Have a language expert improve your writing
Run a free plagiarism check in 10 minutes, automatically generate references for free.
- Knowledge Base
- Methodology
- Data Collection Methods | Step-by-Step Guide & Examples
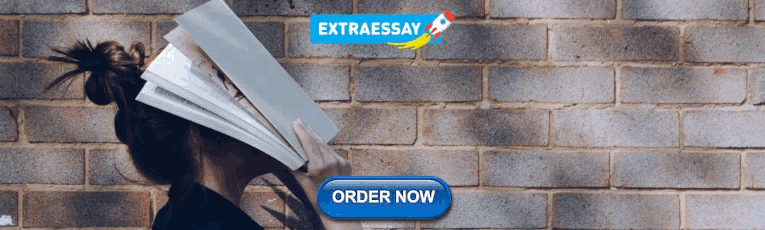
Data Collection Methods | Step-by-Step Guide & Examples
Published on 4 May 2022 by Pritha Bhandari .
Data collection is a systematic process of gathering observations or measurements. Whether you are performing research for business, governmental, or academic purposes, data collection allows you to gain first-hand knowledge and original insights into your research problem .
While methods and aims may differ between fields, the overall process of data collection remains largely the same. Before you begin collecting data, you need to consider:
- The aim of the research
- The type of data that you will collect
- The methods and procedures you will use to collect, store, and process the data
To collect high-quality data that is relevant to your purposes, follow these four steps.
Table of contents
Step 1: define the aim of your research, step 2: choose your data collection method, step 3: plan your data collection procedures, step 4: collect the data, frequently asked questions about data collection.
Before you start the process of data collection, you need to identify exactly what you want to achieve. You can start by writing a problem statement : what is the practical or scientific issue that you want to address, and why does it matter?
Next, formulate one or more research questions that precisely define what you want to find out. Depending on your research questions, you might need to collect quantitative or qualitative data :
- Quantitative data is expressed in numbers and graphs and is analysed through statistical methods .
- Qualitative data is expressed in words and analysed through interpretations and categorisations.
If your aim is to test a hypothesis , measure something precisely, or gain large-scale statistical insights, collect quantitative data. If your aim is to explore ideas, understand experiences, or gain detailed insights into a specific context, collect qualitative data.
If you have several aims, you can use a mixed methods approach that collects both types of data.
- Your first aim is to assess whether there are significant differences in perceptions of managers across different departments and office locations.
- Your second aim is to gather meaningful feedback from employees to explore new ideas for how managers can improve.
Prevent plagiarism, run a free check.
Based on the data you want to collect, decide which method is best suited for your research.
- Experimental research is primarily a quantitative method.
- Interviews , focus groups , and ethnographies are qualitative methods.
- Surveys , observations, archival research, and secondary data collection can be quantitative or qualitative methods.
Carefully consider what method you will use to gather data that helps you directly answer your research questions.
Method | When to use | How to collect data |
---|---|---|
Experiment | To test a causal relationship. | Manipulate variables and measure their effects on others. |
Survey | To understand the general characteristics or opinions of a group of people. | Distribute a list of questions to a sample online, in person, or over the phone. |
Interview/focus group | To gain an in-depth understanding of perceptions or opinions on a topic. | Verbally ask participants open-ended questions in individual interviews or focus group discussions. |
Observation | To understand something in its natural setting. | Measure or survey a sample without trying to affect them. |
Ethnography | To study the culture of a community or organisation first-hand. | Join and participate in a community and record your observations and reflections. |
Archival research | To understand current or historical events, conditions, or practices. | Access manuscripts, documents, or records from libraries, depositories, or the internet. |
Secondary data collection | To analyse data from populations that you can’t access first-hand. | Find existing datasets that have already been collected, from sources such as government agencies or research organisations. |
When you know which method(s) you are using, you need to plan exactly how you will implement them. What procedures will you follow to make accurate observations or measurements of the variables you are interested in?
For instance, if you’re conducting surveys or interviews, decide what form the questions will take; if you’re conducting an experiment, make decisions about your experimental design .
Operationalisation
Sometimes your variables can be measured directly: for example, you can collect data on the average age of employees simply by asking for dates of birth. However, often you’ll be interested in collecting data on more abstract concepts or variables that can’t be directly observed.
Operationalisation means turning abstract conceptual ideas into measurable observations. When planning how you will collect data, you need to translate the conceptual definition of what you want to study into the operational definition of what you will actually measure.
- You ask managers to rate their own leadership skills on 5-point scales assessing the ability to delegate, decisiveness, and dependability.
- You ask their direct employees to provide anonymous feedback on the managers regarding the same topics.
You may need to develop a sampling plan to obtain data systematically. This involves defining a population , the group you want to draw conclusions about, and a sample, the group you will actually collect data from.
Your sampling method will determine how you recruit participants or obtain measurements for your study. To decide on a sampling method you will need to consider factors like the required sample size, accessibility of the sample, and time frame of the data collection.
Standardising procedures
If multiple researchers are involved, write a detailed manual to standardise data collection procedures in your study.
This means laying out specific step-by-step instructions so that everyone in your research team collects data in a consistent way – for example, by conducting experiments under the same conditions and using objective criteria to record and categorise observations.
This helps ensure the reliability of your data, and you can also use it to replicate the study in the future.
Creating a data management plan
Before beginning data collection, you should also decide how you will organise and store your data.
- If you are collecting data from people, you will likely need to anonymise and safeguard the data to prevent leaks of sensitive information (e.g. names or identity numbers).
- If you are collecting data via interviews or pencil-and-paper formats, you will need to perform transcriptions or data entry in systematic ways to minimise distortion.
- You can prevent loss of data by having an organisation system that is routinely backed up.
Finally, you can implement your chosen methods to measure or observe the variables you are interested in.
The closed-ended questions ask participants to rate their manager’s leadership skills on scales from 1 to 5. The data produced is numerical and can be statistically analysed for averages and patterns.
To ensure that high-quality data is recorded in a systematic way, here are some best practices:
- Record all relevant information as and when you obtain data. For example, note down whether or how lab equipment is recalibrated during an experimental study.
- Double-check manual data entry for errors.
- If you collect quantitative data, you can assess the reliability and validity to get an indication of your data quality.
Data collection is the systematic process by which observations or measurements are gathered in research. It is used in many different contexts by academics, governments, businesses, and other organisations.
When conducting research, collecting original data has significant advantages:
- You can tailor data collection to your specific research aims (e.g., understanding the needs of your consumers or user testing your website).
- You can control and standardise the process for high reliability and validity (e.g., choosing appropriate measurements and sampling methods ).
However, there are also some drawbacks: data collection can be time-consuming, labour-intensive, and expensive. In some cases, it’s more efficient to use secondary data that has already been collected by someone else, but the data might be less reliable.
Quantitative research deals with numbers and statistics, while qualitative research deals with words and meanings.
Quantitative methods allow you to test a hypothesis by systematically collecting and analysing data, while qualitative methods allow you to explore ideas and experiences in depth.
Reliability and validity are both about how well a method measures something:
- Reliability refers to the consistency of a measure (whether the results can be reproduced under the same conditions).
- Validity refers to the accuracy of a measure (whether the results really do represent what they are supposed to measure).
If you are doing experimental research , you also have to consider the internal and external validity of your experiment.
In mixed methods research , you use both qualitative and quantitative data collection and analysis methods to answer your research question .
Operationalisation means turning abstract conceptual ideas into measurable observations.
For example, the concept of social anxiety isn’t directly observable, but it can be operationally defined in terms of self-rating scores, behavioural avoidance of crowded places, or physical anxiety symptoms in social situations.
Before collecting data , it’s important to consider how you will operationalise the variables that you want to measure.
Cite this Scribbr article
If you want to cite this source, you can copy and paste the citation or click the ‘Cite this Scribbr article’ button to automatically add the citation to our free Reference Generator.
Bhandari, P. (2022, May 04). Data Collection Methods | Step-by-Step Guide & Examples. Scribbr. Retrieved 29 August 2024, from https://www.scribbr.co.uk/research-methods/data-collection-guide/
Is this article helpful?
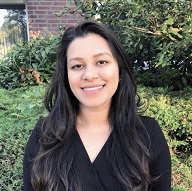
Pritha Bhandari
Other students also liked, qualitative vs quantitative research | examples & methods, triangulation in research | guide, types, examples, what is a conceptual framework | tips & examples.
Data collection in research: Your complete guide
Last updated
31 January 2023
Reviewed by
Cathy Heath
Short on time? Get an AI generated summary of this article instead
In the late 16th century, Francis Bacon coined the phrase "knowledge is power," which implies that knowledge is a powerful force, like physical strength. In the 21st century, knowledge in the form of data is unquestionably powerful.
But data isn't something you just have - you need to collect it. This means utilizing a data collection process and turning the collected data into knowledge that you can leverage into a successful strategy for your business or organization.
Believe it or not, there's more to data collection than just conducting a Google search. In this complete guide, we shine a spotlight on data collection, outlining what it is, types of data collection methods, common challenges in data collection, data collection techniques, and the steps involved in data collection.
Analyze all your data in one place
Uncover hidden nuggets in all types of qualitative data when you analyze it in Dovetail
- What is data collection?
There are two specific data collection techniques: primary and secondary data collection. Primary data collection is the process of gathering data directly from sources. It's often considered the most reliable data collection method, as researchers can collect information directly from respondents.
Secondary data collection is data that has already been collected by someone else and is readily available. This data is usually less expensive and quicker to obtain than primary data.
- What are the different methods of data collection?
There are several data collection methods, which can be either manual or automated. Manual data collection involves collecting data manually, typically with pen and paper, while computerized data collection involves using software to collect data from online sources, such as social media, website data, transaction data, etc.
Here are the five most popular methods of data collection:
Surveys are a very popular method of data collection that organizations can use to gather information from many people. Researchers can conduct multi-mode surveys that reach respondents in different ways, including in person, by mail, over the phone, or online.
As a method of data collection, surveys have several advantages. For instance, they are relatively quick and easy to administer, you can be flexible in what you ask, and they can be tailored to collect data on various topics or from certain demographics.
However, surveys also have several disadvantages. For instance, they can be expensive to administer, and the results may not represent the population as a whole. Additionally, survey data can be challenging to interpret. It may also be subject to bias if the questions are not well-designed or if the sample of people surveyed is not representative of the population of interest.
Interviews are a common method of collecting data in social science research. You can conduct interviews in person, over the phone, or even via email or online chat.
Interviews are a great way to collect qualitative and quantitative data . Qualitative interviews are likely your best option if you need to collect detailed information about your subjects' experiences or opinions. If you need to collect more generalized data about your subjects' demographics or attitudes, then quantitative interviews may be a better option.
Interviews are relatively quick and very flexible, allowing you to ask follow-up questions and explore topics in more depth. The downside is that interviews can be time-consuming and expensive due to the amount of information to be analyzed. They are also prone to bias, as both the interviewer and the respondent may have certain expectations or preconceptions that may influence the data.
Direct observation
Observation is a direct way of collecting data. It can be structured (with a specific protocol to follow) or unstructured (simply observing without a particular plan).
Organizations and businesses use observation as a data collection method to gather information about their target market, customers, or competition. Businesses can learn about consumer behavior, preferences, and trends by observing people using their products or service.
There are two types of observation: participatory and non-participatory. In participatory observation, the researcher is actively involved in the observed activities. This type of observation is used in ethnographic research , where the researcher wants to understand a group's culture and social norms. Non-participatory observation is when researchers observe from a distance and do not interact with the people or environment they are studying.
There are several advantages to using observation as a data collection method. It can provide insights that may not be apparent through other methods, such as surveys or interviews. Researchers can also observe behavior in a natural setting, which can provide a more accurate picture of what people do and how and why they behave in a certain context.
There are some disadvantages to using observation as a method of data collection. It can be time-consuming, intrusive, and expensive to observe people for extended periods. Observations can also be tainted if the researcher is not careful to avoid personal biases or preconceptions.
Automated data collection
Business applications and websites are increasingly collecting data electronically to improve the user experience or for marketing purposes.
There are a few different ways that organizations can collect data automatically. One way is through cookies, which are small pieces of data stored on a user's computer. They track a user's browsing history and activity on a site, measuring levels of engagement with a business’s products or services, for example.
Another way organizations can collect data automatically is through web beacons. Web beacons are small images embedded on a web page to track a user's activity.
Finally, organizations can also collect data through mobile apps, which can track user location, device information, and app usage. This data can be used to improve the user experience and for marketing purposes.
Automated data collection is a valuable tool for businesses, helping improve the user experience or target marketing efforts. Businesses should aim to be transparent about how they collect and use this data.
Sourcing data through information service providers
Organizations need to be able to collect data from a variety of sources, including social media, weblogs, and sensors. The process to do this and then use the data for action needs to be efficient, targeted, and meaningful.
In the era of big data, organizations are increasingly turning to information service providers (ISPs) and other external data sources to help them collect data to make crucial decisions.
Information service providers help organizations collect data by offering personalized services that suit the specific needs of the organizations. These services can include data collection, analysis, management, and reporting. By partnering with an ISP, organizations can gain access to the newest technology and tools to help them to gather and manage data more effectively.
There are also several tools and techniques that organizations can use to collect data from external sources, such as web scraping, which collects data from websites, and data mining, which involves using algorithms to extract data from large data sets.
Organizations can also use APIs (application programming interface) to collect data from external sources. APIs allow organizations to access data stored in another system and share and integrate it into their own systems.
Finally, organizations can also use manual methods to collect data from external sources. This can involve contacting companies or individuals directly to request data, by using the right tools and methods to get the insights they need.
- What are common challenges in data collection?
There are many challenges that researchers face when collecting data. Here are five common examples:
Big data environments
Data collection can be a challenge in big data environments for several reasons. It can be located in different places, such as archives, libraries, or online. The sheer volume of data can also make it difficult to identify the most relevant data sets.
Second, the complexity of data sets can make it challenging to extract the desired information. Third, the distributed nature of big data environments can make it difficult to collect data promptly and efficiently.
Therefore it is important to have a well-designed data collection strategy to consider the specific needs of the organization and what data sets are the most relevant. Alongside this, consideration should be made regarding the tools and resources available to support data collection and protect it from unintended use.
Data bias is a common challenge in data collection. It occurs when data is collected from a sample that is not representative of the population of interest.
There are different types of data bias, but some common ones include selection bias, self-selection bias, and response bias. Selection bias can occur when the collected data does not represent the population being studied. For example, if a study only includes data from people who volunteer to participate, that data may not represent the general population.
Self-selection bias can also occur when people self-select into a study, such as by taking part only if they think they will benefit from it. Response bias happens when people respond in a way that is not honest or accurate, such as by only answering questions that make them look good.
These types of data bias present a challenge because they can lead to inaccurate results and conclusions about behaviors, perceptions, and trends. Data bias can be avoided by identifying potential sources or themes of bias and setting guidelines for eliminating them.
Lack of quality assurance processes
One of the biggest challenges in data collection is the lack of quality assurance processes. This can lead to several problems, including incorrect data, missing data, and inconsistencies between data sets.
Quality assurance is important because there are many data sources, and each source may have different levels of quality or corruption. There are also different ways of collecting data, and data quality may vary depending on the method used.
There are several ways to improve quality assurance in data collection. These include developing clear and consistent goals and guidelines for data collection, implementing quality control measures, using standardized procedures, and employing data validation techniques. By taking these steps, you can ensure that your data is of adequate quality to inform decision-making.
Limited access to data
Another challenge in data collection is limited access to data. This can be due to several reasons, including privacy concerns, the sensitive nature of the data, security concerns, or simply the fact that data is not readily available.
Legal and compliance regulations
Most countries have regulations governing how data can be collected, used, and stored. In some cases, data collected in one country may not be used in another. This means gaining a global perspective can be a challenge.
For example, if a company is required to comply with the EU General Data Protection Regulation (GDPR), it may not be able to collect data from individuals in the EU without their explicit consent. This can make it difficult to collect data from a target audience.
Legal and compliance regulations can be complex, and it's important to ensure that all data collected is done so in a way that complies with the relevant regulations.
- What are the key steps in the data collection process?
There are five steps involved in the data collection process. They are:
1. Decide what data you want to gather
Have a clear understanding of the questions you are asking, and then consider where the answers might lie and how you might obtain them. This saves time and resources by avoiding the collection of irrelevant data, and helps maintain the quality of your datasets.
2. Establish a deadline for data collection
Establishing a deadline for data collection helps you avoid collecting too much data, which can be costly and time-consuming to analyze. It also allows you to plan for data analysis and prompt interpretation. Finally, it helps you meet your research goals and objectives and allows you to move forward.
3. Select a data collection approach
The data collection approach you choose will depend on different factors, including the type of data you need, available resources, and the project timeline. For instance, if you need qualitative data, you might choose a focus group or interview methodology. If you need quantitative data , then a survey or observational study may be the most appropriate form of collection.
4. Gather information
When collecting data for your business, identify your business goals first. Once you know what you want to achieve, you can start collecting data to reach those goals. The most important thing is to ensure that the data you collect is reliable and valid. Otherwise, any decisions you make using the data could result in a negative outcome for your business.
5. Examine the information and apply your findings
As a researcher, it's important to examine the data you're collecting and analyzing before you apply your findings. This is because data can be misleading, leading to inaccurate conclusions. Ask yourself whether it is what you are expecting? Is it similar to other datasets you have looked at?
There are many scientific ways to examine data, but some common methods include:
looking at the distribution of data points
examining the relationships between variables
looking for outliers
By taking the time to examine your data and noticing any patterns, strange or otherwise, you can avoid making mistakes that could invalidate your research.
- How qualitative analysis software streamlines the data collection process
Knowledge derived from data does indeed carry power. However, if you don't convert the knowledge into action, it will remain a resource of unexploited energy and wasted potential.
Luckily, data collection tools enable organizations to streamline their data collection and analysis processes and leverage the derived knowledge to grow their businesses. For instance, qualitative analysis software can be highly advantageous in data collection by streamlining the process, making it more efficient and less time-consuming.
Secondly, qualitative analysis software provides a structure for data collection and analysis, ensuring that data is of high quality. It can also help to uncover patterns and relationships that would otherwise be difficult to discern. Moreover, you can use it to replace more expensive data collection methods, such as focus groups or surveys.
Overall, qualitative analysis software can be valuable for any researcher looking to collect and analyze data. By increasing efficiency, improving data quality, and providing greater insights, qualitative software can help to make the research process much more efficient and effective.
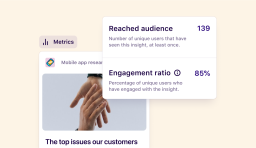
Learn more about qualitative research data analysis software
Should you be using a customer insights hub.
Do you want to discover previous research faster?
Do you share your research findings with others?
Do you analyze research data?
Start for free today, add your research, and get to key insights faster
Editor’s picks
Last updated: 18 April 2023
Last updated: 27 February 2023
Last updated: 22 August 2024
Last updated: 5 February 2023
Last updated: 16 August 2024
Last updated: 9 March 2023
Last updated: 30 April 2024
Last updated: 12 December 2023
Last updated: 11 March 2024
Last updated: 4 July 2024
Last updated: 6 March 2024
Last updated: 5 March 2024
Last updated: 13 May 2024
Latest articles
Related topics, .css-je19u9{-webkit-align-items:flex-end;-webkit-box-align:flex-end;-ms-flex-align:flex-end;align-items:flex-end;display:-webkit-box;display:-webkit-flex;display:-ms-flexbox;display:flex;-webkit-flex-direction:row;-ms-flex-direction:row;flex-direction:row;-webkit-box-flex-wrap:wrap;-webkit-flex-wrap:wrap;-ms-flex-wrap:wrap;flex-wrap:wrap;-webkit-box-pack:center;-ms-flex-pack:center;-webkit-justify-content:center;justify-content:center;row-gap:0;text-align:center;max-width:671px;}@media (max-width: 1079px){.css-je19u9{max-width:400px;}.css-je19u9>span{white-space:pre;}}@media (max-width: 799px){.css-je19u9{max-width:400px;}.css-je19u9>span{white-space:pre;}} decide what to .css-1kiodld{max-height:56px;display:-webkit-box;display:-webkit-flex;display:-ms-flexbox;display:flex;-webkit-align-items:center;-webkit-box-align:center;-ms-flex-align:center;align-items:center;}@media (max-width: 1079px){.css-1kiodld{display:none;}} build next, decide what to build next, log in or sign up.
Get started for free
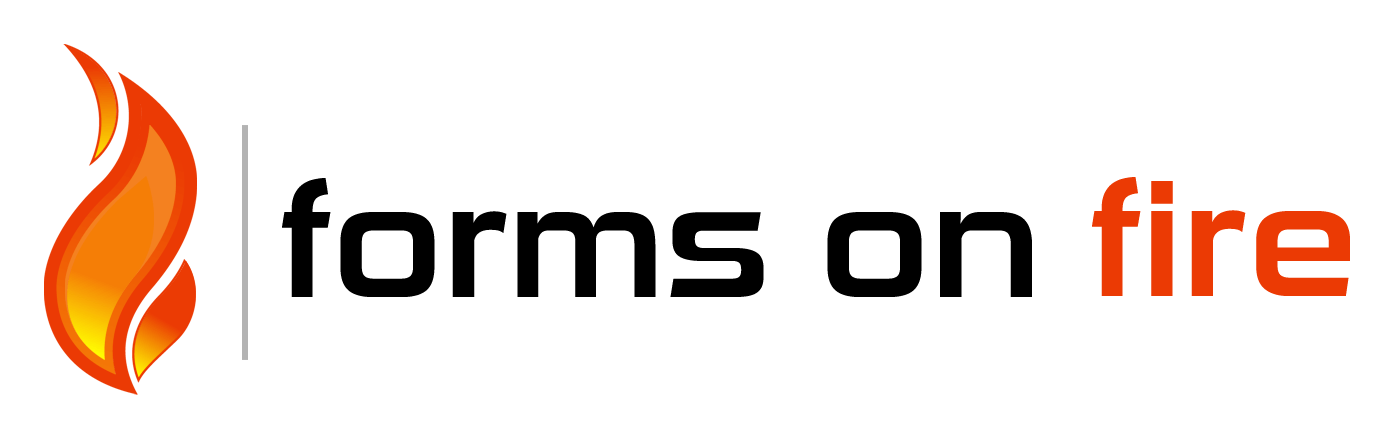
How to Write a Data Collection Plan (Templates and Examples Included)
In a world where data drives decisions, how do you make sure you're gathering the right information? With a clear data collection plan in place, you ensure that the collected data leads to actionable insights.
Effective data collection is key to smart decision-making, grounding strategies in solid evidence rather than guesses. A well-designed data collection plan guarantees that you're collecting not just any data, but the right data, crucial for spotting trends, refining processes, and deeply understanding customer needs in any sector.
By the end of this article, you'll understand the importance of planning your data collection and how to do it effectively.
What is a data collection plan?
A data collection plan is a roadmap for identifying what data you need, the ways in which you'll collect it, and how you'll analyze it. The core purpose is to ensure that your data collection is targeted, efficient, and reliable, providing meaningful insights for your project or study.
Data collection plans should be developed at the start of a project or study, before any data is collected. Typically, this responsibility falls to project leaders, researchers, data analysts, or a designated team member with expertise in data management.
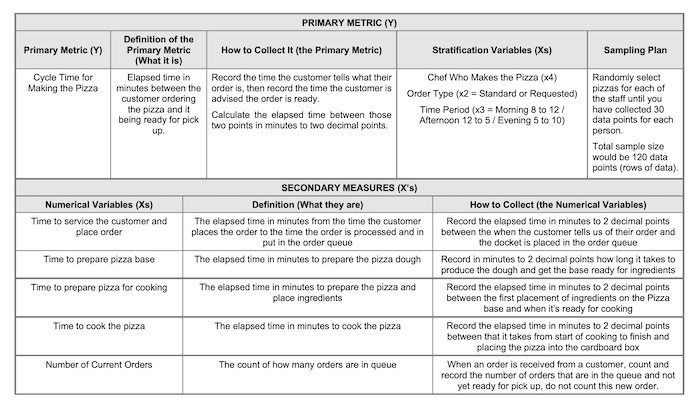
What does a typical data collection plan document cover
From setting clear objectives to establishing robust communication channels, each section of the plan is a stepping stone towards having a thorough data collection strategy:
- Objectives: Start with a specific goal for your data collection. Clearly state why this data is crucial and how it will impact your project or decision-making. This step ensures that every part of your plan aligns with your end goal.
- Data typology: Decide whether you need quantitative (numerical) or qualitative (descriptive) data. Clarify the importance of each data type in the context of your objectives. This clarity helps in selecting the right tools and methods for data collection.
- Collection methodology: Select appropriate methods like surveys, interviews, or analysis of existing data. Prioritize data quality; for surveys, this means clear, unbiased questions; for interviews, standardized interviewing techniques; etc.
- Data management protocols: Plan for the storage, organization, and protection of your data. Address ethical considerations, especially for sensitive information. Include a system for updating and correcting data to maintain its accuracy over time.
- Project timeline : Outline a realistic timeline with start and end dates, including key milestones. Incorporate flexibility for unforeseen delays or challenges.
- Needed resources: Identify the team, tools, and budget required. Clearly define roles and responsibilities to ensure a smooth data collection process.
- Data analysis strategy: Determine how you'll analyze the collected data. Include methods for dealing with unexpected findings, like ambiguous, conflicting, corrupted, or incomplete data.
- Feedback mechanisms: Establish a mechanism for ongoing assessment and adjustment of your data collection methods. This allows you to adapt and refine your approach as needed.
- Communication framework: Decide how and when you'll communicate your findings. Depending on the project, you might need to keep stakeholders updated throughout the process, not just at the end, to maintain engagement and transparency.
Try to meticulously address each of these elements to set the stage for successful data gathering.
Ways to collect data
Collecting data is akin to gathering and sorting the pieces for a puzzle. Each piece, or data point, is critical to form a complete and accurate picture of the subject under study.
To ensure that this picture is as clear and precise as possible, researchers and analysts employ a variety of data collection methods outlined in the image below.
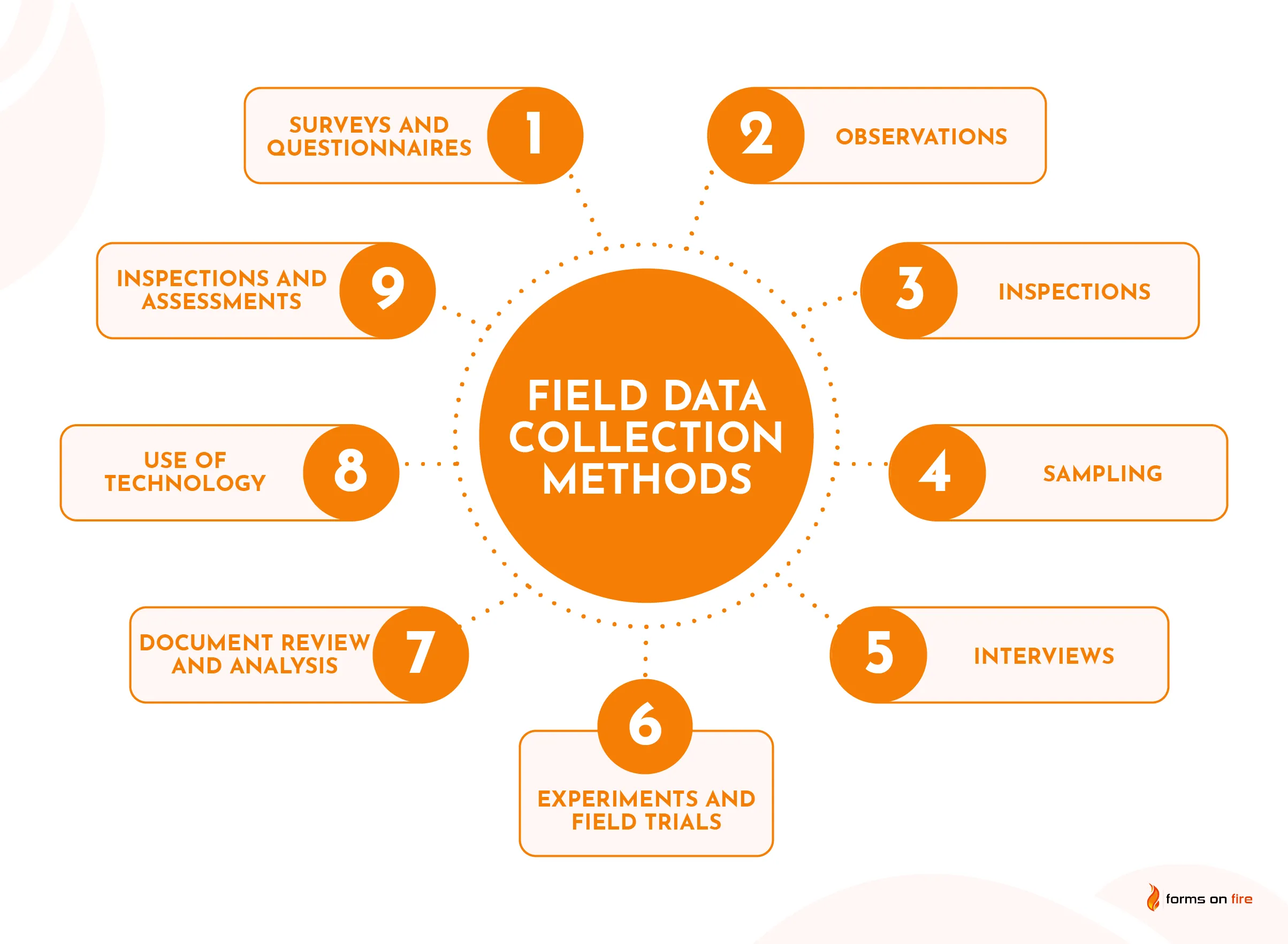
- Surveys and questionnaires: These involve asking structured questions to a large group of people. Consider the timing of your survey distribution — sending out surveys at a time when your target audience is likely to be available and attentive can significantly improve the response quality.
- Interviews: One-on-one conversations that allow for deep dives into subjects' thoughts and experiences. Record interviews (with permission) and note non-verbal cues. These can provide context often lost in written notes, like the respondent's tone or hesitation.
- Focus groups: Small groups of people discuss specific topics, providing qualitative data on opinions and behaviors. Use a skilled moderator who can encourage quieter members to speak up and keep dominant personalities from overtaking the conversation.
- Observations: Watching and recording behavior or events as they naturally occur. If possible, conduct observations at different times or in varied settings. This helps in understanding if the observed behavior is consistent or situation-dependent.
- Inspections and assessments: Examining objects, processes, or places in detail, often using a structured approach supported by pre-made checklists.
- Document review and analysis: Systematically reviewing and interpreting existing documents to extract data. Cross-reference information from different documents for a more comprehensive understanding. This triangulation can validate findings and reveal deeper insights.
Each of these methods offers a unique way to gather data and comes with its own set of pros and cons. Take your time to decide which data collection methods are the best fit for your use case.
Steps for writing an effective data collection plan
With the theory out of the way, let’s see how to write a proper data collection plan, step by step.
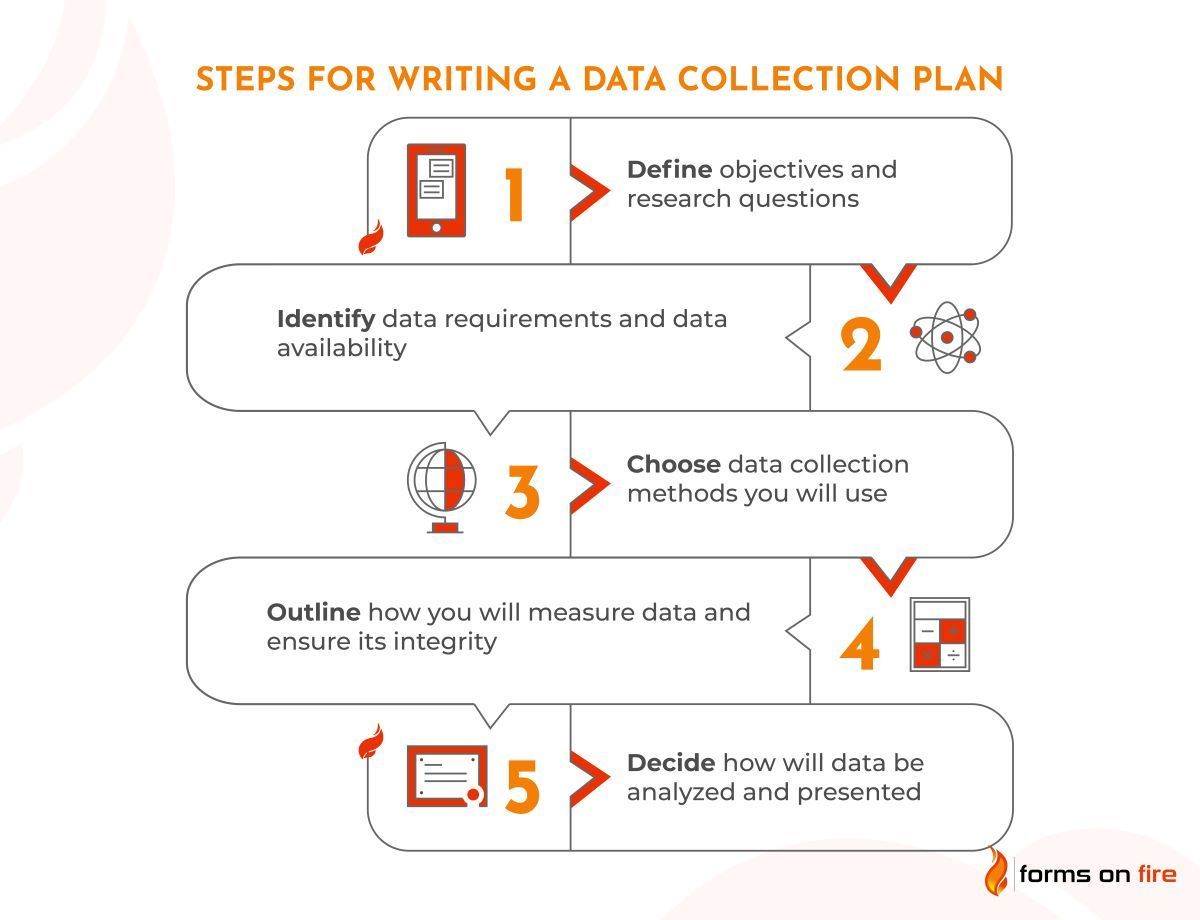
1. Define objectives and research questions
Write down a statement of purpose that explains what you intend to discover, decide, or achieve. This statement will act as the compass for your data collection journey.
Your research questions must be clear, focused, and aligned with your stated objectives. For every objective, draft at least one research question that, when answered, will bring you closer to your goal.
When finalizing your list of research questions, don't overlook the "so what?" factor. For each one, ask yourself what the implications are if the question is answered or the objective is met. How will it change your understanding, decision-making, or actions? This ensures that your plan has practical value and isn't just an academic exercise.
2. Identify data requirements and availability
Identifying your data requirements is a two-part process: you need to understand the type of data you need and assess the data that is already available to you.
Here's how to understand the type of data you need:
- Consider the nature of your research questions: What data will provide the answers? Is it demographic information, behavioral metrics, financial statistics, etc.?
- Determine the data quantity: How much data is enough to make your results reliable? This can depend on the statistical methods you plan to use and the scale of your project.
- Think about the data quality: What level of accuracy is required? Does the data need to be current, historical, or predictive?
Create a data inventory list. For each research question, list the types of data that could potentially answer it. Next to each type, note down the attributes of the data you need (timeframe, demographic details, granularity, etc.).
To assess the data that is already available to you, follow these:
- Look internally first: Does your organization already have some of the data you need? This could be sales records, customer feedback, or past survey results.
- Consider external sources: Is there public data available that fits your needs, such as government databases, research papers, or industry reports?
- Evaluate accessibility: Can you easily access this data, or are there barriers (e.g., paywalls, privacy laws, data sharing agreements) that you need to consider?
For each piece of required data, try to record its source, format, any costs associated with obtaining it, and any potential challenges in accessing it. If data is not available, note down what proxies could be used or whether secondary data collection is necessary.
Completing this step will form the backbone of your data collection strategy, guiding you on where to focus your resources.
3. Choose how you will collect data
Based on your data requirements, select the most suitable collection methods. Will you use surveys, interviews, observations, experiments, or a combination of multiple methods?
Match data collection methods to the type of data you need. For quantitative data, you might use surveys or sensor data. For qualitative data, consider interviews or focus groups. Think about the context of your research — does it call for controlled experiments, or would field studies yield better results?
Once you've selected a method, it's time to think about who will shoulder the task. The 'who' could range from your own team members to external professionals, depending on the expertise required.
Incorporate quality control measures right from the start. This should include when and where data will be collected, the tools or technologies used, and the step-by-step process for gathering the data.
Finally, address ethical considerations, especially if you’re dealing with human subjects or sensitive data. Obtain necessary permissions and ensure you’re compliant with relevant laws and regulations.
4. Outline how you will measure data and ensure its integrity
Clearly specify what you are measuring and how it will be quantified. Are you looking at frequencies, averages, percentages, or growth rates? Ensure that the chosen metrics align directly with your research questions and objectives.
Develop and document standardized procedures for data measurement: define operational terms, detail measurement techniques, and specify the equipment or software used.
For each variable, write down a clear operational definition, which is a detailed description of the procedures used to measure it . For example, if you're measuring customer satisfaction, define what constitutes satisfaction and the scale you're using (e.g., 1-5 likert scale ).
To ensure data integrity, team members tasked with collecting and analyzing data really need to know what they’re doing. If you’re using instruments or software, ensure they are calibrated and tested before data collection begins. Consider running a pilot study or trial to test your measurement processes and make adjustments where necessary. This helps you catch potential issues before you roll out large-scale data collection.
Create a data log that records when and by whom data was collected, entered, and verified. Make sure to regularly check a sample of data entries against the original data to ensure accuracy. If you’re using mobile forms or other digital tools to collect data, most of this can be automated.
Lastly, decide in advance how you will deal with missing data or outliers. Will you use imputation methods , or will you exclude it? Make sure your approach is consistent and documented.
5. Decide how will data be analyzed and presented
Outlines each step of your analysis process: the methods you'll use, the required tools, and the sequence of analysis.
Choose analysis methods that align with your data types and objectives. For analyzing quantitative data , statistical methods like regression analysis, ANOVA, or cluster analysis might be appropriate. For analyzing qualitative data , try content analysis, thematic analysis, or discourse analysis.
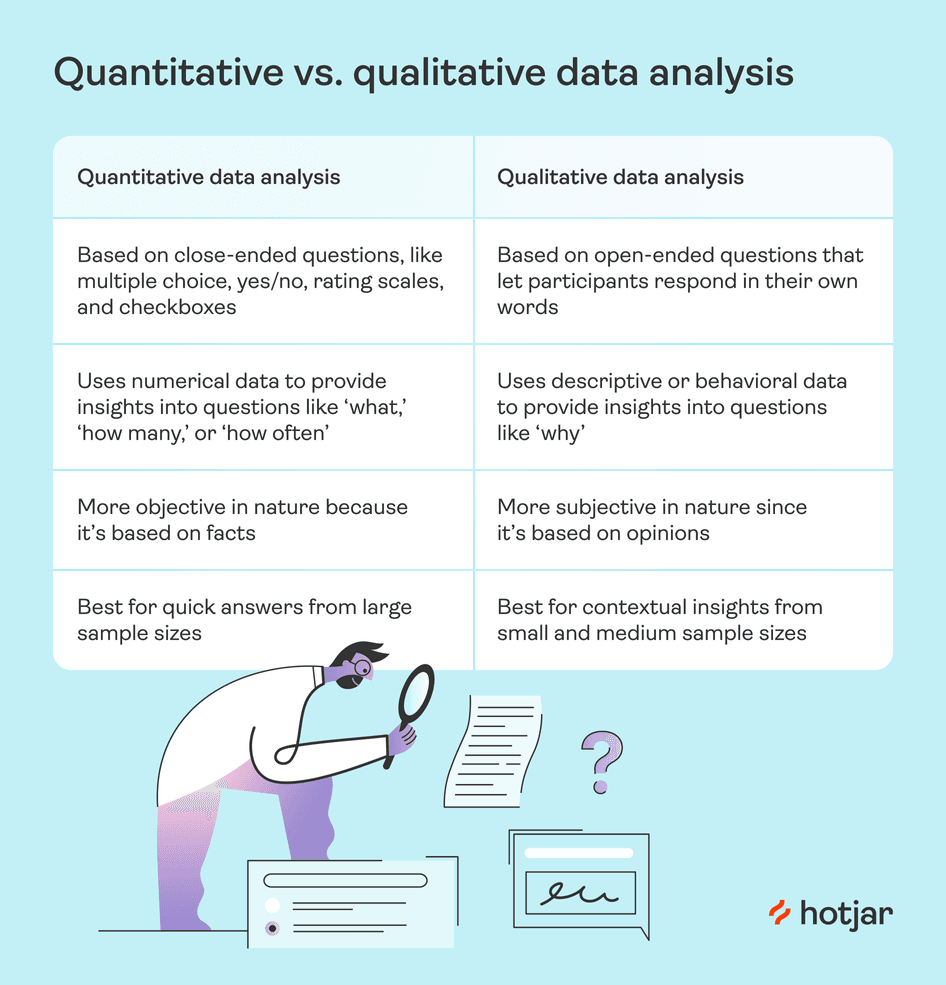
If you have a complex project and plan to use specific software to analyze data, decide which one that is going to be. Options could range from statistical software like SPSS or R for quantitative analysis to software like NVivo for qualitative data analysis.
Think about how you will present your data. This could be in the form of reports, infographics, dashboards, or presentations. Choose the format with your audience in mind — what format will be most clear and persuasive to them?
Try sketching out a draft of your final report or presentation early in the planning process. This helps you visualize the end product and ensure that your data collection and analysis will support this outcome.
Data collection plan examples and templates
Below are four different examples and templates you can use to build your own data collection plans.
Streamline data collection process with Forms On Fire
Forms on Fire offers a flexible, accessible, and efficient platform for automating and simplifying data collection and management:
- Customizable forms and mobile accessibility: Design forms specific to your needs and capture data on the go, even offline, with mobile device support.
- Automated workflows and integration capabilities: Automate processes like notifications and data analysis upon form submission, while seamlessly integrating with over 1,500 systems.
- Real-time data access and reporting: Access and analyze data instantly for timely decision-making, trend spotting, and addressing issues quickly.
- Cloud-based data storage: Securely store all data in the cloud, ensuring safety and availability from any location at any time.
- User-friendly interface: Enjoy an intuitive platform that requires no coding skills, making it easy for anyone in your organization to create and use forms.
Ready to transform your data collection process? Start a free trial below or schedule a product demo and see how our solution can answer your data management needs!
< Older Post
Newer Post >
+1 (425) 214-1920
10900 NE 4th Street, Suite 2300
Bellevue, WA 98004
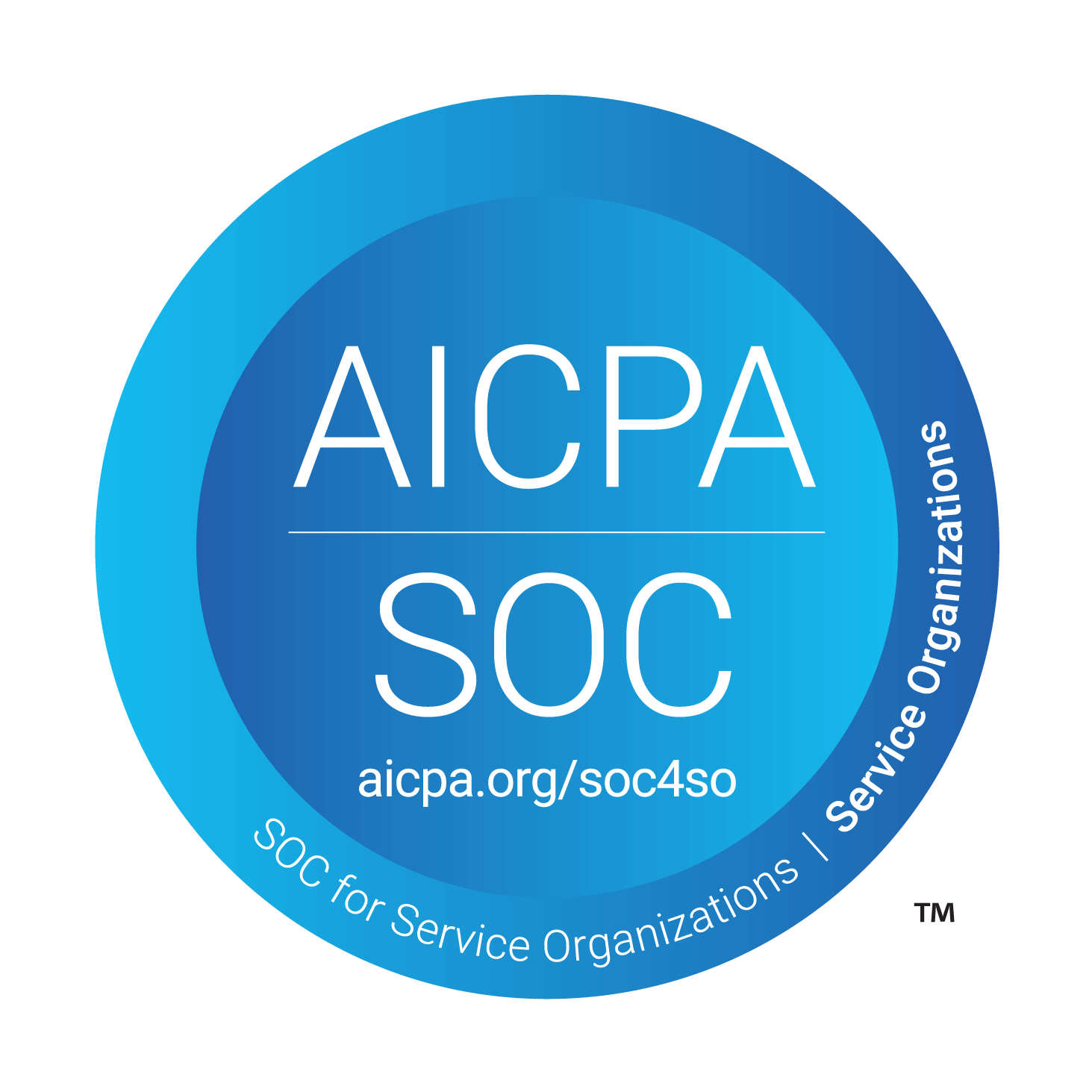
© 2024 Forms On Fire, All Rights Reserved
Policy Center | Terms of Service | Privacy

