- Privacy Policy

Home » Case Study – Methods, Examples and Guide
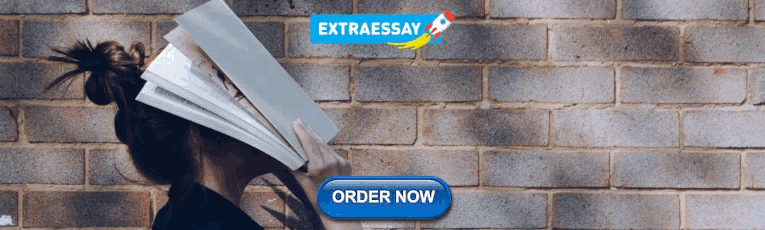
Case Study – Methods, Examples and Guide
Table of Contents

A case study is a research method that involves an in-depth examination and analysis of a particular phenomenon or case, such as an individual, organization, community, event, or situation.
It is a qualitative research approach that aims to provide a detailed and comprehensive understanding of the case being studied. Case studies typically involve multiple sources of data, including interviews, observations, documents, and artifacts, which are analyzed using various techniques, such as content analysis, thematic analysis, and grounded theory. The findings of a case study are often used to develop theories, inform policy or practice, or generate new research questions.
Types of Case Study
Types and Methods of Case Study are as follows:
Single-Case Study
A single-case study is an in-depth analysis of a single case. This type of case study is useful when the researcher wants to understand a specific phenomenon in detail.
For Example , A researcher might conduct a single-case study on a particular individual to understand their experiences with a particular health condition or a specific organization to explore their management practices. The researcher collects data from multiple sources, such as interviews, observations, and documents, and uses various techniques to analyze the data, such as content analysis or thematic analysis. The findings of a single-case study are often used to generate new research questions, develop theories, or inform policy or practice.
Multiple-Case Study
A multiple-case study involves the analysis of several cases that are similar in nature. This type of case study is useful when the researcher wants to identify similarities and differences between the cases.
For Example, a researcher might conduct a multiple-case study on several companies to explore the factors that contribute to their success or failure. The researcher collects data from each case, compares and contrasts the findings, and uses various techniques to analyze the data, such as comparative analysis or pattern-matching. The findings of a multiple-case study can be used to develop theories, inform policy or practice, or generate new research questions.
Exploratory Case Study
An exploratory case study is used to explore a new or understudied phenomenon. This type of case study is useful when the researcher wants to generate hypotheses or theories about the phenomenon.
For Example, a researcher might conduct an exploratory case study on a new technology to understand its potential impact on society. The researcher collects data from multiple sources, such as interviews, observations, and documents, and uses various techniques to analyze the data, such as grounded theory or content analysis. The findings of an exploratory case study can be used to generate new research questions, develop theories, or inform policy or practice.
Descriptive Case Study
A descriptive case study is used to describe a particular phenomenon in detail. This type of case study is useful when the researcher wants to provide a comprehensive account of the phenomenon.
For Example, a researcher might conduct a descriptive case study on a particular community to understand its social and economic characteristics. The researcher collects data from multiple sources, such as interviews, observations, and documents, and uses various techniques to analyze the data, such as content analysis or thematic analysis. The findings of a descriptive case study can be used to inform policy or practice or generate new research questions.
Instrumental Case Study
An instrumental case study is used to understand a particular phenomenon that is instrumental in achieving a particular goal. This type of case study is useful when the researcher wants to understand the role of the phenomenon in achieving the goal.
For Example, a researcher might conduct an instrumental case study on a particular policy to understand its impact on achieving a particular goal, such as reducing poverty. The researcher collects data from multiple sources, such as interviews, observations, and documents, and uses various techniques to analyze the data, such as content analysis or thematic analysis. The findings of an instrumental case study can be used to inform policy or practice or generate new research questions.
Case Study Data Collection Methods
Here are some common data collection methods for case studies:
Interviews involve asking questions to individuals who have knowledge or experience relevant to the case study. Interviews can be structured (where the same questions are asked to all participants) or unstructured (where the interviewer follows up on the responses with further questions). Interviews can be conducted in person, over the phone, or through video conferencing.
Observations
Observations involve watching and recording the behavior and activities of individuals or groups relevant to the case study. Observations can be participant (where the researcher actively participates in the activities) or non-participant (where the researcher observes from a distance). Observations can be recorded using notes, audio or video recordings, or photographs.
Documents can be used as a source of information for case studies. Documents can include reports, memos, emails, letters, and other written materials related to the case study. Documents can be collected from the case study participants or from public sources.
Surveys involve asking a set of questions to a sample of individuals relevant to the case study. Surveys can be administered in person, over the phone, through mail or email, or online. Surveys can be used to gather information on attitudes, opinions, or behaviors related to the case study.
Artifacts are physical objects relevant to the case study. Artifacts can include tools, equipment, products, or other objects that provide insights into the case study phenomenon.
How to conduct Case Study Research
Conducting a case study research involves several steps that need to be followed to ensure the quality and rigor of the study. Here are the steps to conduct case study research:
- Define the research questions: The first step in conducting a case study research is to define the research questions. The research questions should be specific, measurable, and relevant to the case study phenomenon under investigation.
- Select the case: The next step is to select the case or cases to be studied. The case should be relevant to the research questions and should provide rich and diverse data that can be used to answer the research questions.
- Collect data: Data can be collected using various methods, such as interviews, observations, documents, surveys, and artifacts. The data collection method should be selected based on the research questions and the nature of the case study phenomenon.
- Analyze the data: The data collected from the case study should be analyzed using various techniques, such as content analysis, thematic analysis, or grounded theory. The analysis should be guided by the research questions and should aim to provide insights and conclusions relevant to the research questions.
- Draw conclusions: The conclusions drawn from the case study should be based on the data analysis and should be relevant to the research questions. The conclusions should be supported by evidence and should be clearly stated.
- Validate the findings: The findings of the case study should be validated by reviewing the data and the analysis with participants or other experts in the field. This helps to ensure the validity and reliability of the findings.
- Write the report: The final step is to write the report of the case study research. The report should provide a clear description of the case study phenomenon, the research questions, the data collection methods, the data analysis, the findings, and the conclusions. The report should be written in a clear and concise manner and should follow the guidelines for academic writing.
Examples of Case Study
Here are some examples of case study research:
- The Hawthorne Studies : Conducted between 1924 and 1932, the Hawthorne Studies were a series of case studies conducted by Elton Mayo and his colleagues to examine the impact of work environment on employee productivity. The studies were conducted at the Hawthorne Works plant of the Western Electric Company in Chicago and included interviews, observations, and experiments.
- The Stanford Prison Experiment: Conducted in 1971, the Stanford Prison Experiment was a case study conducted by Philip Zimbardo to examine the psychological effects of power and authority. The study involved simulating a prison environment and assigning participants to the role of guards or prisoners. The study was controversial due to the ethical issues it raised.
- The Challenger Disaster: The Challenger Disaster was a case study conducted to examine the causes of the Space Shuttle Challenger explosion in 1986. The study included interviews, observations, and analysis of data to identify the technical, organizational, and cultural factors that contributed to the disaster.
- The Enron Scandal: The Enron Scandal was a case study conducted to examine the causes of the Enron Corporation’s bankruptcy in 2001. The study included interviews, analysis of financial data, and review of documents to identify the accounting practices, corporate culture, and ethical issues that led to the company’s downfall.
- The Fukushima Nuclear Disaster : The Fukushima Nuclear Disaster was a case study conducted to examine the causes of the nuclear accident that occurred at the Fukushima Daiichi Nuclear Power Plant in Japan in 2011. The study included interviews, analysis of data, and review of documents to identify the technical, organizational, and cultural factors that contributed to the disaster.
Application of Case Study
Case studies have a wide range of applications across various fields and industries. Here are some examples:
Business and Management
Case studies are widely used in business and management to examine real-life situations and develop problem-solving skills. Case studies can help students and professionals to develop a deep understanding of business concepts, theories, and best practices.
Case studies are used in healthcare to examine patient care, treatment options, and outcomes. Case studies can help healthcare professionals to develop critical thinking skills, diagnose complex medical conditions, and develop effective treatment plans.
Case studies are used in education to examine teaching and learning practices. Case studies can help educators to develop effective teaching strategies, evaluate student progress, and identify areas for improvement.
Social Sciences
Case studies are widely used in social sciences to examine human behavior, social phenomena, and cultural practices. Case studies can help researchers to develop theories, test hypotheses, and gain insights into complex social issues.
Law and Ethics
Case studies are used in law and ethics to examine legal and ethical dilemmas. Case studies can help lawyers, policymakers, and ethical professionals to develop critical thinking skills, analyze complex cases, and make informed decisions.
Purpose of Case Study
The purpose of a case study is to provide a detailed analysis of a specific phenomenon, issue, or problem in its real-life context. A case study is a qualitative research method that involves the in-depth exploration and analysis of a particular case, which can be an individual, group, organization, event, or community.
The primary purpose of a case study is to generate a comprehensive and nuanced understanding of the case, including its history, context, and dynamics. Case studies can help researchers to identify and examine the underlying factors, processes, and mechanisms that contribute to the case and its outcomes. This can help to develop a more accurate and detailed understanding of the case, which can inform future research, practice, or policy.
Case studies can also serve other purposes, including:
- Illustrating a theory or concept: Case studies can be used to illustrate and explain theoretical concepts and frameworks, providing concrete examples of how they can be applied in real-life situations.
- Developing hypotheses: Case studies can help to generate hypotheses about the causal relationships between different factors and outcomes, which can be tested through further research.
- Providing insight into complex issues: Case studies can provide insights into complex and multifaceted issues, which may be difficult to understand through other research methods.
- Informing practice or policy: Case studies can be used to inform practice or policy by identifying best practices, lessons learned, or areas for improvement.
Advantages of Case Study Research
There are several advantages of case study research, including:
- In-depth exploration: Case study research allows for a detailed exploration and analysis of a specific phenomenon, issue, or problem in its real-life context. This can provide a comprehensive understanding of the case and its dynamics, which may not be possible through other research methods.
- Rich data: Case study research can generate rich and detailed data, including qualitative data such as interviews, observations, and documents. This can provide a nuanced understanding of the case and its complexity.
