Academia.edu no longer supports Internet Explorer.
To browse Academia.edu and the wider internet faster and more securely, please take a few seconds to upgrade your browser .
Enter the email address you signed up with and we'll email you a reset link.
- We're Hiring!
- Help Center
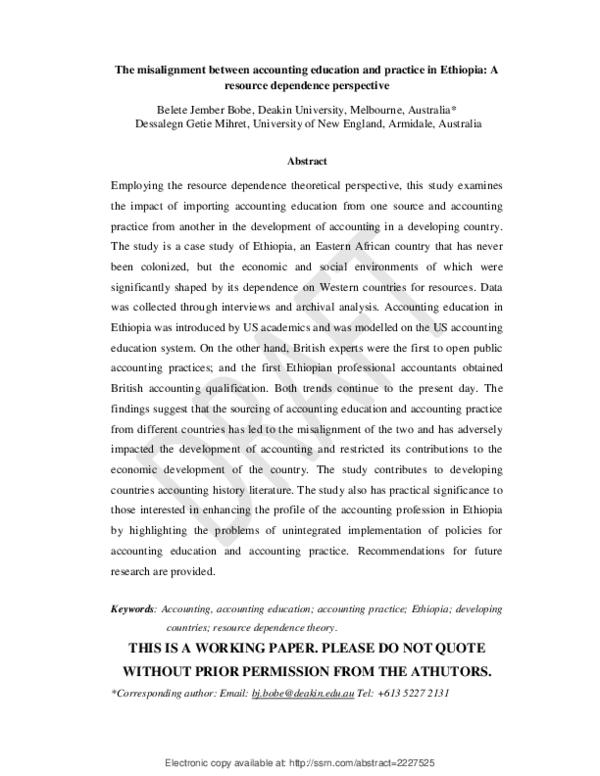
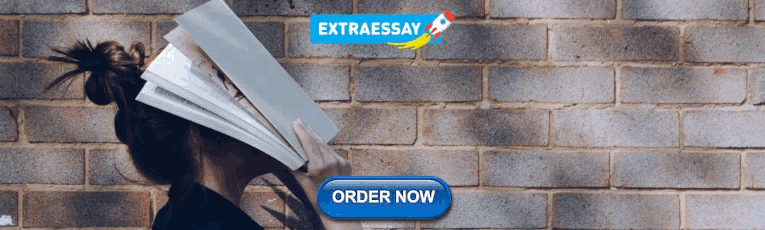
The Misalignment between Accounting Education and Practice in Ethiopia: A Resource Dependence Perspective

2011, SSRN Electronic Journal
Related Papers
Accounting, Auditing & Accountability Journal
firehiwot batisso
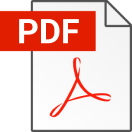
endale bersisa
International journal of business and social research
Deresse Mersha
engida bayou
The objective of the research was to identify the threats that impede adherence to professional ethics among selected Authorized Accountants and Authorized Auditors in Ethiopia. The pillars of ethical values rest on integrity, objectivity, professional competence and due care, confidentiality and professional behavior. Observance of these pillars of ethics was evaluated in light of threats and safeguards. The research approach employed for the study was mixed research augmented with simple statistical analysis. Respondent Authorized Accountants and Authorized Auditors were selected using snowballing technique. The data collected from the subjects of the study was validated by triangulation. Bankers and Tax Auditors were identified as principal users of the financial reports and thus used to validate the data. Key informants interview with persons having close ties with the profession was conducted to enrich the data. The findings indicate that there are lapses in the observance of p...
obsinet tadesse
IFRS FITABILITY IN OUR COUNTRY ETHIOPIA
International Journal of Research in Commerce, IT and Management
Akanksha Khanna
Financial Studies
Business, Management and Education
Purpose: The demand for accountants is increasing from time to time because of the increase in the complexity of the business environment. Higher education institutions are responsible for producing well qualified and ethical accountant required by different organisations. Specifically, Technical and Vocational education and Training (TVET) colleges play a great role in training middle and lower level accountant in Ethiopia. However, academicians, practitioner and employers are raising concern on the excellence and significance of training given in TVET colleges. The objective of this research is to evaluate the education and training in accounting offered by public and private colleges in West Oromia region, Ethiopia. Research Methodology: This study used the cross-sectional survey to obtain the opinion of the trainee, trainers and college deans on how TVET in accounting program is performing about each of the components of CIPP model and identify the knowledge and skill gap as com...
Maru Ayanaw
International Financial Reporting Standards (IFRSs) have been adopted by more than 200 countries across the globe. The main objective of IFRSs is to enhance comparable, transparent and accountable financial information for users. However, its adoption and implementation bring opportunities and pose challenges to the adopter(s). The objective of this study is to assess key challenges of IFRS adoption in public utility enterprises in Ethiopia. A descriptive survey design is used for this study. The target population of the study was the three public utility enterprises which are Ethiopian power utility, Ethio-telecom and Addis Ababa water and sewerage authority as target population for this study. But the Addis Ababa water and sewerage authority are yet to start conversion to IFRS. Due to this excluded from target population. 36 respondents from IFRS project team members and finance staffs of the enterprises who were fully engaged in IFRS adoption and who have IFRS knowledge were selected using purposive sampling. Primary data were collected through questionnaire while secondary data were collected through documentary evidence and primary data is analyzed by using different descriptive statistical tools. Finally, the result of the study revealed that cost of IFRS implementation, system incompatibility and support from regulatory body and professional association, nature of IFRS requirement, weak institutional level support, knowledge gap and Poor project management were key challenges that hinder the adoption of IFRS in public utility enterprises. And recommend on importance of strong professional accountancy body that can have a professional membership with IFAC, collaboration and coordination of AABE with different stakeholders.
Loading Preview
Sorry, preview is currently unavailable. You can download the paper by clicking the button above.
RELATED PAPERS
Tariku Burka
International Journal of Financial Management
Publishing India Group , Dakito Alemu , Abraham J Bayeh
Business Excellence and Management
Alemu Tadesse
Critical Perspectives on Accounting
Fatima Mohamed Mahmoud AlShingiti
European Journal of Business and Management
Nila Hidayat
Asian Journal of Basic Science & Research
Addisu Gemeda
Amos Nyabiosi
refera matewosk
Amanuel Tsegaye
Accounting History
Vincent De Castro
Bayou Tsegaw
Biniyam Abate
Mohammed Adem
International Journal of Business Ethics in Developing Economies
Publishing India Group
Tekalign N E G A S H Kebede
Amanuel Gezmu
Hamdu Kedir Mohammed
Alem Legese
Konan Anderson Seny Kan
Eshetu Teka
Dr.Eshetu T E K A Anchemo
International journal of scientific and research publications
Netsanet Shiferaw
Silamlak Desta
Kanbiro Orkaido
Department of accountancy, the malawi polytechnic
john chilima , DICKSON FYOP
Research Papers
Trevor Hopper , Philippe Lassou
Samuel Mulugeta
International journal of social science and economic research
Dr. Preeti Kulshrestha
TAMRAT AFESHA
john chilima
The Impact of IFRS on Audit Quality Measurement: a review of two selected private commercial bank of Ethiopia
Tilahun Minwuyelet Terefe
Ezra Kakozi
RELATED TOPICS
- We're Hiring!
- Help Center
- Find new research papers in:
- Health Sciences
- Earth Sciences
- Cognitive Science
- Mathematics
- Computer Science
- Academia ©2024
Academia.edu no longer supports Internet Explorer.
To browse Academia.edu and the wider internet faster and more securely, please take a few seconds to upgrade your browser .
- We're Hiring!
- Help Center
Accounting and Finance
- All Departments
- 17 Documents
- 86 Researchers
Enter the email address you signed up with and we'll email you a reset link.
- Academia.edu Journals
- We're Hiring!
- Help Center
- Find new research papers in:
- Health Sciences
- Earth Sciences
- Cognitive Science
- Mathematics
- Computer Science
- Academia ©2024

- Amharic Audio Bible
(BA Degree Accounting) Sample Research Papers
43 comments:.

It is so nice work!
Thank you Micky Man for your comment!!!
It is so nice work
ya good paper
it is good.
I learn from this paper,thank you
I usually keep my mouth shut in the face of the many hilarious errors that crop up in the burgeoning world of datasets for cultural analytics, but this one is too good to pass up.
Buddy, the model researches were a lot helpful in conducting my study. Thanks a lot, God bless!!
I noticed a lot of students asking on Social medial platforms about the B.A research papers. Good to see, you share those research papers here. I must share link of this blog with them. They definitely try this out and happy to get the research papers.
I am impressed with your work and skill. Thank you so much for sharing and keep on shearing that types of interesting articles. Get cardboard hang tags net worth
It will be fine in PDF form
Nice to be visiting your blog again, it has been months for me. Well this article that i’ve been waited for so long. I need this article to complete my assignment in the college, and it has same topic with your article. Thanks, great share. BA final year time table 2019
I would like to have best and brief samples of research proposals on accounting and finance
WOW so nice paper
So beautiful
Best for your contribution
Is there any titile for Bsc in accounting
how we can the PDF one
My name is Mrs Nadia Albert from Russia, And i am a happy woman today through the help of a Loan lender, Mr Russ Harry. I will refer any person that is looking for loan to this Loan firm. He gave happiness to me and my family, i was in need of a loan of $500,000.00 to start my life all over as i am a single mother with 2 kids I met this honest and GOD fearing man loan lender that help me with a loan of $500,000.00 US Dollar, at a low Rate. He is a God fearing man, if you are in need of loan and you will pay back the loan please contact him Via [email protected] /Whatsapp number+393511617486
RU BA Final Year Time Table 2020 Rajasthan University BA 3rd Year Time Table 2020 Uniraj BA 3rd Exam Date Sheet 2020
Enter your comment...v.good paper
Good to see you
Its so better
Hello. Take this opportunity to get credit if you need it and you are a trusted person who doesn't want protocol credit. * Financial credits * Home loans * Investment loans * Automatic loans * Consolidation debt * Personal credits. Do not hesitate to submit your request if you are interested in an amount ranging from 5,000 to 8,000,000.00 euros with a repayment period of 1 year to 35 years with an interest rate of 1.5% Thank you very much . WhatsApp contact number: +33 7 52 33 67 24 Email address: [email protected]
Thank you so much. Today I saved from big challenge because of you.
I want to share with you all on how I got my loan from a very good and honest loan company registered in the USA, as I was applying as a start-up loan it took me several documents to meet up with their conditions but God Willing I got through and it was smooth to work with as an entrepreneur.Contact [email protected] for all kinds of financial assistance such as loans and investment facilities.
