applied to convey increasing values for increasing darkness ( Figures 1 B and 1F). Diverging color schemes are those that have two sequential schemes that represent two extremes, often with a white or neutral color in the middle ( Figure 1 E). A classic example of a diverging color scheme is the red to blue hues applied to jurisdictions in order to show voting preference in a two-party political system. Finally, qualitative color schemes are found when the intensity of the color is not of primary importance, but rather the objective is to use different and otherwise unrelated colors to convey qualitative group differences ( Figures 1 A and 1G).
While it is recommended to use color and capture the power that colors convey, there exist some technical recommendations. First, it is always recommended to design color figures that work effectively in both color and black-and-white formats ( Figures 1 B and 1F). In other words, whenever possible, use color that can be converted to an effective grayscale such that no information is lost in the conversion. Along with this approach, colors can be combined with symbols, line types, and other design elements to share the same information that the color was sharing. It is also good practice to use color schemes that are effective for colorblind readers ( Figures 1 A and 1E). Excellent resources, such as ColorBrewer, 22 exist to help in selecting color schemes based on colorblind criteria. Finally, color transparency is another powerful tool, much like a volume knob for color ( Figures 1 D and 1E). Not all colors have to be used at full value, and when not part of a sequential or diverging color scheme—and especially when a figure has more than one colored geometry—it can be very effective to increase the transparency such that the information of the color is retained but it is not visually overwhelming or outcompeting other design elements. Color will often be the first visual information a reader gets, and with this knowledge color should be strategically used to amplify your visual message.
Principle #5 Include Uncertainty
Not only is uncertainty an inherent part of understanding most systems, failure to include uncertainty in a visual can be misleading. There exist two primary challenges with including uncertainty in visuals: failure to include uncertainty and misrepresentation (or misinterpretation) of uncertainty.
Uncertainty is often not included in figures and, therefore, part of the statistical message is left out—possibly calling into question other parts of the statistical message, such as inference on the mean. Including uncertainty is typically easy in most software programs, and can take the form of common geometries such as error bars and shaded intervals (polygons), among other features. 15 Another way to approach visualizing uncertainty is whether it is included implicitly into the existing geometries, such as in a box plot ( Figure 1 E) or distribution ( Figures 1 B and 1G), or whether it is included explicitly as an additional geometry, such as an error bar or shaded region ( Figure 1 D).
Representing uncertainty is often a challenge. 23 Standard deviation, standard error, confidence intervals, and credible intervals are all common metrics of uncertainty, but each represents a different measure. Expressing uncertainty requires that readers be familiar with metrics of uncertainty and their interpretation; however, it is also the responsibility of the figure author to adopt the most appropriate measure of uncertainty. For instance, standard deviation is based on the spread of the data and therefore shares information about the entire population, including the range in which we might expect new values. On the other hand, standard error is a measure of the uncertainty in the mean (or some other estimate) and is strongly influenced by sample size—namely, standard error decreases with increasing sample size. Confidence intervals are primarily for displaying the reliability of a measurement. Credible intervals, almost exclusively associated with Bayesian methods, are typically built off distributions and have probabilistic interpretations.
Expressing uncertainty is important, but it is also important to interpret the correct message. Krzywinski and Altman 23 directly address a common misconception: “a gap between (error) bars does not ensure significance, nor does overlap rule it out—it depends on the type of bar.” This is a good reminder to be very clear not only in stating what type of uncertainty you are sharing, but what the interpretation is. Others 16 even go so far as to recommend that standard error not be used because it does not provide clear information about standard errors of differences among means. One recommendation to go along with expressing uncertainty is, if possible, to show the data (see Use an Effective Geometry and Show Data ). Particularly when the sample size is low, showing a reader where the data occur can help avoid misinterpretations of uncertainty.
Principle #6 Panel, when Possible (Small Multiples)
A particularly effective visual approach is to repeat a figure to highlight differences. This approach is often called small multiples , 7 and the technique may be referred to as paneling or faceting ( Figure 1 G). The strategy behind small multiples is that because many of the design elements are the same—for example, the axes, axes scales, and geometry are often the same—the differences in the data are easier to show. In other words, each panel represents a change in one variable, which is commonly a time step, a group, or some other factor. The objective of small multiples is to make the data inevitably comparable, 7 and effective small multiples always accomplish these comparisons.
Principle #7 Data and Models Are Different Things
Plotted information typically takes the form of raw data (e.g., scatterplot), summarized data (e.g., box plot), or an inferential statistic (e.g., fitted regression line; Figure 1 D). Raw data and summarized data are often relatively straightforward; however, a plotted model may require more explanation for a reader to be able to fully reproduce the work. Certainly any model in a study should be reported in a complete way that ensures reproducibility. However, any visual of a model should be explained in the figure caption or referenced elsewhere in the document so that a reader can find the complete details on what the model visual is representing. Although it happens, it is not acceptable practice to show a fitted model or other model results in a figure if the reader cannot backtrack the model details. Simply because a model geometry can be added to a figure does not mean that it should be.
Principle #8 Simple Visuals, Detailed Captions
As important as it is to use high data-ink ratios, it is equally important to have detailed captions that fully explain everything in the figure. A study of figures in the Journal of American Medicine 8 found that more than one-third of graphs were not self-explanatory. Captions should be standalone, which means that if the figure and caption were looked at independent from the rest of the study, the major point(s) could still be understood. Obviously not all figures can be completely standalone, as some statistical models and other procedures require more than a caption as explanation. However, the principle remains that captions should do all they can to explain the visualization and representations used. Captions should explain any geometries used; for instance, even in a simple scatterplot it should be stated that the black dots represent the data ( Figures 1 C–1E). Box plots also require descriptions of their geometry—it might be assumed what the features of a box plot are, yet not all box plot symbols are universal.
Principle #9 Consider an Infographic
It is unclear where a figure ends and an infographic begins; however, it is fair to say that figures tend to be focused on representing data and models, whereas infographics typically incorporate text, images, and other diagrammatic elements. Although it is not recommended to convert all figures to infographics, infographics were found 20 to have the highest memorability score and that diagrams outperformed points, bars, lines, and tables in terms of memorability. Scientists might improve their overall information transfer if they consider an infographic where blending different pieces of information could be effective. Also, an infographic of a study might be more effective outside of a peer-reviewed publication and in an oral or poster presentation where a visual needs to include more elements of the study but with less technical information.
Even if infographics are not adopted in most cases, technical visuals often still benefit from some text or other annotations. 16 Tufte's works 7 , 24 provide great examples of bringing together textual, visual, and quantitative information into effective visualizations. However, as figures move in the direction of infographics, it remains important to keep chart junk and other non-essential visual elements out of the design.
Principle #10 Get an Opinion
Although there may be principles and theories about effective data visualization, the reality is that the most effective visuals are the ones with which readers connect. Therefore, figure authors are encouraged to seek external reviews of their figures. So often when writing a study, the figures are quickly made, and even if thoughtfully made they are not subject to objective, outside review. Having one or more colleagues or people external to the study review figures will often provide useful feedback on what readers perceive, and therefore what is effective or ineffective in a visual. It is also recommended to have outside colleagues review only the figures. Not only might this please your colleague reviewers (because figure reviews require substantially less time than full document reviews), but it also allows them to provide feedback purely on the figures as they will not have the document text to fill in any uncertainties left by the visuals.
What About Tables?
Although often not included as data visualization, tables can be a powerful and effective way to show data. Like other visuals, tables are a type of hybrid visual—they typically only include alphanumeric information and no geometries (or other visual elements), so they are not classically a visual. However, tables are also not text in the same way a paragraph or description is text. Rather, tables are often summarized values or information, and are effective if the goal is to reference exact numbers. However, the interest in numerical results in the form of a study typically lies in comparisons and not absolute numbers. Gelman et al. 25 suggested that well-designed graphs were superior to tables. Similarly, Spence and Lewandowsky 26 compared pie charts, bar graphs, and tables and found a clear advantage for graphical displays over tabulations. Because tables are best suited for looking up specific information while graphs are better for perceiving trends and making comparisons and predictions, it is recommended that visuals are used before tables. Despite the reluctance to recommend tables, tables may benefit from digital formats. In other words, while tables may be less effective than figures in many cases, this does not mean tables are ineffective or do not share specific information that cannot always be displayed in a visual. Therefore, it is recommended to consider creating tables as supplementary or appendix information that does not go into the main document (alongside the figures), but which is still very easily accessed electronically for those interested in numerical specifics.
Conclusions
While many of the elements of peer-reviewed literature have remained constant over time, some elements are changing. For example, most articles now have more authors than in previous decades, and a much larger menu of journals creates a diversity of article lengths and other requirements. Despite these changes, the demand for visual representations of data and results remains high, as exemplified by graphical abstracts, overview figures, and infographics. Similarly, we now operate with more software than ever before, creating many choices and opportunities to customize scientific visualizations. However, as the demand for, and software to create, visualizations have both increased, there is not always adequate training among scientists and authors in terms of optimizing the visual for the message.
Figures are not just a scientific side dish but can be a critical point along the scientific process—a point at which the figure maker demonstrates their knowledge and communication of the data and results, and often one of the first stopping points for new readers of the information. The reality for the vast majority of figures is that you need to make your point in a few seconds. The longer someone looks at a figure and doesn't understand the message, the more likely they are to gain nothing from the figure and possibly even lose some understanding of your larger work. Following a set of guidelines and recommendations—summarized here and building on others—can help to build robust visuals that avoid many common pitfalls of ineffective figures ( Figure 2 ).

Overview of the Principles Presented in This Article
The two principles in yellow (bottom) are those that occur first, during the figure design phase. The six principles in green (middle) are generally considerations and decisions while making a figure. The two principles in blue (top) are final steps often considered after a figure has been drafted. While the general flow of the principles follows from bottom to top, there is no specific or required order, and the development of individual figures may require more or less consideration of different principles in a unique order.
All scientists seek to share their message as effectively as possible, and a better understanding of figure design and representation is undoubtedly a step toward better information dissemination and fewer errors in interpretation. Right now, much of the responsibility for effective figures lies with the authors, and learning best practices from literature, workshops, and other resources should be undertaken. Along with authors, journals play a gatekeeper role in figure quality. Journal editorial teams are in a position to adopt recommendations for more effective figures (and reject ineffective figures) and then translate those recommendations into submission requirements. However, due to the qualitative nature of design elements, it is difficult to imagine strict visual guidelines being enforced across scientific sectors. In the absence of such guidelines and with seemingly endless design choices available to figure authors, it remains important that a set of aesthetic criteria emerge to guide the efficient conveyance of visual information.
Acknowledgments
Thanks go to the numerous students with whom I have had fun, creative, and productive conversations about displaying information. Danielle DiIullo was extremely helpful in technical advice on software. Finally, Ron McKernan provided guidance on several principles.
Author Contributions
S.R.M. conceived the review topic, conducted the review, developed the principles, and wrote the manuscript.
Steve Midway is an assistant professor in the Department of Oceanography and Coastal Sciences at Louisiana State University. His work broadly lies in fisheries ecology and how sound science can be applied to management and conservation issues. He teaches a number of quantitative courses in ecology, all of which include data visualization.
- School Guide
- Mathematics
- Number System and Arithmetic
- Trigonometry
- Probability
- Mensuration
- Maths Formulas
- Class 8 Maths Notes
- Class 9 Maths Notes
- Class 10 Maths Notes
- Class 11 Maths Notes
- Class 12 Maths Notes
Graphical Representation of Data
Graphical Representation of Data: Graphical Representation of Data,” where numbers and facts become lively pictures and colorful diagrams . Instead of staring at boring lists of numbers, we use fun charts, cool graphs, and interesting visuals to understand information better. In this exciting concept of data visualization, we’ll learn about different kinds of graphs, charts, and pictures that help us see patterns and stories hidden in data.
There is an entire branch in mathematics dedicated to dealing with collecting, analyzing, interpreting, and presenting numerical data in visual form in such a way that it becomes easy to understand and the data becomes easy to compare as well, the branch is known as Statistics .
The branch is widely spread and has a plethora of real-life applications such as Business Analytics, demography, Astro statistics, and so on . In this article, we have provided everything about the graphical representation of data, including its types, rules, advantages, etc.
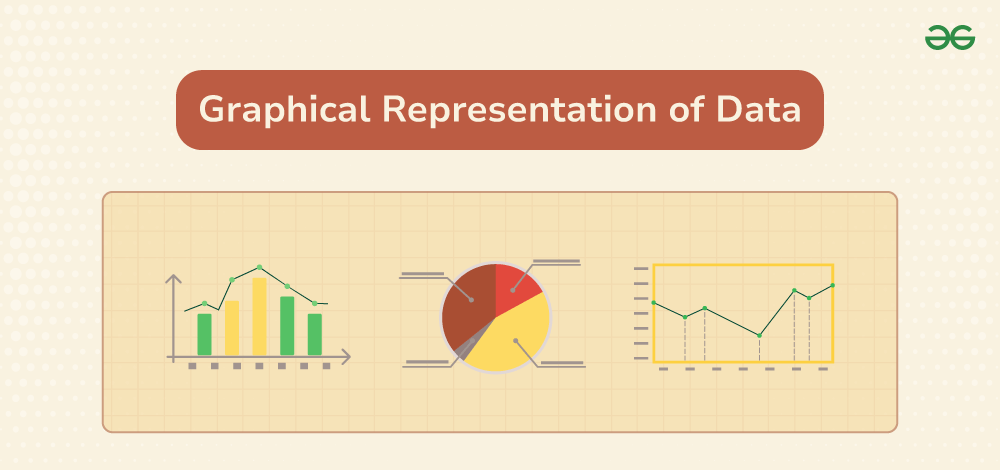
Table of Content
What is Graphical Representation
Types of graphical representations, line graphs, histograms , stem and leaf plot , box and whisker plot .
- Graphical Representations used in Maths
Value-Based or Time Series Graphs
Frequency based, principles of graphical representations, advantages and disadvantages of using graphical system, general rules for graphical representation of data, frequency polygon, solved examples on graphical representation of data.
Graphics Representation is a way of representing any data in picturized form . It helps a reader to understand the large set of data very easily as it gives us various data patterns in visualized form.
There are two ways of representing data,
- Pictorial Representation through graphs.
They say, “A picture is worth a thousand words”. It’s always better to represent data in a graphical format. Even in Practical Evidence and Surveys, scientists have found that the restoration and understanding of any information is better when it is available in the form of visuals as Human beings process data better in visual form than any other form.
Does it increase the ability 2 times or 3 times? The answer is it increases the Power of understanding 60,000 times for a normal Human being, the fact is amusing and true at the same time.
Check: Graph and its representations
Comparison between different items is best shown with graphs, it becomes easier to compare the crux of the data about different items. Let’s look at all the different types of graphical representations briefly:
A line graph is used to show how the value of a particular variable changes with time. We plot this graph by connecting the points at different values of the variable. It can be useful for analyzing the trends in the data and predicting further trends.
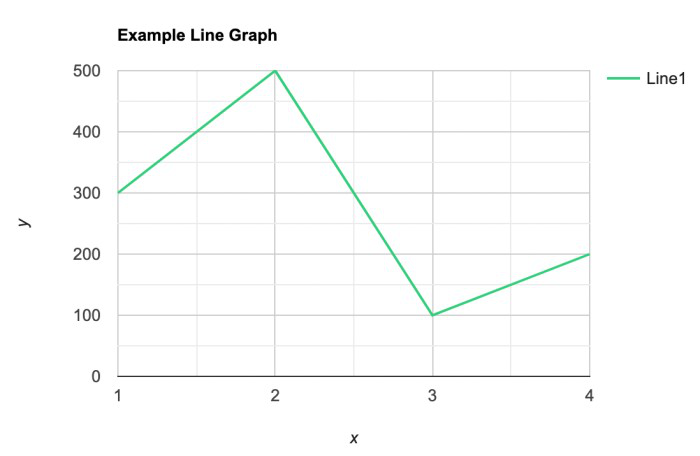
A bar graph is a type of graphical representation of the data in which bars of uniform width are drawn with equal spacing between them on one axis (x-axis usually), depicting the variable. The values of the variables are represented by the height of the bars.