- Research Data Lifecycle Guide
Data Collection
Data collection is the process of gathering and measuring information used for research. Collecting data is one of the most important steps in the research process, and is part of all disciplines including physical and social sciences, humanities, business, etc. Data comes in many forms with different ways to store and record data, either written in a lab notebook and or recorded digitally on a computer system.
While methods may differ across disciplines, good data management processes begin with accurately and clearly describing the information recorded, the process used to collect the data, practices that ensure the quality of the data, and sharing data to enable reproducibility. This section breaks down different topics that need to be addressed while collecting and managing data for research.
Learn more about what’s required for data collection as a researcher at Case Western Reserve University.
Ensuring Accurate and Appropriate Data Collection
Accurate data collection is vital to ensure the integrity of research . It is important when planning and executing a research project to consider methods collection and the storage of data to ensure that results can be used for publications and reporting. The consequences from improper data collection include:
- inability to answer research questions accurately
- inability to repeat and validate the study
- distorted findings resulting in wasted resources
- misleading other researchers to pursue fruitless avenues of investigation
- compromising decisions for public policy
- causing harm to human participants and animal subjects
While the degree of impact from inaccurate data may vary by discipline, there is a potential to cause disproportionate harm when data is misrepresented and misused. This includes fraud or scientific misconduct.
Any data collected in the course of your research should follow RDM best practices to ensure accurate and appropriate data collection. This includes as appropriate, developing data collection protocols and processes to ensure inconsistencies and other errors are caught and corrected in a timely manner.
Examples of Research Data
Research data is any information that has been collected, observed, generated or created in association with research processes and findings.
Much research data is digital in format, but research data can also be extended to include non-digital formats such as laboratory notebook, diaries, or written responses to surveys. Examples may include (but are not limited to):
- Excel spreadsheets that contains instrument data
- Documents (text, Word), containing study results
- Laboratory notebooks, field notebooks, diaries
- Questionnaires, transcripts, codebooks
- Audiotapes, videotapes
- Photographs, films
- Protein or genetic sequences
- Test responses
- Slides, artifacts, specimens, samples
- Collection of digital objects acquired and generated during the process of research
- Database contents (video, audio, text, images)
- Models, algorithms, scripts
- Contents of an application (input, output, logfiles for analysis software, simulation software, schemas)
- Source code used in application development
To ensure reproducibility of experiments and results, be sure to include and document information such as:
- Methodologies and workflows
- Standard operating procedures and protocols
Data Use Agreements
When working with data it is important to understand any restrictions that need to be addressed due to the sensitivity of the data. This includes how you download and share with other collaborators, and how it needs to be properly secured.
Datasets can include potentially sensitive data that needs to be protected, and not openly shared. In this case, the dataset cannot be shared and or downloaded without permission from CWRU Research Administration and may require an agreement between collaborators and their institutions. All parties will need to abide by the agreement terms including the destruction of data once the collaboration is complete.
Storage Options
UTech provides cloud and on-premise storage to support the university research mission. This includes Google Drive , Box , Microsoft 365 , and various on-premise solutions for high speed access and mass storage. A listing of supported options can be found on UTech’s website .
In addition to UTech-supported storage solutions, CWRU also maintains an institutional subscription to OSF (Open Science Framework) . OSF is a cloud-based data storage, sharing, and project collaboration platform that connects to many other cloud services like Drive, Box, and Github to amplify your research and data visibility and discoverability. OSF storage is functionally unlimited.
When selecting a storage platform it is important to understand how you plan to analyze and store your data. Cloud storage provides the ability to store and share data effortlessly and provides capabilities such as revisioning and other means to protect your data. On-premise storage is useful when you have large storage demands and require a high speed connection to instruments that generate data and systems that process data. Both types of storage have their advantages and disadvantages that you should consider when planning your research project.
Data Security
Data security is a set of processes and ongoing practices designed to protect information and the systems used to store and process data. This includes computer systems, files, databases, applications, user accounts, networks, and services on institutional premises, in the cloud, and remotely at the location of individual researchers.
Effective data security takes into account the confidentiality, integrity, and availability of the information and its use. This is especially important when data contains personally identifiable information, intellectual property, trade secrets, and or technical data supporting technology transfer agreements (before public disclosure decisions have been made).
Data Categorization
CWRU uses a 3-tier system to categorize research data based on information types and sensitivity . Determination is based upon risk to the University in the areas of confidentiality, integrity, and availability of data in support of the University's research mission. In this context, confidentiality measures to what extent information can be disclosed to others, integrity is the assurance that the information is trustworthy and accurate, and availability is a guarantee of reliable access to the information by authorized users.
Information (or data) owners are responsible for determining the impact levels of their information, i.e. what happens if the data is improperly accessed or lost accidentally, implementing the necessary security controls, and managing the risk of negative events including data loss and unauthorized access.
Classification | Examples |
---|---|
| |
| |
|
Loss, corruption, or inappropriate access to information can interfere with CWRU's mission, interrupt business and damage reputations or finances.
Securing Data
The classification of data requires certain safeguards or countermeasures, known as controls, to be applied to systems that store data. This can include restricting access to the data, detecting unauthorized access, preventative measures to avoid loss of data, encrypting the transfer and storage of data, keeping the system and data in a secure location, and receiving training on best practices for handling data. Controls are classified according to their characteristics, for example:
- Physical controls e.g. doors, locks, climate control, and fire extinguishers;
- Procedural or administrative controls e.g. policies, incident response processes, management oversight, security awareness and training;
- Technical or logical controls e.g. user authentication (login) and logical access controls, antivirus software, firewalls;
- Legal and regulatory or compliance controls e.g. privacy laws, policies and clauses.
Principal Investigator (PI) Responsibilities
The CWRU Faculty Handbook provides guidelines for PIs regarding the custody of research data. This includes, where applicable, appropriate measures to protect confidential information. It is everyone’s responsibility to ensure that our research data is kept securely and available for reproducibility and future research opportunities.
University Technology provides many services and resources related to data security including assistance with planning and securing data. This includes processing and storing restricted information used in research.
Data Collected as Part of Human Subject Research
To ensure the privacy and safety of the individual participating in a human subject research study, additional rules and processes are in place that describe how one can use and disclose data collected, The Office of Research Administration provides information relevant to conducting this type of research. This includes:
- Guidance on data use agreements and processes for agreements that involve human-related data or human-derived samples coming in or going out of CWRU.
- Compliance with human subject research rules and regulations.
According to 45 CFR 46 , a human subject is "a living individual about whom an investigator (whether professional or student) conducting research:
- Obtains information or biospecimens through intervention or interaction with the individual, and uses, studies, or analyzes the information or biospecimens; or
- Obtains, uses, studies, analyzes, or generates identifiable private information or identifiable biospecimens."
The CWRU Institutional Review Board reviews social science/behavioral studies, and low-risk biomedical research not conducted in a hospital setting for all faculty, staff, and students of the University. This includes data collected and used for human subjects research.
Research conducted in a hospital setting including University Hospitals requires IRB protocol approval.
Questions regarding the management of human subject research data should be addressed to the CWRU Institutional Review Board .
Getting Help With Data Collection
If you are looking for datasets and other resources for your research you can contact your subject area librarian for assistance.
- Kelvin Smith Library
If you need assistance with administrative items such as data use agreements or finding the appropriate storage solution please contact the following offices.
- Research Administration
- UTech Research Computing
- Information Security Office
Guidance and Resources
- Information Security Policy
- Research Data Protection
- CWRU Faculty Handbook
- CWRU IRB Guidance
Tips for writing your data collection procedures
Your data collection plan is a crucial key to developing a sound study. The plan indicates how you will access and gather information from your participants. A clear data collection plan at the proposal stage can alleviate stress and ensure that future researchers can replicate your study. Additionally, a clear data collection plan will help ensure that you obtain the information you need to answer your research questions. Below are some suggestions for creating a solid data collection plan.
First, it may be helpful to outline your steps. This allows you to see where your data collection procedures must begin and end. This should include all of the steps that you will take from the time that you obtain Institutional Review Board (IRB) approval to the time that your data is collected and ready for analysis. A simple bulleted list of the steps you plan to conduct will suffice for this step.
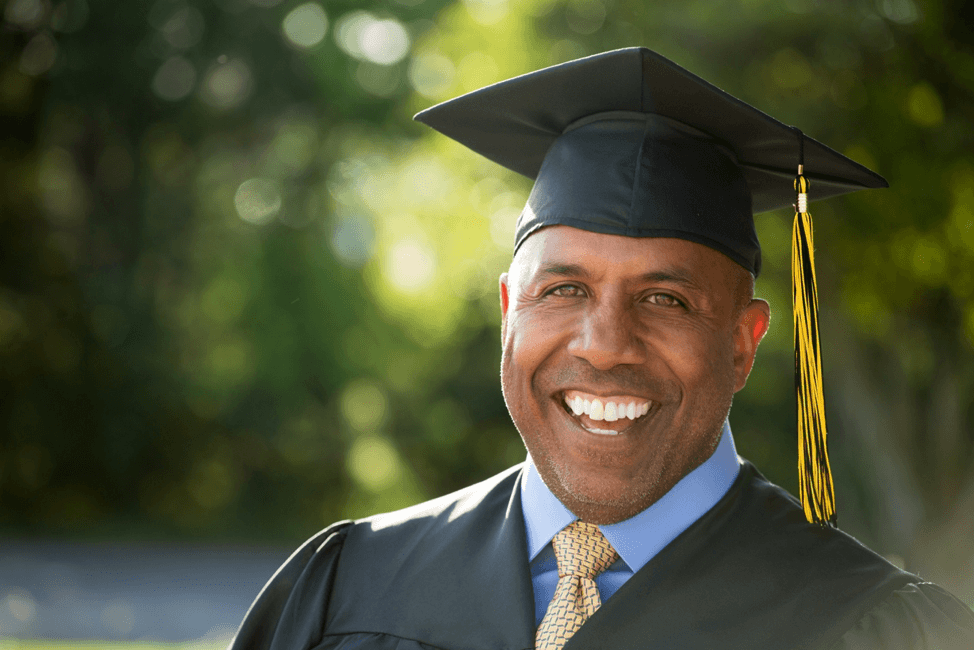
Discover How We Assist to Edit Your Dissertation Chapters
Aligning theoretical framework, gathering articles, synthesizing gaps, articulating a clear methodology and data plan, and writing about the theoretical and practical implications of your research are part of our comprehensive dissertation editing services.
- Bring dissertation editing expertise to chapters 1-5 in timely manner.
- Track all changes, then work with you to bring about scholarly writing.
- Ongoing support to address committee feedback, reducing revisions.
From there, cross reference this list with your research questions and the variables in each research question. Make sure you have an instrument to measure each variable and you have included each of these instruments in your outline. Once you have developed an outline that includes all of the necessary instruments, you can move on to writing a full detailed draft of your data collection procedures. However, before you do that, you may want to take some time to have an accountability partner review your work. This should be a person who can be a sounding board and who can provide basic feedback on your work. Describe the purpose of your study, the research questions, and the data you will need to access to address your research questions. Let them review your outline and double check to ensure that all necessary data collection steps are presented.
Now you are ready to turn your outline into the data collection draft. Observe the appropriate tone and wording as you turn your outline into a doctoral level narrative. Imagine this as a recipe that your dissertation committee, IRB, and future researchers can use to understand and replicate your study. The draft should be succinct, clear, and comprehensive.
Once you have completed the narrative, you can compare it to the outline to make sure everything is addressed. You should also review your school’s template or guidelines for the data collection section to ensure that all the required points have been addressed.
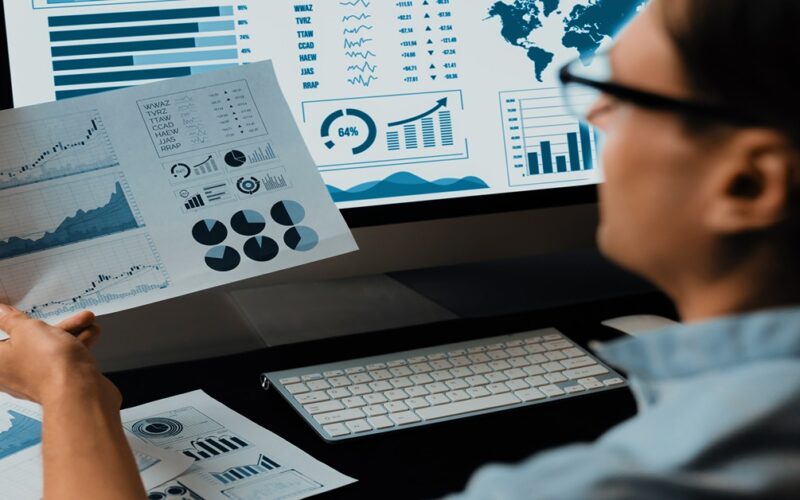
Data Collection: A Comprehensive Guide
- 7 minute read
- August 27, 2024