My name is Mr. Klaus Dieter, I work for the French financial institution Crédit Mutuel with the partnership of the bank BNP Paribas, we offer you offers at an interest rate of 3% per year, to start with new projects: - You have unpaid debt. - Buying a new house. - buy a new car. - Buying a new apartment or property. Get personal loans, business loans. If you would like loans for setting up your business and project, do not hesitate to send us an email: [email protected] Whatsapp: +4917694954827

I was searching for loan to sort out my bills& debts, then i saw comments about Blank ATM Credit Card that can be hacked to withdraw money from any ATM machines around you . I doubted thus but decided to give it a try by contacting ([email protected]} they responded with their guidelines on how the card works. I was assured that the card can withdraw $5,000 instant per day & was credited with$50,000,000.00 so i requested for one & paid the delivery fee to obtain the card, after 24 hours later, i was shock to see the UPS agent in my resident with a parcel{card} i signed and went back inside and confirmed the card work's after the agent left. This is no doubts because i have the card & has made used of the card. This hackers are USA based hackers set out to help people with financial freedom!! Contact these email if you wants to get rich with this Via: [email protected] or WhatsApp +1(360)6370612

We offer private, commercial and personal loans with an annual interest rate of very low temperatures up to 2% per year with a maturity of 50 years, anywhere in the world. We offer loans between US $ 5,000 and US $ 100 million. Lender Email Address: [email protected] Sincerely, Mr. Garry Jones. [email protected]

Wow best proposed financial
INSTEAD OF BITCOIN INVESTMENT SCAM WHY DON'T YOU CONTACT MR OSCAR FOR A REAL BLANK ATM CARD [email protected] or whats-app +1(209)-643-1515. My name is Morgan Williams am from Alabama United State,this is so real and wonderful, at first i thought is a scam , because have been scam by several people claiming they can help me invest my money in bitcoin trading , that is how i lost my $25,000 last week on investment , but with the help of Mr Oscar White Blank ATM Card [email protected] , i was able to withdraw $50,000 from ATM machine without trace more than the money i lost last week , indeed Mr Oscar your Blank ATM card is real and genuine , i will keep telling people about you as i promise to do , if you are in any financial problem to pay up bills and start up a new life , kindly contact Mr Oscar white on how you can obtain his Blank ATM card , he does not charge big , trust him and contact him today through email [email protected] ,whats-app +1(209)-643-1515
Thank you for sharing it igot information iam looking for it.
I recently needed a private investigator or a hacker to help me pull one or two strings for the successful completion of my goal. Over the years I have came in contact with different types of scammers and users until I almost gave up then I heard of the name WHITEHATS- To my greatest surprise it came out that the WHITECOLLARS are all I have been searching for many years. Contact them for any hacking services for ease and fast delivery of any operation that involves a computer, other services like Phone Spy, Phone hack, Phone tracking, Credit boost up with all credit bureaus, Credit card top up, Bank Loading and many more. Mail- WhitehatspytechATcyberservicesDOTcom or WhitehatstechAtgmailDotcom, YOU WILL BE SURE TO THANK ME LATER
Give me clear example of research on management
How download research
Free must download Apps
The Negative Effect of Temperature Variability on Household Wealth in Low- and Middle-Income Countries
- Open access
- Published: 28 August 2024
Cite this article
You have full access to this open access article
- Ida Brzezinska 1 &
- Paul Jasper 1
Climate change distributes its negative impacts on societies unequally. Low- and middle-income countries (LMICs) are disproportionately affected by climate change, which threatens to undo global progress in poverty reduction. Temperature variability has been shown to reduce macro-economic growth and to negatively affect household wealth. Climate models predict that LMICs are located in areas that will experience the largest increases in temperature variability. Understanding the effects of an increasingly variable climate on social and economic outcomes is thus of key importance. Yet current literature on the link between temperature variability and poverty has limited geographical coverage, with evidence from many LMICs still missing. In addition, the data used in studies have tended to be at a low level of spatial disaggregation. This paper combines remote sensing temperature data from the Reanalysis Fifth Generation (ERA5) global climate dataset for 2018 and micro-estimates of relative household wealth at a 2.4 km spatial resolution predicted by machine learning (ML) algorithms from (Chi et al., 2022 ) in a Spatial First Differences (SFD) research design. We find a remarkably robust negative effect of increases in day-to-day temperature variability on household wealth across 89 LMICs. Our heterogeneity analysis shows that the magnitude of negative effects of day-to-day temperature variability on household wealth is largest in Sub-Saharan Africa, in low-income countries, and in tropical climates. Altogether our findings highlight the need to build climate resilience across LMICs, for instance through climate-responsive social protection programmes – particularly in the most affected geographies and climates .
Similar content being viewed by others
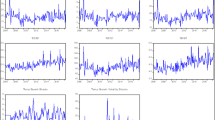
Climate shocks and wealth inequality in the UK: evidence from monthly data
The Effects of Climate Change on Poverty and Income Distribution: A Case Study for Rural Mexico
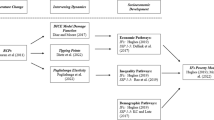
How many people will live in poverty because of climate change? A macro-level projection analysis to 2070
Avoid common mistakes on your manuscript.
Introduction
Climate change continues to increase the frequency and intensity of extreme weather events, exerting widespread negative impacts on societies around the world – particularly on people living in poverty (Jafino et al. 2020 ). Recent climate models show that low- and middle-income countries (LMICs) are located in ‘hot-spot’ areas, where increases in temperature variability due to climate change are projected to be the greatest (Bathiany et al. 2018 ). According to projections of relative changes in temperature variability until the end of the twenty-first century in Bathiany et al. ( 2018 ), climate variability is expected to decrease in most high-income countries – while LMICs will experience the largest increases in variability. This is one of the exemplifiers of the issue of climate injustice, with countries that contributed the least to climate change likely to be the ones most affected by its negative effects.
Given the projected changes in climate variability, a crucial question relates to the impact of increased temperature variability on economic and social outcomes. Recent evidence shows that variations in temperature can negatively affect macro-economic growth. Kotz et al. ( 2021 ) analyse subnational economic data for 1,537 regions worldwide over a 40 year period, finding that, on average, an extra degree of day-to-day temperature variability reduces the regional economic growth rate by 5 percentage points. Linsenmeier ( 2021 ) uses satellite data on nightlights as a proxy for economic activity and similarly finds that day-to-day variability has negative effects on economic growth at most temperature levels. Consistent with the unequal impacts of climate change mentioned above, Dell et al. ( 2008 ) show that temperature fluctuations reduce economic growth in low-income countries but have little effect in rich countries.
Alongside those looking at macro-economic outcomes, many studies have analysed the impact of temperature variability on household poverty. Agriculture is a common focus area. Temperature variability has been associated with lower agricultural productivity, decreased yields and thus reduced farm income in Togo (Yevessé, 2021 ), Nigeria (Ishaya et al. 2014 ), and El Salvador (Ibanez et al. 2021 ). Also, temperature variability and temperature shocks have been directly linked to reductions of food and non-food consumption in Vietnam (Narloch & Bangalore 2016 ), Mexico (Skoufias et al. 2011 ), and Malawi (Asfaw & Maggio 2018 ). Other studies find that temperature variability can negatively affect educational outcomes, including the likelihood of a child completing any education in Ethiopia (Randell & Gray 2016 ). Finally, adverse effects of temperature variability on poverty can materialise through lower labour productivity (Ibanez et al. 2021 ; Markham & Markham 2005 ; Cachon et al. 2012 ).
Although there is a sizeable literature on the link between temperature variability and poverty, geographical coverage remains limited, with evidence from most LMICs still lacking. In addition, studies to date have typically considered poverty outcomes at a coarse level of spatial disaggregation, obscuring the spatial distribution of poverty at smaller areas. We leverage a novel high-resolution dataset on household wealth and poverty from Chi et al. ( 2022 ). This consists of micro-estimates of relative household asset wealth for 89 LMICs at an unprecedented 2.4 km spatial resolution predicted from machine-learning (ML) algorithms on the basis of Demographic and Health Surveys (DHS), connectivity data from Facebook, demographic maps, and geo-spatial datasets. We combine this with remote sensing temperature data from 2018 at a 0.5 degrees spatial resolution taken from the Reanalysis Fifth Generation (ERA5) global climate time-series data. We apply a Spatial First Differences (SFD) approach that addresses unobserved heterogeneity in cross-sectional data that is dense in space (Druckenmiller & Hsiang 2018 ), and a variety of robustness checks, to identify the causal impact of day-to-day temperature variability on household wealth in LMICs.
Through the use of high-resolution ‘big data’ on climate and household wealth, as well as novel analytical approaches, we aim to address the following research question:
Is there a causal relationship between day-to-day temperature variability and wealth in LMICs and does it vary across regions?
Existing Literature
The literature that links temperature variability to poverty and wealth in LMICs is broad. Although many studies have looked at the effects of absolute changes in temperature and temperature extremes on wealth and poverty, we focus on the literature that specifically investigates temperature variability . We first very briefly touch on the literature that investigates factors that explain variation and changes in temperature variability, then review theoretical approaches that seek to explain the link between temperature variability and poverty or wealth in LMICs, before turning to a summary of existing empirical evidence related to this issue.
Factors Affecting Changes in Temperature Variability
Like many climatic variables, temperature variability is affected by local, regional, and global factors whose impacts materialise at different time-scales. For instance, studies that have identified global factors affecting changes in temperature variability have highlighted the effects of climate change and how these materialise differently across regions (Bathiany et al. 2018 ). More locally, studies have identified urbanisation (Tam et al. 2015 ), deforestation (Ge et al. 2022 ), and changes in soil moisture and sea-ice loss (Bathiany et al. 2018 ) as factors associated with a change in temperature variability. Hence, while changes in temperature variability can generally be explained by global drivers, there is some evidence that at a local level they might also be affected by human activity, such as for example urban build up.
Theory: Temperature Variability Affecting Poverty and Wealth in LMICs
Three main theoretical mechanisms via which temperature variability might affect poverty have been discussed in the literature. First, temperature variability has been linked to the concept of poverty traps (Banerjee & Duflo 2011 ). Given that households experiencing extreme poverty are more likely to live in rural areas and to be engaged in agriculture than other households (The World Bank 2022 ), temperature variability increases uncertainty and volatility of farm income for many poor households. This, in turn, makes it more difficult for households to break out of poverty traps, and increases the likelihood of families being pushed into them. Second, and in a similar vein, theoretical modelling has linked climate change, temperature increases, and increased temperature variability to a reduction of farm productivity and rents, hence reducing a key source of income for most of the world’s poor (Mendelsohn et al. 1994 ). Third, increases in temperature variability and the likelihood of temperature shocks have been theoretically linked to increases in firm production costs, in turn reducing firm productivity and, via this channel, wealth (Melitz 2003 ; Foltz et al. 2018 ).
Existing Empirical Evidence on the Effects of Temperature Variability
There is a growing body of evidence that temperature variability negatively affects aggregate levels of economic growth. For instance, Kotz et al. ( 2021 ), find that an extra degree of day-to-day variability results in a 5-percentage point reduction in growth rates in the regions included in their study. Other studies find similar negative relationships between temperature variability and growth, although effects vary by location, with LMICs, often in hotter climates, being more severely affected (Linsenmeier 2021 ; Dell et al. 2008 ). Other studies have found evidence for negative effects of temperature variability on health-related outcomes, such as mortality rates and birth outcomes (Andalón et al. 2014 ; Hovdahl 2020 ).
At the household level, studies have directly linked increases in temperature variability and temperature shocks with reductions of food and non-food consumption in Vietnam (Narloch & Bangalore 2016 ), Mexico (Skoufias et al. 2011 ), and Malawi (Asfaw & Maggio 2018 ). Others have focused explicitly on rural households and linked temperature to decreases in agricultural income in Ghana (Nkegbe & Kuunibe 2014 ), and rural consumption growth in Tanzania (Letta et al. 2018 ). Haile et al. ( 2018 ) find that negative effects of heat waves on rural households vary by climatic condition, when comparing results from Ghana, Uganda, and Tanzania. This highlights the need for broad analyses that cover a variety of different contextual conditions while also allowing for localised disaggregation of effect estimates.
