| | , and Additional contact information Michela Piccarozzi: Department of Economics, Engineering, Society and Business Organization, University of ‘Tuscia’ of Viterbo, 01100 Viterbo, Italy Barbara Aquilani: Department of Economics, Engineering, Society and Business Organization, University of ‘Tuscia’ of Viterbo, 01100 Viterbo, Italy , 2018, vol. 10, issue 10, 1-24 Recent developments in production processes and their automation have led to the definition of the Fourth Industrial Revolution, commonly known as “Industry 4.0”. Industry 4.0 is a very broad domain that includes: production processes, efficiency, data management, relationship with consumers, competitiveness, and much more. At the same time, obviously, Industry 4.0 has become a new theme for management scholars and business economics disciplines and a number of contributions covering various issues and aspects have been published. However, a systematic formulation of all these contributions is still lacking in management literature. Therefore, the aim of the paper is to analyze and classify the main contributions published on the topic of Industry 4.0 in management literature, seeking to give it a unique definition, discover the gaps still remaining in literature and outline future avenues of research in this domain. A systematic review of the literature of the major academic and research databases has been used as methodology to achieve the aim of the paper. This work contributes theoretically to the development of literature on Industry 4.0 and from a managerial perspective it could support entrepreneurs in better understanding the implications and fields of application of the Fourth Industrial Revolution as well as the interplay among them. ; ; ; ; ; (search for similar items in EconPapers) (search for similar items in EconPapers) 2018 (15) (external link) (application/pdf) (text/html) This item may be available elsewhere in EconPapers: for items with the same title. BibTeX RIS (EndNote, ProCite, RefMan) HTML/Text for this article Sustainability is currently edited by in Sustainability from Bibliographic data for series maintained by MDPI Indexing Manager ( ). | | You are using an outdated browser. Please upgrade your browser to improve your experience. Industry 4.0 in Management Studies: A Systematic Literature Review- Piccarozzi, Michela 1
- Gatti, Corrado 2
- 1. Università degli Studi della 'Tuscia' di Viterbo
- 2. Sapienza Università di Roma
DescriptionPiccarozzi, M.; Aquilani, B.; Gatti, C. Industry 4.0 in Management Studies: A Systematic Literature Review. Sustainability 2018 , 10 , 3821. doi: 10.3390/su10103821 ( https://doi.org/10.3390/su10103821 ) Abstract: Recent developments in production processes and their automation have led to the definition of the Fourth Industrial Revolution, commonly known as “Industry 4.0”. Industry 4.0 is a very broad domain that includes: production processes, efficiency, data management, relationship with consumers, competitiveness, and much more. At the same time, obviously, Industry 4.0 has become a new theme for management scholars and business economics disciplines and a number of contributions covering various issues and aspects have been published. However, a systematic formulation of all these contributions is still lacking in management literature. Therefore, the aim of the paper is to analyze and classify the main contributions published on the topic of Industry 4.0 in management literature, seeking to give it a unique definition, discover the gaps still remaining in literature and outline future avenues of research in this domain. A systematic review of the literature of the major academic and research databases has been used as methodology to achieve the aim of the paper. This work contributes theoretically to the development of literature on Industry 4.0 and from a managerial perspective it could support entrepreneurs in better understanding the implications and fields of application of the Fourth Industrial Revolution as well as the interplay among them. Paper pubblicato Sustainability_ sustainability-10-03821.pdfFiles (1.0 mb). Name | Size | Download all | | 1.0 MB | Preview Download | This site uses cookies. Find out more on how we use cookies Login to your accountChange password, your password must have 8 characters or more and contain 3 of the following:. - a lower case character,
- an upper case character,
- a special character
Password Changed SuccessfullyYour password has been changed Create a new accountCan't sign in? Forgot your password? Enter your email address below and we will send you the reset instructions If the address matches an existing account you will receive an email with instructions to reset your password Request UsernameCan't sign in? Forgot your username? Enter your email address below and we will send you your username If the address matches an existing account you will receive an email with instructions to retrieve your username 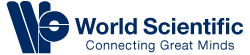 - This Journal
-
- Institutional Access
Cookies NotificationOur site uses javascript to enchance its usability. you can disable your ad blocker or whitelist our website www.worldscientific.com to view the full content., select your blocker:, adblock plus instructions. - Click the AdBlock Plus icon in the extension bar
- Click the blue power button
- Click refresh
Adblock Instructions- Click the AdBlock icon
- Click "Don't run on pages on this site"
uBlock Origin Instructions- Click on the uBlock Origin icon in the extension bar
- Click on the big, blue power button
- Refresh the web page
uBlock Instructions- Click on the uBlock icon in the extension bar
Adguard Instructions- Click on the Adguard icon in the extension bar
- Click on the toggle next to the "Protection on this website" text
Brave Instructions- Click on the orange lion icon to the right of the address bar
- Click the toggle on the top right, shifting from "Up" to "Down
Adremover Instructions- Click on the AdRemover icon in the extension bar
- Click the "Don’t run on pages on this domain" button
- Click "Exclude"
Adblock Genesis Instructions- Click on the Adblock Genesis icon in the extension bar
- Click on the button that says "Whitelist Website"
Super Adblocker Instructions- Click on the Super Adblocker icon in the extension bar
- Click on the "Don’t run on pages on this domain" button
- Click the "Exclude" button on the pop-up
Ultrablock Instructions- Click on the UltraBlock icon in the extension bar
- Click on the "Disable UltraBlock for ‘domain name here’" button
Ad Aware Instructions- Click on the AdAware icon in the extension bar
- Click on the large orange power button
Ghostery Instructions- Click on the Ghostery icon in the extension bar
- Click on the "Trust Site" button
Firefox Tracking Protection Instructions- Click on the shield icon on the left side of the address bar
- Click on the toggle that says "Enhanced Tracking protection is ON for this site"
Duck Duck Go Instructions- Click on the DuckDuckGo icon in the extension bar
- Click on the toggle next to the words "Site Privacy Protection"
Privacy Badger Instructions- Click on the Privacy Badger icon in the extension bar
- Click on the button that says "Disable Privacy Badger for this site"
Disconnect Instructions- Click on the Disconnect icon in the extension bar
- Click the button that says "Whitelist Site"
Opera Instructions- Click on the blue shield icon on the right side of the address bar
- Click the toggle next to "Ads are blocked on this site"
 System Upgrade on Tue, May 28th, 2024 at 2am (EDT)Industry 4.0 maturity and readiness models: a systematic literature review and future framework. Department of Management Studies, Indian Institute of Science, Bangalore, India E-mail Address: [email protected] Search for more papers by this author Over the last five years, Industry 4.0 (I4.0) has gained a lot of attention from industry leaders, policymakers, and government officials worldwide. In an era where new concepts and techniques are introduced continuously, there is a lack of systematic literature review (SLR) on identifying main dimensions, levels, methods to assess the maturity and readiness level toward I4.0. To address this gap, we have chosen our primary objective to provide a critical review of existing literature on dimensions, methods, levels, and current trends to evaluate the I4.0 maturity and readiness models. A Preferred Reporting Items for Systematic Reviews and Meta-Analysis (PRISMA) methodology was adopted to make sure that there is no replication and to maintain complete transparency in the review process. A total of 53 papers were deemed relevant for thematic analysis. From the literature, we have found and proposed 10 main dimensions — Strategy and Organization, Manufacturing and Operations, Supply Chain, Business Model, IT, People, Customers, Product, Services, and Culture, to assess the I4.0 maturity and readiness levels of an organization. Further, a conceptual framework was proposed for the same. This study contributes theoretically to the development of I4.0 maturity and readiness models. So far, this is the first review paper on dimensions of I4.0 maturity and readiness models and is expected to give future researchers and practitioners a holistic guideline to design and develop I4.0 maturity and readiness models. - Literature review
- Industry 4.0
- Industry 4.0 maturity model
- Industry 4.0 readiness model
1. IntroductionCurrently, manufacturing companies worldwide face severe challenges due to environmental, societal, and technological development [ Erol et al. ( 2016 )]. A growing phenomenon and worldwide movement have attracted a lot of attention among policymakers, practitioners, and industrial leaders [ Kagermann et al. ( 2013 ); Xu et al. ( 2018 )]. This movement is called Industry 4.0 (I4.0) or the fourth industrial revolution. This industrial revolution is going to transform the industrial production system with the introduction of new technologies such as autonomous robots, Internet of Things (IoT), cloud computing, big data, simulation, artificial intelligence, additive manufacturing, and virtual reality [ Rüßmann et al. ( 2015 )]. These technologies will increase the connectivity within and outside the organization, thus creating a network of physical systems called as Cyber-physical system [ Xu et al. ( 2018 )]. Again, this revolution has brought the next level of energy efficiency, operational efficiency, and productivity in manufacturing organizations. Hence, all organizational functions are undergoing sea changes in the way it functions, operates, and produces products both internally and externally [ Sony and Naik ( 2019 ); Rajnai and Kocsis ( 2018 )]. Of late, countries across the globe are spearheading new policies, schemes, and agendas to ramify their manufacturing sector in the context of I4.0 [ Liao et al. ( 2017 ); Kusiak ( 2018 )]. However, it is difficult to correctly predict this revolution’s implications on industries, society, and the country’s economy. The concept of “Industry 4.0” came into being in Hannover Fair in the year 2011 when the German government along with industry leaders and practitioners came together to form a “High Tech Strategy Group” to transform the German manufacturing ecosystem [ Rojko ( 2017 ); Schumacher et al. ( 2016 ); Kohler and Weisz ( 2016 ); Alexopoulos et al. ( 2016 ); Qin et al. ( 2016 ); Li ( 2017 )]. In simple terms, the transformation of organizational work processes and practices into digital form is known as I4.0 [ Sony and Naik ( 2019 )]. The main objective behind the initiative of “Industrie 4.0” or “Industry 4.0” was to transform the existing German industry by adopting digital technologies that will empower firms for better connectivity between machines, supply systems, and customers and result in better production output. Besides, the primary goals of I4.0 are to achieve higher levels of automation in the existing operation and increase productivity [ Thames and Schaefer ( 2016 )]. Furthermore, to facilitate and provide step-by-step implementation guidelines, I4.0 maturity and readiness level assessment is a first step toward understanding the status quo of the present status and future desirable levels of an organization [ Schumacher et al. ( 2016 )]. Moreover, manufacturing organizations need to assess their current systems, processes, and practices to move toward the next level of automation, i.e. I4.0. There is a lack of systematically reviewing the state-of-the-art literature on I4.0 maturity and readiness assessment dimensions, methods, levels, contribution, limitations, and current trends in the current context of literature. This paper aims to contribute in the following ways: (a) | To provide a holistic review of existing I4.0 maturity and readiness models. | (b) | To find out the most important dimensions, levels of assessment, methods, the focused field of study, contribution, and limitations of the existing I4.0 maturity and readiness models adopted by researchers and consultancy firms. | (c) | To comparatively analyze existing I4.0 maturity and readiness models in three focused areas-descriptive, prescriptive, and comparative purposes. | (d) | To provide current trends in its country of origin and frequency of publications. | (e) | To provide a comprehensive I4.0 maturity and readiness framework with the identified dimensions for future implementation. |
Hence to address this gap, we have adopted a Preferred Reporting Items for Systematic Reviews and Meta-Analysis (PRISMA) methodology to analyze the existing literature and come up with a holistic framework for industries and researchers on the I4.0 maturity and readiness assessment model. We have found 10 dimensions during our literature review — Strategy and Organization, Manufacturing and Operations, Supply Chain, Business Model, IT, People, Customers, Product, Services, and Culture most widely used by researchers and practitioners. However, 60% of the papers accounted for conceptual paper, while 31% are case studies, 7% are survey-based, and 2% accounted for literature survey study. We have seen the variation of maturity levels from model to model, but on average, five levels were mostly used by many researchers and practitioners. Germany leads with the highest number of publications on I4.0 maturity and readiness models in terms of frequency of publications. Therefore, to provide a clear understanding and meaningful information, this paper is organized as follows. Section 2 provides an overview and detailed background on maturity and readiness models and its importance in the current context, followed by a thorough review of the methodology and its screening process in Sec. 3 . Section 4 illustrates the results obtained from the included papers and their specific data analysis on our objectives. Section 5 provides a detailed discussion and the proposed assessment framework on I4.0 maturity and readiness. Section 6 ends with concluding remarks, limitations, and implications. 2. Literature Review2.1. the concept of industry 4.0 maturity and readiness models. The maturity model’s initial concept was popularized with the Capability Maturity Model’s introduction by the Carnegie Mellon Software Engineering Institute (SEI) in the 1990s [ Mettler (2011) ]. This model was most widely used and well-known among information science researchers and practitioners. Maturity models are generally used for prescriptive, descriptive, and comparative purposes [ Röglinger et al. ( 2012 ); De Bruin et al. ( 2005 )]. It serves as an illustrative purpose when used for as-it-is assessment and serves as a prescriptive purpose when it is used as a step by step to achieve the desired path or identify future desirables or level of granularity. In contrast, the comparative sense is used for internal and external benchmarking of the organization. According to Carnegie Mellon University [2012] , the Maturity model is defined as “A set of characteristics, attributes, indicators and patterns that represent progression and achievement in a particular domain or discipline”. The maturity model concept dates to 1973, wherein Harvard University Professor Richard L. Nolan [ 1973 ] first published stages of growth model for software. After Nolan’s growth model’s initial success, Watts Humphrey further extended to develop a process maturity model at IBM. However, significant development of process maturity took place after 1986 when Watts Humphrey joined SEI Carnegie Mellon University to develop a standard process maturity framework for the Department of Defense, United States of America. In the United States of America, the defense department used the process maturity model developed by SEI to evaluate software contractors. The above framework was based on Crosby’s earlier studies on the quality maturity grid [ Crosby ( 1979 )]. In the early part of the 1990s, due to the emergence of the latest technologies, the process maturity framework was redeveloped to call it a “Capability Maturity Model (CMM)”. The main objectives behind the CMM were to capture and evaluate the software development process, adoption, and practices in an organization [ Paulk et al. ( 2011 )]. Later, CMM was rechristened to call it Capability Maturity Model Integration (CMMI). This model integrates components to address specific areas like system engineering, software engineering, and service delivery. In 1993, the International organization for standardization (ISO) developed and introduced a standard software process improvement and capability determination (SPICE) for the users to support software development, validation, and transition for software process improvement. This SPICE project resulted in the publication of process standard “ISO/IEC 15504” [ Silva et al. (2004) ]. However, due to its complexity and criticality, it was widely criticized by many researchers and practitioners. The inception of I4.0 maturity and readiness started after a formal announcement of “High Tech Strategy” by the German government in 2011. Since then, many researchers from academics and practitioners from industry began to develop and deploy their maturity models for the organization to assess and capture the level of maturity and readiness. The maturity model is a technique or a series of sequential levels that form a logical path from an initial state to a final state of maturity [ Proença and Borbinha (2016) ]. It provides guidelines for the organizations to develop best practices to achieve the desired goals. Earlier studies done by Geissbauer et al. [2016] and PwC [2015] proposed a readiness assessment model focusing on digitalization strategies based on four stages-digital novices, vertical integration, horizontal collaborator, and digital champion. To assess the readiness of manufacturing organization, they have identified seven key dimensions, namely (1) digital business models, (2) digitalization of products and services, (3) integration of vertical and horizontal value chains, (4) data and analytics, (5) agile IT infrastructure, (6) compliance security-legal and tax, and (7) organization-employee culture. Rockwell Automation [2014] introduced a “Connected Enterprise Maturity Model” with four dimensions — information infrastructure, hardware and software, control, and devices like sensors, actuators, and security. In the above model, their focus was on information technology as a primary enabler and five-stage action plans for organizations to assess an organization’s maturity. The five-stage action plan focuses on evaluating existing IT networks, security, network controls, organized network data capital, real-time data analytics, and collaboration. However, their focus was on SME’s maturity assessment. VDMA (Mechanical Engineering Industry Association of Germany) and its partners have developed a company-specific maturity model to assess the German small-scale, medium enterprises (SMEs) following five different stages. This study adopted a step-by-step process for firms’ vision toward I4.0 from product and process point of view. However, the research was done among senior managers, cross-disciplinary teams, and used a toolbox based on product and process to assess the company maturity and readiness. The procedure followed five stages, namely (1) Preparation-knowledge base, (2) Analysis-competencies and internal projects, (3) Creativity, (4) Evaluation, and (5) Implementation. Qin et al. [2016] developed a roadmap for organizations in the transition toward I4.0. However, the above roadmap was not clear as the journey toward I4.0 is not only confined toward roadmap without long-term implementation plans in place. The above roadmap covers five levels of maturity toward building new capabilities in a manufacturing organization. Level 1 (L1) looks at single station automated cells, Level 2 (L2) looks at automated assembly system, Level 3 (L3) looks at computer-integrated manufacturing, and Level 5 (L5) looks at reconfigurable manufacturing system. The study mostly looks at systems and process gaps by adopting smart automation practices toward I4.0. Schumacher et al. [2016] developed a maturity index adapted to measure German SMEs’ readiness levels in terms of digital and smart manufacturing practices. The maturity index is composed of nine key dimensions concerning the organization. They are the organization’s strategy, leadership, products, customers, operations, governance, people, and technology. However, the above model was specially designed for SMEs in the German perspective. Adopting I4.0 technologies within the organization depends on organizational leadership, employee’s readiness to change, skill sets, ability to adapt to the changing culture, and understanding of the technologies surrounding business standards and the respective country’s laws. Kannan et al. [2017] developed a model-based requirement engineering (gap analysis) focusing on standardization in the automotive sector. However, the prerequisite for the I4.0 assessment is missing and does not clearly define the guidelines for the same. Jung et al. [2016] developed a four-dimension manufacturing readiness index to measure organizational readiness toward I4.0. The dimensions considered for assessing the organization are (1) Organization, (2) Information Technology, (3) Performance management, and (4) Information connectivity. However, the above study was mainly focused on maturity for multi-national enterprises (MNEs), and steps to achieve the next level of maturity was missing and was not empirically tested either. Ganzarain and Errasti [2016] introduced the three-stage maturity model leading toward I4.0, considering diversification as a primary enabler in the process. The tools are considered three levels, namely initial, managed, and defined. However, further details regarding assessment steps were not discussed, and it was not empirically tested either. Lee et al. [2017] developed a conceptual model known as the “Analytic Network Process”, considering multi-criteria or building blocks for the Korean manufacturing industry considering five-level. This five-level maturity model devised a path to achieve I4.0 by categorizing industries based on the checking, monitoring, control, optimization, and autonomy. However, readiness to move toward I4.0 was missing from the model. Schuh et al. [2017] under Acatech, designed a conceptual “Industry 4.0 Maturity Index,” taking six levels of assessment to measure the maturity of a manufacturing organization. The six-maturity model consists of computerization, connectivity, visibility, transparency, predictive capacity, and adaptability. Besides, it mentions digitalization as a strategic blueprint with a case study in a manufacturing organization. Four dimensions of digital maturity were considered for the study. They are resources, information systems, organizational structure, and organizational culture. Digital Maturity Model developed by Gill and VanBoskirk [2016] as part of their benchmark study undertaken by Forrester focused on four main dimensions to determine the digital maturity of an organization. The four dimensions consist of the company’s culture, technology, organization, and insights. In this study, the maturity level was segmented into four stages: skeptics, adopters, collaborators, and differentiators. The maturity score ranged from 0 to 33 as the first level, 34 to 52 as adopters for the second level, 53 to 71 as collaborators for the third level, and top maturity segment as differentiators from low to high. The skeptics were those who have just begun the digital journey within the organization; secondly, adopters were those investing in skills and infrastructure development within the organization with a particular strategy on prioritizing customer relationships overproduction. Thirdly, collaborators break down the traditional silos by investing in newer technologies for process improvement to create a competitive advantage. Fourthly, the highest level of maturity segment-differentiators where digital and physical are connected to give a data-driven customer obsession with strong revenue growth. Their top priority is to generate revenue with real-time data processing capability for customer insights. However, the above study was undertaken as a consultancy project by Forrester. Gökalp et al. [2017] proposed a two-dimensional model based on the existing model of SPICE. The two-dimension model takes care of the capability and aspect dimensions of an organization. The capability dimension consists of six maturity levels from 0 to 5. Accordingly, the maturity levels include 0 = incomplete , 1 = performed , 2 = managed , 3 = established , 4 = predictable , and 5 = optimizing . However, the aspect dimension takes care of asset management, data governance, application management, process transformation, and specific organizational focus areas. De Carolis et al. [2017] put forward a model to assess a manufacturing firm’s digital readiness based on CMMI maturity levels. This model incorporates five manufacturing firm areas, particularly in design and engineering, production management, quality management, maintenance management, and logistics management, to assess an organization’s maturity toward I4.0. This model has classified organizations based on five levels, namely initial, managed, define, integrated, interoperable, and digital-oriented. Akdil et al. [2018] compared four models and instituted the I4.0 maturity model considering three major dimensions — (1) strategy and organization, (2) products and services, and (3) processes. The above model considered four levels of assessment — absence, existence, survival, and maturity. A case study was also undertaken in the retail industry in Turkey to validate the model. Of late, Lin et al. [2020] developed a smart manufacturing readiness using a maturity model to assess Taiwan enterprise by classifying into four categories. Meanwhile, Wagire et al. [2020] developed a maturity model using seven dimensions to assess the auto manufacturing company but lacked its applicability with a limited number of expert’s opinions. Furthermore, Caiado et al. [2020] and Rafael et al. [2020] extended the earlier model to develop a specific maturity assessment tool for manufacturing companies and machine tools in Brazil and Spain. 2.2. Importance of Industry 4.0 maturity and readiness modelsMaturity models are an essential tool to assess an organization’s current capabilities and figure out self-improvement guidelines for the future [ Schumacher et al. ( 2016 )]. It enables internal assessment and benchmark with the external organization to provide continuous improvement in processes and practices [ Rübel et al. ( 2018 )]. Today’s organization evolves continuously and is driven by forever changing factors such as technology, customers, society, the global economy, and various others [ Baker ( 2002 )]. In this scenario, it has become essential for organizations to understand and evaluate their organizational needs, the status of change, and future technological needs to match other market competitors. Companies often face a lack of strategic guidance and failure to assess the I4.0 capabilities [ Akdil et al. (2018) ]. There are three important and fundamental purposes of maturity models [ De Bruin et al. ( 2005 )]. The first important purpose is for prescriptive purposes. The second fundamental purpose is for descriptive purpose, and the third purpose is for a comparative purpose or benchmarking purpose. Apart from the above, maturity models are used to establish a framework for establishing a direction for future improvement following the domain’s prescribed best practices. And it also demonstrates a critical understanding of the organization’s current position compared with the other competitors in the market. It is also critically essential for organizations to establish and understand the current position to benchmark themselves against the best to determine their direction. 3. MethodologyA step-by-step systematic literature review (SLR) method was adopted to ensure no replication and maintain complete transparency in the review process [ Sony and Naik ( 2019 ); Tranfield et al. (2003) ]. We adopted a literature search strategy using the keywords “Industry 4.0 readiness,”; “Industry 4.0 maturity model,”; “assessing Industry 4.0,”; “CPPS readiness,”; “dimensions for Industry 4.0 maturity and readiness”. Figure 1 illustrates the flow chart of various stages of the literature review. To cover a wide range of papers from different databases, a review protocol was followed to reduce bias [ Kitchenham ( 2012 )] and cover many articles, papers, reports, and white papers. We followed the inclusion and exclusion criteria for our search on database selection. We have included papers, articles, and white reports written only in English with a cut off year from 2011 to October 2020 and align with keywords, main themes, topics, abstracts, and content relevance on the I4.0 maturity and readiness assessment model. Meanwhile, we have excluded studies that do not focus on the I4.0 maturity and readiness assessment model and those papers that mention keywords only in abstract and titles. To ensure that relevant literature is not left out, we followed a cross-referencing procedure to find out the literature [ Emodi et al. ( 2017 ); van der Merwe et al. (2018) ]. 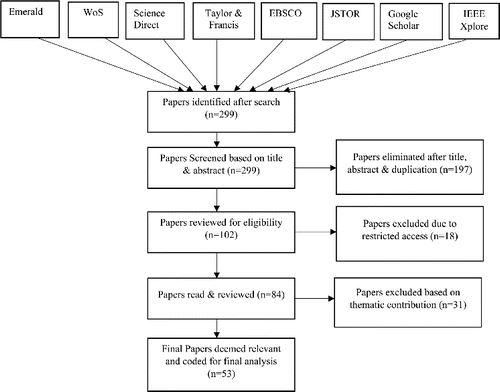 Fig. 1. SLR methodology flow chart. 3.1. Literature search strategy and screeningThe first phase of the literature review involved finalizing the database and keywords. The databases included in this study were EBSCO, Emerald, IEEE Xplore digital library, JSTOR, Google Scholar, Science Direct, Taylor & Francis, and Web of Science. The keywords used for searching the papers, reports, journals, and white papers were as follows: Industry 4.0 Maturity Models, Industry 4.0 Readiness, Cyber physical production system readiness, Dimensions for Industry 4.0 maturity and readiness, Assessing Industry 4.0. We followed the PRISMA to analyze reporting literature review results by critically examining, unearthing gaps, and discussing the most widely used dimensions to assess the maturity and readiness of industry toward I4.0. Table 1 summarizes published academic papers on dimensions of assessing I4.0 maturity and readiness models from 2011 to October 2020. We have identified 53 scholarly published articles and white reports for our analysis. Initially, a total of 292 articles and reports were extracted from database sources and screened for abstract and title relevance. However, a total of 197 articles and reports were eliminated based on the eligibility criteria, relevance, and duplication. Further screening was done to find out the actual relevance in the content of the articles and reports. After the additional screening, 18 papers and reports were excluded for the analysis due to access restrictions on full text and unavailability of the content from the source (mostly consulting firms report). The remaining 83 papers were further reviewed to find out the paper’s contribution to the literature. All those papers that are not providing any contribution were extracted from the final analysis. Finally, 53 articles were accepted and coded for analysis as per the criteria and relevance of the content, title, abstract, and objectives. The literature review process’s coding was a step-by-step process following the inductive method of analysis. Following the inductive method of analysis, the main idea is to bring out raw data to emerge out as dominant and structured without any constraints [ Thomas ( 2006 )]. The summary of maturity and readiness models from 2011 to October 2020 are depicted in Tables 2 – 4 . Initially, a total of 299 articles and reports were extracted from database sources and screened for abstract and title relevance. However, a total of 197 articles and reports were eliminated based on the eligibility criteria, relevance, and duplication. Further screening was done to find out the actual relevance in the content of the articles and reports. After the additional screening, 18 papers and reports were excluded for the analysis due to access restrictions on full text and unavailability of the content from the source. Finally, 53 articles were accepted and coded for analysis as per the criteria and relevance of the content, title, abstract, type of paper, design, methodology, and objectives. The literature review process’s coding was a step-by-step process following the inductive method of analysis. The main idea behind the inductive method of analysis is to bring out raw data to emerge out as dominant and structured without constraints [ Thomas ( 2006 )]. The summary of maturity and readiness models from 2011 to October 2020 are depicted in Tables 3 – 4 , respectively. Country-wise frequency of publications on Industry 4.0 maturity and readiness models.Country | Count | Percentage | Cumulative percentage (%) |