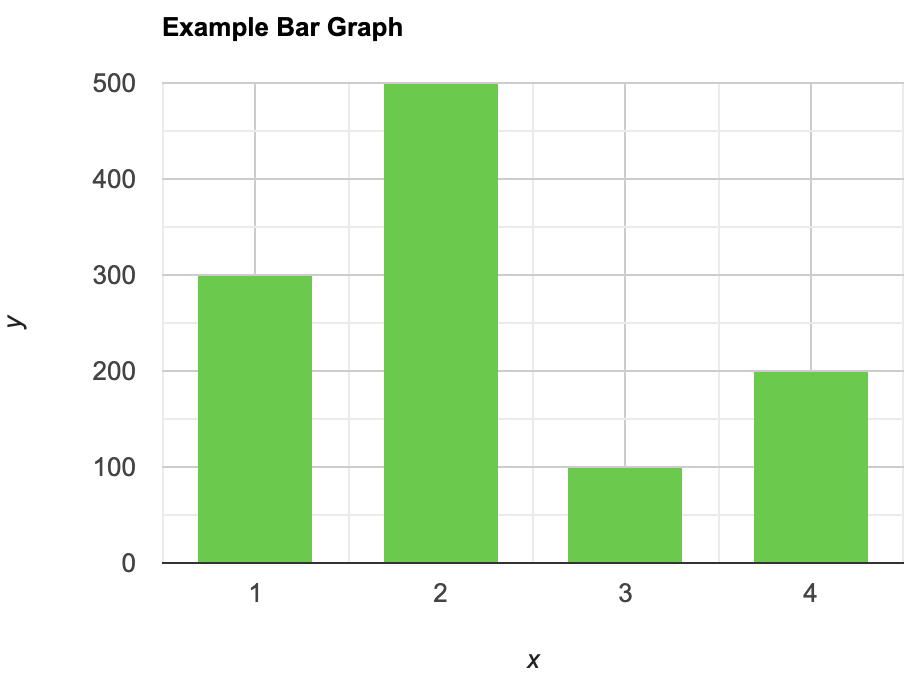
This is similar to bar graphs, but it is based frequency of numerical values rather than their actual values. The data is organized into intervals and the bars represent the frequency of the values in that range. That is, it counts how many values of the data lie in a particular range.
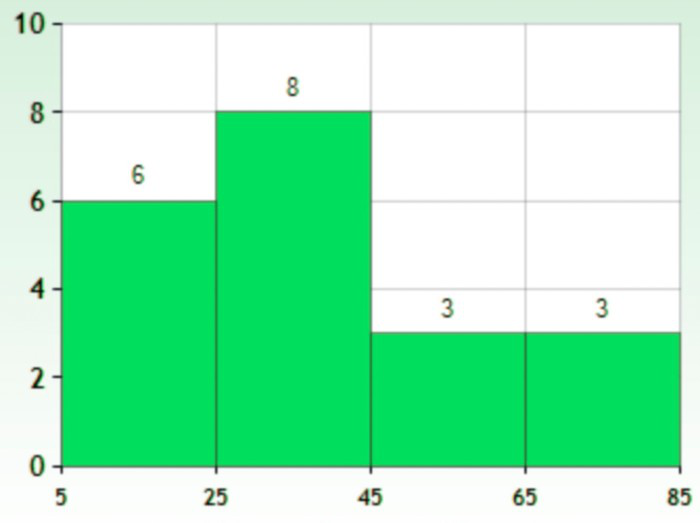
It is a plot that displays data as points and checkmarks above a number line, showing the frequency of the point.
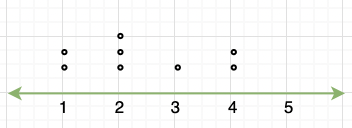
This is a type of plot in which each value is split into a “leaf”(in most cases, it is the last digit) and “stem”(the other remaining digits). For example: the number 42 is split into leaf (2) and stem (4).
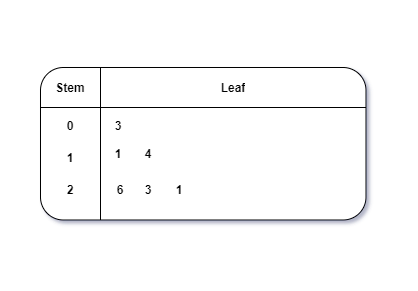
These plots divide the data into four parts to show their summary. They are more concerned about the spread, average, and median of the data.
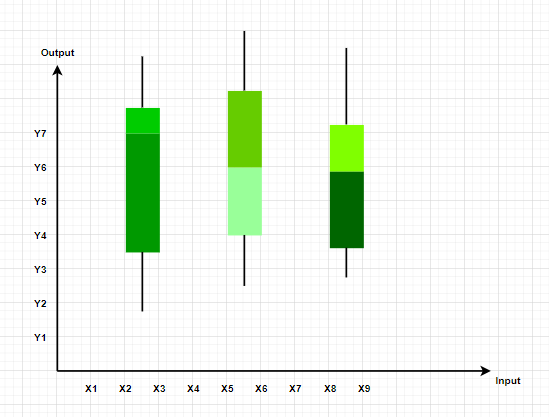
It is a type of graph which represents the data in form of a circular graph. The circle is divided such that each portion represents a proportion of the whole.
Graphical Representations used in Math’s
Graphs in Math are used to study the relationships between two or more variables that are changing. Statistical data can be summarized in a better way using graphs. There are basically two lines of thoughts of making graphs in maths:
- Value-Based or Time Series Graphs
These graphs allow us to study the change of a variable with respect to another variable within a given interval of time. The variables can be anything. Time Series graphs study the change of variable with time. They study the trends, periodic behavior, and patterns in the series. We are more concerned with the values of the variables here rather than the frequency of those values.
Example: Line Graph
These kinds of graphs are more concerned with the distribution of data. How many values lie between a particular range of the variables, and which range has the maximum frequency of the values. They are used to judge a spread and average and sometimes median of a variable under study.
Also read: Types of Statistical Data
- All types of graphical representations follow algebraic principles.
- When plotting a graph, there’s an origin and two axes.
- The x-axis is horizontal, and the y-axis is vertical.
- The axes divide the plane into four quadrants.
- The origin is where the axes intersect.
- Positive x-values are to the right of the origin; negative x-values are to the left.
- Positive y-values are above the x-axis; negative y-values are below.
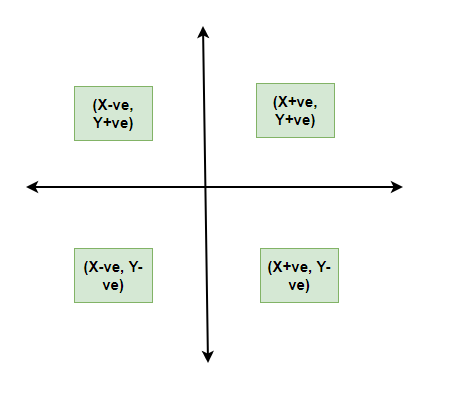
- It gives us a summary of the data which is easier to look at and analyze.
- It saves time.
- We can compare and study more than one variable at a time.
Disadvantages
- It usually takes only one aspect of the data and ignores the other. For example, A bar graph does not represent the mean, median, and other statistics of the data.
- Interpretation of graphs can vary based on individual perspectives, leading to subjective conclusions.
- Poorly constructed or misleading visuals can distort data interpretation and lead to incorrect conclusions.
Check : Diagrammatic and Graphic Presentation of Data
We should keep in mind some things while plotting and designing these graphs. The goal should be a better and clear picture of the data. Following things should be kept in mind while plotting the above graphs:
- Whenever possible, the data source must be mentioned for the viewer.
- Always choose the proper colors and font sizes. They should be chosen to keep in mind that the graphs should look neat.
- The measurement Unit should be mentioned in the top right corner of the graph.
- The proper scale should be chosen while making the graph, it should be chosen such that the graph looks accurate.
- Last but not the least, a suitable title should be chosen.
A frequency polygon is a graph that is constructed by joining the midpoint of the intervals. The height of the interval or the bin represents the frequency of the values that lie in that interval.
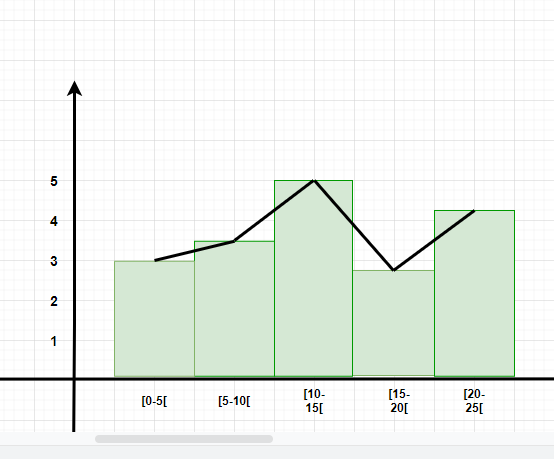
Question 1: What are different types of frequency-based plots?
Types of frequency-based plots: Histogram Frequency Polygon Box Plots
Question 2: A company with an advertising budget of Rs 10,00,00,000 has planned the following expenditure in the different advertising channels such as TV Advertisement, Radio, Facebook, Instagram, and Printed media. The table represents the money spent on different channels.
Draw a bar graph for the following data.
- Put each of the channels on the x-axis
- The height of the bars is decided by the value of each channel.
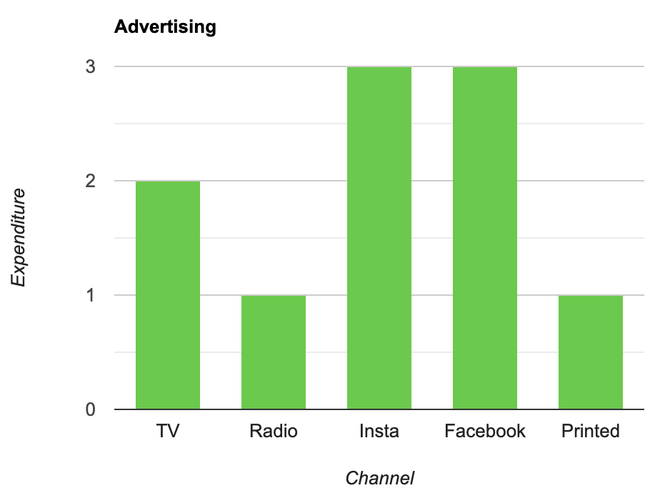
Question 3: Draw a line plot for the following data
- Put each of the x-axis row value on the x-axis
- joint the value corresponding to the each value of the x-axis.
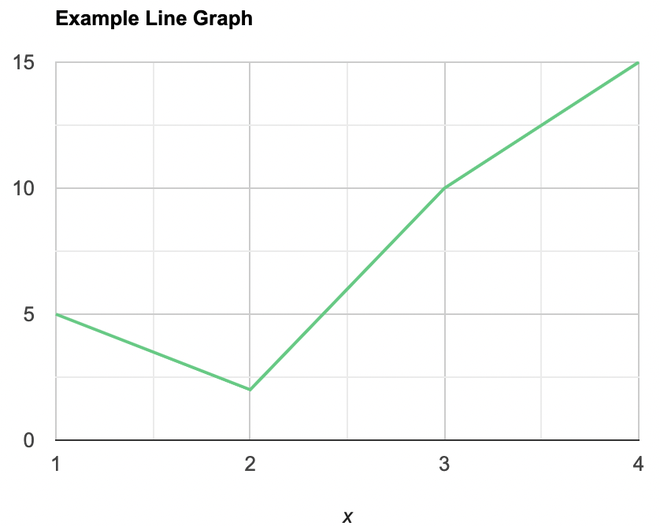
Question 4: Make a frequency plot of the following data:
- Draw the class intervals on the x-axis and frequencies on the y-axis.
- Calculate the midpoint of each class interval.
Class Interval | Mid Point | Frequency |
0-3 | 1.5 | 3 |
3-6 | 4.5 | 4 |
6-9 | 7.5 | 2 |
9-12 | 10.5 | 6 |
Now join the mid points of the intervals and their corresponding frequencies on the graph.
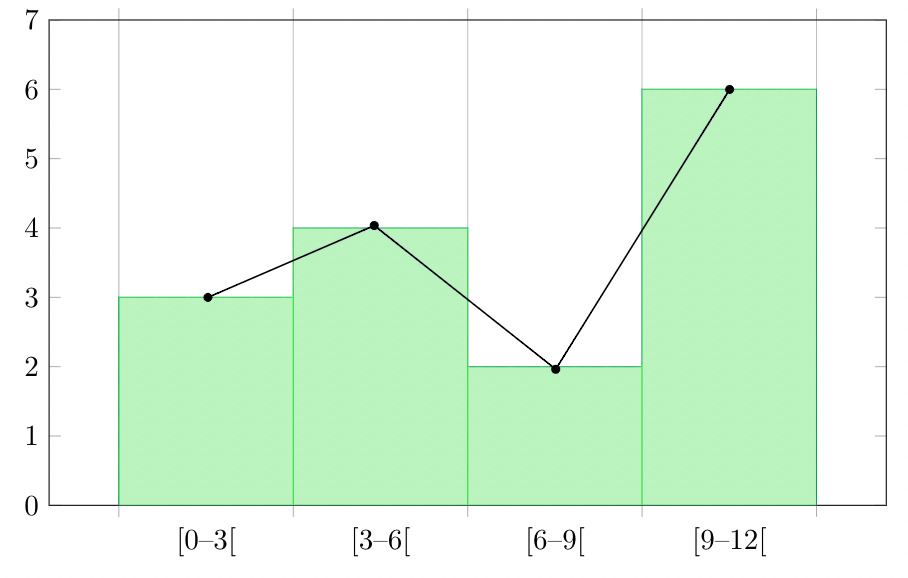
This graph shows both the histogram and frequency polygon for the given distribution.
Related Article:
Graphical Representation of Data| Practical Work in Geography Class 12 What are the different ways of Data Representation What are the different ways of Data Representation? Charts and Graphs for Data Visualization
Conclusion of Graphical Representation
Graphical representation is a powerful tool for understanding data, but it’s essential to be aware of its limitations. While graphs and charts can make information easier to grasp, they can also be subjective, complex, and potentially misleading . By using graphical representations wisely and critically, we can extract valuable insights from data, empowering us to make informed decisions with confidence.
Graphical Representation of Data – FAQs
What are the advantages of using graphs to represent data.
Graphs offer visualization, clarity, and easy comparison of data, aiding in outlier identification and predictive analysis.
What are the common types of graphs used for data representation?
Common graph types include bar, line, pie, histogram, and scatter plots , each suited for different data representations and analysis purposes.
How do you choose the most appropriate type of graph for your data?
Select a graph type based on data type, analysis objective, and audience familiarity to effectively convey information and insights.
How do you create effective labels and titles for graphs?
Use descriptive titles, clear axis labels with units, and legends to ensure the graph communicates information clearly and concisely.
How do you interpret graphs to extract meaningful insights from data?
Interpret graphs by examining trends, identifying outliers, comparing data across categories, and considering the broader context to draw meaningful insights and conclusions.
Please Login to comment...
Similar reads.
- School Learning
- Maths-Class-9
- How to Get a Free SSL Certificate
- Best SSL Certificates Provider in India
- Elon Musk's xAI releases Grok-2 AI assistant
- What is OpenAI SearchGPT? How it works and How to Get it?
- Content Improvement League 2024: From Good To A Great Article
Improve your Coding Skills with Practice
What kind of Experience do you want to share?
- Documentation
Data Visualization Essentials: Tips, Techniques, and Tools
Written by Tom Czaban | May 17, 2023
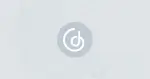
Table of Contents
What Is Data Visualization?
Why is data visualization important, types of data visualization, out-of-the-box visualizations, fully-customized visualizations, what is real-time data visualization, what is interactive data visualization, advantages and disadvantages of data visualization, what tools do i need for data visualization, examples of data visualization, data visualization best practices, ready to get started with data visualization, discover more about data visualization.
Data visualization is the use of visual representations to display information. This definition might sound modern, but data visualization has been around for centuries. One of the earliest and most obvious examples is maps, which developed out of the need to graphically display geographic data. Since then data visualization has continued to develop to meet the needs of today’s users.
There are multiple ways to visualize data (including charts, graphs, and infographics), and technology is constantly evolving to present information in more eye-catching and useful ways. Examples of this include making visualizations interactive and allowing the end user to filter and display different metrics. Regardless of these updates, the aim remains the same: to present key insights and make it easier to engage with and understand data.
Any discussion of the meaning of data visualization would be incomplete without a mention of creating dashboards . It is important to note that although data visualization and dashboards are closely related they are not in fact the same thing. The aim of a dashboard is to offer an overview of the key performance indicators (KPIs) of the presented area. Typically a dashboard will contain multiple visualizations, which together provide an overview of the key insights — as shown in the image below.