Written by:

Aashi Verma has dedicated herself to covering the forefront of enterprise and cloud technologies. As an Passionate researcher, learner, and writer, Aashi Verma interests extend beyond technology to include a deep appreciation for the outdoors, music, literature, and a commitment to environmental and social sustainability.
Summary: This blog provides a comprehensive overview of data collection, covering its definition, importance, methods, and types of data. It also discusses tools and techniques for effective data collection, emphasising quality assurance and control. Understand how to gather accurate and reliable data for informed decision-making and research integrity.
Introduction
Data collection is a fundamental component of any research or business endeavour, enabling organisations to gather and analyse information that informs decision-making, drives strategic planning, and evaluates outcomes.
Data collection involves using systematic methods to gather observations or measurements. We use these observations to answer questions, analyze performance, and predict future trends. This blog aims to provide a comprehensive overview of data collection, including its definition, importance, methods, types of data, tools, and techniques for effective data collection.
Read More: Why Data Driven Mindset is Your Key To Success
Definition of Data Collection
Data collection is the process of gathering and measuring information on targeted variables in an established system. This process allows researchers and organisations to capture evidence that enables them to formulate credible answers to the questions they have posed.
Data collection can be as routine as logging patient information into an electronic medical record system or as specific as keeping a record of mosquito nets delivered to rural households. It encompasses a wide range of activities, from automated data collection functions built into business applications to manual procedures such as surveys and observations.
Importance of Data Collection
Data collection is essential for maintaining research integrity and ensuring that subsequent decisions are based on valid data. Here are some key reasons why data collection is important:
Informed Decision-Making
Accurate data collection provides the necessary information to answer relevant questions and evaluate outcomes. This allows organisations to base their decisions on solid evidence.
Research Integrity
Ensuring accurate and honest data collection is crucial for maintaining research integrity. It helps in preventing errors and ensuring that the findings are reliable and valid.
Strategic Planning
Data collection is vital for strategic planning in businesses. It helps in analysing business performance, understanding customer preferences, and predicting future trends.
Evaluation of Outcomes
Data collection allows organisations to evaluate the effectiveness of their interventions or strategies. This feedback loop is essential for continuous improvement and optimization.
Methods of Data Collection
Data collection methods vary widely depending on the field of study, the nature of the data needed, and the resources available. Here are some common methods:
Surveys and Questionnaires
Researchers use structured tools like surveys to collect numerical or categorical data from many participants. They can conduct surveys online, in person, or via phone and email.
Interviews involve one-on-one conversations with participants to gather detailed information about their experiences and perspectives. You can structure surveys as structured, semi-structured, or unstructured.
Observations
Observational methods involve collecting data by observing the behaviour of participants without direct interaction. This can be done in natural or controlled settings.
Focus Groups
Focus groups are group discussions led by a moderator to explore collective opinions and experiences. They are useful for understanding social dynamics and group interactions.
Experiments
Experimental methods involve controlled studies designed to test hypotheses and measure the effects of variables. These are often used in scientific research to establish cause-and-effect relationships.
Secondary Data Analysis
This involves analysing existing data from sources such as databases, archives, or previous studies. Secondary data can be quicker and less expensive to obtain but may lack the specificity and control of primary data collection.
Types of Data
Understanding the different types of data is crucial for effective data collection. This section delves into quantitative and qualitative data , exploring their characteristics, examples, and applications to help you choose the right type for your research or business needs.
Quantitative Data
Quantitative data is numerical and measurable. It is collected through structured methods such as surveys, questionnaires, and experiments. This type of data is analysed statistically to identify patterns, test hypotheses, and make predictions.
Examples of Quantitative Data
- Numerical Survey Responses: Answers to multiple-choice questions or rating scales.
- Test Scores: Results from standardised tests or assessments.
- Website Traffic Data: Metrics such as page views, click-through rates, and time spent on a website.
- Sales Data: Numerical data on sales figures.
Qualitative Data
Qualitative data is non-numerical and provides a deeper understanding of human thoughts, feelings, and behaviours. It is collected through open-ended methods such as interviews, focus groups, and observations. This type of data is analysed through interpretation and thematic analysis to understand themes and insights.
Examples of Qualitative Data
- Interview Transcripts : Detailed records of conversations with participants.
- Observation Notes : Records of behaviours and interactions observed in a natural or controlled setting.
- Open-Ended Survey Responses: Answers to questions that allow participants to provide detailed, unstructured responses.
- Diaries and Journals: Personal accounts of experiences and thoughts over time.
Tools for Data Collection
The choice of data collection tools depends on the specific needs of your project, including the type of data you are collecting, the scale of your project, and your budget. Here are some widely used tools across different categories:
Survey Tools
These tools are designed to collect quantitative data from a large audience through structured questionnaires. They are ideal for gathering customer feedback, employee opinions, or market research insights.
- QuestionPro: Offers advanced survey features and analytics, including customizable templates and real-time analytics.
- SurveyMonkey: Known for its user-friendly interface and customizable survey options.
- Google Forms : Free and easy to use, suitable for simple surveys.
Interview and Focus Group Tools
It is one of the common methods of data collection. These tools facilitate the collection of qualitative data through guided conversations and group discussions.
- Zoom : Great for virtual interviews and focus group discussions, offering features for recording and collaboration.
- Microsoft Teams: Offers features for collaboration and recording sessions, making it suitable for virtual interviews and focus groups.
Observation and Field Data Collection Tools
If you are looking for a more relevant data collection method that gives you real output, field data collection takes the lead. These tools are used for collecting data in field settings or through observational methods.
- Open Data Kit (ODK) : Ideal for mobile data collection in field settings, allowing offline data collection and synchronisation when an internet connection is available.
- REDCap: A secure web application for building and managing online surveys, particularly useful for research environments.
Mobile Data Collection Tools
These tools leverage smartphones and tablets to gather data on the go, often used in remote areas or fieldwork.
- KoboToolbox: Designed for humanitarian work, useful for field data collection with features like GPS tagging and photo capture.
- SurveyCTO: Provides offline data collection capabilities for mobile devices, ensuring data integrity in areas with limited internet connectivity.
Data Analysis, Visualisation, Reporting, & Workflow Tools
These tools assist in processing and interpreting the collected data, providing features for data visualisation, reporting, and workflow management.
- Tableau : A powerful data visualisation tool to analyse survey results and other quantitative data.
- SPSS : Widely used for statistical analysis in research, offering advanced features for data interpretation.
- Power BI : Helps in visualising data in a user-friendly format, making it easier to identify trends and patterns.
Qualitative Data Analysis Tools
These tools help researchers organise, code, and interpret non-numerical data, such as text, images, and videos, facilitating thematic analysis, sentiment analysis, and visualisation of qualitative patterns.
- NVivo: For analysing qualitative data like interviews or open-ended survey responses, offering features for thematic analysis and sentiment analysis.
- Dedoose: Useful for mixed-methods research, combining qualitative and quantitative data.
General Data Collection and Management Tools
These tools provide comprehensive solutions for collecting, storing, and organising data from various sources.
- Airtable: Combines spreadsheet and database functionalities for organising data, making it versatile for different types of projects.
- Microsoft Excel: A widely used tool for data entry, analysis, and visualisation, suitable for various data collection needs.
Techniques for Effective Data Collection
Effective data collection involves several key techniques to ensure accuracy, reliability, and validity. Discover key techniques to enhance data collection. Learn how to ensure accuracy, reliability, and validity in your data collection processes for informed decision-making and research integrity.
Quality Assurance (QA)
Ensure data integrity by implementing quality assurance measures that prevent errors and maintain consistency throughout the data collection process. Quality assurance focuses on preventing errors before data collection begins. This includes:
- Standardisation of Protocol: Ensuring that the data collection protocol is standardised with comprehensive and detailed procedure descriptions.
- Training Staff: Providing rigorous training for staff members responsible for data collection to ensure they understand the procedures and instruments.
- Clear Instructions: Ensuring that instructions for using data collection instruments are clear and step-by-step to avoid confusion.
Quality Control (QC)
Quality control involves actions taken during and after data collection to ensure data integrity. This includes:
- Monitoring Systems: Establishing a clearly defined communication structure to monitor the data collection process and identify errors promptly.
- Documentation: Documenting all details of the data collection process to ensure transparency and accountability.
- Corrective Actions : Identifying and implementing corrective actions to address any issues that arise during data collection.
Operationalisation
Operationalization involves turning abstract conceptual ideas into measurable observations. For example, the concept of social anxiety can be operationally defined in terms of self-rating scores, behavioural avoidance of crowded places, or physical anxiety symptoms in social situations.
Choosing the right sampling method is crucial to gather representative and relevant data. Common sampling methods include random sampling, stratified sampling, and purposive sampling.
Data collection is a critical process that underpins research and business decision-making. By understanding the different methods, types of data, and tools available, organisations can ensure that their data collection processes are effective, reliable, and valid.
Implementing quality assurance and quality control measures, operationalizing variables, and selecting appropriate sampling methods are essential techniques for ensuring the integrity of the data collected. As technology continues to evolve, leveraging advanced tools for data collection, analysis, and visualisation will remain vital for extracting meaningful insights from the data.
Frequently Asked Questions
What is data collection, and why is it important.
Data collection is the systematic process of gathering observations or measurements to answer questions, analyse performance, and predict future trends. It is crucial for maintaining research integrity, informing decision-making, and evaluating outcomes.
What are the Main Differences Between Quantitative and Qualitative Data Collection Methods?
Quantitative data collection involves structured methods to gather numerical data, which is analysed statistically. Qualitative data collection involves open-ended methods to gather non-numerical data, which is analysed through interpretation and thematic analysis.
Quantitative methods are used to test hypotheses and identify patterns, while qualitative methods are used to explore complex phenomena and understand subjective experiences.
How can Technology Enhance the Data Collection Process?
Technology can significantly enhance the data collection process by providing tools for creating and distributing surveys, managing and analysing data, and visualising results. Digital survey platforms, database systems, and mobile data collection platforms streamline the process, reduce errors, and improve data quality.
Additionally, statistical software and data visualisation tools facilitate the analysis and interpretation of the collected data.
Reviewed by:

Post written by: Aashi Verma
- August 23, 2024
Deep Learning Explainability and Interpretability
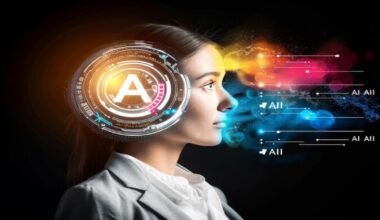
Artificial Intelligence What is Knowledge Representation in AI?
You may also like.