---|
Germany | 13 | 25 | 25 | USA | 7 | 13 | 38 | Switzerland | 3 | 5 | 43 | UK | 3 | 5 | 49 | Canada | 2 | 4 | 53 | Turkey | 2 | 4 | 57 | Brazil | 2 | 4 | 60 | India | 2 | 4 | 64 | Portugal | 2 | 4 | 68 | Denmark | 2 | 4 | 72 | Finland | 2 | 4 | 75 | Italy | 2 | 4 | 79 | Spain | 2 | 4 | 83 | Czech Republic | 2 | 2 | 85 | Ireland | 1 | 2 | 87 | Malaysia | 1 | 2 | 89 | Namibia | 1 | 2 | 91 | South Korea | 1 | 2 | 92 | Taiwan | 1 | 2 | 94 | Sri Lanka | 1 | 2 | 96 | Sweden | 1 | 2 | 98 | Thailand | 1 | 2 | 100 | Summary of Industry 4.0 maturity and readiness dimensions based on published articles in academia. | Summary of Industry 4.0 maturity and readiness models based on consultancy firms. | 2011 | 2014 | 2015 | 2016 | 2017 | 2018 | |
---|
Dimensions | PwC | Rockwell Automation | IBM | McKinsey | PwC | KPMG | Forrester | Accenture | BCG | Deloitte | EY | Capgemini | Total |
---|
Digital input | | | | | | | | | | | | | 1 | Org. strategy | | | | | | | | | | | | | 6 | Leadership | | | | | | | | | | | | | 1 | Infrastructure | | | | | | | | | | | | | 1 | Digital output | | | | | | | | | | | | | 1 | Digital processing | | | | | | | | | | | | | 1 | Business model | | | | | | | | | | | | | 2 | Customers | | | | | | | | | | | | | 7 | People | | | | | | | | | | | | | 2 | Process | | | | | | | | | | | | | 2 | Organizational culture | | | | | | | | | | | | | 6 | Compliance, legal, tax | | | | | | | | | | | | | 3 | Technology | | | | | | | | | | | | | 5 | IT | | | | | | | | | | | | | 1 | Operations | | | | | | | | | | | | | 3 | Asset management | | | | | | | | | | | | | 1 | Value chain integration | | | | | | | | | | | | | 1 | Production | | | | | | | | | | | | | 1 | Marketing | | | | | | | | | | | | | 3 | Sales | | | | | | | | | | | | | 1 | Development and purchasing | | | | | | | | | | | | | 1 | Data driven insights | | | | | | | | | | | | | 2 | Digital enabler | | | | | | | | | | | | | 2 | Product | | | | | | | | | | | | | 2 | Service | | | | | | | | | | | | | 2 | IT architecture | | | | | | | | | | | | | 1 | Digitize the core | | | | | | | | | | | | | 1 | Innovation growth | | | | | | | | | | | | | 1 | Supply chain | | | | | | | | | | | | | 1 | Finance | | | | | | | | | | | | | 1 | Security | | | | | | | | | | | | | 1 | Enterprise enabler | | | | | | | | | | | | | 1 | Governance | | | | | | | | | | | | | 1 | Analytics | | | | | | | | | | | | | 1 | Analysis of existing maturity and readiness models of Industry 4.0.Researcher | Year | Country | Maturity model/title | Levels of maturity | No. of dimensions | Maturity definitions | Assessment method | Sample | Focused field of study | Contribution and limitations |
---|
Kubrick | 2012 | Canada | dStrategy Digital Maturity Model | No | 6 | No | No | Conceptual | General | Descriptive analysis with lack of empirical validation and assessment method | Lichtblau | 2015 | Germany | IMPULS — Industrie 4.0 readiness | 6 | 6 | No | No | Survey — 232 response from employees | Manufacturing firm | Used for prescriptive analysis but lacked on assessment method | Anderl | 2015 | Germany | RAMI4.0 | No | 6 | No | No | Conceptual | General | Study is conceptual in nature with no description on assessment method and empirical validation | Westermann | 2016 | Germany | No details | 5 | | No | No | Conceptual | Mechanical firms | Limited applicability and lack of details | Schumacher | 2016 | Germany | Industry 4.0 readiness and maturity of manufacturing enterprises | No | 9 | Yes | Yes | Case study — 400 employees’ sample | Manufacturing firm | The model is linear and prescriptive but lacked on levels of maturity | Ganzarain and Errasti | 2016 | Spain | Three Stage Maturity Model | 5 | 3 | No | No | Conceptual | SMEs | The study is prescriptive and provides precise dimensions but lacks empirical validation and definition | Leyh | 2016 | Germany | SIMMI 4.0 | 5 | 3 | No | No | Conceptual | IT in manufacturing | The study focuses on technological aspects and lacked empirical validation | Jung | 2016 | USA | SMRL | 10 | 4 | No | Yes | Case study | ICT of manufacturing firm | The study contributes to quantitative analysis but lack the exact maturity assessment method | Weber | 2017 | Germany | M2DDM | 6 | 6 | No | No | Conceptual | Data-driven manufacturing firm | The study focuses on data-driven firm and is conceptual, lacked on the maturity assessment method | Klötzer and Pflaum | 2017 | Germany | No details | 5 | 10 | No | No | Conceptual | Supply chain | It covers a conceptual study on supply chain but lacked on empirical validation and assessment method | Gökalp | 2017 | Turkey | Industry 4.0 MM | 6 | 5 | No | No | Conceptual | Manufacturing firm | Contributes to the concept but no details on the assessment method with empirical validation | Passi | 2017 | Finland | VTT Model of Digi Maturity | 4 | 6 | No | No | Conceptual | General | It contributes to formulation and development of maturity model but lacked on assessment and validation | Schuh | 2017 | Germany | Industry 4.0 Maturity Index | 6 | 4 | No | Yes | Conceptual | Manufacturing firm | Developed index but lacked details on assessment methods and validation | Lee | 2017 | South Korea | Smart Assessment using Analytical Network Process | 5 | 4 | No | Yes | Case study | SMEs | Contributes to SMEs on analytical assessment but lacked an assessment method | De Carolis | 2017 | Italy | DREAMY | 5 | 4 | Yes | Yes | Conceptual | General | The model contributes to conceptual descriptive analysis and lacks empirical validation | Agca | 2017 | UK | Industry 4.0 Readiness assessment tool | 6 | 4 | Yes | Yes | Case study | General | The model provides an assessment of comparative and prescriptive purposes on Industry 4.0 readiness but lacked on considering the importance of dimensions and validation. | Blatz | 2018 | Germany | MM | 3 | 6 | Yes | Yes | Case study | SMEs | The model contributes to maturity assessment but limited to SMEs | Sjödin | 2018 | Sweden | Preliminary Maturity Model | 4 | 3 | No | Yes | Case study | Automobile | The model is linear and specific and lacked on the maturity assessment method | Hamidi | 2018 | Malaysia | SMEs Maturity Model Assessment | 6 | 6 | Yes | Yes | Survey | SMEs | The model contributes to maturity assessment but limited to SMEs | Scremin | 2018 | Canada | MM | 5 | 3 | No | No | Case study | General | The model contributes to earlier literature but lacked an assessment method | Akdil | 2018 | Turkey | Maturity and Readiness Model for Industry 4.0 Strategy | 4 | 3 | Yes | Yes | Case study | Retail | The model focuses on the strategy part of the assessment in the retail sector but lacked on multiple dimensions | Bibby and Dehe | 2018 | UK | Industry 4.0 Assessment | 4 | 3 | No | Yes | Case study | Defense | The model contributes to the existing maturity assessment on technology but lacked on the generalization of the model | Methavitakul and Santiteerakul | 2018 | Thailand | No details | No | 9 | No | No | Conceptual | Supply chain | The model is based on existing literature and does not have any empirical evidence | Canetta | 2018 | Switzerland | No details | No | 5 | No | No | Conceptual | Manufacturing | The study is conceptual and lacked on assessment method and empirical evidence | Rajnai and Kocsis | 2018 | Hungary | MM | 4 | 4 | Yes | No | Case study | General | The model brings in major dimensions but lacked on the maturity assessment method | Akdil | 2018 | Turkey | Industry 4.0 Maturity and Readiness Model | 4 | 3 | Yes | Yes | Case study | Retail | The model is granular with limited dimensions and is focused on retail | Bandara | 2019 | Sri Lanka | MM | 5 | 7 | Yes | Yes | Conceptual | Banking sector | The model contributes to the banking sector but lacked empirical evidence | Colli | 2018 | Denmark | 360 DMA | 6 | 5 | Yes | Yes | Case study | General | The model is linear and contributes to maturity assessment but lacked on the generalization of the model | Sony and Naik | 2019 | Namibia | No details | — | 6 | No | No | Literature review | General | The study contributes to existing literature but lacked empirical validation | Basl and Doucek | 2019 | Czech Republic | Meta Model for Industry 4.0 Readiness | 7 | 6 | No | Yes | Conceptual | General | The model is linear and contributes to readiness assessment but lacked empirical validation | Castelo-Branco | 2019 | Portugal | Industry 4.0 Readiness | No | 2 | No | No | Survey | Manufacturing | The model is linear and contributes to readiness assessment but lacked dimensions and assessment method | Trotta and Garengo | 2019 | Italy | Industry 4.0 Maturity | 5 | 5 | No | No | Conceptual | SMEs | The model is linear and contributes to maturity development on SMEs but lacked on assessment method and empirical validation | Santos and Martinho | 2019 | Brazil | Industry 4.0 Maturity | 6 | 5 | Yes | Yes | Case study | Automobile | The model provides a maturity tool for assessment but lacked on a sample size | Pacchini | 2019 | Brazil | Degree of Readiness | 6 | 1 | Yes | Yes | Case study | Automobile | The model focuses on readiness assessment with eight key technologies but lacks in dimensions | Lin | 2020 | Taiwan | Industry 4.0 Readiness | 6 | 3 | Yes | Yes | Case study | Manufacturing | The model focuses on readiness index calculation using the clustering technique on three main dimensions — process, technology, and organization. However, the study has limited dimensions | Moura and Kohl | 2020 | Brazil | Maturity assessment | 6 | 6 | Yes | Yes | Case study | Manufacturing | The model contributes to the comparative assessment of German and Brazilian companies but lacked a clear maturity plan for implementation | Caiado | 2020 | Brazil | OSCM4.0 | 5 | 3 | Yes | Yes | Case study | Manufacturing organization | The study adopted a Fuzzy rule-based assessment for operations and supply chain. The study is limited to a single organization and has less sample size | Harmoko | 2020 | Germany | Industry 4.0 Readiness | 5 | 3 | Yes | Yes | Conceptual | SMEs | The model contributes to Industry 4.0 readiness assessment in SMEs and has limited dimensions and focuses more on data-driven assessment | Wagire | 2020 | India | Maturity Model | 4 | 7 | Yes | Yes | Case study | Auto-component manufacturing company | The study contributes to the maturity assessment tool but lacked sample size and its applicability in other organizations | Hajoary and Akhilesh | 2020 | India | Industry 4.0 Maturity and Readiness | — | 8 | Yes | Yes | Conceptual | — | Contributes to conceptual theory building and lacked on empirical validation and levels of assessment | Rafael | 2020 | Spain | Industry 4.0 maturity | 6 | 6 | Yes | Yes | Case study | Machine tools | Contributes to the existing literature but lacked on wider applicability in other organizations | 4. Descriptive Analysis and ResultsThis literature review research’s primary goal was to critically review the existing literature on dimensions to assess the maturity and readiness of a manufacturing organization toward I4.0. We have identified dimensions, country of origin, assessment levels, methods, focus areas, and remarks and its drawbacks of the existing maturity and readiness models. Figure 2 shows the country-wise distribution of published articles on I4.0 maturity and readiness. Germany has taken the lead by producing most articles (25%) on I4.0 maturity and readiness models. Hence, it is evident from Table 1 that the concept got early attention in Germany and then to other European countries. Other counties like the USA (13%), Switzerland (5%), and United Kingdom (5%) are catching up on published articles on I4.0 maturity and readiness dimensions from 2011 to October 2020. Since the concept is at an early stage of full-blown implementation, countries worldwide are leapfrogging gradually to implement I4.0 implementation policies. 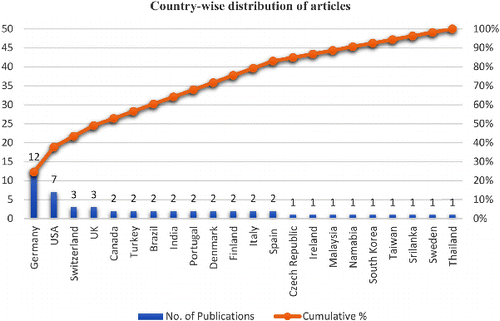 Fig. 2. Country-wise publications of Industry 4.0 maturity and readiness models. 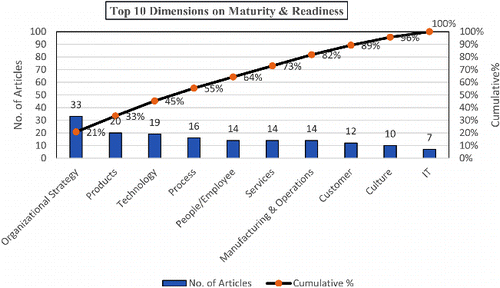 Fig. 3. Pareto chart showing top 10 dimensions on maturity and readiness models. Tables 2 and 3 critically find out and list down the dimensions of I4.0 maturity and readiness models concerning a manufacturing organization. We found out 56 dimensions from various published articles in academia. We have noticed organizational strategy (33) as the most important dimensions to assess the I4.0 maturity and readiness level of an organization, followed by-product (20), technology (19), and process (16), and people capability (14). This undermines the need to assess the organizational strategy, process, and people to understand the current and future skill requirements in I4.0. We have arrived at the top 10 dimensions by summing up the list of dimensions and ranking them from highest to lowest. Table 3 provides a summary of I4.0 maturity and readiness developed by consultancy firms. There are 34 dimensions of I4.0 maturity and readiness models identified from consultancy firms. Likewise, the organizational strategy was the most important dimension repeated six times, along with the organizational culture. About 33 papers referred organizational strategy as main dimensions to evaluate the I4.0 maturity and readiness of an organization followed by-products, people, process, technology, services, operations, IT, customer, and culture. The analysis presented in Tables 4 and 5 illustrates and uncovers the state-of-the-art review of currently existing I4.0 maturity and readiness models. It provides a detailed understanding of its characteristics, focus areas, levels, scope, definition, and draws contributions and limitations for the same. To classify the above models, we have categorized them into three categories as per the purpose of the maturity and readiness models-descriptive, prescriptive, and comparative (Table 6 ). Descriptive maturity models are usually focused on understanding and evaluating the present status i.e. as-it-is, and do not indicate suggestions and recommendations for future improvement [ De Bruin et al. ( 2005 ); Becker et al. ( 2009 )]. Generally, maturity and readiness models are used as a diagnostic tool that directly helps organizations to identify an internal process for improvement [ Maier et al. ( 2009 )]. Analysis of existing maturity and readiness model by consultancy firms.Company | Year | Country | Maturity model/title | Levels of maturity | No. of dimensions | Maturity definitions | Assessment method | Sample | Focused field of study | Contribution and limitations |
---|
Booz and Company | 2011 | Netherlands | Measuring Industry Digitization | No | 4 | No | No | Conceptual | General | The model focuses on digital aspects of organization but lacked on levels of maturity and assessment method along with empirical validation | Rockwell Automation | 2014 | USA | The connected Enterprise Maturity Model | 5 | 6 | No | No | Conceptual | IT capability on manufacturing firm | The study contributes to existing literature on maturity development but lacked on assessment method and empirical validation | IBM | 2014 | USA | Big Data and Analytics Maturity Model | 5 | 8 | No | No | Conceptual | General | The study focuses on big data analytics but lacked maturity assessment method and empirical validation | McKinsey | 2015 | USA | DQ | No | 6 | No | No | Conceptual | General | The study contributes to digital maturity model but lacked on empirical evidence | Neuland | 2015 | India | No details | — | — | — | — | — | — | Restricted assess | Strategy and Transformation Consulting | 2015 | Germany | Digital Maturity Assessment | — | — | — | — | Conceptual | General | The study focuses on prescriptive purpose but lacked on dimensions, assessment method and empirical validation | PwC | 2015 | Germany | Industry 4.0/Digital Operations Self-Assessment | 5 | 6 | No | No | Conceptual | General | Restricted Access | Forrester | 2016 | UK | The Digital Maturity Model 4.0 | 4 | 4 | No | No | Conceptual | General | The study develops a digital maturity model with limited dimensions. The model lacked assessment method and empirical validation | KPMG | 2016 | Switzerland | Digital Readiness Assessment | No | 4 | No | No | Conceptual | General | The study is conceptual and focuses on digital readiness but lacked on assessment method and empirical validation | Accenture | 2017 | Ireland | — | — | — | — | — | Conceptual | General | Restricted access | BCG | 2018 | USA | Digital Acceleration Index | 4 | 4 | No | No | Conceptual | General | Developed a digital readiness index with main dimensions but lacked on empirical validation and assessment method | Deloitte | 2018 | USA | Digital Maturity Model | No | 5 | No | No | Conceptual | General | The model contributes to digital maturity model development but lacked on empirical validation and assessment method | EY | 2018 | UK | Digital Maturity Check | — | — | — | — | Conceptual | General | The model is a conceptual digital checker tool and lacks on rigorous empirical validation | Capgemini | 2018 | Finland | Industry 4.0 Maturity Model | 4 | No | No | No | Conceptual | General | The study contributes to the existing Industry 4.0 maturity model with four main dimensions, but the model lacked on assessment method and empirical validation | Industry 4.0 maturity and readiness model classification.Model name | Descriptive | Prescriptive | Comparative |
---|
dStrategy Digital Maturity Model | Yes | | | IMPULS — Industrie 4.0 readiness | Yes | Yes | | RAMI4.0 | Yes | | | Industry 4.0 readiness and maturity of manufacturing enterprise | Yes | Yes | | DMM | Yes | | | Three Stage Maturity Model | | Yes | | SMRL | Yes | | | M2DDM | Yes | | | Industry 4.0 MM | Yes | | | VTT Model of Digimaturity | Yes | | | Industry 4.0 Maturity Index | Yes | | | Smart Assessment using Analytical Network Process | Yes | | | DREAMY | Yes | | | Industry 4.0 Readiness assessment tool | | Yes | Yes | MM | Yes | | | Preliminary Maturity Model | Yes | | | SMEs Maturity Model Assessment | Yes | | | Maturity and Readiness Model for Industry 4.0 Strategy | Yes | | | Industry 4.0 Assessment | Yes | | | Industry 4.0 Maturity and Readiness Model | Yes | Yes | | 360 DMA | Yes | | | Digital Maturity | Yes | | | Meta Model for Industry 4.0 Readiness | Yes | Yes | | Industry 4.0 Readiness | Yes | | | Industry 4.0 Maturity | Yes | Yes | | Measuring Industry Digitization | Yes | | | The connected Enterprise Maturity Model | Yes | Yes | | Big Data and Analytics Maturity Model | Yes | Yes | | Digital Maturity Assessment | Yes | | | Industry 4.0/Digital Operations Self-Assessment | Yes | Yes | | The Digital Maturity Model 4.0 | Yes | | | Digital Readiness Assessment | Yes | Yes | | Digital Acceleration Index | Yes | | | Digital Maturity Model | Yes | | | Digital Maturity Check | Yes | | | Industry 4.0 Maturity Model | Yes | Yes | | On the other hand, prescriptive maturity and readiness models help us to identify the present status or current levels and thus provide a complete guideline or step-by-step course of action for future improvement. Moreover, in comparative purpose, a maturity and readiness model will help the organizations assess and benchmark the internal and external organizations within similar business units [ De Bruin et al. ( 2005 ); Maier et al. ( 2009 )]. In this analysis, we have considered only those models that have a name and detailed purpose. Table 6 clearly brings out the main purposes of the existing maturity and readiness models toward I4.0. Most of the earlier published models are descriptive and prescriptive. In contrast, there is a lack of a comparative model used to benchmark with the other organizations. We have noticed only one model, the “Industry 4.0 readiness assessment tool”, developed by Agca et al. [2017] that concentrates on the comparative as the maturity model’s prescriptive purpose. Typically, I4.0 maturity and readiness models have been structured into five levels or stages (32%), while some models are structured into four and six levels (23%, 15%) and rest about 30%. To date, none of them have developed a well-structured and widely accepted framework for assessing the maturity and readiness level of I4.0. Hence the need for a well-structured and standard maturity model assessment remains valid. Of late, we have seen the rapid growth in the development of new models on the I4.0 maturity and readiness model relevant to the current context. VDMA developed the “IMPULS-Industry 4.0 readiness” model as the earliest model in Germany to assess a manufacturing organization’s readiness level toward I4.0. The above model was used for descriptive and prescriptive purposes. Schumacher et al. [2016] developed “Industry 4.0 readiness and maturity of manufacturing enterprise”, which is one such model that concentrates on assessing both the maturity and readiness level of a manufacturing organization. While most of the earlier models focused on assessing the maturity used a descriptive approach to evaluate the present status. 4.1. Number of publications by yearFrom the year 2011 to 2020, the number of academic publications has drastically increased. At the same time, I4.0 maturity and readiness model publications were nil in the year 2013. However, research articles and publications improved exponentially in 2017 (16%) and 2018 (25%) (Table 7 ). It is likely to increase further in the coming years, with 16% already published in 2019 and 11% in 2020. These show the rate of increase in publications from both academic and industry-related reports on I4.0 maturity and readiness models. Such growth is due to the widespread adoption and awareness of I4.0 benefits and future objectives by many industry leaders and policymakers. Figure 4 displays the yearly percentage of publications on I4.0 maturity and readiness models. It is evident from Fig. 4 that the concept of I4.0 maturity and readiness assessment is at a nascent stage and is growing year after year. 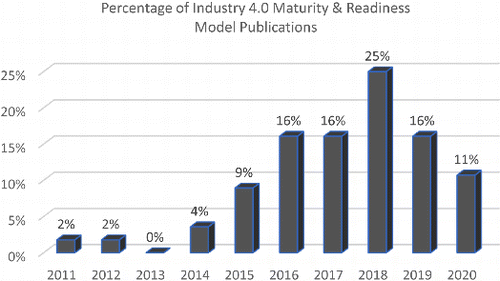 Fig. 4. Yearly percentage of publications on Industry 4.0 maturity and readiness models. Yearly percentage of publications on Industry 4.0 maturity and readiness models.Year | 2011 | 2012 | 2013 | 2014 | 2015 | 2016 | 2017 | 2018 | 2019 | 2020 |
---|
Count | 1 | 1 | 0 | 2 | 5 | 9 | 9 | 14 | 9 | 6 | Percentage | 2% | 2% | 0% | 4% | 9% | 16% | 16% | 25% | 16% | 11% | 4.2. Distribution of publicationsFigure 5 depicts the percentage of 53 published data types from the literature. Most of the articles and reports are conceptual. The total articles and reports accounted for 60% that are conceptual, which reflects that the concept of maturity and readiness is gaining momentum across various principles of I4.0. Meanwhile, the case study accounted for 31%, while 7% of the total accounted for survey-based research, and 2% accounted for a literature review paper. The bar diagram (Fig. 5 ) results are a combination of research articles from academia and white reports from consultancy firms. The total articles from academia have 34 publications. Besides, we have also found 14 consultancy firms reports that concentrated on I4.0 maturity and readiness assessment. However, two reports were excluded from the analysis since it does not contain the necessary criteria for my study. 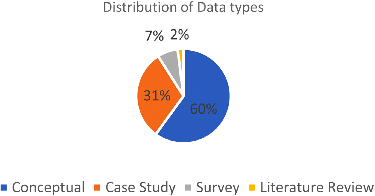 Fig. 5. Distribution of data types. 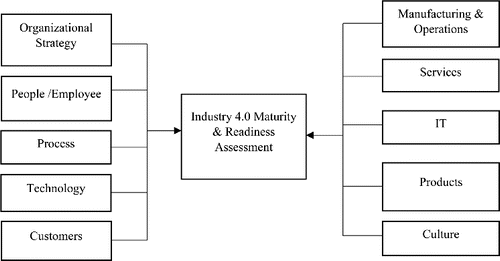 Fig. 6. 10 key dimensions to assess Industry 4.0 maturity and readiness. 5. Discussions and Proposed FrameworkThe very definition of “Industry 4.0” lacks a standard and well-accepted definition accepted by practitioners and researchers. The concept is entirely new and fuzzy [ Hofmann and Rüsch (2017) ] and needs to mature to be well received by academics and industry leaders. It is believed that most academicians and industry leaders understand the benefits of transition toward I4.0 [ Morteza ( 2018 )] and are taking steps to move and assess their present status and future-readiness. This paper’s main contribution is to bring out the essential dimensions required to evaluate organizations’ maturity and readiness level toward I4.0 and provide a holistic understanding for future researchers and practitioners on I4.0 maturity and readiness. It also brings out the current trends, assessment methods, definitions, and gaps of published articles from various countries and consultancy firms. Thus, highlighting the research gaps based on the literature, the following are some of this paper’s findings. The database search resulted in the extrapolation of exciting figures on the scholarly publication of I4.0 maturity and readiness models over the years. Firstly, we have noticed exponential growth in academic publications and white reports from consultancy firms. Secondly, we have witnessed the highest number of publications in the year 2018. Thirdly, we noticed zero publications in 2013. Fourthly, it is likely that the publication rate will go up as 2020 data is still incomplete since the research was carried out in the middle of the current year. Fifthly, we have also noticed conceptual paper dominating with 60% of publications. Such a trend highlights that the I4.0 maturity and readiness model is an emerging topic of interest among scholars and practitioners. A total of 53 published articles and white reports from academia and consultancy firms were finally selected for analysis. After the review, we found out 62 dimensions (56 dimensions — academic publications and 6 dimensions — consultancy firms). However, those similar dimensions between academic publications and consultancy firms were considered as one dimension for the analysis. Out of the 62 dimensions (Tables 3 and 4 ), the top 10 dimensions were highlighted for future researchers as an essential dimension to measure and assess the I4.0 maturity and readiness level of an organization. Apart from the above, we have also found those countries leading in research in the areas of I4.0 maturity and readiness models. We found Germany leading with 13 publications since 2011, followed by the USA with 7 publications. There was an exponential growth in the publication of articles and reports in 2018, with 25% of the total publication coming in the same year, whereas it was nil in 2013. These show that researchers and industry leaders understand the benefits of assessing the maturity and readiness levels of I4.0 to close the gap with the existing market competition across the globe and be an innovative organization. Companies usually experience problems and challenges in determining their current state and future developmental stages to incorporate changes in I4.0 implementation [ Erol et al. ( 2016 )]. Hence to facilitate and provide step-by-step implementation guidelines, I4.0 maturity and readiness level assessment is a first step toward understanding the present status and future desirable levels of an organization. Based on the existing models’ literature review and analysis, we categorized models into three categories: descriptive, prescriptive, and comparative. Hence, the I4.0 maturity and readiness assessment model must be designed, keeping in mind the above purposes. Apart from the above, it must provide a step-by-step implementation guideline in the transition toward I4.0. To successfully implement and adopt I4.0 technologies, organizations must have long-term and short-term organizational strategy to reap the benefits of I4.0. The leadership team needs to understand the benefits and challenges of I4.0 implementation and adopt best practices and cultures. It also requires cross-functional teams within the organization to collaborate and work in tandem with each other. Organizations must have relevant tools to measure the performance at a strategic and operational level. Moreover, it is also equally important to invest in new technologies and reskill employees with the latest technological skill sets. I4.0 acceptance will have an impact on the existing employees and their roles and responsibilities. Future researchers must study the amount of impact it will have on employees. We have come up with key dimensions that are essential and can be used to assess the I4.0 maturity and readiness levels of an organization. In this regard, the top 10 dimensions widely used by many scholars and practitioners were considered. All 10 dimensions are vital for the successful implementation of I4.0 in an organization and are interrelated. Production technology has evolved over the years from the craft-based production system to job shop, Flexible Manufacturing System (FMS), and Manufacturing Execution System (MES). Implementation of I4.0 in the supply chain will bring better visibility, shorter delivery time, and transparency by real-time inventory control and monitoring systems. All supply chain networks are integrated into one platform from supplier to the customers. The cyber-physical system will help the organizations monitor remotely from any part of the world and maintain a strong security network and predictive maintenance of the systems’ health. Of late, the difference between products and services is blurring due to the advancement of technologies. At present, products are produced as per the customers’ needs, i.e. customized, and technologies like sensors and RFID are used to track the quality, location, and status. I4.0 provides a wide range of opportunities by providing integrated marketing channels; contemporary business models like “as a service business model”. A lot of data is generated from customers, and this data can be used to predict customers, automate scheduling, real-time tracking of products to enhance the customer touchpoints. Current I4.0 maturity models lacked in identifying the most critical dimensions and standard levels, methods, and company-specific target states. Future researchers need to create a toolbox to assess each department in an organization toward I4.0 maturity and readiness and provide an achievable roadmap, action plans, and strategies. 5.1. Organizational strategyThe success of the implementation of I4.0 in an organization depends on the leadership team’s awareness and ability to undertake long-term and short-term strategic decisions toward the I4.0 roadmap. The Leadership team must understand the value of I4.0 and prioritize and align with the overall business strategy. The organization must map out a digital strategy and evaluate the present status in terms of the degree of strategy implementation. Prioritize and evaluate the future requirements as per the organizational goals by implementing key performance indicator (KPI) tools at both strategic and operational levels within the organization. Establish cross-functional teams within the organization and run pilot projects on I4.0 technology implementation. Adopt digital ecosystem work culture and contemporary business models. Invest in newer technologies by aligning with technology strategy. 5.2. People/employeeEmployees are an important asset to the organization. It is equally essential for the organizations to understand its employees’ current skill sets, roles, and qualifications and reskill them with the latest skill sets as per the I4.0 requirements. Another aspect is concerned with its ability to change the mindset of the employees and willingness to change. Hence, the success of the I4.0 implementation depends on the employees’ ability to adopt digital technologies and adapt to the changing environment. Automation will replace most manual works, and new roles will be created depending on the business needs. Thinking critically, learning and unlearn new skills, and understanding its needs are critical for the employees in the current context. 5.3. ProcessThe traditional manufacturing process was rigid and manual. However, I4.0 has brought a drastic change in the manufacturing process by transforming it into a more agile and automated manufacturing process. Hence organizations need to transform the current processes into more dynamic and agile manufacturing processes. Every business’s success metrics will depend on achieving low cost-efficacy while executing a high return on investment. In this scenario, industries need to invest in automation, adopt I4.0 technologies, and reduce labor costs by implementing robots in the manufacturing process. It is also equally important to assess the manufacturing process’s current capabilities and implement virtualization of work processes by using augmented reality, virtual plant monitoring, predictive maintenance, and exchange of information and data with the help of cloud computing. 5.4. TechnologyManufacturing production technology has evolved over the years from traditional craft-based to job shop, assembly line, just-in-time to flexible manufacturing systems. This evolution has transformed the manufacturing industry over the years. Eight vital enabling technologies will drive manufacturing organization toward I4.0 — (1) IoT, (2) big data, (3) cloud computing, (4) cyber-physical system, (5) autonomous robot, (6) additive manufacturing, (7) augmented reality, and (8) artificial intelligence. 5.5. CustomersA lot of customer data is generated daily from sales and services. Organizations need to use these data by analyzing the use of analytics tools to understand customer behavior. The digitalization of sales and services is equally important for organizations to connect with the customers seamlessly. Real-time information on products and services increases the customer experience and empowers them to make more informed decisions. 