- Holistic perspective: Case study research allows for a holistic perspective of the case, taking into account the various factors, processes, and mechanisms that contribute to the case and its outcomes. This can help to develop a more accurate and comprehensive understanding of the case.
- Theory development: Case study research can help to develop and refine theories and concepts by providing empirical evidence and concrete examples of how they can be applied in real-life situations.
- Practical application: Case study research can inform practice or policy by identifying best practices, lessons learned, or areas for improvement.
- Contextualization: Case study research takes into account the specific context in which the case is situated, which can help to understand how the case is influenced by the social, cultural, and historical factors of its environment.
Limitations of Case Study Research
There are several limitations of case study research, including:
- Limited generalizability : Case studies are typically focused on a single case or a small number of cases, which limits the generalizability of the findings. The unique characteristics of the case may not be applicable to other contexts or populations, which may limit the external validity of the research.
- Biased sampling: Case studies may rely on purposive or convenience sampling, which can introduce bias into the sample selection process. This may limit the representativeness of the sample and the generalizability of the findings.
- Subjectivity: Case studies rely on the interpretation of the researcher, which can introduce subjectivity into the analysis. The researcher’s own biases, assumptions, and perspectives may influence the findings, which may limit the objectivity of the research.
- Limited control: Case studies are typically conducted in naturalistic settings, which limits the control that the researcher has over the environment and the variables being studied. This may limit the ability to establish causal relationships between variables.
- Time-consuming: Case studies can be time-consuming to conduct, as they typically involve a detailed exploration and analysis of a specific case. This may limit the feasibility of conducting multiple case studies or conducting case studies in a timely manner.
- Resource-intensive: Case studies may require significant resources, including time, funding, and expertise. This may limit the ability of researchers to conduct case studies in resource-constrained settings.
About the author
Muhammad Hassan
Researcher, Academic Writer, Web developer
You may also like

Basic Research – Types, Methods and Examples

Questionnaire – Definition, Types, and Examples

Observational Research – Methods and Guide

Descriptive Research Design – Types, Methods and...

Mixed Methods Research – Types & Analysis

Experimental Design – Types, Methods, Guide
Have a language expert improve your writing
Run a free plagiarism check in 10 minutes, generate accurate citations for free.
- Knowledge Base
Methodology
- What Is a Case Study? | Definition, Examples & Methods
What Is a Case Study? | Definition, Examples & Methods
Published on May 8, 2019 by Shona McCombes . Revised on November 20, 2023.
A case study is a detailed study of a specific subject, such as a person, group, place, event, organization, or phenomenon. Case studies are commonly used in social, educational, clinical, and business research.
A case study research design usually involves qualitative methods , but quantitative methods are sometimes also used. Case studies are good for describing , comparing, evaluating and understanding different aspects of a research problem .
Table of contents
When to do a case study, step 1: select a case, step 2: build a theoretical framework, step 3: collect your data, step 4: describe and analyze the case, other interesting articles.
A case study is an appropriate research design when you want to gain concrete, contextual, in-depth knowledge about a specific real-world subject. It allows you to explore the key characteristics, meanings, and implications of the case.
Case studies are often a good choice in a thesis or dissertation . They keep your project focused and manageable when you don’t have the time or resources to do large-scale research.
You might use just one complex case study where you explore a single subject in depth, or conduct multiple case studies to compare and illuminate different aspects of your research problem.
Research question | Case study |
---|---|
What are the ecological effects of wolf reintroduction? | Case study of wolf reintroduction in Yellowstone National Park |
How do populist politicians use narratives about history to gain support? | Case studies of Hungarian prime minister Viktor Orbán and US president Donald Trump |
How can teachers implement active learning strategies in mixed-level classrooms? | Case study of a local school that promotes active learning |
What are the main advantages and disadvantages of wind farms for rural communities? | Case studies of three rural wind farm development projects in different parts of the country |
How are viral marketing strategies changing the relationship between companies and consumers? | Case study of the iPhone X marketing campaign |
How do experiences of work in the gig economy differ by gender, race and age? | Case studies of Deliveroo and Uber drivers in London |
Here's why students love Scribbr's proofreading services
Discover proofreading & editing
Once you have developed your problem statement and research questions , you should be ready to choose the specific case that you want to focus on. A good case study should have the potential to:
- Provide new or unexpected insights into the subject
- Challenge or complicate existing assumptions and theories
- Propose practical courses of action to resolve a problem
- Open up new directions for future research
TipIf your research is more practical in nature and aims to simultaneously investigate an issue as you solve it, consider conducting action research instead.
Unlike quantitative or experimental research , a strong case study does not require a random or representative sample. In fact, case studies often deliberately focus on unusual, neglected, or outlying cases which may shed new light on the research problem.
Example of an outlying case studyIn the 1960s the town of Roseto, Pennsylvania was discovered to have extremely low rates of heart disease compared to the US average. It became an important case study for understanding previously neglected causes of heart disease.
However, you can also choose a more common or representative case to exemplify a particular category, experience or phenomenon.
Example of a representative case studyIn the 1920s, two sociologists used Muncie, Indiana as a case study of a typical American city that supposedly exemplified the changing culture of the US at the time.
While case studies focus more on concrete details than general theories, they should usually have some connection with theory in the field. This way the case study is not just an isolated description, but is integrated into existing knowledge about the topic. It might aim to:
- Exemplify a theory by showing how it explains the case under investigation
- Expand on a theory by uncovering new concepts and ideas that need to be incorporated
- Challenge a theory by exploring an outlier case that doesn’t fit with established assumptions
To ensure that your analysis of the case has a solid academic grounding, you should conduct a literature review of sources related to the topic and develop a theoretical framework . This means identifying key concepts and theories to guide your analysis and interpretation.
There are many different research methods you can use to collect data on your subject. Case studies tend to focus on qualitative data using methods such as interviews , observations , and analysis of primary and secondary sources (e.g., newspaper articles, photographs, official records). Sometimes a case study will also collect quantitative data.
Example of a mixed methods case studyFor a case study of a wind farm development in a rural area, you could collect quantitative data on employment rates and business revenue, collect qualitative data on local people’s perceptions and experiences, and analyze local and national media coverage of the development.
The aim is to gain as thorough an understanding as possible of the case and its context.
In writing up the case study, you need to bring together all the relevant aspects to give as complete a picture as possible of the subject.
How you report your findings depends on the type of research you are doing. Some case studies are structured like a standard scientific paper or thesis , with separate sections or chapters for the methods , results and discussion .
Others are written in a more narrative style, aiming to explore the case from various angles and analyze its meanings and implications (for example, by using textual analysis or discourse analysis ).
In all cases, though, make sure to give contextual details about the case, connect it back to the literature and theory, and discuss how it fits into wider patterns or debates.
If you want to know more about statistics , methodology , or research bias , make sure to check out some of our other articles with explanations and examples.
- Normal distribution
- Degrees of freedom
- Null hypothesis
- Discourse analysis
- Control groups
- Mixed methods research
- Non-probability sampling
- Quantitative research
- Ecological validity
Research bias
- Rosenthal effect
- Implicit bias
- Cognitive bias
- Selection bias
- Negativity bias
- Status quo bias
Cite this Scribbr article
If you want to cite this source, you can copy and paste the citation or click the “Cite this Scribbr article” button to automatically add the citation to our free Citation Generator.
McCombes, S. (2023, November 20). What Is a Case Study? | Definition, Examples & Methods. Scribbr. Retrieved August 26, 2024, from https://www.scribbr.com/methodology/case-study/
Is this article helpful?
Shona McCombes
Other students also liked, primary vs. secondary sources | difference & examples, what is a theoretical framework | guide to organizing, what is action research | definition & examples, get unlimited documents corrected.
✔ Free APA citation check included ✔ Unlimited document corrections ✔ Specialized in correcting academic texts
Educational resources and simple solutions for your research journey

What is a Case Study in Research? Definition, Methods, and Examples
Case study methodology offers researchers an exciting opportunity to explore intricate phenomena within specific contexts using a wide range of data sources and collection methods. It is highly pertinent in health and social sciences, environmental studies, social work, education, and business studies. Its diverse applications, such as advancing theory, program evaluation, and intervention development, make it an invaluable tool for driving meaningful research and fostering positive change.[ 1]
Table of Contents
What is a Case Study?
A case study method involves a detailed examination of a single subject, such as an individual, group, organization, event, or community, to explore and understand complex issues in real-life contexts. By focusing on one specific case, researchers can gain a deep understanding of the factors and dynamics at play, understanding their complex relationships, which might be missed in broader, more quantitative studies.
When to do a Case Study?
A case study design is useful when you want to explore a phenomenon in-depth and in its natural context. Here are some examples of when to use a case study :[ 2]
- Exploratory Research: When you want to explore a new topic or phenomenon, a case study can help you understand the subject deeply. For example , a researcher studying a newly discovered plant species might use a case study to document its characteristics and behavior.
- Descriptive Research: If you want to describe a complex phenomenon or process, a case study can provide a detailed and comprehensive description. For instance, a case study design could describe the experiences of a group of individuals living with a rare disease.
- Explanatory Research: When you want to understand why a particular phenomenon occurs, a case study can help you identify causal relationships. A case study design could investigate the reasons behind the success or failure of a particular business strategy.
- Theory Building: Case studies can also be used to develop or refine theories. By systematically analyzing a series of cases, researchers can identify patterns and relationships that can contribute to developing new theories or refining existing ones.
- Critical Instance: Sometimes, a single case can be used to study a rare or unusual phenomenon, but it is important for theoretical or practical reasons. For example , the case of Phineas Gage, a man who survived a severe brain injury, has been widely studied to understand the relationship between the brain and behavior.
- Comparative Analysis: Case studies can also compare different cases or contexts. A case study example involves comparing the implementation of a particular policy in different countries to understand its effectiveness and identifying best practices.

How to Create a Case Study – Step by Step
Step 1: select a case .
Careful case selection ensures relevance, insight, and meaningful contribution to existing knowledge in your field. Here’s how you can choose a case study design :[ 3]
- Define Your Objectives: Clarify the purpose of your case study and what you hope to achieve. Do you want to provide new insights, challenge existing theories, propose solutions to a problem, or explore new research directions?