Looking at other welfare-related outcomes, studies that use household-level data have found evidence that temperature fluctuations are associated with lower education completion rates (Randell & Gray 2016 ), lower levels of income from crops (Yevessé, 2021 ), and lower cereal yields (Ishaya et al. 2014 ). In addition, temperature variability has been found to lead to lower labour productivity in non-agricultural sectors, both in LMICs and in rich economies, particularly in precarious employment, such as at industrial plants or construction sites, where exposure to health risks is higher (Ibanez et al. 2021 ; Markham & Markham 2005 ; Cachon et al. 2012 ).
Gaps and our Contribution
There is hence a significant body of evidence for negative effects of temperature variability and shocks on aggregate economic indicators and household-level outcomes, including consumption, expenditure, and income. The size of these effects seems to vary, depending on the agroecological and climatological characteristics of the area a particular study covers.
As far as we know, no large-scale study has been conducted so far that aggregates data across a global set of LMICs, while at the same time focussing on high resolution, i.e. small area measurements of household wealth. Our study aims to close this gap, providing both a globe-circumventing analysis of this relationship between temperature variability and household wealth in LMICs, and a high-resolution perspective on this. This allows for disaggregating effect estimates by a variety of different contextual variables.
Data and Methods
Climatic data, data sources.
The main data source for climatic variables is the Reanalysis Fifth Generation (ERA5) global climate time-series data for the years 1979 to 2019 taken from the European Centre for Medium-Range Weather Forecasts (ECMWF). ERA5 combines satellite data with historical observations from land stations, aircrafts and radars into global estimates using advanced modelling and data assimilation systems (Hersbach et al. 2020 ). For our main estimation, we use only the climatic data for 2018 to match the time period of our household wealth data (see below). Variables are recorded on a regular grid of 0.5 by 0.5 degrees spatial resolution with global geographical coverage. For our purposes, a crucial advantage of this dataset is the highly disaggregated hourly temporal resolution. This allows us to construct our measure of day-to-day temperature variability from the temperature variable, defined as the temperature of air at two metres above the surface of land, sea, or inland waters, in Kelvins (K). We also process the rainfall flux variable, defined as the rate of rain that falls to the Earth’s surface. For additional analyses that incorporates historical and long-term measures of temperature variability, we make use of pre-2018 observations of this time-series.
To remove the influence of the seasonal temperature cycle, we use an additional climatic dataset to construct a measure of seasonal temperature difference, which serves as a control variable in our main analytical specification. This data comes from the Climatic Research Unit (CRU) Version 4 gridded time-series climatic dataset on a 0.5 latitude by 0.5 longitude regular grid over all land domains of the world except Antarctica. The spatial resolution of this climatic time-series matches that of our main data source – ERA5. Observations are derived by the interpolation of monthly climate anomalies from extensive networks of weather station measurements (Harris et al. 2020 ). The available time range spans 1901–2018. We concentrate on monthly values of temperature, defined as mean two meters above-surface, in the historical reference period of 1979 to 2018. All values are defined in degrees Celsius. Additionally, we rely on a global 0.5 degree resolution dataset with the Koppen-Geiger climate classification in order to identify climatic zones into which grid-cell observations of climatic variables fall (Beck et al. 2018 ).
Constructing Temperature Variability Indicators
Following Kotz et al. ( 2021 ), we construct our main measure of day-to-day temperature variability from the ERA5 dataset as the deviation of daily temperatures from their monthly means, averaged for 2018. For each grid cell, we implement the following steps: 1) aggregate hourly measurements to a mean daily temperature, 2) calculate monthly standard deviations, and 3) average the values of monthly standard deviations for the whole year. This approach is a better measure of day-to-day variability than absolute changes between days, which can be sensitive to changes in observational procedures and not fully reflect seasonal variation (Moberg et al. 2000 ). The result is presented in Fig. 1 . Day-to-day temperature variability displays a strong latitudinal dependence, being the smallest around the equator and increasing at higher latitudes.
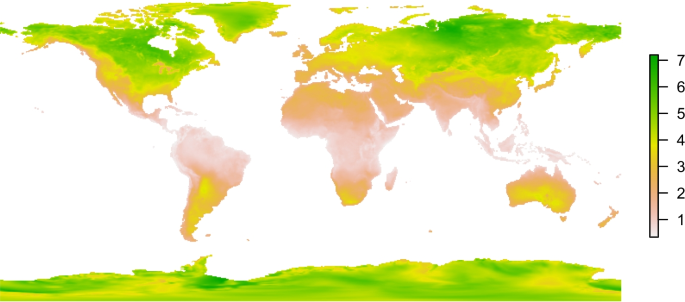
Day-to-day temperature variability (in Kelvins)
For some analyses, we also include long-term measures of day-to-day temperature variability, which are averages of the day-to-day temperature variability measure described above for two historical periods: 1) 1989–2001; and 2) 1989–2017. For our main dependent variable, we rely on estimates of household wealth produced by machine learning (ML) algorithms trained on the latest available “ground truth” DHS data and various Big Data sources. While the DHS data used is mostly from 2018, in some cases this data is not available for that year and an earlier DHS is used. The earliest DHS used to train the algorithm is from 2002–03. Footnote 1 For analyses using historical temperature variability data, and to check whether results are sensitive to a change in how we look at historical temperature variability, we therefore choose two historical periods to construct long-term measures of temperature variability: First, 1989–2001, which excludes any time-period for which DHS data is included in the construction of our main dependent variable. Second, 1989–2017, which covers a longer time span, but which overlaps to a limited extent with DHS data collection years.
Day-to-day variations in temperature could also be driven by the variation introduced by the seasonal temperature cycle. We include a measure of seasonal temperature difference as a control variable in our main specification to filter out the confounding effect of seasonal variation. This is calculated using the CRU Version 4 dataset at the grid-cell level as the difference between the maximum and minimum average monthly temperature in a year. In order to capture the historical trend and minimise the influence of anomalies in a particular year, we calculate this difference for every year during the period 1979 to 2018 and average the values to yield one unique observation per grid cell. We convert temperature units from Celsius to Kelvin for consistency with our main dataset, ERA5. The result is plotted in Fig. 2 . Similar to day-to-day temperature variability, seasonal variation is generally larger at higher latitudes. Note that this dataset does not cover land masses in Antarctica.
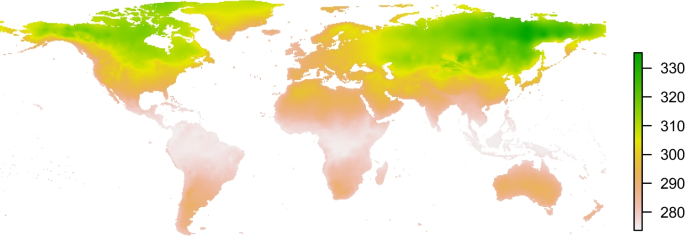
Seasonal temperature difference (in Kelvins)
Apart from temperature variability, previous research has found that rainfall has a significant impact on poverty in LMICs, particularly through droughts. For example, Brunckhorst ( 2020 ) finds that the 2015 drought in Ethiopia reduced annual consumption of affected households by 12 percent, while Hirvonen et al. ( 2020 ) show that chronic undernutrition rates increased in drought-exposed areas with limited road networks. Drought was also found to have negatively affected household consumption in Somalia (Pape & Wollburg 2019 ) and Malawi (Baquie & Fuje 2020 ) and reduced agricultural productivity in Zambia (Chonabayashi et al. 2020 ). Given the importance of rainfall for poverty outcomes, and its potential relationship with other climatic variables such as temperature variability, we include daily rainfall as an additional control in our key regressions below. This variable is constructed on the basis of the hourly rainfall flux observations from the ERA5 dataset for every grid cell. These values, originally expressed in kg m-2 s-1, are converted into daily rainfall in millimetres for ease of interpretation.
Household Wealth
Data source.
As a measure of relative wealth, we rely on micro-estimates of relative wealth for LMICs at a 2.4 km spatial resolution provided by Chi et al. ( 2022 ). These estimates are produced by applying machine learning algorithms to the ‘ground truth’ of household survey data coming from the USAID Demographic and Health Surveys (DHS), data from satellites, mobile phone networks, and topographic maps, as well as deidentified connectivity data from Facebook. The DHS, which is available for 56 LMICs, is geo-referenced, linked with alternative data sources, and fed into the algorithm, which then predicts relative wealth for all 135 LMICs at a 2.4 km grid-cell spatial resolution. The resulting output is cross-sectional in nature and relies on the latest available DHS in any given country, with the latest information coming from 2018. Footnote 2 Importantly, 5 out of the 10 most predicative features in the model used to produce relative wealth predictions are related to Facebook connectivity data, rather than any geo-spatial covariates that could directly capture our measure of day-to-day temperature variability. Footnote 3
A crucial question relates to the accuracy of these micro-estimates of relative wealth. Chi et al. ( 2022 ) conduct validation exercises comparing their household wealth estimates to actual values from four independent data sources that are not used to train the ML algorithm: 1) census data collected from 15 LMICs since 2001; 2) nationally representative samples collected by the Government of Togo from 6,172 households in 2018/2019; 3) nationally representative samples collected by the Government of Nigeria from 22,104 households in 2019; and 4) data from 5,703 Kenyan households in 2018 collected by GiveDirectly, a non-profit humanitarian aid organisation. For the census data, the prediction model explains between 72–86% of the variation in household wealth. Footnote 4 For Togo and Nigeria, the model explains 76–84% and 50–71% Footnote 5 of variation, respectively. Finally, in Kenya, the coefficient of correlation between the wealth predicted by the ML model and actual values in the survey is between 0.41 and 0.78. In addition to validity tests with external data sources, the data set includes an estimated average model error for each 2.4 km grid cell, which we refer to in more detail when accounting for prediction error.
Variables Used
The measure of wealth produced by micro-estimates in Chi et al. ( 2022 ) is the Relative Wealth Index (RWI), derived from ‘ground truth’ DHS data. This is an asset-based measure of wealth constructed by implementing a Principal Component Analysis (PCA) of questions related to asset holdings of households surveyed in the DHS (such as electricity, TV, motorcycle, roof material etc.) (Rutstein & Johnson 2004 ). The resulting RWI is in itself a standardised value, with a mean of zero and a standard deviation of one. Crucially, the RWI is defined relative to other locations within the country. It is therefore not comparable across countries, unless transformed into an absolute wealth index. Since a conversion into absolute values requires relying on per capita GDP and Gini coefficient estimates, which are unavailable or unreliable at the right temporal or geographical resolution in many LMICs, we use the RWI in LMICs as our main measure of household wealth. Because we are particularly interested in how temperature variability affects poverty, we would ideally want to directly observe households’ poverty status, which is typically measured using consumption or income aggregates. However, such data is unavailable at a sufficiently high spatial resolution needed for this study. While we acknowledge that using a consumption or income-based measure would be more suitable to analyses of poverty outcomes, asset-based measures can nonetheless provide important insights into the relationship between temperature variability and household wealth, all while being available at an unprecedented spatial granularity and covering areas across LMICs with relatively higher levels of poverty, compared to high-income countries.