5.6. Manufacturing and operationsManufacturing and operations form the base and is regarded as the heart of the vision of I4.0. Every manufacturing enterprise dreams of producing products autonomously in a self-optimizing environment. An IoT enabled manufacturing and operations connects with machine-to-machine to self-predict and optimize using data generated and collected from processes. Hence, the organizations need to assess the current level and future desirable level of automation in terms of digital modeling capability, self-optimizing processes, automatic material handling, decentralization of processes, digital modeling and simulation used in prototyping and product development, predictive maintenance, and artificial intelligence. 5.7. Products and servicesOver the years, slowly, the difference between products and services has been blurring with technology advancement. At present, products and services go hand in hand as products are equipped with smart sensors, RFID- Radio Frequency Identification, GPS- Global Positioning System, embedded systems, etc. to track the status, quality, and location. In the early days, traditional manufacturing organizations produced products with standard mass production without late differentiation capability, but now with the use of advanced technologies, products are customized as per the needs of the customer. Companies use historical data to generate revenue out of it from customers using analytics. Products are transformed into a smart product wherein customers can track the product from any part of the world. In this regard, I4.0 maturity and readiness models help the organization to track hold of the current product and service capabilities, thus providing future roadmaps for implementation. 5.8. Information technologyInformation technology came into existence with the advent of the computers and invention of the world wide web in 1989. This led to the transformation and extensive use of information technology in every manufacturing enterprise. Technologies like enterprise resource planning (ERP), MES, FMS, computer-aided manufacturing (CAM), Numerical Control (NC), etc. are enhancing productivity, operational efficiency and increasing the visibility in the manufacturing process. The use of mobile devices to remotely monitor and control complex processes is reducing the failure rate and reducing cost for the organization. 5.9. CultureI4.0 will have a significant implication in the way the organization functions and organize itself for its work culture. The culture of open innovation and cross-company collaboration will be more relevant in this era. An internal culture such as infrastructure, intellectual property, digital culture, standards, norms, and certifications will be the key to implementing I4.0 culture in the organization. Hence, organizations must adopt and imbibe the digital culture and benchmark itself from other external organizations regarding their current culture and future desirables. 6. Concluding Remarks, Implications, and LimitationsThe first insight that appears clear from this literature review is that the concept of I4.0 maturity and readiness is a new and emerging topic of interest among researchers and practitioners. It appears that the topic involves both design research and information science. As new theories and methods emerge from time to time, it is evident that the concept is gaining momentum among researchers and industry practitioners for assessing the present status and future desirables of the organization. This paper provides several contributions to the existing literature from a theoretical point of view. First, using well-structured and replicable PRISMA methodology, the previous literature review has been updated and extended further by giving more impetus on dimensions, assessment methods, levels, definitions, country of origin, academic publications, consulting firms, and gaps. Secondly, we can conclude that Germany is currently leading in spearheading I4.0 maturity and readiness model development. Meanwhile, we have seen the variation of maturity levels depending on the focus areas and their purposes. Typically, I4.0 maturity and readiness models have been structured into five levels or stages: level 1 as outsider or absence, level 2 as beginner or existence, level 3 as intermediate or survival, level 4 as experience or maturity, level 5 as an expert or top performer. Meanwhile, some authors have named the level based on their understanding; e.g. Pacchini et al. [2019] have called -level 1 as embryonic, level 2 as initial, level 3 as primary, level 4 as intermediate, level 5 as advanced, and level 6 as ready. We have also proposed framework development dimensions for future researchers and practitioners on I4.0 maturity and readiness model development, assessment, and levels. We intend to highlight the best practices and hands-on library for industrial practitioners and academicians in assessing I4.0 maturity and readiness models for an organization by presenting this paper. This paper makes a noble contribution in identifying relevant dimensions that are frequently used, levels, methodology, contributions, and gaps in assessing an organization’s maturity and readiness level toward I4.0. It also brings out the granularity of maturity levels and focuses areas for future researchers. Of late, there has been an enormous interest among researchers and practitioners since its inception of the term I4.0 in the year 2011 in assessing their industry maturity and readiness toward I4.0. The dimensions, levels, assessment methods, country of origin, contribution, and gaps unearth in this study can be used as a holistic direction for future researchers and practitioners in assessing organization in the domain of I4.0 maturity and readiness (refer Tables 4 – 6 ). Moreover, this study demonstrates the importance of SLR ability as an important and efficient form of research in bringing out state-of-the-art research. Updating and bringing out comprehensive reviews on the I4.0 maturity and readiness models will assist and guide future researchers in developing and designing maturity and readiness models in the I4.0 perspective. It was also evident from this study that procedures, levels of assessment, scope, and accuracy of the models were not uniform. Hence it is difficult to propagate and use them in other organizations. Hence, researchers need to design assessment models identifying the standard procedures, levels, and methods for I4.0 maturity and readiness models. This paper also provides attention, particularly in the areas where manufacturing organizations can focus on assessing the present status to move toward the next level of automation, i.e. I4.0 Fig. 3 . In this regard, industry leaders and practitioners can articulate to design, develop, and implement a robust assessment tool to assess the readiness and maturity level of manufacturing organizations toward I4.0. Based on the analysis, we have identified the existing contributions and limitations of maturity and readiness models. About 57% of the relevant papers were published in the last three years, which means that the concept of I4.0 gained wider acceptance, and it is evident that the trend is growing rapidly. Meanwhile, Germany tops the list with 25% of the total publications on I4.0 maturity and readiness models. We have identified 62 dimensions from the literature that are used to assess and design I4.0 maturity and readiness models. Most of the earlier models followed the step-by-step assessment method i.e. linear approach, and most of these models are descriptive, prescriptive, and comparative in nature. Strategy and organizational practices have become the foremost important for organizations to assess the I4.0 maturity and readiness. Out of the major publications, 60% of the papers were conceptual, while 31% were case studies, and 7% were survey-based. In addition, this study is also particularly important for researchers, practitioners, and policymakers in formulating standard policy roadmap and implementation toward I4.0. Researchers can further explore designing and developing a robust assessment tool to measure and assess the readiness and maturity level of organizations toward I4.0 (Fig. 3 ). The top 10 dimensions identified can be used by industry leaders in assessing their organizations at the micro-level and benchmark themselves from external practices toward I4.0. Lastly, this study can be useful to managers who intend to move their organization toward the next level of automation i.e. I4.0. It will also help them formulate strategies and step-by-step implementation plans, approaches, and practices to transition toward I4.0. This paper’s main limitations are database search from eight sources does not qualify or cover all the databases. In this case, some of the scholarly articles might be left out in the process, as multiple terminologies are used for I4.0. Secondly, white reports and company publications keep on changing each year. Hence, it is difficult to find out details or is restricted in most cases. Thirdly, the term I4.0 is not well defined; some countries, industrial practitioners and scholars called it “Smart Manufacturing”, “Factories of Future”, “Industrial Internet of Things,” etc. In this regard, the keywords used might not bring out exclusive articles and publications for the same. Fourthly, this paper does not have an exploratory study to verify its proposed framework. Despite the above limitations, we believe the proposed conceptual framework on dimensions is justified based only on present literature. However, the content might be biased with respect to other maturity models. Hence, in the future, we planned to undertake an exploratory study to verify and validate the proposed framework with the help of a case study in a manufacturing organization. There is a broader scope for future researchers to verify the above model and design models using primary and secondary data on various manufacturing organizations. To be specific, researchers can work on standardization, design, tool kit, index, and strategic roadmap for assessing maturity and readiness toward I4.0. - AIMA, A. and KPMG, A. (2018). Industry 4.0 India Inc. Gearing up for change. Available at http://resources.aima.in/presentations/AIMA-KPMG-industry-4-0-report.pdf [accessed on 12 July 2019]. Google Scholar
- Agca, O., Gibson, J., Godsell, J., Ignatius, J., Wyn Davies, C. and Xu, O. [ 2017 ] An industry 4 readiness assessment tool . International Institute for Product and Service Innovation , 1–19. Google Scholar
- Akdil, K. Y., Ustundag, A., Cevikcan, E. [ 2018 ] Maturity and readiness model for Industry 4.0 strategy . In Industry 4.0: Managing the Digital Transformation . Springer Series in Advanced Manufacturing . Springer, Cham. https://doi.org/10.1007/978-3-319-57870-5_4 Crossref , Google Scholar
- Alexopoulos, K., Makris, S., Xanthakis, V., Sipsas, K. and Chryssolouris, G. [ 2016 ] A concept for context-aware computing in manufacturing: The white goods case . International Journal of Computer Integrated Manufacturing , 29 , 8: 839–849. Crossref , Google Scholar
- BCG (2018). Digital Accelerator Index. Available at https://www.bcg.com/en-in/capabilities/technology-digital/digital-acceleration-index.aspx [accessed on 25 August 2018]. Google Scholar
- Baker, K. A. (2002). Management Benchmark Study, Chapter 14 — Innovation, Office of Planning and Analysis. Available at http://www.au.af.mil/au/awc/awcgate/doe/benchmark, 2002 [accessed on 8 June 2018]. Google Scholar
- Bandara, O., Vidanagamachchi, K. and Wickramarachchi, R. [ 2019 ] A model for assessing maturity of industry 4.0 in the banking sector . In Proceedings of the International Conference on Industrial Engineering and Operations Management , Bangkok, Thailand , March 2019 . Google Scholar
- Basl, J. and Doucek, P. [ 2019 ] A metamodel for evaluating enterprise readiness in the context of industry 4.0 . Information (Switzerland) , 10 , 3: 1–13. https://doi.org/10.3390/info10030089 Google Scholar
- Becker, J., Knackstedt, R. and Pöppelbuß, J. [ 2009 ] Developing maturity models for IT management — A procedure model and its application . Business & Information Systems Engineering , 1 , 3: 213–222. Crossref , Google Scholar
- Bibby, L. and Dehe, B. [ 2018 ] Defining and assessing industry 4.0 maturity levels — case of the defence sector . Production Planning and Control , 29 , 12: 1030–1043. https://doi.org/10.1080/09537287.2018.1503355 Crossref , Google Scholar
- Bitkom, VDMA and ZVEI. (2016). Implementation strategy Industry 4.0. In report on the results of the Industrie 4.0 Platform. Retrieved from https://www.zvei.org/fileadmin/user_upload/Presse_und_Medien/Publikationen/2016/januar/Implementation_Strategy_Industrie_4.0_-_Report_on_the_results_of_Industrie_4.0_Platform/Implementation-Strategy-Industrie-40-ENG.pdf. Google Scholar
- Blatz, F., Bulander, R. and Dietel, M. [ 2018 ] Maturity model of digitization for SMEs . In IEEE International Conference on Engineering, Technology and Innovation, ICE/ITMC 2018 — Proceedings 2004 . https://doi.org/10.1109/ICE.2018.8436251 Crossref , Google Scholar
- Boston Consulting Group. (2018). Digital Acceleration Index. Available at https://www.bcg.com/capabilities/technology-digital/digital-accelerationindex.aspx [accessed 9 June 2019]. Google Scholar
- CGI. (2014). Industry 4.0. Business and Information Systems Engineering , 6 , 4: 239. Available at https://www.cgi.com/sites/default/files/white-papers/manufacturing_industry-4_white-paper.pdf. Google Scholar
- Caiado, R. G. G., Scavarda, L. F., Gavião, L. O., Ivson, P., de Mattos Nascimento, D. L. and Garza-Reyes, J. A. [ 2020 ] A fuzzy rule-based Industry 4.0 maturity model for operations and supply chain management . International Journal of Production Economics , 231 : 107883. Crossref , Google Scholar
- Canetta, L., Barni, A. and Montini, E. [ 2018 ] Development of a digitalization maturity model for the manufacturing sector . In IEEE International Conference on Engineering, Technology and Innovation, ICE/ITMC 2018 — Proceedings , Stuttgart, Germany , pp. 1–7. https://doi.org/10.1109/ICE.2018.8436292 Crossref , Google Scholar
- Carnegie Mellon University (2012). McKinsey Digital: Raising Your Digital Quotient 136. Available at http://www.mckinsey.com/business-functions/digital-mckinsey/how-we-help-clients/December-136. Google Scholar
- Castelo-Branco, I., Cruz-Jesus, F. and Oliveira, T. [ 2019 ] Assessing Industry 4.0 readiness in manufacturing: Evidence for the European Union . Computers in Industry , 107 : 22–32. https://doi.org/10.1016/j.compind.2019.01.007 Crossref , Google Scholar
- Choi, S., Kang, G., Jung, K. and Kulvatunyou, B. [ 2016 ] Applications of the factory design and improvement reference activity model . In IFIP International Conference on Advances in Production Management Systems . Springer, Cham, USA , pp. 697–704. Crossref , Google Scholar
- Colli, M., Madsen, O., Berger, U., Møller, C., Wæhrens, B. V. and Bockholt, M. [ 2018 ] Contextualizing the outcome of a maturity assessment for Industry 4.0 . IFAC-PapersOnLine , 51 , 11: 1347–1352. https://doi.org/10.1016/j.ifacol.2018.08.343 Crossref , Google Scholar
- Crosby, B. Y. P. B. [ 1979 ] How to manage quality so that it becomes a source of business profits. The executive who spends half a may find it one of the most . Quality Is Free , NY: Penguin Books, pp. 21–136. Google Scholar
- De Bruin, T., Rosemann, M., Freeze, R. and Kulkarni, U. [ 2005 ] Understanding the main phases of developing a maturity assessment model . In Australasian Conference on Information Systems (ACIS) , Sydney, Australia . Google Scholar
- De Carolis, A., Macchi, M., Kulvatunyou, B., Brundage, M. P. and Terzi, S. [ 2017 ] Maturity models and tools for enabling smart manufacturing systems: Comparison and reflections for future developments . In Ifip International Conference on Product Lifecycle Management . Springer, Cham, pp. 23–35. Crossref , Google Scholar
- Deloitte (2018). Deloitte Digital Maturity Model. Available at https://www2.deloitte.com/content/dam/Deloitte/global/Documents/Technology-Media-Telecommunications/deloitte-digital-maturity-model.pdf [accessed on 14 November 2018]. Google Scholar
- Emodi, N. V., Murthy, G. P., Emodi, C. C. and Emodi, A. S. A. [ 2017 ] A literature review on the factors influencing patent propensity . International Journal of Innovation and Technology Management , 14 , 3: 1750015. Link , Google Scholar
- Ernst and Young (2018). Digital Readiness Assessment — Does your business strategy work in a digital world?, 2018. Available at https://digitalreadiness.ey.com/[accessed 25 June 2019]. Google Scholar
- Erol, S., Schumacher, A. and Sihn, W. [ 2016 ] Strategic guidance towards Industry 4.0 — A three-stage process model . In Proceedings of International Conference on Competitive Manufacturing , Vol. 9 , No. 1, pp. 495–501. Google Scholar
- Gökalp, E., Şener, U. and Eren, P. E. [ 2017 ] Development of an assessment model for Industry 4.0: Industry 4.0-MM . In International Conference on Software Process Improvement and Capability Determination . Springer, Cham, pp. 128–142. Crossref , Google Scholar
- Ganzarain, J. and Errasti, N. [ 2016 ] Three stage maturity model in SME’s towards Industry 4.0 . Journal of Industrial Engineering and Management , 9 , 5: 1119–1128. https://doi.org/10.3926/jiem.2073 Crossref , Google Scholar
- Geissbauer, R., Vedso, J. and Schrauf, S. [ 2016 ] Industry 4.0: Building the digital enterprise . Retrieved from PwC Website , https://www.pwc.com/gx/en/industries/industries-4.0/landing-page/industry-4.0-building-your-digital-enterprise-april-2016.pdf . Google Scholar
- Gilchrist, A. [ 2016 ] Industry 4.0 . Apress, Berkeley, CA, pp. 161–177. https://doi.org/10.1007/978-1-4842-2047-4 Crossref , Google Scholar
- Gill, M. and VanBoskirk, S. [ 2016 ] The digital maturity model 4.0 . Forrester , Jan 2016 , 1–17. Google Scholar
- Hajoary, P. K. and Akhilesh, K. B. [ 2020 ] Conceptual framework to assess the maturity and readiness towards Industry 4.0 . Industry 4.0 and Advanced Manufacturing , Lecture Notes in Mechanical Engineering , eds. A. ChakrabartiM. Arora . Springer, Singapore. https://doi.org/10.1007/978-981-15-5689-0_2 Google Scholar
- Hamidi, S. R., Aziz, A. A., Shuhidan, S. M., Aziz, A. A. and Mokhsin, M. [ 2018 ] SMEs maturity model assessment of IR4.0 digital transformation . Advances in Intelligent Systems and Computing , 739 : 721–732. https://doi.org/10.1007/978-981-10-8612-0_75 Crossref , Google Scholar
- Harmoko, H. [ 2020 ] Industry 4.0 readiness assessment: Comparison of tools and introduction of new tool for SME . Tehnički Glasnik , 14 , 2: 212–217. Crossref , Google Scholar
- Hofmann, E. and Rüsch, M. [ 2017 ] Industry 4.0 and the current status as well as future prospects on logistics . Computers in industry , 89 , 23–34. Crossref , Google Scholar
- IBM. (2014).Big Data & Analytics Maturity Mode. Available at https://www.ibm.com/developerworks/community/blogs/bigdataanalytics/entry/big_data_analytics_maturity_model?lang=en [accessed on 13 August 2019]. Google Scholar
- Jovanović, M., Mesquida, A.-L., Mas, A. and Lalić, B. [ 2017 ] Towards the development of a sequential framework for agile adoption . In SPICE: International Conference on Software Process Improvement and Capability Determination , pp. 30–42. Springer, Spain, October 2017 . https://doi.org/10.1007/978-3-319-67383-7 Crossref , Google Scholar
- Jung, K., Kulvatunyou, B., Choi, S. and Brundage, M. P. [ 2016 ] An overview of smart manufacturing system readiness assessment . Advances in Production Management Systems. Initiatives for a Sustainable World , eds. I. Nääs et al. Springer, pp. 705–712. https://doi.org/10.1007/978-3-319-51133-7_83 Crossref , Google Scholar
- Kagermann, H., Wahlster, W. and Helbig, J. [ 2013 ] Recommendations for Implementing the Strategic Initiative Industry 4.0 . National Academy of Science and Engineering, Berlin, Germany. Google Scholar
- Kannan, S. M., Suri, K., Cadavid, J., Barosan, I., Van Den Brand, M., Alferez, M. and Gerard, S. (2017). Towards industry 4.0: Gap analysis between current automotive MES and industry standards using model-based requirement engineering. In 2017 IEEE International Conference on Software Architecture Workshops (ICSAW) , pp. 29–35. Google Scholar
- Kitchenham, B. A. [ 2012 ] Systematic review in software engineering — Where we are and where we should be going . In Proceedings of the 2nd International Workshop on Evidential Assessment of Software Technologies , pp. 1–2. Crossref , Google Scholar
- Klötzer, C. and Pflaum, A. [ 2017 ] Toward the development of a maturity model for digitalization within the manufacturing industry supply chain . In Proceedings of the 50th Hawaii International Conference on System Sciences 2017 , Hawaii, USA , Jan 2017 , pp. 4210–4219. https://doi.org/10.24251/hicss.2017.509 Crossref , Google Scholar
- Kohler, D. and Weisz, J.-D. [ 2016 ] Industrie: Les défis de la Transformation Numérique du Modèle Industriel Allemand . La documentation Française, Paris. Google Scholar
- Kubrick, K. (2012). Introducing the Six Dimensions of Digital Maturity, aka the dStrategy Digital Maturity Model TM . Availabe at https://www.onlineauthority.com/blog/introducing-dstrategy-digital-maturity-model/[accessed on 12 August 2018]. Google Scholar
- Kusiak, A. [ 2018 ] Smart manufacturing . International Journal of Production Research , 56 , 1–2: 508–517. Crossref , Google Scholar
- Lee, J., Jun, S., Chang, T. W. and Park, J. [ 2017 ] A smartness assessment framework for smart factories using analytic network process . Sustainability (Switzerland) , 9 , 5: 1–15. https://doi.org/10.3390/su9050794 Google Scholar
- Leyh, C., Schäffer, T., Bley, K. and Forstenhäusler, S. (2016). SIMMI 4.0 — A maturity model for classifying the enterprise-wide IT and software landscape focusing on Industry 4.0. 8 : 1297–1302, https://doi.org/10.15439/2016F478. Google Scholar
- Leyh, C., Bley, K., Schäffer, T. and Bay, L. [ 2017 ] The application of the maturity model SIMMI 4.0 in selected enterprises . Amcis 2017 Proceedings . https://doi.org/10.1007/978-3-319-51133-7_82 , Boston, US . Google Scholar
- Li, D. X., Eric, L. X. and Ling, L. [ 2018 ] Industry 4.0: State of the art and future trends . International Journal of Production Research , 56 , 8: 2941–2962. https://doi.org/10.1080/00207543.2018.1444806 Crossref , Google Scholar
- Li, L. (2017). China’s manufacturing locus in 2025: With a comparison of “Made-in-China 2025” and “Industry 4.0.” Technological Forecasting and Social Change . Online Published, https://doi.org/10.1016/j.techfore.2017.05.028. Google Scholar
- Liao, Y., Deschamps, F., Loures, E. R. and Ramos, L. F. [ 2017 ] Past, present and future of Industry 4.0: A systematic literature review and research agenda proposal . International Journal of Production Research , 55 , 12: 3609–3629. https://doi.org/10.1080/00207543.2017.1308576 Crossref , Google Scholar
- Lichtblau, K., Stich, V., Bertenrath, R., Blum, M., Bleider, M., Millack, A. et al. (2015). IMPULS-Industrie 4.0-Readiness, Impuls-Stiftung des VDMA, Aachen-Köln. Available at http://www.impulsstiftung.de/documents/3581372/4875835/Industrie+4.0+Readniness+IMPULS+Studie+Oktober+2015.pdf/447a6187-9759-4f25-b186-b0f5eac69974 [accessed on 2 June 2018]. Google Scholar
- Lin, T. C., Wang, K. J. and Sheng, M. L. [ 2020 ] To assess smart manufacturing readiness by maturity model: A case study on Taiwan enterprises . International Journal of Computer Integrated Manufacturing , 33 , 1: 102–115. Crossref , Google Scholar
- Machadoa, C. G., Winrotha, M., Carlssonb, D., Almströma, P., Centerholtb, V. and Hallin, M. [ 2019 ] Industry 4.0 readiness in manufacturing companies: Challenges and enablers towards increased digitalization . Way , 1 , 2: 3–4. Google Scholar
- Maier, A. M., Moultrie, J. and Clarkson, P. J. [ 2009 ] Developing maturity grids for assessing organisational capabilities: Practitioner guidance . In Proceedings of the 4th International Conference on Management Consulting, Academy of Management (MCD) , Vienna, Austria . Google Scholar
- Mettler, T. [ 2011 ] Maturity assessment models: A design science research approach . International Journal of Society Systems Science , 3 , 1–2: 81–98. Crossref , Google Scholar
- Methavitakul, B. and Santiteerakul, S. [ 2018 ] Analysis of key dimension and sub-dimension for supply chian of industry to fourth industry performance measurement . In Proceedings of the 2018 IEEE International Conference on Service Operations and Logistics, and Informatics, SOLI 2018 , Singapore , pp. 191–195. https://doi.org/10.1109/SOLI.2018.8476765 Crossref , Google Scholar
- Morteza, G. [ 2018 ] The future of manufacturing industry: A strategic roadmap toward Industry 4.0 . Journal of Manufacturing Technology Management , 29 , 6: 910–936. https://doi.org/10.1108/JMTM-02-2018-0057 Crossref , Google Scholar
- Moura, L. R. and Kohl, H. [ 2020 ] Maturity assessment in Industry 4.0 — A comparative analysis of Brazilian and German companies . Emerging Science Journal , 4 , 5: 365–375. Crossref , Google Scholar
- Nolan, R. L. [ 1973 ] Managing the computer resources: A strategic hyothesis . Communication of the ACM , 16 , 7: 399–405. Crossref , Google Scholar
- Paasi, J. [2017] Towards a new era in manufacturing. Final Report of VTT?S For Industry Spearhead Programme . VTT Technical Research Centre of Finland, Vol. 288 http://www.vtt.fi/inf/pdf/technology/2017/T288.pdf,. pp. 41–46. Google Scholar
- Pacchini, A. P. T., Lucato, W. C., Facchini, F. and Mummolo, G. [ 2019 ] The degree of readiness for the implementation of Industry 4.0 . Computers in Industry , 113 : 103125. Crossref , Google Scholar
- Paulk, M. C., Curtis, B., Chrissis, M. B. and Weber, C. V. [ 2011 ] The capability maturity model for software . Software Process Improvement , IEEE, pp. 49–88. https://doi.org/10.1109/9781118156667.ch2 Google Scholar
- PricewaterhouseCoopers. (2015). Industry 4.0 — Enabling Digital Operations. Available at https://i40-self-assessment.pwc.de/i40/landing/[accessed on 10 May 2018]. Google Scholar
- Proença, D. and Borbinha, J. [ 2016 ] Maturity models for information systems — A state of the art . Procedia Computer Science , 100 , June 2017 : 1042–1049. https://doi.org/10.1016/j.procs.2016.09.279 Crossref , Google Scholar
- Qin, J., Liu, Y. and Grosvenor, R. [ 2016 ] A categorical framework of manufacturing for Industry 4.0 and beyond . Procedia CIRP , 52 : 173–178. https://doi.org/10.1016/j.procir.2016.08.005 Crossref , Google Scholar
- Röglinger, M., Pöppelbuß, J. and Becker, J. [ 2012 ] Maturity models in business process management . Business Process Management Journal , 18 , 2: 328–346. Crossref , Google Scholar
- Rübel, S., Emrich, A., Klein, S. and Loos, P. (2018). A maturity model for business model management in Industry 4.0. In Multikonferenz Wirtschaftsinformatik . Lüneburg, Germany. Google Scholar
- Rüßmann, M., Lorenz, M., Gerbert, P., Waldner, M., Justus, J., Engel, P. and Harnisch, M. [ 2015 ] Industry 4.0: The Future of Productivity and Growth in Manufacturing . Boston Consulting Group. Google Scholar
- Rafael, L. D., Jaione, G. E., Cristina, L. and Ibon, S. L. [ 2020 ] An Industry 4.0 maturity model for machine tool companies . Technological Forecasting and Social Change , 159 : 120203. Crossref , Google Scholar
- Rajnai, Z. and Kocsis, I. [ 2018 ] Assessing industry 4.0 readiness of enterprises . In SAMI 2018 — IEEE 16th World Symposium on Applied Machine Intelligence and Informatics Dedicated to the Memory of Pioneer of Robotics Antal (Tony) K. Bejczy, Proceedings , Kosice, Slovakia , February 2018 , pp. 225–230. https://doi.org/10.1109/SAMI.2018.8324844 Crossref , Google Scholar
- Rockwell Automation (2014). The Connected Enterprise Maturity. Retrieved from https://literature.rockwellautomation.com/idc/groups/literature/documents/wp/cie-wp002_-en-p.pdf. Google Scholar
- Rojko, A. [ 2017 ] Industry 4.0 concept: Background and overview . International Journal of Interactive Mobile Technologies , 11 , 5: 77. https://doi.org/10.3991/ijim.v11i5.7072 Crossref , Google Scholar
- Santos, R. C. and Martinho, J. L. [ 2019 ] An industry 4.0 maturity model proposal . Journal of Manufacturing Technology Management , 31 , 5: 1023–1043. Crossref , Google Scholar
- Schuh, G., Anderl, R., Gausemeier, J. and ten Hompel, W. W. [ 2017 ] Industrie 4.0 maturity index . Managing the Digital Transformation of Companies , Munich: Herbert Utz. https://doi.org/10.1136/bmj.3.5765.46 Google Scholar
- Schumacher, A., Erol, S. and Sihn, W. [ 2016 ] A maturity model for assessing Industry 4.0 readiness and maturity of manufacturing enterprises . Procedia CIRP , 52 : 161–166. https://doi.org/10.1016/j.procir.2016.07.040 Crossref , Google Scholar
- Schweikl, S. and Obermaier, R. [ 2019 ] Lessons from three decades of IT productivity research: Towards a better understanding of IT-induced productivity effects . Management Review Quarterly , 70 : 461–507. https://doi.org/10.1007/s11301-019-00173-6 Crossref , Google Scholar
- Scremin, L., Armellini, F., Brun, A., Solar-Pelletier, L. and Beaudry, C. [ 2018 ] Towards a framework for assessing the maturity of manufacturing companies in Industry 4.0 adoption . In Analyzing the Impacts of Industry 4.0 in Modern Business Environments , IGI Global, pp. 224–254. Crossref , Google Scholar
- Silva, J. G., Rodríguez, M., Costa, D., Loon, H., Rodríguez-Dapena, P., Pederson, K. and Aldea-Montero, F. [ 2004 ] Product assurance of software reuse in the SPICE for space framework . In Proc. of the 4th International SPICE Conference on Process Assessment and Improvement (SPICE 2004) , April, Lisbon, Portugal . Google Scholar
- Sjödin, D. R., Parida, V., Leksell, M. and Petrovic, A. [ 2018 ] Smart factory implementation and process innovation: A preliminary maturity model for leveraging digitalization in manufacturing moving to smart factories presents specific challenges that can be addressed through a structured approach focused on people . Research Technology Management , 61 , 5: 22–31. https://doi.org/10.1080/08956308.2018.1471277 Crossref , Google Scholar
- Sony, M. and Naik, S. [ 2019 ] Key ingredients for evaluating Industry 4.0 readiness for organizations: A literature review . Benchmarking: An International Journal , 27 , 7: 2213–2232. https://doi.org/10.1108/BIJ-09-2018-0284 Crossref , Google Scholar
- Thames, L. and Schaefer, D. [ 2016 ] Software-defined cloud manufacturing for Industry 4.0 . Procedia CIRP , 52 : 12–17. https://doi.org/10.1016/j.procir.2016.07.041 Crossref , Google Scholar
- Thomas, D. R. [ 2006 ] A general inductive approach for analyzing qualitative evaluation data . American Journal of Evaluation , 27 , 2: 237–246. https://doi.org/10.1177/1098214005283748 Crossref , Google Scholar
- Tranfield, D., Denyer, D. and Smart, P. [ 2003 ] Towards a methodology for developing evidence-informed management knowledge by means of systematic review . British Journal of Management , 14 , 3: 207–222. Crossref , Google Scholar
- Trotta, D. and Garengo, P. [ 2019 ] Assessing Industry 4.0 maturity: An essential scale for SMEs . In Proceedings of 2019 8th International Conference on Industrial Technology and Management, ICITM 2019 , pp. 69–74. https://doi.org/10.1109/ICITM.2019.8710716 Crossref , Google Scholar
- van der Merwe, M. D., Grobbelaar, S. S., Schutte, C. S. and von Leipzig, K. H. [ 2018 ] Toward an enterprise growth framework for entering the base of the pyramid market: A systematic review . International Journal of Innovation and Technology Management , 15 , 4: 1850035. Link , Google Scholar
- Wagire, A. A., Joshi, R., Rathore, A. P. S. and Jain, R. [ 2020 ] Development of maturity model for assessing the implementation of Industry 4.0: Learning from theory and practice . Production Planning & Control . https://doi.org/10.1080/09537287.2020.1744763 Crossref , Google Scholar
- Weber, C., Königsberger, J., Kassner, L. and Mitschang, B. [ 2017 ] M2DDM - A maturity model for data-driven manufacturing . Procedia CIRP , 63 : 173–178. https://doi.org/10.1016/j.procir.2017.03.309 Crossref , Google Scholar
- Westermann, T., Anacker, H., Dumitrescu, R. and Czaja, A. (2016). Reference architecture and maturity levels for cyber-physical systems in the mechanical engineering industry. In ISSE 2016 — 2016 International Symposium on Systems Engineering — Proceedings Papers , Edinburgh, UK, pp. 1–6, https://doi.org/10.1109/SysEng.2016.7753153. Google Scholar
- Xu, L. Da, Xu, E. L. and Li, L. [ 2018 ] Industry 4.0: State of the art and future trends . International Journal of Production Research , 56 , 8: 2941–2962. https://doi.org/10.1080/00207543.2018.1444806 Crossref , Google Scholar
Pinosh Kumar Hajoary is a Senior Research Fellow at the Department of Management Studies, Indian Institute of Science Bangalore. He works in the areas of Industry 4.0, MIS, and Technology Management. He holds B. Tech and MBA from Central Institute of Technology, Kokrajhar and University of Hyderabad, Hyderabad. - Smart manufacturing maturity assessment: a Turkish case study in glass balcony manufacturing enterprise Göknur Arzu Akyüz and Dursun Balkan 23 September 2023 | Smart Science, Vol. 12, No. 1
- Az ipar 4.0 felkészültség értékelési rendszere a témamodellezés segítségével – középpontban a kis- és középvállalatok Andrea Gelei, Szabina Fodor and Katalin Ternai 20 Nov 2023 | Közgazdasági Szemle, Vol. 70, No. 11
- Hazai vállalatok ipar 4.0 felkészültségének értékelése : Modelljavaslat és annak esettanulmányokon keresztül történő vizsgálata Andrea Gelei, Katalin Ternai and Elizabeth Lengyel 15 September 2023 | Vezetéstudomány / Budapest Management Review, Vol. 54, No. 9