- Consider Unusual or Outlying Cases: Focus on unusual, neglected, or outlying cases that can provide unique insights.
- Choose a Representative Case: Alternatively, select a common or representative case to exemplify a particular category, experience, or phenomenon.
- Avoid Bias: Ensure your selection process is unbiased using random or criteria-based selection.
- Be Clear and Specific: Clearly define the boundaries of your study design , including the scope, timeframe, and key stakeholders.
- Ethical Considerations: Consider ethical issues, such as confidentiality and informed consent.
Step 2: Build a Theoretical Framework
To ensure your case study has a solid academic foundation, it’s important to build a theoretical framework:
- Conduct a Literature Review: Identify key concepts and theories relevant to your case study .
- Establish Connections with Theory: Connect your case study with existing theories in the field.
- Guide Your Analysis and Interpretation: Use your theoretical framework to guide your analysis, ensuring your findings are grounded in established theories and concepts.
Step 3: Collect Your Data
To conduct a comprehensive case study , you can use various research methods. These include interviews, observations, primary and secondary sources analysis, surveys, and a mixed methods approach. The aim is to gather rich and diverse data to enable a detailed analysis of your case study .
Step 4: Describe and Analyze the Case
How you report your findings will depend on the type of research you’re conducting. Here are two approaches:
- Structured Approach: Follows a scientific paper format, making it easier for readers to follow your argument.
- Narrative Approach: A more exploratory style aiming to analyze meanings and implications.
Regardless of the approach you choose, it’s important to include the following elements in your case study :
- Contextual Details: Provide background information about the case, including relevant historical, cultural, and social factors that may have influenced the outcome.
- Literature and Theory: Connect your case study to existing literature and theory in the field. Discuss how your findings contribute to or challenge existing knowledge.
- Wider Patterns or Debates: Consider how your case study fits into wider patterns or debates within the field. Discuss any implications your findings may have for future research or practice.
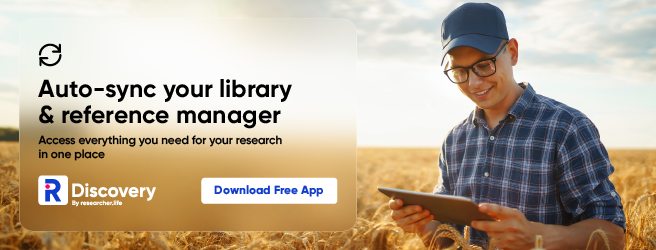
What Are the Benefits of a Case Study
Case studies offer a range of benefits , making them a powerful tool in research.
1. In-Depth Analysis
- Comprehensive Understanding: Case studies allow researchers to thoroughly explore a subject, understanding the complexities and nuances involved.
- Rich Data: They offer rich qualitative and sometimes quantitative data, capturing the intricacies of real-life contexts.
2. Contextual Insight
- Real-World Application: Case studies provide insights into real-world applications, making the findings highly relevant and practical.
- Context-Specific: They highlight how various factors interact within a specific context, offering a detailed picture of the situation.
3. Flexibility
- Methodological Diversity: Case studies can use various data collection methods, including interviews, observations, document analysis, and surveys.
- Adaptability: Researchers can adapt the case study approach to fit the specific needs and circumstances of the research.
4. Practical Solutions
- Actionable Insights: The detailed findings from case studies can inform practical solutions and recommendations for practitioners and policymakers.
- Problem-Solving: They help understand the root causes of problems and devise effective strategies to address them.
5. Unique Cases
- Rare Phenomena: Case studies are particularly valuable for studying rare or unique cases that other research methods may not capture.
- Detailed Documentation: They document and preserve detailed information about specific instances that might otherwise be overlooked.
What Are the Limitations of a Case Study
While case studies offer valuable insights and a detailed understanding of complex issues, they have several limitations .
1. Limited Generalizability
- Specific Context: Case studies often focus on a single case or a small number of cases, which may limit the generalization of findings to broader populations or different contexts.
- Unique Situations: The unique characteristics of the case may not be representative of other situations, reducing the applicability of the results.
2. Subjectivity
- Researcher Bias: The researcher’s perspectives and interpretations can influence the analysis and conclusions, potentially introducing bias.
- Participant Bias: Participants’ responses and behaviors may be influenced by their awareness of being studied, known as the Hawthorne effect.
3. Time-Consuming
- Data Collection and Analysis: Gathering detailed, in-depth data requires significant time and effort, making case studies more time-consuming than other research methods.
- Longitudinal Studies: If the case study observes changes over time, it can become even more prolonged.
4. Resource Intensive
- Financial and Human Resources: Conducting comprehensive case studies may require significant financial investment and human resources, including trained researchers and participant access.
- Access to Data: Accessing relevant and reliable data sources can be challenging, particularly in sensitive or proprietary contexts.
5. Replication Difficulties
- Unique Contexts: A case study’s specific and detailed context makes it difficult to replicate the study exactly, limiting the ability to validate findings through repetition.
- Variability: Differences in contexts, researchers, and methodologies can lead to variations in findings, complicating efforts to achieve consistent results.
By acknowledging and addressing these limitations , researchers can enhance the rigor and reliability of their case study findings.
Key Takeaways
Case studies are valuable in research because they provide an in-depth, contextual analysis of a single subject, event, or organization. They allow researchers to explore complex issues in real-world settings, capturing detailed qualitative and quantitative data. This method is useful for generating insights, developing theories, and offering practical solutions to problems. They are versatile, applicable in diverse fields such as business, education, and health, and can complement other research methods by providing rich, contextual evidence. However, their findings may have limited generalizability due to the focus on a specific case.
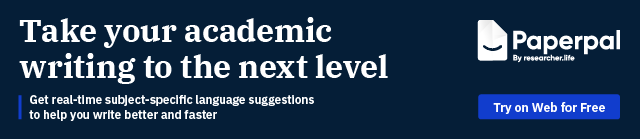
Frequently Asked Questions
Q: What is a case study in research?
A case study in research is an impactful tool for gaining a deep understanding of complex issues within their real-life context. It combines various data collection methods and provides rich, detailed insights that can inform theory development and practical applications.
Q: What are the advantages of using case studies in research?
Case studies are a powerful research method, offering advantages such as in-depth analysis, contextual insights, flexibility, rich data, and the ability to handle complex issues. They are particularly valuable for exploring new areas, generating hypotheses, and providing detailed, illustrative examples that can inform theory and practice.
Q: Can case studies be used in quantitative research?
While case studies are predominantly associated with qualitative research, they can effectively incorporate quantitative methods to provide a more comprehensive analysis. A mixed-methods approach leverages qualitative and quantitative research strengths, offering a powerful tool for exploring complex issues in a real-world context. For example , a new medical treatment case study can incorporate quantitative clinical outcomes (e.g., patient recovery rates and dosage levels) along with qualitative patient interviews.
Q: What are the key components of a case study?
A case study typically includes several key components:
- Introductio n, which provides an overview and sets the context by presenting the problem statement and research objectives;
- Literature review , which connects the study to existing theories and prior research;
- Methodology , which details the case study design , data collection methods, and analysis techniques;
- Findings , which present the data and results, including descriptions, patterns, and themes;
- Discussion and conclusion , which interpret the findings, discuss their implications, and offer conclusions, practical applications, limitations, and suggestions for future research.
Together, these components ensure a comprehensive, systematic, and insightful exploration of the case.
References
- de Vries, K. (2020). Case study methodology. In Critical qualitative health research (pp. 41-52). Routledge.
- Fidel, R. (1984). The case study method: A case study. Library and Information Science Research , 6 (3), 273-288.
- Thomas, G. (2021). How to do your case study. How to do your case study , 1-320.
Editage All Access is a subscription-based platform that unifies the best AI tools and services designed to speed up, simplify, and streamline every step of a researcher’s journey. The Editage All Access Pack is a one-of-a-kind subscription that unlocks full access to an AI writing assistant, literature recommender, journal finder, scientific illustration tool, and exclusive discounts on professional publication services from Editage.
Based on 22+ years of experience in academia, Editage All Access empowers researchers to put their best research forward and move closer to success. Explore our top AI Tools pack, AI Tools + Publication Services pack, or Build Your Own Plan. Find everything a researcher needs to succeed, all in one place – Get All Access now starting at just $14 a month !
Related Posts

What are the Best Research Funding Sources

Inductive vs. Deductive Research Approach

The Ultimate Guide to Qualitative Research - Part 1: The Basics
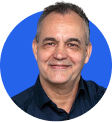
- Introduction and overview
- What is qualitative research?
- What is qualitative data?
- Examples of qualitative data
- Qualitative vs. quantitative research
- Mixed methods
- Qualitative research preparation
- Theoretical perspective
- Theoretical framework
- Literature reviews
Research question
- Conceptual framework
- Conceptual vs. theoretical framework
Data collection
- Qualitative research methods
- Focus groups
- Observational research
What is a case study?
Applications for case study research, what is a good case study, process of case study design, benefits and limitations of case studies.
- Ethnographical research
- Ethical considerations
- Confidentiality and privacy
- Power dynamics
- Reflexivity
Case studies
Case studies are essential to qualitative research , offering a lens through which researchers can investigate complex phenomena within their real-life contexts. This chapter explores the concept, purpose, applications, examples, and types of case studies and provides guidance on how to conduct case study research effectively.