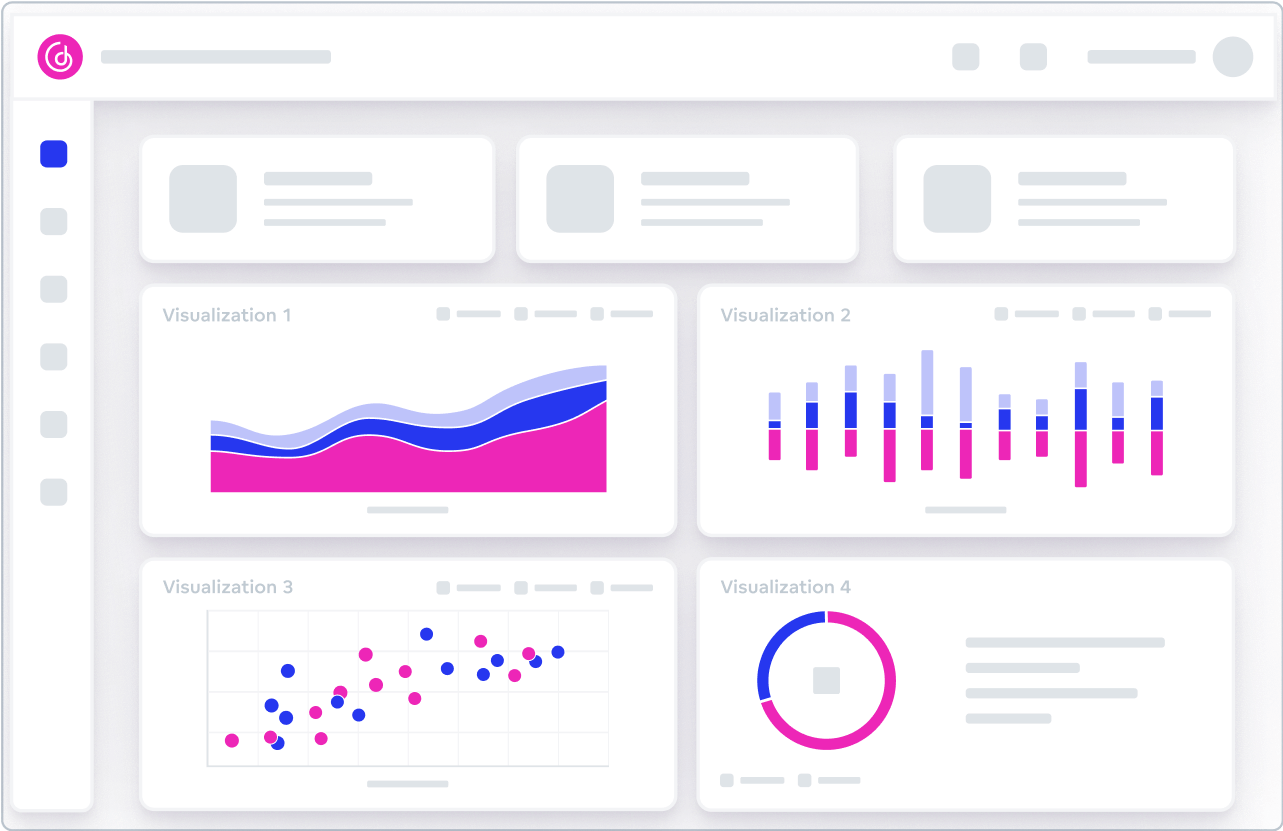
Data visualization helps to ensure data insights aren’t lost in delivery; most of us can’t process big blocks of statistics, our brains aren’t built like that. Anyone who has looked at a long list of numbers will understand the disconnect this can cause. Graphical representation solves this pain point by making statistics and data easier to absorb.
Data visualization is not only about creating simple and attractive visuals. It can be used to create insights by identifying patterns and trends that would otherwise be difficult to spot. Displaying a set of data on a scatter plot , for example, might reveal connections between outliers that previously went unnoticed when the statistics were in a table.
Data visualization is also an important business intelligence (BI) tool, allowing companies to effectively communicate their data and improve decision-making. High-quality visualizations can help an organization promote a data culture because the insights needn’t be explained to non-technical end users. Decisions can be made with increased accuracy and speed because everyone is on the same page. As well as improving internal processes, visualizations help to increase external engagement by making the data more accessible to partners and customers.
There are different types of visualizations to choose from, and each is better suited to showcasing certain attributes and metrics. It is important to use the visualization that makes the most sense for the insights you aim to convey. Below is a brief overview of the visualizations you might choose for some typical scenarios. For more on this, check out our post on how to choose the best chart type to visualize your data .
There are instances when you may need to display one key figure , for example, the number of customers, or the number of returned items. A KPI visualization is best suited to this purpose because it shows one big number. However, this number will mean nothing on its own; you have to, at the very least, provide a date range and compare it with another metric to give it some context.
To show comparisons between categories , for example, the number of sales each staff member has made in the last month, it is best to use a bar chart or column chart . A stacked bar chart gives you the option to add another category, so as well as showing how many sales each staff member has made, you might also include the product type they sold by adding color and a key.
When comparing parts to the whole it is best to use a pie chart , donut chart , or treemap . An example of part-to-whole comparison is the number of people who answered ‘yes’ or ‘no’ to a specific question. Generally speaking, it is a bad idea to use a pie chart or donut chart for more than three categories because it becomes difficult for users to accurately absorb the data. With more categories, it is better to use a treemap.
To show changes over time the most effective options are line charts , area charts, or column charts. You might, for instance, choose one of these to display month-by-month revenue. If you want to add an additional category (such as product type) you can use a line chart with multiple lines or a stacked area chart. But it's best to tread carefully with these because they can become confusing if not properly executed.
To show the details of many items it is best to use a table. Some people avoid using tables because they seem too basic, but when you have many items (such as a lot of customer details) a table can be the right choice. Amid the myriad of visualization options available, tables can be quite striking when combined with other types of charts and graphs on a dashboard.
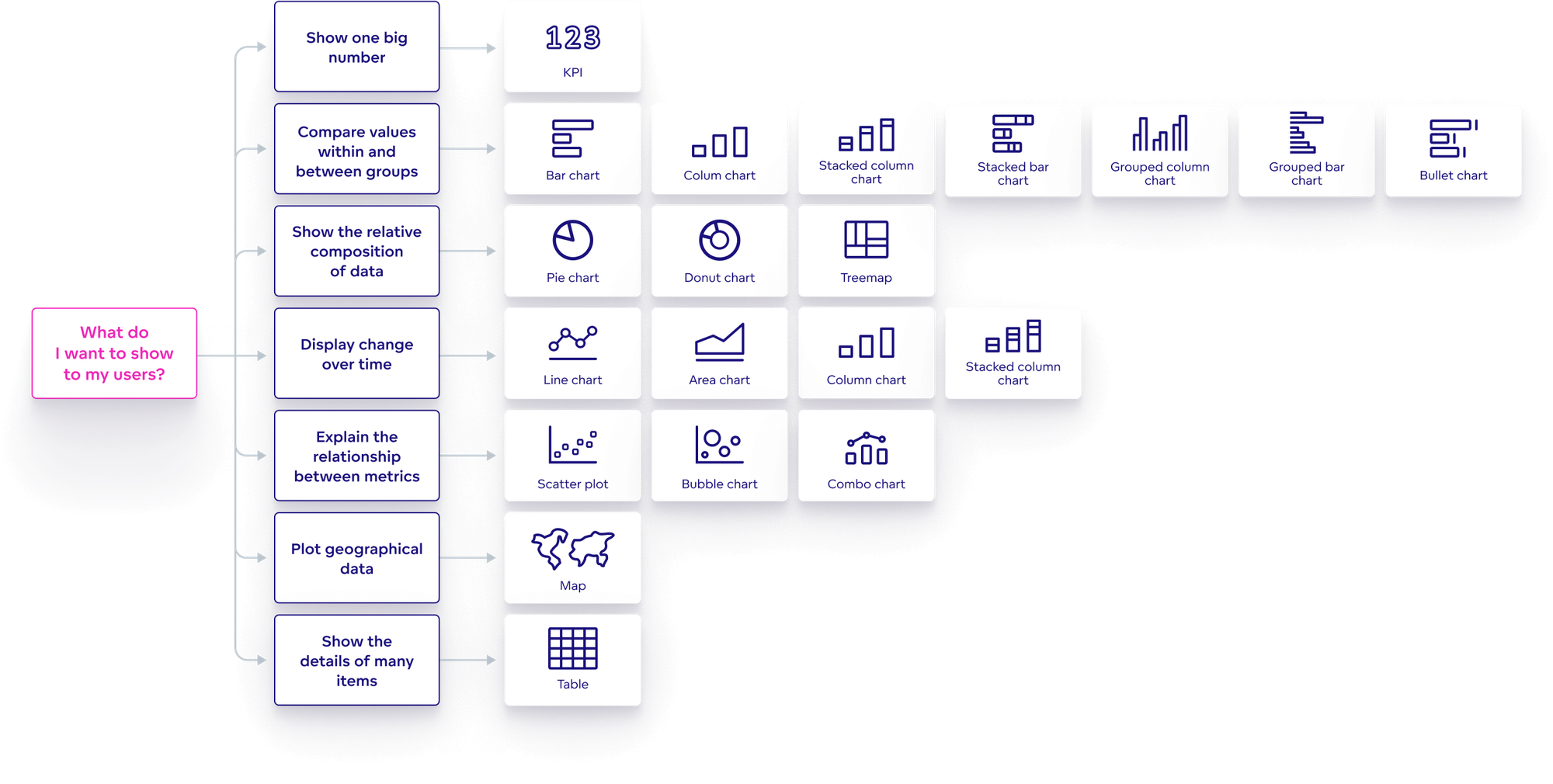
Most analytics platforms offer out-of-the-box visualizations that you can use to display your data. Out-of-the-box refers to the standard visualizations available to all customers who have purchased the BI tool. These options typically include tables, column charts, bar charts, line charts, donut charts, etc. Not all out-of-the-box offerings are created equal, so when choosing a BI tool, it is helpful if it has at least some of the following capabilities:
- An interface that makes it easy to change metrics, dimensions, or data visualization types from the out-of-the-box options, such as drag-and-drop.
- The ability to add filters and drill into visualizations.
- The option to adjust certain aspects to match your brand, for example, fonts, colors, and logos.
- The potential to populate visualizations with live data.
While you can do plenty with out-of-the-box visualizations, your options are limited to the standard charts and graphs that come with the tool. For this reason, you’re often better off with a solution that allows you more creative options. With custom visualization, you can tailor your visualizations to your exact needs. You are no longer limited to standard chart types, and the only barrier to creating highly original visuals is your imagination.
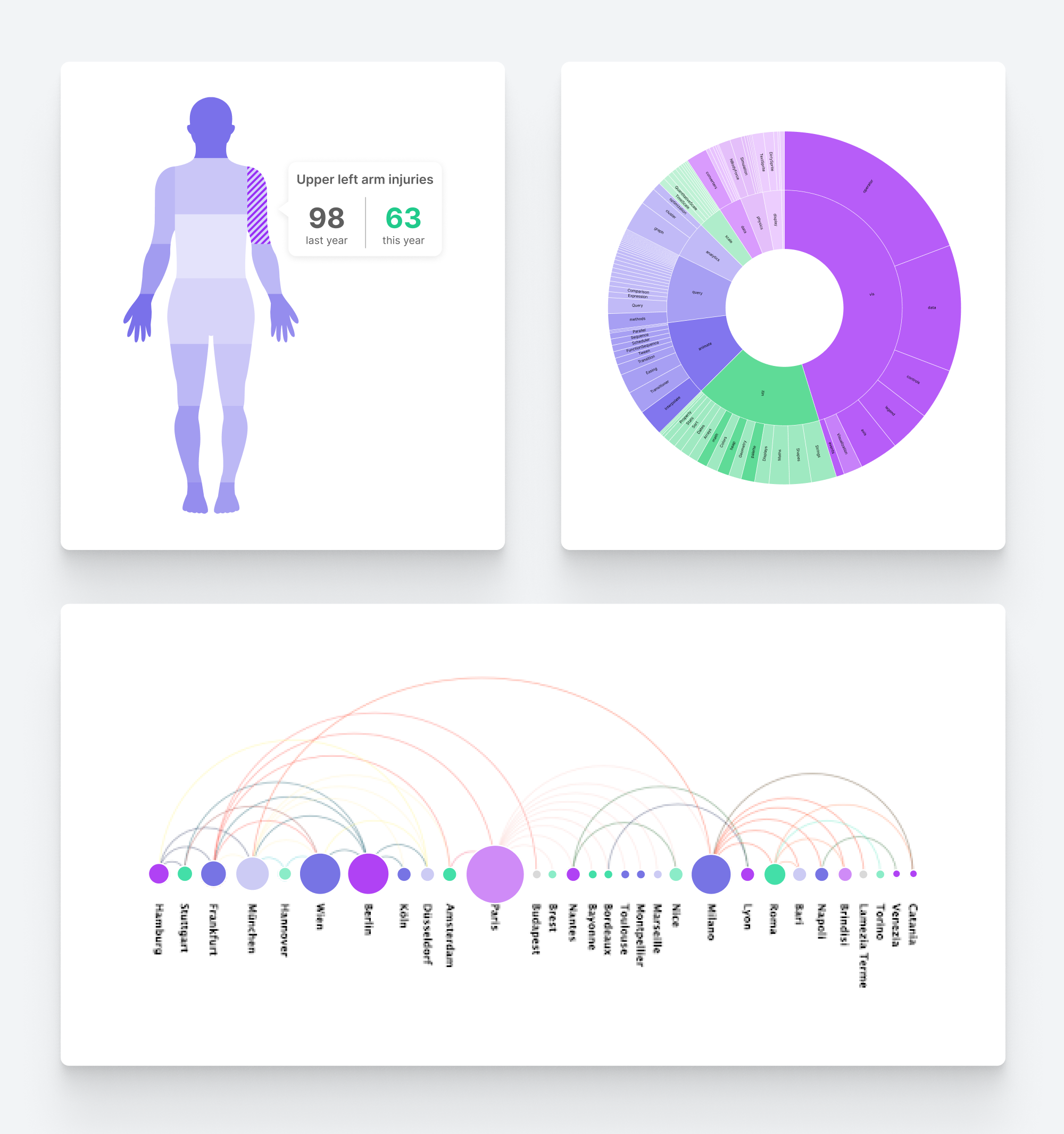
A good analytics platform achieves custom visualization by enabling access to third-party charting libraries, such as D3.js, Chart.js, Fusion Charts, Google Charts, and more. These libraries are the best route to creating advanced visuals that are graphically amazing.
Want to see what GoodData can do for you?
Get a guided tour and ask us about GoodData’s features, implementation, and pricing.
With real-time data visualization, users can see the data changing as it is being updated or generated. For example, they might see the height of bar charts changing, or colors adjusting themselves on a heatmap.
To create this kind of visualization, a company needs the ability to perform real-time data reporting. In other words, their data architecture must operate in real-time to build up-to-the-minute visuals. This architecture includes components such as data processing, data streaming, and all the logic of the defined analysis that leads to the displayed insights.
Real-time analytics and their visualization can be crucial under certain circumstances. On other occasions, this can be unnecessary and even confusing. Before deciding whether to employ automated real-time visualization, a company might ask themselves the following questions:
- Do we really need to see in-the-moment data to make decisions?
- Do we have enough new data at frequent intervals to necessitate real-time updates?
- Will these instant updates make our decision-making processes more confusing and create delays?
- Will real-time visualization help our users, i.e., what value will they get from the live updates?
Based on the above questions, scenarios where real-time visualizations may be beneficial include:
- Security and fraud prevention, e.g., when monitoring for major security breaches.
- A situation where a company needs to act promptly to a crisis.
- Goal monitoring (but only if these goals are affected by rapidly changing information)
- In financial teams – where it is crucial for team members to receive up-to-the-minute financial information (for example, stock markets).
Below is an example of real-time visualization in action:
On the surface, interactive data visualization tends to look similar to regular (or static) visualization. The difference is there is an option to click a button or move a slider, so the user can interact with the data, rather than just look at it. This ability to manipulate charts, graphs, and maps can positively influence user experience (UX).
Sometimes interactive features are custom-designed for a specific purpose, but generally speaking, the most common interactive features are:
- Filtering: Allows you to filter for the exact information required, highlighting the relevant data and reducing the data that is currently unimportant.
- Drilling: Enables you to move between different visualizations and send an action from the dashboard.
- Zooming and panning: Creates the possibility to hone in on a particular detail; you can zoom in on a specific part of the visualization and pan across it.
Interactive features can be extremely useful, for example, when users quickly need to answer specific queries, which is why they’re often used in BI reports. However, there are also times when a static visualization might be the best choice; for instance, when visualizations need to be printed and shared as reports, or when it is unnecessary for users to manipulate the information and all they need to do is to look at it. For more on this, check out our article that compares interactive and non-interactive visualizations using the example of world happiness levels.
While there are huge benefits to visualizing data, if not done properly the technique can spread misinformation. Below we look at some of the main advantages of data visualization and how to mitigate its downsides.
Benefits of data visualization
- Easy to spot trends. Visualization allows users to see patterns in the data they might otherwise have missed.
- Simple sharing of information. It is far easier to share data with charts, graphs, and infographics.
- Makes data accessible to non-technical users. With visualization, you no longer need to be a mathematician to understand the data insights.
- Easy to remember. Charts and graphs are not only easier to digest; they also tend to stay in the memory more easily than lists of numbers and statistics.