Figure 3 plots the micro-estimates of the RWI derived from machine learning models in Chi et al. ( 2022 ) for all LMICs. Each data point represents a grid cell with a 2.4 km spatial resolution and the associated predicted value of relative wealth. Values should be interpreted as relative to other locations within the same country, where a value of 0 represents the mean. Given the relative nature of our wealth measure, we apply our econometric framework, Spatial First Differences, within countries, i.e. we are only comparing and differencing with regards to observational points that lie within the same country.
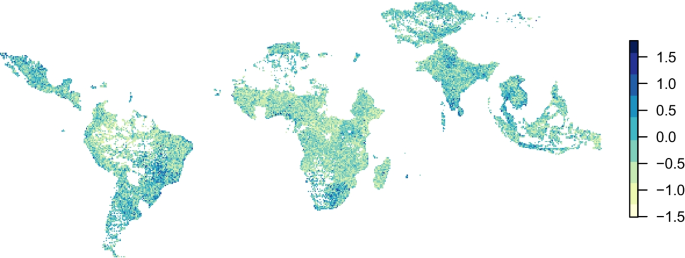
Relative Wealth Index at 2.4 km spatial resolution
Aggregating Data to the Right Spatial Resolution
The first step to processing our temperature and household wealth data for analysis was aggregation to the same spatial resolution. While the ERA5 temperature dataset offers observations at a regular grid of 0.5 longitude by 0.5 latitude (around 50 km at the equator), the micro-estimates of wealth from Chi et al. ( 2022 ) are produced at an exceptionally highly disaggregated spatial level of an irregular grid with a 2.4 km spatial resolution. To obtain comparable units of observation, we aggregated the wealth data to a regular grid of 0.5 × 0.5 degrees. Given our earlier discussion on the RWI being a relative measure of wealth, and therefore its non-comparability across borders, we perform this aggregation within countries. For each 0.5 × 0.5 degrees grid cell, we calculate the mean values of the RWI and ML model error across all data points at a 2.4 km spatial resolution that fall within that grid cell. The process is visualised in Fig. 4 for the example of Ethiopia. The left side shows the initial structure of our household wealth data, with an irregular grid of data points at a 2.4 km spatial disaggregation, each representing an estimate of the RWI. The right-hand side is the outcome of the aggregation, with averaged values overlaid on a regular 0.5 degrees grid.
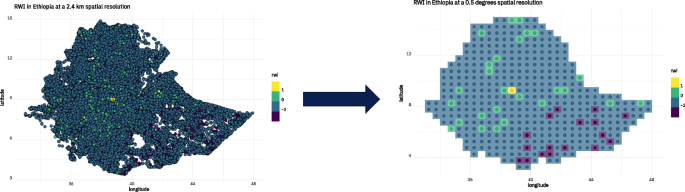
Aggregating household wealth data to a 0.5 degrees spatial resolution
Once both datasets had the same spatial resolution of 0.5 degrees, our next step was to set up a geographical panel structure. This is analogous to methods used with longitudinal panel data in relation to time, except that the geographical panel is defined in space, exploiting the spatial structure of the data. The two identifying dimensions of our panel are: 1) the country code (for which we use i as an index); and 2) ordered grid cells starting from the most north-western observation in each country, moving east, then turning south and reversing the order to go east–west in a ‘snake-like’ manner (for which we use g as an index). A sketch of this process is presented in Fig. 5 .
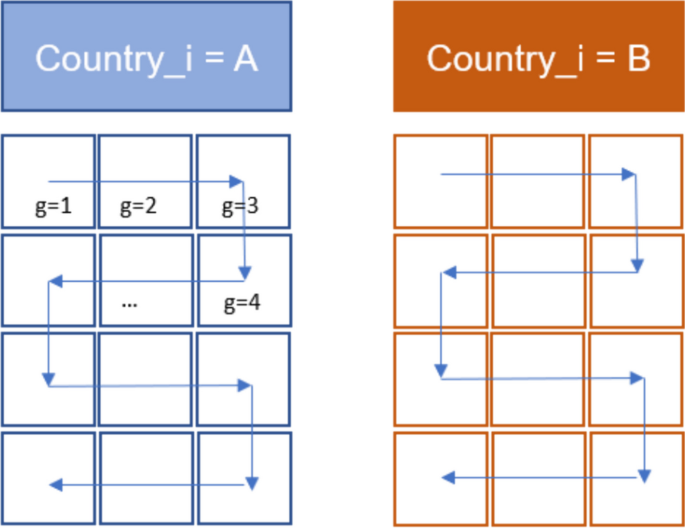
Building a geospatial panel dataset
A crucial question relates to a case where a single temperature grid cell crosses borders, falling within two different countries – and which country it should be assigned to. This is illustrated in Fig. 6 , where yellow dots represent data points assigned to Ethiopia, red dots to Eritrea, and the orange dots for those that fall at the border between the two countries. In this case, we would count the grid cell twice : once as a data point forming part of the panel for Ethiopia, and once as part of the panel for Eritrea. We aggregate the higher resolution wealth data in such a way, however, that each country’s data is only counted once. This means that we have duplicate temperature observations in our data for these overlapping grid cells, but no duplicate wealth observations.
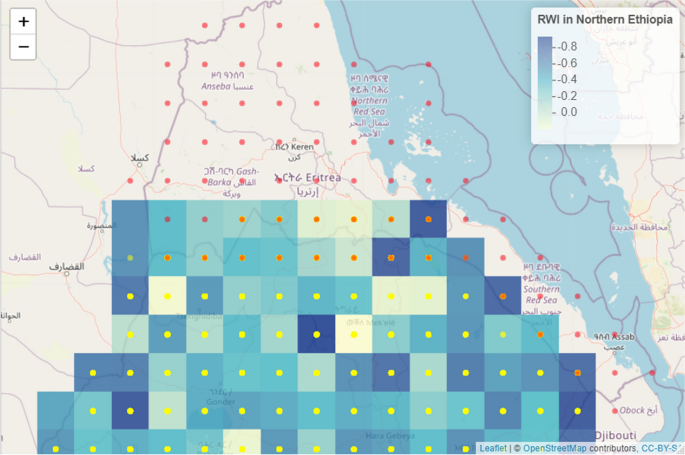
Grid cells crossing borders
Population Density Estimates
To control for population density in a sub-set of our regressions, we use UN-adjusted population density estimates at a 30 arc spatial resolution (approximately 1 km at the equator) for 2018 produced by WorldPop ( 2018 ). These high-resolution estimates are available for all 89 countries in our sample, listed in Table 10 in the Annex. The original units are number of people per square kilometre based on country totals adjusted to match the corresponding official United Nations population estimates that have been prepared by the Population Division of the Department of Economic and Social Affairs of the United Nations Secretariat. In order to match the spatial resolution of the temperature data, we aggregate the population estimates to a 0.5-degree resolution using the “sum” resampling algorithm and divide the population estimates by 1,000. The resulting unit is number of people (1,000s) per 0.5-degree grid cell.
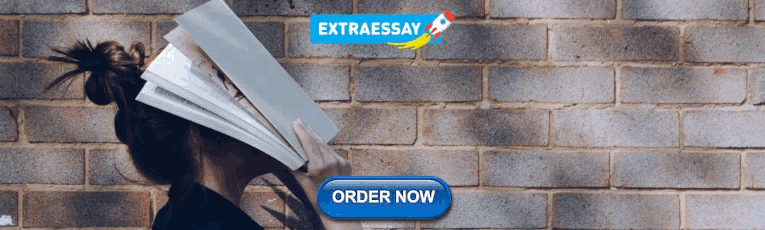
The Final Dataset
The structure of our dataset is shown in Table 1 . At the original spatial resolution of 2.4 km, we have 2,428,569 micro-estimates of wealth. After spatial aggregation and calculation of first differences, we obtain a total of 18,594 grid cells across 89 LMICs spread among six regions. Although in the original (Chi et al. 2022 ) paper, the micro-estimates of wealth are calculated for all 135 LMICs, only 89 of those were made publicly available for download at the time we accessed the data. Footnote 6 The regions that are represented in our data are: Sub-Saharan Africa, Latin America and the Caribbean, South Asia, Middle East and North Africa, East Asia and the Pacific, and Europe and Central Asia. See Table 10 in the Annex for a full list of countries in our data and corresponding regions.
Our Analytical Approach
Spatial first differences.
To identify the causal effect of temperature variability on household wealth in LMICs, we adopt a SFD research design, which can be used to reduce the effect of unobserved heterogeneity in settings with cross-sectional data that is dense in space (Druckenmiller & Hsiang 2018 ). This methodology is conceptually analogous to first differences in time, except the difference is calculated between two spatially adjacent units, i.e. the time index is replaced by a location in space. Since only the neighbouring observations in space are compared, the conditional mean assumption necessary for identification only needs to hold locally , as shown in the equation below .
where observations are indexed by \(i\) (the country they belong to), \(g\) (their location within the country), \(Y\) is the outcome variable (relative household wealth), \(T\) is the treatment variable (temperature variability in our case), and X represents a set of control variables (rainfall and seasonal temperature variation in our main specification, other controls in additional robustness checks and sensitivity analyses). This assumption states that conditional on receiving the same treatment and a set of controls, two spatially adjacent observations should have the same expected outcome. This is a much weaker identifying assumption than the conditional mean assumption in cross-sectional regressions in levels, which requires that all observations with the same treatment conditional on observable covariates need to have the same expected outcome. The SFD design thus reduces the bias due to omitted variables by exploiting the variation between two units adjacent in space that are ‘comparable’ and differencing out the error term that might contain unobserved heterogeneity.
Spatial First Differences using Temperature Variability and Micro-Data on Household Wealth
Main approach.
Our main specification is a linear model of the relationship between temperature variability and asset-based household wealth, with observations indexed by their location in space \(g\) within each country \(i\) .
Here, \(\Delta\) denotes first order differences with respect to \(g\) , \(rwi\) represents the RWI, \(temp\) is the degree of temperature variability, \(rain\) additionally controls for rainfall, \(season\) filters out the influence of seasonal temperature variation, and \(\varepsilon\) is the error term. The unit of observation is the 0.5 degrees latitude by 0.5 degrees longitude grid cell. Standard errors are clustered at the country level to account for spatial autocorrelation across units. The coefficient of interest is \({\beta }_{1}\) , which identifies the causal impact of a one-unit change in the degree of temperature variability on the RWI. Given the nature of this RWI, this coefficient should be interpreted as the average unit change in the wealth index at grid-cell level relative to other grid cells within a country caused by a one-unit change in the degree of temperature variability.
The SFD design relies on calculating first differences between spatially adjacent units, indexed by \(g\) . To check that our results are not sensitive to a specific implementation of the indexing approach, i.e. the snake approach presented in Fig. 5 and hence the direction in which first differences are defined, we implement the SFD estimation with a second indexing approach in addition to the one presented in Fig. 5 . If the identifying assumption of SFD is met, the coefficients obtained by different ways of indexing the data should be similar. For instance, running the ‘snake’ in a different direction (e.g. going south-north first and then east–west) should not lead to different results, as we would still be differencing between neighbours. In this paper, we present results from two approaches:
Method 1: Snake : within each country, we defined the order in which observations should be numbered by the ‘snake’ approach as presented in Fig. 5 . We started from the north-western most observation, moving east while keeping latitude constant, then turning south and reversing the order to go east–west in a ‘snake’. At each step, the first differences are calculated. Note that though observations are dense in space, in some cases the next available grid cell might be far away, potentially violating the local conditional mean assumption. In order to ensure comparability between every two adjacent observations, an empty row was inserted whenever the next observation fell outside of a 100 km radius and no difference was calculated.