- Digital Transformation Journey Guidance: A Holistic Digital Maturity Model Based on a Systematic Literature Review Arzu Aras and Gülçin Büyüközkan 20 April 2023 | Systems, Vol. 11, No. 4
- A smart modern construction enterprise maturity model for business scenarios leading to Industry 4.0 Priyadarshini Das, Srinath Perera, Sepani Senaratne and Robert Osei-Kyei 13 March 2023 | Smart and Sustainable Built Environment, Vol. 18
- Assessing Lean 4.0 for Industry 4.0 Readiness Using PLS-SEM towards Sustainable Manufacturing Supply Chain Karishma M. Qureshi, Bhavesh G. Mewada, Sumeet Kaur and Mohamed Rafik Noor Mohamed Qureshi 21 February 2023 | Sustainability, Vol. 15, No. 5
- A strategic framework for digital maturity of design and construction through a systematic review and application Srinath Perera, Xiaohua Jin, Priyadarshini Das, Kasun Gunasekara and Marini Samaratunga 1 Feb 2023 | Journal of Industrial Information Integration, Vol. 31
- Evaluating Government Readiness for Industry 4.0: A Case Study of Batam City Nofriani Fajrah, Muhammat Rasid Ridho, Lubna Salsabila, D. Mutiarin and M. Alam et al. 1 November 2023 | E3S Web of Conferences, Vol. 440
- Reindustrialization Using Industry 4.0 Maturity Models in Msmes and Tenets of Digital Transformation Phases Kavita Bhatt and S. Mohan Kumar 26 Dec 2022
- A systematic review of the implementation of industry 4.0 from the organisational perspective Hamed Nayernia, Hanna Bahemia and Savvas Papagiannidis 26 November 2021 | International Journal of Production Research, Vol. 60, No. 14
- Sarah Philipson
- Development of a Human-Centered Implementation Strategy for Industry 4.0 Exemplified by Digital Shopfloor Management Magnus Kandler, Marvin Carl May, Julian Kurtz, Andreas Kuhnle and Gisela Lanza 1 November 2021
- Digital Transformation Models for the I4.0 Transition: Lessons from the Change Management Literature Nicola Bellantuono, Angela Nuzzi, Pierpaolo Pontrandolfo and Barbara Scozzi 23 November 2021 | Sustainability, Vol. 13, No. 23
- Diagnosis of the Maturity Level of Implementing Industry 4.0 Solutions in Selected Functional Areas of Management of Automotive Companies in Poland Ewa Stawiarska, Danuta Szwajca, Mirosław Matusek and Radosław Wolniak 26 April 2021 | Sustainability, Vol. 13, No. 9
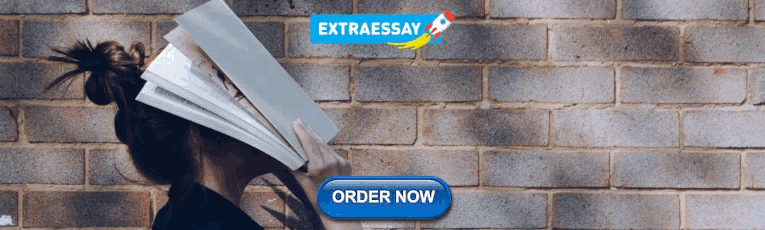 Recommended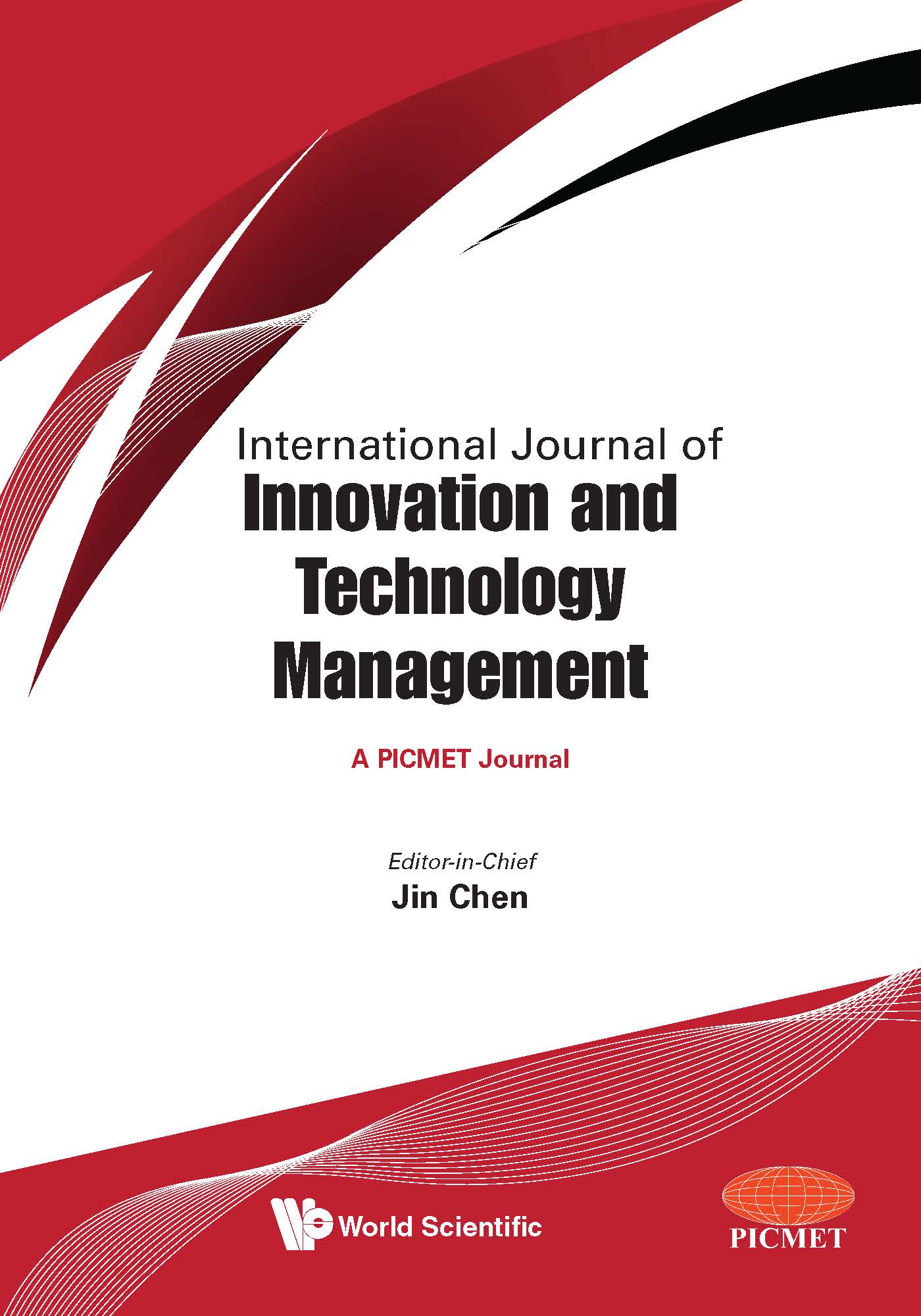 Received 10 March 2020 Revised 11 November 2020 Accepted 11 November 2020 Published: 9 January 2021 Academia.edu no longer supports Internet Explorer. To browse Academia.edu and the wider internet faster and more securely, please take a few seconds to upgrade your browser . Enter the email address you signed up with and we'll email you a reset link. 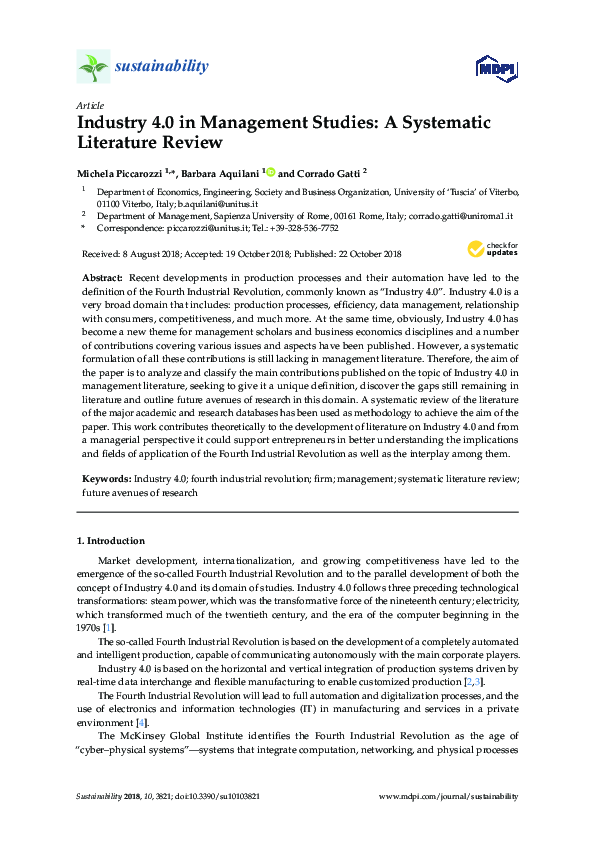 Industry 4.0 in Management Studies: A Systematic Literature Review Sustainability Recent developments in production processes and their automation have led to the definition of the Fourth Industrial Revolution, commonly known as “Industry 4.0”. Industry 4.0 is a very broad domain that includes: production processes, efficiency, data management, relationship with consumers, competitiveness, and much more. At the same time, obviously, Industry 4.0 has become a new theme for management scholars and business economics disciplines and a number of contributions covering various issues and aspects have been published. However, a systematic formulation of all these contributions is still lacking in management literature. Therefore, the aim of the paper is to analyze and classify the main contributions published on the topic of Industry 4.0 in management literature, seeking to give it a unique definition, discover the gaps still remaining in literature and outline future avenues of research in this domain. A systematic review of the literature of the major academic and re... Related PapersMichela PICCAROZZI Advanced manufacturing solutions, augmented reality, and cloud and big data are technologies pertaining to Industry 4.0. These technologies improve working conditions, create new business models, and increase both productivity and firm quality production. However, they can also improve life and society as a whole. This new perspective, oriented toward social and global well-being, is called Society 5.0. As has happened for all past industrial revolutions, Industry 4.0 will support the transition to a different society, i.e., Society 5.0. In this transition, open innovation and value co-creation can play an important role. The aim of the study was twofold: to examine how Industry 4.0 features and enabling technologies can support the transition to Society 5.0 and to investigate the roles of both open innovation and value co-creation within this transition. A conceptual framework was developed to jointly consider for the first time Industry 4.0, Society 5.0, open innovation, and value... 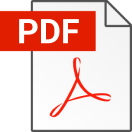 Marco Savastano The digital transformation of manufacturing (a phenomenon also known as “Industry 4.0” or “Smart Manufacturing”) is finding a growing interest both at practitioner and academic levels, but is still in its infancy and needs deeper investigation. Even though current and potential advantages of digital manufacturing are remarkable, in terms of improved efficiency, sustainability, customization, and flexibility, only a limited number of companies has already developed ad hoc strategies necessary to achieve a superior performance. Through a systematic review, this study aims at assessing the current state of the art of the academic literature regarding the paradigm shift occurring in the manufacturing settings, in order to provide definitions as well as point out recurring patterns and gaps to be addressed by future research. For the literature search, the most representative keywords, strict criteria, and classification schemes based on authoritative reference studies were used. The fin... Applied System Innovation Dag Øivind Madsen This study provides an exploratory bibliometric analysis of the emerging literature on Industry 5.0, which is a new visionary concept on the future of industry. Industry 5.0 has in recent years begun to attract the interest of both practitioners and academics, but this new field can still be considered embryonic and not well documented. Therefore, this study aims to map the field and provide a preliminary picture of the emergence and status of the scientific literature on Industry 5.0. Bibliometric data covering the period from 2015 to 2021 were extracted from the Scopus database. Bibliometric analyses of overall publication volume and growth trajectory, influential documents, authors, sources and countries are performed. The exploratory analysis provides a preliminary overview of the birth and emergence of this new research area. The results are discussed in relation to theories on the emergence and evolution of new management concepts. The article closes with some speculations abo... Paola De Bernardi The concept of Industry 4.0 has been mainly addressed by the current literature from a technological perspective, overlooking the organisational and even ethical challenges related to this recent paradigm. In order to become ‘4.0 compliant’, an enterprise must adapt its organisation and business approaches, and these changes may lead to a significant impact on sustainability. Therefore, we performed a systematic literature review to investigate the most recent Industry 4.0 research streams by adopting a multi-perspective approach. This analysis led to collect insights on the key traits of an Enterprise 4.0: integration, decomposed hierarchy, flexibility, and autonomy. Each of these keywords involves work environments, business and organisational models, and educational approaches, which constitute the key traits of the novel framework proposed in this study. Industry 4.0 (I4.0) technologies have been highlighted in recent literature as enablers of servitisation. Simultaneously, businesses are advised to implement a circular economy (CE) to bring new opportunities. However, it is pertinent to mention that little attention has been given to assess the role of I4.0 in adopting the CE and servitisation in a fully integrated manner. This research fills this gap by developing a conceptual framework through a systematic literature review of 139 studies investigating the relationship between the I4.0, CE, and servitisation. This study identifies the impact of these variables on a firm’s operational and financial performance (revenue stream, growth, and profitability). Our research findings advocate that adopting I4.0 technologies to the business and manufacturing model enables sustainability, energy and resource efficiency while enhancing performance and offering innovative products through smart services. Thus, firms must systematically adopt ... In 2020, the whole world had to face a pandemic with inevitable profound changes in all aspects of life, from the social to the economic sphere. The profound economic crisis that followed the rise of the pandemic has pushed firms and researchers to question the necessary changes and new challenges for the survival of businesses. In this scenario, the aim of the paper is to analyze and classify the main contributions published on the topic of COVID-19 in managerial literature, seeking to discover the perspective and the gaps and outline future avenues of research. A systematic review of the literature has been performed. The results highlight the orientation of studies in this field and the various links between different aspects that emerged. Limitations and implications complete the research. Applied Sciences Carlo Andrea Bassani The enabling role of Digital Technologies towards the Circular Economy transition has been recognized. Nonetheless, to support the transition, the operationalization of the discourse is still needed. The present study performs a systematic literature review, deepening the knowledge on the role of Digital Technologies in operationalizing the Circular Economy transition. The analysis is shaped according to the ReSOLVE framework, as it has been recognized as able to operationally guide industrial firms towards the Circular Economy transition. Despite the broad focus on the topic by the extant literature, the results of the analysis show limited Circular Economy aspects addressed and specific technologies considered, making it difficult to have a complete overview on the implementation of Digital Technologies in the Circular Economy transition, operatively addressing it. Shortcomings are identified regarding the lack of an integrated and holistic analysis of the relationships, the need ... Administrative Sciences (ISSN 2076-3387) (ESCI & Scopus indexing) The Industry 4.0 (I4.0) concept is concerned with the fourth industrial revolution in manufacturing, in which technological trends such as digitalization, automation and artificial intelligence are transforming production processes. Since the concept's introduction at the Hannover Fair in Germany in 2011, I4.0 has enjoyed a meteoric rise in popularity and is currently high on the agenda of governments, politicians and business elites. In light of these observations, some commentators have asked the question of whether I4.0 is a concept that is hyped up and possibly just the latest in a long line of fashionable management concepts introduced over the course of the last few decades. Therefore, the aim of this paper is to provide a critical outside-in look at the emergence and rise of I4.0. Theoretically, these processes are viewed through the lens of management fashion, a theoretical perspective well suited to examinations of evolutionary trajectories of management concepts and ideas. The findings indicate that the I4.0 concept has quickly become highly popular and is dominating much of the popular management discourse. The concept has migrated out of the specialized manufacturing discourse to become a more general concept with mainstream appeal and applicability, evidenced by a multitude of neologisms such as Work 4.0 and Innovation 4.0. The numbers 4.0 have spread in a meme-like fashion, evidenced by the fact that the combination of a noun and the numbers 4.0 are used to signal and usher in discussions about the future of business and society. While there is much evidence that clearly shows that the concept has had a wide-ranging impact at the discursive level, the currently available research is less clear about what impact the concept has had so far on industries and organizations worldwide. Veljko Mijušković Industry 4.0 has been a major force framing the societal, economic and technological environment after 2010. Exposed to ongoing digital transformation, companies are able to exploit opportunities offered by Industry 4.0, and are forced to manage immanent risks and barriers. However, studies on opportunities and challenges relevant for the implementation of Industry 4.0 for companies are scarce. In response to this literature gap, the aim of this exploratory research is to provide a deeper analysis of the level of digital transformation of companies in Serbia based on a digital maturity model, and examine their managers’ opinions on the most important driving forces and implementation barriers. The paper uses exploratory research design based on a survey responded to by 122 high-level managers within the Serbian manufacturing sector. Findings show that, contrary to expectations, digitally transforming enterprises do not see human resources as a driving force, but rather as an obstacl... maria grazia saporito The present review retraces the steps of the industrial and agriculture revolution that have taken place up to the present day, giving ideas and considerations for the future. This paper analyses the specific challenges facing agriculture along the farming supply chain to permit the operative implementation of Industry 4.0 guidelines. The subsequent scientific value is an investigation of how Industry 4.0 approaches can be improved and be pertinent to the agricultural sector. However, industry is progressing at a much faster rate than agriculture. In fact, already today experts talk about Industry 5.0. On the other hand, the 4.0 revolution in agriculture is still limited to a few innovative firms. For this reason, this work deals with how technological development affects different sectors (industry and agriculture) in different ways. In this innovative background, despite the advantages of industry or agriculture 4.0 for large enterprises, small- and medium-sized enterprises (SMEs)... Loading Preview Sorry, preview is currently unavailable. You can download the paper by clicking the button above. RELATED PAPERSVojko Potocan Information Blanka Klimova Kwangho Jung Aldona Kluczek Shahryar Sorooshian Marian MOCAN VINCENZO VARRIALE Valeria Mininno Horst Treiblmaier DORIS MARIELA SILVA DELVASTO Yevgeniy Lukhmanov Marta Travaglioni Irena Pawłyszyn Sensors (Basel, Switzerland) Katalin Puskas Khetani Martin Pech Oshane Thorpe Pierpaolo Pontrandolfo Parkash Tambare Patricia Guarnieri Maximilian Espuny Paulo Peças Cristiano Jesus Loredana Paunescu Claudio Sassanelli Premaratne Samaranayake Luis Jesús Belmonte-Ureña Roman Kmieciak Nemer Rumman Anabela Alves Michał Organa layalee qtaishat Vasja Roblek , Maja Meško Luis Pérez-Domínguez Okechukwu Okorie RELATED TOPICS- We're Hiring!
- Help Center
- Find new research papers in:
- Health Sciences
- Earth Sciences
- Cognitive Science
- Mathematics
- Computer Science
- Academia ©2024
From catch to consumer: enhancing seafood processing management with Industry 4.0 innovations- Open access
- Published: 18 June 2024
- Volume 4 , article number 43 , ( 2024 )
Cite this articleYou have full access to this open access article  - Abhirami Subash 1 ,
- Hareesh N. Ramanathan 2 &
- Marko Šostar 3
51 Accesses Explore all metrics The incorporation of Industry 4.0 technologies into the seafood processing sector presents a transformative potential for improving efficiency, sustainability, and transparency throughout the supply chain. This study aims to examine the impact of such technological advancements, including automation, robotics, blockchain, computer vision, AI, and IoT, on the seafood industry's operations, ranging from sorting, cleaning, and packing to quality assessment and product preservation. Conducted through a Systematic Literature Review (SLR) of Web of Science-indexed papers, this research assesses the synergy between innovation and business 4.0 technologies in seafood processing. Our findings suggest that these technologies not only promise enhanced operational efficiency and product quality but also offer sustainable practices through energy-efficient methods, waste management techniques, and innovative packaging solutions. Additionally, the study addresses the workforce implications, underscoring the shift in job roles towards more specialized and value-added positions rather than outright displacement. The primary data collected and analyzed herein underscores the critical role of Industry 4.0 in promoting sustainable resource management, optimizing processes, and ensuring ecological responsibility within the seafood sector, thereby contributing to the broader goals of food security and environmental sustainability. Similar content being viewed by others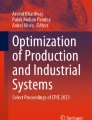 Enhancing Supply Chain Sustainability Through Industry 4.0 and Additive Manufacturing Technologies: A Bibliometric-Based Review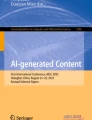 Industry 4.0 Technology-Supported Framework for Sustainable Supply Chain Management in the Textile Industry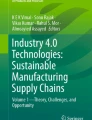 Industry 4.0 Technologies: Opportunities in the Sustainable Supply Chain ManagementAvoid common mistakes on your manuscript. 1 IntroductionThe food business has grown and undergone revolutions around the supply chain including food manufacturing technologies, methods, and customer needs. Human nutrition technology has improved significantly, from harvesting to stem cell technology, and 3D printing, which are slowly getting accepted in the industries. With the high demand and needs of customers like ready to eat ready to cook products, value-addition is also coming to its peak in many countries [ 1 , 2 ]. Artificial intelligence (AI), smart sensors machine learning (ML), robotics, and extensive data analysis are among the critical technologies used in the industry 4.0 (4th Industrial Revolution) [ 2 ], microwaves, ultrasound sensitive computerized sensors high pressure-based technologies, biotechnology, spectroscopy, spectrometry nanotechnology, chromatography, [ 2 ], and other technical innovations are being developed and applied in the food industry [ 3 ]. In a Systematic Literature Review, this article explores the significance of technical advancements in the seafood sector in relation to industry 4.0. A systematic literature review (SLR) finds, selects, and critically evaluates material on a certain topic. Before beginning the investigation, the systematic review should follow an established approach or plan with well-defined criteria. It requires creating a well-considered search approach with a specific focus or resolving a specific problem. The overview explains the information obtained, assessed, and reported within the parameters stated. The evaluation must strictly contain search keywords, tactics (database names, platforms, and search dates), and strict restrictions [ 4 ]. A bibliometric analysis was also carried out using “VOSviewer Application”, emphasizing various elements of the advancement of scientific literature and finding 10 clusters, notably theories, technologies, techniques, nations, and research themes: "Seafood," "Industry 4.0," "Technology," and "Sustainability." [ 5 ], and current and future implications, from which the discussion on Industry 4.0 was more required [ 6 ]. The after-effects of the coronavirus pandemic scenario have also aided in the rapid development and acceleration of Industry 4.0, and the acceptance of its technologies was discussed by [ 7 ]. This paper undertakes a tier-one analysis of worldwide food and beverage publications, identifying trends and changes [ 8 ]. Seafood being highly perishable and spoils quickly, the fourth industrial revolution has a crucial element in addressing numerous emergent difficulties. These technologies have the potential to address all the problems while also extending the shelf life of fish and other seafood, according to studies from numerous research articles that are provided in this publication. Hassoun et al. [ 2 ] addressed this issue emphasized the need for thorough research and understanding on the subject. The following questions are listed and explored in the study. What are the present technology adaptations in seafood processing industry? How will technological innovation under Industry 4.0 affect the future of the seafood industry? Will the seafood sector accept the industrial revolution under the 4th generation? How will the technology introduced into the processing industry replace the skilled workers in the seafood processing sector? How can Industry 4.0 application in the seafood industry lead to a sustainable resources management effect? 2 Materials and methodsThe Systematic Literature Review approach is used in this study to assess the areas under consideration. According to the theoretical framework offered, an exact SLR generally assists in generating a solid understanding of what is known in a subject field, detecting research trends and trajectories, and setting a research plan [ 9 ]. “The growth of evidence-based practice has resulted in an increasing variety of review types" [ 10 ]. A pool of academic contributions was obtained by conducting a keyword search of Web of Science-indexed papers. Web of Science was chosen as the database because it offers complete scientific, technological, and sociological content from all relevant scientific publications [ 6 ]. Finally, the search implication was restricted to a single covering period, which included all industry 4.0 research until 2023. Progressively a deeper understanding of the growth of the interaction between innovation and business 4.0 technologies in seafood processing companies is gained. The study followed a definite path in the systematic literature. The initial stage was the identification of the study questions, followed using the SLR technique. Then the identified papers were analyzed on different parameters using the software and then the analysis and discussion were done. 3 Result and discussion3.1 analysis using vosviewer. A bibliometric analysis was also carried out, emphasizing various elements of the advancement of scientific literature and finding 10 clusters, notably theories, technologies, techniques, nations, and research themes: "Seafood," "Industry 4.0," "Technology," and "Sustainability." Although VOSviewer is primarily meant for bibliometric network analysis, it may also be used to construct, visualize, and explore maps based on network data. The clusters shown in Table 1 depicts the relationship between author keywords obtained by Fig. 2 , where the interaction between 4 major categories have been mentioned and they are: Seafood, Technology, Sustainability and Safety and Quality. Figure 1 depicts approaches for investigating technical innovation in the context of the 4.0 paradigm for the seafood processing sector. However, there is a disparity in the cited theories and conceptual frameworks employed to analyze the topic. [ 6 ]. Each cluster illustrates a topic-based explanation that directly and indirectly connects the paper keywords Fig. 1 . The figure shows 200+ keywords divided into four groups with four color variations. The primary connecting words are “SEAFOOD,” “FOOD SAFETY,” “SUSTAINABILITY,” “NON-HUMAN,” “FOOD INDUSTRY,” “SUSTAINABLE DEVELOPMENT,” “BLOCKCHAIN,” and “INDUSTRY 4.0”. 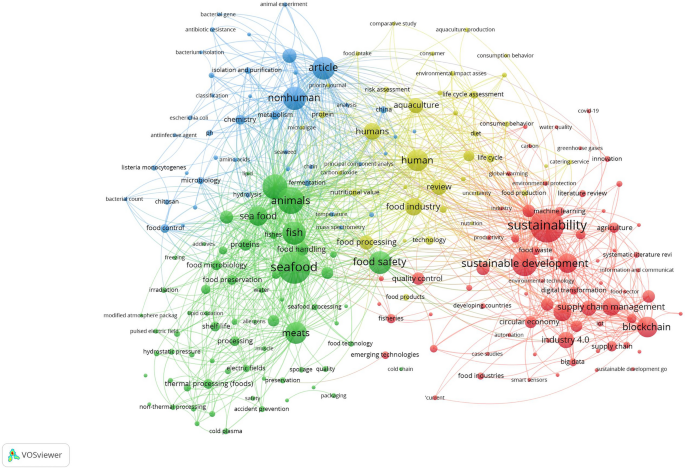 Source: Map created through the VOSviewer application Network based on Co-occurrence Keyword Map. The figure was emphasized and used to investigate the significance of sustainability in the framework of business 4.0 in the seafood processing business (Fig. 2 ). 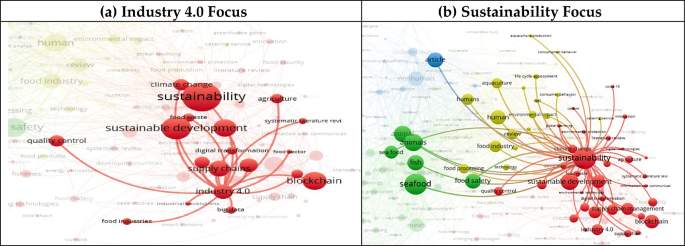 Highlights sustainability and Industry 4.0 from Network based on Co-occurrence Keyword Map. a Industry 4.0 Focus. b Sustainability Focus. Figure 3 a displays the interaction and linkages between the 10 clusters constructed using input data from the Web of Science. On the map, the author's name and the year of publication have been filtered.  Interaction and linkages between the 10 clusters. a Network based on Co-authorship documents Map. b Map highlighting the paper authorship relation on Industry. The clusters in Table 2 correspond to the major and minor conceptual frameworks employed in each research papers, which were chosen and divided based on keyword variation. The topic chosen for review here is seafood industry in line with Industry 4.0, the papers obtained include different clusters, and an outcome of 10 groups was obtained from the VOSviewer application. The inverse of co-citation is bibliographic coupling. If both publications cite a third publication, the two publications are bibliographically connected. Bibliographic coupling refers to the overlap in published reference lists illustrated in Fig. 4 . This bibliographic coupling is based on the same database configuring the other maps. These maps were used to address the research questions thus formed and are explained under.  Bibliographic-Coupling Map. Source: Map created through the VOSviewer application 3.1.1 What are the present technology adaptations in seafood processing industry?Seafood is an essential component of a completely healthy diet. The technology discussed in this research paper has been shown because of its high protein content, it has been demonstrated to include micronutrients like vitamins and minerals. It also contains a high concentration of LC-PUFAs (or Omega-3), namely eicosapentaenoic acid (EPA) and docosahexaenoic acid (DHA) [ 11 ]. Seafood, on the other hand, is very perishable and rapidly degrades. Innovative technology like ultrasonic, irradiation, high-pressure manufacturing, cold pulsed electric field, plasma, radiofrequency, and pulsed frequency are being used to overcome these challenges with the advent of Industry 4.0 [ 12 ]. These forms of technology can overcome the barriers and extend the life of fish for food [ 2 ]. Formaintaining fish quality and sustainable fishing methods, sophisticated processing and post-harvest technologies are required. Hence the sophistication technology has brought up great importance due to the requirement of the customers. Wild-caught fish is first washed and cleaned onboard the fishing vessel and then sorted according to size and species before being shifted to the fish hold for storage [ 13 ]. Farm-raised fish should be stunned first, as soon as it is harvested, and then bled in specific tanks with running water facilities built for the purpose before being further processed. This will reduce postmortem alterations and retain nutritional content. Bleeding is carried out in specialized tanks with fresh seawater circulation. Processing starts from de-heading, scaling, filleting, forming and freezing, the process stages and style vary according to the customer requirements. The seafood processing sector could achieve several innovations through automation and ICT applications, which enabled the industry to uphold high quality standards and get rid of production line defects in processing of various seafood items like fillets, where unit get reduced thereby increasing the value and shelf life of catch. The ability to objectively assess the fillet quality is necessary for a machine to automatically identify and eliminate quality flaws. With the application of cameras, sensors, and lighting technologies to simulate human vision, computer vision has advanced significantly in recent years [ 14 ]. As Einarsdóttir et al. (2022) highlighted that AI and vision technologies have also been utilized in shellfish processing to identify bits of shell following shrimp peeling. Papers that link with the article are from Fig. 3 a. Zhang et al. [ 15 ] on Chaof ranges in chemical gel from silver carp fillets while processing it through setting and heating. The influence of increased gel strength on the effects of different washing solutions is demonstrated by the calcium chloride 0–2% washing solution [ 16 ]. This work examines knowledge transfer to small and medium-sized (SMEs) using a dynamic research model that can improve future seafood processing technology preservation. The remaining papers in the first cluster discuss new technology for preserving muscle and meat quality through high-pressure processing and extending the product's shelf life. The second cluster from Fig. 3 a (reference to Table 2 ) includes seven major papers [ 17 ] illustrating on innovative and interactive packaging and IMedBox, the authors' universal and preventative medication management system. Controlled delamination (CDM) seals and regulated wireless technology were also studied in new medication packaging [ 17 ]. Li et al. [ 18 ] evaluated that presently there are various types of packaging. Smart, interactive, active, ergonomic, and intelligent packaging advances all have an influence on passive packaging. Intelligent packaging is a fully integrated system with intelligent features such as detecting, sensing, recording, tracing, and communicating. The primary goals are to increase shelf life, improve safety and quality, gives information, and send alerts on possible concerns and thereby assuring environment sustainable material. As a result, wireless connectivity through biological sensors could categorize critical characteristics. High-performance design enables immediate care and prediction of these crucial aspects. The employment of statistics and modelling in tandem improved the efficacy of the quality characterization process [ 19 , 20 ] as well as the pragmatic application resulting the food quality monitoring. To confirm the quality of food, key public issue, and dependable sensors or systems for monitoring are required [ 21 ]. Zaragozás et al. [ 20 ] in his paper, refers to the simultaneous use of statistics and modeling as extremely helpful for the quality characterization process. To confirm the quality of diverse food commodities, a document addressing the safety and quality of food, a key public issue, and dependable sensors or systems for monitoring are required [ 22 ]. Chemosensors are a novel sensing device being researched and developed in the food sector, with immense potential for enabling onsite monitoring of food quality and safety. One strategy to assist SMEs in developing and increasing their competitiveness in the global market is through technology transfer. The new approaches are needed with the adoption of revolutionary technologies and getting around persistent barriers between researchers and industry [ 16 ]. It can be concluded that, technology improvements have been significantly reflected in the biochemical processes that have been in use in the seafood industry from the traditional time. New mythological launches obtained greater result acceptability, demonstrating the need of comprehensive modification of machinery and other accessories in the business. The requirement for better waste management practices in the company premises with better technology interaction is also a case to be discussed. With the introduction of Industry 4.0, advanced technologies mentioned above would be ultimately applied to the seafood processing sector. 