- Increase revenue. When all the decision-makers have the information at their fingertips, it empowers management to make quick and accurate decisions.
Problems with data visualization
- Information still needs to be accurate. Great visualizations [/blog/8-ways-turn-good-data-great-visualizations/] don’t make up for bad data. If best practices are not followed then visualizations can fall into the trap of becoming style over substance.
- Data visualization is an investment. Companies that want to effectively organize and visualize their data, or provide this ability to their customers, will either need a lot of involvement from analytics engineers (if they have the resources)), or an integrated analytics solution. Neither of these options comes without its costs, and pricing can vary depending on requirements. This then raises the question of whether to build the analytics solution in-house or buy off the shelf .
- Correlation does not equal causation. Visualizations often show the correlation between two or more metrics, so users often assume causation. But just because there is a correlation it doesn’t necessarily mean that one is caused by the other. There may be several other factors at play that aren’t included in the visualization.
- Users can still misinterpret the information. While visualization makes it easier for users to absorb data, it is still open to misinterpretation. For example, users might focus on the wrong thing when viewing it. This once again highlights the importance of using the right visualization type for the data displayed and the desired outcome.
- Confusing visualizations. Visualizations are supposed to simplify data, but if done badly they can make matters even more complicated. Perhaps the wrong chart type has been chosen, or there is too much information — as in the picture below.
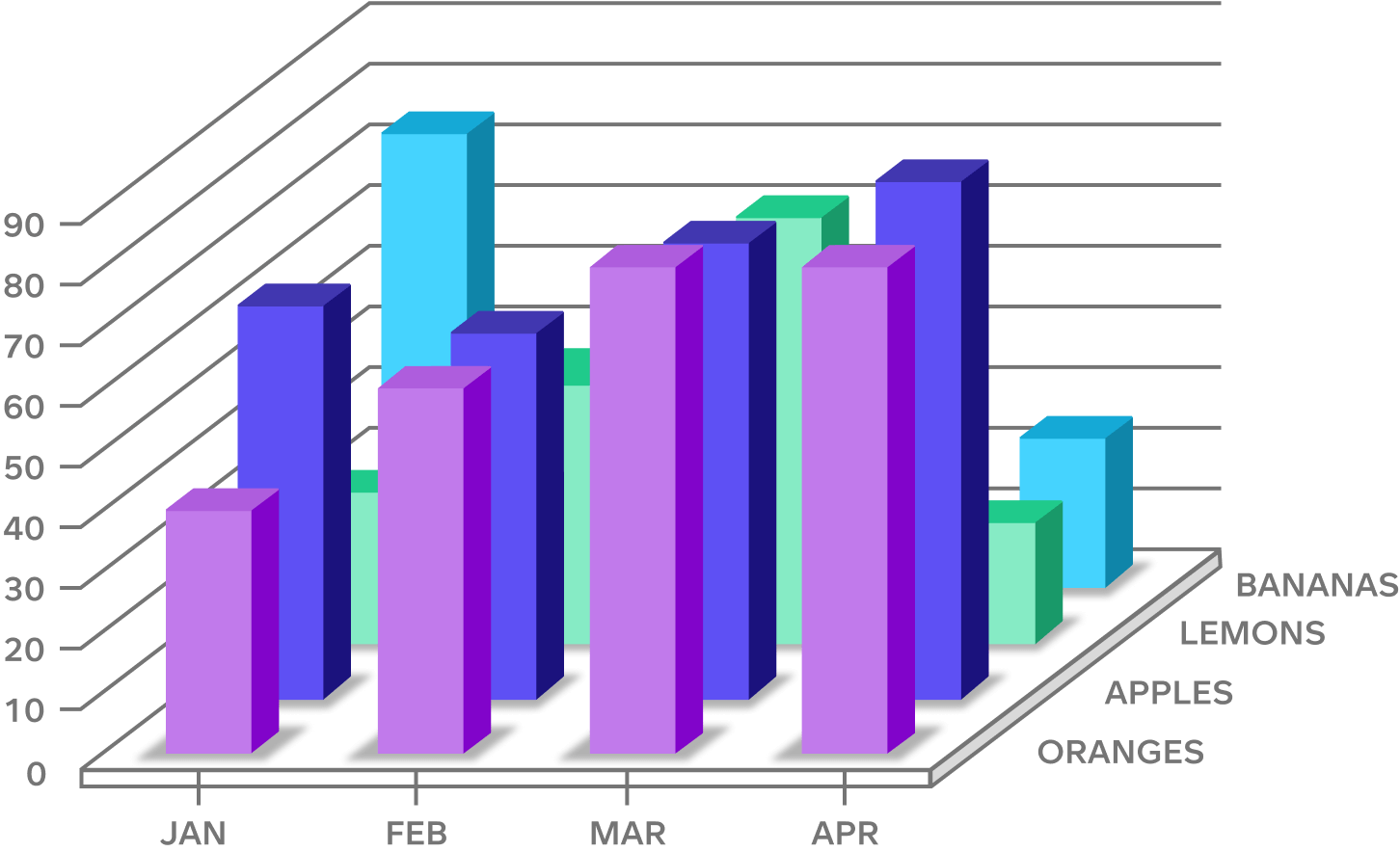
The tools required to visualize data will depend on the project and the complexities of what you need to achieve. If you’d like to visualize some basic data for a presentation, you can use Excel to create some simple charts and graphs. If your data is more complex, you’ll need to create the insights first, which may involve data analysis or data mining. To achieve this, you will likely need to learn a programming language like Python. Alternatively, you can invest in a BI solution(s) such as those listed below.
- Chart generators or plugins: These tend to be used by developers and data engineers because the software requires a more advanced level of expertise. The plugins have many visualization types to choose from and there may even be a data-processing API that allows you to create actionable insights from your data. These tools usually have the capability to categorize and analyze basic data, and so can be used as the foundation of a company’s BI platform.
- Visualization reporting software: This is most often used by report developers and BI engineers. The software creates business and data analysis reports, which can then be turned into visualizations using a selection of built-in charts.
- A fully integrated BI and analytics solution: As the name suggests, this is the most complete solution. A good BI platform will allow you to easily explore data on your own, and create interactive dashboards and charts via a user-friendly no-code UI. The top solutions offer plug-and-play integrations, no-code tools, and flexible embedding options (such as React, Iframes, and Web Components) that allow you to seamlessly embed visualizations and dashboards into your product in a way that matches the brand.
To find out more about some of the best BI solutions with visualization tools, check out Gartner’s Magic Quadrant , which provides an objective analysis of the market leaders.
Data visualization has become so omnipresent that we use it every day without even noticing. Weather maps, bus schedules, computer audio levels, and fitness trackers – all employ visualizations to provide information in a more palatable way.
The above are just some of the ways companies use customer-facing visualizations to improve UX. But they also call upon data visualization to make internal decisions, for example, when looking at how to improve the supply chain , checking product sales across different countries, or how a specific marketing campaign or project is performing. Data visualization is also useful to get a better sense of external factors that might impact the company, such as the economic climate.
Data visualization use cases across different industries
Data visualization is used across all industries, from banking to healthcare . Below we look at how it can be used to improve processes in four different sectors.
- Software as a service (SAAS): Data is the beating heart of most software companies, and many of these embed analytics into their applications. They can then share the data with their customers through visualizations in a user-friendly way. A good example of this is Zendesk, whose software is all about improving the relationship between a business and its customers. Using advanced analytics and visualization tools, Zendesk gives their customers immediate access to the insights they need, which in turn speeds up response times and increases satisfaction among the clients of their customers.
- E-commerce: Data visualization helps brands and suppliers streamline their logistics and supply chains and optimize their operations. An e-commerce platform might use visualization to both improve the consumer experience and optimize brand performance. They could, for example, use their data to get a better understanding of how shoppers are using their site, helping them to better target customers and grow their business. Visualization is necessary to share these customer insights both internally and externally.
- Financial services: Financial firms leverage data and analytics to meet compliance requirements, manage risk, improve efficiency, and grow their business. Time is money, so the quicker they can make insights available to their employees and partners, the better. Visualization can also be used to help mitigate risk in real-time, and create highly personalized experiences for customers within financial service products.
- Insurance: Insurance firms rely on analysts to create impactful insights. These insights are then shared across the enterprise with people such as adjusters, underwriters, and marketers. Data visualization is crucial for insurance companies as it allows them to present clear insights, increase speed, and reduce inaccuracy.
With the right analytics platform, it is easy to create insights from your data and beautifully visualize them on dashboards. So why do some visualizations fail to achieve what they set out to do? The simple answer is that you need to be intentional about the information you want to convey. Good data visualization requires thoughtful human design. To that end, it can be useful to ask yourself the following questions to ensure best practice.
- What story am I trying to tell?
Visualization is a form of storytelling , only you’re using visuals instead of words. You need to find a clear way to tell the story to ensure it has the greatest impact. Data metrics and attributes must be relevant to the story that you are trying to tell. If you’re allowing users to filter, consider carefully how this will allow them to create their own stories from one source of truth.
- Have I designed the visualization for the viewer’s eye?
Once you’re clear on who the visualization is designed for and what you want them to take from it, you can focus on UX. Big-picture clarity is crucial, but it’s important not to neglect the details either. For example, what do the colors you’re using mean? Red usually implies danger, green is considered more positive. Can you customize the visualization to fit the brand? What about shortening numbers to make them easier to read (e.g., using K, M, B for thousands, millions, billions)?
- Am I trying to display too much data?
When it comes to data visualization, less is more. Although it’s tempting to present as many insights as possible, there is a fine line to be walked between insightful and overwhelming.
- Have I provided context?
Without a goal or a benchmark, users may miss the key insights. It is important to standardize benchmarks so that comparisons are not being made between two different things – which can lead to misleading takeaways.
The above are just some of the questions you might consider when creating visualizations. For a deeper dive, check out our article on visualization best practices .
Our analytics platform allows you to fully integrate real-time customized dashboards into your app or product. To see how this works and get your questions instantly answered, book a demo to help you decide whether this is the right tool for your needs. Alternatively, for some first-hand experience with data visualization, sign up for a free GoodData trial .
To learn more about data visualization and how it can help your business, you might like to check out some of our other resources:
Interactive vs. non-interactive data visualizations
7 ways to create great data visualizations
Related content
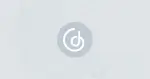
Subscribe to GoodData Updates
Receive regular insights from GoodData delivered directly to your inbox.
You are now subscribed to GoodData’s Product Updates .
You are now subscribed to GoodData’s Updates .
- Artificial Intelligence
- Generative AI
- Business Operations
- Cloud Computing
- Data Center
- Data Management
- Emerging Technology
- Enterprise Applications
- IT Leadership
- Digital Transformation
- IT Strategy
- IT Management
- Diversity and Inclusion
- IT Operations
- Project Management
- Software Development
- Vendors and Providers
- Enterprise Buyer’s Guides
- United States
- Middle East
- España (Spain)
- Italia (Italy)
- Netherlands
- United Kingdom
- New Zealand
- Data Analytics & AI
- Newsletters
- Foundry Careers
- Terms of Service
- Privacy Policy
- Cookie Policy
- Copyright Notice
- Member Preferences
- About AdChoices
- Your California Privacy Rights
Our Network
- Computerworld
- Network World
What is data visualization? Presenting data for decision-making
Data visualization is the presentation of data in a graphical format to make it easier for decision makers to see and understand trends, outliers, and patterns in data..

Data visualization definition
Data visualization is the presentation of data in a graphical format such as a plot, graph, or map to make it easier for decision makers to see and understand trends, outliers, and patterns in data.
Maps and charts were among the earliest forms of data visualization. One of the most well-known early examples of data visualization was a flow map created by French civil engineer Charles Joseph Minard in 1869 to help understand what Napoleon’s troops suffered in the disastrous Russian campaign of 1812. The map used two dimensions to depict the number of troops, distance, temperature, latitude and longitude, direction of travel, and location relative to specific dates.
Today, data visualization encompasses all manners of presenting data visually, from dashboards to reports, statistical graphs, heat maps, plots, infographics, and more.
What is the business value of data visualization?
Data visualization helps people analyze data, especially large volumes of data, quickly and efficiently.
By providing easy-to-understand visual representations of data, it helps employees make more informed decisions based on that data. Presenting data in visual form can make it easier to comprehend, enable people to obtain insights more quickly. Visualizations can also make it easier to communicate those insights and to see how independent variables relate to one another. This can help you see trends, understand the frequency of events, and track connections between operations and performance, for example.
Key data visualization benefits include:
- Unlocking the value big data by enabling people to absorb vast amounts of data at a glance
- Increasing the speed of decision-making by providing access to real-time and on-demand information
- Identifying errors and inaccuracies in data quickly
What are the types of data visualization?
There are myriad ways of visualizing data, but data design agency The Datalabs Agency breaks data visualization into two basic categories:
- Exploration: Exploration visualizations help you understand what the data is telling you.
- Explanation: Explanation visualizations tell a story to an audience using data .
It is essential to understand which of those two ends a given visualization is intended to achieve. The Data Visualisation Catalogue , a project developed by freelance designer Severino Ribecca, is a library of different information visualization types.
Some of the most common specific types of visualizations include:
2D area: These are typically geospatial visualizations. For example, cartograms use distortions of maps to convey information such as population or travel time. Choropleths use shades or patterns on a map to represent a statistical variable, such as population density by state.
Temporal: These are one-dimensional linear visualizations that have a start and finish time. Examples include a time series, which presents data like website visits by day or month, and Gantt charts, which illustrate project schedules.
Multidimensional: These common visualizations present data with two or more dimensions. Examples include pie charts, histograms, and scatter plots.
Hierarchical: These visualizations show how groups relate to one another. Tree diagrams are an example of a hierarchical visualization that shows how larger groups encompass sets of smaller groups.
Network: Network visualizations show how data sets are related to one another in a network. An example is a node-link diagram, also known as a network graph , which uses nodes and link lines to show how things are interconnected.
What are some data visualization examples?
Tableau has collected what it considers to be 10 of the best data visualization examples . Number one on Tableau’s list is Minard’s map of Napoleon’s march to Moscow, mentioned above. Other prominent examples include:
- A dot map created by English physician John Snow in 1854 to understand the cholera outbreak in London that year. The map used bar graphs on city blocks to indicate cholera deaths at each household in a London neighborhood. The map showed that the worst-affected households were all drawing water from the same well, which eventually led to the insight that wells contaminated by sewage had caused the outbreak.
- An animated age and gender demographic breakdown pyramid created by Pew Research Center as part of its The Next America project , published in 2014. The project is filled with innovative data visualizations. This one shows how population demographics have shifted since the 1950s, with a pyramid of many young people at the bottom and very few older people at the top in the 1950s to a rectangular shape in 2060.
- A collection of four visualizations by Hanah Anderson and Matt Daniels of The Pudding that illustrate gender disparity in pop culture by breaking down the scripts of 2,000 movies and tallying spoken lines of dialogue for male and female characters. The visualizations include a breakdown of Disney movies, the overview of 2,000 scripts, a gradient bar with which users can search for specific movies, and a representation of age biases shown toward male and female roles.
Data visualization tools
Data visualization software encompasses many applications, tools, and scripts. They provide designers with the tools they need to create visual representations of large data sets. Some of the most popular include the following:
Domo: Domo is a cloud software company that specializes in business intelligence tools and data visualization. It focuses on business-user deployed dashboards and ease of use, making it a good choice for small businesses seeking to create custom apps.
Dundas BI: Dundas BI is a BI platform for visualizing data, building and sharing dashboards and reports, and embedding analytics.
Infogram: Infogram is a drag-and-drop visualization tool for creating visualizations for marketing reports, infographics, social media posts, dashboards, and more. Its ease-of-use makes it a good option for non-designers as well.
Klipfolio: Klipfolio is designed to enable users to access and combine data from hundreds of services without writing any code. It leverages pre-built, curated instant metrics and a powerful data modeler, making it a good tool for building custom dashboards.
Looker: Now part of Google Cloud, Looker has a plug-in marketplace with a directory of different types of visualizations and pre-made analytical blocks. It also features a drag-and-drop interface.
Microsoft Power BI: Microsoft Power BI is a business intelligence platform integrated with Microsoft Office. It has an easy-to-use interface for making dashboards and reports. It’s very similar to Excel so Excel skills transfer well. It also has a mobile app.
Qlik: Qlik’s Qlik Sense features an “associative” data engine for investigating data and AI-powered recommendations for visualizations. It is continuing to build out its open architecture and multicloud capabilities.
Sisense: Sisense is an end-to-end analytics platform best known for embedded analytics. Many customers use it in an OEM form.
Tableau: One of the most popular data visualization platforms on the market, Tableau is a platform that supports accessing, preparing, analyzing, and presenting data. It’s available in a variety of options, including a desktop app, server, and hosted online versions, and a free, public version. Tableau has a steep learning curve but is excellent for creating interactive charts.