Method 2: 100 km radius : for each observation, we also identified all observations that lie within a 100 km radius of the initial point and fall within the same country and calculated the average of the outcome variable (RWI), temperature variability, and control variables (rainfall and seasonal temperature variation). We then subtracted the values for the initial observation from this 100km average and regressed the first differences. In this case, the ‘neighbouring’ cell is an artificial unit that represents averages across neighbours in all directions, hence being a good robustness check on our first method.
The indexing approach for both methods is shown with the example of Northern Ethiopia in Fig. 7 : the ‘snake’ in the left-hand side panel and the radius approach in the right-hand side panel.
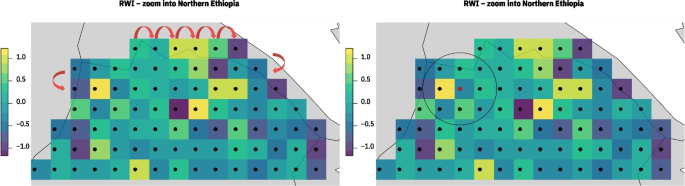
Two methods for indexing data
Heterogeneity Analysis by Region, Income Classification, and Koppen-Geiger Climate Classification
We also conduct heterogeneity analyses to understand if estimated effects vary by geographical region, by running our main specification, but additionally including an interaction term between the spatially differenced day-to-day temperature variability at grid cell level and a categorical variable indicating the region each country and grid cell is located in. The full model used for heterogeneity analysis is shown in Eq. ( 3 ) below. We follow the World Bank Country Classification Footnote 7 for the fiscal year 2024 to define which region each of the countries in our sample belongs to: Sub-Saharan Africa, Latin America and the Caribbean, South Asia, Middle East and North Africa, East Asia and the Pacific, and Europe and Central Asia. A full list of countries and regions in our dataset is shown in Table 10 in the Annex.
Our coefficients of interest are \({{\varvec{\upbeta}}}_{5}\) , which is the vector of individual coefficients for each of the interaction terms of regions and differenced temperature variability included in the regression (represented by the \(\text{i}.region\) term, with the exception of the base region). Each of these coefficients in \({{\varvec{\upbeta}}}_{5}\) represent how the impact of temperature variability on the RWI changes when comparing the respective region with the base region. If our estimated coefficients ( \({\widehat{{\varvec{\upbeta}}}}_{5})\) are significantly different from zero, this indicates that effects vary significantly across regions – compared to the base region. We set the base region to Europe & Central Asia. We are also interested in the sum of \({\upbeta }_{1}\) with each of the individual components of \({{\varvec{\upbeta}}}_{5}\) (e.g. \({\upbeta }_{1}+{\beta }_{5,Latin America}\) ), which represents the full effect of temperature variability on the RWI in each region. If estimates of combinations of these coefficients are different from zero in any given region, this indicates that there is a significant effect of temperature variability in this region. Footnote 8
We replicate this approach for an additional analysis using a different type of country grouping: the World Bank’s 2018 income classification system. Footnote 9 This system assigns countries to low-income, lower-middle-income, and upper-middle-income groups based on national per-capita income. For countries in our sample, the 2018 classification can be found in Table 10 in the Annex. We use the same specification as in Eq. ( 3 ), just changing the groupings of countries from regions to income groups. Again, we are interested in whether effects vary across groupings and whether effects are significantly different from zero within each group.
Similarly, we conduct heterogeneity analysis by climate zones, using the Koppen-Geiger climate classification. We estimate Eq. ( 4 ) below, where \(KG\) indicates a binary variable which assigns each grid cell to the Koppen-Geiger climate classification. We rely on a global 0.5 degree resolution dataset, which classifies each grid cell according to the Koppen-Geiger climate classification (Beck et al. 2018 ). We use the newest release of the dataset, which covers the present-day classification (1991–2020). The five main groups according to the Koppen-Geiger climate classification are: A (tropical), B (arid), C (temperate), D (continental), and E (polar).
The coefficients in the vector \({{\varvec{\upbeta}}}_{5}\) indicate how the impact of day-to-day temperature variability on household wealth differs for each of the Koppen-Geiger climate classifications, when compared to the base category. We set the base category to be Group A (tropical climate). The sum of coefficients \({\upbeta }_{1}\) and each individual component of \({{\varvec{\upbeta}}}_{5}\) – as above – shows the full effect of temperature variability on the RWI for each of the Koppen-Geiger climate categories.
Main Robustness Checks
Spatial double difference.
We estimate a few key variations to our main specification defined above for robustness check purposes. First, we follow Druckenmiller and Hsiang ( 2018 ) and take higher order differences of observations in adjacent units within countries and estimate the SFD on these. This means that we rely on the same linear model as our main specification but take double spatial differences of all variables. This so-called Spatial Double Difference (SDD) model is given by the equation below:
Here, \({\Delta }^{2}\) represent the spatial ‘double-difference’ transformations, i.e. for example: \({{\Delta }^{2} rwi}_{ig}= {\Delta rwi}_{ig}-\Delta rw{i}_{i\left(g-1\right)}.\) Druckenmiller and Hsiang ( 2018 ) argue that if the estimated coefficients of the simple SFD procedure and SDD are similar, it is likely that local conditional independence holds.
Controlling for Modelling Uncertainty in Underlying Wealth Data
In a second robustness check, we re-estimate all our main specifications using an amended household wealth dataset. Given that our household wealth data is based on modelling output, i.e. predictions produced by Chi et al. ( 2022 ), one could argue that our estimates are influenced by prediction uncertainty or prediction modelling error. In order to assess the plausibility of this, we use the modelling error data at 2.4 km grid-level shared by Chi et al. ( 2022 ) and re-estimate our specification on two new datasets: 1) excluding all countries with average model error in the top 50% of the distribution, and 2) excluding all 2.4 km grid-cells that lie in the top 50% of the modelling error distribution. These datasets are hence comprised of ‘high-quality’ predictions of our key outcome variable only.
Reverse Causality
It is possible that the relationship between temperature variability and relative household wealth could be driven by reverse causality. For example, if wealthier households build up their living environment with increased infrastructure, which in turn affects temperature variability, then higher levels of household wealth in certain grid cells might be driving differences in temperature variability across cells and not vice-versa. Inspired by Linsenmeier's ( 2021 ) approach to tackle a similar problem, we deal with this by re-running our main specifications with two different measures of long-term historical temperature variability: one covering the years 1989–2001 and one covering the years 1989–2017. Both measures precede the year for which the relative wealth index is estimated (2018), while the first one precedes any time-period in which underlying ground-truth DHS data was collected. We argue that there is no possibility of reverse causality in the form of observed wealth distributions affecting temperature variability in these specifications.
Controlling for Population Density
We run additional specifications in which we include population density as a control variable to investigate and address two key issues: first, our main outcome variable is measured using predictions from a machine learning algorithm. Some of the features (though as we explain in the above section the less important ones) used to produce these predictions – such as road density, population, and if urban or built up – could be related to temperature variability. Our analysis might hence be influenced by these underlying relationships between predictors and temperature variability. We argue that including population density in our specifications allows us to assess how sensitive our results are to controlling for some of these features. Second, it is possible that our SFD approach is not able to appropriately account for how temperature variability might be related both to household wealth and the location people live in. The relationship we identify might simply reflect how wealthier households live in more densely populated areas – which have different temperature variability characteristics than less densely populated areas. Including population density in our specifications means that we are comparing grid-cells with similar density levels when trying to identify the effect of temperature variability on wealth, hence controlling for this issue.
Headline Results
Ordinary least squares.
To illustrate the potential Omitted Variable Bias (OVB) problem that could be introduced by unobserved heterogeneity in cross-sectional regressions in levels, we first run a simple Ordinary Least Squares (OLS) regression of temperature variability on the RWI, without taking first differences. Table 2 shows the results for four models: (1) no control variables, (2) including a control for rainfall, (3) additionally controlling for seasonal temperature variability, and (4) including country fixed effects. Standard errors are clustered at the country level to allow for autocorrelation. In all cases, the coefficient of day-to-day temperature variability on the RWI is positive and statistically significant at the 1% level, indicating a positive impact of temperature variability on the asset-based index of wealth. More specifically, a one-unit increase in the degree of day-to-day temperature variability is found to be related to an increase in the RWI of 0.04–0.10 units.
This result is not only counterintuitive, as it suggests that higher degrees of climate variability possibly increase wealth, but also most likely does not have a meaningful interpretation due to the unobserved heterogeneity that we expect in the error term, biasing the coefficient. The identifying assumption for the cross-sectional regression in levels states that all units of observation that receive the same treatment (temperature variability) should have the same expected outcome conditional on control variables. This is unlikely to hold, as we are regressing data points from 89 LMICs. Each country (and – in the case of column (4) – each region within a country) has different characteristics that might affect household wealth through channels other than temperature variability. The omission of these factors in the regression will cause OVB. On the other hand, in an SFD setting, the conditional mean assumption only needs to hold locally, between points close in physical space. We look at results from our SFD estimations next.
Spatial First Differences Method 1: Snake
In Table 3 , we present our main SFD estimation results, based on specification Eq. ( 2 ) and on Method 1 (‘snake’ SFD) indexing method presented in Fig. 5 . We present three models, which only differ by covariates included in the regressions. The results vary starkly from OLS results in Table 2 . First, the coefficient on the day-to-day temperature variability variable has now turned negative, and significantly so. Across all three models, it is highly significant.
These results imply that – once accounting for unobserved heterogeneity by performing spatial first differences – an increase in temperature variability is significantly and negatively related with average relative wealth among households in each geographical grid-cell in our sample. The negative effect of a one-unit increase in temperature variability on relative wealth lies between -0.16 and -0.19 on average across all countries in our sample. We interpret these results to imply that temperature variability negatively affects household wealth and – as a consequence – likely increases poverty in LMICs.
Spatial First Differences Method 2: 100 km radius
To demonstrate that these results are not a feature of our ‘snake’ indexing approach, Table 4 presents SFD results implemented using Method 2 (100 km radius) approach. This means that we run specification Eq. ( 2 ) by taking first differences of outcomes between every unit of observation and the 100 km radius average. As before, the first model in column (1) considers the relationship between temperature variability and household wealth without any control variables. Column (2) adds a control for rainfall, and column (3) additionally adjusts for the seasonal temperature cycle. These results confirm the findings derived from implementing SFD using our ‘snake’ approach: in all cases, there is a highly statistically significant (at 1%) negative impact of day-to-day temperature variation on the RWI. In fact, our main coefficient estimates of interest are remarkably similar to the ‘snake’ approach, varying between -0.16 and -0.19.
Heterogeneity Analysis by Region and Income Classification
We present results from our heterogeneity analysis by region in Fig. 8 . We plot estimated combined coefficients on temperature variability and the regional interaction terms in specification Eq. ( 3 ) – i.e. \(\widehat{{\beta }_{1}+{{\varvec{\beta}}}_{5}}\) – together with the 95% confidence interval, indicated by the width of the whiskers in the graph below. A full regression table that shows coefficients, including the specific coefficients estimated for each interaction term and control variables included in this specification, is presented in Table 13 in the Annex.