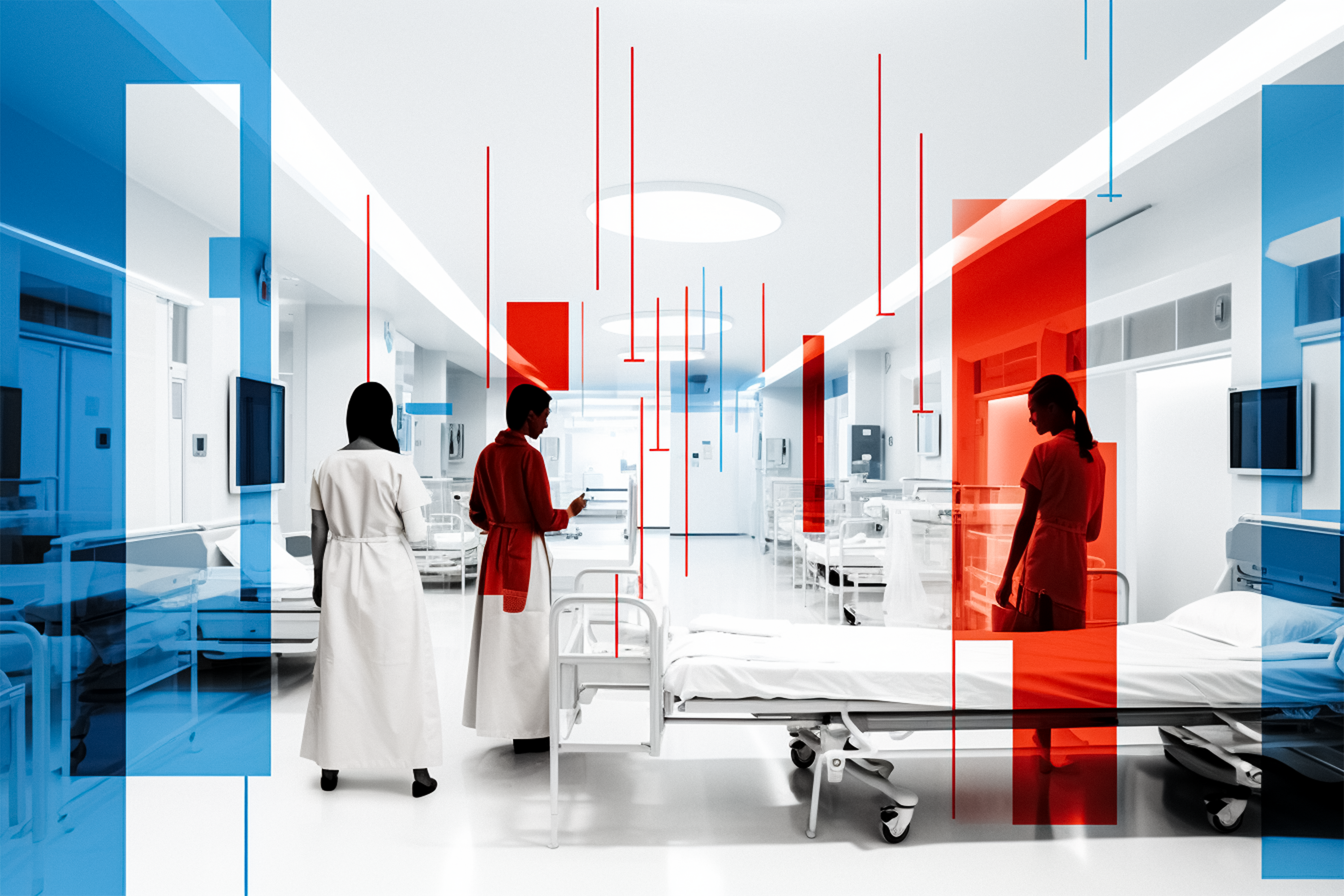
Whereas quantitative methods look at phenomena at scale, case study research looks at a concept or phenomenon in considerable detail. While analyzing a single case can help understand one perspective regarding the object of research inquiry, analyzing multiple cases can help obtain a more holistic sense of the topic or issue. Let's provide a basic definition of a case study, then explore its characteristics and role in the qualitative research process.
Definition of a case study
A case study in qualitative research is a strategy of inquiry that involves an in-depth investigation of a phenomenon within its real-world context. It provides researchers with the opportunity to acquire an in-depth understanding of intricate details that might not be as apparent or accessible through other methods of research. The specific case or cases being studied can be a single person, group, or organization – demarcating what constitutes a relevant case worth studying depends on the researcher and their research question .
Among qualitative research methods , a case study relies on multiple sources of evidence, such as documents, artifacts, interviews , or observations , to present a complete and nuanced understanding of the phenomenon under investigation. The objective is to illuminate the readers' understanding of the phenomenon beyond its abstract statistical or theoretical explanations.
Characteristics of case studies
Case studies typically possess a number of distinct characteristics that set them apart from other research methods. These characteristics include a focus on holistic description and explanation, flexibility in the design and data collection methods, reliance on multiple sources of evidence, and emphasis on the context in which the phenomenon occurs.
Furthermore, case studies can often involve a longitudinal examination of the case, meaning they study the case over a period of time. These characteristics allow case studies to yield comprehensive, in-depth, and richly contextualized insights about the phenomenon of interest.
The role of case studies in research
Case studies hold a unique position in the broader landscape of research methods aimed at theory development. They are instrumental when the primary research interest is to gain an intensive, detailed understanding of a phenomenon in its real-life context.
In addition, case studies can serve different purposes within research - they can be used for exploratory, descriptive, or explanatory purposes, depending on the research question and objectives. This flexibility and depth make case studies a valuable tool in the toolkit of qualitative researchers.
Remember, a well-conducted case study can offer a rich, insightful contribution to both academic and practical knowledge through theory development or theory verification, thus enhancing our understanding of complex phenomena in their real-world contexts.
What is the purpose of a case study?
Case study research aims for a more comprehensive understanding of phenomena, requiring various research methods to gather information for qualitative analysis . Ultimately, a case study can allow the researcher to gain insight into a particular object of inquiry and develop a theoretical framework relevant to the research inquiry.
Why use case studies in qualitative research?
Using case studies as a research strategy depends mainly on the nature of the research question and the researcher's access to the data.
Conducting case study research provides a level of detail and contextual richness that other research methods might not offer. They are beneficial when there's a need to understand complex social phenomena within their natural contexts.
The explanatory, exploratory, and descriptive roles of case studies
Case studies can take on various roles depending on the research objectives. They can be exploratory when the research aims to discover new phenomena or define new research questions; they are descriptive when the objective is to depict a phenomenon within its context in a detailed manner; and they can be explanatory if the goal is to understand specific relationships within the studied context. Thus, the versatility of case studies allows researchers to approach their topic from different angles, offering multiple ways to uncover and interpret the data .
The impact of case studies on knowledge development
Case studies play a significant role in knowledge development across various disciplines. Analysis of cases provides an avenue for researchers to explore phenomena within their context based on the collected data.
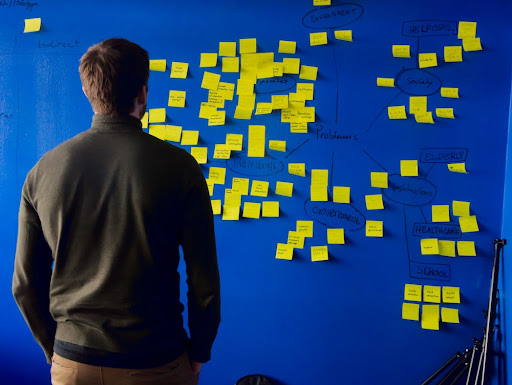
This can result in the production of rich, practical insights that can be instrumental in both theory-building and practice. Case studies allow researchers to delve into the intricacies and complexities of real-life situations, uncovering insights that might otherwise remain hidden.
Types of case studies
In qualitative research , a case study is not a one-size-fits-all approach. Depending on the nature of the research question and the specific objectives of the study, researchers might choose to use different types of case studies. These types differ in their focus, methodology, and the level of detail they provide about the phenomenon under investigation.
Understanding these types is crucial for selecting the most appropriate approach for your research project and effectively achieving your research goals. Let's briefly look at the main types of case studies.
Exploratory case studies
Exploratory case studies are typically conducted to develop a theory or framework around an understudied phenomenon. They can also serve as a precursor to a larger-scale research project. Exploratory case studies are useful when a researcher wants to identify the key issues or questions which can spur more extensive study or be used to develop propositions for further research. These case studies are characterized by flexibility, allowing researchers to explore various aspects of a phenomenon as they emerge, which can also form the foundation for subsequent studies.
Descriptive case studies
Descriptive case studies aim to provide a complete and accurate representation of a phenomenon or event within its context. These case studies are often based on an established theoretical framework, which guides how data is collected and analyzed. The researcher is concerned with describing the phenomenon in detail, as it occurs naturally, without trying to influence or manipulate it.
Explanatory case studies
Explanatory case studies are focused on explanation - they seek to clarify how or why certain phenomena occur. Often used in complex, real-life situations, they can be particularly valuable in clarifying causal relationships among concepts and understanding the interplay between different factors within a specific context.
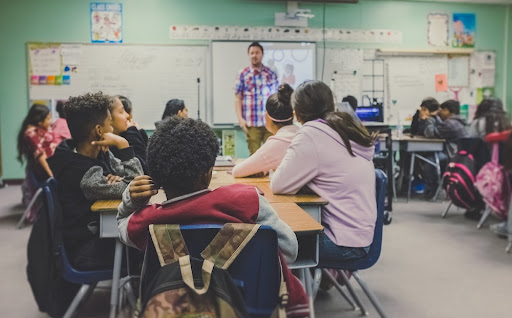
Intrinsic, instrumental, and collective case studies
These three categories of case studies focus on the nature and purpose of the study. An intrinsic case study is conducted when a researcher has an inherent interest in the case itself. Instrumental case studies are employed when the case is used to provide insight into a particular issue or phenomenon. A collective case study, on the other hand, involves studying multiple cases simultaneously to investigate some general phenomena.
Each type of case study serves a different purpose and has its own strengths and challenges. The selection of the type should be guided by the research question and objectives, as well as the context and constraints of the research.
The flexibility, depth, and contextual richness offered by case studies make this approach an excellent research method for various fields of study. They enable researchers to investigate real-world phenomena within their specific contexts, capturing nuances that other research methods might miss. Across numerous fields, case studies provide valuable insights into complex issues.
Critical information systems research
Case studies provide a detailed understanding of the role and impact of information systems in different contexts. They offer a platform to explore how information systems are designed, implemented, and used and how they interact with various social, economic, and political factors. Case studies in this field often focus on examining the intricate relationship between technology, organizational processes, and user behavior, helping to uncover insights that can inform better system design and implementation.
Health research
Health research is another field where case studies are highly valuable. They offer a way to explore patient experiences, healthcare delivery processes, and the impact of various interventions in a real-world context.