3.1.2 How will technological innovation under Industry 4.0 affect the future of the Seafood Industry?When the seafood sector is considered, it enormously contributes to the world economy, accounting for about 8% of the global demand for protein [ 23 ]. With increasing customer demands, emerging techniques are being studied to supplement preservation procedures to ensure non-hazardous food and increase shelf life without compromising food safety with quality. In this way, modern cold-based storage techniques like chilling and super chilling contrast traditional storage methods like freezing and refrigeration are being adopting the latest materials for construction and endorsement in the technological sides Zhang et al. [ 15 ] suggested two advanced systems of compression aided ammonia water absorption refrigeration circuits that use the ocean's energy to satisfy seafood freezing and storage refrigeration requirements in coastal and offshore island areas. Cascade refrigeration systems (CRS) have been identified as promising to increase energy efficiency while fulfilling multiple temperature targets [ 24 ]. Investigations were also made to assess the in-fluence of alternating pressure on energy efficiency and primary energy rate ratio in refrigeration systems. The use of innovative food packaging techniques (edible films and coatings) and differential scanning calorimetry (DSC) studies of glass transition temperatures for mixed zein films over the water content range revealed that sunflower oil has a plasticity influence on the film [ 25 , 26 ], as well as a new method of storing food called storage under extreme conditions, which uses storage pressure control to thwart microbial growth and subzero refrigeration and room temperatures both postpone organoleptic deterioration [ 27 , 28 ]. The development of intelligent packaging [ 29 , 30 ] was classified and mapped using circular economy principles, including the primary motivations and hurdles that might aid or impede the increase in packing with increased traits and potential benefits businesses can obtain. While a model for sophisticated monitoring of freshness with continuous surveillance food freshness is preserved as a barrier for both food producers and end users due to the lack of portable detection devices, affordable, and efficient have yet to be commercialized, have developed a simple sensor system based on a cell phone app that includes a real-time deeper learning model freshness of food monitoring (see Fig. 3 a), [ 31 ]. The utilization of cellulose paper for colorimetric indicator bars was introduced through a novel approach involving UV-induced crosslinking of synthesized Gelatine methacryloyl (GleMA) along with encapsulated bromocresol green (BCG). The third cluster shown in Fig. 2 b directly deals with Industry 4.0, as illustrated in papers [ 32 , 33 ]. The phrase "Industry 4.0" was coined by a German federal government project, including academics and commercial businesses, in 2011. This project was related to a strategy program to build the most sophisticated production methods to enhance domestic industry productivity and efficiency in Germany [ 34 ]. The study presented here provides an understanding of Industry 4.0 by text mining of associated materials [ 35 ]. The journal papers, 660 in number, were identified as those having the keywords “Industry 4.0″ or “fourth industrial revolution” from the Web of Science Core Collections, containing all available data published was taken from [ 36 ]. The seafood industry is working on improved food safety and shelf-life strategies. Cold storage technologies, environmentally friendly, [ 13 ] creative food packaging, and the creation of smart packaging are all being researched. Using smartphone app sensor technologies, continuous food freshness tracking is being created. Hence a major focus is Industry 4.0 in future. 3.1.3 Will the seafood sector accept the industrial revolution under the 4th generation?Industry 4.0 has received much attention in the recent literature. However, there is a dearth of systematic and comprehensive research reviews that reflect the topic's dynamic nature [ 37 , 38 ]. Figures 1 and 2 b establishes the connection between industry 4.0 and its importance in the current situations through the latest publication, with the present twentieth century proving its state and importance. Various cutting-edge technologies are included in Industry 4.0, including ERP, sensory monitoring systems [ 39 ], and modernized artificial intelligence technology for improved quality control systems. AI accommodates innovation analytics [ 40 ], the fundamental process of generating insights, models, and visualizations through computer-based data analysis, coupled with creative problem-solving, fosters innovation and facilitates progress [ 41 ]. Digital, biological, and physical worlds and new technologies distinguish Industry 4.0 [ 42 ].Incorporating Artificial Intelligence (AI) and blockchain technology (BCT) into supply chains offers substantial benefits, including improved operational capabilities, support for sustainable growth, data monetization, and leveraging cutting-edge technologies like cloud computing, robotics, 3D printing, advanced wireless technologies, and the Internet of Things (IoT) [ 43 ]. Furthermore, upcoming technologies such as fifth generation (5G) communications, which include the Internet of Things (IoT) [ 44 ], blockchain, artificial intelligence, and so on, will hasten global business change. 5G technology is intended to unlock gigantic IoT ecosystems by enabling immense connections for many IoT devices with excellent data rates, ultra-low latency, and low-cost access. The 5G networks will provide the performance required for massive IoT and allow a perceived ultimately linked world. Meanwhile, blockchain is being pushed as the foundation for a new business model in future IoT (IoT) [ 45 ]. This Fig. 2 b is generated using a group of keywords with reference to Table 1 , generally expressed from Industry 4.0 that emphasizes the significant linkages of other keywords that directly relate to the relevance of Industry 4.0. It is directly associated with seafood processing, where cutting-edge technologies like ohmic heating, pulsed electric field high-pressure processing, and nanotechnology are well discussed in these papers [ 46 , 47 ], advanced mass spectrometry, Smart labels are mentioned in [ 48 ], Others were discussed and mentioned [ 49 ]. Electronic noses that replicate the human nose have been created to detect food quality and infection. The e-nose gadget appeared to be easier to use, more effective, and less expensive; it was an effective tool for environmental monitoring, proposed by Choudhari et al. [ 47 ]. Clusters four, five, six, and seven from Figs. 1 , 2 b and 3 a discuss other emerging technologies in processing sectors based on nutritional values of food and quality maintenance, like the green technique for valuing chestnut shells developed [ 21 ]. Another experiment that came to be effective and like the devised approach was used to determine trace Bisphenols in canned meat, with recoveries ranging from 73 to 117%, indicating a possible use for pretreatment in complicated materials [ 50 ]. This research paper demonstrates the utilization of nonthermal processing techniques, such as pulsed electric field (PEF), dense phase carbon dioxide (DPCD), high hydrostatic pressure (HHP), membrane technology, ultrasound-assisted extraction (UAE), and enzyme-assisted extraction, to extract bioactive compounds from marine byproducts [ 51 ]. Another idea put forward by [ 52 ] is the presence of polyunsaturated fatty acids and carotenoids in prawn oil makes it healthier than other fish oils. The technique of extracting SPBP oil can be improved by lowering the requirement for solvents and increasing yield. By modifying the procedures, drawbacks can be managed. A product with added value for the sustainability of the prawn industry and marine resources can be a stable form produced employing techniques at the industrial level. Comparing non-thermal technologies to conventional techniques, they produced more favorable results in terms of output, quality, energy, and time costs for extracting bioactive chemicals from marine by-products. This shows that implementing non-thermal alternatives could potentially improve food security while reducing environmental issues [ 53 ]. A review of the latest knowledge about hydrophobic marine-derived bioactive compounds' nanostructured delivery vehicles and methods of the production highlighted their potential use in functional food since they enhance their organoleptic properties and lower their toxicity was mentioned by Hosseini et al. [ 54 ]. In commercial and research applications, HPP technology is utilized to combine pressure with bio-preservation to achieve certain product features. This study also discusses new results in microbiology, chemistry, and molecular biology [ 55 ]. Astaxanthin, a xanthophyll carotenoid pigment found in shellfish, crabs, and seaweeds, can be utilized as an ingredient in food products to maintain freshness, color, flavor, quality, and other factors. According to current research on the combination of astaxanthin and nanotechnology, the encapsulation of astaxanthin in nanocarriers improves the solubility, stability and permits a wide range of applications due to its enhanced bioavailability in digestive, endocrine, and nervous systems [ 56 ]. Texture modified foods are becoming more and more popular among the elderly since they are easier for old and dysphagic individuals to chew. A study on application of herbal extract to improve the consistency of texture of yellowfin sole (Pleuronectes aspera), prior to grilling with superheated steam, revealed that marinating fish with herbal extracts results in soft texture, improved sensory quality, and ease of ingest for elderly people [ 57 ]. Figure 1 describes the paper connection and its correlation through Co-Citation mapping on the above-mapped clusters. Each paper shows a link to the technological aspects of the seafood industry, thereby stating the importance of technology and its adoption. It demonstrates the relationship between the citations and the associated paper conjunction, which is then visually shown. Nevertheless, the progress in technology has significantly influenced various food matrices, showcasing advantageous improvements in terms of their physicochemical attributes, sensory characteristics, nutritional composition, and extension of shelf life [ 58 ]. The study focuses on current developments in biosensing techniques fand their application in food safety to establish relationship between small molecule pollutants in water and biological samples acquired from aquaculture and fisheries, such as heavy metals, antibiotics, biotoxins, and different seafood degradation factors. Also, it seeks the importance of advances in the biorecognition process, signal transduction approaches, and the quick advancement of element integration in each unique strategy, emphasizing the critical roles of revolutionary material science, simple platform manufacture, and intelligent readout [ 59 ]. Through comprehensive investigations into the functionalities, applications, and production techniques of FPCP protein hydrolysates, significant research patterns and future directions have emerged to capitalize on the economic and environmental benefits for the fish processing industry. FPCP hydrolysates have been found to possess desirable physical and chemical properties, such as emulsifying, foaming, and oil and water binding capacities. Additionally, they exhibit a diverse range of intriguing bioactivities, including anti-oxidative, anti-hypertensive, anti-microbial, and anti-anemia effects, which make them applicable in various food, nutritional, and pharmaceutical products. While chemical hydrolysis remains the most used method for producing crude FPCP protein hydrolysates, its limited control over product quality necessitates exploration of more precise alternatives [ 60 ]. Clusters eight, nine from Fig. 3 a and b, and ten discuss food quality issues like the contamination of dried seafood during processing as well as handling in the storage can be affected by mycotoxins which are naturally poisonous secondary metabolites generated by molds or filamentous fungal species, the most dangerous of which include aflatoxins, ochratoxin A, deoxynivalenol, T-2 toxin, zearalenone, and fumonisins. Recent trends show an increase in the reports of mycotoxin contamination in dried fish products. To increase food safety, mycotoxins in dried fish items sold in markets must be monitored [ 61 ]. The other papers already show the interconnection with the previously mentioned cluster groups. As a result of technological advancements, the adoption of new high-tech robotic equipment has already influenced the food business. As quality and safety go hand in hand with technology, this creates a route for other sectors, such as seafood, to expand and meet customer needs. Once the system becomes trendy, it will steadily spread for better marketing and product demand. Hence leading to the adaptation of the industry to 4.0, 5.0, and so on with time. 3.1.4 How will the technology introduce into the processing industry replace the skilled workers in the Seafood processing sector?Professional employees are required for filleting, trimming, peeling, visual inspection, and quality control throughout the seafood processing process, from raw materials to consumable goods. According to an examination of Figs. 1 and 2 b, the fish industry frequently requires assistance in hiring workers for processing plants. Jobs are often tedious, repetitious, and even hazardous. Seafood quality degrades fast, significantly, when temperatures rise. As a result, the product is constantly maintained cool and, the working environment is cold and wet, making it difficult for employees to stay for the entire shift [ 13 ]. Historically, the fish business has been labor-intensive, needing specialized workers to turn entire fish into consumable items. Because of the biological variety found in food items, automation in food production has been slower than in specific industries such as automobiles. Since machines will never obtain the finishing provided by a skilled worker in their product, skilled labor has always been the fundamental foundation of prawns and other qualified processing requirements. Recent advancements in automated inspection, artificial intelligence, and robots are reshaping the food manufacturing business, providing new automation capabilities that have the potential to boost throughput and output. In the processing of crustaceans and shellfishes, AI and vision technology have also been utilized to detect shell fragments after prawns and mussels have been peeled says [ 13 ]. The changes brought up in the seafood industry and its transformation in the future is related in Fig. 3 b. Gripper technology is the design of tooling that can manipulate food products, such as picking products from a conveyor and placing in a tray. Gripping technology today often tries to resemble elements from nature, such as suction cups on octopuses’ arms or grasping capabilities of human hands. Other advances that are on the horizon are mobile robots [ 62 ]; (Rubio et al., 2019) and collaborative robots, capable of performing in the harsh food production environment. Due to the unsanitary practices of trained personnel, completed items may pose a disease threat to customers, causing trust and quality concerns for the company. The integration of AI and automation in industries can lead to both economic and social sustainability. While automation can improve efficiency and reduce costs, it can also lead to job displacement, causing unemployment and income inequality. Additionally, workers may need to acquire new skills or retrain to stay relevant, necessitating access to training and education opportunities. Therefore, balancing economic stability and social sustainability is crucial for ensuring the long-term success of the industry. As a result, appropriate resource management, monitoring systems, robotic interactions, and machine learning among expert employees can endure changing people's wants. 3.1.5 How can Industry 4.0 application in the seafood industry lead to a sustainable resources management effect?Figure 2 and Table 1 illustrates the author key words correlating about 200+ papers related to sustainability, sustainable development, seafood, fish, food safety, food industry, and Industry 4.0 and supply chain management. Based on recent studies, the advancements in industry 4.0 technologies have the potential to enhance food sustainability, leading to more environmentally friendly food production and consumption practices. Intelligent applications in the food manufacturing sector (smart factories) and agricultural production systems (brilliant/precision agriculture and farming) hold the promise to accelerate the adoption and integration of Industry 4.0 technology [ 32 ]. This indeed creates easy access of seafood sources to the demand for ready-to-eat, ready-to-cook products. Numerous recent publications have highlighted the pivotal significance of Industry 4.0 technologies in reducing and upcycling food waste, enhancing food quality, enabling better food traceability, and fostering the creation of healthier and ecologically sustainable food products. The emerging notion of "Food Quality 4.0" entails leveraging Industry 4.0 technologies in food analysis to achieve rapid, reliable, and unbiased evaluations of food quality. This concept was thoroughly explored and discussed in the mentioned source [ 63 ]. The figure depicts the fundamental technologies of Industry 4.0 utilized to develop intelligent food factories and farms, enhance food quality, safety, and traceability, reduce food waste and energy consumption, as well as create novel and healthier food options [ 5 ]. The research encompassed peer-reviewed publications, grey literature, relevant networking websites, and conference proceedings focusing on the application of eco-friendly technology and Industry 4.0 enablers in promoting food sustainability. The primary aim was to underscore the significance of these cutting-edge solutions as essential elements of increasingly sustainable agricultural system [ 5 ]. Hence technological advancements with a parallel focus on sustainability can meet demand by future generations for seafood resources. 4 ConclusionThe seafood sector is predicted to benefit from sector 4.0, which includes automation and robots to improve operations like sorting, cleaning, and packing, decreasing labor costs and increasing productivity. Blockchain technology is being investigated for transparency, while sophisticated technologies like computer vision and AI are being utilized to evaluate the quality of marine items. Energy-efficient technology and waste management techniques are among the sustainable practices that are being used. The shelf life of products is being extended by using innovative packaging techniques including modified environment and vacuum packing. While cutting-edge processing methods are being developed, IoT devices are being utilized to monitor and regulate parameters. Hygiene precautions are being improved, and energy efficiency is being optimized. Direct sales to consumers and online marketplaces are also used. Overall, the implementation of industry 4.0 technologies in the seafood sector has the potential to increase efficiency, quality, sustainability, and transparency across the supply chain. It is crucial to emphasize, however, that these developments are not without hurdles, such as the need for early investment, labor adaptability, and cybersecurity issues. The integration of digital, green, and innovation strategies for sustainable economic development is also a key factor for future revolutionary change [ 64 ]. Compared to the Industrial Revolution, other sectors, such as communications systems, textile systems, and so on, have secured the fifth revolutionary voyage. The advent of technology in the seafood processing business can have an influence on the jobs of experienced personnel, although it does not necessarily completely replace them. Instead, it frequently changes their jobs, forcing people to adapt to new methods of functioning. Automation of repetitive jobs, shift towards technical competence, quality control, maintenance, data management, training, innovation, process improvement, cooperation with technology, complicated problem-solving, and regulatory compliance are all examples of this. Instead of displacing talented individuals, technology changes their positions within the sector to more specialized and value-added jobs. Hassoun et al. [ 65 ] presents an overview of Industry 4.0 technologies in the food processing industry, focusing on robotics, smart sensors, artificial intelligence, the Internet of Things, and Big Data as key enablers. These technologies include quality control, safety, and production efficiency. They emphasize the need for detailed studies to address challenges and provide insights into each enabler. This indeed also highlights the need for workers to acquire new skills or retrain to stay relevant in the workforce, emphasizing the importance of access to training and education opportunities. Industry 4.0 technologies have the potential to greatly enhance sustainable resource management in the seafood sector. These technologies can enable ecologically responsible and sustainable marine resource management by optimizing procedures, minimizing waste, and improving traceability. Benefits include effective resource utilization, decreased waste and byproducts, sustainable fishing practices, traceability and transparency, ecosystem monitoring, energy efficiency, predictive analytics, closed-loop systems, cooperation, knowledge sharing, and compliance monitoring. These developments lead to a more sustainable approach to seafood production, which benefits both the industry and the environment. Data availabilityNo datasets were generated or analysed during the current study. Streams PN. The industrial revolution in world history. New York and London: Routledge; 2021. Google Scholar Hassoun A, Siddiqui SA, Smaoui S, Ucak I, Arshad RN, Garcia-Oliveira P, Prieto MA, Aït-Kaddour A. Seafood processing, preservation, and analytical techniques in the age of Industry 4.0. Appl Sci. 2022;12(3):1703. Article CAS Google Scholar Onibonoje MO, Nwulu N, Bokoro PN. Artificial Intelligence and IoT-based technologies for sustainable farming and smart agriculture. Hershey: IGI Global Publisher of Timely Knowledge; 2021. p. 18. Dewey A, Drahota A. Cochrane.org. 2016. https://training.cochrane.org/interactivelearning/module-1-introduction-conducting-systematic-reviews . Hassoun A, Prieto MA, Carpena M, Bouzembrak Y, Marvin HJ, Pallares N, Barba FJ, Bangar SP, Chaudhary V, Ibrahim S, Bono G. Exploring the role of green and Industry 4.0 technologies in achieving sustainable development goals in food sectors. Food Res Int. 2022;162: 112068. Article PubMed Google Scholar Cannavacciuolo L, Ferraro G, Ponsiglione C, Primario S, Quinto I. Technological innovation-enabling industry 4.0 paradigm: a systematic literature review. TTechnovation. 2023;124: 102733. Article Google Scholar Ojo OO, Shah S, Coutroubis A, Jiménez MT, Ocana YM, Ocana YM. Potential Impact of Industry 4.0 in Sustainable Food Supply Chain Environment, Marrakech, Morocco: International Conference on Technology Management, Operations and Decisions (ICTMOD), 2018. Viswanathan R, Telukdarie A. The impact of 4IR on the future skills in food and beverage industry. In: Portland International Conference on Management of Engineering and Technology (PICMET), Portland, 2022. Massaro M, Handley K, Bangnoli C, Dumay J. Knowledge management in small and medium enterprises: a structured literature review. J Knowl Manag. 2016;20:258–91. Grant MJ, Booth A. A typology of reviews: an analysis of 14 review types and associated methodologies. Health Info Libr J. 2009;26(2):91–108. Zárate R, Jaber-Vazdekis N, Tejera N, Pérez JA, Rodríguez C. Significance of long chain polyunsaturated fatty acids in human health. Clin Transl Med. 2017;6(1):25. Article PubMed PubMed Central Google Scholar Hassoun A, Guðjónsdóttir M, Prieto MA, Garcia-Oliveira P, Simal-Gandara J, Marini F, Donato FD, Archivio D, Biancolillo AA. Application of novel techniques for monitoring quality changes in meat and fish products during traditional processing processes: reconciling novelty and tradition. Processes. 2020;8(8):988. Einarsdóttir H, Guðmundsson B, Ómarsson V. Automation in the fish industry. Animal Front. 2022;12:32–9. Kopparapu SK. Lighting design for machine vision application. Image Vision Comput. 2006;24:720–6. Zhang L, Li Q, Jing S, Zhu B, Luo Y. Changes in chemical interactions and gel properties of heat-induced surimi gels from silver carp ( Hypophthalmichthys molitrix ) fillets during setting and heating: Effects of different washing solutions. Food Hydrocolloids. 2018: 116–124. Morrissey MT, Almonacid S. Rethinking technology transfer. J Food Eng. 2005;67:135–45. Chelliah R, Wei S, Daliri EB-M, Rubab M, Elahi F, Yem S-J, Yan P, Jo KH, Oh DH. Development of nanosensors based intelligent packaging systems: food quality and medicine. Nanomaterials. 2021;11(6):1515. Article CAS PubMed PubMed Central Google Scholar Li Y, Chu F, Côté J-F, Coelho LC, Chu C. The multi-plant perishable food production routing with packaging consideration. Int J Prod Econ. 2020;221: 107472. Lotta K, Marc S, Solimeo A, Pérez-Fernández R, Vanderroost M, Baest BD, Meulenaer BD, Ragaert P, Devlieghere F. Spoilage evaluation of raw Atlantic salmon ( Salmo salar ) stored under modified atmospheres by multivariate statistics and augmented ordinal regression. Int J Food Microbiol. 2019;303:46–57. Zaragozá P, Fuentes A, Ruiz-Ric M, Vivancos J-L, Fernández-Segovia I, Ros-Lis JV, Barat JM, Manez RM. Development of a colorimetric sensor array for squid spoilage assessment. Food Chem. 2015;175:315–21. Conidi C, Donato L, Algieri C, Cassano A. Valorization of chestnut processing by-products: a membrane-assisted green strategy for purifying valuable compounds from shells. Valoriz J Cleaner Prod. 2022;378: 134564. Pavase TR, Lin H, Hussain S, Li Z, Ahmed I, Hussain L, Shah SB, Kalhoro MT. Recent advances of conjugated polymer (CP) nanocomposite-based chemical sensors and their applications in food spoilage detection: a comprehensive review. Sensors Actuators B Chem. 2018;273:1113–38. Sensarma S. Fishereis and aquculture. Invest India - National Investment promotion & Faciltation Agency; 2023. Saini SK, Dasgupta MS, Widell KN, Bhattacharyya S. Comparative investigation of low GWP pure fluids as potential refrigerant options for a cascade system in seafood application. Mitig Adapt Strateg Glob Change. 2022. https://doi.org/10.1007/s11027-022-10036-3 . Mouzakitis C-K, Sereti V, Matsakidou A, Kotsiou K, Biliaderis CG, Lazaridou A. Physicochemical properties of zein-based edible films and coatings for extending wheat bread shelf life. Food Hydrocolloids. 2022;132: 107856. Bizymis A-P, Tzia C. Edible films and coatings: properties for the selection of the components, evolution through composites and nanomaterials, and safety issues. Crit Rev Food Sci Nutr. 2021;63(31):8777–92. Tavares J, Martins A, Fidalgo LG, Lima V, Silva RA, Pinto CA, Silva AA, Saeaiva JA. Fresh fish degradation and advances in preservation using physical emerging technologies. Food. 2021;10(4):780. Palazzo M, Vollero A, Siano A. Intelligent packaging in the transition from linear to circular economy: driving research in practice. J Cleaner Prod. 2023;388: 135984. Lee SJ, Raham AM. Innovations in Food Packaging (Second Edition). 2014: 171–209. Ghoshal G. Chapter 10 - Recent Trends in Active, Smart, and Intelligent Packaging for Food Products. Food Packaging and Preservation. 2018:343–374. Gong W, Yao H-B, Chen T, Yu Y, Fang Y, Zhang H-Y, Hu J-N. Smartphone platform based on gelatin methacryloyl (GelMA)combined with deep learning models for real-time monitoring of food freshness. Talanta. 2023;253: 124057. Kazancoglu Y, Sezer MD, Ozkan-Ozen YD, Mangla SK, Kumar A. Industry 4.0 impacts on responsible environmental and societal management in the family business. Technol Forecast Soc Change. 2021;173: 121108. Li X, Nosheen S, Haq NU, Gao X. Value creation during fourth industrial revolution: Use of intellectual capital by most innovative companies of the world. Technol Forecast Soc Change. 2021;163: 120479. Sarbu M. The impact of industry 4.0 on innovation performance: insights from German manufacturing and service firms. Technovation. 2022;113: 102415. Lee C, Lim C. From technological development to social advance: a review of Industry. Technol Forecast Soc Change. 2021;167: 120653. Laubengaier DA, Cagliano R, Canterino F. It takes two to Tango: analyzing the relationship between technological and administrative process innovations in industry 4.0. Technol Forecast Soc Change. 2022;180: 121675. Strazzullo S, Cricelli L, Grimaldi M, Ferruzzi G. Connecting the path between open innovation and industry 4.0: a review of the literature. In IEEE Transactions on Engineering Management, 2021. Sachin KS, Angappa G, Gawankar AS. Sustainable Industry 4.0 framework: a systematic literature review identifying the current trends and future perspectives. Process Saf Environ Prot. 2018;117:408–25. Khan A, Parya E, Rhim J-WR. Alizarin: prospects and sustainability for food safety and quality monitoring applications. Colloids Surf B: Bionterfaces. 2023;223: 113169. Lepore D, Spigarelli F. Integrating Industry 4.0 plans into regional innovation strategies. J Local Econ Policy Unit. 2020;35(5):496–510. Kakatkar C, Bilgram V, Fülle J. AI accommodate innovation analytics, which are the basic process of developing computer-enabled, data-driven insights, models, and visualisations as part of the innovation process. Business Horizon. 2020: 171–181. Tsolakis N, Schumacher R, Dora M, Kumar M. Artificial intelligence and blockchain implementation in supply chains: a pathway to sustainability and data monetisation? Ann Oper Res. 2022;327(1):157–210. Tenol Alpha. Embracing Industry 4.0 To Thrive. Tenol Alpha, 2020. Vern P, Miftah N, Panghal A. Digital technology: implementation challenges and strategies in agri-food supply chain. Emerald Publishing Limited. 2022: 17–30. Li S, Iqbal M, Saxena N. Future industry internet of things with zero-trust security. Inf Syt Front. 2022. Sachdev D, Kumar V, Maheshwari PH, Pasricha R, Baghel DN. Silver based nanomaterial, as a selective colorimetric sensor for visual detection of post-harvest spoilage in onion. Sensors Actuators B: Chem. 2016;228:471–9. Choudhari U, Jagtap S, Rane S. Emerging applications of nanotechnology for e-nos. Nanotechnology- Based E-Nose, 2023: 57–100. Chen B, Min Z, Chen H, Mujumdar AS, Guo Z. Progress in smart labels for rapid quality detection of fruit and vegetables: a review. Postharvest Biol Technol. 2023;198: 112261. Hassoun A, Aït-Kaddour A, Abu-Mahfouz AM, Rathod NB, Bader FJ, Biancolillo A, Cropotova J, Galanakis CM, Jambrak AR, Lorenzo JM, Måge N. The fourth industrial revolution in the food industry—Part I: industry 4.0 technologies. Crit Rev Food Sci Nutr. 2022;63(23):6547–63. Qiao J-Y, Pang Y-H, Yan Z-Y, Shen X-F. Electro-enhanced solid-phase microextraction with membrane protection for enrichment of bisphenols in canned meat. J Chromatogr A. 2022;1685: 463592. Article CAS PubMed Google Scholar Ali A, Wei S, Liu Z, Fan X, Sun Q, Xia Q, Liu S, Hao J, Deng C. Non-thermal processing technologies for the recovery of bioactive compounds from marine by-products. LWT. 2021;147: 111549. Gulzar S, Raju N, Nagarajarao RC, Benjakul S. Oil and pigments from shrimp processing by-products: extraction, composition, bioactivities and its application- a review. Trends Food Sci Technol. 2020;100:307–19. Picart-Palmade L, Cunault C, Chevalier-Lucia D, Belleville M-P, Marchesseau S. Potentialities and limits of some non-thermal technologies to improve sustainability of food processing. Nutr Sustain Diets. 2019;5:130. Hosseini SF, Ramezanzade L, McClements DJ. Nanoliposomes, nanoemulsions, lipid nanoparticles, and polymeric nanoparticles are evaluated for bioavailability, sensory characteristics, and potential toxicity. Trends Food Sci Technol. 2021;109:322–39. Wang C-Y, Huang H-W, Hsu C-P, Yang BB. Recent advances in food processing using high hydrostatic pressure technology. Crit Rev Food Sci Nutr. 2016;56(4):527–40. Chen Y, Su W, Tie S, Zhang L, Tan M. Advances of astaxanthin-based delivery systems for precision nutrition. Trends Food Sci Technol. 2022;127:63–73. Cho W-H, Yoon S-J, Choi J-S. Optimization of texture-modified yellowfin sole (Pleuronectes aspera) by enzymatic treatment and superheated steam treating to improve quality characteristics. Processes. 2021;9(5):763. Wang Z, Zhou D, Liu D, Zhu B. Food-grade encapsulated polyphenols: recent advances as novel additives in foodstuffs. Crit Rev Food Sci Nutr. 2022;63(33):11545–60. Huang L, Liu G, Fu Y. Recent developments in biosensing strategies for the detection of small molecular contaminants to ensure food safety in aquaculture and fisheries. Trends Food Sci Technol. 2023;133:15–27. He S, Franco C, Zhang W. Functions, applications and production of protein hydrolysates from fish processing co-products (FPCP). Food Res Int. 2013;50:289–97. Deng Y, Wang Y, Deng Q, Sun L, Wang R, Wang X, Liao J, Gooneratne R. Simultaneous quantification of aflatoxin B1, T-2 Toxin, Ochratoxin A and deoxynivalenol in dried seafood products by LC-MS/MS. Toxuns. 2020;12:488. Gao X, Li J, Fan L, Zhou Q, Yin K, Wang J, Song C, Huang L, Wang Z. Review of wheeled mobile robots’ navigation problems and application prospects in agriculture. Rev WMRs Navig Problems Appl Prospects Agric. 2018;6:49248–68. Hassoun A, Jagtap S, Garcia-Garcia G, Trollman H, Pateiro M, Lorenzo JM, Trif M, Rusu AV, Aadil RM, Rusu V, Šimat V, Cropotova J, Câmara JS. Food quality 4.0: from traditional approaches to digitalized automated analysis. J Food Eng. 2023;337: 111216. Yin S, Liu L, Mahmood T. New trends in sustainable development for industry 5.0: digital green innovation economy. Green Low-Carbon Econ. 2023. https://doi.org/10.47852/bonviewGLCE32021584 . Hassoun A, Jagtap S, Trollman H, Garcia-Garcia G, Abdullah NA, Goksen G, Bader F, Ozogul F, Barba FJ, Cropotova J, Munekata PES, Lorenzo JM. Food processing 4.0: current and future developments spurred by the fourth industrial revolution. Food Control. 2023;145: 109507. Download references This research received no external funding. Author informationAuthors and affiliations. Research Scholar, School of Industrial Fisheries, Cochin University of Science and Technology, Kochi, Kerala, India Abhirami Subash Associate Professor, Business Management, School of Industrial Fisheries, Cochin University of Science and Technology, Kochi, Kerala, India Hareesh N. Ramanathan Associate Professor, Faculty of Tourism and Rural Development in Pozega, Josip Juraj Strossmayer University of Osijek, Osijek, Croatia Marko Šostar You can also search for this author in PubMed Google Scholar ContributionsAbhirami Subash: Original data drafting, editing and reviewing. Hareesh N Ramanathan: VOSviewer bibliographic data analysis, review and supervision. Marko Šostar: Review, editing, visualization, and supervision. Corresponding authorCorrespondence to Marko Šostar . Ethics declarationsEthics approval and consent to participate. The study was conducted in accordance with the Declaration of Helsinki. Consent for publicationNot applicable. Competing interestsThe author declares no conflict of interests. Additional informationPublisher's note. Springer Nature remains neutral with regard to jurisdictional claims in published maps and institutional affiliations. Rights and permissionsOpen Access This article is licensed under a Creative Commons Attribution 4.0 International License, which permits use, sharing, adaptation, distribution and reproduction in any medium or format, as long as you give appropriate credit to the original author(s) and the source, provide a link to the Creative Commons licence, and indicate if changes were made. The images or other third party material in this article are included in the article's Creative Commons licence, unless indicated otherwise in a credit line to the material. If material is not included in the article's Creative Commons licence and your intended use is not permitted by statutory regulation or exceeds the permitted use, you will need to obtain permission directly from the copyright holder. To view a copy of this licence, visit http://creativecommons.org/licenses/by/4.0/ . Reprints and permissions About this articleSubash, A., Ramanathan, H.N. & Šostar, M. From catch to consumer: enhancing seafood processing management with Industry 4.0 innovations. Discov Food 4 , 43 (2024). https://doi.org/10.1007/s44187-024-00115-6 Download citation Received : 29 March 2024 Accepted : 31 May 2024 Published : 18 June 2024 DOI : https://doi.org/10.1007/s44187-024-00115-6 Share this articleAnyone you share the following link with will be able to read this content: Sorry, a shareable link is not currently available for this article. Provided by the Springer Nature SharedIt content-sharing initiative - Find a journal