Data visualization certifications
Data visualization skills are in high demand. Individuals with the right mix of experience and skills can demand high salaries. Certifications can help.
Some of the popular certifications include the following:
- Data Visualization Nanodegree (Udacity)
- Professional Certificate in IBM Data Science (IBM)
- Data Visualization with Python (DataCamp)
- Data Analysis and Visualization with Power BI (Udacity)
- Data Visualization with R (Dataquest)
- Visualize Data with Python (Codecademy)
- Professional Certificate in Data Analytics and Visualization with Excel and R (IBM)
- Data Visualization with Tableau Specialization (UCDavis)
- Data Visualization with R (DataCamp)
- Excel Skills for Data Analytics and Visualization Specialization (Macquarie University)
Data visualization jobs and salaries
Here are some of the most popular job titles related to data visualization and the average salary for each position, according to data from PayScale .
- Data analyst: $64K
- Data scientist: $98K
- Data visualization specialist: $76K
- Senior data analyst: $88K
- Senior data scientist: $112K
- BI analyst: $65K
- Analytics specialist: $71K
- Marketing data analyst: $61K
Related content
Tableau further democratizes analytics with ai-fueled features, the secret to successful citizen data science programs: good governance, tableau’s new slack capabilities democratize data, from our editors straight to your inbox, show me more, going public: corporate cios make the shift to government it leadership.

Legendary CIO Anil Cheriyan on delivering innovation that matters

What tech Infrastructure NSW’s ICT director aligns with business objectives

CIO Leadership Live Australia with Nazih Battal, Chief Information and Technology Officer at Rashays

Mike Aiello, CTO at Secureworks, joins CIO Leadership Live from Foundry's CIO100 event NEW

CIO Leadership Live Australia with Andrew Dome, Chief Digital Information Officer at Uniting

Kubecost helps firms monitor, optimize their Kubernetes and cloud spend

Mike Aiello, CTO at Secureworks, joins CIO Leadership Live from Foundry's CIO100 event

Sponsored Links
- Everybody's ready for AI except your data. Unlock the power of AI with Informatica
- Everyone’s moving to the cloud. Are they realizing expected value?
- The cloud shouldn’t be complicated. Unlock its potential with SAS.
- The future of identity is here. Unlock brand growth with Merkury
What is Data Visualization? Definition, Examples, Best Practices
This guide provides an introduction to data visualization, including real-world examples, best practices and editable templates.
- Share on LinkedIn
- Share through Email
- Print this page
- Bookmark this page
Resource Details
June 5, 2020
Data visualization is the visual presentation of data or information. The goal of data visualization is to communicate data or information clearly and effectively to readers. Typically, data is visualized in the form of a chart, infographic, diagram, or map.
The field of data visualization combines both art and data science. While data visualization can be creative and pleasing to look at, it should also be functional in its visual communication of the data.
This resource explains the fundamentals of data visualization, including examples of different types of data visualizations and when and how to use them to illustrate findings and insights.
Do you have feedback on this resource?
Thank you for your feedback as we strive to curate and publish resources to help social impact organizations succeed with data.
Send us a note
Explore More
Related Guides & Resources
Getting started with data visualization.
Visualizing data is one of the most effective ways of communicating data. This can take many forms from live digital data dashboards to static charts shared in social media channels.
Communicating Results: Design your Visualization
Let’s get visual: nonprofit data visualization.
All resources
- Blog Articles
- Use Cases & Examples
- Guides & Resources
- Dashboard Templates
- Case Studies
- Testimonials
What is data visualization
Why use data visualization, the advantages and benefits of good data visualization, principles of successful data visualization, how to choose a chart type, how to tell the visual story, designing the dashboard, why data visualization is important, reporting and visualization software tools comparison, looker studio by google, google sheets, key takeaways, what is data visualization: definition, principles, examples, tools.
Vlada Malysheva , Creative Writer @ OWOX
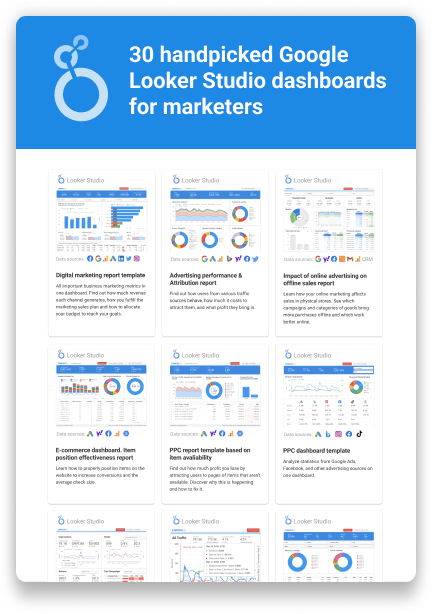
Top 30 Handpicked Google Looker Studio Dashboards for Marketers
65% of people are visual learners , making data visualization an effective way to communicate information.
When Excel spreadsheets aren’t enough to connect the dots between your data and there’s no possibility to involve data or digital analyst to get the report quickly, data visualization software tools and tools is what you need to become data-savvy.
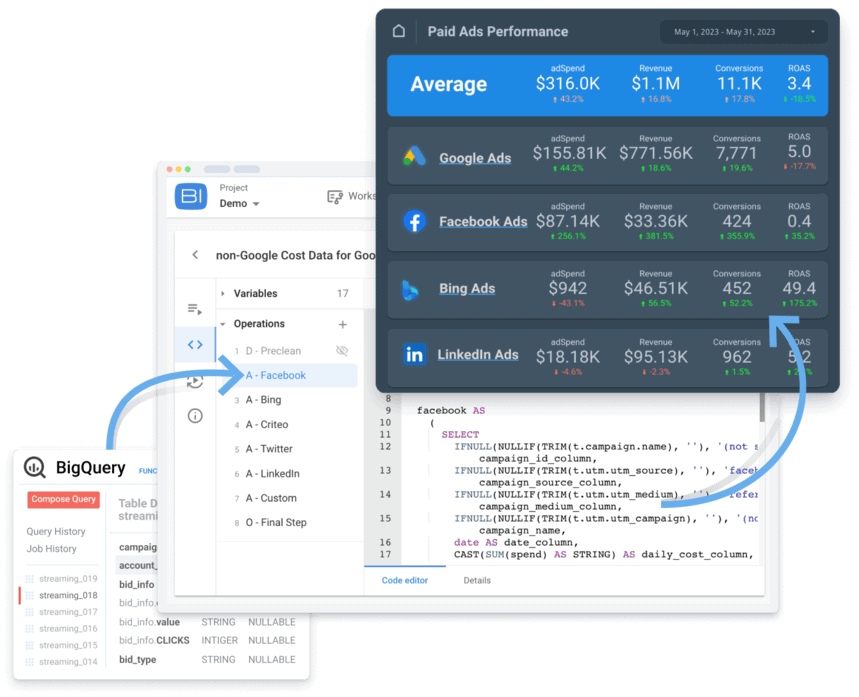
In this article, which was last updated in January 2024, we’ll show you what data visualization techniques are available, how to visualize data correctly, which tools can be used for engaging and interactive visualizations without any help from developers or data professionals, and how to choose a tool that suits your specific needs.
The definition of data visualization is the visual representation of your data. With the help of charts, maps, and other graphical elements these tools provide a simple and comprehensible way to clearly see and easily discover insights and patterns in your data.
Data visualization is the graphical representation of data using visual elements such as charts, graphs, and maps.
It is a way to communicate complex information in a visual and intuitive manner, making it easier for people to understand and analyze the data. By transforming raw data into visual representations, data visualization allows patterns, trends, and insights to be easily identified and interpreted.
Data visualization is also a powerful storytelling tool. Visual storytelling helps to uncover hidden patterns, relationships, and correlations that may not be apparent, or not visible in raw data. Through visualizations, data can be presented in a way that is engaging, impactful, and memorable, enabling effective communication and data-driven decision-making .
Data visualization is not limited to a specific field or industry. It's not only about marketing data and is used in various domains such as business, finance, healthcare, education, or journalism. In business, data visualization is used to analyze sales trends, key performance indicators, and present business metrics. In healthcare, it is used to visualize patient data, monitor disease outbreaks, and analyze medical research. In journalism, it is used to create better stories and increase reach and consumption.
If you want your Facebook post to be read by as many people as possible, what will you do? You’ll add an interesting visual. This trick works perfectly with reports too. Data-driven visuals attract more attention, are easier to understand, and assist in getting your message across to the audience quickly.
With the help of descriptive graphics and dashboards, even difficult information can be clear and comprehensible.
Why is that?
Most people are visual learners. So if you want the majority of your partners, colleagues, and clients to be able to interact with your data, you should turn boring charts into beautiful graphics. Here are some noteworthy numbers, based on research, that confirm the importance of visualization:
- People get 90% of information about their environment from the eyes.
- 50% of brain neurons take part in visual data processing.
- Pictures increase the wish to read a text up to 80%.
- People remember 10% of what they hear, 20% of what they read, and 80% of what they see.
- If a package insert doesn’t contain any data illustrations, people will remember 70% of the information. With pictures added, they’ll remember up to 95%.
With OWOX BI , your data is collected, normalized, attributed & prepared for reporting.
Use our templates to get reports built in minutes, or use your data to prepare the data for any report you need and visualize it in Looker Studio (formerly Google Data Studio), Google Sheets or the BI tool of your choice. Save 70+ hours on data preparation every month and automate your entire digital marketing reporting.
Relevant visualization brings lots of advantages for your business:
- Fast decision-making. Summing up data is easy and fast with graphics, which let you quickly see that a column or touchpoint is higher than others without looking through several pages of statistics in Google Sheets or Excel... or even a database or a CRM or CMS system.
- More stakeholders are involved. Most people are better at perceiving and remembering information presented visually and delivered on time in a visual-appealing format.
- Higher level of involvement. Beautiful and bright graphics with clear messages attract readers’ attention.
- Better understanding. Perfect reports are transparent not only for technical specialists, analysts, and data scientists but also for CMOs, CEOs and other C-levels or managers, and help each and every worker make decisions in their area of responsibility.
The first thing to do before creating any chat is to check all information for accuracy and consistency.
For example, if the scaling factor is 800%, whereas the average is 120–130%, you should check where this number is coming from. Maybe it’s some kind of an outlier that you need to delete from the graph so it doesn’t skew the overall picture: 800% downplays the difference between 120% and 130%. This kind of outlying data in a report can lead to incorrect decisions made.
To increase the chances of success in marketing, the right message should be delivered to the right person at the right time.
The same three rules are applied for data visualization:
- Choose the right chart to visualize the answer to specific question based on your goal.
- Confirm that the message to deliver the result of your report suits your audience (the stakeholder).
- Use an appropriate design for the chart to deliver that message.
If your message is timely but the chat or graphic isn’t dynamic, or it provides incorrect insights. or the design is not attractive, then you won’t achieve the results you were dreaming of.
If you choose the wrong chart or graph, your readers will be confused or interpret or read the results incorrectly. That’s why before creating a report with charts, it’s important to decide what data you want to visualize and for what purpose, for example:
- To compare different data points
- To show data distribution : for instance, which data points are frequent and which are not
- To show the structure of something with the help of data
- To follow the connections, references or correlation between data points
Let’s have a look at the most popular types of charts and the goals they can help you achieve.
1. Line chart
A line chart is a type of data visualization that uses a series of data points connected by straight lines. It is commonly used to show the relationship between two variables over a continuous period of time. Foe example, the x-axis represents the time or the independent variable, while the y-axis represents the value or the dependent variable.
By plotting the data points and connecting them with lines, the line chart provides a visual representation of how the values change over time.
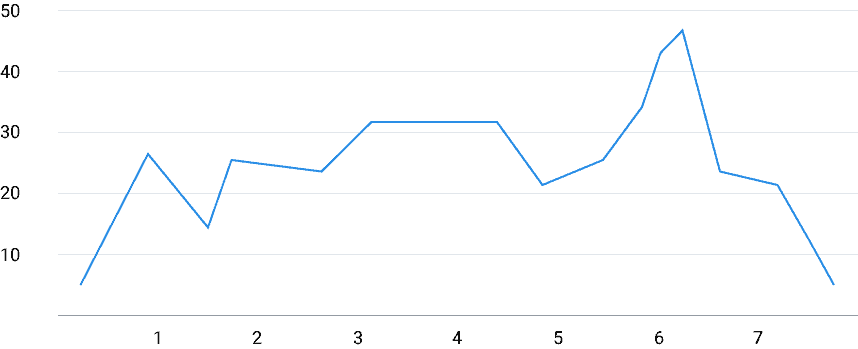
Pros of Line Charts
One of the main advantages of line charts is their ability to display trends and patterns in data . They make it easy to identify the overall direction of change, whether it is increasing, decreasing, or remaining stable.
Line charts also allow for the comparison of multiple data series on the same chart, making it simple to analyze the correlation between different variables .
Additionally, line charts are visually appealing and easy to understand, making them accessible to a wide range of audiences.
Cons of Line Charts
However, line charts also have some limitations. They are most effective when used with continuous data, such as time series data, and may not be suitable for categorical or discrete data .
Line charts can become cluttered and confusing if there are too many data points or series plotted on the chart. They may also not be the best choice for displaying data with irregular or inconsistent intervals. It is important to consider these factors when deciding whether to use a line chart for data visualization.
Use cases of Line Charts
The best use cases for line charts include analyzing sales or revenue data over time , tracking website traffic or user engagement metrics , visualizing stock market trends, or monitoring changes in weather patterns.
Line charts are particularly useful when there is a need to understand the overall trend or pattern in the data and identify any significant changes or anomalies. They are also effective for presenting data to a non-technical stekholders, as they provide a clear and really easy and intuitive representation of the data.
2. Bar chart
Type of diagram that represents data using rectangular bars is called bar chart. Each bar corresponds to a specific metric or variable, while its length or height represents the value associated with that metric.
Bar charts are typically used to compare different metrics or track changes over time providing simplicity and versatility.
Horizontal bar charts are often used when you need to compare lots of data sets or to visually emphasize the distinct advantage of one of the data sets.
Vertical bar charts display how data points change over time — for example, how the annual company profit has changed over the past few years.
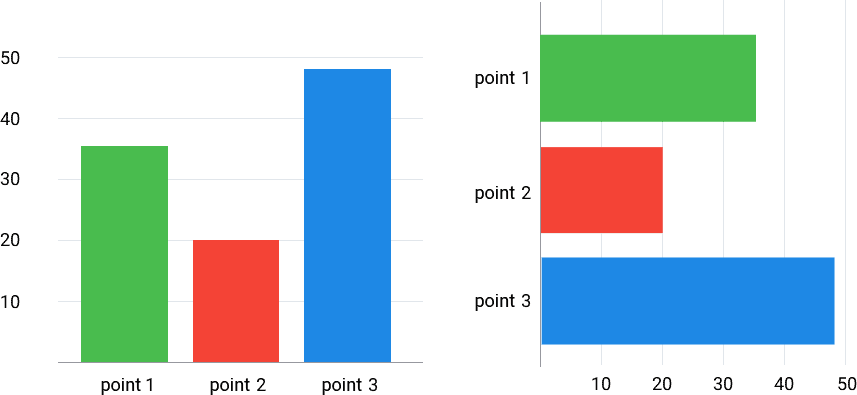
Pros of Bar Charts
Bar charts are ease of read and consume, no background in data analysis is required.
The clear and straightforward presentation of data in bar charts allows for quick insights and understanding.
Additionally, bar charts can accommodate large datasets and display multiple variables simultaneously (and stay usable).
Cons of Bar Charts
Continuous data, such as temperature measurements over time, may not be as suitable for bar charts.
Bar charts may also not be the best choice for displaying complex relationships or correlations between variables, as they primarily focus on comparing values within categories.
Use cases of Bar Charts
Some common use cases include sales analysis, market research, financial reporting, and survey results. For example, a bar chart can be used to compare the market share of different companies in a specific industry , or to visualize the responses to a survey question with multiple answer options.
A bar chart can also represent the sales figures of different products in a given month , with each bar representing a product or a category, and its height indicating the sales quantity. This visual representation allows for easy comparison and identification of trends or patterns in the data.
The of bar charts make them a valuable tool for data visualization in various domains.
3. Histogram
A histogram is often mistaken for a bar chart due to their visual similarities, but the goals of these charts are different.
A histogram shows the distribution of a dataset across a continuous interval or a definite time period. It is a graphical representation of the frequency of data values in different intervals or bins. The x-axis of a histogram represents the range of values in the dataset, divided into equal intervals, while the y-axis represents the frequency or count of data values falling within each interval. The height of each bar in the histogram corresponds to the frequency of data values in that interval. This chart provides a visual summary of the underlying data distribution.