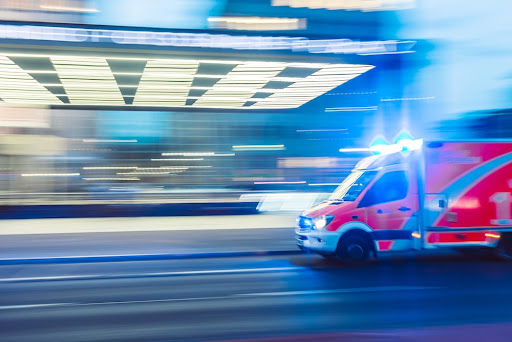
Case studies can provide a deep understanding of a patient's journey, giving insights into the intricacies of disease progression, treatment effects, and the psychosocial aspects of health and illness.
Asthma research studies
Specifically within medical research, studies on asthma often employ case studies to explore the individual and environmental factors that influence asthma development, management, and outcomes. A case study can provide rich, detailed data about individual patients' experiences, from the triggers and symptoms they experience to the effectiveness of various management strategies. This can be crucial for developing patient-centered asthma care approaches.
Other fields
Apart from the fields mentioned, case studies are also extensively used in business and management research, education research, and political sciences, among many others. They provide an opportunity to delve into the intricacies of real-world situations, allowing for a comprehensive understanding of various phenomena.
Case studies, with their depth and contextual focus, offer unique insights across these varied fields. They allow researchers to illuminate the complexities of real-life situations, contributing to both theory and practice.
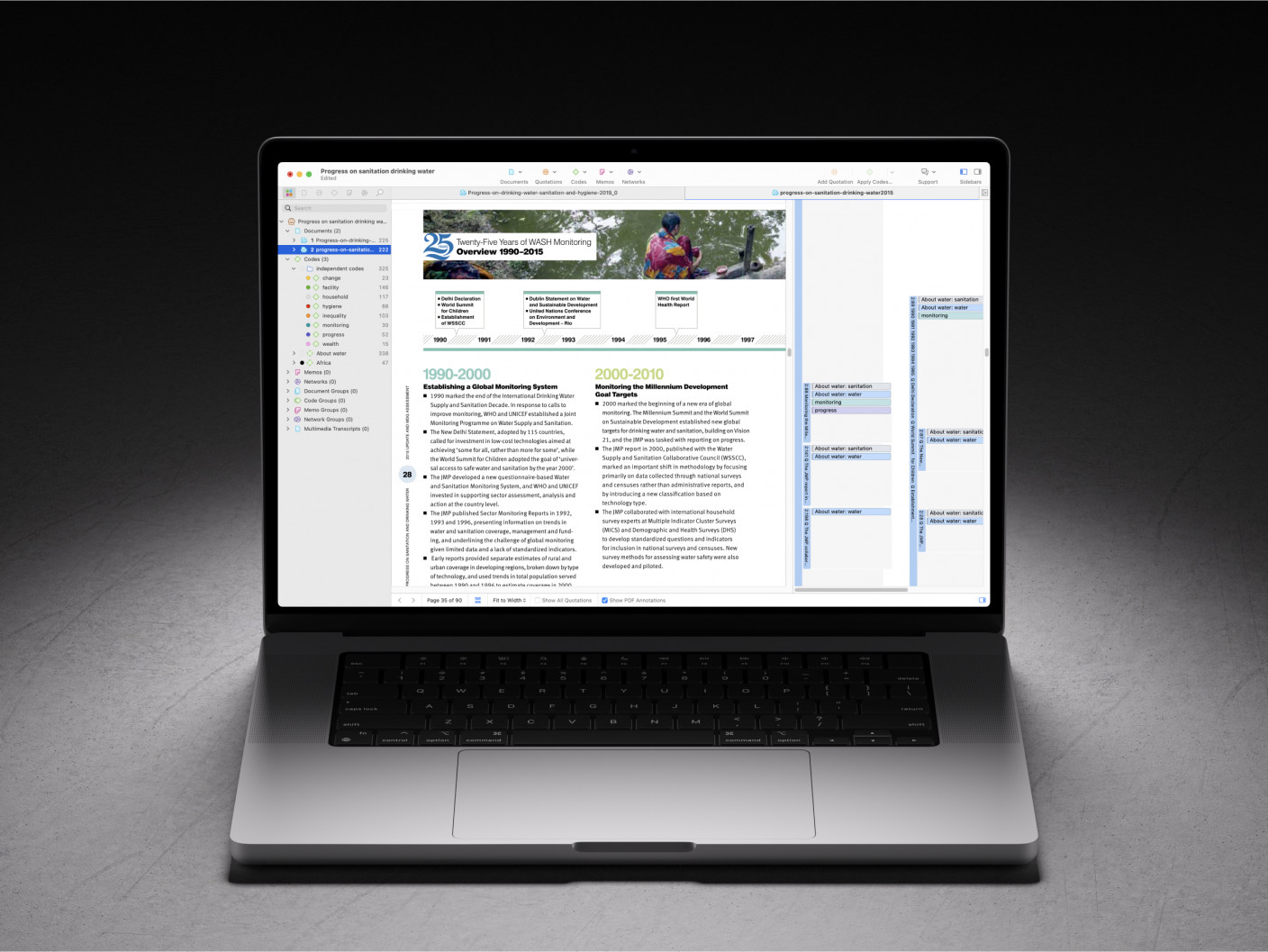
Whatever field you're in, ATLAS.ti puts your data to work for you
Download a free trial of ATLAS.ti to turn your data into insights.
Understanding the key elements of case study design is crucial for conducting rigorous and impactful case study research. A well-structured design guides the researcher through the process, ensuring that the study is methodologically sound and its findings are reliable and valid. The main elements of case study design include the research question , propositions, units of analysis, and the logic linking the data to the propositions.
The research question is the foundation of any research study. A good research question guides the direction of the study and informs the selection of the case, the methods of collecting data, and the analysis techniques. A well-formulated research question in case study research is typically clear, focused, and complex enough to merit further detailed examination of the relevant case(s).
Propositions
Propositions, though not necessary in every case study, provide a direction by stating what we might expect to find in the data collected. They guide how data is collected and analyzed by helping researchers focus on specific aspects of the case. They are particularly important in explanatory case studies, which seek to understand the relationships among concepts within the studied phenomenon.
Units of analysis
The unit of analysis refers to the case, or the main entity or entities that are being analyzed in the study. In case study research, the unit of analysis can be an individual, a group, an organization, a decision, an event, or even a time period. It's crucial to clearly define the unit of analysis, as it shapes the qualitative data analysis process by allowing the researcher to analyze a particular case and synthesize analysis across multiple case studies to draw conclusions.
Argumentation
This refers to the inferential model that allows researchers to draw conclusions from the data. The researcher needs to ensure that there is a clear link between the data, the propositions (if any), and the conclusions drawn. This argumentation is what enables the researcher to make valid and credible inferences about the phenomenon under study.
Understanding and carefully considering these elements in the design phase of a case study can significantly enhance the quality of the research. It can help ensure that the study is methodologically sound and its findings contribute meaningful insights about the case.
Ready to jumpstart your research with ATLAS.ti?
Conceptualize your research project with our intuitive data analysis interface. Download a free trial today.
Conducting a case study involves several steps, from defining the research question and selecting the case to collecting and analyzing data . This section outlines these key stages, providing a practical guide on how to conduct case study research.
Defining the research question
The first step in case study research is defining a clear, focused research question. This question should guide the entire research process, from case selection to analysis. It's crucial to ensure that the research question is suitable for a case study approach. Typically, such questions are exploratory or descriptive in nature and focus on understanding a phenomenon within its real-life context.
Selecting and defining the case
The selection of the case should be based on the research question and the objectives of the study. It involves choosing a unique example or a set of examples that provide rich, in-depth data about the phenomenon under investigation. After selecting the case, it's crucial to define it clearly, setting the boundaries of the case, including the time period and the specific context.
Previous research can help guide the case study design. When considering a case study, an example of a case could be taken from previous case study research and used to define cases in a new research inquiry. Considering recently published examples can help understand how to select and define cases effectively.
Developing a detailed case study protocol
A case study protocol outlines the procedures and general rules to be followed during the case study. This includes the data collection methods to be used, the sources of data, and the procedures for analysis. Having a detailed case study protocol ensures consistency and reliability in the study.
The protocol should also consider how to work with the people involved in the research context to grant the research team access to collecting data. As mentioned in previous sections of this guide, establishing rapport is an essential component of qualitative research as it shapes the overall potential for collecting and analyzing data.
Collecting data
Gathering data in case study research often involves multiple sources of evidence, including documents, archival records, interviews, observations, and physical artifacts. This allows for a comprehensive understanding of the case. The process for gathering data should be systematic and carefully documented to ensure the reliability and validity of the study.
Analyzing and interpreting data
The next step is analyzing the data. This involves organizing the data , categorizing it into themes or patterns , and interpreting these patterns to answer the research question. The analysis might also involve comparing the findings with prior research or theoretical propositions.
Writing the case study report
The final step is writing the case study report . This should provide a detailed description of the case, the data, the analysis process, and the findings. The report should be clear, organized, and carefully written to ensure that the reader can understand the case and the conclusions drawn from it.
Each of these steps is crucial in ensuring that the case study research is rigorous, reliable, and provides valuable insights about the case.
The type, depth, and quality of data in your study can significantly influence the validity and utility of the study. In case study research, data is usually collected from multiple sources to provide a comprehensive and nuanced understanding of the case. This section will outline the various methods of collecting data used in case study research and discuss considerations for ensuring the quality of the data.
Interviews are a common method of gathering data in case study research. They can provide rich, in-depth data about the perspectives, experiences, and interpretations of the individuals involved in the case. Interviews can be structured , semi-structured , or unstructured , depending on the research question and the degree of flexibility needed.
Observations
Observations involve the researcher observing the case in its natural setting, providing first-hand information about the case and its context. Observations can provide data that might not be revealed in interviews or documents, such as non-verbal cues or contextual information.
Documents and artifacts
Documents and archival records provide a valuable source of data in case study research. They can include reports, letters, memos, meeting minutes, email correspondence, and various public and private documents related to the case.