- Data Engineering
- Data Science
Data Science vs. Data Engineering: Unraveling the Key Differences
- Aishwarya Kurre
- July 26, 2023
- 6 minute read

Introduction to R Programming For Data Science
- July 10, 2023
- 9 minute read
- Privacy Policy

Home » Research Methodology – Types, Examples and writing Guide
Research Methodology – Types, Examples and writing Guide
Table of Contents

Research Methodology
Definition:
Research Methodology refers to the systematic and scientific approach used to conduct research, investigate problems, and gather data and information for a specific purpose. It involves the techniques and procedures used to identify, collect , analyze , and interpret data to answer research questions or solve research problems . Moreover, They are philosophical and theoretical frameworks that guide the research process.
Structure of Research Methodology
Research methodology formats can vary depending on the specific requirements of the research project, but the following is a basic example of a structure for a research methodology section:
I. Introduction
- Provide an overview of the research problem and the need for a research methodology section
- Outline the main research questions and objectives
II. Research Design
- Explain the research design chosen and why it is appropriate for the research question(s) and objectives
- Discuss any alternative research designs considered and why they were not chosen
- Describe the research setting and participants (if applicable)
III. Data Collection Methods
- Describe the methods used to collect data (e.g., surveys, interviews, observations)
- Explain how the data collection methods were chosen and why they are appropriate for the research question(s) and objectives
- Detail any procedures or instruments used for data collection
IV. Data Analysis Methods
- Describe the methods used to analyze the data (e.g., statistical analysis, content analysis )
- Explain how the data analysis methods were chosen and why they are appropriate for the research question(s) and objectives
- Detail any procedures or software used for data analysis
V. Ethical Considerations
- Discuss any ethical issues that may arise from the research and how they were addressed
- Explain how informed consent was obtained (if applicable)
- Detail any measures taken to ensure confidentiality and anonymity
VI. Limitations
- Identify any potential limitations of the research methodology and how they may impact the results and conclusions
VII. Conclusion
- Summarize the key aspects of the research methodology section
- Explain how the research methodology addresses the research question(s) and objectives
Research Methodology Types
Types of Research Methodology are as follows:
Quantitative Research Methodology
This is a research methodology that involves the collection and analysis of numerical data using statistical methods. This type of research is often used to study cause-and-effect relationships and to make predictions.
Qualitative Research Methodology
This is a research methodology that involves the collection and analysis of non-numerical data such as words, images, and observations. This type of research is often used to explore complex phenomena, to gain an in-depth understanding of a particular topic, and to generate hypotheses.
Mixed-Methods Research Methodology
This is a research methodology that combines elements of both quantitative and qualitative research. This approach can be particularly useful for studies that aim to explore complex phenomena and to provide a more comprehensive understanding of a particular topic.
Case Study Research Methodology
This is a research methodology that involves in-depth examination of a single case or a small number of cases. Case studies are often used in psychology, sociology, and anthropology to gain a detailed understanding of a particular individual or group.
Action Research Methodology
This is a research methodology that involves a collaborative process between researchers and practitioners to identify and solve real-world problems. Action research is often used in education, healthcare, and social work.
Experimental Research Methodology
This is a research methodology that involves the manipulation of one or more independent variables to observe their effects on a dependent variable. Experimental research is often used to study cause-and-effect relationships and to make predictions.
Survey Research Methodology
This is a research methodology that involves the collection of data from a sample of individuals using questionnaires or interviews. Survey research is often used to study attitudes, opinions, and behaviors.
Grounded Theory Research Methodology
This is a research methodology that involves the development of theories based on the data collected during the research process. Grounded theory is often used in sociology and anthropology to generate theories about social phenomena.
Research Methodology Example
An Example of Research Methodology could be the following:
Research Methodology for Investigating the Effectiveness of Cognitive Behavioral Therapy in Reducing Symptoms of Depression in Adults
Introduction:
The aim of this research is to investigate the effectiveness of cognitive-behavioral therapy (CBT) in reducing symptoms of depression in adults. To achieve this objective, a randomized controlled trial (RCT) will be conducted using a mixed-methods approach.
Research Design:
The study will follow a pre-test and post-test design with two groups: an experimental group receiving CBT and a control group receiving no intervention. The study will also include a qualitative component, in which semi-structured interviews will be conducted with a subset of participants to explore their experiences of receiving CBT.
Participants:
Participants will be recruited from community mental health clinics in the local area. The sample will consist of 100 adults aged 18-65 years old who meet the diagnostic criteria for major depressive disorder. Participants will be randomly assigned to either the experimental group or the control group.
Intervention :
The experimental group will receive 12 weekly sessions of CBT, each lasting 60 minutes. The intervention will be delivered by licensed mental health professionals who have been trained in CBT. The control group will receive no intervention during the study period.
Data Collection:
Quantitative data will be collected through the use of standardized measures such as the Beck Depression Inventory-II (BDI-II) and the Generalized Anxiety Disorder-7 (GAD-7). Data will be collected at baseline, immediately after the intervention, and at a 3-month follow-up. Qualitative data will be collected through semi-structured interviews with a subset of participants from the experimental group. The interviews will be conducted at the end of the intervention period, and will explore participants’ experiences of receiving CBT.
Data Analysis:
Quantitative data will be analyzed using descriptive statistics, t-tests, and mixed-model analyses of variance (ANOVA) to assess the effectiveness of the intervention. Qualitative data will be analyzed using thematic analysis to identify common themes and patterns in participants’ experiences of receiving CBT.
Ethical Considerations:
This study will comply with ethical guidelines for research involving human subjects. Participants will provide informed consent before participating in the study, and their privacy and confidentiality will be protected throughout the study. Any adverse events or reactions will be reported and managed appropriately.
Data Management:
All data collected will be kept confidential and stored securely using password-protected databases. Identifying information will be removed from qualitative data transcripts to ensure participants’ anonymity.
Limitations:
One potential limitation of this study is that it only focuses on one type of psychotherapy, CBT, and may not generalize to other types of therapy or interventions. Another limitation is that the study will only include participants from community mental health clinics, which may not be representative of the general population.
Conclusion:
This research aims to investigate the effectiveness of CBT in reducing symptoms of depression in adults. By using a randomized controlled trial and a mixed-methods approach, the study will provide valuable insights into the mechanisms underlying the relationship between CBT and depression. The results of this study will have important implications for the development of effective treatments for depression in clinical settings.
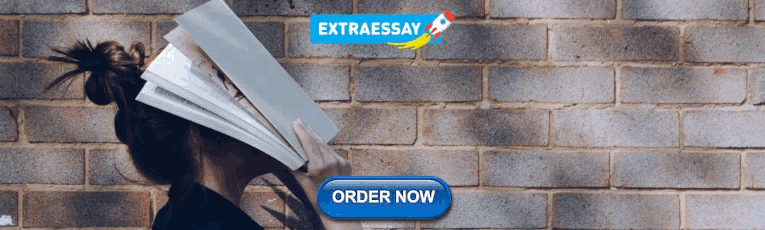
How to Write Research Methodology
Writing a research methodology involves explaining the methods and techniques you used to conduct research, collect data, and analyze results. It’s an essential section of any research paper or thesis, as it helps readers understand the validity and reliability of your findings. Here are the steps to write a research methodology:
- Start by explaining your research question: Begin the methodology section by restating your research question and explaining why it’s important. This helps readers understand the purpose of your research and the rationale behind your methods.
- Describe your research design: Explain the overall approach you used to conduct research. This could be a qualitative or quantitative research design, experimental or non-experimental, case study or survey, etc. Discuss the advantages and limitations of the chosen design.
- Discuss your sample: Describe the participants or subjects you included in your study. Include details such as their demographics, sampling method, sample size, and any exclusion criteria used.
- Describe your data collection methods : Explain how you collected data from your participants. This could include surveys, interviews, observations, questionnaires, or experiments. Include details on how you obtained informed consent, how you administered the tools, and how you minimized the risk of bias.
- Explain your data analysis techniques: Describe the methods you used to analyze the data you collected. This could include statistical analysis, content analysis, thematic analysis, or discourse analysis. Explain how you dealt with missing data, outliers, and any other issues that arose during the analysis.
- Discuss the validity and reliability of your research : Explain how you ensured the validity and reliability of your study. This could include measures such as triangulation, member checking, peer review, or inter-coder reliability.
- Acknowledge any limitations of your research: Discuss any limitations of your study, including any potential threats to validity or generalizability. This helps readers understand the scope of your findings and how they might apply to other contexts.
- Provide a summary: End the methodology section by summarizing the methods and techniques you used to conduct your research. This provides a clear overview of your research methodology and helps readers understand the process you followed to arrive at your findings.
When to Write Research Methodology
Research methodology is typically written after the research proposal has been approved and before the actual research is conducted. It should be written prior to data collection and analysis, as it provides a clear roadmap for the research project.
The research methodology is an important section of any research paper or thesis, as it describes the methods and procedures that will be used to conduct the research. It should include details about the research design, data collection methods, data analysis techniques, and any ethical considerations.
The methodology should be written in a clear and concise manner, and it should be based on established research practices and standards. It is important to provide enough detail so that the reader can understand how the research was conducted and evaluate the validity of the results.
Applications of Research Methodology
Here are some of the applications of research methodology:
- To identify the research problem: Research methodology is used to identify the research problem, which is the first step in conducting any research.
- To design the research: Research methodology helps in designing the research by selecting the appropriate research method, research design, and sampling technique.
- To collect data: Research methodology provides a systematic approach to collect data from primary and secondary sources.
- To analyze data: Research methodology helps in analyzing the collected data using various statistical and non-statistical techniques.
- To test hypotheses: Research methodology provides a framework for testing hypotheses and drawing conclusions based on the analysis of data.
- To generalize findings: Research methodology helps in generalizing the findings of the research to the target population.
- To develop theories : Research methodology is used to develop new theories and modify existing theories based on the findings of the research.
- To evaluate programs and policies : Research methodology is used to evaluate the effectiveness of programs and policies by collecting data and analyzing it.
- To improve decision-making: Research methodology helps in making informed decisions by providing reliable and valid data.
Purpose of Research Methodology
Research methodology serves several important purposes, including:
- To guide the research process: Research methodology provides a systematic framework for conducting research. It helps researchers to plan their research, define their research questions, and select appropriate methods and techniques for collecting and analyzing data.
- To ensure research quality: Research methodology helps researchers to ensure that their research is rigorous, reliable, and valid. It provides guidelines for minimizing bias and error in data collection and analysis, and for ensuring that research findings are accurate and trustworthy.
- To replicate research: Research methodology provides a clear and detailed account of the research process, making it possible for other researchers to replicate the study and verify its findings.
- To advance knowledge: Research methodology enables researchers to generate new knowledge and to contribute to the body of knowledge in their field. It provides a means for testing hypotheses, exploring new ideas, and discovering new insights.
- To inform decision-making: Research methodology provides evidence-based information that can inform policy and decision-making in a variety of fields, including medicine, public health, education, and business.
Advantages of Research Methodology
Research methodology has several advantages that make it a valuable tool for conducting research in various fields. Here are some of the key advantages of research methodology:
- Systematic and structured approach : Research methodology provides a systematic and structured approach to conducting research, which ensures that the research is conducted in a rigorous and comprehensive manner.
- Objectivity : Research methodology aims to ensure objectivity in the research process, which means that the research findings are based on evidence and not influenced by personal bias or subjective opinions.
- Replicability : Research methodology ensures that research can be replicated by other researchers, which is essential for validating research findings and ensuring their accuracy.
- Reliability : Research methodology aims to ensure that the research findings are reliable, which means that they are consistent and can be depended upon.
- Validity : Research methodology ensures that the research findings are valid, which means that they accurately reflect the research question or hypothesis being tested.
- Efficiency : Research methodology provides a structured and efficient way of conducting research, which helps to save time and resources.
- Flexibility : Research methodology allows researchers to choose the most appropriate research methods and techniques based on the research question, data availability, and other relevant factors.
- Scope for innovation: Research methodology provides scope for innovation and creativity in designing research studies and developing new research techniques.
Research Methodology Vs Research Methods
Research Methodology | Research Methods |
---|---|
Research methodology refers to the philosophical and theoretical frameworks that guide the research process. | refer to the techniques and procedures used to collect and analyze data. |
It is concerned with the underlying principles and assumptions of research. | It is concerned with the practical aspects of research. |
It provides a rationale for why certain research methods are used. | It determines the specific steps that will be taken to conduct research. |
It is broader in scope and involves understanding the overall approach to research. | It is narrower in scope and focuses on specific techniques and tools used in research. |
It is concerned with identifying research questions, defining the research problem, and formulating hypotheses. | It is concerned with collecting data, analyzing data, and interpreting results. |
It is concerned with the validity and reliability of research. | It is concerned with the accuracy and precision of data. |
It is concerned with the ethical considerations of research. | It is concerned with the practical considerations of research. |
About the author
Muhammad Hassan
Researcher, Academic Writer, Web developer
You may also like

Appendices – Writing Guide, Types and Examples

Limitations in Research – Types, Examples and...

Research Findings – Types Examples and Writing...

Scope of the Research – Writing Guide and...

How to Publish a Research Paper – Step by Step...

Research Paper Outline – Types, Example, Template

An official website of the United States government
The .gov means it’s official. Federal government websites often end in .gov or .mil. Before sharing sensitive information, make sure you’re on a federal government site.
The site is secure. The https:// ensures that you are connecting to the official website and that any information you provide is encrypted and transmitted securely.
- Publications
- Account settings
Preview improvements coming to the PMC website in October 2024. Learn More or Try it out now .
- Advanced Search
- Journal List
- J Korean Med Sci
- v.37(16); 2022 Apr 25