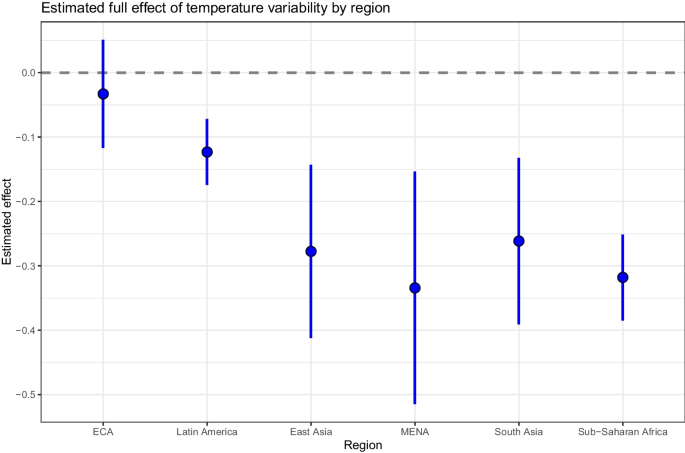
Effect of day-to-day temperature variability by region
The results show that in Sub-Saharan Africa, Middle East & North Africa (MENA), East Asia & the Pacific, and South Asia the effects of day-to-day temperature variability on household wealth are negative and significantly different from zero. As shown by the value of the coefficients, the magnitude of the negative effect is the largest in Sub-Saharan Africa, followed by MENA, East Asia & the Pacific, and South Asia. Effects are not statistically significantly different from zero in Europe & Central Asia.
In Fig. 9 , we present results from a similar heterogeneity analysis, this time grouping countries according to the World Bank’s income classification system (2018). Again, we plot full effects, where this time the interaction terms are over country income groupings. A full regression table is presented in Table 18 in the Annex. The results show that while across all groupings we estimate effects of temperature variability on wealth to be negative, the magnitude of the effect is largest among low-income countries. Because poverty levels are highest among this group of countries, and our findings imply that temperature variability negatively affects wealth most significantly here, we interpret this as evidence that suggests that temperature variability also likely increases poverty.
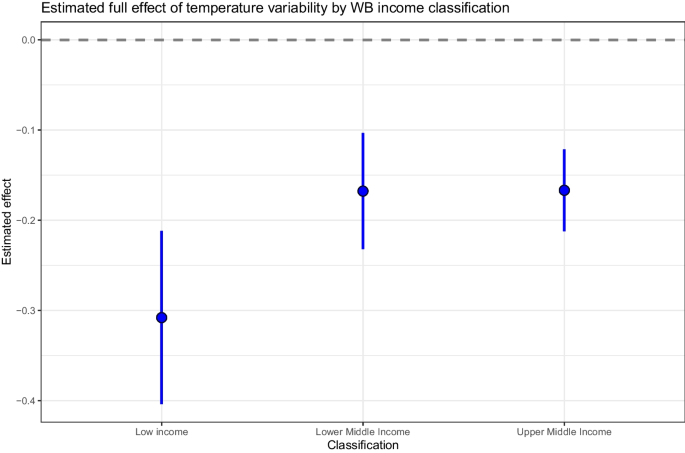
Effect of day-to-day temperature variability by World Bank income classification
Heterogeneity Analysis by Koppen-Geiger Climate Classification
In addition to heterogeneity by region, we show how the effects of temperature variability on household wealth vary by climatic zones into which grid-cells fall, which might cross country and regional boundaries. We use the Koppen-Geiger climate classification, which has five main groups: A (tropical), B (arid), C (temperate), D (continental), and E (polar) (Beck et al. 2018 ). As before, we plot estimated combined coefficients on temperature variability and the climate classification interaction terms from specification Eq. ( 4 ) – i.e. \(\widehat{{\beta }_{1}+{{\varvec{\beta}}}_{5}}\) – together with the 95% confidence interval, in Fig. 10 . The full regression table is shown in Table 14 in the Annex.
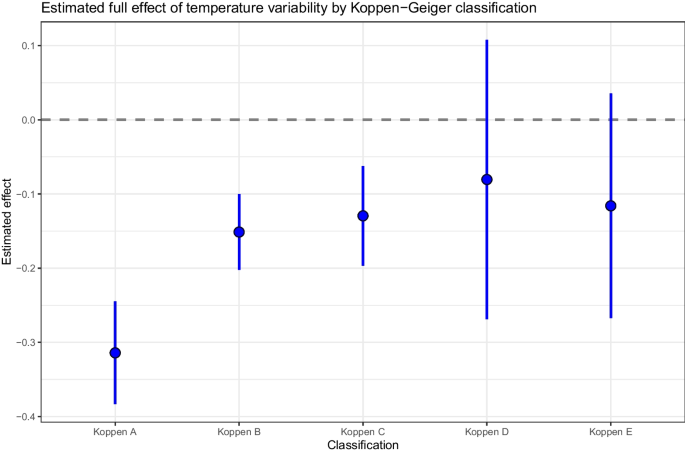
Effect of day-to-day temperature variability by Koppen-Geiger climate classification
The results show that the negative effect of temperature variability on household wealth is largest for group A in the Koppen-Geiger climate classification. This means that households in tropical areas are most impacted by temperature variability. After group A, households in group B (arid) and group C (temperate) experience the largest magnitude of the negative effect of temperature variability on wealth, although the confidence intervals of the two estimates overlap – meaning that there is no statistically significant difference between the effects estimated for arid and temperate areas. In the case of groups D (continental), and E (polar), the estimated effect is not statistically different from zero. Footnote 10
Robustness Checks
We also implemented a ‘double-difference’ transformation to our data to then implement the SDD estimation as a robustness check. We present results in Table 5 , together with the original SFD results. The SDD results are presented in the rows with the tag (2nd diff). The key finding here is that the significant negative relationship between temperature variability and relative wealth persists. Estimated coefficients across our models are now smaller (-0.11 to -0.14), but this is expected, given possible attenuation bias that results from the double-difference transformation (Druckenmiller & Hsiang 2018 ). Again, these results strengthen our headline findings of the significant negative effects that increases in temperature variability have on household wealth.
Limiting the Wealth Data to ‘High-Quality’ Predictions
We also re-estimate our main specifications for observations with ‘high-quality’ RWI predictions only. We determine ‘high-quality’ observations based on model error at two levels of disaggregation: 1) country level, and 2) 2.4 km grid-cell level.
Table 6 presents the results for the Method 1 (‘snake’ SFD) approach and SDD using ‘high-quality’ predictions at the country level. This involves re-running our main regression models for a sub-set of countries that have average model error in the bottom 50% of the distribution. Despite the lower number of observations resulting from this, our key coefficient estimates are in line with previous findings and remain highly statistically significant. Across specifications including first- and second-order differences and a set of control variables, we obtain a negative effect of day-to-day temperature variability on household wealth in the range of -0.15 to -0.19.
The results from applying Method 2 (100 km radius SFD) to the sub-set of ‘high-quality’ country level observations is shown in Table 7 . The coefficients on day-to-day temperature variability have values between -0.16 and -0.19, similar to those obtained with Method 1 (‘snake’ SFD) in Table 6 . Our second approach to accounting for model error is to re-estimate our main specifications for a sub-set of 2.4 km grid-cells that have model error in the bottom 50% of the distribution. Results for Method 1 (‘snake’ SFD) and Method 2 (100 km radius SFD) are shown in Table 11 and Table 12 in the Annex, respectively. Using only ‘high-quality’ observations at the grid-cell level, we obtain key coefficients largely in line with our previous findings, in the range of -0.09 to –0.13. This suggests that our headline results were unlikely to be significantly influenced by uncertainty related to predictions of RWI, as measured by the grid-cell level model error.
One concern with the interpretation of our earlier results relates to reverse causality. As described above, we deal with this by regressing the RWI on a long-term measure of day-to-day temperature variability for two time periods which precede the household wealth data. We use the average day-to-day temperature variability in two periods: 1) 1989–2001; and 2) 1989–2017. For the temperature variability measure averaged for 1989–2001, the results from an SFD regression using the ‘snake’ method, as well as the spatial double difference, are shown in Table 8 . The coefficient on day-to-day temperature variability remains negative and highly statistically significant. Full regression tables, including for the average between 1989–2017 and using the 100 km radius approach are shown in Table 15 , Table 16 , Table 17 in the Annex. Our results remain unchanged across different specifications. We interpret this to mean that this relationship is unlikely to be driven by reverse causality.
In column (4) of Table 9 , we present results of our main SFD specification (using Method 1 ‘snake’) when including population density. Our main coefficient of interest on temperature variability and related significance levels do not change meaningfully when compared to the specification in column (3), which excludes population density as an explanatory variable. This can be interpreted in three ways. First, we argue that this indicates that our results are not sensitive to the inclusion of and are unlikely to be driven by a set of predictors included in the original algorithm used by Chi et al. ( 2022 ). Basically, this is evidence that our results are not resurfacing a relationship between household wealth and temperature variability that was previously introduced by the algorithm used to predict the RWI across LMICs. Second, we argue that this is evidence that our results are not driven by a simple correlation between temperature variability, wealth, and high population density – and by implication urbanisation – which our SFD approach might not have been able to deal with appropriately. Finally, these results indicate that location choice cannot explain the mechanism by which temperature variability might be affecting household wealth: even when holding population density levels constant, and after the SFD transformation, we find that higher temperature variability is associated with lower household wealth.
We leverage a newly available high-resolution ‘big data’ dataset on relative household wealth and poverty from Chi et al. ( 2022 ) and remote sensing temperature data from the ERA5 global climate time-series data in a SFD framework to explore a key question around the causal impact of day-to-day temperature variability on household wealth in LMICs. In the age of climate change, a key concern is understanding the effects of increasing temperature variability on social and economic outcomes in the regions that are projected to be most affected. Our research uses an innovative approach which shows that high-resolution estimates of household wealth, derived from ML algorithms, as well as novel analytical methods in causal inference, can be used in downstream research to investigate policy-relevant questions – particularly around climate-responsive social protection, as discussed below.
Our findings show a highly statistically significant negative effect of increases in day-to-day temperature variability on household wealth in LMICs, which is remarkably robust across different econometric specifications. This result provides evidence that households in LMICs will likely be negatively impacted by increases in temperature variability. In light of this, our findings highlight the importance of building climate resilience amongst vulnerable populations in LMICs. One potential channel is through climate-responsive social protection programmes, such as anticipatory cash transfers triggered by climatic shocks, or agricultural insurance schemes indexed to weather conditions.
Climate projections predict an unequal effect of climate change on temperature variability trends globally, with LMICs expected to see the largest increase in temperature variations (Bathiany et al. 2018 ). This highlights the need to identify geographies where the impact of day-to-day temperature variability on household wealth is strongest – and conversely, weakest. Our heterogeneity analysis shows that the magnitude of negative effects of day-to-day temperature variability on household wealth is largest in Sub-Saharan Africa, followed by Middle East & North Africa, East Asia & Pacific, and South Asia, when compared to Europe & Central Asia. In addition, our results show that negative effects are largest among low-income countries, compared to lower-middle- or upper-middle-income countries. We also conduct heterogeneity analysis by climate zones and show that households in tropical climates will be most affected by the negative effects of temperature variability on household wealth, followed by arid and temperate climates. This implies that the importance of climate-responsive social protection is largest in those geographies and climate zones. Future studies could undertake a more spatially disaggregated heterogeneity analysis. The high resolution of household wealth data could be used to indicate small areas that will be most impacted, up to a 2.4 km spatial resolution. This disaggregation in the data could inform geographical targeting of social policy, allowing for identification of populations most affected by climatic shocks.