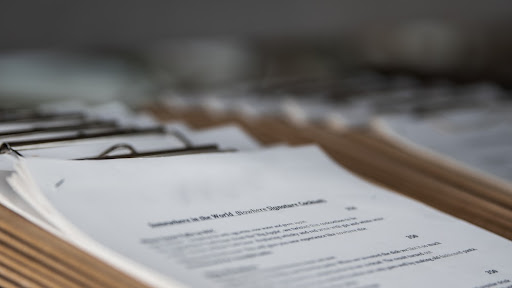
These records can provide historical context, corroborate evidence from other sources, and offer insights into the case that might not be apparent from interviews or observations.
Physical artifacts refer to any physical evidence related to the case, such as tools, products, or physical environments. These artifacts can provide tangible insights into the case, complementing the data gathered from other sources.
Ensuring the quality of data collection
Determining the quality of data in case study research requires careful planning and execution. It's crucial to ensure that the data is reliable, accurate, and relevant to the research question. This involves selecting appropriate methods of collecting data, properly training interviewers or observers, and systematically recording and storing the data. It also includes considering ethical issues related to collecting and handling data, such as obtaining informed consent and ensuring the privacy and confidentiality of the participants.
Data analysis
Analyzing case study research involves making sense of the rich, detailed data to answer the research question. This process can be challenging due to the volume and complexity of case study data. However, a systematic and rigorous approach to analysis can ensure that the findings are credible and meaningful. This section outlines the main steps and considerations in analyzing data in case study research.
Organizing the data
The first step in the analysis is organizing the data. This involves sorting the data into manageable sections, often according to the data source or the theme. This step can also involve transcribing interviews, digitizing physical artifacts, or organizing observational data.
Categorizing and coding the data
Once the data is organized, the next step is to categorize or code the data. This involves identifying common themes, patterns, or concepts in the data and assigning codes to relevant data segments. Coding can be done manually or with the help of software tools, and in either case, qualitative analysis software can greatly facilitate the entire coding process. Coding helps to reduce the data to a set of themes or categories that can be more easily analyzed.
Identifying patterns and themes
After coding the data, the researcher looks for patterns or themes in the coded data. This involves comparing and contrasting the codes and looking for relationships or patterns among them. The identified patterns and themes should help answer the research question.
Interpreting the data
Once patterns and themes have been identified, the next step is to interpret these findings. This involves explaining what the patterns or themes mean in the context of the research question and the case. This interpretation should be grounded in the data, but it can also involve drawing on theoretical concepts or prior research.
Verification of the data
The last step in the analysis is verification. This involves checking the accuracy and consistency of the analysis process and confirming that the findings are supported by the data. This can involve re-checking the original data, checking the consistency of codes, or seeking feedback from research participants or peers.
Like any research method , case study research has its strengths and limitations. Researchers must be aware of these, as they can influence the design, conduct, and interpretation of the study.
Understanding the strengths and limitations of case study research can also guide researchers in deciding whether this approach is suitable for their research question . This section outlines some of the key strengths and limitations of case study research.
Benefits include the following:
- Rich, detailed data: One of the main strengths of case study research is that it can generate rich, detailed data about the case. This can provide a deep understanding of the case and its context, which can be valuable in exploring complex phenomena.
- Flexibility: Case study research is flexible in terms of design , data collection , and analysis . A sufficient degree of flexibility allows the researcher to adapt the study according to the case and the emerging findings.
- Real-world context: Case study research involves studying the case in its real-world context, which can provide valuable insights into the interplay between the case and its context.
- Multiple sources of evidence: Case study research often involves collecting data from multiple sources , which can enhance the robustness and validity of the findings.
On the other hand, researchers should consider the following limitations:
- Generalizability: A common criticism of case study research is that its findings might not be generalizable to other cases due to the specificity and uniqueness of each case.
- Time and resource intensive: Case study research can be time and resource intensive due to the depth of the investigation and the amount of collected data.
- Complexity of analysis: The rich, detailed data generated in case study research can make analyzing the data challenging.
- Subjectivity: Given the nature of case study research, there may be a higher degree of subjectivity in interpreting the data , so researchers need to reflect on this and transparently convey to audiences how the research was conducted.
Being aware of these strengths and limitations can help researchers design and conduct case study research effectively and interpret and report the findings appropriately.
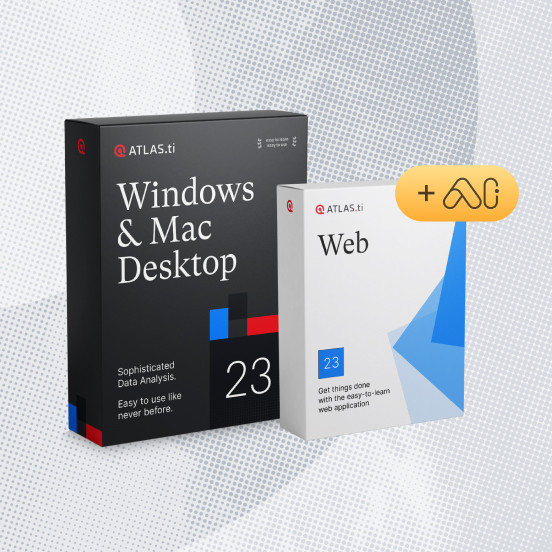
Ready to analyze your data with ATLAS.ti?
See how our intuitive software can draw key insights from your data with a free trial today.
Case Study Research Method in Psychology
Saul McLeod, PhD
Editor-in-Chief for Simply Psychology
BSc (Hons) Psychology, MRes, PhD, University of Manchester
Saul McLeod, PhD., is a qualified psychology teacher with over 18 years of experience in further and higher education. He has been published in peer-reviewed journals, including the Journal of Clinical Psychology.
Learn about our Editorial Process
Olivia Guy-Evans, MSc
Associate Editor for Simply Psychology
BSc (Hons) Psychology, MSc Psychology of Education
Olivia Guy-Evans is a writer and associate editor for Simply Psychology. She has previously worked in healthcare and educational sectors.
On This Page:
Case studies are in-depth investigations of a person, group, event, or community. Typically, data is gathered from various sources using several methods (e.g., observations & interviews).
The case study research method originated in clinical medicine (the case history, i.e., the patient’s personal history). In psychology, case studies are often confined to the study of a particular individual.
The information is mainly biographical and relates to events in the individual’s past (i.e., retrospective), as well as to significant events that are currently occurring in his or her everyday life.
The case study is not a research method, but researchers select methods of data collection and analysis that will generate material suitable for case studies.
Freud (1909a, 1909b) conducted very detailed investigations into the private lives of his patients in an attempt to both understand and help them overcome their illnesses.
This makes it clear that the case study is a method that should only be used by a psychologist, therapist, or psychiatrist, i.e., someone with a professional qualification.
There is an ethical issue of competence. Only someone qualified to diagnose and treat a person can conduct a formal case study relating to atypical (i.e., abnormal) behavior or atypical development.
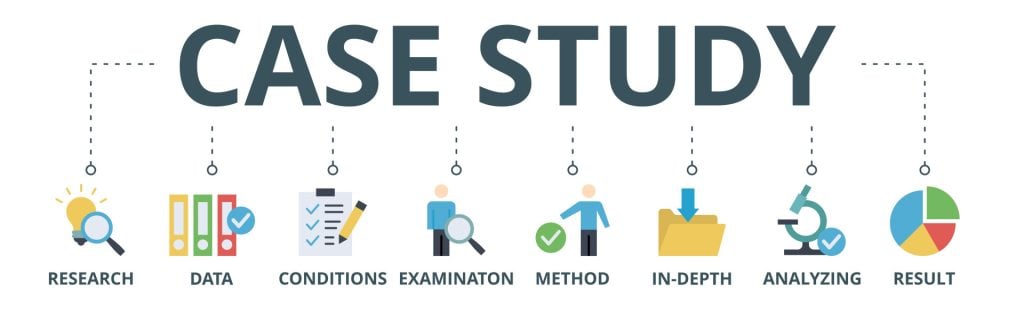
Famous Case Studies
- Anna O – One of the most famous case studies, documenting psychoanalyst Josef Breuer’s treatment of “Anna O” (real name Bertha Pappenheim) for hysteria in the late 1800s using early psychoanalytic theory.
- Little Hans – A child psychoanalysis case study published by Sigmund Freud in 1909 analyzing his five-year-old patient Herbert Graf’s house phobia as related to the Oedipus complex.
- Bruce/Brenda – Gender identity case of the boy (Bruce) whose botched circumcision led psychologist John Money to advise gender reassignment and raise him as a girl (Brenda) in the 1960s.
- Genie Wiley – Linguistics/psychological development case of the victim of extreme isolation abuse who was studied in 1970s California for effects of early language deprivation on acquiring speech later in life.
- Phineas Gage – One of the most famous neuropsychology case studies analyzes personality changes in railroad worker Phineas Gage after an 1848 brain injury involving a tamping iron piercing his skull.
Clinical Case Studies
- Studying the effectiveness of psychotherapy approaches with an individual patient
- Assessing and treating mental illnesses like depression, anxiety disorders, PTSD
- Neuropsychological cases investigating brain injuries or disorders
Child Psychology Case Studies
- Studying psychological development from birth through adolescence
- Cases of learning disabilities, autism spectrum disorders, ADHD
- Effects of trauma, abuse, deprivation on development
Types of Case Studies
- Explanatory case studies : Used to explore causation in order to find underlying principles. Helpful for doing qualitative analysis to explain presumed causal links.
- Exploratory case studies : Used to explore situations where an intervention being evaluated has no clear set of outcomes. It helps define questions and hypotheses for future research.
- Descriptive case studies : Describe an intervention or phenomenon and the real-life context in which it occurred. It is helpful for illustrating certain topics within an evaluation.
- Multiple-case studies : Used to explore differences between cases and replicate findings across cases. Helpful for comparing and contrasting specific cases.
- Intrinsic : Used to gain a better understanding of a particular case. Helpful for capturing the complexity of a single case.
- Collective : Used to explore a general phenomenon using multiple case studies. Helpful for jointly studying a group of cases in order to inquire into the phenomenon.
Where Do You Find Data for a Case Study?
There are several places to find data for a case study. The key is to gather data from multiple sources to get a complete picture of the case and corroborate facts or findings through triangulation of evidence. Most of this information is likely qualitative (i.e., verbal description rather than measurement), but the psychologist might also collect numerical data.