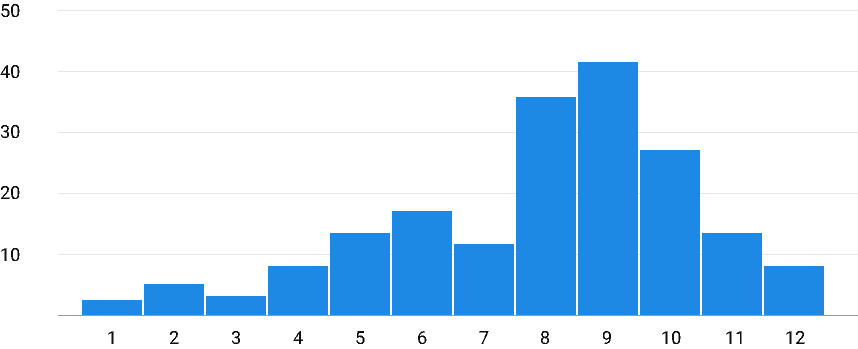
Unlike a histogram, a bar chart doesn’t show any continuous interval; each column displays a category of its own. It’s easier to demonstrate the number of purchases in different years with the help of a bar chart.
If you want to know the number of order beween $10 and 100, $101 and 200, $201 and 300, etc. of purchases, it’s better to choose a histogram. The histogram will show you the frequency of orders falling within each price range, allowing us to identify patterns such as a normal distribution, skewed distribution, or outliers.
Histogram allows you to quickly identify the central tendency, spread, and shape of the dataset. Histograms are particularly useful when dealing with large datasets or continuous data, as they provide a visual summary without overwhelming the viewer with individual data points.
What are the limitation of the histogram?
First, the choice of bin size or interval width can impact the interpretation of the data. A smaller bin size can provide more detailed information but may also result in a cluttered or noisy chart . At the same time, a larger bin size can oversimplify the data distribution .
Second, histograms may not be suitable for datasets with categorical or ordinal variables , as they require numerical data to create meaningful intervals.
4. Pie chart
A pie chart is a type of data visualization that displays shares of each value in a data set.
It is divided into slices, where each slice represents a proportion or percentage of the whole. The size of each slice is determined by the value it represents in relation to the total value of the data set. Pie charts are commonly used to show the distribution or composition of a categorical variable.
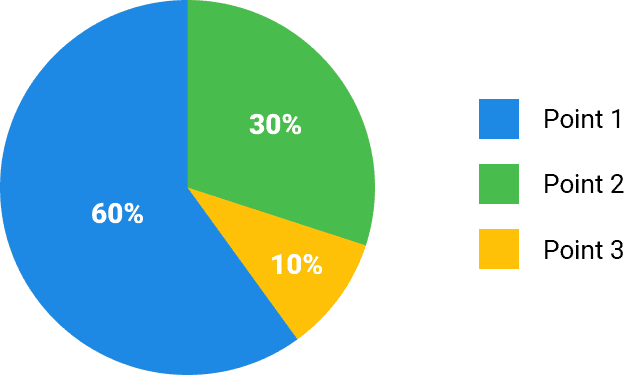
Pie chart visually displays the relative proportions of different categories within a data set. It allows viewers to quickly grasp the overall distribution of the data and easily compare the sizes of different categories.
The angles of the slices in the pie chart represent the proportions of the categories, making it easy to understand the relationship between the parts and the whole. For instance, what percentage of general sales is attributed to each product category?
Pie charts are particularly useful when dealing with data that has a small number of categories or when the emphasis is on comparing the parts to the whole. They can also be useful for highlighting a specific category or identifying outliers.
The biggest pie chart limitation is that they can become difficult to interpret when there are too many categories or when the differences between the categories are small. It can be challenging to accurately compare the sizes of the slices, especially if they are similar in magnitude.
Additionally, pie charts do not easily don't represent the trends over time. Pie charts are commonly used in business and marketing to represent market share, customer demographics, or product sales by category. Pie charts are also used in survey data to display the distribution of responses for multiple-choice questions. Overall, pie charts are most effective when the data is simple, the categories are distinct, and the emphasis is on comparing the parts to the whole.
5. Scatter plot
A scatter plot chart displays the relationship between two numerical variables . It uses a Cartesian coordinate system, where each data point is represented by a dot or marker on the chart.
The x-axis represents one variable, while the y-axis represents the other variable. By plotting the data points on the chart, you can visually analyze the correlation or pattern between the variables.
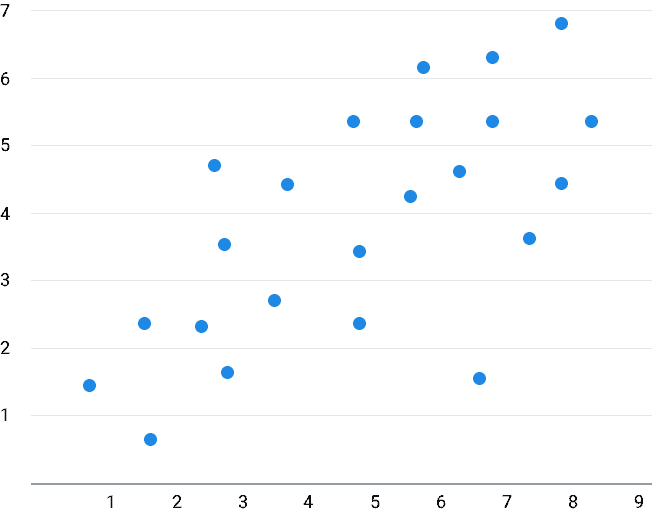
The scatter plot chart allows you to identify trends, clusters, or outliers in the data.
Additionally, scatter plots can be used to detect any patterns or irregularities in the data distribution.
The main limitation is that it can only represent two variables at a time. If there are more than two variables to analyze, additional charts are required. Also, scatter plots may not be suitable for large datasets, as the overlapping data points can make it difficult to make decisions based on the chart accurately.
For example, with the help of a scatter plot, you can find out how the conversion rate changes depending on the size of the product discount.
6. Bubble chart
This is an interesting chart that allows you to compare two parameters by means of a third.
It is a variation of a scatter plot, where the size of the bubbles is used to convey additional information. The bubble chart is particularly useful when visualizing three variables, as it allows for the representation of two continuous variables on the x and y axes, while the size of the bubbles represents the third variable. This makes it easy to identify patterns and relationships between the variables in a single chart.
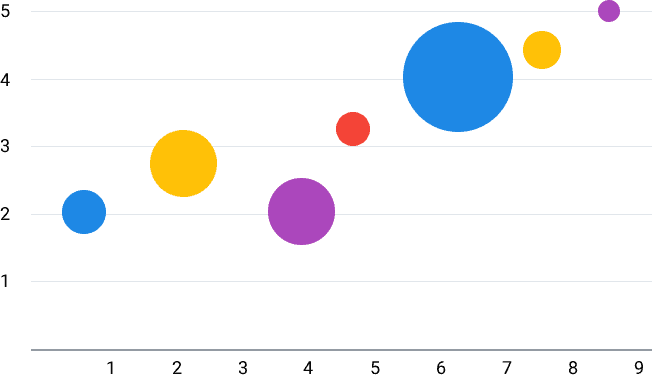
Bubble chat allows you to display large amounts of data in a visually appealing and intuitive way. By using different colors or shades, you can also incorporate a fourth variable into the chart, further enhancing the information conveyed. The size of the bubbles provides a quick visual cue, allowing for easy comparisons between data points.
Additionally, the bubble chart can be interactive, allowing users to hover over or click on the bubbles to reveal more detailed information.
Basically, the main drawback of the bubble charts is that the size of the bubbles can sometimes be misleading, as it may not accurately represent the magnitude of the data point. This can be mitigated by scaling the size of the bubbles appropriately or by providing a clear legend or scale.
Also, it is important to strike a balance between the number of data points and the readability of the chart.
The best use cases for bubble charts are situations where you want to visualize relationships between three variables.
For example, you can use a bubble chart to show the relationship between the price, size, and number of orders of different products. It can also be used to compare data across different categories or groups, such as comparing the revenue, market share, and growth rate of different companies in an industry.
Bubble charts are particularly effective when the size of the bubbles is meaningful and provides valuable insights into the data.
7. Geo chart
The geo chart is a simple one. It’s used when you need to demonstrate a certain data distribution across regions, countries, and continents.
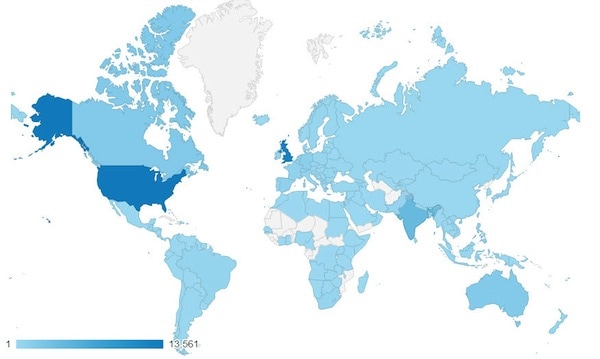
By visualizing data on a map, a geo chart provides a clear and intuitive way to understand spatial patterns and user behavior. For example, a geo chart can show shopping frequency across countries, GDP per capita by country, or election results by region. It allows viewers to quickly grasp the variations and disparities between different locations. Basically, geo chart works best if metric dimension is geographical.
By mapping data onto a familiar geographic locations, it becomes easier for viewers to interpret and remember the information.
Since a geo chart relies on colors or patterns to represent data, it is important to choose appropriate color schemes and legends to avoid confusion or bias. Furthermore, a geo chart may not be suitable for displaying complex or detailed data, as the level of granularity is often limited to the size and boundaries of the regions on the map. It is important to carefully select the level of detail and aggregation that best suits the purpose of the report.
For example, when analyzing sales data, a geo chart can show the distribution of sales across different regions , helping businesses identify potential markets or areas of improvement.
Overall, geo charts are particularly effective when the spatial dimension of the data is crucial for decision-making or storytelling.
The second important thing that you have to take into account while working with visualization is choosing the right message for the audience . The information you talk about, the story you tell in the report should be clear and informative for your readers.
Here’s a chart that was awarded the prestigious Data Journalism Award.
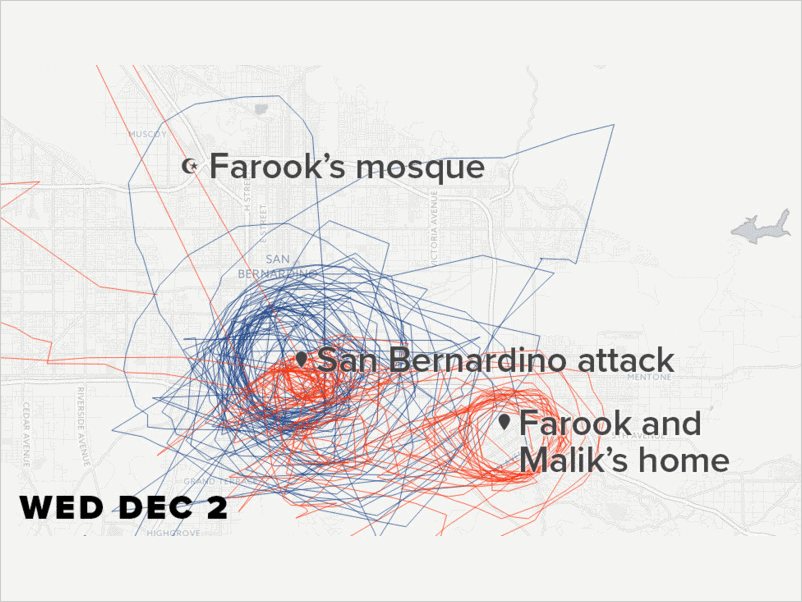
For people who aren’t familiar with the background to the story, this chart looks like a picture made by a three-year-old. However, when you find out a little bit more about it, you can see the huge amount of work done by its authors.
Charles Seife and Peter Aldhous, Buzzfeed News editors, used the R language to visualize flight data obtained by FBI and DHS agents as part of air surveillance. Specifically, this chart shows flights above the house and mosque of those responsible for the mass shooting in December 2015 in San Bernardino, California.
While choosing the parameters you want to visualize on one chart, you have to confirm that they can be combined. Some combinations just aren’t logical, though at first sight the information correlates perfectly. Here’s an example of such a chart with a faulty correlation. It shows that the number of people who drowned by falling into a pool correlates with the number of Nicolas Cage films.
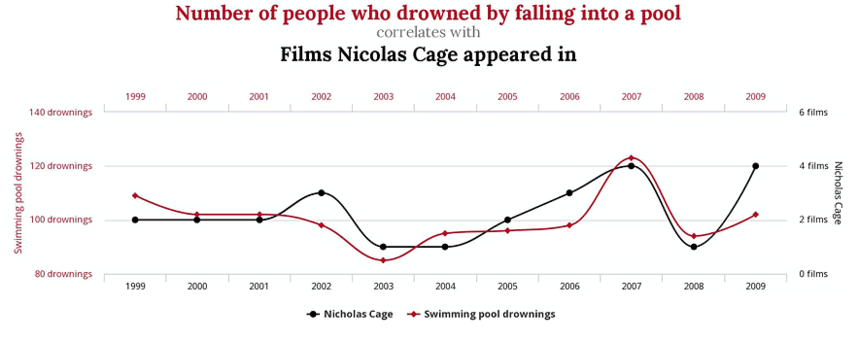
The next things you should take into account when creating a chart are the scale and scope. People are used to the fact that measurements on axes start from the bottom and from the left. If you change the direction of measurement, it will confuse an inattentive audience. Although we should mention that reversing the measurement is possible when used as a tactical maneuver, as in this example:
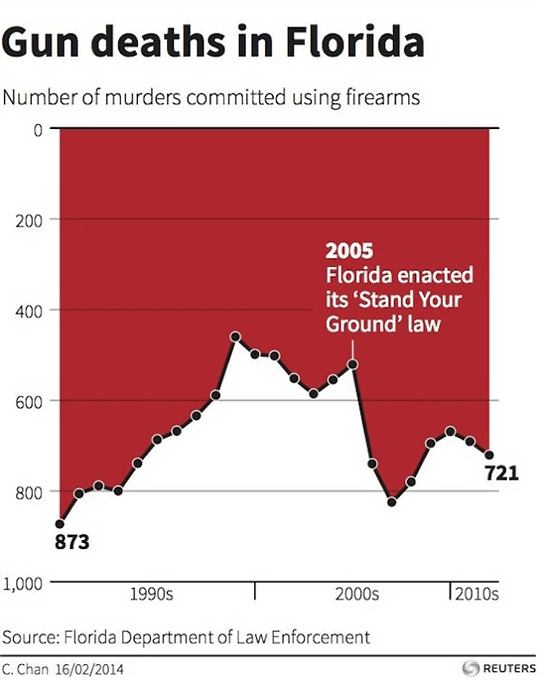
At first sight, it may seem that the number of murders committed using firearms has been decreasing over the years. In fact, it’s the opposite, as the scale starts from the top. Perhaps the author of the chart did this on purpose to decrease the negative response to the results shown.
A suitable scale also makes your chart clearer. If a report shows data points that are too close and you can’t see any movement, try to change the scale. Start the measurements not from zero or divide the scale into smaller parts and the picture will clear up.
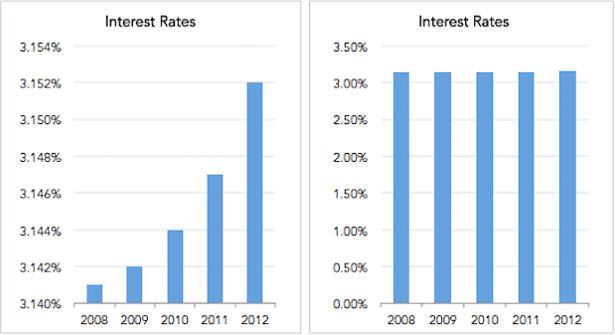
Before giving a report to the stakeholder, make sure that the chart loads fast. Slow loading kills all your efforts.
For example, if you’re visualizing data in Google Sheets, most likely your data is stored on the same page or on the next page and doesn’t come from a third-party source.
But when you create a report in Looker Studio (ex. Data Studio) or Power BI, data will be imported from somewhere else. In this case, you have to pay close attention to the source accessibility and the data flow rate. Otherwise, you’ll see a sad looking picture when there’s a chart template but data hasn’t been loaded.
Remember, the golden rule when you're crafting your chart design is to keep it simple.
When you're tasked with putting together a standard report, don’t fret about making it look fancy. You don't need to dress it up.
Avoid any extra elements that only clutter the chart: too many colors and structures, 3D volume, shadows, gradients, etc.