A Practical Guide to Writing Quantitative and Qualitative Research Questions and Hypotheses in Scholarly Articles
Edward barroga.
1 Department of General Education, Graduate School of Nursing Science, St. Luke’s International University, Tokyo, Japan.
Glafera Janet Matanguihan
2 Department of Biological Sciences, Messiah University, Mechanicsburg, PA, USA.
The development of research questions and the subsequent hypotheses are prerequisites to defining the main research purpose and specific objectives of a study. Consequently, these objectives determine the study design and research outcome. The development of research questions is a process based on knowledge of current trends, cutting-edge studies, and technological advances in the research field. Excellent research questions are focused and require a comprehensive literature search and in-depth understanding of the problem being investigated. Initially, research questions may be written as descriptive questions which could be developed into inferential questions. These questions must be specific and concise to provide a clear foundation for developing hypotheses. Hypotheses are more formal predictions about the research outcomes. These specify the possible results that may or may not be expected regarding the relationship between groups. Thus, research questions and hypotheses clarify the main purpose and specific objectives of the study, which in turn dictate the design of the study, its direction, and outcome. Studies developed from good research questions and hypotheses will have trustworthy outcomes with wide-ranging social and health implications.
INTRODUCTION
Scientific research is usually initiated by posing evidenced-based research questions which are then explicitly restated as hypotheses. 1 , 2 The hypotheses provide directions to guide the study, solutions, explanations, and expected results. 3 , 4 Both research questions and hypotheses are essentially formulated based on conventional theories and real-world processes, which allow the inception of novel studies and the ethical testing of ideas. 5 , 6
It is crucial to have knowledge of both quantitative and qualitative research 2 as both types of research involve writing research questions and hypotheses. 7 However, these crucial elements of research are sometimes overlooked; if not overlooked, then framed without the forethought and meticulous attention it needs. Planning and careful consideration are needed when developing quantitative or qualitative research, particularly when conceptualizing research questions and hypotheses. 4
There is a continuing need to support researchers in the creation of innovative research questions and hypotheses, as well as for journal articles that carefully review these elements. 1 When research questions and hypotheses are not carefully thought of, unethical studies and poor outcomes usually ensue. Carefully formulated research questions and hypotheses define well-founded objectives, which in turn determine the appropriate design, course, and outcome of the study. This article then aims to discuss in detail the various aspects of crafting research questions and hypotheses, with the goal of guiding researchers as they develop their own. Examples from the authors and peer-reviewed scientific articles in the healthcare field are provided to illustrate key points.
DEFINITIONS AND RELATIONSHIP OF RESEARCH QUESTIONS AND HYPOTHESES
A research question is what a study aims to answer after data analysis and interpretation. The answer is written in length in the discussion section of the paper. Thus, the research question gives a preview of the different parts and variables of the study meant to address the problem posed in the research question. 1 An excellent research question clarifies the research writing while facilitating understanding of the research topic, objective, scope, and limitations of the study. 5
On the other hand, a research hypothesis is an educated statement of an expected outcome. This statement is based on background research and current knowledge. 8 , 9 The research hypothesis makes a specific prediction about a new phenomenon 10 or a formal statement on the expected relationship between an independent variable and a dependent variable. 3 , 11 It provides a tentative answer to the research question to be tested or explored. 4
Hypotheses employ reasoning to predict a theory-based outcome. 10 These can also be developed from theories by focusing on components of theories that have not yet been observed. 10 The validity of hypotheses is often based on the testability of the prediction made in a reproducible experiment. 8
Conversely, hypotheses can also be rephrased as research questions. Several hypotheses based on existing theories and knowledge may be needed to answer a research question. Developing ethical research questions and hypotheses creates a research design that has logical relationships among variables. These relationships serve as a solid foundation for the conduct of the study. 4 , 11 Haphazardly constructed research questions can result in poorly formulated hypotheses and improper study designs, leading to unreliable results. Thus, the formulations of relevant research questions and verifiable hypotheses are crucial when beginning research. 12
CHARACTERISTICS OF GOOD RESEARCH QUESTIONS AND HYPOTHESES
Excellent research questions are specific and focused. These integrate collective data and observations to confirm or refute the subsequent hypotheses. Well-constructed hypotheses are based on previous reports and verify the research context. These are realistic, in-depth, sufficiently complex, and reproducible. More importantly, these hypotheses can be addressed and tested. 13
There are several characteristics of well-developed hypotheses. Good hypotheses are 1) empirically testable 7 , 10 , 11 , 13 ; 2) backed by preliminary evidence 9 ; 3) testable by ethical research 7 , 9 ; 4) based on original ideas 9 ; 5) have evidenced-based logical reasoning 10 ; and 6) can be predicted. 11 Good hypotheses can infer ethical and positive implications, indicating the presence of a relationship or effect relevant to the research theme. 7 , 11 These are initially developed from a general theory and branch into specific hypotheses by deductive reasoning. In the absence of a theory to base the hypotheses, inductive reasoning based on specific observations or findings form more general hypotheses. 10
TYPES OF RESEARCH QUESTIONS AND HYPOTHESES
Research questions and hypotheses are developed according to the type of research, which can be broadly classified into quantitative and qualitative research. We provide a summary of the types of research questions and hypotheses under quantitative and qualitative research categories in Table 1 .
Quantitative research questions | Quantitative research hypotheses |
---|---|
Descriptive research questions | Simple hypothesis |
Comparative research questions | Complex hypothesis |
Relationship research questions | Directional hypothesis |
Non-directional hypothesis | |
Associative hypothesis | |
Causal hypothesis | |
Null hypothesis | |
Alternative hypothesis | |
Working hypothesis | |
Statistical hypothesis | |
Logical hypothesis | |
Hypothesis-testing | |
Qualitative research questions | Qualitative research hypotheses |
Contextual research questions | Hypothesis-generating |
Descriptive research questions | |
Evaluation research questions | |
Explanatory research questions | |
Exploratory research questions | |
Generative research questions | |
Ideological research questions | |
Ethnographic research questions | |
Phenomenological research questions | |
Grounded theory questions | |
Qualitative case study questions |
Research questions in quantitative research
In quantitative research, research questions inquire about the relationships among variables being investigated and are usually framed at the start of the study. These are precise and typically linked to the subject population, dependent and independent variables, and research design. 1 Research questions may also attempt to describe the behavior of a population in relation to one or more variables, or describe the characteristics of variables to be measured ( descriptive research questions ). 1 , 5 , 14 These questions may also aim to discover differences between groups within the context of an outcome variable ( comparative research questions ), 1 , 5 , 14 or elucidate trends and interactions among variables ( relationship research questions ). 1 , 5 We provide examples of descriptive, comparative, and relationship research questions in quantitative research in Table 2 .
Quantitative research questions | |
---|---|
Descriptive research question | |
- Measures responses of subjects to variables | |
- Presents variables to measure, analyze, or assess | |
What is the proportion of resident doctors in the hospital who have mastered ultrasonography (response of subjects to a variable) as a diagnostic technique in their clinical training? | |
Comparative research question | |
- Clarifies difference between one group with outcome variable and another group without outcome variable | |
Is there a difference in the reduction of lung metastasis in osteosarcoma patients who received the vitamin D adjunctive therapy (group with outcome variable) compared with osteosarcoma patients who did not receive the vitamin D adjunctive therapy (group without outcome variable)? | |
- Compares the effects of variables | |
How does the vitamin D analogue 22-Oxacalcitriol (variable 1) mimic the antiproliferative activity of 1,25-Dihydroxyvitamin D (variable 2) in osteosarcoma cells? | |
Relationship research question | |
- Defines trends, association, relationships, or interactions between dependent variable and independent variable | |
Is there a relationship between the number of medical student suicide (dependent variable) and the level of medical student stress (independent variable) in Japan during the first wave of the COVID-19 pandemic? |
Hypotheses in quantitative research
In quantitative research, hypotheses predict the expected relationships among variables. 15 Relationships among variables that can be predicted include 1) between a single dependent variable and a single independent variable ( simple hypothesis ) or 2) between two or more independent and dependent variables ( complex hypothesis ). 4 , 11 Hypotheses may also specify the expected direction to be followed and imply an intellectual commitment to a particular outcome ( directional hypothesis ) 4 . On the other hand, hypotheses may not predict the exact direction and are used in the absence of a theory, or when findings contradict previous studies ( non-directional hypothesis ). 4 In addition, hypotheses can 1) define interdependency between variables ( associative hypothesis ), 4 2) propose an effect on the dependent variable from manipulation of the independent variable ( causal hypothesis ), 4 3) state a negative relationship between two variables ( null hypothesis ), 4 , 11 , 15 4) replace the working hypothesis if rejected ( alternative hypothesis ), 15 explain the relationship of phenomena to possibly generate a theory ( working hypothesis ), 11 5) involve quantifiable variables that can be tested statistically ( statistical hypothesis ), 11 6) or express a relationship whose interlinks can be verified logically ( logical hypothesis ). 11 We provide examples of simple, complex, directional, non-directional, associative, causal, null, alternative, working, statistical, and logical hypotheses in quantitative research, as well as the definition of quantitative hypothesis-testing research in Table 3 .
Quantitative research hypotheses | |
---|---|
Simple hypothesis | |
- Predicts relationship between single dependent variable and single independent variable | |
If the dose of the new medication (single independent variable) is high, blood pressure (single dependent variable) is lowered. | |
Complex hypothesis | |
- Foretells relationship between two or more independent and dependent variables | |
The higher the use of anticancer drugs, radiation therapy, and adjunctive agents (3 independent variables), the higher would be the survival rate (1 dependent variable). | |
Directional hypothesis | |
- Identifies study direction based on theory towards particular outcome to clarify relationship between variables | |
Privately funded research projects will have a larger international scope (study direction) than publicly funded research projects. | |
Non-directional hypothesis | |
- Nature of relationship between two variables or exact study direction is not identified | |
- Does not involve a theory | |
Women and men are different in terms of helpfulness. (Exact study direction is not identified) | |
Associative hypothesis | |
- Describes variable interdependency | |
- Change in one variable causes change in another variable | |
A larger number of people vaccinated against COVID-19 in the region (change in independent variable) will reduce the region’s incidence of COVID-19 infection (change in dependent variable). | |
Causal hypothesis | |
- An effect on dependent variable is predicted from manipulation of independent variable | |
A change into a high-fiber diet (independent variable) will reduce the blood sugar level (dependent variable) of the patient. | |
Null hypothesis | |
- A negative statement indicating no relationship or difference between 2 variables | |
There is no significant difference in the severity of pulmonary metastases between the new drug (variable 1) and the current drug (variable 2). | |
Alternative hypothesis | |
- Following a null hypothesis, an alternative hypothesis predicts a relationship between 2 study variables | |
The new drug (variable 1) is better on average in reducing the level of pain from pulmonary metastasis than the current drug (variable 2). | |
Working hypothesis | |
- A hypothesis that is initially accepted for further research to produce a feasible theory | |
Dairy cows fed with concentrates of different formulations will produce different amounts of milk. | |
Statistical hypothesis | |
- Assumption about the value of population parameter or relationship among several population characteristics | |
- Validity tested by a statistical experiment or analysis | |
The mean recovery rate from COVID-19 infection (value of population parameter) is not significantly different between population 1 and population 2. | |
There is a positive correlation between the level of stress at the workplace and the number of suicides (population characteristics) among working people in Japan. | |
Logical hypothesis | |
- Offers or proposes an explanation with limited or no extensive evidence | |
If healthcare workers provide more educational programs about contraception methods, the number of adolescent pregnancies will be less. | |
Hypothesis-testing (Quantitative hypothesis-testing research) | |
- Quantitative research uses deductive reasoning. | |
- This involves the formation of a hypothesis, collection of data in the investigation of the problem, analysis and use of the data from the investigation, and drawing of conclusions to validate or nullify the hypotheses. |
Research questions in qualitative research
Unlike research questions in quantitative research, research questions in qualitative research are usually continuously reviewed and reformulated. The central question and associated subquestions are stated more than the hypotheses. 15 The central question broadly explores a complex set of factors surrounding the central phenomenon, aiming to present the varied perspectives of participants. 15
There are varied goals for which qualitative research questions are developed. These questions can function in several ways, such as to 1) identify and describe existing conditions ( contextual research question s); 2) describe a phenomenon ( descriptive research questions ); 3) assess the effectiveness of existing methods, protocols, theories, or procedures ( evaluation research questions ); 4) examine a phenomenon or analyze the reasons or relationships between subjects or phenomena ( explanatory research questions ); or 5) focus on unknown aspects of a particular topic ( exploratory research questions ). 5 In addition, some qualitative research questions provide new ideas for the development of theories and actions ( generative research questions ) or advance specific ideologies of a position ( ideological research questions ). 1 Other qualitative research questions may build on a body of existing literature and become working guidelines ( ethnographic research questions ). Research questions may also be broadly stated without specific reference to the existing literature or a typology of questions ( phenomenological research questions ), may be directed towards generating a theory of some process ( grounded theory questions ), or may address a description of the case and the emerging themes ( qualitative case study questions ). 15 We provide examples of contextual, descriptive, evaluation, explanatory, exploratory, generative, ideological, ethnographic, phenomenological, grounded theory, and qualitative case study research questions in qualitative research in Table 4 , and the definition of qualitative hypothesis-generating research in Table 5 .
Qualitative research questions | |
---|---|
Contextual research question | |
- Ask the nature of what already exists | |
- Individuals or groups function to further clarify and understand the natural context of real-world problems | |
What are the experiences of nurses working night shifts in healthcare during the COVID-19 pandemic? (natural context of real-world problems) | |
Descriptive research question | |
- Aims to describe a phenomenon | |
What are the different forms of disrespect and abuse (phenomenon) experienced by Tanzanian women when giving birth in healthcare facilities? | |
Evaluation research question | |
- Examines the effectiveness of existing practice or accepted frameworks | |
How effective are decision aids (effectiveness of existing practice) in helping decide whether to give birth at home or in a healthcare facility? | |
Explanatory research question | |
- Clarifies a previously studied phenomenon and explains why it occurs | |
Why is there an increase in teenage pregnancy (phenomenon) in Tanzania? | |
Exploratory research question | |
- Explores areas that have not been fully investigated to have a deeper understanding of the research problem | |
What factors affect the mental health of medical students (areas that have not yet been fully investigated) during the COVID-19 pandemic? | |
Generative research question | |
- Develops an in-depth understanding of people’s behavior by asking ‘how would’ or ‘what if’ to identify problems and find solutions | |
How would the extensive research experience of the behavior of new staff impact the success of the novel drug initiative? | |
Ideological research question | |
- Aims to advance specific ideas or ideologies of a position | |
Are Japanese nurses who volunteer in remote African hospitals able to promote humanized care of patients (specific ideas or ideologies) in the areas of safe patient environment, respect of patient privacy, and provision of accurate information related to health and care? | |
Ethnographic research question | |
- Clarifies peoples’ nature, activities, their interactions, and the outcomes of their actions in specific settings | |
What are the demographic characteristics, rehabilitative treatments, community interactions, and disease outcomes (nature, activities, their interactions, and the outcomes) of people in China who are suffering from pneumoconiosis? | |
Phenomenological research question | |
- Knows more about the phenomena that have impacted an individual | |
What are the lived experiences of parents who have been living with and caring for children with a diagnosis of autism? (phenomena that have impacted an individual) | |
Grounded theory question | |
- Focuses on social processes asking about what happens and how people interact, or uncovering social relationships and behaviors of groups | |
What are the problems that pregnant adolescents face in terms of social and cultural norms (social processes), and how can these be addressed? | |
Qualitative case study question | |
- Assesses a phenomenon using different sources of data to answer “why” and “how” questions | |
- Considers how the phenomenon is influenced by its contextual situation. | |
How does quitting work and assuming the role of a full-time mother (phenomenon assessed) change the lives of women in Japan? |
Qualitative research hypotheses | |
---|---|
Hypothesis-generating (Qualitative hypothesis-generating research) | |
- Qualitative research uses inductive reasoning. | |
- This involves data collection from study participants or the literature regarding a phenomenon of interest, using the collected data to develop a formal hypothesis, and using the formal hypothesis as a framework for testing the hypothesis. | |
- Qualitative exploratory studies explore areas deeper, clarifying subjective experience and allowing formulation of a formal hypothesis potentially testable in a future quantitative approach. |
Qualitative studies usually pose at least one central research question and several subquestions starting with How or What . These research questions use exploratory verbs such as explore or describe . These also focus on one central phenomenon of interest, and may mention the participants and research site. 15
Hypotheses in qualitative research
Hypotheses in qualitative research are stated in the form of a clear statement concerning the problem to be investigated. Unlike in quantitative research where hypotheses are usually developed to be tested, qualitative research can lead to both hypothesis-testing and hypothesis-generating outcomes. 2 When studies require both quantitative and qualitative research questions, this suggests an integrative process between both research methods wherein a single mixed-methods research question can be developed. 1
FRAMEWORKS FOR DEVELOPING RESEARCH QUESTIONS AND HYPOTHESES
Research questions followed by hypotheses should be developed before the start of the study. 1 , 12 , 14 It is crucial to develop feasible research questions on a topic that is interesting to both the researcher and the scientific community. This can be achieved by a meticulous review of previous and current studies to establish a novel topic. Specific areas are subsequently focused on to generate ethical research questions. The relevance of the research questions is evaluated in terms of clarity of the resulting data, specificity of the methodology, objectivity of the outcome, depth of the research, and impact of the study. 1 , 5 These aspects constitute the FINER criteria (i.e., Feasible, Interesting, Novel, Ethical, and Relevant). 1 Clarity and effectiveness are achieved if research questions meet the FINER criteria. In addition to the FINER criteria, Ratan et al. described focus, complexity, novelty, feasibility, and measurability for evaluating the effectiveness of research questions. 14
The PICOT and PEO frameworks are also used when developing research questions. 1 The following elements are addressed in these frameworks, PICOT: P-population/patients/problem, I-intervention or indicator being studied, C-comparison group, O-outcome of interest, and T-timeframe of the study; PEO: P-population being studied, E-exposure to preexisting conditions, and O-outcome of interest. 1 Research questions are also considered good if these meet the “FINERMAPS” framework: Feasible, Interesting, Novel, Ethical, Relevant, Manageable, Appropriate, Potential value/publishable, and Systematic. 14
As we indicated earlier, research questions and hypotheses that are not carefully formulated result in unethical studies or poor outcomes. To illustrate this, we provide some examples of ambiguous research question and hypotheses that result in unclear and weak research objectives in quantitative research ( Table 6 ) 16 and qualitative research ( Table 7 ) 17 , and how to transform these ambiguous research question(s) and hypothesis(es) into clear and good statements.
Variables | Unclear and weak statement (Statement 1) | Clear and good statement (Statement 2) | Points to avoid |
---|---|---|---|
Research question | Which is more effective between smoke moxibustion and smokeless moxibustion? | “Moreover, regarding smoke moxibustion versus smokeless moxibustion, it remains unclear which is more effective, safe, and acceptable to pregnant women, and whether there is any difference in the amount of heat generated.” | 1) Vague and unfocused questions |
2) Closed questions simply answerable by yes or no | |||
3) Questions requiring a simple choice | |||
Hypothesis | The smoke moxibustion group will have higher cephalic presentation. | “Hypothesis 1. The smoke moxibustion stick group (SM group) and smokeless moxibustion stick group (-SLM group) will have higher rates of cephalic presentation after treatment than the control group. | 1) Unverifiable hypotheses |
Hypothesis 2. The SM group and SLM group will have higher rates of cephalic presentation at birth than the control group. | 2) Incompletely stated groups of comparison | ||
Hypothesis 3. There will be no significant differences in the well-being of the mother and child among the three groups in terms of the following outcomes: premature birth, premature rupture of membranes (PROM) at < 37 weeks, Apgar score < 7 at 5 min, umbilical cord blood pH < 7.1, admission to neonatal intensive care unit (NICU), and intrauterine fetal death.” | 3) Insufficiently described variables or outcomes | ||
Research objective | To determine which is more effective between smoke moxibustion and smokeless moxibustion. | “The specific aims of this pilot study were (a) to compare the effects of smoke moxibustion and smokeless moxibustion treatments with the control group as a possible supplement to ECV for converting breech presentation to cephalic presentation and increasing adherence to the newly obtained cephalic position, and (b) to assess the effects of these treatments on the well-being of the mother and child.” | 1) Poor understanding of the research question and hypotheses |
2) Insufficient description of population, variables, or study outcomes |
a These statements were composed for comparison and illustrative purposes only.
b These statements are direct quotes from Higashihara and Horiuchi. 16
Variables | Unclear and weak statement (Statement 1) | Clear and good statement (Statement 2) | Points to avoid |
---|---|---|---|
Research question | Does disrespect and abuse (D&A) occur in childbirth in Tanzania? | How does disrespect and abuse (D&A) occur and what are the types of physical and psychological abuses observed in midwives’ actual care during facility-based childbirth in urban Tanzania? | 1) Ambiguous or oversimplistic questions |
2) Questions unverifiable by data collection and analysis | |||
Hypothesis | Disrespect and abuse (D&A) occur in childbirth in Tanzania. | Hypothesis 1: Several types of physical and psychological abuse by midwives in actual care occur during facility-based childbirth in urban Tanzania. | 1) Statements simply expressing facts |
Hypothesis 2: Weak nursing and midwifery management contribute to the D&A of women during facility-based childbirth in urban Tanzania. | 2) Insufficiently described concepts or variables | ||
Research objective | To describe disrespect and abuse (D&A) in childbirth in Tanzania. | “This study aimed to describe from actual observations the respectful and disrespectful care received by women from midwives during their labor period in two hospitals in urban Tanzania.” | 1) Statements unrelated to the research question and hypotheses |
2) Unattainable or unexplorable objectives |
a This statement is a direct quote from Shimoda et al. 17
The other statements were composed for comparison and illustrative purposes only.
CONSTRUCTING RESEARCH QUESTIONS AND HYPOTHESES
To construct effective research questions and hypotheses, it is very important to 1) clarify the background and 2) identify the research problem at the outset of the research, within a specific timeframe. 9 Then, 3) review or conduct preliminary research to collect all available knowledge about the possible research questions by studying theories and previous studies. 18 Afterwards, 4) construct research questions to investigate the research problem. Identify variables to be accessed from the research questions 4 and make operational definitions of constructs from the research problem and questions. Thereafter, 5) construct specific deductive or inductive predictions in the form of hypotheses. 4 Finally, 6) state the study aims . This general flow for constructing effective research questions and hypotheses prior to conducting research is shown in Fig. 1 .

Research questions are used more frequently in qualitative research than objectives or hypotheses. 3 These questions seek to discover, understand, explore or describe experiences by asking “What” or “How.” The questions are open-ended to elicit a description rather than to relate variables or compare groups. The questions are continually reviewed, reformulated, and changed during the qualitative study. 3 Research questions are also used more frequently in survey projects than hypotheses in experiments in quantitative research to compare variables and their relationships.
Hypotheses are constructed based on the variables identified and as an if-then statement, following the template, ‘If a specific action is taken, then a certain outcome is expected.’ At this stage, some ideas regarding expectations from the research to be conducted must be drawn. 18 Then, the variables to be manipulated (independent) and influenced (dependent) are defined. 4 Thereafter, the hypothesis is stated and refined, and reproducible data tailored to the hypothesis are identified, collected, and analyzed. 4 The hypotheses must be testable and specific, 18 and should describe the variables and their relationships, the specific group being studied, and the predicted research outcome. 18 Hypotheses construction involves a testable proposition to be deduced from theory, and independent and dependent variables to be separated and measured separately. 3 Therefore, good hypotheses must be based on good research questions constructed at the start of a study or trial. 12
In summary, research questions are constructed after establishing the background of the study. Hypotheses are then developed based on the research questions. Thus, it is crucial to have excellent research questions to generate superior hypotheses. In turn, these would determine the research objectives and the design of the study, and ultimately, the outcome of the research. 12 Algorithms for building research questions and hypotheses are shown in Fig. 2 for quantitative research and in Fig. 3 for qualitative research.