1. Primary sources
- Interviews – Interviewing key people related to the case to get their perspectives and insights. The interview is an extremely effective procedure for obtaining information about an individual, and it may be used to collect comments from the person’s friends, parents, employer, workmates, and others who have a good knowledge of the person, as well as to obtain facts from the person him or herself.
- Observations – Observing behaviors, interactions, processes, etc., related to the case as they unfold in real-time.
- Documents & Records – Reviewing private documents, diaries, public records, correspondence, meeting minutes, etc., relevant to the case.
2. Secondary sources
- News/Media – News coverage of events related to the case study.
- Academic articles – Journal articles, dissertations etc. that discuss the case.
- Government reports – Official data and records related to the case context.
- Books/films – Books, documentaries or films discussing the case.
3. Archival records
Searching historical archives, museum collections and databases to find relevant documents, visual/audio records related to the case history and context.
Public archives like newspapers, organizational records, photographic collections could all include potentially relevant pieces of information to shed light on attitudes, cultural perspectives, common practices and historical contexts related to psychology.
4. Organizational records
Organizational records offer the advantage of often having large datasets collected over time that can reveal or confirm psychological insights.
Of course, privacy and ethical concerns regarding confidential data must be navigated carefully.
However, with proper protocols, organizational records can provide invaluable context and empirical depth to qualitative case studies exploring the intersection of psychology and organizations.
- Organizational/industrial psychology research : Organizational records like employee surveys, turnover/retention data, policies, incident reports etc. may provide insight into topics like job satisfaction, workplace culture and dynamics, leadership issues, employee behaviors etc.
- Clinical psychology : Therapists/hospitals may grant access to anonymized medical records to study aspects like assessments, diagnoses, treatment plans etc. This could shed light on clinical practices.
- School psychology : Studies could utilize anonymized student records like test scores, grades, disciplinary issues, and counseling referrals to study child development, learning barriers, effectiveness of support programs, and more.
How do I Write a Case Study in Psychology?
Follow specified case study guidelines provided by a journal or your psychology tutor. General components of clinical case studies include: background, symptoms, assessments, diagnosis, treatment, and outcomes. Interpreting the information means the researcher decides what to include or leave out. A good case study should always clarify which information is the factual description and which is an inference or the researcher’s opinion.
1. Introduction
- Provide background on the case context and why it is of interest, presenting background information like demographics, relevant history, and presenting problem.
- Compare briefly to similar published cases if applicable. Clearly state the focus/importance of the case.
2. Case Presentation
- Describe the presenting problem in detail, including symptoms, duration,and impact on daily life.
- Include client demographics like age and gender, information about social relationships, and mental health history.
- Describe all physical, emotional, and/or sensory symptoms reported by the client.
- Use patient quotes to describe the initial complaint verbatim. Follow with full-sentence summaries of relevant history details gathered, including key components that led to a working diagnosis.
- Summarize clinical exam results, namely orthopedic/neurological tests, imaging, lab tests, etc. Note actual results rather than subjective conclusions. Provide images if clearly reproducible/anonymized.
- Clearly state the working diagnosis or clinical impression before transitioning to management.
3. Management and Outcome
- Indicate the total duration of care and number of treatments given over what timeframe. Use specific names/descriptions for any therapies/interventions applied.
- Present the results of the intervention,including any quantitative or qualitative data collected.
- For outcomes, utilize visual analog scales for pain, medication usage logs, etc., if possible. Include patient self-reports of improvement/worsening of symptoms. Note the reason for discharge/end of care.
4. Discussion
- Analyze the case, exploring contributing factors, limitations of the study, and connections to existing research.
- Analyze the effectiveness of the intervention,considering factors like participant adherence, limitations of the study, and potential alternative explanations for the results.
- Identify any questions raised in the case analysis and relate insights to established theories and current research if applicable. Avoid definitive claims about physiological explanations.
- Offer clinical implications, and suggest future research directions.
5. Additional Items
- Thank specific assistants for writing support only. No patient acknowledgments.
- References should directly support any key claims or quotes included.
- Use tables/figures/images only if substantially informative. Include permissions and legends/explanatory notes.
- Provides detailed (rich qualitative) information.
- Provides insight for further research.
- Permitting investigation of otherwise impractical (or unethical) situations.
Case studies allow a researcher to investigate a topic in far more detail than might be possible if they were trying to deal with a large number of research participants (nomothetic approach) with the aim of ‘averaging’.
Because of their in-depth, multi-sided approach, case studies often shed light on aspects of human thinking and behavior that would be unethical or impractical to study in other ways.
Research that only looks into the measurable aspects of human behavior is not likely to give us insights into the subjective dimension of experience, which is important to psychoanalytic and humanistic psychologists.
Case studies are often used in exploratory research. They can help us generate new ideas (that might be tested by other methods). They are an important way of illustrating theories and can help show how different aspects of a person’s life are related to each other.
The method is, therefore, important for psychologists who adopt a holistic point of view (i.e., humanistic psychologists ).
Limitations
- Lacking scientific rigor and providing little basis for generalization of results to the wider population.
- Researchers’ own subjective feelings may influence the case study (researcher bias).
- Difficult to replicate.
- Time-consuming and expensive.
- The volume of data, together with the time restrictions in place, impacted the depth of analysis that was possible within the available resources.
Because a case study deals with only one person/event/group, we can never be sure if the case study investigated is representative of the wider body of “similar” instances. This means the conclusions drawn from a particular case may not be transferable to other settings.
Because case studies are based on the analysis of qualitative (i.e., descriptive) data , a lot depends on the psychologist’s interpretation of the information she has acquired.
This means that there is a lot of scope for Anna O , and it could be that the subjective opinions of the psychologist intrude in the assessment of what the data means.
For example, Freud has been criticized for producing case studies in which the information was sometimes distorted to fit particular behavioral theories (e.g., Little Hans ).
This is also true of Money’s interpretation of the Bruce/Brenda case study (Diamond, 1997) when he ignored evidence that went against his theory.
Breuer, J., & Freud, S. (1895). Studies on hysteria . Standard Edition 2: London.
Curtiss, S. (1981). Genie: The case of a modern wild child .
Diamond, M., & Sigmundson, K. (1997). Sex Reassignment at Birth: Long-term Review and Clinical Implications. Archives of Pediatrics & Adolescent Medicine , 151(3), 298-304
Freud, S. (1909a). Analysis of a phobia of a five year old boy. In The Pelican Freud Library (1977), Vol 8, Case Histories 1, pages 169-306
Freud, S. (1909b). Bemerkungen über einen Fall von Zwangsneurose (Der “Rattenmann”). Jb. psychoanal. psychopathol. Forsch ., I, p. 357-421; GW, VII, p. 379-463; Notes upon a case of obsessional neurosis, SE , 10: 151-318.
Harlow J. M. (1848). Passage of an iron rod through the head. Boston Medical and Surgical Journal, 39 , 389–393.
Harlow, J. M. (1868). Recovery from the Passage of an Iron Bar through the Head . Publications of the Massachusetts Medical Society. 2 (3), 327-347.
Money, J., & Ehrhardt, A. A. (1972). Man & Woman, Boy & Girl : The Differentiation and Dimorphism of Gender Identity from Conception to Maturity. Baltimore, Maryland: Johns Hopkins University Press.
Money, J., & Tucker, P. (1975). Sexual signatures: On being a man or a woman.
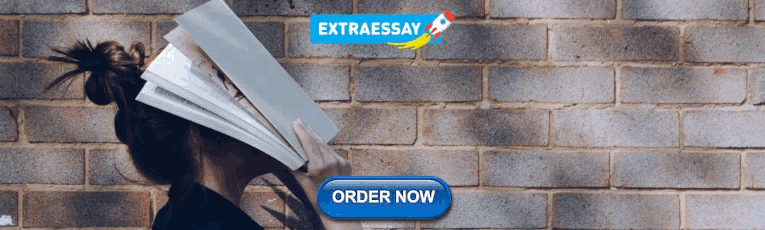
Further Information
- Case Study Approach
- Case Study Method
- Enhancing the Quality of Case Studies in Health Services Research
- “We do things together” A case study of “couplehood” in dementia
- Using mixed methods for evaluating an integrative approach to cancer care: a case study

Have a language expert improve your writing
Run a free plagiarism check in 10 minutes, automatically generate references for free.
- Knowledge Base
- Methodology
- Case Study | Definition, Examples & Methods
Case Study | Definition, Examples & Methods
Published on 5 May 2022 by Shona McCombes . Revised on 30 January 2023.
A case study is a detailed study of a specific subject, such as a person, group, place, event, organisation, or phenomenon. Case studies are commonly used in social, educational, clinical, and business research.
A case study research design usually involves qualitative methods , but quantitative methods are sometimes also used. Case studies are good for describing , comparing, evaluating, and understanding different aspects of a research problem .
Table of contents
When to do a case study, step 1: select a case, step 2: build a theoretical framework, step 3: collect your data, step 4: describe and analyse the case.
A case study is an appropriate research design when you want to gain concrete, contextual, in-depth knowledge about a specific real-world subject. It allows you to explore the key characteristics, meanings, and implications of the case.
Case studies are often a good choice in a thesis or dissertation . They keep your project focused and manageable when you don’t have the time or resources to do large-scale research.
You might use just one complex case study where you explore a single subject in depth, or conduct multiple case studies to compare and illuminate different aspects of your research problem.
Research question | Case study |
---|---|
What are the ecological effects of wolf reintroduction? | Case study of wolf reintroduction in Yellowstone National Park in the US |
How do populist politicians use narratives about history to gain support? | Case studies of Hungarian prime minister Viktor Orbán and US president Donald Trump |
How can teachers implement active learning strategies in mixed-level classrooms? | Case study of a local school that promotes active learning |
What are the main advantages and disadvantages of wind farms for rural communities? | Case studies of three rural wind farm development projects in different parts of the country |
How are viral marketing strategies changing the relationship between companies and consumers? | Case study of the iPhone X marketing campaign |
How do experiences of work in the gig economy differ by gender, race, and age? | Case studies of Deliveroo and Uber drivers in London |
Prevent plagiarism, run a free check.