Finally, it is important to keep in mind that our analysis remains cross-sectional in nature. Therefore, it provides only a snapshot of the relationship between day-to-day temperature variability and asset-based household wealth at a particular point in time, rather than an insight into poverty dynamics over time. This is because the micro-estimates of wealth that we rely on were only produced for the latest available ‘ground truth’ DHS data. Such small area estimates of poverty, produced either using machine learning or statistical modelling techniques, often rely on non-traditional ‘big data’ sources of information as its predictors. This offers an opportunity to leverage high-resolution datasets that are frequently updated, such as geo-spatial and mobile phone data, in order to produce dynamic high-resolution and high-frequency ‘poverty maps’, which change the spatial distribution of poverty as new information becomes available. Our analysis, as well as any future studies looking at the relationship between climate change and poverty using high-resolution data, could then investigate poverty dynamics. Small area estimation techniques could also be used to estimate high-resolution consumption or income data, in addition to asset-based wealth measures used in this study.
Data Availability
Data is provided within the manuscript.
This is the case for Indonesia, where the latest DHS data available to the authors is from 2002–03.
The detailed list of all ground truth data sources can be found in Table S1 of the online Appendix . The latest DHS data comes from 2018. However, in some countries the survey from that time period was not available. In that case, an earlier DHS was used.
These are: number of mobile devices, number of WiFi access points, number of cell towers, number of Android devices, number of iOS devices. The remaining 5 features are: radiance, road density, population, if urban or built up, and one out of over 100 satellite images.
Specifically, the ML model explains 72% of variation when data from 15 census countries is pooled together and 86%, on average, when countries are considered individually.
The lower figure is obtained when aggregating individual geo-referenced data points from independent data sources to the 2.4 km grid-cell level and the higher figure comes from aggregation at the lowest administrative unit in those countries – cantons for Togo and local government areas for Nigeria.
The data is publicly available to download from the Humanitarian Data Exchange site: Relative Wealth Index—Humanitarian Data Exchange (humdata.org) . At the time we accessed the data, the latest version was from 8th April 2021. All the analysis in this paper is based on this version. Since then, new micro-estimates for countries not originally available for download were added to the website. We will continue accessing new micro-estimates of wealth as they become available for public download and update our results.
Full list of countries and regions under the World Bank classification can be found here: World Bank Country and Lending Groups – World Bank Data Help Desk .
In practice, we estimate the combinations of these coefficients using the ‘lincom’ command in R, after running specification (4). See https://search.r-project.org/CRAN/refmans/biostat3/html/lincom.html .
A full list of countries by income classification, including for 2018, can be found here: https://datahelpdesk.worldbank.org/knowledgebase/articles/906519 .
Note that most of our grid cells fall into groups A-C, with only a small number of grid cells classified as either group D or group E. The exact numbers are: 9,260 (50%) in Group A, 6,069 (33%) in Group B, 2,658 (14%) in Group C, 276 (2%) in Group D, and 315 (2%) in Group E.
Andalón M, Azevedo JP, Rodríguez-Castelán C, Sanfelice V, Valderrama D (2014) Weather shocks and health at birth in Colombia. Policy Research Working Papers. The World Bank. https://doi.org/10.1596/1813-9450-7081
Asfaw S, Maggio G (2018) Gender, weather shocks and welfare: evidence from Malawi. J Dev Stud 54(2):271–291
Article Google Scholar
Banerjee A, Duflo E (2011) Poor economics. PublicAffairs
Baquie S, Fuje H (2020) Vulnerability to poverty following extreme weather events in Malawi. Working Paper. World Bank, Washington, DC. https://doi.org/10.1596/1813-9450-9435
Bathiany S, Dakos V, Scheffer M, Lenton T (2018) Climate Models Predict Increasing Temperature Variability in Poor Countries. Sci Adv. https://doi.org/10.1126/sciadv.aar5809
Beck HE, Zimmermann NE, McVicar TR, Vergopolan N, Berg A, Wood EF (2018) Present and Future Köppen-Geiger Climate Classification Maps at 1-Km Resolution. Sci Data 5(1):180214. https://doi.org/10.1038/sdata.2018.214
Brunckhorst B (2020) Rural mobility and climate vulnerability: evidence from the 2015 drought in Ethiopia. 2020–17. CSAE Working Paper Series. Centre for the Study of African Economies, University of Oxford. https://ideas.repec.org/p/csa/wpaper/2020-17.html
Cachon GP, Gallino S, Olivares M (2012) Severe weather and automobile assembly productivity. SSRN Scholarly Paper ID 2099798. Social Science Research Network, Rochester, NY. https://doi.org/10.2139/ssrn.2099798
Chi G, Fang H, Chatterjee S, Blumenstock JE (2022) Microestimates of Wealth for All Low- and Middle-Income Countries. Proc Natl Acad Sci 119(3):e2113658119. https://doi.org/10.1073/pnas.2113658119
Chonabayashi S, Jithitikulchai T, Qu Y (2020) Does agricultural diversification build economic resilience to drought and flood? Evidence from poor households in Zambia. African Journal of Agricultural and Resource Economics 15(1):65–80. https://doi.org/10.53936/afjare.2020.15(1).05
Dell M, Jones B, Olken B (2008) Climate change and economic growth: evidence from the last half century. Am Econ J Macroecon 4 (July). https://doi.org/10.3386/w14132
Druckenmiller H, Hsiang S (2018) Accounting for unobservable heterogeneity in cross section using spatial first differences. Working Paper. Working Paper Series. National Bureau of Economic Research. https://doi.org/10.3386/w25177
Foltz JD, Traore N (2018) Temperatures, productivity, and firm competitiveness in developing countries: evidence from Africa. In: 2018 annual meeting, August 5–7, Washington, D.C. Agricultural and Applied Economics Association, p 274434. https://ideas.repec.org/p/ags/aaea18/274434.html
Ge J, Liu Qi, Zan B, Lin Z, Sha Lu, Qiu Bo, Guo W (2022) Deforestation Intensifies Daily Temperature Variability in the Northern Extratropics. Nat Commun 13(1):5955. https://doi.org/10.1038/s41467-022-33622-0
Haile B, Signorelli S, Azzarri C, Johnson T (2018) Welfare Effects of Weather Variability: Multi-Country Evidence from Africa South of the Sahara. PLoS ONE. https://doi.org/10.1371/journal.pone.0206415
Harris I, Osborn TJ, Jones P, Lister D (2020) Version 4 of the CRU TS Monthly High-Resolution Gridded Multivariate Climate Dataset. Sci Data 7(1):109. https://doi.org/10.1038/s41597-020-0453-3
Hersbach H, Bell B, Berrisford P, Hirahara S, Horányi A, Muñoz-Sabater J, Nicolas J et al (2020) The ERA5 Global Reanalysis. Q J R Meteorol Soc 146(730):1999–2049. https://doi.org/10.1002/qj.3803
Hirvonen K, Sohnesen TP, Bundervoet T (2020) Impact of Ethiopia’s 2015 Drought on Child Undernutrition. World Dev 131:104964. https://doi.org/10.1016/j.worlddev.2020.104964
Hovdahl I (2020) Deadly variation: the effect of temperature variability on mortality. No 01/2020. Working Papers. Centre for Applied Macro- and Petroleum economics (CAMP), BI Norwegian Business School. https://ideas.repec.org/p/bny/wpaper/0084.html
Ibanez AM, Romero J, Velasquez A (2021) Temperature shocks, labor markets and migratory decisions in El Salvador. https://conference.iza.org/conference_files/ClimateChange_2021/velasquez_a26669.pdf
Ishaya (2014) Post-Adaptation Vulnerability of Cereals to Rainfall and Temperature Variability in the Federal Capital Territory of Nigeria. Ethiop J Environ Stud Manag 7:532. https://doi.org/10.4314/ejesm.v7i5.7
Jafino BA, Walsh B, Rozenberg J, Hallegatte S (2020) Revised estimates of the impact of climate change on extreme poverty by 2030. The World Bank
Kotz M, Wenz L, Stechemesser A, Kalkuhl M, Levermann A (2021) Day-to-Day Temperature Variability Reduces Economic Growth. Nat Clim Chang 11(4):319–325. https://doi.org/10.1038/s41558-020-00985-5
Letta Marco, Montalbano Pierluigi, Tol Richard (2018) Temperature Shocks, Short-Term Growth and Poverty Thresholds: Evidence from Rural Tanzania. World Dev 112:13–32
Linsenmeier M (2021) Temperature variability and long-run economic development. 110499. LSE Research Online Documents on Economics. London School of Economics and Political Science, LSE Library. https://ideas.repec.org/p/ehl/lserod/110499.html
Markham S, Markham I (2005) Biometeorological Effects on Worker Absenteeism. Int J Biometeorol 49:317–324. https://doi.org/10.1007/s00484-004-0246-y
Melitz MJ (2003) The Impact of Trade on Intra-Industry Reallocations and Aggregate Industry Productivity. Econometrica 71(6):1695–1725
Mendelsohn R, Nordhaus W, Shaw D (1994) The Impact of Global Warming on Agriculture: A Ricardian Analysis. Am Econ Rev 84(4):753–771
Google Scholar
Moberg A, Jones PD, Barriendos M, Bergström H, Camuffo D, Cocheo C, Davies TD et al (2000) Day-to-Day Temperature Variability Trends in 160- to 275-Year-Long European Instrumental Records. J Geophys Res Atmos 105(D18):22849–22868. https://doi.org/10.1029/2000JD900300
Narloch U, Bangalore M (2016) Environmental risks and poverty: analyzing geo-spatial and household data from Vietnam. World Bank Policy Research Working Paper No. 7763. https://papers.ssrn.com/sol3/papers.cfm?abstract_id=2814397
Nkegbe PK, Kuunibe N (2014) Climate variability and household welfare in Northern Ghana. Working Paper 2014/027. WIDER Working Paper. https://doi.org/10.35188/UNU-WIDER/2014/748-6
Pape U, Wollburg P (2019) Impact of drought on poverty in Somalia. World Bank Working Paper, January.