- Publish with us
- Track your research
The fourth industrial revolution (Industry 4.0): technologies disruption on operations and supply chain managementInternational Journal of Operations & Production Management ISSN : 0144-3577 Article publication date: 20 November 2019 Issue publication date: 20 November 2019 Koh, L. , Orzes, G. and Jia, F.(J). (2019), "The fourth industrial revolution (Industry 4.0): technologies disruption on operations and supply chain management", International Journal of Operations & Production Management , Vol. 39 No. 6/7/8, pp. 817-828. https://doi.org/10.1108/IJOPM-08-2019-788 Emerald Publishing Limited Copyright © 2019, Emerald Publishing Limited 1. The fourth industrial revolution (Industry 4.0): technologies disruption on operations and supply chain management1.1 context. During the last five years, journals in robotics, electronics, computer science and production engineering have devoted significant attention to Industry 4.0 and related subjects, including additive manufacturing/3D printing, intelligent manufacturing and big data ( Lee et al. , 2014 ; Xi et al. , 2015; Pfeiffer et al. , 2016 ; Mosterman and Zander, 2016 ; Chen and Zhang, 2015 ; Jia et al. , 2016 ). A systematic literature review on Industry 4.0 or on some of its specific technologies (e.g. additive manufacturing) is provided by Liao et al. (2017) , Strozzi et al. (2017) and Khorram Niaki and Nonino (2017) among others. Although prominent scholars have acknowledged the relevance of Industry 4.0 for management in general, as well as for Operations and Production Management (O&PM) specifically ( Brennan et al. , 2015 ; Fawcett and Waller, 2014 ; Holmström and Romme, 2012 ; Melnyk et al. , 2018 ), relatively little consideration has been given to these topics by mainstream O&PM journals, especially on Industry 4.0 technologies’ disruption on operations and supply chain management. A few prominent exceptions are represented by the recent attempts to shed lights on: the link between Industry 4.0 and lean manufacturing ( Buer et al. , 2018 ; Tortorella and Fettermann, 2018 ); the link between Internet of Things (IoT) and supply chain management ( Ben-Daya et al. , 2017 ); the impact of additive manufacturing on supply chain processes and performances ( Liu et al. , 2014 ; Oettmeier and Hofmann, 2016 ; Li et al. , 2017 ); and the short-term supply chain scheduling in smart factories ( Ivanov et al. , 2016 ). While in the past there were very few pilot Industry 4.0 projects, the number of applications has significantly increased, both in terms of demonstration and “real” factories hence give rise to more empirical studies. Demonstration factories include Factory 2050 at the University of Sheffield (UK), Demonstration Factory at Aachen University (Germany), TRUMPF Group Factory in Chicago (USA) and SmartFactoryKL in Kaiserslautern (Germany), whilst “real” factories are at Audi’s Ingolstadt factory (Core77, 2016), Arla Foods ( ARC, 2016 ), Siemens’ Amberg plant ( Siemens, 2016 ) and Bosch’s Feuerbach plant in Stuttgart ( Automotive World, 2016 ). A recent survey conducted by PwC on more than 2,000 companies from 26 countries showed an overall adoption rate of Industry 4.0 concepts (e.g. digitization and integration) of 33 percent, and forecasted that it will reach 72 percent by 2020 ( PwC, 2015 ). This growth will be further fostered by the funding and innovation plans launched by several countries leading this industrial revolution, e.g., Manufacturing USA in the USA, Industrie du Futur in France, Industrie 4.0 in Germany, Industria 4.0 in Italy, Made in China 2025, Made Smarter UK. It is argued that different industrial sectors have different pace of adopting Industry 4.0. for instance, the aerospace sector has sometimes been characterized as “too low volume for extensive automation” however Industry 4.0 principles have been investigated by several aerospace companies, technologies have been developed to improve productivity where the upfront cost of automation cannot be justified, one example of this is the aerospace parts manufacturer Meggitt PLC’s project, M4. Here, the fourth industrial revolution (Industry 4.0) refers to the “confluence of technologies ranging from a variety of digital technologies (e.g. 3D printing, IoT, advanced robotics) to new materials (e.g. bio or nano-based) to new processes (e.g. data driven production, Artificial Intelligence, synthetic biology)” ( OECD, 2016 ). These technologies have the potential to revolutionize operations and supply chain management ( Brennan et al. , 2015 ; Holmström et al. , 2016 ; Rüßmann et al. , 2015 ; Fawcett and Waller, 2014 ; Waller and Fawcett, 2013 ). Industry 4.0 is not merely about integrating technologies, but it is about the whole concept of how future customer demands, resources and data are shared, owned, used, regenerated, exploited, organized and recycled to make a product or deliver a service, faster, cheaper, more efficiently and more sustainably ( Spath, 2013 ). As such, Industry 4.0 requires a rethinking and shift in mindset of how products are manufactured and services are produced, distributed/supplied, sold and used in the supply chain; thus, it will drive significant structural theoretical evolution and revolution for operations and supply chain management. Whilst classical theories such as resource based view, institutional theory, chaos theory, systems theory, stakeholder theory, transaction economic cost theory, evolutionary theory to name a few may need reshaping, the issues of trust will become prominent in such a disruptive digital environment, driving major evolvement of technological singularity in the transformation process, where blockchain may play a central role with IoT and Artificial Intelligence (AI) ( Carter and Koh, 2018 ). 2. IntroductionSo far, all the industrial revolutions that took place in the past two centuries is promoted by altering production mode enabled by a specific emerging technology at that time ( Liao et al. , 2017 ). The arrival of steam engine promoted the first industrial revolution; the application of electricity led to the second revolution, and the widespread use of information technology and electronics products support the third revolution ( Liao et al. , 2017 ). The recent popularization of the IoT and cyber-physical system (CPS) ( Khaitan and McCalley, 2014 ) has attracted the attention of both enterprise and academics. Leveraging those two emerging technologies is promising to enable the higher level of connection between information, products and people ( Ibarra et al. , 2018 ), thereby making contributions to the current production mode. This phenomenon is considered as the fourth industrial revolution, also known as industry 4.0, which is about to bring about an extensive range of innovation from a variety of digital technologies ( Lu, 2017 ), advanced materials ( Schumacher et al. , 2016 ), innovative products ( Pereira and Romero, 2017 ), to new manufacturing processes ( Wagner et al. , 2017 ). Industry 4.0 is an emerging concept deriving from technological advancement and disruptive developments in the industrial sector worldwide in the past few years (Dallasega et al. , 2017). It defines a methodology applying emerging technologies to revolutionize the current production that transits from machine dominant manufacturing to digital manufacturing ( Oztemel and Gursev, 2018 ). Some consider it as the integration of technologies such as CPS, IoT, Big Dara and Cloud manufacturing ( Pereira and Romero, 2017 ). However, there is a discourse arguing that industry 4.0 is not only regarding integrating technologies but concerning the whole concept of how to acquire, share, use, organize data and resource to make the product/service deliver faster, cheaper, more effective and more sustainable ( Piccarozzi et al. , 2018 ). As the interest in the Industry 4.0 research is growing rapidly, these studies do not limit their focus on industry 4.0 itself, but seek to find the relationship between industry 4.0 and other topics. For instance, Piccarozzi et al. (2018) try to link industry 4.0 with management studies; Dallasega et al. (2018) investigate industry 4.0 in the context of the supply chain. Müller et al. (2018) and Kamble et al. (2018) explore the relationship between industry 4.0 and sustainable development. This position paper intends to summarize the major topics in the current research regarding Industry 4.0 and charts key thematic future research directions and paradigms. In the following section, the paradigms and principles of industry 4.0 are concluded. Five technologies that are widely discussed in the current research are identified and the outcomes of industry 4.0 are discussed at the end of this position paper. 3. Paradigms in industry 4.0According to Weyer et al. (2015) , industry 4.0 can be subdivided into three paradigms: the smart product, the smart machine and the augmented operator. This conclusion of the major paradigm of industry 4.0 is also agreed by Longo et al. (2017) and Mrugalska and Wyrwicka (2017) . The first paradigm is the smart products, it refers to objects and machines that are equipped with sensors and microchips, controlled by software, and connected to the internet ( Lu, 2017 ; Kamble et al. , 2018 ). Smart products can store the operational data and requirements independently, and further, the product can inform the machine-related manufacturing information, for instance, when to produce, where to produce, or what parameter should be adopted to complete the product manufacturing. In this case, smart product shifts the role of the workpiece in a system from passive to an active part ( Loskyll et al. , 2012 ). The second paradigm is the Smart Machine. It refers to a device equipped with machine-to-machine and/or cognitive computing technologies (i.e. AI and machine learning (ML)). Through leveraging these technologies, machines can reason, problem-solve, make decision ad eventually take action. Smart machine brought decentralized self-organization, thus replacing the previous traditional production hierarchy ( Mrugalska and Wyrwicka, 2017 ). In such innovative system, the use of open networks and semantic descriptions allow the communication among the autonomic components ( Oztemel and Gursev, 2018 ), while the local control intelligence communicate with other devices, production modules and products, thereby, contributing to the improvement of flexibility and modularity of the production line ( Pereira and Romero, 2017 ). The third paradigm of industry 4.0 is the augmented operator. This concept emphasizes the technological support of the worker in the production system with higher flexibility and modularity ( Weyer et al. , 2015 ). Mrugalska and Wyrwicka (2017) state that augmented operator addresses the knowledge automation in the system, therefore making them the most flexible and adaptive part in the production system. Workers in such production system are likely to encounter with varieties of tasks including specification, monitoring and verification of production strategy. Meanwhile, they may have to annually intervene in the self-organized production system. Under the support of mobile, context-sensitive user interfaces and user-focused assistance system ( Gorecky et al. , 2014 ), such workers play the role of strategic decision-makers and flexible problem-solvers in the circumstance of increasing technical complexity ( Mrugalska and Wyrwicka, 2017 ). 4. Design principles in industry 4.0Based on the three paradigms mentioned above, some researchers further conclude six principles that should be considered when designing the implementation of industry 4.0 ( Oztemel and Gursev, 2018 ). Those principles include interoperability, virtualization, decentralization, real-time capability, service orientation and modularity ( Lu, 2017 , Oztemel and Gursev, 2018 ). Kamble et al. (2018) conduct a systematic literature review to develop a framework of sustainable industry 4.0 and further justify the role of these principles on industry 4.0 implementation. First, interoperability is the first principle for industry 4.0. Interoperability refers to the ability of two systems to communicate with and understand each other and use the functions of one another ( Hermann et al. , 2016 ; Lu, 2017 ). It addresses the capability of data exchanging and information and knowledge sharing among systems ( Lu, 2017 ). It is assumed that interoperability is the key advantages of industry 4.0 as it ensures the connection and communication among products, machines and humans ( Mrugalska and Wyrwicka, 2017 ) throughout the diversified autonomous procedure ( Lu, 2017 ). Further, Lu (2017) proposes a framework of interoperability of industry 4.0 and concludes four levels of interoperability in industry 4.0, including operational, systematic, technical and semantic interoperability. The author gives specific explanations for each level of interoperability. Operational interoperability indicates the concepts, standards, languages and relationships within the system. Systematic interoperability describes the methodologies, standards and models; technical interoperability illustrates tools and platforms for technical development, and the semantic interoperability ensures the exchanged information is well understood among different groups. Qin et al. (2016) confirmed that interoperability constructs a trusted environment in a manufacturing system, in which information is accurately and swiftly shared among partners ( Kamble et al. , 2018 ), therefore resulting in a cost-saving operation with higher productivity ( Lu, 2017 ). Virtualization is used for process monitoring and machine-to-machine communication. It indicates that devices have the capability of monitoring the physical process. The sensor data is linked to virtual plant models and simulation models, thus constructing the virtual copy of physical objects ( Mrugalska and Wyrwicka, 2017 ). Meanwhile, each device can be virtualized and become a part of the plant model. The virtual model can simulate various scenarios based on the monitored data. Once the potential risks or failures are detected in the virtual models, operators are informed and they can take the pre-emptive action ( Kamble et al. , 2018 ), thus reducing the actual error rate and smoothing the inter-company operations ( Brettel et al. , 2014 ). Third, decentralization denotes that companies, operation staff, and even devices are able to make independent decision rather than depending on the centralized decision-making, It can be achieved with the use of embedded computer, which provides the operation staff or devices the capability of individual control and independent decision-making ( Marques et al. , 2017 ). As the development of customization and product variety, the flexible production line is expected to be extensively adopted. Overall control of the production line is less advisable. However, the embedded control system can empower each device or the unit of the device to make independent decisions, thus making the decision-making efficient and offering more flexibility ( Kamble et al. , 2018 ). Fourth, real-time capability refers to the immediacy of data collection and analysis, and the real-time of data transmission. Smart factory requires continuous real-time data monitoring and analyzing, to detect the errors timely and satisfy the new demand. The collection of real-time data relies on big data technology ( Kamble et al. , 2018 ). The huge amount of data regarding machines, equipment, and products are collected from factories, and data regarding customers are collected from multiple sources such as social media or outlets. The analysis of those real-time data may alter the ways of decision-making and pose an impact on the profitability of the companies implementing industry 4.0. Fifth, service orientation required that devices are capable of satisfying the needs of users through the internet of service. As all the entities in the production system are interconnected, and therefore, the concept of the product will extend from the product itself to product-service ( Lasi et al. , 2014 ). Service orientation indicates that product should be considering the users’ practical needs, such as user-friendly or convenience for maintenance, at the very beginning of product design. Moreover, through service orientation, corporate can achieve flexibility and agility and thus to have a quick response to the market change ( Kamble et al. , 2018 ). Sixth, modularity refers to the device or the components of a device is produced following standards. Therefore, they can be assembled, replaced and expanded as needed in the modular production system ( Qin et al. , 2016 ). In this case, modularity provides smart factories with the capability of adapting capacity at a lower cost to cope with seasonal fluctuation and changes in production needs ( Mrugalska and Wyrwicka, 2017 ). 5. Technologies in industry 4.0Lu (2017) defines industry 4.0 as an integrated, adapted, optimized, service-oriented and interoperable manufacturing process in which algorithms, big data and high technologies are included. Technologies are considered as the very heart of industry 4.0 as the interconnection in the industry 4.0 is supported by the adoption of software, sensor, processor and communication technologies ( Bahrin et al. , 2016 ). Five technologies are frequently discussed in the literature: IoT, big data analytics, cloud, 3D printing and robotic systems ( Piccarozzi et al. , 2018 ; Kamble et al. 2018 ), where technologies such as AI, ML, digital twin and 5G are emerging. Internet of Things (IoT)The IoT is an emerging industrial ecosystem. It facilitates the combination of intelligent machines, advanced predictive analytics and machine-human collaboration, aiming at promoting productivity, efficiency and reliability ( Kamble et al. , 2018 ). In industry 4.0, IoT can support the smart factory. It can lead to the creation of virtual networks to support the smart factory ( Xu et al. , 2018 ); meanwhile, it provides the factory with the ability to collect real-time data and transmit the data swiftly ( Yang et al. , 2017 ). Therefore, it enables the remote operation of manufacturing activities and affects collaboration among stakeholders ( Yang et al. , 2017 ). IoT can benefit the integration and coordination of product and information flow ( Tao et al. , 2014 ), and enable the decentralization of decision-making, interconnected devised can perform automatic analytics and decision-making, thus improving the responsiveness to the environment change ( Wang et al. , 2014 ). Big data analyticsManufacturing companies have realized that data analytics capabilities are imperative for their competitive advantage in the era of digitization. Therefore, they devote themselves to improving skills for algorithms development and data interpretation ( Lee et al. , 2017 ). Big data analytics and technologies can promote data collection from multiple sources, and the ability of comprehensive data analysis and real-time decision making based on the data analysis results ( Bahrin et al. , 2016 ). It has been widely adopted in manufacturing to monitor the process. Also, big data is used for failure detection, thus supporting new capabilities such as predictive analytics ( Lee et al. , 2017 ). Data quality and qualified data analysis capabilities are key to achieve the desired outcomes of big data analytics ( Kamble et al. , 2018 ). Therefore, leveraging the intelligence in big data to improve agility will require new challenges, for example how to ensure the data consistency and confidentiality in a long and complex supply chain ( Kamble et al. , 2018 ). Cloud computing is a computing technology. Cloud computing centers can store and compute a huge amount of data, therefore promoting the manufacturing and production and further bringing organizations higher performance and lower cost ( Mitra et al. , 2017 ). Cloud computing is supported by virtualization technology, as it provides cloud computing with resource pooling, resource sharing, dynamic allocation, flexible extension and other capabilities ( Xu et al. , 2018 ). Xu et al. (2018) also address the usefulness of cloud computing in facilitating efficient data exchange and sharing. Through cloud computing, data can be stored in either private cloud or public cloud servers, and thus cloud computing can promote complex decision-making ( Xu et al. , 2018 ). Cloud-based manufacturing is key to the success Industry 4.0 implementation. It enables the modularization and service-orientation in the field of manufacturing ( Xu et al. , 2018 ), where system orchestration and sharing of service and components are essential considerations and are affected by modularization and service-orientation ( Xu et al. , 2018 ). Branger and Pang (2015) assumed that cloud manufacturing is expected to be the next paradigm in manufacturing in Industry 4.0. 3D printing3D printing relies on additive manufacturing (as opposed to subtractive manufacturing). Final products in 3D printing are built up with successive layers of materials ( Oztemel and Gursev, 2018 ), thus avoiding the component assembly in the production process. Additive manufacturing techniques can make contributions to industry 4.0 in terms of offering organizations construction advantages, as it allows to produce small batches of customized products with complex and lightweight design ( Kamble et al. , 2018 ). Chen and Lin (2017) state that the exploitation of 3D technology can optimize smart manufacturing and lean manufacturing. However, there are technical challenges in the use of 3D printing, namely, limited accuracy and productivity, and limited available material ( Chen and Lin, 2017 ). Because of the technical challenges, additive manufacturing (3D printing) is still in the initial stage. However, once the challenges have been solved, it is expected to see wider adoption of this technology in Industry 4.0 ( Kamble et al. , 2018 ). Robotic systemsHowever, robotics has been used for production in many manufacturing industries, the modern robotics systems are more flexible, autonomous and smart and are able to communicate and cooperate with one another and even have learning ability ( Kamble et al. , 2018 ), leading to the next generation of robotic systems, namely, cobot (collaborative robots). Pei et al. (2017) state that the modern robotics can perform well in most of the processes in the smart factory, for instance, Mueller et al. (2017) proposed that it is feasible to use programmable dual-arm robots to efficiently distribute and allocate materials in the assembly line. Therefore, the application of modern robots can provide the factory with cost advantages and a wide range of capabilities ( Pei et al. , 2017 ). To ensure the safe operation of the robotics system, a device named safety eye is equipped. Once the device has detected any disturbance in the operation, it will stop the robot and will not reactivate the robot before the operators remove the objects that disturb the operation ( Kamble et al. , 2018 ). 6. Outcomes of industry 4.0Considering industry 4.0 can revolutionize the products and manufacturing system in terms of operation, product, design, production processes and services across the supply chain, it is expected that implementing industry 4.0 can positively impact the industry, markets and multiple participants (Dallasega et al. , 2017). Pereira and Romero (2017) conclude six areas on which industry 4.0 may exert influence. Those areas include: industry, products and service, business model and market, economy, work environment and skills development. Kamble et al. (2018) further link industry 4.0 with sustainable development and argued that industry 4.0 can generate sustainable outcomes in terms of environmental, social and economic. Industry 4.0 has brought manufacturing industry new decentralized and digitalized production patterns, in which the production elements are highly autonomous, and therefore they can trigger actions and respond to the environment change independently ( Pereira and Romero, 2017 ). Industry 4.0 also promote the integration of products and processes, thus transforming the production pattern from mass production to mass customization ( Lu, 2017 ). Additionally, production processes and operations are significantly affected by the emergence of smart factories and emerging technologies, such as IoT, 3D printing and robotic systems. In this case, Industry 4.0 can improve the flexibility in operations and efficiency in resource allocation ( Pereira and Romero, 2017 ). Dallasega et al. (2018) state that Industry 4.0 will not only affect the productivity in the manufacturing industry but also influence the entire supply chain from product development and manufacturing process to the product distribution. Products and services are also affected by industry 4.0. The principle of modularisation makes the products modular and configurable, and as a result, products and services are more customized to satisfy specific customer needs ( Jazdi, 2014 ). Industry 4.0 has brought a number of new disruptive technologies that have altered the approaches of delivering products or services, hence affecting the traditional business models and encouraging the new business models ( Pereira and Romero, 2017 ). For instance, system integration and complexity in industry 4.0 will result in the emergence of more complex and digital market models, in which the barriers between information and physical structure are reduced ( Ibarra et al. , 2018 ). Industry 4.0 is transforming jobs and required skills, which have impacts on the working environment and skills development. With more robots and smart machines is involved in the daily operation, the physical and virtual world are fusing together, thus launching transformation in the working environment. For example, as human-machine interfere requires the communication among smart machines, smart products and employees, ergonomic issues should be considered in the future system should stress the workers and their importance in the system ( Pereira and Romero, 2017 ). For skills development, as in the context of industry 4.0, interdisciplinary thinking and qualified skills in the social and technical field are required. These new competencies should be included in the employee training and education ( Pereira and Romero, 2017 ), to make workers and managers well prepared for this new industrial paradigm. Moreover, Kamble et al. (2018) state that Industry 4.0 can lead to sustainable development. With the support of cloud computing and big data analytics, organizations can achieve cost reduction and lean production, thus realising the economic sustainability; Employing technologies such as sensing, detection and tracing analysis can help to mitigate the problem of industrial waste disposal, which facilitates the environmental sustainability; technologies (risk maps or wearable technologies) for improving the safety of employees in hazardous work areas helps to ensure the process safety and promote the social sustainability. 7. Methodological approaches adopted by Industry 4.0 researchIndustry 4.0 literature is characterized by a prevalence of conceptual papers. Piccarozzi et al. (2018) found for instance in their systematic review on Industry 4.0 in management studies 54 percent of conceptual papers, mainly literature reviews and developments of models/frameworks. As far as empirical papers are concerned, qualitative methods (mainly case studies) and quantitative methods (surveys) are almost equally adopted (25 vs 21 percent, respectively). An agreed definition and operationalization of the Industry 4.0 construct is missing ( Culot et al. , 2018 ). While some authors have indeed sought to develop maturity models and readiness indexes, which identify incremental levels of Industry 4.0 implementation (for a review see Mittal et al. , 2018 ), Industry 4.0 literature still relies on different operationalizations of the concept. As an example, the bunch of technologies considered as Industry 4.0 varies significantly from one paper to the other. This poses serious limitations to theory building and research comparability. Finally, Industry 4.0 papers belong to a wide set of disciplinary domains. Muhuri et al. (2019) identified in their bibliometric analysis of Industry 4.0 the top 10 subject areas in the Scopus database. At the first place there is Engineering (65 percent [1] ), followed by Computer Science (45 percent), Business, Management and Accounting (16 percent) and Decision Sciences (14 percent). While these disciplines were the most important ones also in the previous investigation conducted by Liao et al. (2017) , their relative importance has significantly changed (Engineering was at the second place after Computer Science; Business, Management and Accounting and Decision Sciences were significantly less frequent). Besides this wide set of disciplines involved, there is however a limited number of interdisciplinary papers. 8. Suggestions for future Industry 4.0 research – methodological approachAs we pointed out in this position paper, Industry 4.0 research so far is still characterized by a prevalence of conceptual papers in the operations and production field. However paradigms, design principles and technologies prevalent to industry 4.0 have been examined. Whilst this might be partially justified by the novelty of the topic and the consequent limited adoption by companies (the Industry 4.0 concept was indeed introduced at the Hannover Fair in 2011), the scientific research cannot overlook the contact with the industrial world. One of the main challenges for future Industry 4.0 research is therefore to carry out more empirical investigations as well as large-scale data analysis. For this reason, we decided not to accept any conceptual contribution in our special issue (even though we received some high-quality conceptual papers). Alongside the traditional empirical methods (i.e. case study and survey), other exploratory methodologies – such as Delphi studies or focus groups – could bring significant insights given the interdisciplinary and “futuristic” nature of the topic. A further potential methodological limitation of current Industry 4.0 research is the absence of agreed definitions and operationalizations of the main constructs. Without these operationalizations, there is a risk that the significant relationships observed are just due to the specific definitions considered and are not reproducible in other studies. A second significant challenge for future Industry 4.0 research is therefore to define the main Industry 4.0 constructs (e.g. Industry 4.0 adoption, Industry 4.0 maturity, Industry 4.0 readiness) and empirically validate them. This challenge will not be easy since both the technological landscape and the application fields of Industry 4.0 are rapidly evolving. Researchers should however find a way to define a common set of constructs to support further theory building and theory testing efforts. The issue pointed out above is particularly significant in quantitative research, which is usually based on closed-ended questions or secondary data (requiring a precise operationalization of the measured constructs). The almost equal representation of qualitative and quantitative research might in this sense signal a potential issue. We therefore think that qualitative theory building papers should be particularly welcome in this stage, to develop a set of constructs and relationships to be tested on larger samples in a later stage. Finally, Industry 4.0 is a highly interdisciplinary topic, involving a wide set of knowledge domains (e.g. automatic controls, robotics, sensors, computer science, and management) and actors (e.g. researchers, companies, technology providers, policy makers, schools). The successful transition toward Industry 4.0 requires indeed a joint effort of the above-mentioned actors to create a successful ecosystem ( Xu et al. , 2018 ). Interdisciplinary research should therefore be significantly encouraged at all levels. First, Industry 4.0 researchers should for instance try to aim in their paper more at the policy makers and the managers. Research should indeed support the different authorities to take better decision to support the digital transformation. Second, authors from different disciplines or affiliations (universities, applied research centers, companies, technology providers, governments and regulatory bodies) should try to systematically integrate the different perspectives and point of views. Finally, the reviewing and editorial board of journals might also be broadened/hybridized by involving experts from the industrial and the policy making worlds. 