EXAMPLES OF RESEARCH QUESTIONS FROM PUBLISHED ARTICLES
- EXAMPLE 1. Descriptive research question (quantitative research)
- - Presents research variables to be assessed (distinct phenotypes and subphenotypes)
- “BACKGROUND: Since COVID-19 was identified, its clinical and biological heterogeneity has been recognized. Identifying COVID-19 phenotypes might help guide basic, clinical, and translational research efforts.
- RESEARCH QUESTION: Does the clinical spectrum of patients with COVID-19 contain distinct phenotypes and subphenotypes? ” 19
- EXAMPLE 2. Relationship research question (quantitative research)
- - Shows interactions between dependent variable (static postural control) and independent variable (peripheral visual field loss)
- “Background: Integration of visual, vestibular, and proprioceptive sensations contributes to postural control. People with peripheral visual field loss have serious postural instability. However, the directional specificity of postural stability and sensory reweighting caused by gradual peripheral visual field loss remain unclear.
- Research question: What are the effects of peripheral visual field loss on static postural control ?” 20
- EXAMPLE 3. Comparative research question (quantitative research)
- - Clarifies the difference among groups with an outcome variable (patients enrolled in COMPERA with moderate PH or severe PH in COPD) and another group without the outcome variable (patients with idiopathic pulmonary arterial hypertension (IPAH))
- “BACKGROUND: Pulmonary hypertension (PH) in COPD is a poorly investigated clinical condition.
- RESEARCH QUESTION: Which factors determine the outcome of PH in COPD?
- STUDY DESIGN AND METHODS: We analyzed the characteristics and outcome of patients enrolled in the Comparative, Prospective Registry of Newly Initiated Therapies for Pulmonary Hypertension (COMPERA) with moderate or severe PH in COPD as defined during the 6th PH World Symposium who received medical therapy for PH and compared them with patients with idiopathic pulmonary arterial hypertension (IPAH) .” 21
- EXAMPLE 4. Exploratory research question (qualitative research)
- - Explores areas that have not been fully investigated (perspectives of families and children who receive care in clinic-based child obesity treatment) to have a deeper understanding of the research problem
- “Problem: Interventions for children with obesity lead to only modest improvements in BMI and long-term outcomes, and data are limited on the perspectives of families of children with obesity in clinic-based treatment. This scoping review seeks to answer the question: What is known about the perspectives of families and children who receive care in clinic-based child obesity treatment? This review aims to explore the scope of perspectives reported by families of children with obesity who have received individualized outpatient clinic-based obesity treatment.” 22
- EXAMPLE 5. Relationship research question (quantitative research)
- - Defines interactions between dependent variable (use of ankle strategies) and independent variable (changes in muscle tone)
- “Background: To maintain an upright standing posture against external disturbances, the human body mainly employs two types of postural control strategies: “ankle strategy” and “hip strategy.” While it has been reported that the magnitude of the disturbance alters the use of postural control strategies, it has not been elucidated how the level of muscle tone, one of the crucial parameters of bodily function, determines the use of each strategy. We have previously confirmed using forward dynamics simulations of human musculoskeletal models that an increased muscle tone promotes the use of ankle strategies. The objective of the present study was to experimentally evaluate a hypothesis: an increased muscle tone promotes the use of ankle strategies. Research question: Do changes in the muscle tone affect the use of ankle strategies ?” 23
EXAMPLES OF HYPOTHESES IN PUBLISHED ARTICLES
- EXAMPLE 1. Working hypothesis (quantitative research)
- - A hypothesis that is initially accepted for further research to produce a feasible theory
- “As fever may have benefit in shortening the duration of viral illness, it is plausible to hypothesize that the antipyretic efficacy of ibuprofen may be hindering the benefits of a fever response when taken during the early stages of COVID-19 illness .” 24
- “In conclusion, it is plausible to hypothesize that the antipyretic efficacy of ibuprofen may be hindering the benefits of a fever response . The difference in perceived safety of these agents in COVID-19 illness could be related to the more potent efficacy to reduce fever with ibuprofen compared to acetaminophen. Compelling data on the benefit of fever warrant further research and review to determine when to treat or withhold ibuprofen for early stage fever for COVID-19 and other related viral illnesses .” 24
- EXAMPLE 2. Exploratory hypothesis (qualitative research)
- - Explores particular areas deeper to clarify subjective experience and develop a formal hypothesis potentially testable in a future quantitative approach
- “We hypothesized that when thinking about a past experience of help-seeking, a self distancing prompt would cause increased help-seeking intentions and more favorable help-seeking outcome expectations .” 25
- “Conclusion
- Although a priori hypotheses were not supported, further research is warranted as results indicate the potential for using self-distancing approaches to increasing help-seeking among some people with depressive symptomatology.” 25
- EXAMPLE 3. Hypothesis-generating research to establish a framework for hypothesis testing (qualitative research)
- “We hypothesize that compassionate care is beneficial for patients (better outcomes), healthcare systems and payers (lower costs), and healthcare providers (lower burnout). ” 26
- Compassionomics is the branch of knowledge and scientific study of the effects of compassionate healthcare. Our main hypotheses are that compassionate healthcare is beneficial for (1) patients, by improving clinical outcomes, (2) healthcare systems and payers, by supporting financial sustainability, and (3) HCPs, by lowering burnout and promoting resilience and well-being. The purpose of this paper is to establish a scientific framework for testing the hypotheses above . If these hypotheses are confirmed through rigorous research, compassionomics will belong in the science of evidence-based medicine, with major implications for all healthcare domains.” 26
- EXAMPLE 4. Statistical hypothesis (quantitative research)
- - An assumption is made about the relationship among several population characteristics ( gender differences in sociodemographic and clinical characteristics of adults with ADHD ). Validity is tested by statistical experiment or analysis ( chi-square test, Students t-test, and logistic regression analysis)
- “Our research investigated gender differences in sociodemographic and clinical characteristics of adults with ADHD in a Japanese clinical sample. Due to unique Japanese cultural ideals and expectations of women's behavior that are in opposition to ADHD symptoms, we hypothesized that women with ADHD experience more difficulties and present more dysfunctions than men . We tested the following hypotheses: first, women with ADHD have more comorbidities than men with ADHD; second, women with ADHD experience more social hardships than men, such as having less full-time employment and being more likely to be divorced.” 27
- “Statistical Analysis
- ( text omitted ) Between-gender comparisons were made using the chi-squared test for categorical variables and Students t-test for continuous variables…( text omitted ). A logistic regression analysis was performed for employment status, marital status, and comorbidity to evaluate the independent effects of gender on these dependent variables.” 27
EXAMPLES OF HYPOTHESIS AS WRITTEN IN PUBLISHED ARTICLES IN RELATION TO OTHER PARTS
- EXAMPLE 1. Background, hypotheses, and aims are provided
- “Pregnant women need skilled care during pregnancy and childbirth, but that skilled care is often delayed in some countries …( text omitted ). The focused antenatal care (FANC) model of WHO recommends that nurses provide information or counseling to all pregnant women …( text omitted ). Job aids are visual support materials that provide the right kind of information using graphics and words in a simple and yet effective manner. When nurses are not highly trained or have many work details to attend to, these job aids can serve as a content reminder for the nurses and can be used for educating their patients (Jennings, Yebadokpo, Affo, & Agbogbe, 2010) ( text omitted ). Importantly, additional evidence is needed to confirm how job aids can further improve the quality of ANC counseling by health workers in maternal care …( text omitted )” 28
- “ This has led us to hypothesize that the quality of ANC counseling would be better if supported by job aids. Consequently, a better quality of ANC counseling is expected to produce higher levels of awareness concerning the danger signs of pregnancy and a more favorable impression of the caring behavior of nurses .” 28
- “This study aimed to examine the differences in the responses of pregnant women to a job aid-supported intervention during ANC visit in terms of 1) their understanding of the danger signs of pregnancy and 2) their impression of the caring behaviors of nurses to pregnant women in rural Tanzania.” 28
- EXAMPLE 2. Background, hypotheses, and aims are provided
- “We conducted a two-arm randomized controlled trial (RCT) to evaluate and compare changes in salivary cortisol and oxytocin levels of first-time pregnant women between experimental and control groups. The women in the experimental group touched and held an infant for 30 min (experimental intervention protocol), whereas those in the control group watched a DVD movie of an infant (control intervention protocol). The primary outcome was salivary cortisol level and the secondary outcome was salivary oxytocin level.” 29
- “ We hypothesize that at 30 min after touching and holding an infant, the salivary cortisol level will significantly decrease and the salivary oxytocin level will increase in the experimental group compared with the control group .” 29
- EXAMPLE 3. Background, aim, and hypothesis are provided
- “In countries where the maternal mortality ratio remains high, antenatal education to increase Birth Preparedness and Complication Readiness (BPCR) is considered one of the top priorities [1]. BPCR includes birth plans during the antenatal period, such as the birthplace, birth attendant, transportation, health facility for complications, expenses, and birth materials, as well as family coordination to achieve such birth plans. In Tanzania, although increasing, only about half of all pregnant women attend an antenatal clinic more than four times [4]. Moreover, the information provided during antenatal care (ANC) is insufficient. In the resource-poor settings, antenatal group education is a potential approach because of the limited time for individual counseling at antenatal clinics.” 30
- “This study aimed to evaluate an antenatal group education program among pregnant women and their families with respect to birth-preparedness and maternal and infant outcomes in rural villages of Tanzania.” 30
- “ The study hypothesis was if Tanzanian pregnant women and their families received a family-oriented antenatal group education, they would (1) have a higher level of BPCR, (2) attend antenatal clinic four or more times, (3) give birth in a health facility, (4) have less complications of women at birth, and (5) have less complications and deaths of infants than those who did not receive the education .” 30
Research questions and hypotheses are crucial components to any type of research, whether quantitative or qualitative. These questions should be developed at the very beginning of the study. Excellent research questions lead to superior hypotheses, which, like a compass, set the direction of research, and can often determine the successful conduct of the study. Many research studies have floundered because the development of research questions and subsequent hypotheses was not given the thought and meticulous attention needed. The development of research questions and hypotheses is an iterative process based on extensive knowledge of the literature and insightful grasp of the knowledge gap. Focused, concise, and specific research questions provide a strong foundation for constructing hypotheses which serve as formal predictions about the research outcomes. Research questions and hypotheses are crucial elements of research that should not be overlooked. They should be carefully thought of and constructed when planning research. This avoids unethical studies and poor outcomes by defining well-founded objectives that determine the design, course, and outcome of the study.
Disclosure: The authors have no potential conflicts of interest to disclose.
Author Contributions:
- Conceptualization: Barroga E, Matanguihan GJ.
- Methodology: Barroga E, Matanguihan GJ.
- Writing - original draft: Barroga E, Matanguihan GJ.
- Writing - review & editing: Barroga E, Matanguihan GJ.
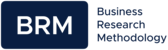
Data Collection Methods
Data collection is a process of collecting information from all the relevant sources to find answers to the research problem, test the hypothesis (if you are following deductive approach ) and evaluate the outcomes. Data collection methods can be divided into two categories: secondary methods of data collection and primary methods of data collection.
Secondary Data Collection Methods
Secondary data is a type of data that has already been published in books, newspapers, magazines, journals, online portals etc. There is an abundance of data available in these sources about your research area in business studies, almost regardless of the nature of the research area. Therefore, application of appropriate set of criteria to select secondary data to be used in the study plays an important role in terms of increasing the levels of research validity and reliability.
These criteria include, but not limited to date of publication, credential of the author, reliability of the source, quality of discussions, depth of analyses, the extent of contribution of the text to the development of the research area etc. Secondary data collection is discussed in greater depth in Literature Review chapter.
Secondary data collection methods offer a range of advantages such as saving time, effort and expenses. However they have a major disadvantage. Specifically, secondary research does not make contribution to the expansion of the literature by producing fresh (new) data.
Primary Data Collection Methods
Primary data is the type of data that has not been around before. Primary data is unique findings of your research. Primary data collection and analysis typically requires more time and effort to conduct compared to the secondary data research. Primary data collection methods can be divided into two groups: quantitative and qualitative.
Quantitative data collection methods are based on mathematical calculations in various formats. Methods of quantitative data collection and analysis include questionnaires with closed-ended questions, methods of correlation and regression, mean, mode and median and others.
Quantitative methods are cheaper to apply and they can be applied within shorter duration of time compared to qualitative methods. Moreover, due to a high level of standardisation of quantitative methods, it is easy to make comparisons of findings.
Qualitative research methods , on the contrary, do not involve numbers or mathematical calculations. Qualitative research is closely associated with words, sounds, feeling, emotions, colours and other elements that are non-quantifiable.
Qualitative studies aim to ensure greater level of depth of understanding and qualitative data collection methods include interviews, questionnaires with open-ended questions, focus groups, observation, game or role-playing, case studies etc.
Your choice between quantitative or qualitative methods of data collection depends on the area of your research and the nature of research aims and objectives.
My e-book, The Ultimate Guide to Writing a Dissertation in Business Studies: a step by step assistance offers practical assistance to complete a dissertation with minimum or no stress. The e-book covers all stages of writing a dissertation starting from the selection to the research area to submitting the completed version of the work within the deadline.
John Dudovskiy
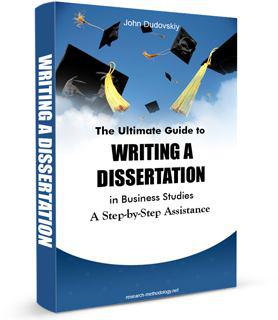
- Skip to main content
- Skip to primary sidebar
- Skip to footer
- QuestionPro

- Solutions Industries Gaming Automotive Sports and events Education Government Travel & Hospitality Financial Services Healthcare Cannabis Technology Use Case AskWhy Communities Audience Contactless surveys Mobile LivePolls Member Experience GDPR Positive People Science 360 Feedback Surveys
- Resources Blog eBooks Survey Templates Case Studies Training Help center