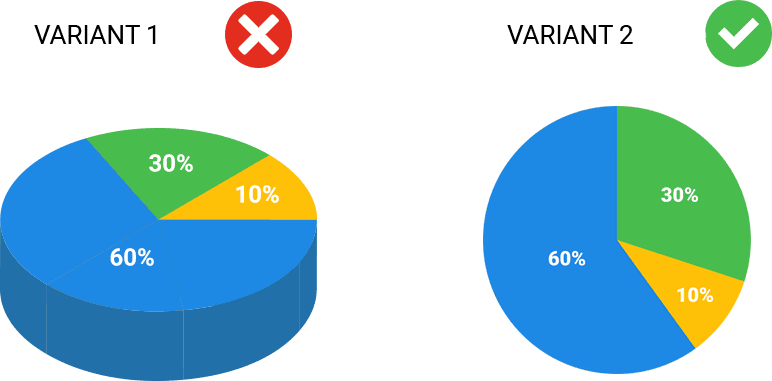
The simpler a chart is - the easier it is for the readers to understand the information you want to share.
Don’t make your visualizations too small, and don’t put all charts on the same dashboard page. It’s considered bad style to use more than three types of charts on one slide or the same dashboard page. If you really need so many chart types, put them on different pages, or make a clear separation, so it’s easy to understand them.
Don’t be afraid to experiment. If you have a task that's not typical, perhaps your solution should also be non-standard. In the infographic below, we can see the wing movement patterns of different animals. The dynamic visualization is totally relevant.
Let’s have a look at some data visualization tools examples and discuss how to choose the right one for your goals.
Visualizing data is an undeniable benefit in any niche, and it doesn’t matter if you’re building a career in marketing, design, retail or anything else.
Making information easy to consume and quickly make smart decisions is one of the keys to finding growth zones and developing your business.
When your colleagues would see the visual charts outlining the current state of the main metrics for you, it’s easier to make sure that all of the team members are on the same page and everyone understands the strong and weak points of the current strategy.
While visualizing reports itself cannot fix the issues, it gives you the wheel to drive the car, to make the necessary changes and improve the KPIs.
Nowadays, there are lots of data visualization and reporting tools on the market. Some of them are paid, others are available for free. Some of them work fully on the web, others can be installed on a desktop but work online, and others are offline only.
Best Reporting Tools
We’ve crafted a list of 10 most popular reporting and data visualization software:
1. Google Spreadsheets
Explore BigQuery Data in Google Sheets
Bridge the gap between corporate BigQuery data and business decisions. Simplify reporting in Google Sheets without manual data blending and relying on digital analyst resources availability
2. Looker Studio (ex. Google Data Studio)
3. Tableau
4. Power BI
6. QlikView
7. R Studio
8. Visual.ly
First six tools and services are created by companies specializing in visualization.
Numbers seven through ten are quite interesting tools, mostly free and online. They offer non-standard types of data visualization and may offer new ways of approaching your business information.
How to select a reporting tool
What to look for when choosing a reporting tool:
- Start from the goals and tasks you want to accomplish. For example, a major trend on the market nowadays is dynamic reports. If a tool cannot work with dynamic reports, that’s a strike against it.
- Consider the amount of money you’re ready to pay. If your team is big enough and every employee has to work with the visualization tool, then the cost per user may be a stop sign.
- Decide who will use the tool and how:
- Is there a possibility for group editing?
- How simple is it to start working with the tool?
- Is the interface user-friendly?
- Is there a possibility to create a report without any knowledge of programming?
For example, R Studio is a great service, especially for searching for trends and building attribution and correlation models. But if you are not familiar with coding, you won't be able to connect any specific libraries, and it would be difficult for you to start working with R Studio.
We'll dive deeper into a few services and guide you through their pros & cons, as well as the main features and advantages. But before we start, let us explain how dynamic data visualization and dynamic reports differs.
Dynamic reports refer to the possibility to import data from different sources in real time.
For example, Looker Studio (formerly Google Data Studio) doesn’t have dynamic reports in place. Let’s say we’ve connected a Looker Studio request from Google BigQuery and then changed something in this request. To record these changes in the report, we need to at least refresh the page.
However, if we add or delete some fields in Google BigQuery (not just change the logic of the calculation but change the table structure), then Looker Studio would show an error. You’ll have to rebuild the dashboard to get the visualizations in place.
Dynamic visualization concept refers to the possibility to look at summary statistics over different dates during one session.
For example, in Google Analytics 4 you can change the time period and get statistics for the date range you need.
OWOX BI is a comprehensive analytics platform that covers everything from data collection and streaming to attribution modeling and reporting. With OWOX BI, companies get a complete view of their marketing activities across various channels, empowering advertising specialists to optimize their ad spending and achieve better ROI.
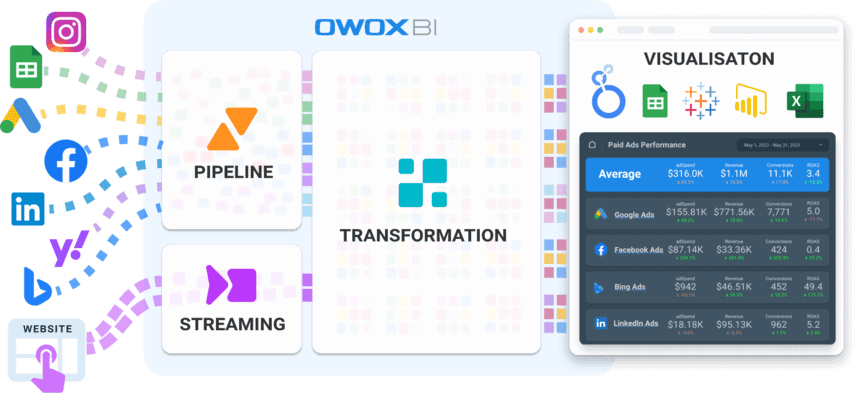
3 whales of data management and analysis
OWOX BI Pipelines facilitates seamless data collection from various advertising platforms, CRMs, and website builders, enabling organizations to consolidate all their data in one place in order to have a data source of truth and gain better insights.
OWOX BI Streaming is a cookieless real-time user behavior tracking system, ensuring privacy compliance with regulation and extending the lifespan of cookies. Marketers can accurately track the entire conversion journey, find the true sources of conversions , and gain a deeper understanding of customer behavior.
OWOX BI Transformation saves time on data preparation (avg. of 70 hours per month). With pre-built low- or no-code transformation templates (based on 100’s delivered projects across multiple industries), businesses can quickly produce trusted datasets for reporting , modeling, and operational workflows:
- Sessionization: Group on-site events into sessions to find conversion sources
- Cost data blending: Merge ad cost data across channels to compare campaign KPIs in a single report
- Attribute ad costs to sessions to measure cohorts and pages' ROI;
- Create cross-device user profiles across different devices
- Identify new and returning user types for accurate analysis
- Apply a set of attribution models : Choose from standard attribution models like First-Click, LNDC, Linear, U-shape, and Time Decay, or create a custom Machine Learning Funnel-based attribution model
- Use modeled conversion for cookieless measurements and conversion predictions
- Prepare data for marketing reports in minutes
Lastly, OWOX BI integrates with visualization tools like Looker Studio, Tableau, or Power BI, enhancing data-driven decision-making by building customizable reports & keeping the data always up-to-date.
OWOX BI Advantages & Benefits
- No technical background, coding experience or knowledge of SQL is required.
- Simple and user-friendly interface: you can collect all of the data and generate reports using our dashboard templates and customize what matters for you the most.
- If you want to working with your data in Google Sheets, you can easily export an aggregated dataset from BigQuery to Google Sheets with our reports add-on .
- You can copy SQL queries generated by OWOX BI.
- You can then modify those queries or use them, for example, to automate a data-based report in Google Sheets or BigQuery.
- You retain complete control over access to that data .
- You can merge digital marketing data with CRM/CMS data.
- Full transperancy.
Note: For enterprise customers, OWOX BI expert team will set up a data model tailored to your business. You’ll be able to evaluate the impact of all marketing efforts — both online and offline.
Types of data you can use
User actions on your site:
- You can set up the collection of raw data from the site in Google BigQuery using OWOX BI Streaming.
- Or you can use the native standard export from Google Analytics 360 or Google Analytics 4 to Google BigQuery.
Transactions Data:
- Google Analytics → Google BigQuery
- Google Sheets → Google BigQuery
- CRM → Google BigQuery
Advertising campaign costs:
- Advertising services → Google Analytics 4
- Advertising services → Google BigQuery
- Other marketing tools → Google BigQuery
Looker Studio, also known as data studio allows you to connect data sources, easily build charts, reports and add elements to visualize and share reports with colleagues in a way that’s similar to other Google products.
Advantages:
- Free (with paid version announced in 2023)
- More than 860 connectors to the data sources that are easy to integrate
- Allows to use data from several sources via one dashboard
- Convenient to share reports
Looker Studio is a free tool with 21 native connectors provided by Google:
- Connect to your Looker semantic models.
- Connect to Google Analytics 4 reporting views.
- Connect to Google Ads performance report data.
- Connect Google Sheets .
- Connect to BigQuery tables and custom queries.
- Connect to AppSheet app data.
- File Upload - Use CSV ( comma-separated values ) files.
- Connect to Amazon Redshift .
- Connect to Campaign Manager 360 data.
- Connect to MySQL databases.
- Connect to Display & Video 360 report data.
- Connect to Microsoft SQL Server databases.
- Connect to PostgreSQL databases.
- Connect to Search Console data.
- Connect to YouTube Analytics data.
and more...
They’re checked, approbated, work well, and perfectly suit to the most common reporting tasks.
There are also connectors provided by Google partners, though you have to understand that connectors can be presented by developers with different skill levels and there’s no guarantee they’ll perform correctly.
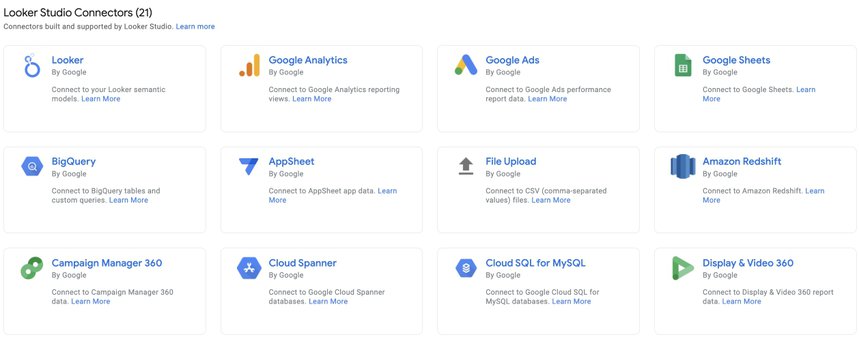
By the way, if you want to see any Facebook or Yahoo Gemini statistics in reports built in Looker Studio, you can import ad cost data into Google BigQuery with OWOX BI. While you may lose some of the important data with other data connectors, with our Facebook Ads to Google BigQuery pipeline you receive complete data ready for analysis and reporting from your Facebook account.
You can also merge your Facebook Ads data with the advertising cost data from Google Ads, Twitter Ads, and LinkedIn ads and get a helicopter view of your marketing performance and optimize your cross-channel budget easily.
Automate your digital marketing reporting
Manage and analyze all your data in one place! Access fresh & reliable data with OWOX BI — an all-in-one reporting and analytics tool
We also have a ready-to-use dashboard templates of our own that we want to share.
We've prepared a comprehensive Looker Studio dashboard template gallery with ready-to-use templates so that you can quickly create a guide to your business results, KPIs and performance.
The first is a All-in-one Performance Dashboard . With this dashboard, you can find all of the basic metrics and metrics to stay on top of your advertising and marketing performance and achieve the desired ROI.
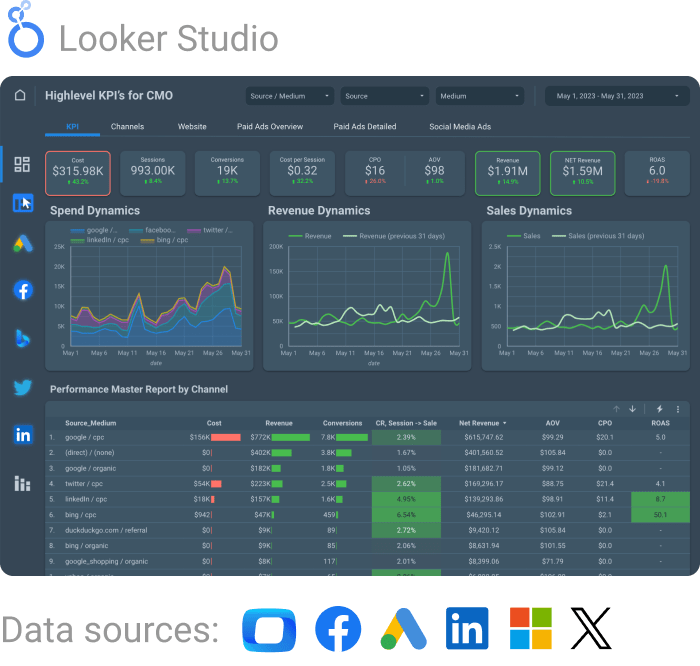
All-in-one Digital marketing Dashboard
Another dashboard template we'd like to share is the Digital Marketing Paid Channels KPI dashboard , which is segmented by data sources (shown in detail). In other words, it shows filtered data on Facebook marketing campaigns, etc.
Those are the dashboard templates. Make a copy, change the data sources to your own, and use them to build beautiful reports based on your data.
One of the recent Looker Studio updates adds the possibility to filter information by view. For example, you can compare data points over the current period and the previous year.
One more interesting update allows you to change the type of an already created chart, graph or element. Earlier, when changing a chart, you had to delete it and create a new one.
Useful links:
- Webinar: Mastering Marketing KPIs
- Google Looker Studio dashboard template gallery
Mastering Marketing KPIs: How to Evaluate Your Marketing Performance
You'll learn how you can effectively evaluate your marketing performance to fuel your business growth.
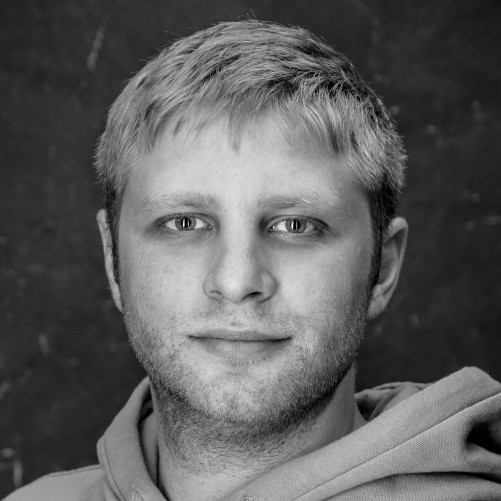
Marketing Ninja @ OWOX
This is one of the two most popular data reporting tools (together with Microsoft Excel) that’s used by any marketing specialist at least once. The Google Sheets interface is quite simple and easy-to-use, especially for those who just starting analytics out.
- Flexible — supports dynamic parameters, vlookups, pivot tables, formulas, app scripts etc.
- Easy to integrate with data sources (but not so easy to automate updates)
- Convenient to share reports via links
The charts and reports types in Google Sheets are the same as that is in Looker Studio.
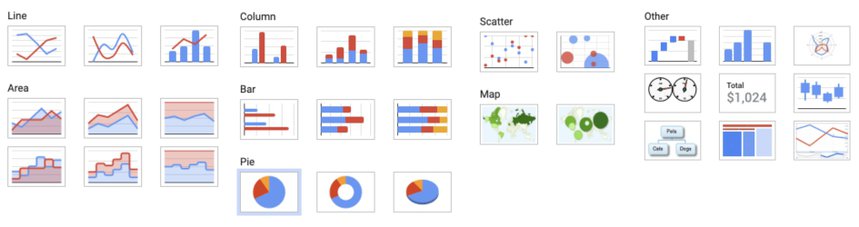
Conditional Formatting
Conditional formatting in Google Sheets allows users to apply formatting rules to cells based on specific conditions .
These conditions can be based on the cell's value, text, or even a formula . By using conditional formatting, users can visually highlight important data, identify trends , and make their spreadsheets more visually appealing and easier to understand.
For example, let's say you have a sales report in Google Sheets and you want to highlight all the cells that have sales numbers above a certain threshold . With conditional formatting, you can set a rule that applies a different background color to those cells automatically. This makes it easier to quickly identify the high-performing sales figures without manually scanning through the entire spreadsheet.