9. ConclusionThe purpose of this position paper is to summarize the major topics of recent research on industry 4.0. First, three paradigms and six principles of industry 4.0 are identified, and five technologies that are frequently discussed in industry 4.0 are concluded. The outcomes and impacts of industry 4.0 are discussed at the end. In addition, the methodological approaches in industry 4.0 research has been discussed, and future research directions and paradigms of industry 4.0 methodological approach have been proposed. Although industry 4.0 has been widely discussed from multiple perspectives, as technology advancement still takes place constantly, thus continuously shaping the industry and organizations, there are abundant research opportunities in this topic. Meanwhile, with the increasingly in-depth understanding of industry 4.0, there are more research potentials to combine industry 4.0 with other research fields, to further investigate the industry 4.0 with a wider scope. The sum of percentages exceeds 100 percent since some papers are categorized by Scopus in more than one category. ARC ( 2016 ), “ Arla foods reduces packing machine integration costs using PackML ”, available at: www.arcweb.com/Blog/Post/519/Arla-Foods-Reduces-Packing-Machine-Integration-Costs-Using-PackML Automotive World ( 2016 ), “ Industry 4.0 and the rise of smart manufacturing ”, available at www.automotiveworld.com/analysis/industry-4-0-rise-smart-manufacturing/ Bahrin , M.A.K. , Othman , M.F. , Azli , N.N. and Talib , M.F. ( 2016 ), “ Industry 4.0: a review on industrial automation and robotic ”, Jurnal Teknologi , Vol. 78 Nos 6-13 , pp. 137 - 143 . Ben-Daya , M. , Hassini , E. and Bahroun , Z. ( 2017 ), “ Internet of things and supply chain management: a literature review ”, International Journal of Production Research , doi: 10.1080/00207543.2017.1402140 . Branger , J. and Pang , Z. ( 2015 ), “ From automated home to sustainable, healthy and manufacturing home: a new story enabled by the Internet-of-Things and Industry 4.0 ”, Journal of Management Analytics , Vol. 2 No. 4 , pp. 314 - 332 . Brennan , L. , Ferdows , K. , Godsell , J. , Golini , R. , Keegan , R. , Kinkel , S. , Srai , S.J. and Taylor , M. ( 2015 ), “ Manufacturing in the world: where next? ”, International Journal of Operations & Production Management , Vol. 35 No. 9 , pp. 1253 - 1274 . Brettel , M. , Friederichsen , N. , Keller , M. and Rosenberg , M. ( 2014 ), “ How virtualization, decentralization and network building change the manufacturing landscape: an Industry 4.0 perspective ”, International Journal of Mechanical, Industrial Science and Engineering , Vol. 8 No. 1 , pp. 37 - 44 . Buer , S.V. , Strandhagen , J.O. and Chan , F.T. ( 2018 ), “ The link between Industry 4.0 and lean manufacturing: mapping current research and establishing a research agenda ”, International Journal of Production Research , Vol. 56 No. 8 , pp. 2924 - 2940 . Carter , C. and Koh , L. ( 2018 ), Blockchain Disruption on Transport: Are you Decentralised Yet? , Transport Systems Catapult and The University of Sheffield . Chen , G. and Zhang , J. ( 2015 ), “ Study on training system and continuous improving mechanism for mechanical engineering ”, The Open Mechanical Engineering Journal , Vol. 9 No. 1 , pp. 7 - 14 . Chen , T. and Lin , Y.C. ( 2017 ), “ Feasibility evaluation and optimization of a smart manufacturing system based on 3D printing: a review ”, International Journal of Intelligent Systems , Vol. 32 No. 4 , pp. 394 - 413 . Culot , G. , Nassimbeni , G. , Orzes , G. and Sartor , M. ( 2018 ), “ Industry 4.0: Ambiguities and limits of current definitions ”, Decision Sciences for the New Global Economy, 9th Annual EDSI Conference Proceedings . Dallasega , P. , Rauch , E. and Linder , C. ( 2018 ), “ Industry 4.0 as an enabler of proximity for construction supply chains: a systematic literature review ”, Computers in Industry , Vol. 99 , pp. 205 - 225 . Fawcett , S.E. and Waller , M.A. ( 2014 ), “ Can we stay ahead of the obsolescence curve? On inflection points, proactive preemption, and the future of supply chain management ”, Journal of Business Logistics , Vol. 35 No. 1 , pp. 17 - 22 . Gorecky , D. , Schmitt , M. , Loskyll , M. and Zühlke , D. ( 2014 ), “ Human-machine-interaction in the industry 4.0 era ”, 2014 12th IEEE International Conference on Industrial Informatics (INDIN), IEEE , pp. 289 - 294 . Hermann , M. , Pentek , T. and Otto , B. ( 2016 ), “ Design principles for industrie 4.0 scenarios ”, 2016 49th Hawaii International Conference on System Sciences (HICSS), IEEE , pp. 3928 - 3937 . Holmström , J. and Romme , A.G.L. ( 2012 ), “ Guest editorial: five steps towards exploring the future of operations management ”, Operations Management Research , Vol. 5 No. 1 , pp. 37 - 42 . Holmström , J. , Holweg , M. , Khajavi , S.H. and Partanen , J. ( 2016 ), “ The direct digital manufacturing (r) evolution: definition of a research agenda ”, Operations Management Research , Vol. 9 No. 1 , pp. 1 - 10 . Ibarra , D. , Ganzarain , J. and Igartua , J.I. ( 2018 ), “ Business model innovation through Industry 4.0: a review ”, Procedia Manufacturing , Vol. 22 , pp. 4 - 10 . Ivanov , D. , Dolgui , A. , Sokolov , B. , Werner , F. and Ivanova , M. ( 2016 ), “ A dynamic model and an algorithm for short-term supply chain scheduling in the smart factory industry 4.0 ”, International Journal of Production Research , Vol. 54 No. 2 , pp. 386 - 402 . Jazdi , N. ( 2014 ), “ Cyber physical systems in the context of Industry 4.0 ”, 2014 IEEE International Conference on Automation, Quality and Testing, Robotics, IEEE , pp. 1 - 4 . Jia , F. , Wang , X. , Mustafee , N. and Hao , L. ( 2016 ), “ Investigating the feasibility of supply chain-centric business models in 3D chocolate printing: a simulation study ”, Technological Forecasting and Social Change , Vol. 102 , pp. 202 - 213 . Kamble , S.S. , Gunasekaran , A. and Gawankar , S.A. ( 2018 ), “ Sustainable Industry 4.0 framework: a systematic literature review identifying the current trends and future perspectives ”, Process Safety and Environmental Protection , Vol. 117 , pp. 408 - 425 . Khaitan , S.K. and McCalley , J.D. ( 2014 ), “ Design techniques and applications of cyberphysical systems: a survey ”, IEEE Systems Journal , Vol. 9 No. 2 , pp. 350 - 365 . Khorram Niaki , M. and Nonino , F. ( 2017 ), “ Additive manufacturing management: a review and future research agenda ”, International Journal of Production Research , Vol. 55 No. 5 , pp. 1419 - 1439 . Lasi , H. , Fettke , P. , Kemper , H.G. , Feld , T. and Hoffmann , M. ( 2014 ), “ Industry 4.0 ”, Business & Information Systems Engineering , Vol. 6 No. 4 , pp. 239 - 242 . Lee , J. , Kao , H.A. and Yang , S. ( 2014 ), “ Service innovation and smart analytics for industry 4.0 and big data environment ”, Procedia CIRP , Vol. 16 , pp. 3 - 8 . Lee , J.Y. , Yoon , J.S. and Kim , B.H. ( 2017 ), “ A big data analytics platform for smart factories in small and medium-sized manufacturing enterprises: an empirical case study of a die casting factory ”, International Journal of Precision Engineering and Manufacturing , Vol. 18 No. 10 , pp. 1353 - 1361 . Li , Y. , Jia , G. , Cheng , Y. and Hu , Y. ( 2017 ), “ Additive manufacturing technology in spare parts supply chain: a comparative study ”, International Journal of Production Research , Vol. 55 No. 5 , pp. 1498 - 1515 . Liao , Y. , Deschamps , F. , Loures , E.D.F.R. and Ramos , L.F.P. ( 2017 ), “ Past, present and future of Industry 4.0-a systematic literature review and research agenda proposal ”, International Journal of Production Research , Vol. 55 No. 12 , pp. 3609 - 3629 . Liu , P. , Huang , S.H. , Mokasdar , A. , Zhou , H. and Hou , L. ( 2014 ), “ The impact of additive manufacturing in the aircraft spare parts supply chain: supply chain operation reference (SCOR) model based analysis ”, Production Planning & Control , Vol. 25 Nos 13-14 , pp. 1169 - 1181 . Longo , F. , Nicoletti , L. and Padovano , A. ( 2017 ), “ Smart operators in industry 4.0: a human-centered approach to enhance operators’ capabilities and competencies within the new smart factory context ”, Computers & Industrial Engineering , Vol. 113 , pp. 144 - 159 . Loskyll , M. , Heck , I. , Schlick , J. and Schwarz , M. ( 2012 ), “ Context-based orchestration for control of resource-efficient manufacturing processes ”, Future Internet , Vol. 4 No. 3 , pp. 737 - 761 . Lu , Y. ( 2017 ), “ Industry 4.0: a survey on technologies, applications and open research issues ”, Journal of Industrial Information Integration , Vol. 6 , pp. 1 - 10 . Marques , M. , Agostinho , C. , Zacharewicz , G. and Jardim-Gonçalves , R. ( 2017 ), “ Decentralized decision support for intelligent manufacturing in Industry 4.0 ”, Journal of Ambient Intelligence and Smart Environments , Vol. 9 No. 3 , pp. 299 - 313 . Melnyk , S.A. , Flynn , B.B. and Awaysheh , A. ( 2018 ), “ The best of times and the worst of times: empirical operations and supply chain management research ”, International Journal of Production Research , Vol. 56 Nos 1-2 , pp. 164 - 192 . Mitra , A. , Kundu , A. , Chattopadhyay , M. and Chattopadhyay , S. ( 2017 ), “ A cost-efficient one time password-based authentication in cloud environment using equal length cellular automata ”, Journal of Industrial Information Integration , Vol. 5 , pp. 17 - 25 . Mittal , S. , Khan , M. , Romero , D. and Wuest , T. ( 2018 ), “ A critical review of smart manufacturing and Industry 4.0 maturity models: implications for small and medium-sized enterprises (SMEs) ”, Journal of Manufacturing Systems , Vol. 49 , pp. 194 - 214 . Mosterman , P.J. and Zander , J. ( 2016 ), “ Industry 4.0 as a cyber-physical system study ”, Software & Systems Modeling , Vol. 15 No. 1 , pp. 17 - 29 . Mrugalska , B. and Wyrwicka , M.K. ( 2017 ), “ Towards lean production in industry 4.0 ”, Procedia Engineering , Vol. 182 , pp. 466 - 473 . Muhuri , P.K. , Shukla , A.K. and Abraham , A. ( 2019 ), “ Industry 4.0: a bibliometric analysis and detailed overview ”, Engineering Applications of Artificial Intelligence , Vol. 78 , pp. 218 - 235 . Müller , J.M. , Kiel , D. and Voigt , K.I. ( 2018 ), “ What drives the implementation of Industry 4.0? The role of opportunities and challenges in the context of sustainability ”, Sustainability , Vol. 10 No. 1 , p. 247 . OECD ( 2016 ), “ Enabling the next production revolution: the future of manufacturing and services – interim report ”, available at: www.oecd.org/mcm/documents/Enabling-the-next-production-revolution-the-future-of-manufacturing-and-services-interim-report.pdf Oettmeier , K. and Hofmann , E. ( 2016 ), “ Impact of additive manufacturing technology adoption on supply chain management processes and components ”, Journal of Manufacturing Technology Management , Vol. 27 No. 7 , pp. 944 - 968 . Oztemel , E. and Gursev , S. ( 2018 ), “ Literature review of Industry 4.0 and related technologies ”, Journal of Intelligent Manufacturing , pp. 1 - 56 . Pei , F.Q. , Tong , Y.F. , He , F. and Li , D.B. ( 2017 ), “ Research on design of the smart factory for forging enterprise in the industry 4.0 environment ”, Mechanics , Vol. 23 No. 1 , pp. 146 - 152 . Pereira , A.C. and Romero , F. ( 2017 ), “ A review of the meanings and the implications of the Industry 4.0 concept ”, Procedia Manufacturing , Vol. 13 , pp. 1206 - 1214 . Pfeiffer , T. , Hellmers , J. , Schön , E.M. and Thomaschewski , J. ( 2016 ), “ Empowering User Interfaces for Industrie 4.0 ”, Proceedings of the IEEE , Vol. 104 No. 5 , pp. 986 - 996 . Piccarozzi , M. , Aquilani , B. and Gatti , C. ( 2018 ), “ Industry 4.0 in management studies: a systematic literature review ”, Sustainability , Vol. 10 No. 10 , p. 3821 . PwC ( 2015 ), “ Industry 4.0: building the digital enterprise ”, available at: www.pwc.com/gx/en/industries/industries-4.0/landing-page/industry-4.0-building-your-digital-enterprise-april-2016.pdf Qin , J. , Liu , Y. and Grosvenor , R. ( 2016 ), “ A categorical framework of manufacturing for industry 4.0 and beyond ”, Procedia CIRP , Vol. 52 , pp. 173 - 178 . Rüßmann , M. , Lorenz , M. , Gerbert , P. , Waldner , M. , Justus , J. , Engel , P. and Harnisch , M. ( 2015 ), Industry 4.0: The Future of Productivity and Growth in Manufacturing Industries , Boston Consulting Group . Schumacher , A. , Erol , S. and Sihn , W. ( 2016 ), “ A maturity model for assessing Industry 4.0 readiness and maturity of manufacturing enterprises ”, Procedia CIRP , Vol. 52 , pp. 161 - 166 . Siemens ( 2016 ), “ ‘Industrie 4.0’: seven facts to know about the future of manufacturing ”, available at: www.siemens.com/innovation/en/home/pictures-of-the-future/industry-and-automation/digtial-factory-trends-industrie-4-0.html Spath , D. ( 2013 ), Produktionsarbeit der Zukunft – Industrie 4.0 , IOA , Stuttgart , available at: www.produktionsarbeit.de/content/dam/produktionsarbeit/de/documents/Fraunhofer-IAO-Studie_Produktionsarbeit_der_Zukunft-Industrie_4_0.pdf (accessed August 18, 2015 ). Strozzi , F. , Colicchia , C. , Creazza , A. and Noè , C. ( 2017 ), “ Literature review on the ‘Smart Factory’ concept using bibliometric tools ”, International Journal of Production Research , Vol. 55 No. 22 , pp. 6572 - 6591 . Tao , F. , Zuo , Y. , Da Xu , L. and Zhang , L. ( 2014 ), “ IoT-based intelligent perception and access of manufacturing resource toward cloud manufacturing ”, IEEE Transactions on Industrial Informatics , Vol. 10 No. 2 , pp. 1547 - 1557 . Tortorella , G.L. and Fettermann , D. ( 2018 ), “ Implementation of Industry 4.0 and lean production in Brazilian manufacturing companies ”, International Journal of Production Research , Vol. 56 No. 8 , pp. 2975 - 2987 . Wagner , T. , Herrmann , C. and Thiede , S. ( 2017 ), “ Industry 4.0 impacts on lean production systems ”, Procedia CIRP , Vol. 63 , pp. 125 - 131 . Waller , M.A. and Fawcett , S.E. ( 2013 ), “ Data science, predictive analytics, and big data: a revolution that will transform supply chain design and management ”, Journal of Business Logistics , Vol. 34 No. 2 , pp. 77 - 84 . Wang , C. , Bi , Z. and Da Xu , L. ( 2014 ), “ IoT and cloud computing in automation of assembly modeling systems ”, IEEE Transactions on Industrial Informatics , Vol. 10 No. 2 , pp. 1426 - 1434 . Weyer , S. , Schmitt , M. , Ohmer , M. and Gorecky , D. ( 2015 ), “ Towards Industry 4.0-standardization as the crucial challenge for highly modular, multi-vendor production systems ”, Ifac-Papersonline , Vol. 48 No. 3 , pp. 579 - 584 . Xu , L.D. , Xu , E.L. and Li , L. ( 2018 ), “ Industry 4.0: state of the art and future trends ”, International Journal of Production Research , Vol. 56 No. 8 , pp. 2941 - 2962 . Yang , C. , Lan , S. , Shen , W. , Huang , G.Q. , Wang , X. and Lin , T. ( 2017 ), “ Towards product customization and personalization in IoT-enabled cloud manufacturing ”, Cluster Computing , Vol. 20 No. 2 , pp. 1717 - 1730 . Further readingKagermann , H. ( 2015 ), “ Change through digitization – value creation in the age of Industry 4.0 ”, Management of Permanent Change , Springer Gabler , Wiesbaden , pp. 23 - 45 . Tjahjono , B. , Esplugues , C. , Ares , E. and Pelaez , G. ( 2017 ), “ What does industry 4.0 mean to supply chain? ”, Procedia Manufacturing , Vol. 13 , pp. 1175 - 1182 . Related articlesAll feedback is valuable. Please share your general feedback Report an issue or find answers to frequently asked questionsContact Customer Support InformationInitiativesYou are accessing a machine-readable page. In order to be human-readable, please install an RSS reader. All articles published by MDPI are made immediately available worldwide under an open access license. No special permission is required to reuse all or part of the article published by MDPI, including figures and tables. For articles published under an open access Creative Common CC BY license, any part of the article may be reused without permission provided that the original article is clearly cited. For more information, please refer to https://www.mdpi.com/openaccess . Feature papers represent the most advanced research with significant potential for high impact in the field. A Feature Paper should be a substantial original Article that involves several techniques or approaches, provides an outlook for future research directions and describes possible research applications. Feature papers are submitted upon individual invitation or recommendation by the scientific editors and must receive positive feedback from the reviewers. Editor’s Choice articles are based on recommendations by the scientific editors of MDPI journals from around the world. Editors select a small number of articles recently published in the journal that they believe will be particularly interesting to readers, or important in the respective research area. The aim is to provide a snapshot of some of the most exciting work published in the various research areas of the journal. Original Submission Date Received: . - Active Journals
- Find a Journal
- Proceedings Series
- For Authors
- For Reviewers
- For Editors
- For Librarians
- For Publishers
- For Societies
- For Conference Organizers
- Open Access Policy
- Institutional Open Access Program
- Special Issues Guidelines
- Editorial Process
- Research and Publication Ethics
- Article Processing Charges
- Testimonials
- Preprints.org
- SciProfiles
- Encyclopedia
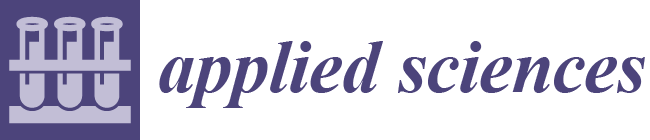 Article Menu - Subscribe SciFeed
- Recommended Articles
- Google Scholar
- on Google Scholar
- Table of Contents
Find support for a specific problem in the support section of our website. Please let us know what you think of our products and services. Visit our dedicated information section to learn more about MDPI. JSmol ViewerExamining the response to covid-19 in logistics and supply chain processes: insights from a state-of-the-art literature review and case study analysis. 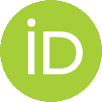 1. Introduction- RQ1 (scientific): How have researchers studied the impact of COVID-19 on logistics and supply chain processes? Which industrial sectors were mostly studied and why? Which additional topics can be related to COVID-19 and logistics/supply chain?
- RQ2 (practical): What effects of COVID-19 on logistics and supply chain processes were experienced by companies?
2. Materials and Methods2.1. systematic literature review, 2.1.1. sample creation, 2.1.2. descriptive analyses, 2.1.3. paper classification. - Macro theme: sustainability, resilience, risk, information technology, economics, performance, planning and food security. This classification represents paper’s core topic.
- Industrial sector: aerospace, agri-food, apparel, automotive, construction, e-commerce, electronic, energy, fast-moving consumer goods, food, healthcare, logistics, manufacturing and service.
- Data collection method: questionnaire/interview, third-party sources or case study. This classification represents the method used by the authors to collect the data useful to their study.
- Research method: statistical, decision-making, simulation, empirical, literature review or economic. This category describes the tool used by the authors to conduct the study and reach the related goals.
- Specific method, e.g., descriptive statistics, structural equation modeling (SEM), multi-criteria decision making (MCDM), etc.; this feature describes more accurately the type of work carried out by the authors and the tools used.
- Country: it reflects the geographical area in which the study was carried out, in terms, for instance, of the country in which a sample of people has been interviewed or where empirical data were collected, or where the simulation was set. This method of classification, although more elaborated, was preferred over traditional approaches, in which the country of the study is defined based merely on the affiliation of the first author of the paper, because the exact knowledge of the country in which the study was carried out is, for sure, a more representative source of information about the research. This is true in general, but it is even more important for this subject matter, as the management of the COVID-19 pandemic was made on a country or regional basis, with significant differences from country to country; knowing the exact location of the study helps in better interpreting the research outcomes. Possible entries in this field also include “multiple countries” and “not specified”, with the obvious meanings of the terms.
2.1.4. Cross-Analyses2.1.5. interrelated aspects, 2.2. case study, 2.2.1. data collection. - Economic data: some key economic data were retrieved from the company’s balance sheet, from 2019 up to the latest available document, which refers to 2022.
- Organizational data: these data describe changes in the operational, decision-making and business structure of the company in terms, e.g., of number of employees hired, number of drivers, etc.
- The related data were collected and elaborated between July and September 2023.
2.2.2. Survey Phase2.2.3. analysis and summary, 3. results—systematic literature review, 3.1. descriptive statistics, 3.2. common classification fields, 3.2.1. macro theme, 3.2.2. industrial sector, 3.2.3. data collection method, 3.2.4. research method, 3.2.5. country, 3.3. cross-analyses, 3.3.1. macro theme vs. industrial sector, 3.3.2. research method vs. macro theme, 3.4. interrelated aspects, 4. results—case study, 4.1. company overview, 4.2. pre-covid-19 period, 4.3. covid-19 period, 4.4. post-covid-19 period, 4.5. analysis and summary. - Strengths : at present, Company A benefits from a robust network of relationships with customers and suppliers (e.g., drivers), which was leveraged during the pandemic period to provide a rapid response to the increased request by the consumers. The company has also leveraged the usage of digital technologies, which made logistics activities more efficient and, again, allowed the company to respond to consumer demand in the pandemic period.
- Weaknesses : Company A has suffered from low economic results, in particular in the post-COVID-19 period, mainly due to the high production costs. Efforts must be made by the company to reduce expenses. At the same time, however, the service level, in terms of delivery lead time or on-time delivery, should be safeguarded.
- Opportunities : the growth of e-commerce, experienced in the COVID-19 period but expected to last over time, creates opportunities for increasing the volume of items handled by Company A. Indeed, the survey phase demonstrated that the company’s consumers have shifted towards the usage of online sales; hence, the company could consider investing in this area to increase its market share. By leveraging the e-commerce logistics and diversifying service, expansions could also be possible at an international level. Even if the company has already embraced the implementation of digital technologies, some emerging technologies (e.g., drones or advanced traceability systems) could also be introduced for further improving the logistics efficiency. Finally, sustainability is another opportunity to be leveraged, because of the current push towards the adoption of environmental-friendly logistics solutions. Examples of those solutions include a reduction in CO 2 emissions, and the usage of electric vehicles or zero-impact materials.
- Threats : the growth of e-commerce can be seen as an opportunity, but because many logistics companies have already entered this field, the sector is characterized by very high competition, which could limit the market share of Company A; this could instead be seen as a threat needing to be properly managed. Another threat comes from the increased cost of fuel, which, for sure, for a logistics company plays an important role in determining the cost of the transport activities (also, having previously observed that the company suffered from a limited revenue in recent years). This factor could further push towards the adoption of environmentally friendly transport modes (e.g., electric vehicles), which have been previously mentioned as an opportunity for leveraging in the logistics sector.
5. Conclusions5.1. answer to the research questions, 5.2. scientific and practical implications, 5.3. suggestions for future research directions, author contributions, institutional review board statement, informed consent statement, data availability statement, conflicts of interest. - Christopher, M. Logistics and Supply Chain Management: Strategies for Reducing Cost and Improving Service Financial Times ; Pitman Publishing: London, UK, 1998; ISBN 0 273 63049 0. [ Google Scholar ]
- Gechevski, D.; Kochov, A.; Popovska-Vasilevska, S.; Polenakovik, R.; Donev, V. Reverse Logistics and Green Logistics Way to Improving the Environmental Sustainability. Acta Tech. Corviniensis-Bull. Eng. 2016 , 9 , 63. [ Google Scholar ]
- Mbang, A. A New Introduction to Supply Chains and Supply Chain Management: Definitions and Theories Perspective. Int. Bus. Res. 2011 , 5 , 194–207. [ Google Scholar ] [ CrossRef ]
- Jones, T.C.; Riley, D.W. Using Inventory for Competitive Advantage through Supply Chain Management. Int. J. Phys. Distrib. Mater. Manag. 1985 , 15 , 16–26. [ Google Scholar ] [ CrossRef ]
- Monczka, R.M.; Trent, R.J.; Handfield, R.B. Purchasing and Supply Chain Management ; South Western Educational Publishing: Natorp Blvd Cincinnati, OH, USA, 2002; ISBN 0-324-02315-4. [ Google Scholar ]
- Belhadi, D.; Peiffer-Smadja, N.; Lescure, F.-X.; Yazdanpanah, Y.; Mentré, F.; Laouénan, C. A Brief Review of Antiviral Drugs Evaluated in Registered Clinical Trials for COVID-19. MedRxiv 2020 . [ Google Scholar ] [ CrossRef ]
- Shen, Z.; Sun, Y. Strengthening Supply Chain Resilience during COVID-19: A Case Study of JD.Com. J. Oper. Manag. 2021 , 69 , 359–383. [ Google Scholar ] [ CrossRef ]
- Dohale, V.; Verma, P.; Gunasekaran, A.; Ambilkar, P. COVID-19 and Supply Chain Risk Mitigation: A Case Study from India. Int. J. Logist. Manag. 2023 , 34 , 417–442. [ Google Scholar ] [ CrossRef ]
- Mishra, P.K. COVID-19, Black Swan Events and the Future of Disaster Risk Management in India. Prog. Disaster Sci. 2020 , 8 , 100137. [ Google Scholar ] [ CrossRef ]
- Badhotiya, G.K.; Soni, G.; Jain, V.; Joshi, R.; Mittal, S. Assessing Supply Chain Resilience to the Outbreak of COVID-19 in Indian Manufacturing Firms. Oper. Manag. Res. 2022 , 15 , 1161–1180. [ Google Scholar ] [ CrossRef ]
- Mahmoud, M.A.; Mahmoud, A.; Abubakar, S.L.; Garba, A.S.; Daneji, B.A. COVID-19 Operational Disruption and SMEs’ Performance: The Moderating Role of Disruption Orientation and Government Support. Benchmarking Int. J. 2022 , 29 , 2641–2664. [ Google Scholar ] [ CrossRef ]
- Aldrighetti, R.; Battini, D.; Ivanov, D. Increasing Supply Chain Resilience through Efficient Redundancy Allocation: A Risk-Averse Mathematical Model. Ifac-papersonline 2021 , 54 , 1011–1016. [ Google Scholar ]
- Tang, O.; Nurmaya Musa, S. Identifying Risk Issues and Research Advancements in Supply Chain Risk Management. Int. J. Prod. Econ. 2011 , 133 , 25–34. [ Google Scholar ] [ CrossRef ]
- Rinaldi, M.; Murino, T.; Gebennini, E.; Morea, D.; Bottani, E. A Literature Review on Quantitative Models for Supply Chain Risk Management: Can They Be Applied to Pandemic Disruptions? Comput. Ind. Eng. 2022 , 170 , 108329. [ Google Scholar ] [ CrossRef ]
- Corallo, A.; Lazoi, M.; Lezzi, M.; Luperto, A. Cybersecurity Awareness in the Context of the Industrial Internet of Things: A Systematic Literature Review. Comput. Ind. 2022 , 137 , 103614. [ Google Scholar ] [ CrossRef ]
- Rinaldi, M.; Bottani, E. How Did COVID-19 Affect Logistics and Supply Chain Processes? Immediate, Short and Medium-Term Evidence from Some Industrial Fields of Italy. Int. J. Prod. Econ. 2023 , 262 , 108915. [ Google Scholar ] [ CrossRef ]
- Chowdhury, P.; Paul, S.K.; Kaisar, S.; Moktadir, M.A. COVID-19 pandemic related supply chain studies: A systematic review. Transp. Res. Part E—Logist. Transp. Rev. 2021 , 148 , 102271. [ Google Scholar ] [ CrossRef ]
- Nandi, S.; Sarkis, J.; Hervani, A.A.; Helms, M.M. Redesigning supply chains using blockchain-enabled circular economy and COVID-19 experiences. Sustain. Prod. Consum. 2021 , 27 , 10–22. [ Google Scholar ] [ CrossRef ]
- Kraus, S.; Clauss, T.; Breier, M.; Gast, J.; Zardini, A.; Tiberius, V. The economics of COVID-19: Initial empirical evidence on how family firms in five European countries cope with the corona crisis. Int. J. Entrep. Behav. Res. 2020 , 26 , 1067–1092. [ Google Scholar ] [ CrossRef ]
- De Vet, J.M.; Nigohosyan, D.; Ferrer, J.N.; Gross, A.K.; Kuehl, S.; Flickenschild, M. Impacts of the COVID-19 Pandemic on EU Industries. 2021. Available online: https://www.europarl.europa.eu/RegData/etudes/STUD/2021/662903/IPOL_STU(2021)662903_EN.pdf (accessed on 29 April 2024).
- Manuj, I.; Mentzer, J.T. Global Supply Chain Risk Management. J. Bus. Logist. 2008 , 29 , 133–155. [ Google Scholar ] [ CrossRef ]
- Ali, I.; Golgeci, I.; Arslan, A. Achieving Resilience through Knowledge Management Practices and Risk Management Culture in Agri-Food Supply Chains. Supply Chain. Manag. Int. J. 2023 , 28 , 284–299. [ Google Scholar ] [ CrossRef ]
- Madhavika, N.; Jayasinghe, N.; Ehalapitiya, S.; Wickramage, T.; Fernando, D.; Jayasinghe, V. Operationalizing Resilience through Collaboration: The Case of Sri Lankan Tea Supply Chain during Covid-19. Qual. Quant. 2023 , 57 , 2981–3018. [ Google Scholar ] [ CrossRef ]
- Aman, S.; Seuring, S. Analysing Developing Countries Approaches of Supply Chain Resilience to COVID-19. Int. J. Logist. Manag. 2023 , 34 , 909–934. [ Google Scholar ] [ CrossRef ]
- Maharjan, R.; Kato, H. Resilient Supply Chain Network Design: A Systematic Literature Review. Transp. Rev. 2022 , 42 , 739–761. [ Google Scholar ] [ CrossRef ]
- Zidi, S.; Hamani, N.; Kermad, L. Antecedents and Enablers of Supply Chain Reconfigurability and Their Effects on Performance. Int. J. Adv. Manuf. Technol. 2022 , 120 , 3027–3043. [ Google Scholar ] [ CrossRef ]
- Nabipour, M.; Ülkü, M.A. On Deploying Blockchain Technologies in Supply Chain Strategies and the COVID-19 Pandemic: A Systematic Literature Review and Research Outlook. Sustainability 2021 , 13 , 10566. [ Google Scholar ] [ CrossRef ]
- Rokicki, T.; Bórawski, P.; Bełdycka-Bórawska, A.; Szeberényi, A.; Perkowska, A. Changes in Logistics Activities in Poland as a Result of the COVID-19 Pandemic. Sustainability 2022 , 14 , 10303. [ Google Scholar ] [ CrossRef ]
- Figura, J.; Gądek-Hawlena, T. The Impact of the COVID-19 Pandemic on the Development of Electromobility in Poland. The Perspective of Companies in the Transport-Shipping-Logistics Sector: A Case Study. Energies 2022 , 15 , 1461. [ Google Scholar ] [ CrossRef ]
- Baldrighi, E.; Monferdini, L.; Bottani, E. The Response to COVID-19 in Logistics and Supply Chain Processes: Evidence from a Review of the Literature. In Proceedings of the International Conference on Harbour, Maritime and Multimodal Logistics Modelling and Simulation, Athens, Greece, 18–20 September 2023. [ Google Scholar ]
- Bottani, E.; Monferdini, L. Studies related to Covid-19 in logistics and supply chain processes (2021–2023). Mendeley Data 2024 , V1. [ Google Scholar ] [ CrossRef ]
- Kesmodel, U.S. Cross-Sectional Studies—What Are They Good For? Acta Obstet. Gynecol. Scand. 2018 , 97 , 388–393. [ Google Scholar ] [ CrossRef ]
- Thompson, M.L.; Myers, J.; Kriebel, D. Prevalence Odds Ratio or Prevalence Ratio in the Analysis of Cross Sectional Data: What Is to Be Done? Occup. Environ. Med. 1998 , 55 , 272–277. [ Google Scholar ] [ CrossRef ]
- Robson, C. Real World Research: A Resource for Social Scientists and Practitioner-Researchers ; Blackwell Publishing: Hoboken, NJ, USA, 1993; ISBN 978-0-631-17689-3. [ Google Scholar ]
- Yin, R. Case Studies. Int. Encycl. Soc. Behav. Sci. 2015 , 2 , 194–201. [ Google Scholar ] [ CrossRef ]
- Wohlin, C. Case Study Research in Software Engineering—It Is a Case, and It Is a Study, but Is It a Case Study? Inf. Softw. Technol. 2021 , 133 , 106514. [ Google Scholar ] [ CrossRef ]
- Grabot, B.; Vallespir, B.; Samuel, G.; Bouras, A.; Kiritsis, D. Advances in Production Management Systems: Innovative and Knowledge-Based Production Management in a Global-Local World: IFIP WG 5.7 International Conference, APMS 2014, Ajaccio, France, 20–24 September 2014, Proceedings, Part II ; Springer: Berlin/Heidelberg, Germany, 2014; Volume 439, ISBN 3-662-44736-3. [ Google Scholar ]
- Alicke, K.; Azcue, X.; Barriball, E.; Supply-Chain Recovery in Coronavirus Times—Plan for Now and the Future. McKinsey & Company. 2020. Available online: https://www.mckinsey.com (accessed on 29 April 2024).