Home Market Research
Qualitative Data Collection: What it is + Methods to do it
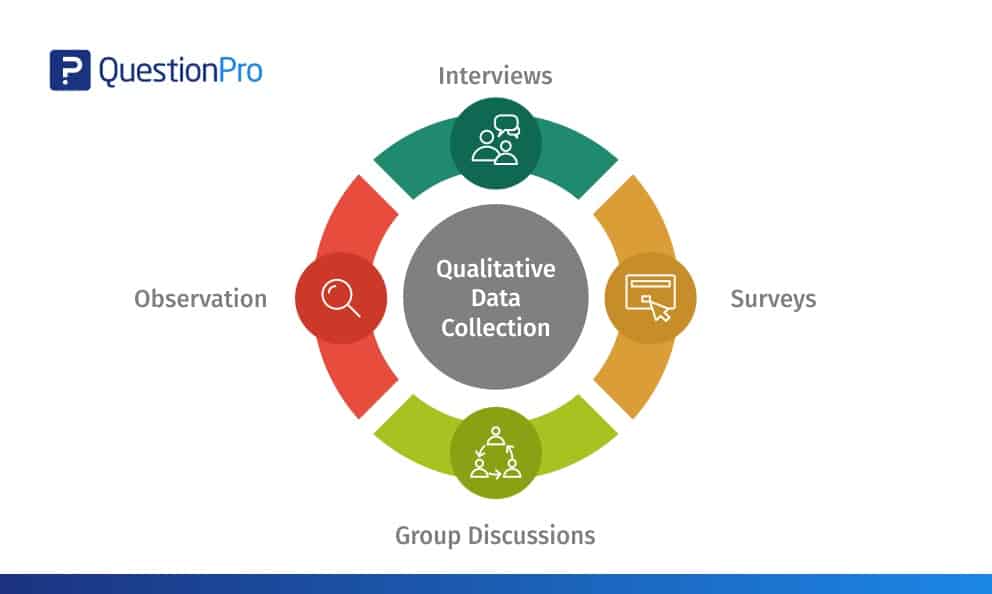
Qualitative data collection is vital in qualitative research. It helps researchers understand individuals’ attitudes, beliefs, and behaviors in a specific context.
Several methods are used to collect qualitative data, including interviews, surveys, focus groups, and observations. Understanding the various methods used for gathering qualitative data is essential for successful qualitative research.
In this post, we will discuss qualitative data and its collection methods of it.
Content Index
What is Qualitative Data?
What is qualitative data collection, what is the need for qualitative data collection, effective qualitative data collection methods, qualitative data analysis, advantages of qualitative data collection.
Qualitative data is defined as data that approximates and characterizes. It can be observed and recorded.
This data type is non-numerical in nature. This type of data is collected through methods of observations, one-to-one interviews, conducting focus groups, and similar methods.
Qualitative data in statistics is also known as categorical data – data that can be arranged categorically based on the attributes and properties of a thing or a phenomenon.
It’s pretty easy to understand the difference between qualitative and quantitative data. Qualitative data does not include numbers in its definition of traits, whereas quantitative research data is all about numbers.
- The cake is orange, blue, and black in color (qualitative).
- Females have brown, black, blonde, and red hair (qualitative).
Qualitative data collection is gathering non-numerical information, such as words, images, and observations, to understand individuals’ attitudes, behaviors, beliefs, and motivations in a specific context. It is an approach used in qualitative research. It seeks to understand social phenomena through in-depth exploration and analysis of people’s perspectives, experiences, and narratives. In statistical analysis , distinguishing between categorical data and numerical data is essential, as categorical data involves distinct categories or labels, while numerical data consists of measurable quantities.
The data collected through qualitative methods are often subjective, open-ended, and unstructured and can provide a rich and nuanced understanding of complex social phenomena.
Qualitative research is a type of study carried out with a qualitative approach to understand the exploratory reasons and to assay how and why a specific program or phenomenon operates in the way it is working. A researcher can access numerous qualitative data collection methods that he/she feels are relevant.
LEARN ABOUT: Best Data Collection Tools
Qualitative data collection methods serve the primary purpose of collecting textual data for research and analysis , like the thematic analysis. The collected research data is used to examine:
- Knowledge around a specific issue or a program, experience of people.
- Meaning and relationships.
- Social norms and contextual or cultural practices demean people or impact a cause.
The qualitative data is textual or non-numerical. It covers mostly the images, videos, texts, and written or spoken words by the people. You can opt for any digital data collection methods , like structured or semi-structured surveys, or settle for the traditional approach comprising individual interviews, group discussions, etc.
Data at hand leads to a smooth process ensuring all the decisions made are for the business’s betterment. You will be able to make informed decisions only if you have relevant data.
Well! With quality data, you will improve the quality of decision-making. But you will also enhance the quality of the results expected from any endeavor.
Qualitative data collection methods are exploratory. Those are usually more focused on gaining insights and understanding the underlying reasons by digging deeper.
Although quantitative data cannot be quantified, measuring it or analyzing qualitative data might become an issue. Due to the lack of measurability, collection methods of qualitative data are primarily unstructured or structured in rare cases – that too to some extent.
Let’s explore the most common methods used for the collection of qualitative data:
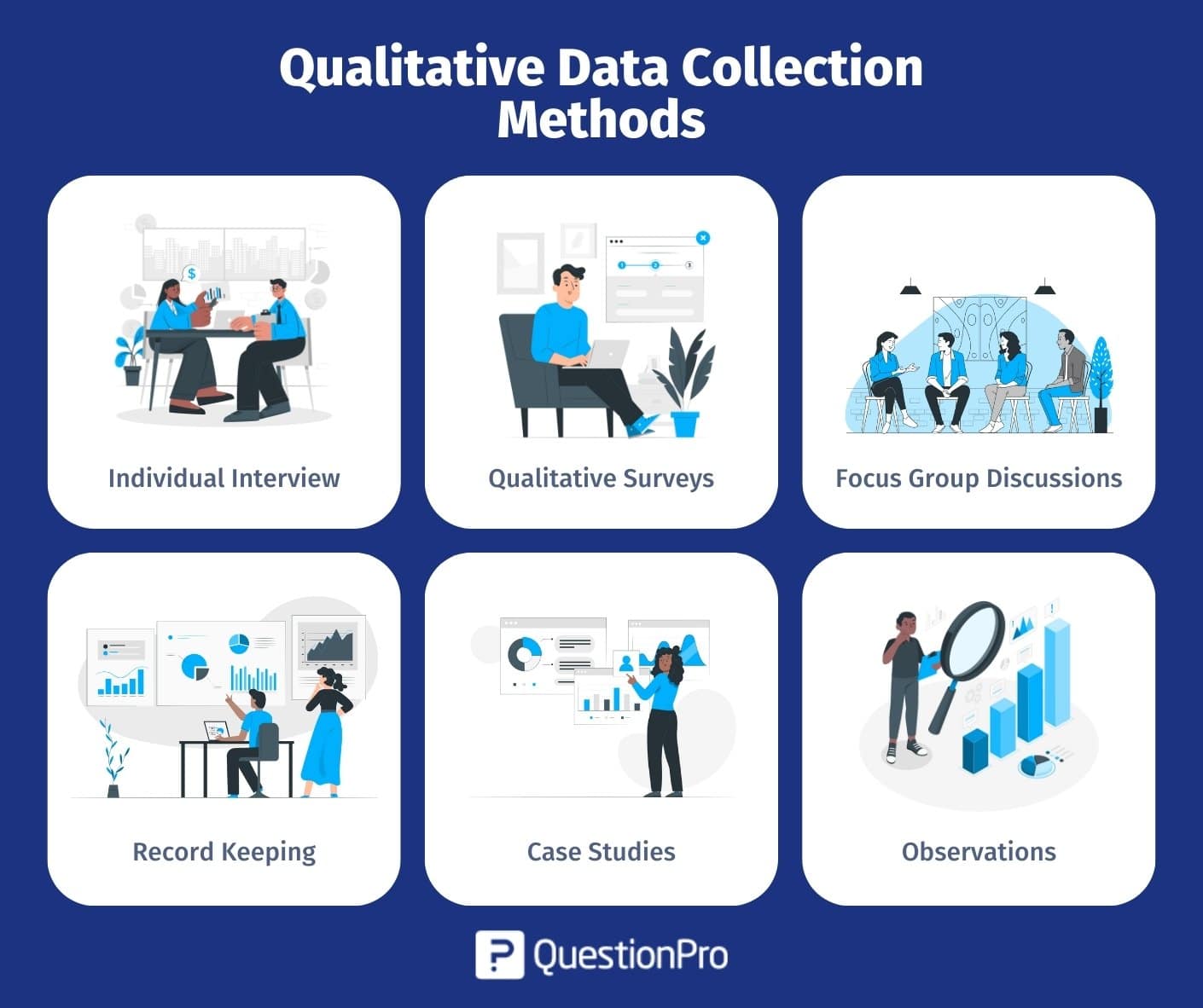
Individual interview
It is one of the most trusted, widely used, and familiar qualitative data collection methods primarily because of its approach. An individual or face-to-face interview is a direct conversation between two people with a specific structure and purpose.
The interview questionnaire is designed in the manner to elicit the interviewee’s knowledge or perspective related to a topic, program, or issue.
At times, depending on the interviewer’s approach, the conversation can be unstructured or informal but focused on understanding the individual’s beliefs, values, understandings, feelings, experiences, and perspectives on an issue.
More often, the interviewer chooses to ask open-ended questions in individual interviews. If the interviewee selects answers from a set of given options, it becomes a structured, fixed response or a biased discussion.
The individual interview is an ideal qualitative data collection method. Particularly when the researchers want highly personalized information from the participants. The individual interview is a notable method if the interviewer decides to probe further and ask follow-up questions to gain more insights.
Qualitative surveys
To develop an informed hypothesis, many researchers use qualitative research surveys for data collection or to collect a piece of detailed information about a product or an issue. If you want to create questionnaires for collecting textual or qualitative data, then ask more open-ended questions .
LEARN ABOUT: Research Process Steps
To answer such qualitative research questions , the respondent has to write his/her opinion or perspective concerning a specific topic or issue. Unlike other collection methods, online surveys have a wider reach. People can provide you with quality data that is highly credible and valuable.
Paper surveys
Online surveys, focus group discussions.
Focus group discussions can also be considered a type of interview, but it is conducted in a group discussion setting. Usually, the focus group consists of 8 – 10 people (the size may vary depending on the researcher’s requirement). The researchers ensure appropriate space is given to the participants to discuss a topic or issue in a context. The participants are allowed to either agree or disagree with each other’s comments.
With a focused group discussion, researchers know how a particular group of participants perceives the topic. Researchers analyze what participants think of an issue, the range of opinions expressed, and the ideas discussed. The data is collected by noting down the variations or inconsistencies (if any exist) in the participants, especially in terms of belief, experiences, and practice.
The participants of focused group discussions are selected based on the topic or issues for which the researcher wants actionable insights. For example, if the research is about the recovery of college students from drug addiction. The participants have to be college students studying and recovering from drug addiction.
Other parameters such as age, qualification, financial background, social presence, and demographics are also considered, but not primarily, as the group needs diverse participants. Frequently, the qualitative data collected through focused group discussion is more descriptive and highly detailed.
Record keeping
This method uses reliable documents and other sources of information that already exist as the data source. This information can help with the new study. It’s a lot like going to the library. There, you can look through books and other sources to find information that can be used in your research.
Case studies
In this method, data is collected by looking at case studies in detail. This method’s flexibility is shown by the fact that it can be used to analyze both simple and complicated topics. This method’s strength is how well it draws conclusions from a mix of one or more qualitative data collection methods.
Observations
Observation is one of the traditional methods of qualitative data collection. It is used by researchers to gather descriptive analysis data by observing people and their behavior at events or in their natural settings. In this method, the researcher is completely immersed in watching people by taking a participatory stance to take down notes.
There are two main types of observation:
- Covert: In this method, the observer is concealed without letting anyone know that they are being observed. For example, a researcher studying the rituals of a wedding in nomadic tribes must join them as a guest and quietly see everything.
- Overt: In this method, everyone is aware that they are being watched. For example, A researcher or an observer wants to study the wedding rituals of a nomadic tribe. To proceed with the research, the observer or researcher can reveal why he is attending the marriage and even use a video camera to shoot everything around him.
Observation is a useful method of qualitative data collection, especially when you want to study the ongoing process, situation, or reactions on a specific issue related to the people being observed.
When you want to understand people’s behavior or their way of interaction in a particular community or demographic, you can rely on the observation data. Remember, if you fail to get quality data through surveys, qualitative interviews , or group discussions, rely on observation.
It is the best and most trusted collection method of qualitative data to generate qualitative data as it requires equal to no effort from the participants.
LEARN ABOUT: Behavioral Research
You invested time and money acquiring your data, so analyze it. It’s necessary to avoid being in the dark after all your hard work. Qualitative data analysis starts with knowing its two basic techniques, but there are no rules.
- Deductive Approach: The deductive data analysis uses a researcher-defined structure to analyze qualitative data. This method is quick and easy when a researcher knows what the sample population will say.
- Inductive Approach: The inductive technique has no structure or framework. When a researcher knows little about the event, an inductive approach is applied.
Whether you want to analyze qualitative data from a one-on-one interview or a survey, these simple steps will ensure a comprehensive qualitative data analysis.
Step 1: Arrange your Data
After collecting all the data, it is mostly unstructured and sometimes unclear. Arranging your data is the first stage in qualitative data analysis. So, researchers must transcribe data before analyzing it.
Step 2: Organize all your Data
After transforming and arranging your data, the next step is to organize it. One of the best ways to organize the data is to think back to your research goals and then organize the data based on the research questions you asked.
Step 3: Set a Code to the Data Collected
Setting up appropriate codes for the collected data gets you one step closer. Coding is one of the most effective methods for compressing a massive amount of data. It allows you to derive theories from relevant research findings.
Step 4: Validate your Data
Qualitative data analysis success requires data validation. Data validation should be done throughout the research process, not just once. There are two sides to validating data:
- The accuracy of your research design or methods.
- Reliability—how well the approaches deliver accurate data.
Step 5: Concluding the Analysis Process
Finally, conclude your data in a presentable report. The report should describe your research methods, their pros and cons, and research limitations. Your report should include findings, inferences, and future research.
QuestionPro is a comprehensive online survey software that offers a variety of qualitative data analysis tools to help businesses and researchers in making sense of their data. Users can use many different qualitative analysis methods to learn more about their data.
Users of QuestionPro can see their data in different charts and graphs, which makes it easier to spot patterns and trends. It can help researchers and businesses learn more about their target audience, which can lead to better decisions and better results.
LEARN ABOUT: Steps in Qualitative Research
Qualitative data collection has several advantages, including:
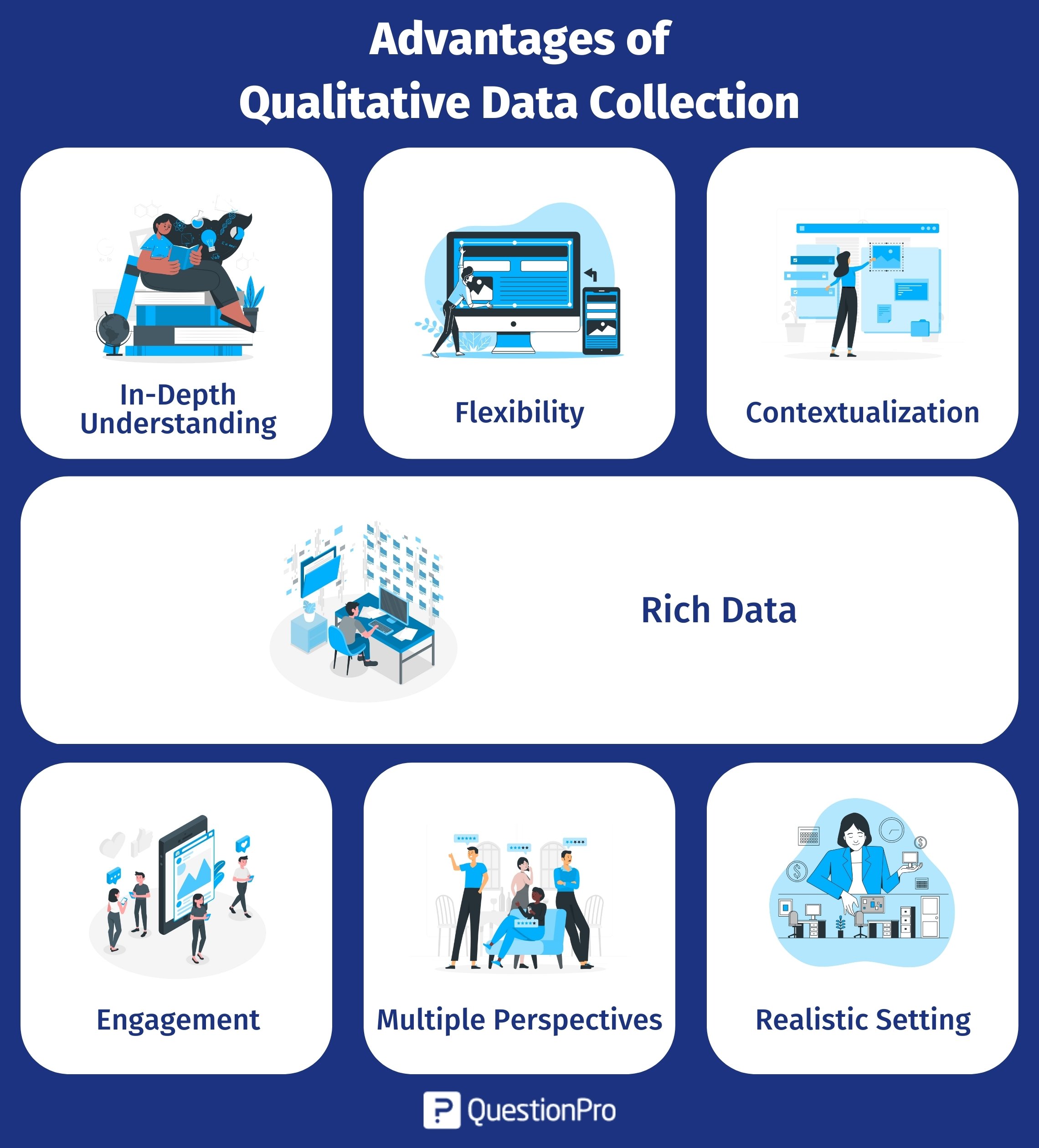
- In-depth understanding: It provides in-depth information about attitudes and behaviors, leading to a deeper understanding of the research.
- Flexibility: The methods allow researchers to modify questions or change direction if new information emerges.
- Contextualization: Qualitative research data is in context, which helps to provide a deep understanding of the experiences and perspectives of individuals.
- Rich data: It often produces rich, detailed, and nuanced information that cannot capture through numerical data.
- Engagement: The methods, such as interviews and focus groups, involve active meetings with participants, leading to a deeper understanding.
- Multiple perspectives: This can provide various views and a rich array of voices, adding depth and complexity.
- Realistic setting: It often occurs in realistic settings, providing more authentic experiences and behaviors.
LEARN ABOUT: 12 Best Tools for Researchers
Qualitative research is one of the best methods for identifying the behavior and patterns governing social conditions, issues, or topics. It spans a step ahead of quantitative data as it fails to explain the reasons and rationale behind a phenomenon, but qualitative data quickly does.
Qualitative research is one of the best tools to identify behaviors and patterns governing social conditions. It goes a step beyond quantitative data by providing the reasons and rationale behind a phenomenon that cannot be explored quantitatively.
With QuestionPro, you can use it for qualitative data collection through various methods. Using Our robust suite correctly, you can enhance the quality and integrity of the collected data.
LEARN MORE FREE TRIAL
MORE LIKE THIS
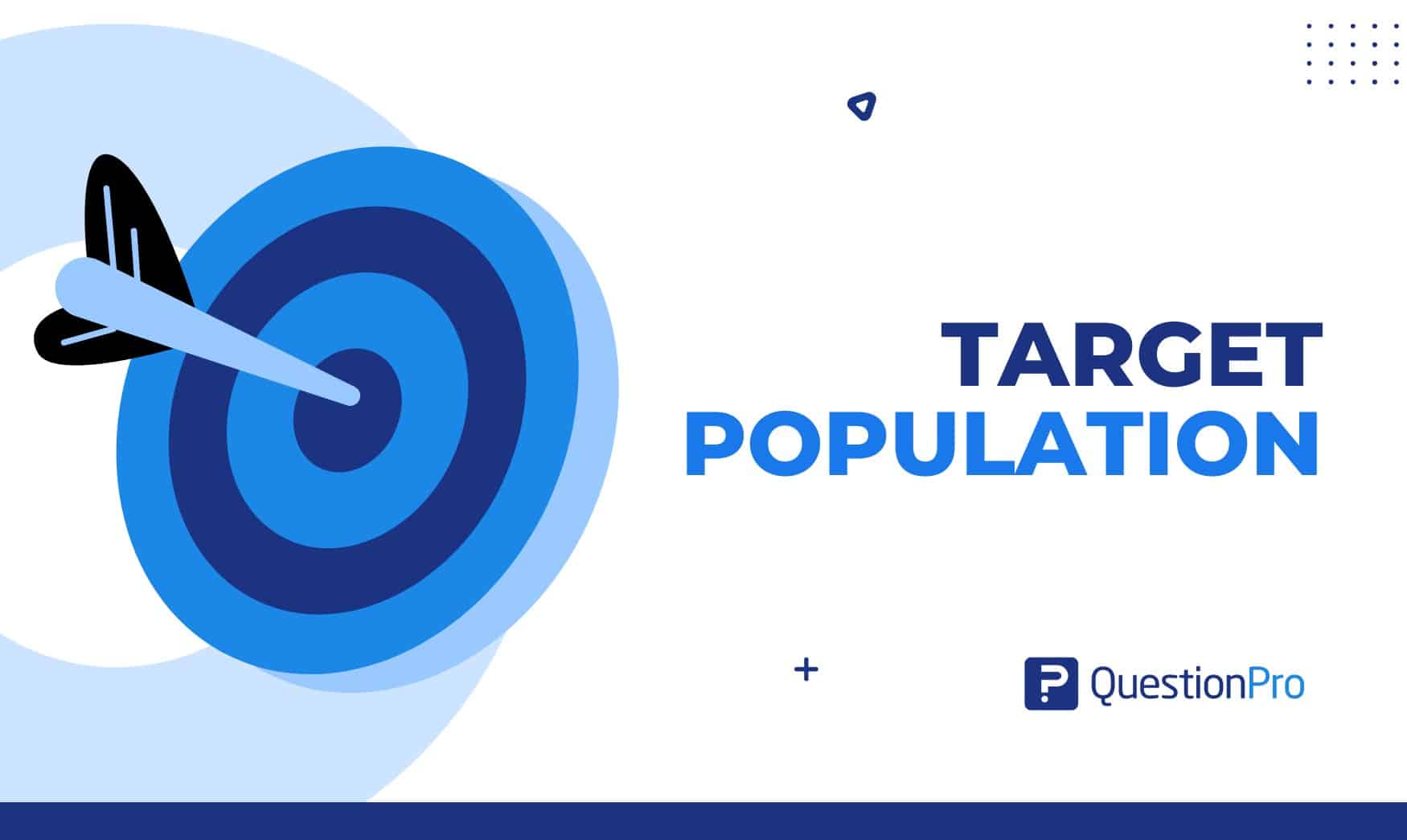
Target Population: What It Is + Strategies for Targeting
Aug 29, 2024
Microsoft Customer Voice vs QuestionPro: Choosing the Best
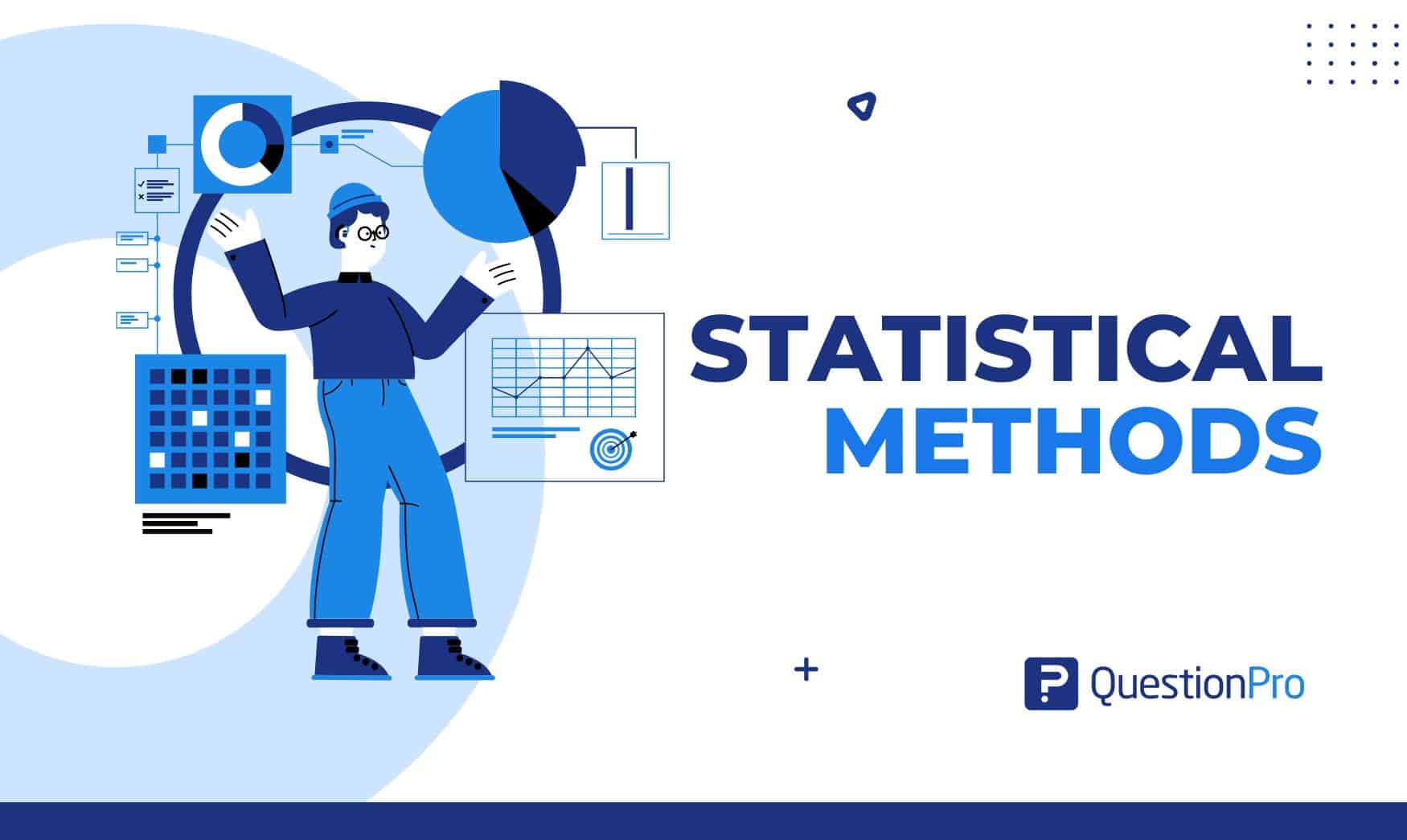
Statistical Methods: What It Is, Process, Analyze & Present
Aug 28, 2024
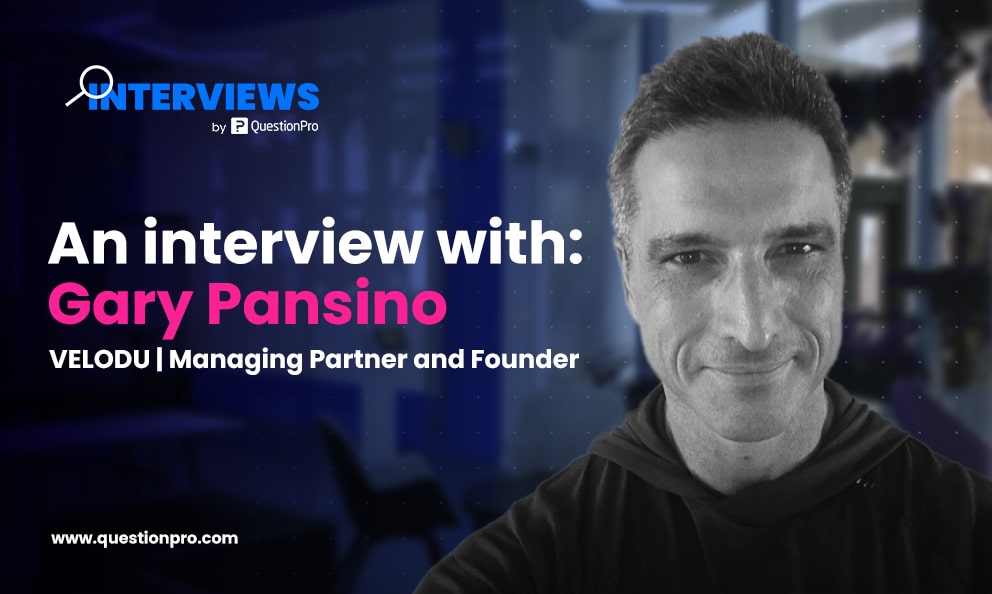
Velodu and QuestionPro: Connecting Data with a Human Touch
Other categories.
- Academic Research
- Artificial Intelligence
- Assessments
- Brand Awareness
- Case Studies
- Communities
- Consumer Insights
- Customer effort score
- Customer Engagement
- Customer Experience
- Customer Loyalty
- Customer Research
- Customer Satisfaction
- Employee Benefits
- Employee Engagement
- Employee Retention
- Friday Five
- General Data Protection Regulation
- Insights Hub
- Life@QuestionPro
- Market Research
- Mobile diaries
- Mobile Surveys
- New Features
- Online Communities
- Question Types
- Questionnaire
- QuestionPro Products
- Release Notes
- Research Tools and Apps
- Revenue at Risk
- Survey Templates
- Training Tips
- Tuesday CX Thoughts (TCXT)
- Uncategorized
- What’s Coming Up
- Workforce Intelligence
- Online panels
- Data-Collection Services
- Full-Service Research
- Global Omnibus
- Case Studies
- Quality Assurance
- Work with us
- Affiliation
- TGM Content Hub
- Bid Request
- Create A Sampling Plan Guide
Create A Survey Sampling Plan In Seven Simple Steps
What is a Sampling Plan in Market Research?
Why is a sampling plan crucial for a market research project, seven steps to create a sampling plan for your research survey.

Step 1: Define Your Survey Goals and Objectives
Step 2: determine your sampling frame, step 3: choose your sampling method, step 4: determine the sample size.
- Qualitative Studies: Typically involve smaller samples, continuing until theoretical saturation is reached—when new data no longer provides additional insights.For example, if you’re interviewing participants for a study on a specific behavioral pattern and you start noticing that each new interview is just repeating what has already been said, you’ve likely reached theoretical saturation.
- Quantitative Studies: Use statistical methods to calculate sample size based on desired confidence levels and margins of error. For example, a 95% confidence level with a 5% margin of error requires precise calculations to ensure validity. Avoid generic rules like "100 subjects is enough"; instead, perform a tailored power analysis or consult detailed research for precise calculations.
Learn more about choosing between qualitative or quantitative research .
Step 5: Select Your Data Collection Method
Step 6: test your survey, step 7: implement your survey.
Discover advanced tools to combat sample and survey fraud and maintain data integrity.
Types of Sampling Methods Used within a Sampling Plan
- Probability Sampling: This method focuses on ensuring that every individual in the population has a fair chance of being selected. Techniques like Simple Random Sampling and Stratified Sampling emphasize randomness and representation. Cluster Sampling and Multistage Sampling add layers of structure, making them particularly useful for large or geographically dispersed populations.
- Non-Probability Sampling: In contrast, this method relies on more subjective criteria, selecting participants based on ease of access ( Convenience Sampling ) or specific characteristics (Purposive Sampling). Quota Sampling and Snowball Sampling build on these principles, with Quota ensuring representation across segments and Snowball leveraging participant networks.
- Mixed Sampling: This approach blends elements from both probability and non-probability methods. For example, Stratified Cluster Sampling combines the stratification of probability sampling with the practicality of cluster sampling, while Sequential Sampling might start with a random selection and then narrow down the sample based on specific criteria, merging both randomization and purposiveness.
For a detailed guide on Survey Sampling Methods, visit https://tgmresearch.com/survey-sampling-methods.html !
What are the Most Important Things to Consider When Creating a Survey Sampling Plan?

1. Understanding the target audience
2. choosing the right sampling method, 3. determining an adequate sample size, 4. addressing non-response bias:, 5. constructing clear questions and statements:, 6. choosing appropriate response types:, 7. using reliable scales and measures:.
- Invalid scales often lack relevance, misalign with research goals, or are inappropriate for the population. For example, a scale that measures employee satisfaction but only includes questions about office decor, rather than job duties or management quality, is likely invalid.
- Unreliable scales produce inconsistent results, show significant variations over time, or have high error rates. An example is a personality test that gives different results for the same person on different days without changes in their traits.
8. Considering analysis techniques
Managing resources for effective sampling and tracking progress.
- Resource Allocation: Assign roles based on expertise, have project managers oversee timelines and progress, and create a detailed budget for participant incentives, tools, and software, monitoring it regularly to avoid overspending.
- Training: Train your team in sampling methods and tools to minimize errors and enhance data quality. Ongoing professional development helps keep your team updated on best practices.
- Time Management: Develop a project timeline with clear deadlines for each phase. Use project management tools to track progress and adjust as needed.
- Monitoring Progress: Utilize software to track sampling progress in real-time. Regular team check-ins can identify and address challenges early.
- Quality Control: Implement quality checks at each stage to ensure accuracy in your sampling process and take corrective actions as needed.
- Contingency Planning: Prepare for unforeseen challenges with a contingency plan, including budget reserves and backup team members to maintain project continuity.
Key Software and Tools for Effective Sampling Plan Development
1. sampling plan templates and guides.
- SurveyMonkey: Offers a range of sampling plan templates and guides to help you design your sampling strategy.
- Qualtrics: Provides sampling plan resources including templates and best practices.
2. Sample Size Calculators
- Raosoft Sample Size Calculator: A user-friendly tool for calculating sample size based on margin of error, confidence level, and population size.
- Epi Info: Offers a sample size calculator for epidemiological studies and surveys.
3. Sampling Methods and Techniques
- TGM Research Blog: Provides detailed explanations of various sampling methods, including probability and non-probability sampling techniques.
- StatTrek: Offers comprehensive guides and examples on sampling methods and their applications.
4. Data Collection Tools
- SurveyMonkey: A popular online survey tool that supports various data collection methods including online surveys.
- Google Forms: A free tool for creating and distributing online surveys with real-time data collection and analysis.
5. Statistical Analysis Software
- SPSS: Provides robust statistical analysis tools for analyzing survey data and determining sample representativeness. Learn more about SPSS.
- R: An open-source programming language and software environment for statistical computing and graphics, useful for analyzing complex sampling data.
A strong sampling plan should include a representative sample, an adequate sample size, a well-defined sampling frame, and appropriate sampling techniques. These elements are crucial for producing high-quality, reliable research results.
A good sample size depends on the population size, the desired confidence level, and the margin of error. Typically, larger sample sizes provide more accurate results, but for most surveys, a sample size of around 350-400 participants is often sufficient to achieve a 95% confidence level with a 5% margin of error for a population of several thousand.
To choose a sampling method, consider the research goals, population characteristics, and resources available. Common methods include random sampling for generalizability, stratified sampling to ensure representation across key subgroups, and convenience sampling when ease and speed are priorities. The choice should align with the need for accuracy, representativeness, and feasibility.
Developing a sampling plan involves challenges such as defining a comprehensive sampling frame, choosing the right method, and determining an adequate sample size. Ensuring representativeness, minimizing bias, managing non-response rates, and addressing logistical constraints are also difficult but essential for effective planning. Overcoming these challenges requires careful planning and a deep understanding of sampling techniques and research goals.
Theoretical saturation is the stage in grounded theory analysis where additional data no longer adds new insights into the topic. In grounded theory, data collection and analysis continue iteratively until no further significant information is gained. Once this point is reached, additional data collection is unnecessary.
The Confidence Level (CL) a statistical measure of the likelihood that test results fall within a specified range. For example, a 95% Confidence Level suggests that outcomes are expected to meet expectations 95% of the time.
The margin of error is a statistic measuring the degree of random sampling error in survey results. A larger margin of error implies less certainty that the survey findings accurately represent the entire population.
Articles to read