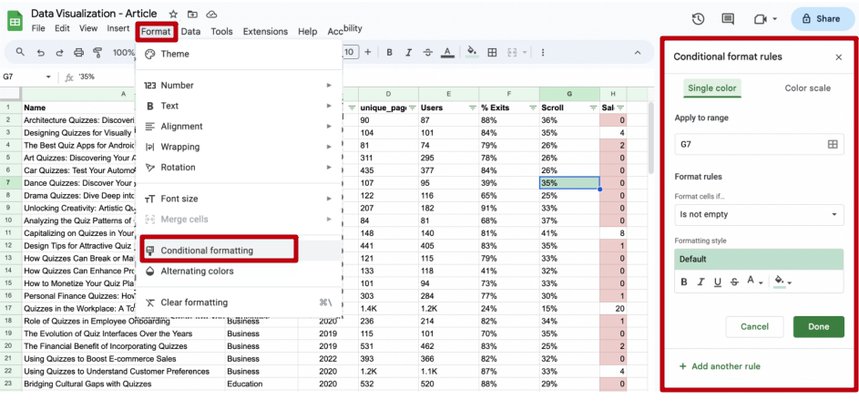
Pivot Tables
Perhaps the main advantage of Google Sheets as your go-to reporting tool is pivot tables.
Pivots allow users to summarize and analyze significant amounts of data. They are used to transform raw flat table data or data sets into insights by organizing and summarizing it in an easy and structured relatively small table.
With Pivot tables you can quickly explore data from different perspectives, change columns and rows, sort values, identify patterns , and uncover trends or anomalies . They are particularly useful for data aggregation tasks.
For example , let's say you have a spreadsheet with sales data for a company. The data includes columns for product names, sales dates, sales quantities, and sales amounts . By creating a pivot table, you can easily summarize this data to answer questions like: ' What are the total sales amounts for each product? ' or ' What are the average sales quantities by month? '
Pivot tables allow you to group and aggregate data based on different criteria , such as product , sales date , or any other relevant attribute.
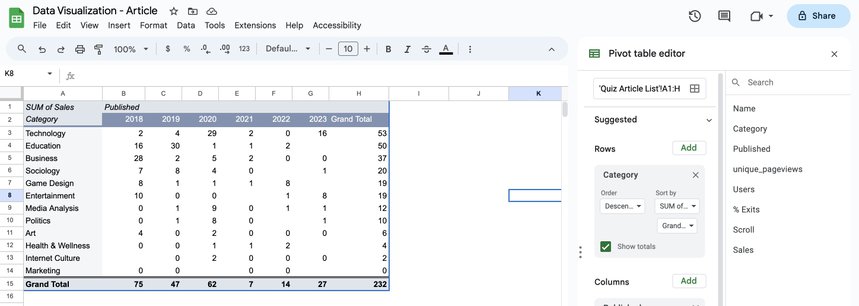
VLOOKUP formula
VLOOKUP is a function in Google Sheets that stands for vertical lookup . It is used to search for a specific value in the leftmost column of a range of cells, and then return a corresponding value from a different column in the same row . Vlookup is commonly used to find and retrieve data from large datasets or tables.
Imagine having a list of products and their corresponding prices. You can use VLOOKUP to search for a specific product name in the leftmost column, and then retrieve the price of that product from a different column in the same row.
This can be useful for tasks such as pricing analysis.
The syntax of the VLOOKUP function in Google Sheets is as follows: =VLOOKUP(search_key, range, index, is_sorted).
The search_key is the value you want to search for, the range is the range of cells where the search will be performed, the index is the column number from which the corresponding value should be returned, and the is_sorted is an optional parameter that specifies whether the range is sorted in ascending order or not.
Everything about VLOOKUP in Google Sheets
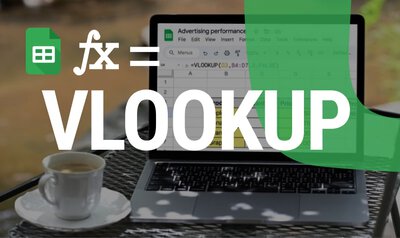
BigQuery <> Google Sheets
If you want to get data from BigQuery to visualized in Google Sheets, there is a Google sheets extension that allows you to query data directly from Google BigQuery and build reports based on the imported data.
Basically, you can request any data stored in your Google BigQuery project directly from the Sheets interface.
Simplify BigQuery Reporting in Sheets
Easily analyze corporate data directly into Google Sheets. Query, run, and automatically update reports aligned with your business needs
Cohort Analysys
Last but not least, we wanted to share with you one of our favorite reports for Google Sheets — the cohort analysis report .
Cohort analysis is a powerful analytical technique used to understand the behavior and common details of a specific group of individuals over time.
It involves dividing a larger audience into smaller groups, or cohorts , based on a common characteristic or event. These cohorts are then analyzed to identify patterns and trends that can help businesses make informed decisions and improve their strategies.
The most common case of cohort analysis in marketing is to track the behavior of customers who made their first purchase in a particular month . By analyzing this cohort, businesses can determine the retention rate, average purchase value, and lifetime value of these customers. This information can be valuable in identifying the most effective marketing channels, optimizing customer acquisition strategies, and improving customer loyalty.
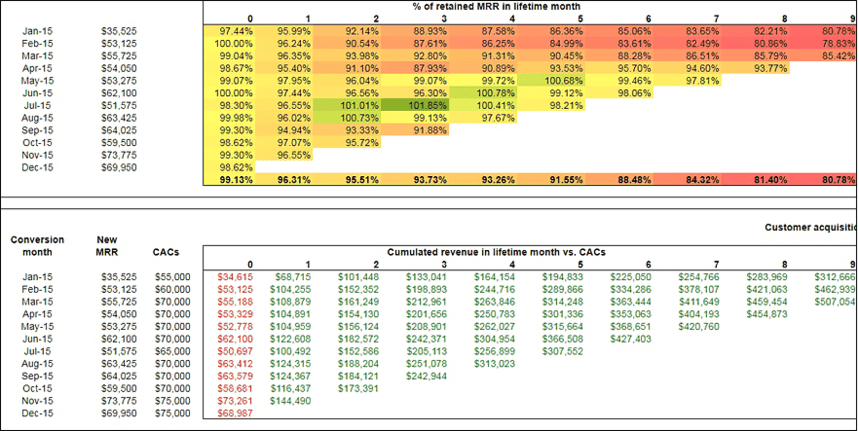
Additionally, you can read our detailed guide to cohort analysis in Google Analytics 4 and Google Sheets, where we provide very detailed instructions. We’ve also hosted a webinar on cohort analysis .
Finally, we want to share some useful links and books on data visualization:
- Edward Tufte, The Visual Display of Quantitative Information
- Stephen Few, Big Data, Big Dupe
- «The Joy of Stats» (documentary film)
Visualization services can help you make your reports visually appealing and comprehensive, you can highlight valuable insights in your data easily.
If you want to keep up with the pace of modern business, adding visual storytelling and data exploration to your reports will allow you to accelerate the process of decision-making.
If you still don’t know which of all data visualization tools would fit your business needs, book a free demo to discuss your specific situation with our data experts and discover the ideal solution designed for you.
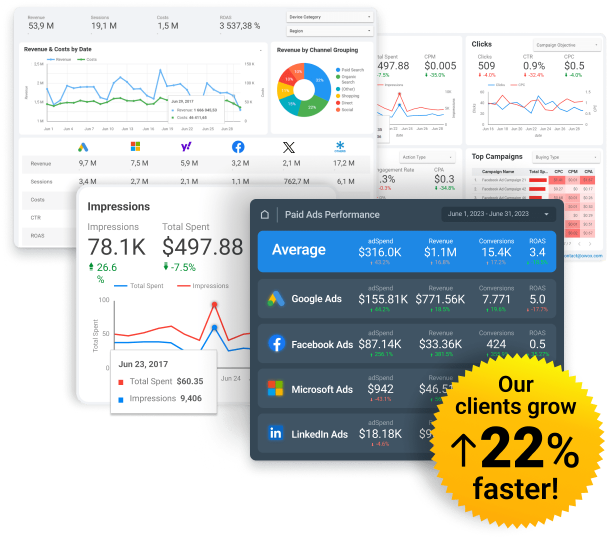
Gain clarity for better decisions without chaos
No switching between platforms. Get the reports you need to focus on campaign optimization
How can I create effective data visualizations?
What are some popular data visualization tools, what are the benefits of data visualization.
Don't forget to share post!
You might also like
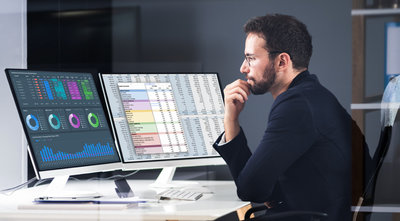
The Role of Business Dashboards: Real-life Examples for Practical Use
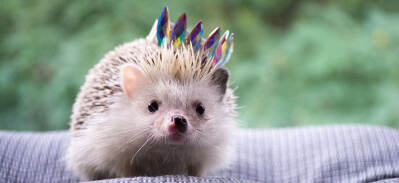
Google Data Studio Templates for Marketers: The Ultimate Guide
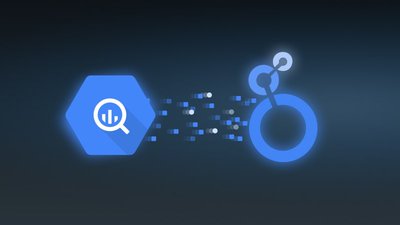
BigQuery to Looker Studio: A New Approach to Dynamic Visualization and Report Automation
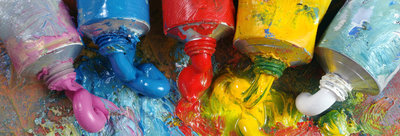
30 Best Marketing Dashboard Examples and Templates [2023]
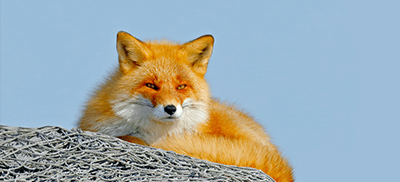
Now You See Me! What is CPA Fraud and How to Fight it
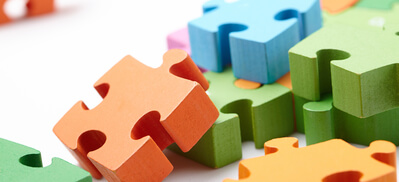
Top 5 Tools for Advertising Reporting
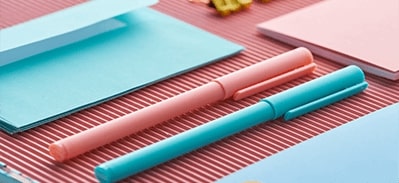
Dashboards for businesses: Why you need one and top services for building it
2,000 companies rely on us
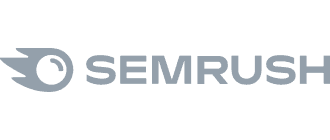
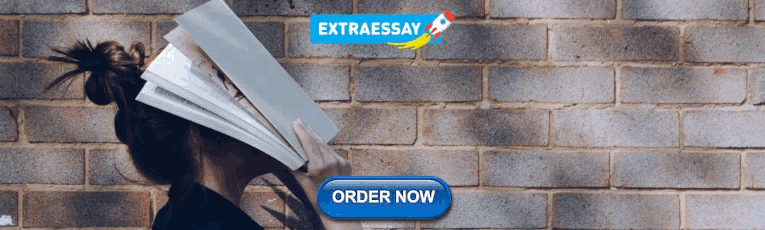
COMMENTS
Data visualization is the graphical representation of information and data. Using visual elements like charts, graphs, and maps, data visualization tools provide an accessible way to see and understand trends, outliers, and patterns in data.
View PDF HTML (experimental) Abstract: Do neural network models of vision learn brain-aligned representations because they share architectural constraints and task objectives with biological vision or because they learn universal features of natural image processing? We characterized the universality of hundreds of thousands of representational dimensions from visual neural networks with ...
eLife assessment. This useful study reports a reanalysis of one experiment of a previously published report to characterize the dynamics of neural population codes during visual working memory in the presence of distracting information. The evidence supporting the claims of dynamic codes is incomplete, as only a subset of the original data is analyzed, there is only modest evidence for dynamic ...
Data visualisation is the graphical representation of information and data. By using visual elements like charts, graphs and maps, data visualisation tools provide an accessible way to see and understand trends, outliers and patterns in data. In the world of big data, data visualisation tools and technologies are essential for analysing massive ...
Data visualization is the graphical representation of information and data using visual elements like charts, graphs, and maps. Learn why data visualization is important, how it helps to make data more accessible and understandable, and what tools and software are available to create and share data visualizations.
Data visualization is the representation of data through use of common graphics, such as charts, plots, infographics and even animations. These visual displays of information communicate complex data relationships and data-driven insights in a way that is easy to understand.
Data visualization is the representation of information and data using charts, graphs, maps, and other visual tools. These visualizations allow us to easily understand any patterns, trends, or outliers in a data set. Data visualization also presents data to the general public or specific audiences without technical knowledge in an accessible ...
Some data visualization tools, however, allow you to add interactivity to your map so the exact values are accessible. 15. Word Cloud. A word cloud, or tag cloud, is a visual representation of text data in which the size of the word is proportional to its frequency. The more often a specific word appears in a dataset, the larger it appears in ...
Data visualization is the graphical representation of information and data. By using visual elements like charts, graphs, and maps, data visualization tools provide an accessible way to see and understand trends, outliers, and patterns in data. This practice is crucial in the data science process, as it helps to make data more understandable ...
The main goal of data visualization is that it helps unify and bring teams onto the same page. The human mind is wired to grasp visual information more effortlessly than raw data in spreadsheets or detailed reports. Thus, graphical representation of voluminous and intricate data is more user-friendly.
The Power of Good Data Visualization. Data visualization involves the use of graphical representations of data, such as graphs, charts, and maps. Compared to descriptive statistics or tables, visuals provide a more effective way to analyze data, including identifying patterns, distributions, and correlations and spotting outliers in complex ...
Data visualization is the visual presentation of data or information. The goal of data visualization is to communicate data or information clearly and effectively to readers. Typically, data is visualized in the form of a chart, infographic, diagram or map. The field of data visualization combines both art and data science.
Data Visualization is a graphic representation of data that aims to communicate numerous heavy data in an efficient way that is easier to grasp and understand. In a way, data visualization is the mapping between the original data and graphic elements that determine how the attributes of these elements vary. The visualization is usually made by ...
Data visualization is important because it breaks down complex data and extracts meaningful insights in a more digestible way. Displaying the data in a more engaging way helps audiences make sense of the information with a higher chance of retention. ... A histogram is a visual representation of the distribution of data. The graph itself ...
Data visualization is the process of turning raw data into graphical representations. Visualizations make it easy to communicate trends in data and draw conclusions. When presented with a graph or chart, stakeholders can easily visualize the story the data is telling, rather than try to glean insights from raw data.
A definition. Data visualization is the graphical or visual representation of data. It helps to highlight the most useful insights from a dataset, making it easier to spot trends, patterns, outliers, and correlations. Imagine you're presented with a spreadsheet containing rows and rows of data.
What is Data Visualization? Data visualization is the graphical representation of different pieces of information or data, using visual elements such as charts, graphs, or maps. Data visualization tools provide the ability to see and understand data trends, outliers, and patterns in an easy, intuitive way. Learn more about data visualization.
Information visualization is the process of representing data in a visual and meaningful way so that a user can better understand it. Dashboards and scatter plots are common examples of information visualization. Via its depicting an overview and showing relevant connections, information visualization allows users to draw insights from abstract ...
Despite these changes, the demand for visual representations of data and results remains high, as exemplified by graphical abstracts, overview figures, and infographics. Similarly, we now operate with more software than ever before, creating many choices and opportunities to customize scientific visualizations. However, as the demand for, and ...
Graphical Representation of Data: Graphical Representation of Data," where numbers and facts become lively pictures and colorful diagrams.Instead of staring at boring lists of numbers, we use fun charts, cool graphs, and interesting visuals to understand information better. In this exciting concept of data visualization, we'll learn about different kinds of graphs, charts, and pictures ...
Data visualization is the use of visual representations to display information. This definition might sound modern, but data visualization has been around for centuries. One of the earliest and most obvious examples is maps, which developed out of the need to graphically display geographic data. Since then data visualization has continued to ...
Data visualization helps people analyze data, especially large volumes of data, quickly and efficiently. By providing easy-to-understand visual representations of data, it helps employees make ...
Overview. Data visualization is the visual presentation of data or information. The goal of data visualization is to communicate data or information clearly and effectively to readers. Typically, data is visualized in the form of a chart, infographic, diagram, or map. The field of data visualization combines both art and data science.
v. t. e. Data and information visualization ( data viz/vis or info viz/vis) [ 2] is the practice of designing and creating easy-to-communicate and easy-to-understand graphic or visual representations of a large amount [ 3] of complex quantitative and qualitative data and information with the help of static, dynamic or interactive visual items.
Data visualization may be described as graphically representing data. It is the act of translating data into a visual context, which can be done using charts, plots, animations, infographics, etc. The idea behind it is to make it easier for us (humans) to identify trends, outliers, and patterns in data.
Data visualization is the graphical representation of data using visual elements such as charts, graphs, and maps. It is a way to communicate complex information in a visual and intuitive manner, making it easier for people to understand and analyze the data. By transforming raw data into visual representations, data visualization allows ...