- Ivanov, D. Supply Chain Viability and the COVID-19 Pandemic: A Conceptual and Formal Generalisation of Four Major Adaptation Strategies. Int. J. Prod. Res. 2021 , 59 , 3535–3552. [ Google Scholar ] [ CrossRef ]
- Adobor, H.; McMullen, R.S. Supply Chain Resilience: A Dynamic and Multidimensional Approach. Int. J. Logist. Manag. 2018 , 29 , 1451–1471. [ Google Scholar ] [ CrossRef ]
- Singh, C.S.; Soni, G.; Badhotiya, G.K. Performance Indicators for Supply Chain Resilience: Review and Conceptual Framework. J. Ind. Eng. Int. 2019 , 15 , 105–117. [ Google Scholar ] [ CrossRef ]
- Mohammed, A.; Jabbour, A.B.L.D.S.; Diabat, A. COVID-19 Pandemic Disruption: A Matter of Building Companies’ Internal and External Resilience. Int. J. Prod. Res. 2023 , 61 , 2716–2737. [ Google Scholar ] [ CrossRef ]
- El Khoury, R.; Nasrallah, N.; Atayah, O.F.; Dhiaf, M.M.; Frederico, G.F. The Impact of Green Supply Chain Management Practices on Environmental Performance during COVID-19 Period: The Case of Discretionary Companies in the G-20 Countries. Benchmarking Int. J. 2022 . ahead of print . [ Google Scholar ] [ CrossRef ]
- Sharma, V.; Singh, A.; Rai, S.S. Disruptions in Sourcing and Distribution Practices of Supply Chains Due to COVID-19 Pandemic: A Sustainability Paradigm. J. Glob. Oper. Strateg. Sourc. 2022 , 15 , 235–261. [ Google Scholar ] [ CrossRef ]
- Karmaker, C.L.; Bari, A.B.M.M.; Anam, M.Z.; Ahmed, T.; Ali, S.M.; de Jesus Pacheco, D.A.; Moktadir, M.A. Industry 5.0 Challenges for Post-Pandemic Supply Chain Sustainability in an Emerging Economy. Int. J. Prod. Econ. 2023 , 258 , 108806. [ Google Scholar ] [ CrossRef ]
- Paul, A.; Shukla, N.; Trianni, A. Modelling Supply Chain Sustainability Challenges in the Food Processing Sector amid the COVID-19 Outbreak. Socio-Econ. Plan. Sci. 2023 , 87 , 101535. [ Google Scholar ] [ CrossRef ]
- Siuta, K.; Kaszyński, D. The Principal-Agent Problem in Supply Chain Management—The Simulation Based Framework. Control. Cybern. 2021 , 50 , 195–221. [ Google Scholar ] [ CrossRef ]
- Kumar, P.; Singh, S.S.; Pandey, A.K.; Singh, R.K.; Srivastava, P.K.; Kumar, M.; Dubey, S.K.; Sah, U.; Nandan, R.; Singh, S.K.; et al. Multi-Level Impacts of the COVID-19 Lockdown on Agricultural Systems in India: The Case of Uttar Pradesh. Agric. Syst. 2021 , 187 , 103027. [ Google Scholar ] [ CrossRef ]
- Atayah, O.F.; Dhiaf, M.M.; Najaf, K.; Frederico, G.F. Impact of COVID-19 on Financial Performance of Logistics Firms: Evidence from G-20 Countries. J. Glob. Oper. Strateg. Sourc. 2022 , 15 , 172–196. [ Google Scholar ] [ CrossRef ]
- Israfilov, N.; Druzyanova, V.; Ermakova, M.; Sinitsyna, A. Key Directions for Transforming Supply Chain Management in Emerging Markets during the PostCOVID-19 Pandemic Period. Oper. Supply Chain. Manag. 2023 , 16 , 498–508. [ Google Scholar ] [ CrossRef ]
- Dwivedi, A.; Chowdhury, P.; Paul, S.K.; Agrawal, D. Sustaining Circular Economy Practices in Supply Chains during a Global Disruption. Int. J. Logist. Manag. 2023 , 34 , 644–673. [ Google Scholar ] [ CrossRef ]
- Ghadge, D.A.; Er, M.; Ivanov, D.; Chaudhuri, A. Visualisation of Ripple Effect in Supply Chains under Long-Term, Simultaneous Disruptions: A System Dynamics Approach. Int. J. Prod. Res. 2021 , 60 , 1987547. [ Google Scholar ] [ CrossRef ]
- Eldem, B.; Kluczek, A.; Bagiński, J. The COVID-19 Impact on Supply Chain Operations of Automotive Industry: A Case Study of Sustainability 4.0 Based on Sense–Adapt–Transform Framework. Sustainability 2022 , 14 , 5855. [ Google Scholar ] [ CrossRef ]
- Gui, D.; Wang, H.; Yu, M. Risk Assessment of Port Congestion Risk during the COVID-19 Pandemic. J. Mar. Sci. Eng. 2022 , 10 , 150. [ Google Scholar ] [ CrossRef ]
- Brdulak, H.; Brdulak, A. Challenges and Threats Faced in 2020 by International Logistics Companies Operating on the Polish Market. Sustainability 2021 , 13 , 359. [ Google Scholar ] [ CrossRef ]
- Paul, S.K.; Chowdhury, P.; Chowdhury, M.T.; Chakrabortty, R.K.; Moktadir, M.A. Operational Challenges during a Pandemic: An Investigation in the Electronics Industry. Int. J. Logist. Manag. 2023 , 34 , 336–362. [ Google Scholar ] [ CrossRef ]
- Klein, M.; Gutowska, E.; Gutowski, P. Innovations in the T&L (Transport and Logistics) Sector during the COVID-19 Pandemic in Sweden, Germany and Poland. Sustainability 2022 , 14 , 3323. [ Google Scholar ] [ CrossRef ]
- Mishrif, A.; Khan, A. Technology Adoption as Survival Strategy for Small and Medium Enterprises during COVID-19. J. Innov. Entrep. 2023 , 12 , 53. [ Google Scholar ] [ CrossRef ]
- Ishak, S.; Shaharudin, M.R.; Salim, N.A.M.; Zainoddin, A.I.; Deng, Z. The Effect of Supply Chain Adaptive Strategies During the COVID-19 Pandemic on Firm Performance in Malaysia’s Semiconductor Industries. Glob. J. Flex. Syst. Manag. 2023 , 24 , 439–458. [ Google Scholar ] [ CrossRef ]
- Hendijani, R.; Norouzi, M. Supply Chain Integration and Firm Performance in the COVID-19 Era: The Mediating Role of Resilience and Robustness. J. Glob. Oper. Strateg. Sourc. 2023 , 16 , 337–367. [ Google Scholar ] [ CrossRef ]
- Vilko, J.; Hallikas, J. Impact of COVID-19 on Logistics Sector Companies. Int. J. Ind. Eng. Oper. Manag. 2024 , 6 , 25–42. [ Google Scholar ] [ CrossRef ]
- Palouj, M.; Lavaei Adaryani, R.; Alambeigi, A.; Movarej, M.; Safi Sis, Y. Surveying the Impact of the Coronavirus (COVID-19) on the Poultry Supply Chain: A Mixed Methods Study. Food Control 2021 , 126 , 108084. [ Google Scholar ] [ CrossRef ]
- Ali, I.; Arslan, A.; Khan, Z.; Tarba, S. The Role of Industry 4.0 Technologies in Mitigating Supply Chain Disruption: Empirical Evidence From the Australian Food Processing Industry. IEEE Trans. Eng. Manag. 2021 , 71 , 10600–10610. [ Google Scholar ] [ CrossRef ]
- Grigorescu, I.; Popovici, E.-A.; Damian, N.; Dumitraşcu, M.; Sima, M.; Mitrică, B.; Mocanu, I. The Resilience of Sub-Urban Small Farming in Bucharest Metropolitan Area in Response to the COVID-19 Pandemic. Land Use Policy 2022 , 122 , 106351. [ Google Scholar ] [ CrossRef ]
- Perrin, A.; Martin, G. Resilience of French Organic Dairy Cattle Farms and Supply Chains to the Covid-19 Pandemic. Agric. Syst. 2021 , 190 , 103082. [ Google Scholar ] [ CrossRef ]
- Coopmans, I.; Bijttebier, J.; Marchand, F.; Mathijs, E.; Messely, L.; Rogge, E.; Sanders, A.; Wauters, E. COVID-19 Impacts on Flemish Food Supply Chains and Lessons for Agri-Food System Resilience. Agric. Syst. 2021 , 190 , 103136. [ Google Scholar ] [ CrossRef ]
- Ababulgu, N.; Abajobir, N.; Wana, H. The Embarking of COVID-19 and the Perishable Products’ Value Chain in Ethiopia. J. Innov. Entrep. 2022 , 11 , 34. [ Google Scholar ] [ CrossRef ]
- Eileen Bogweh, N.; Lutomia, C. COVID-19 Challenges to Sustainable Food Production and Consumption: Future Lessons for Food Systems in Eastern and Southern Africa from a Gender Lens. Sustain. Prod. Consum. 2021 , 27 , 2208–2220. [ Google Scholar ] [ CrossRef ]
- Igberi, C.; Omenyi, L.; Osuji, E.; Egwu, P.; Ibrahim-olesin, S. Comparative Analysis of the Sustainable Dimensions of Food Security with COVID-19 and Climate Change: A Case Study. Int. J. Adv. Appl. Sci. 2022 , 9 , 9–15. [ Google Scholar ] [ CrossRef ]
- Mugabe, P.A.; Renkamp, T.M.; Rybak, C.; Mbwana, H.; Gordon, C.; Sieber, S.; Löhr, K. Governing COVID-19: Analyzing the Effects of Policy Responses on Food Systems in Tanzania. Agric. Food Secur. 2022 , 11 , 47. [ Google Scholar ] [ CrossRef ]
- Nunes, M.; Abreu, A.; Bagnjuk, J.; Nunes, E.; Saraiva, C. A Strategic Process to Manage Collaborative Risks in Supply Chain Networks (SCN) to Improve Resilience and Sustainability. Sustainability 2022 , 14 , 5237. [ Google Scholar ] [ CrossRef ]
- World Economic Forum (WEF). How to Rebound Stronger from COVID-19—Resilience in Manufacturing and Supply Systems ; World Economic Forum: Cologny, Switzerland, 2020. [ Google Scholar ]
- Zulkiffli, S.N.; Zaidi, N.F.; Padlee, S.F.; Sukri, N.K. Eco-Innovation Capabilities and Sustainable Business Performance during the COVID-19 Pandemic. Sustainability 2022 , 14 , 7525. [ Google Scholar ] [ CrossRef ]
- Moosavi, J.; Hosseini, S. Simulation-Based Assessment of Supply Chain Resilience with Consideration of Recovery Strategies in the COVID-19 Pandemic Context. Comput. Ind. Eng. 2021 , 160 , 107593. [ Google Scholar ] [ CrossRef ]
- Ho, W.; Zheng, T.; Yildiz, H.; Talluri, S. Supply Chain Risk Management: A Literature Review. Int. J. Prod. Res. 2015 , 53 , 1030467. [ Google Scholar ] [ CrossRef ]
- Dogbe, C.S.K.; Iddris, F.; Duah, E.; Boateng, P.A.; Kparl, E.M. Analyzing the Health Supply Chain Risks during COVID-19 Pandemic: The Moderating Role of Risk Management. Cogent Bus. Manag. 2023 , 10 , 2281716. [ Google Scholar ] [ CrossRef ]
- Shenoi, V.; Dath, S.; Rajendran, C. Supply Chain Risk Management in Indian Manufacturing Industries: An Empirical Study and a Fuzzy Approach ; Springer Nature Switzerland: Cham, Switzerland, 2021; pp. 107–145. ISBN 978-3-030-69264-3. [ Google Scholar ]
- Jifar, W.; Geneti, G.; Dubale, S. The Impact of COVID-19 on Pharmaceutical Shortages and Supply Disruptions for Non-Communicable Diseases Among Public Hospitals of South West, Oromia, Ethiopia. J. Multidiscip. Healthc. 2022 , 15 , 1933–1943. [ Google Scholar ] [ CrossRef ]
- Goodarzian, F.; Taleizadeh, A.; Ghasemi, P.; Abraham, A. An Integrated Sustainable Medical Supply Chain Network during COVID-19 ; Elsevier: Amsterdam, The Netherlands, 2021; Volume 100. [ Google Scholar ]
- Bump, J.B.; Friberg, P.; Harper, D.R. International Collaboration and Covid-19: What Are We Doing and Where Are We Going? BMJ 2021 , 372 , n180. [ Google Scholar ] [ CrossRef ]
- Abu-Elmagd, K.; Fung, J.; Bueno, J.; Martin, D.; Madariaga, J.R.; Mazariegos, G.; Bond, G.; Molmenti, E.; Corry, R.J.; Starzl, T.E. Logistics and Technique for Procurement of Intestinal, Pancreatic, and Hepatic Grafts from the Same Donor. Ann. Surg. 2000 , 232 , 680–687. [ Google Scholar ] [ CrossRef ]
- Fu, H.; Ke, G.Y.; Lian, Z.; Zhang, L. 3PL Firm’s Equity Financing for Technology Innovation in a Platform Supply Chain. Transp. Res. Part E Logist. Transp. Rev. 2021 , 147 , 102239. [ Google Scholar ] [ CrossRef ]
- Ferrari, P. The Reasons for the Success of Freight Rail Transport through the Swiss Alps|Le Ragioni Del Successo Del Trasporto Ferroviario Delle Merci Attraverso Le Alpi Svizzere. Ing. Ferrov. 2019 , 74 , 9–26. [ Google Scholar ]
- Hess, A.-K.; Schubert, I. Functional Perceptions, Barriers, and Demographics Concerning e-Cargo Bike Sharing in Switzerland. Transp. Res. Part D Transp. Environ. 2019 , 71 , 153–168. [ Google Scholar ] [ CrossRef ]
- Eurostat Sustainable Development in the European Union: Monitoring Report on Progress towards the SDGS in an EU Context ; Publications office of the European Union: Maastricht, The Netherlands, 2017; ISBN 92-79-72288-3.
- European Union. European Union Horizon 2020 in Brief. In The EU Framework Programme for Research and Innovation ; European Union: Maastricht, The Netherlands, 2014. [ Google Scholar ]
- Stoll, J.; Harrison, H.; De Sousa, E.; Callaway, D.; Collier, M.; Harrell, K.; Jones, B.; Kastlunger, J.; Kramer, E.; Kurian, S.; et al. Alternative Seafood Networks During COVID-19: Implications for Resilience and Sustainability. Front. Sustain. Food Syst. 2021 , 5 , 614368. [ Google Scholar ] [ CrossRef ]
- Tendall, D.; Joerin, J.; Kopainsky, B.; Edwards, P.; Shreck, A.; Le, Q.B.; Krütli, P.; Grant, M.; Six, J. Food System Resilience: Defining the Concept. Glob. Food Secur. 2015 , 6 , 17–23. [ Google Scholar ] [ CrossRef ]
- Li, D.; Wang, X.; Chan, H.K.; Manzini, R. Sustainable Food Supply Chain Management. Int. J. Prod. Econ. 2014 , 152 , 1–8. [ Google Scholar ] [ CrossRef ]
- Neven, D. Developing Sustainable Food Value Chains ; FAO: Rome, Italy, 2014; ISBN 92-5-108481-5. [ Google Scholar ]
- Mishra, D.; Gunasekaran, A.; Papadopoulos, T.; Dubey, R. Supply Chain Performance Measures and Metrics: A Bibliometric Study. Benchmarking Int. J. 2018 , 25 , 932–967. [ Google Scholar ] [ CrossRef ]
- Guersola, M.; Pinheiro de Lima, E.; Steiner, M. Supply Chain Performance Measurement: A Systematic Literature Review. Int. J. Logist. Syst. Manag. 2018 , 31 , 109. [ Google Scholar ] [ CrossRef ]
- Perdana, T.; Onggo, B.; Sadeli, A.; Chaerani, D.; Achmad, A.; Rahayu, F.; Gong, Y. Food Supply Chain Management in Disaster Events: A Systematic Literature Review. Int. J. Disaster Risk Reduct. 2022 , 79 , 103183. [ Google Scholar ] [ CrossRef ]
- Rahbari, M.; Arshadi Khamseh, A.; Mohammadi, M. Robust Optimization and Strategic Analysis for Agri-Food Supply Chain under Pandemic Crisis: Case Study from an Emerging Economy. Expert Syst. Appl. 2023 , 225 , 120081. [ Google Scholar ] [ CrossRef ]
- Singh, R.; Sinha, V.; Joshi, P.; Kumar, M. Modelling Agriculture, Forestry and Other Land Use (AFOLU) in Response to Climate Change Scenarios for the SAARC Nations. Environ. Monit. Assess. 2020 , 192 , 1–18. [ Google Scholar ] [ CrossRef ]
- Divergences, N.G. World Economic Outlook. 2023. Available online: https://www.imf.org/en/Publications/WEO/Issues/2023/10/10/world-economic-outlook-october-2023 (accessed on 29 April 2024).
- Ponomarov, S.; Holcomb, M. Understanding the Concept of Supply Chain Resilience. Int. J. Logist. Manag. 2009 , 20 , 124–143. [ Google Scholar ] [ CrossRef ]
- Tarigan, Z.J.H.; Siagian, H.; Jie, F. Impact of Internal Integration, Supply Chain Partnership, Supply Chain Agility, and Supply Chain Resilience on Sustainable Advantage. Sustainability 2021 , 13 , 5460. [ Google Scholar ] [ CrossRef ]
- Zidi, S.; Hamani, N.; Kermad, L. New Metrics for Measuring Supply Chain Reconfigurability. J. Intell. Manuf. 2022 , 33 , 2371–2392. [ Google Scholar ] [ CrossRef ]
- Zhang, S.; Wang, H.; Li, G.; Wang, J. Modeling of the Resilient Supply Chain System from a Perspective of Production Design Changes. Front. Eng. Manag. 2023 , 10 , 96–106. [ Google Scholar ] [ CrossRef ]
- Brundtland, G.H. World Commission on Environment and Development. Environ. Policy Law 1985 , 14 , 26–30. [ Google Scholar ]
- Yao, Q.; Liu, J.; Sheng, S.; Fang, H. Does Eco-Innovation Lift Firm Value? The Contingent Role of Institutions in Emerging Markets. J. Bus. Ind. Mark. 2019 . ahead of print . [ Google Scholar ] [ CrossRef ]
- Althaf, S.; Babbitt, C.W. Disruption Risks to Material Supply Chains in the Electronics Sector. Resour. Conserv. Recycl. 2021 , 167 , 105248. [ Google Scholar ] [ CrossRef ]
- Zeng, X.; Mathews, J.A.; Li, J. Urban Mining of E-Waste Is Becoming More Cost-Effective than Virgin Mining. Environ. Sci. Technol. 2018 , 52 , 4835–4841. [ Google Scholar ] [ CrossRef ]
- Nosalska, K.; Piątek, Z.; Mazurek, G.; Rządca, R. Industry 4.0: Coherent Definition Framework with Technological and Organizational Interdependencies. J. Manuf. Technol. Manag. [ CrossRef ]
- Munongo, S.; Pooe, D. Small and Medium Enterprises’ Adoption of 4IR Technologies for Supply Chain Resilience during the COVID-19 Pandemic. J. Transp. Supply Chain. Manag. 2022 , 14 , 747. [ Google Scholar ] [ CrossRef ]
- Kumar, A.; Singh, R.K. Supply Chain Management Practices, Retail Outlets Attributes and Organisational Performance: A Case of Organised Food Retailers in India. J. Glob. Oper. Strateg. Sourc. 2023 , 16 , 568–589. [ Google Scholar ] [ CrossRef ]
- Nisar, Q.A.; Haider, S.; Ameer, I.; Hussain, M.S.; Gill, S.S.; Usama, A. Sustainable Supply Chain Management Performance in Post COVID-19 Era in an Emerging Economy: A Big Data Perspective. Int. J. Emerg. Mark. 2022; ahead of print . [ Google Scholar ] [ CrossRef ]
- Miljenović, D.; Beriša, B. Pandemics Trends in E-Commerce: Drop Shipping Entrepreneurship during COVID-19 Pandemic. Pomorstvo 2022 , 36 , 31–43. [ Google Scholar ] [ CrossRef ]
- Laborde, D.; Martin, W.; Swinnen, J.; Vos, R. COVID-19 Risks to Global Food Security. Science 2020 , 369 , 500–502. [ Google Scholar ] [ CrossRef ]
- Yearbook, F.S. World Food and Agriculture ; Food and Agriculture Organization of the United Nations: Rome, Italy, 2013; Volume 15. [ Google Scholar ]
- Kim, B.; Kim, G.; Kang, M. Study on Comparing the Performance of Fully Automated Container Terminals during the COVID-19 Pandemic. Sustainability 2022 , 14 , 9415. [ Google Scholar ] [ CrossRef ]
- ISO 9001:2015 ; Quality Management Systems-Requirements. International Organization for Standardization: Geneva, Switzerland, 2015. Available online: https://committee.iso.org/standard/62085.html (accessed on 13 June 2024).
Click here to enlarge figure Source | No. of Papers | Scimago Ranking |
---|
Sustainability (Switzerland) | 10 | Q1–Q2 | International Journal of Logistics Management | 6 | Q1 | Journal of Global Operations and Strategic Sourcing | 5 | Q2 | Agricultural Systems | 5 | Q1 | Benchmarking | 4 | Q1 | International Journal of Production Research | 3 | Q1 | Research Method | No. of Papers |
---|
| | ANOVA | 2 | Contingency analysis and frequency analysis | 1 | Cronbach’s alpha | 1 | Descriptive statistics | 8 | Econometric | 1 | Hypothesis test | 5 | Keyword analysis | 1 | Logistic regression—R software | 1 | Partial Least Square (PLS) | 1 | PLS-SEM | 11 | Random forest regression | 1 | Regression | 3 | SEM | 9 | Descriptive statistics, bias and common method variance test, multiple regression analysis and mediation test | 1 | Analysis with SPSS and Nvivo | 1 | | | Best Worst Method | 1 | Decision-Making Trial and Evaluation Laboratory (DEMATEL) | 1 | DEMATEL—Maximum mean de-entropy (MMDE) | 1 | Fuzzy | 10 | ISM | 1 | ISM-Bayesian network (BN) | 1 | ISM-Cross-Impact Matrix Multiplication Applied to Classification (MICMAC) | 1 | Multi-Attribute Decision Making (MADM) | 1 | Multi-Attribute Utility Theory (MAUT) | 1 | Multi-Criteria Decision Methods (MCDM) | 6 | SWOT analysis | 2 | Total Interpretive Structural Modelling (TISM) + MICMAC analysis | 1 | | | Case study | 7 | Framework and case study | 1 | Product design changes (PDC)—domain modelling | 1 | Qualitative | 5 | | | | | ABC analysis | 2 | Poisson pseudo-maximum likelihood (PPML) | 1 | Method of stochastic factor economic–mathematical analysis | 1 | | | Discrete Event Simulation (DES) | 1 | System dynamics approach | 1 | Multi-period simulation | 1 | | | Industrial Sector | No. of Papers |
---|
Logistics | 13 | Manufacturing | 4 | Food | 4 | Automotive | 3 | Agri-food | 3 | Industrial Sector | No. of Papers |
---|
Logistics | 10 | Food | 7 | Agri-food | 6 | Manufacturing | 6 | Healthcare | 2 | Electronic | 2 | Industrial Sector | No. of Papers |
---|
Logistics | 9 | Food | 3 | Agri-food | 3 | Manufacturing | 2 | | The statements, opinions and data contained in all publications are solely those of the individual author(s) and contributor(s) and not of MDPI and/or the editor(s). MDPI and/or the editor(s) disclaim responsibility for any injury to people or property resulting from any ideas, methods, instructions or products referred to in the content. |
Share and CiteMonferdini, L.; Bottani, E. Examining the Response to COVID-19 in Logistics and Supply Chain Processes: Insights from a State-of-the-Art Literature Review and Case Study Analysis. Appl. Sci. 2024 , 14 , 5317. https://doi.org/10.3390/app14125317 Monferdini L, Bottani E. Examining the Response to COVID-19 in Logistics and Supply Chain Processes: Insights from a State-of-the-Art Literature Review and Case Study Analysis. Applied Sciences . 2024; 14(12):5317. https://doi.org/10.3390/app14125317 Monferdini, Laura, and Eleonora Bottani. 2024. "Examining the Response to COVID-19 in Logistics and Supply Chain Processes: Insights from a State-of-the-Art Literature Review and Case Study Analysis" Applied Sciences 14, no. 12: 5317. https://doi.org/10.3390/app14125317 Article MetricsArticle access statistics, further information, mdpi initiatives, follow mdpi. 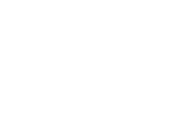 Subscribe to receive issue release notifications and newsletters from MDPI journals 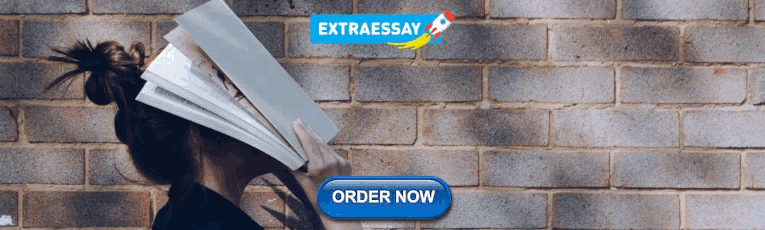 |
IMAGES
VIDEO
COMMENTS
Recent developments in production processes and their automation have led to the definition of the Fourth Industrial Revolution, commonly known as "Industry 4.0". Industry 4.0 is a very broad domain that includes: production processes, efficiency, data management, relationship with consumers, competitiveness, and much more. At the same time, obviously, Industry 4.0 has become a new theme ...
the paper is to analyze and classify the main contributions published on the topic of Industry 4.0 in. management literature, seeking to give it a unique definition, discover the gaps still ...
The state-of-the-art of management studies emerging from this systematic literature review highlights that at the present time the managerial literature on Industry 4.0 is still heavily affected ...
A systematic literature review of the relationship between industry 4.0, sustainability and its pillars," Technological Forecasting and Social Change, Elsevier, vol. 177(C). Dag Øivind Madsen, 2019. " The Emergence and Rise of Industry 4.0 Viewed through the Lens of Management Fashion Theory ," Administrative Sciences , MDPI, vol. 9(3), pages ...
Abstract: Recent developments in production processes and their automation have led to the definition of the Fourth Industrial Revolution, commonly known as "Industry 4.0". Industry 4.0 is a very broad domain that includes: production processes, efficiency, data management, relationship with consumers, competitiveness, and much more.
1. Introduction. Industry 4.0 (I4.0) represents an ongoing transformational phase for manufacturing organisations aiming to fully interlink their business functions and production systems with data from the entire lifecycle or End-to-End Digital Integration (Liao et al. Citation 2017; Castelo-Branco, Cruz-Jesus, and Oliveira Citation 2019).The implementation of I4.0 has been conceptualised not ...
To achieve the study objectives, a systematic literature review (SLR) with a bibliometric analysis of past studies from 2010 to 2022 was performed to identify the emerging themes of corporate reputation in I4.0. ... The findings are aligned with those of the review studies of Veh et al. (2019) and Gomez-Trujillo ... Johl S. K. (2022). Soft and ...
Therefore, the aim of the paper is to analyze and classify the main contributions published on the topic of Industry 4.0 in management literature, seeking to give it a unique definition, discover the gaps still remaining in literature and outline future avenues of research in this domain.
A systematic literature review of 59 studies published between 2011 and December 2020 is conducted. Using the Theory, Context, Characteristics, and Method (TCCM) framework, the review identifies various gaps in research and proposes future research agenda. ... The literature shows that Industry 4.0 impacts strategic management in the long run ...
Findings. By providing a clear picture of what scholars have studied so far on Industry 4.0 transition, in terms of KM, this literature review highlights that among all the studied digital technologies, the big data analytics technology is the one that has been explored the most in each phase of the ACAP process.
Our literature review indicates that the interest in the implications of Industry 4.0 technologies for supply chains grew exponentially from 2015 to 2019 and has remained at a high level since. As a consequence, about three times as many studies on this topic were published in the period 2018 to 2021 as had been published before.
Industry 4.0 in management studies: A systematic literature review: Piccarozzi & Aquilani, 2018: 2018: Review deals with papers published on the Industry 4.0 within management literature. Sustainability: Pharma Industry 4.0: Literature review and research opportunities in sustainable pharmaceutical supply chains: Ding, 2018: 2018
The research is based on a systematic literature review of 111 papers that were published in academic journals between 2015 and 2021. In contrast to previous reviews, we focus on constituting elements of Lean Management and Industry 4.0 to offer a perspective closely related to practical implementations. ... Industry 4.0 in management studies ...
Thus, this study aims to review the integration between LSCM and I4.0 analysing relationship at operative, tactical and strategic levels.,Systematic literature review was conducted to identify and explain the integration of LSCM and I4.0 from scientific sources that were published before March 2021.,The analysis of the literature revealed the ...
Department of Management Studies, Indian Institute of Science, Bangalore, India ... In an era where new concepts and techniques are introduced continuously, there is a lack of systematic literature review (SLR) on identifying main dimensions, levels, methods to assess the maturity and readiness level toward I4.0. ... Industry 4.0 India Inc ...
For this purpose, the study conducts a systematic review of extant literature to identify Industry 4.0 functions for sustainable innovation and applies interpretive structural modeling to devise the promised roadmap. The results offer interesting insights into Industry 4.0 applications for sustainable innovation.
Proposition 2. Industry 4.0 is a social movement that has the German government as one of its major institutional entrepreneurs. At the macro-level of analysis, the concept of varieties of capitalism (Hall & Soskice, 2001) could give clues about the dynamics of the adoption and diffusion of Industry 4.0 in countries.
Probably some interesting papers had been not intentionally missed.,Consultants and managers in developing and implementing their own Quality 4.0 models could use many practical and discussed implications concerning I4.0 technologies and quality management.,This is one of the first papers which employed the systematic literature review for ...
The state-of-the-art of management studies emerging from this systematic literature review highlights that at the present time the managerial literature on Industry 4.0 is still heavily affected by the influence of manufacturing also encompassing its technical and/or engineering aspects.
It is critical for organizations to self-assess their Industry 4.0 readiness to survive and thrive in the age of the Fourth Industrial Revolution. Thereon, conceptualization or development of an Industry 4.0 readiness model with the fundamental model dimensions is needed. This paper used a systematic literature review (SLR) methodology with the Preferred Reporting Items for Systematic Reviews ...
A systematic literature review Chiara Cagnettia*, Tommaso Galloa, Cecilia Silvestria, Alessandro Ruggieria aDepartment of Economy, Engineering, Society and Management (DEIM), University of Tuscia, Via del Paradiso, 47, Viterbo, 01100 , Italy Abstract Lean production and Industry 4.0 are two concepts that have been studied in recent years ...
Abstract. The paper's objective is to review the journal's articles submitted between the years 2010-2019 in reputed journals and conferences in the field of industry 4.0 and its associated technologies. The paper deals with the work carried out by researchers in the area of Industry 4.0, smart supply chain management, digital supply ...
The Systematic Literature Review approach is used in this study to assess the areas under consideration. According to the theoretical framework offered, an exact SLR generally assists in generating a solid understanding of what is known in a subject field, detecting research trends and trajectories, and setting a research plan [].]. "The growth of evidence-based practice has resulted in an ...
7. Methodological approaches adopted by Industry 4.0 research. Industry 4.0 literature is characterized by a prevalence of conceptual papers. Piccarozzi et al. found for instance in their systematic review on Industry 4.0 in management studies 54 percent of conceptual papers, mainly literature reviews and developments of models/frameworks. As ...
This article investigates the impact of the COVID-19 pandemic on logistics and supply chain processes through a two-phase analysis. First, a literature review maps the existing studies, published from 2021 to 2023 (101 papers), offering a view of the multiple challenges faced by supply chains during the pandemic emergency. The literature analysis makes use of descriptive statistics, thematic ...