Randell H, Gray C (2016) Climate Variability and Educational Attainment: Evidence from Rural Ethiopia. Glob Environ Chang 41:111–123. https://doi.org/10.1016/j.gloenvcha.2016.09.006
Rutstein SO, Johnson K (2004) The DHS wealth index August. https://dhsprogram.com/publications/publication-cr6-comparative-reports.cfm
Skoufias E, Rabassa M, Olivieri S (2011) The poverty impacts of climate change: a review of the evidence. World Bank Policy Research Working Paper 5622
Tam BY, Gough WA, Mohsin T (2015) The Impact of Urbanization and the Urban Heat Island Effect on Day to Day Temperature Variation. Urban Clim 12:1–10
The World Bank (2022) Poverty and shared prosperity: correcting course. https://www.worldbank.org/en/publication/poverty-and-shared-prosperity
WorldPop, WorldPop ( www.worldpop.org -School of Geography and Environmental Science, University of Southampton, Department of Geography and Geosciences, University of Louisville, Departement de Geographie, Universite de Namur, and Columbia University (2018) Global high resolution population denominators project - Funded by The Bill and Melinda Gates Foundation (OPP1134076)
Yevessé D (2021) Effects of temperature and rainfall variability on the net income of cereal crops in Togo: semi-parametric approach. AERC | African Economic Research Consortium. Research Paper 435. https://aercafrica.org/aerc-doc/effects-of-temperature-and-rainfall-variability-on-the-net-income-of-cereal-crops-in-togo-semi-parametric-approach/
Download references
Acknowledgements
This paper was funded by the Data and Evidence to End Extreme Poverty (DEEP) project. DEEP is a consortium of the Universities of Cornell, Copenhagen, and Southampton led by Oxford Policy Management, in partnership with the World Bank’s Development Data Group and funded by the UK Foreign, Commonwealth & Development Office.
Author information
Authors and affiliations.
Oxford Policy Management Limited, Ground Floor, 40-41 Park End Street, Oxford, OX1 1JD, United Kingdom
Ida Brzezinska & Paul Jasper
You can also search for this author in PubMed Google Scholar
Contributions
IB and PJ conceived the concept for this project. IB processed the data and produced most analytical code. PJ provided project supervision and reviewed all analytical code. Both authors contributed to interpreting the results. Both authors contributed to writing and approved the final manuscript.
Corresponding author
Correspondence to Ida Brzezinska .
Ethics declarations
Competing interests.
The authors declare no competing interests.
Additional information
Publisher's note.
Springer Nature remains neutral with regard to jurisdictional claims in published maps and institutional affiliations.
See ( Table 10 )
See (Table 11 )
See (Table 12 )
See (Table 13 )
See (Table 14 )
See (Table 15 )
See (Table 16 )
See (Table 17 )
See (Table 18 )
Rights and permissions
Open Access This article is licensed under a Creative Commons Attribution-NonCommercial-NoDerivatives 4.0 International License, which permits any non-commercial use, sharing, distribution and reproduction in any medium or format, as long as you give appropriate credit to the original author(s) and the source, provide a link to the Creative Commons licence, and indicate if you modified the licensed material. You do not have permission under this licence to share adapted material derived from this article or parts of it. The images or other third party material in this article are included in the article’s Creative Commons licence, unless indicated otherwise in a credit line to the material. If material is not included in the article’s Creative Commons licence and your intended use is not permitted by statutory regulation or exceeds the permitted use, you will need to obtain permission directly from the copyright holder. To view a copy of this licence, visit http://creativecommons.org/licenses/by-nc-nd/4.0/ .
Reprints and permissions
About this article
Brzezinska, I., Jasper, P. The Negative Effect of Temperature Variability on Household Wealth in Low- and Middle-Income Countries. EconDisCliCha (2024). https://doi.org/10.1007/s41885-024-00160-6
Download citation
Received : 28 March 2024
Accepted : 26 July 2024
Published : 28 August 2024
DOI : https://doi.org/10.1007/s41885-024-00160-6
Share this article
Anyone you share the following link with will be able to read this content:
Sorry, a shareable link is not currently available for this article.
Provided by the Springer Nature SharedIt content-sharing initiative
- Temperature variability
- Climate change
JEL Classification
- Find a journal
- Publish with us
- Track your research
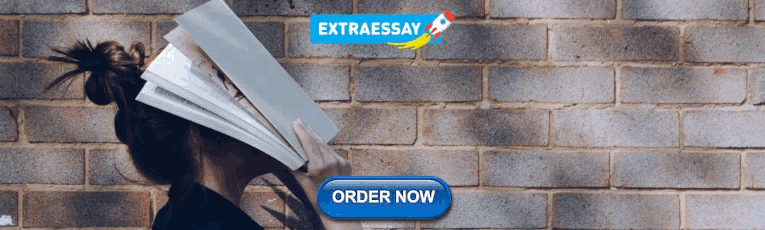
IMAGES
VIDEO
COMMENTS
assessment of electronic banking implementation in selected commercial banks in ethiopia: hailu, seble: jul-2023: determinants of internal audit effectiveness in bank of abyssinia with specific reference to addis ababa districts of the bank: nigussie, samuel: apr-2023: factors affecting of non-performing loans: in the case of development bank ...
AN ASSESSEMENT OF CHARITIS AND SOCIETIES ACCOUNTING IN ETHIOPIA: THE IMPACT OF 70/30 (ADMINISTRATIVE AND OPERATIONAL COST) REGULATION IN ADDIS ABABA. Abeba Edesa Emebet Meselew Woubitu Tafesse. FACULTY OF BUSINESS FACULTY DEPARTMENT OF ACCOUNTING. APPROVED BY THE COMMITTEE OF EXMINER.
commercial bank ethiopia at toba branch) by: dasalegn misgana a research paper submitted to department of accounting and finance in a partial fulfillment of the requirement for ba degree in accounting and finance advisor: sintayu tulu (msc) jimma university college of business and economics departement of accounting and finance june, 2017
establishment and determination of the procedure of the Accounting and Auditing Board of Ethiopia" (AABE), a regulatory government body bestowed with the responsibility of overseeing the implementation of IFRS in Ethiopia by all concerned entities. In Ethiopia, mandatory adoption of IFRS was scheduled in the following phases:
Table 1 & 2 indicates level of education and qualification of the respondents. Accordingly, out of 86 respondents, 76 (88.4%) have BA or BSc degree and 10 (11.6%) of the respondents have Master‟s degree in different educational qualifications. These results indicated that almost all banks possessed first degree.
BA in Accounting and Finance Draft Curriculum 6.3. Degree Nomenclature BA Degree in Accounting and Finance and the Amharic version is: የአርት ባችለር ዲግሪ በአካውንቲንግና ፋይናንስ 6.4. Medium of Instruction The medium of instruction for the program is English Language
and submitted in partial fulfillment of the requirements for the degree of Master of Science in Accounting and Finance complies with the regulations of the University and meets the accepted standards with respect to originality and quality. Approved by: External Examiner: Mehari Mekonnen (PHD) Signature_____Date_____
designed for partial fulfillment for the requirement of Master in Business Administration. This study is conducted to assess the Impact of Digital Banking on P. ofitability in the banking business in Ethiopia, the case of commercial banks in Ethiopia. It will mainly assess the impact of adopting digital banking with.
Other accounting education studies have examined how accounting academicians can be motivated and what should be taught in classrooms (Madawaki, 2015). The accounting profession is emerging and playing an increasingly important role as Ethiopian's economy is being integrated into the world market. Changes in Ethiopia's business environment have
f Finance and Economic DevelopmentSt. Mary's UniversityAbstractThe study was conducted in Addis Ababa University, 6 Kilo campus, Addis Ababa, Ethiopia from February 19, 2014 to May 19 t. assess the practice of fixed asset management of the organization. Descriptive type of the study method was employees through collection of.
ACCOUNTING AND FINANCIAL research - Free download as Word Doc (.doc / .docx), PDF File (.pdf), Text File (.txt) or read online for free. This document is a research paper submitted by Chala Diribsa to the Department of Accounting and Finance at Haramaya University in Ethiopia in partial fulfillment of a bachelor's degree. The paper examines the challenges and opportunities of interest-free ...
on commercial banks in ethiopia a research paper submitted to st. mary's school of graduate studies for partial fulfillment of msc. in accounting and finance by: tigist alemayehu gissila advisor: mohammed seid (ass. professor) st. mary's university school of graduate studies graduate program in accounting and finance march, 2021
This shows the dependence of the profession on this sector.' (Participant 1) 4.3. Accounting education in Ethiopia Historical sketch 14 Accounting education in Ethiopia is examined using the Accounting and Finance Department of Addis Ababa University as the main reference to represent the accounting education component of the study.
Integrated Pharmaceutical Logistics System (IPLS), User Satisfaction, and Logistics Performance: Evidence from Ethiopian Hospitals. This study assessed the effect of IPLS on user satisfaction and logistics performance of HIV/AIDS commodities. Data were collected from 155 respondents in 13 public and private hospitals using questionnaires.
Do not hesitate to submit your request if you are interested in an amount ranging from 5,000 to 8,000,000.00 euros with a repayment period of 1 year to 35 years with an interest rate of 1.5% Thank you very much . WhatsApp contact number: +33 7 52 33 67 24 Email address: [email protected]. Thank you so much.
BA in Accounting and Finance. 1. Background of the Program. Accounting as a profession emerged following the ever increasing needs of mankind in the social, economic and business environment. The accounting profession has shown a continuous and tremendous growth in the business world. This evolution of the accounting practice ranges from the ...
Mary's University a thesis entitled: ""Accounting Information system practice and its impact on accounting and audit reporting quality Case Study in Awash Insurance Company S.C ", in partial fulfillment of the requirements for the MBA in Accounting and Finance of the St Mary's University. Misraku Molla (PhD) Supervisor December, 2021
REQUIREMENTS FOR THE MASTER OF SCIENCE DEGREE IN FINANCE AND INVESTMENT BY CHAINA ALEMAYEHU IDNO PR0017/01 UNDER THE SUPERVISION OF Dr.D.Guruswamy (Asst. Professor) MAY, 2010 MEKELLE, ETHIOPIA View metadata, citation and similar papers at core.ac.uk brought to you by CORE provided by IDS OpenDocs
Dear respondents. ta relating to the"Assessment on credit risk management practice of commercial b. nk of Ethiopia."For fulfillment of the requirements of the thesis for the Masters in Accounting and finance program of St.Mar. University (MSc). The research will be conducted to assess credit risk management practice of commercial bank of ...
School of Management and Accounting Assessing the impact of Core Banking and service quality on Customer Satisfaction in Commercial Bank of Ethiopia (A case of Bishofftu Branch) By: Endalkachew Abebe A research project submitted in partial fulfillment of the requirement for masters of business administration degree in marketing Management
Bank of Ethiopia at Debre Berhan A Thesis submitted to College of Business and Economics of Debre Berhan University in partial fulfillment of the requirement for the Degree of Masters of Business Administration (MBA). By: Zerihun Takele ADVISOR: Shifera Bekele (PHD) May, 2018 Debre Berhan, Ethiopia
Programme name: BA in Accounting & Finance Programme type: undergraduate Mode of delivery: Classroom and community-based Duration: 3 years for regular, 4 years for extension and distance and 5 years for summer programs Graduation Requirements . To graduate from the program the student should take all courses offered in the program, a total of 180 EtCTS for regular and 170 EtCTS for evening and ...
"Financial Reporting Proclamation of Ethiopia" which obliges companies to follow IFRS in their financial statement presentation. The National Bank of Ethiopian (NBE) and the Securities and Exchange Commission also adopted this date for compliance and has issued guidance compliance circulars to ensure full implementation of IFRS in Ethiopia.
The unit of observation is the 0.5 degrees latitude by 0.5 degrees longitude grid cell. Standard errors are clustered at the country level to account for spatial autocorrelation across units. The coefficient of interest is \({\beta }_{1}\) , which identifies the causal impact of a one-unit change in the degree of temperature variability on the RWI.