Transform your approach. Let's talk research!
Have a language expert improve your writing
Run a free plagiarism check in 10 minutes, generate accurate citations for free.
- Knowledge Base
Methodology
- Questionnaire Design | Methods, Question Types & Examples
Questionnaire Design | Methods, Question Types & Examples
Published on July 15, 2021 by Pritha Bhandari . Revised on June 22, 2023.
A questionnaire is a list of questions or items used to gather data from respondents about their attitudes, experiences, or opinions. Questionnaires can be used to collect quantitative and/or qualitative information.
Questionnaires are commonly used in market research as well as in the social and health sciences. For example, a company may ask for feedback about a recent customer service experience, or psychology researchers may investigate health risk perceptions using questionnaires.
Table of contents
Questionnaires vs. surveys, questionnaire methods, open-ended vs. closed-ended questions, question wording, question order, step-by-step guide to design, other interesting articles, frequently asked questions about questionnaire design.
A survey is a research method where you collect and analyze data from a group of people. A questionnaire is a specific tool or instrument for collecting the data.
Designing a questionnaire means creating valid and reliable questions that address your research objectives , placing them in a useful order, and selecting an appropriate method for administration.
But designing a questionnaire is only one component of survey research. Survey research also involves defining the population you’re interested in, choosing an appropriate sampling method , administering questionnaires, data cleansing and analysis, and interpretation.
Sampling is important in survey research because you’ll often aim to generalize your results to the population. Gather data from a sample that represents the range of views in the population for externally valid results. There will always be some differences between the population and the sample, but minimizing these will help you avoid several types of research bias , including sampling bias , ascertainment bias , and undercoverage bias .
Prevent plagiarism. Run a free check.
Questionnaires can be self-administered or researcher-administered . Self-administered questionnaires are more common because they are easy to implement and inexpensive, but researcher-administered questionnaires allow deeper insights.
Self-administered questionnaires
Self-administered questionnaires can be delivered online or in paper-and-pen formats, in person or through mail. All questions are standardized so that all respondents receive the same questions with identical wording.
Self-administered questionnaires can be:
- cost-effective
- easy to administer for small and large groups
- anonymous and suitable for sensitive topics
But they may also be:
- unsuitable for people with limited literacy or verbal skills
- susceptible to a nonresponse bias (most people invited may not complete the questionnaire)
- biased towards people who volunteer because impersonal survey requests often go ignored.
Researcher-administered questionnaires
Researcher-administered questionnaires are interviews that take place by phone, in-person, or online between researchers and respondents.
Researcher-administered questionnaires can:
- help you ensure the respondents are representative of your target audience
- allow clarifications of ambiguous or unclear questions and answers
- have high response rates because it’s harder to refuse an interview when personal attention is given to respondents
But researcher-administered questionnaires can be limiting in terms of resources. They are:
- costly and time-consuming to perform
- more difficult to analyze if you have qualitative responses
- likely to contain experimenter bias or demand characteristics
- likely to encourage social desirability bias in responses because of a lack of anonymity
Your questionnaire can include open-ended or closed-ended questions or a combination of both.
Using closed-ended questions limits your responses, while open-ended questions enable a broad range of answers. You’ll need to balance these considerations with your available time and resources.
Closed-ended questions
Closed-ended, or restricted-choice, questions offer respondents a fixed set of choices to select from. Closed-ended questions are best for collecting data on categorical or quantitative variables.
Categorical variables can be nominal or ordinal. Quantitative variables can be interval or ratio. Understanding the type of variable and level of measurement means you can perform appropriate statistical analyses for generalizable results.
Examples of closed-ended questions for different variables
Nominal variables include categories that can’t be ranked, such as race or ethnicity. This includes binary or dichotomous categories.
It’s best to include categories that cover all possible answers and are mutually exclusive. There should be no overlap between response items.
In binary or dichotomous questions, you’ll give respondents only two options to choose from.
White Black or African American American Indian or Alaska Native Asian Native Hawaiian or Other Pacific Islander
Ordinal variables include categories that can be ranked. Consider how wide or narrow a range you’ll include in your response items, and their relevance to your respondents.
Likert scale questions collect ordinal data using rating scales with 5 or 7 points.
When you have four or more Likert-type questions, you can treat the composite data as quantitative data on an interval scale . Intelligence tests, psychological scales, and personality inventories use multiple Likert-type questions to collect interval data.
With interval or ratio scales , you can apply strong statistical hypothesis tests to address your research aims.
Pros and cons of closed-ended questions
Well-designed closed-ended questions are easy to understand and can be answered quickly. However, you might still miss important answers that are relevant to respondents. An incomplete set of response items may force some respondents to pick the closest alternative to their true answer. These types of questions may also miss out on valuable detail.
To solve these problems, you can make questions partially closed-ended, and include an open-ended option where respondents can fill in their own answer.
Open-ended questions
Open-ended, or long-form, questions allow respondents to give answers in their own words. Because there are no restrictions on their choices, respondents can answer in ways that researchers may not have otherwise considered. For example, respondents may want to answer “multiracial” for the question on race rather than selecting from a restricted list.
- How do you feel about open science?
- How would you describe your personality?
- In your opinion, what is the biggest obstacle for productivity in remote work?
Open-ended questions have a few downsides.
They require more time and effort from respondents, which may deter them from completing the questionnaire.
For researchers, understanding and summarizing responses to these questions can take a lot of time and resources. You’ll need to develop a systematic coding scheme to categorize answers, and you may also need to involve other researchers in data analysis for high reliability .
Question wording can influence your respondents’ answers, especially if the language is unclear, ambiguous, or biased. Good questions need to be understood by all respondents in the same way ( reliable ) and measure exactly what you’re interested in ( valid ).
Use clear language
You should design questions with your target audience in mind. Consider their familiarity with your questionnaire topics and language and tailor your questions to them.
For readability and clarity, avoid jargon or overly complex language. Don’t use double negatives because they can be harder to understand.
Use balanced framing
Respondents often answer in different ways depending on the question framing. Positive frames are interpreted as more neutral than negative frames and may encourage more socially desirable answers.
Positive frame | Negative frame |
---|---|
Should protests of pandemic-related restrictions be allowed? | Should protests of pandemic-related restrictions be forbidden? |
Use a mix of both positive and negative frames to avoid research bias , and ensure that your question wording is balanced wherever possible.
Unbalanced questions focus on only one side of an argument. Respondents may be less likely to oppose the question if it is framed in a particular direction. It’s best practice to provide a counter argument within the question as well.
Unbalanced | Balanced |
---|---|
Do you favor…? | Do you favor or oppose…? |
Do you agree that…? | Do you agree or disagree that…? |
Avoid leading questions
Leading questions guide respondents towards answering in specific ways, even if that’s not how they truly feel, by explicitly or implicitly providing them with extra information.
It’s best to keep your questions short and specific to your topic of interest.
- The average daily work commute in the US takes 54.2 minutes and costs $29 per day. Since 2020, working from home has saved many employees time and money. Do you favor flexible work-from-home policies even after it’s safe to return to offices?
- Experts agree that a well-balanced diet provides sufficient vitamins and minerals, and multivitamins and supplements are not necessary or effective. Do you agree or disagree that multivitamins are helpful for balanced nutrition?
Keep your questions focused
Ask about only one idea at a time and avoid double-barreled questions. Double-barreled questions ask about more than one item at a time, which can confuse respondents.
This question could be difficult to answer for respondents who feel strongly about the right to clean drinking water but not high-speed internet. They might only answer about the topic they feel passionate about or provide a neutral answer instead – but neither of these options capture their true answers.
Instead, you should ask two separate questions to gauge respondents’ opinions.
Strongly Agree Agree Undecided Disagree Strongly Disagree
Do you agree or disagree that the government should be responsible for providing high-speed internet to everyone?
You can organize the questions logically, with a clear progression from simple to complex. Alternatively, you can randomize the question order between respondents.
Logical flow
Using a logical flow to your question order means starting with simple questions, such as behavioral or opinion questions, and ending with more complex, sensitive, or controversial questions.
The question order that you use can significantly affect the responses by priming them in specific directions. Question order effects, or context effects, occur when earlier questions influence the responses to later questions, reducing the validity of your questionnaire.
While demographic questions are usually unaffected by order effects, questions about opinions and attitudes are more susceptible to them.
- How knowledgeable are you about Joe Biden’s executive orders in his first 100 days?
- Are you satisfied or dissatisfied with the way Joe Biden is managing the economy?
- Do you approve or disapprove of the way Joe Biden is handling his job as president?
It’s important to minimize order effects because they can be a source of systematic error or bias in your study.
Randomization
Randomization involves presenting individual respondents with the same questionnaire but with different question orders.
When you use randomization, order effects will be minimized in your dataset. But a randomized order may also make it harder for respondents to process your questionnaire. Some questions may need more cognitive effort, while others are easier to answer, so a random order could require more time or mental capacity for respondents to switch between questions.
Step 1: Define your goals and objectives
The first step of designing a questionnaire is determining your aims.
- What topics or experiences are you studying?
- What specifically do you want to find out?
- Is a self-report questionnaire an appropriate tool for investigating this topic?
Once you’ve specified your research aims, you can operationalize your variables of interest into questionnaire items. Operationalizing concepts means turning them from abstract ideas into concrete measurements. Every question needs to address a defined need and have a clear purpose.
Step 2: Use questions that are suitable for your sample
Create appropriate questions by taking the perspective of your respondents. Consider their language proficiency and available time and energy when designing your questionnaire.
- Are the respondents familiar with the language and terms used in your questions?
- Would any of the questions insult, confuse, or embarrass them?
- Do the response items for any closed-ended questions capture all possible answers?
- Are the response items mutually exclusive?
- Do the respondents have time to respond to open-ended questions?
Consider all possible options for responses to closed-ended questions. From a respondent’s perspective, a lack of response options reflecting their point of view or true answer may make them feel alienated or excluded. In turn, they’ll become disengaged or inattentive to the rest of the questionnaire.
Step 3: Decide on your questionnaire length and question order
Once you have your questions, make sure that the length and order of your questions are appropriate for your sample.
If respondents are not being incentivized or compensated, keep your questionnaire short and easy to answer. Otherwise, your sample may be biased with only highly motivated respondents completing the questionnaire.
Decide on your question order based on your aims and resources. Use a logical flow if your respondents have limited time or if you cannot randomize questions. Randomizing questions helps you avoid bias, but it can take more complex statistical analysis to interpret your data.
Step 4: Pretest your questionnaire
When you have a complete list of questions, you’ll need to pretest it to make sure what you’re asking is always clear and unambiguous. Pretesting helps you catch any errors or points of confusion before performing your study.
Ask friends, classmates, or members of your target audience to complete your questionnaire using the same method you’ll use for your research. Find out if any questions were particularly difficult to answer or if the directions were unclear or inconsistent, and make changes as necessary.
If you have the resources, running a pilot study will help you test the validity and reliability of your questionnaire. A pilot study is a practice run of the full study, and it includes sampling, data collection , and analysis. You can find out whether your procedures are unfeasible or susceptible to bias and make changes in time, but you can’t test a hypothesis with this type of study because it’s usually statistically underpowered .
If you want to know more about statistics , methodology , or research bias , make sure to check out some of our other articles with explanations and examples.
- Student’s t -distribution
- Normal distribution
- Null and Alternative Hypotheses
- Chi square tests
- Confidence interval
- Quartiles & Quantiles
- Cluster sampling
- Stratified sampling
- Data cleansing
- Reproducibility vs Replicability
- Peer review
- Prospective cohort study
Research bias
- Implicit bias
- Cognitive bias
- Placebo effect
- Hawthorne effect
- Hindsight bias
- Affect heuristic
- Social desirability bias
A questionnaire is a data collection tool or instrument, while a survey is an overarching research method that involves collecting and analyzing data from people using questionnaires.
Closed-ended, or restricted-choice, questions offer respondents a fixed set of choices to select from. These questions are easier to answer quickly.
Open-ended or long-form questions allow respondents to answer in their own words. Because there are no restrictions on their choices, respondents can answer in ways that researchers may not have otherwise considered.
A Likert scale is a rating scale that quantitatively assesses opinions, attitudes, or behaviors. It is made up of 4 or more questions that measure a single attitude or trait when response scores are combined.
To use a Likert scale in a survey , you present participants with Likert-type questions or statements, and a continuum of items, usually with 5 or 7 possible responses, to capture their degree of agreement.
You can organize the questions logically, with a clear progression from simple to complex, or randomly between respondents. A logical flow helps respondents process the questionnaire easier and quicker, but it may lead to bias. Randomization can minimize the bias from order effects.
Questionnaires can be self-administered or researcher-administered.
Researcher-administered questionnaires are interviews that take place by phone, in-person, or online between researchers and respondents. You can gain deeper insights by clarifying questions for respondents or asking follow-up questions.
Cite this Scribbr article
If you want to cite this source, you can copy and paste the citation or click the “Cite this Scribbr article” button to automatically add the citation to our free Citation Generator.
Bhandari, P. (2023, June 22). Questionnaire Design | Methods, Question Types & Examples. Scribbr. Retrieved August 29, 2024, from https://www.scribbr.com/methodology/questionnaire/
Is this article helpful?
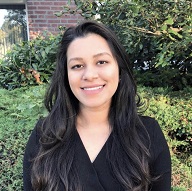
Pritha Bhandari
Other students also liked, survey research | definition, examples & methods, what is a likert scale | guide & examples, reliability vs. validity in research | difference, types and examples, "i thought ai proofreading was useless but..".
I've been using Scribbr for years now and I know it's a service that won't disappoint. It does a good job spotting mistakes”
Recommended pages
- New staff intranet
- Student Welcome
- Online registration
- MyUoB mobile app
- Campus maps
- Lecture timetables
- Study spaces
- Student digital services
- Student support
- Student printing
- Car parking
- Room bookings
- Core systems
- Staff development
Data management plans
What is a data management plan.
A data management plan (DMP), sometimes also referred to as Technical Plan or Data Access Plan, is a document that describes how data will be collected, organised, managed, stored, secured, backed-up, preserved, and where applicable, shared. It is an important tool that facilitates and supports the creation of FAIR data .
The DMP is intended to be a living document which is updated as the project progresses.
Warning: we have been made aware that UKRI may be changing the format required for data management plans in new applications. DMPOnline are liaising with UKRI to ensure templates are updated, but in the meantime, please check the requirements on your application.
What exactly is “research data”?
Following the definition provided in the University's Research Data Management Policy , “Research data are the evidence that underpins the answer to the research question, and can be used to validate findings regardless of its form.” Thus, data covers quantitative and qualitative statements, raw data from measurements and derived data – either cleaned or extracted from your primary dataset or derived from an existing source.
Do I need to write a data management plan?
If your research uses or creates data, yes. The University of Birmingham’s Research Data management Policy states that
“3.4 All funded research must be supported by a DMP or protocol that explicitly addresses data capture, management, integrity, confidentiality, retention, ownership, sharing and publication. This may be either a DMP submitted to the research funder as part of a research application or a document developed via the University’s DMP system after the project receives funding. Unfunded research which is likely to generate Research Data should also be supported by a DMP.”
If you are funded, there might be additional requirements that you need to meet. The main funder requirements are summarised in the data policy section .
How do I write a data management plan?
We recommend you to use the DMPOnline tool to create your data management plan, as it provides you with a variety of templates tailored to funder requirements as well as a basic template if you are unfunded. Guidance on using DMPOnline is available in our Introduction to using DMPonline video (duration 7:11), and the guide to creating a DMP using DMPOnline .
If you are creating a DMP to support a research grant application, you should check whether the funder requires the use of a specific template. Your College Research Support Office can advise.
For PGR students and researchers where no funder requirement applies, the University provides a standard template. You can access it via DMPonline or download a Word version (DOCX file - 64KB) .
Examples of data management plans
The RIO journal allows you to formally publish your data management plan. See their examples of published DMPs.
The Digital Curation Centre provides a collection of example DMPs and guidance .
DMP Review Service
The Scholarly Communications Team has recently launched a Data Management Plan Review Service for Research Staff . We will review drafted data management plans before grant applications submissions and provide feedback.
Please allow at least two weeks for our review.
If you would like us to review your data management plan (DMP), email us a draft of your plan to: [email protected] . Also, make sure that you have included the name of the Principal Investigator, a summary of the project and the associated Funder.
DMPs for PGRs
- College of Arts and Law
- College of Engineering and Physical Sciences
- College of Life and Environmental Sciences
- College of Medicine and Health
- College of Social Sciences
Professional Services
- Academic Services
- Campus Services
- Development and Alumni Relations
- Executive Support
- External Relations
- Human Resources
- IT Services
- Legal Services
- Research Strategy and Services
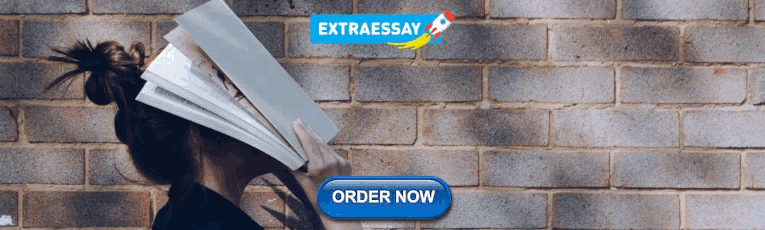
IMAGES
VIDEO
COMMENTS
Step 2: Choose your data collection method. Based on the data you want to collect, decide which method is best suited for your research. Experimental research is primarily a quantitative method. Interviews, focus groups, and ethnographies are qualitative methods. Surveys, observations, archival research, and secondary data collection can be ...
Data collection is the process of gathering and collecting information from various sources to analyze and make informed decisions based on the data collected. This can involve various methods, such as surveys, interviews, experiments, and observation. In order for data collection to be effective, it is important to have a clear understanding ...
1. Decide what data you want to gather. Have a clear understanding of the questions you are asking, and then consider where the answers might lie and how you might obtain them. This saves time and resources by avoiding the collection of irrelevant data, and helps maintain the quality of your datasets. 2.
PDF | Learn how to choose the best data collection methods and tools for your research project, with examples and tips from ResearchGate experts. | Download and read the full-text PDF.
Steps for writing an effective data collection plan. With the theory out of the way, let's see how to write a proper data collection plan, step by step. 1. Define objectives and research questions. Write down a statement of purpose that explains what you intend to discover, decide, or achieve.
Now you need to plan your data collection procedures, especially if you're doing quantitative research! This video will walk you through some key steps for g...
Data collection is the process of gathering and measuring information used for research. Collecting data is one of the most important steps in the research process, and is part of all disciplines including physical and social sciences, humanities, business, etc. Data comes in many forms with different ways to store and record data, either written in a lab notebook and or recorded digitally on ...
Put simply, data collection is the process of gathering information for a specific purpose. It can be used to answer research questions, make informed business decisions, or improve products and services. To collect data, we must first identify what information we need and how we will collect it.
We offer best-practice recommendations for journal reviewers, editors, and authors regarding data collection and preparation. Our recommendations are applicable to research adopting different epistemological and ontological perspectives—including both quantitative and qualitative approaches—as well as research addressing micro (i.e., individuals, teams) and macro (i.e., organizations ...
A clear data collection plan at the proposal stage can alleviate stress and ensure that future researchers can replicate your study. Additionally, a clear data collection plan will help ensure that you obtain the information you need to answer your research questions. Below are some suggestions for creating a solid data collection plan.
It's time to choose your data collection methods. You can choose just one data collection method, or use several methods in the same study. This video will i...
Report all of the procedures applied for administering the study, processing the data, and for planned data analyses. Data collection methods and research design. Data collection methods refers to the general mode of the instruments: surveys, interviews, observations, focus groups, neuroimaging, cognitive tests, and so on. Summarize exactly how ...
Data collection is a crucial stage in any research study, enabling researchers to gather information essential for answering research questions, testing hypotheses, and achieving study objectives.
Data Collection, Research Methodology, Data Collection Methods, Academic Research Paper, Data Collection Techniques. I. INTRODUCTION Different methods for gathering information regarding specific variables of the study aiming to employ them in the data analysis phase to achieve the results of the study, gain the answer of the research ...
Data Collection | Definition, Methods & Examples. Published on June 5, 2020 by Pritha Bhandari.Revised on June 21, 2023. Data collection is a systematic process of gathering observations or measurements. Whether you are performing research for business, governmental or academic purposes, data collection allows you to gain first-hand knowledge and original insights into your research problem.
Summary: This blog provides a comprehensive overview of data collection, covering its definition, importance, methods, and types of data.It also discusses tools and techniques for effective data collection, emphasising quality assurance and control. Understand how to gather accurate and reliable data for informed decision-making and research integrity.
Qualitative Research Methodology. This is a research methodology that involves the collection and analysis of non-numerical data such as words, images, and observations. This type of research is often used to explore complex phenomena, to gain an in-depth understanding of a particular topic, and to generate hypotheses.
4. Provide a summary of the literature relating to the topic and what gaps there may be. Rationale for study. 5. Identify the rationale for the study. The rationale for the use of qualitative methods can be noted here or in the methods section. Objective. 6. Clearly articulate the objective of the study.
In this Rip Out we focus on data collection, but in qualitative research, the entire project must be considered. 1, 2 Careful design of the data collection phase requires the following: deciding who will do what, where, when, and how at the different stages of the research process; acknowledging the role of the researcher as an instrument of ...
It is crucial to have knowledge of both quantitative and qualitative research2 as both types of research involve writing research questions and hypotheses.7 However, these crucial elements of research are sometimes overlooked; ... - This involves data collection from study participants or the literature regarding a phenomenon of interest, using ...
Step 1: Consider your aims and approach. Step 2: Choose a type of research design. Step 3: Identify your population and sampling method. Step 4: Choose your data collection methods. Step 5: Plan your data collection procedures. Step 6: Decide on your data analysis strategies. Other interesting articles.
Data Collection Methods. Data collection is a process of collecting information from all the relevant sources to find answers to the research problem, test the hypothesis (if you are following deductive approach) and evaluate the outcomes. Data collection methods can be divided into two categories: secondary methods of data collection and ...
Qualitative data collection is vital in qualitative research. It helps researchers understand individuals' attitudes, beliefs, and behaviors in a specific context. Several methods are used to collect qualitative data, including interviews, surveys, focus groups, and observations. Understanding the various methods used for gathering ...
sources that already exist. This would further be detailed below.3.3.1 Questionnaire A. questionnaire will be used to obtain primary data from participants at the institution. In so doing, the researcher w. ll be able to evaluate the current level of brand equity the institution (objective 2). In order for the researcher to collect data to ...
Once you have determined your sampling method and sample size, you need to decide on your data collection method. The most common data collection methods include online surveys, telephone surveys, face-to-face surveys, and mail surveys. Each method has its advantages and disadvantages, and you should choose the one that best suits your survey ...
Questionnaires vs. surveys. A survey is a research method where you collect and analyze data from a group of people. A questionnaire is a specific tool or instrument for collecting the data.. Designing a questionnaire means creating valid and reliable questions that address your research objectives, placing them in a useful order, and selecting an appropriate method for administration.
What exactly is "research data"? Following the definition provided in the University's Research Data Management Policy, "Research data are the evidence that underpins the answer to the research question, and can be used to validate findings regardless of its form." Thus, data covers quantitative and qualitative statements, raw data from ...
In the context of prisons research, letter writing serves as a unique way to access prison participants without gaining formal His Majesty's Prison and Probation Service (HMMPS) approval and does not place any demands on the time and resources of prison staff in comparison to in-person data collection methods such as interviews (Burtt, 2020).