Once you have developed your problem statement and research questions , you should be ready to choose the specific case that you want to focus on. A good case study should have the potential to:
- Provide new or unexpected insights into the subject
- Challenge or complicate existing assumptions and theories
- Propose practical courses of action to resolve a problem
- Open up new directions for future research
Unlike quantitative or experimental research, a strong case study does not require a random or representative sample. In fact, case studies often deliberately focus on unusual, neglected, or outlying cases which may shed new light on the research problem.
If you find yourself aiming to simultaneously investigate and solve an issue, consider conducting action research . As its name suggests, action research conducts research and takes action at the same time, and is highly iterative and flexible.
However, you can also choose a more common or representative case to exemplify a particular category, experience, or phenomenon.
While case studies focus more on concrete details than general theories, they should usually have some connection with theory in the field. This way the case study is not just an isolated description, but is integrated into existing knowledge about the topic. It might aim to:
- Exemplify a theory by showing how it explains the case under investigation
- Expand on a theory by uncovering new concepts and ideas that need to be incorporated
- Challenge a theory by exploring an outlier case that doesn’t fit with established assumptions
To ensure that your analysis of the case has a solid academic grounding, you should conduct a literature review of sources related to the topic and develop a theoretical framework . This means identifying key concepts and theories to guide your analysis and interpretation.
There are many different research methods you can use to collect data on your subject. Case studies tend to focus on qualitative data using methods such as interviews, observations, and analysis of primary and secondary sources (e.g., newspaper articles, photographs, official records). Sometimes a case study will also collect quantitative data .
The aim is to gain as thorough an understanding as possible of the case and its context.
In writing up the case study, you need to bring together all the relevant aspects to give as complete a picture as possible of the subject.
How you report your findings depends on the type of research you are doing. Some case studies are structured like a standard scientific paper or thesis, with separate sections or chapters for the methods , results , and discussion .
Others are written in a more narrative style, aiming to explore the case from various angles and analyse its meanings and implications (for example, by using textual analysis or discourse analysis ).
In all cases, though, make sure to give contextual details about the case, connect it back to the literature and theory, and discuss how it fits into wider patterns or debates.
Cite this Scribbr article
If you want to cite this source, you can copy and paste the citation or click the ‘Cite this Scribbr article’ button to automatically add the citation to our free Reference Generator.
McCombes, S. (2023, January 30). Case Study | Definition, Examples & Methods. Scribbr. Retrieved 26 August 2024, from https://www.scribbr.co.uk/research-methods/case-studies/
Is this article helpful?
Shona McCombes
Other students also liked, correlational research | guide, design & examples, a quick guide to experimental design | 5 steps & examples, descriptive research design | definition, methods & examples.
- Open access
- Published: 27 June 2011
The case study approach
- Sarah Crowe 1 ,
- Kathrin Cresswell 2 ,
- Ann Robertson 2 ,
- Guro Huby 3 ,
- Anthony Avery 1 &
- Aziz Sheikh 2
BMC Medical Research Methodology volume 11 , Article number: 100 ( 2011 ) Cite this article
795k Accesses
1104 Citations
42 Altmetric
Metrics details
The case study approach allows in-depth, multi-faceted explorations of complex issues in their real-life settings. The value of the case study approach is well recognised in the fields of business, law and policy, but somewhat less so in health services research. Based on our experiences of conducting several health-related case studies, we reflect on the different types of case study design, the specific research questions this approach can help answer, the data sources that tend to be used, and the particular advantages and disadvantages of employing this methodological approach. The paper concludes with key pointers to aid those designing and appraising proposals for conducting case study research, and a checklist to help readers assess the quality of case study reports.
Peer Review reports
Introduction
The case study approach is particularly useful to employ when there is a need to obtain an in-depth appreciation of an issue, event or phenomenon of interest, in its natural real-life context. Our aim in writing this piece is to provide insights into when to consider employing this approach and an overview of key methodological considerations in relation to the design, planning, analysis, interpretation and reporting of case studies.
The illustrative 'grand round', 'case report' and 'case series' have a long tradition in clinical practice and research. Presenting detailed critiques, typically of one or more patients, aims to provide insights into aspects of the clinical case and, in doing so, illustrate broader lessons that may be learnt. In research, the conceptually-related case study approach can be used, for example, to describe in detail a patient's episode of care, explore professional attitudes to and experiences of a new policy initiative or service development or more generally to 'investigate contemporary phenomena within its real-life context' [ 1 ]. Based on our experiences of conducting a range of case studies, we reflect on when to consider using this approach, discuss the key steps involved and illustrate, with examples, some of the practical challenges of attaining an in-depth understanding of a 'case' as an integrated whole. In keeping with previously published work, we acknowledge the importance of theory to underpin the design, selection, conduct and interpretation of case studies[ 2 ]. In so doing, we make passing reference to the different epistemological approaches used in case study research by key theoreticians and methodologists in this field of enquiry.
This paper is structured around the following main questions: What is a case study? What are case studies used for? How are case studies conducted? What are the potential pitfalls and how can these be avoided? We draw in particular on four of our own recently published examples of case studies (see Tables 1 , 2 , 3 and 4 ) and those of others to illustrate our discussion[ 3 – 7 ].
What is a case study?
A case study is a research approach that is used to generate an in-depth, multi-faceted understanding of a complex issue in its real-life context. It is an established research design that is used extensively in a wide variety of disciplines, particularly in the social sciences. A case study can be defined in a variety of ways (Table 5 ), the central tenet being the need to explore an event or phenomenon in depth and in its natural context. It is for this reason sometimes referred to as a "naturalistic" design; this is in contrast to an "experimental" design (such as a randomised controlled trial) in which the investigator seeks to exert control over and manipulate the variable(s) of interest.
Stake's work has been particularly influential in defining the case study approach to scientific enquiry. He has helpfully characterised three main types of case study: intrinsic , instrumental and collective [ 8 ]. An intrinsic case study is typically undertaken to learn about a unique phenomenon. The researcher should define the uniqueness of the phenomenon, which distinguishes it from all others. In contrast, the instrumental case study uses a particular case (some of which may be better than others) to gain a broader appreciation of an issue or phenomenon. The collective case study involves studying multiple cases simultaneously or sequentially in an attempt to generate a still broader appreciation of a particular issue.
These are however not necessarily mutually exclusive categories. In the first of our examples (Table 1 ), we undertook an intrinsic case study to investigate the issue of recruitment of minority ethnic people into the specific context of asthma research studies, but it developed into a instrumental case study through seeking to understand the issue of recruitment of these marginalised populations more generally, generating a number of the findings that are potentially transferable to other disease contexts[ 3 ]. In contrast, the other three examples (see Tables 2 , 3 and 4 ) employed collective case study designs to study the introduction of workforce reconfiguration in primary care, the implementation of electronic health records into hospitals, and to understand the ways in which healthcare students learn about patient safety considerations[ 4 – 6 ]. Although our study focusing on the introduction of General Practitioners with Specialist Interests (Table 2 ) was explicitly collective in design (four contrasting primary care organisations were studied), is was also instrumental in that this particular professional group was studied as an exemplar of the more general phenomenon of workforce redesign[ 4 ].
What are case studies used for?
According to Yin, case studies can be used to explain, describe or explore events or phenomena in the everyday contexts in which they occur[ 1 ]. These can, for example, help to understand and explain causal links and pathways resulting from a new policy initiative or service development (see Tables 2 and 3 , for example)[ 1 ]. In contrast to experimental designs, which seek to test a specific hypothesis through deliberately manipulating the environment (like, for example, in a randomised controlled trial giving a new drug to randomly selected individuals and then comparing outcomes with controls),[ 9 ] the case study approach lends itself well to capturing information on more explanatory ' how ', 'what' and ' why ' questions, such as ' how is the intervention being implemented and received on the ground?'. The case study approach can offer additional insights into what gaps exist in its delivery or why one implementation strategy might be chosen over another. This in turn can help develop or refine theory, as shown in our study of the teaching of patient safety in undergraduate curricula (Table 4 )[ 6 , 10 ]. Key questions to consider when selecting the most appropriate study design are whether it is desirable or indeed possible to undertake a formal experimental investigation in which individuals and/or organisations are allocated to an intervention or control arm? Or whether the wish is to obtain a more naturalistic understanding of an issue? The former is ideally studied using a controlled experimental design, whereas the latter is more appropriately studied using a case study design.
Case studies may be approached in different ways depending on the epistemological standpoint of the researcher, that is, whether they take a critical (questioning one's own and others' assumptions), interpretivist (trying to understand individual and shared social meanings) or positivist approach (orientating towards the criteria of natural sciences, such as focusing on generalisability considerations) (Table 6 ). Whilst such a schema can be conceptually helpful, it may be appropriate to draw on more than one approach in any case study, particularly in the context of conducting health services research. Doolin has, for example, noted that in the context of undertaking interpretative case studies, researchers can usefully draw on a critical, reflective perspective which seeks to take into account the wider social and political environment that has shaped the case[ 11 ].
How are case studies conducted?
Here, we focus on the main stages of research activity when planning and undertaking a case study; the crucial stages are: defining the case; selecting the case(s); collecting and analysing the data; interpreting data; and reporting the findings.
Defining the case
Carefully formulated research question(s), informed by the existing literature and a prior appreciation of the theoretical issues and setting(s), are all important in appropriately and succinctly defining the case[ 8 , 12 ]. Crucially, each case should have a pre-defined boundary which clarifies the nature and time period covered by the case study (i.e. its scope, beginning and end), the relevant social group, organisation or geographical area of interest to the investigator, the types of evidence to be collected, and the priorities for data collection and analysis (see Table 7 )[ 1 ]. A theory driven approach to defining the case may help generate knowledge that is potentially transferable to a range of clinical contexts and behaviours; using theory is also likely to result in a more informed appreciation of, for example, how and why interventions have succeeded or failed[ 13 ].
For example, in our evaluation of the introduction of electronic health records in English hospitals (Table 3 ), we defined our cases as the NHS Trusts that were receiving the new technology[ 5 ]. Our focus was on how the technology was being implemented. However, if the primary research interest had been on the social and organisational dimensions of implementation, we might have defined our case differently as a grouping of healthcare professionals (e.g. doctors and/or nurses). The precise beginning and end of the case may however prove difficult to define. Pursuing this same example, when does the process of implementation and adoption of an electronic health record system really begin or end? Such judgements will inevitably be influenced by a range of factors, including the research question, theory of interest, the scope and richness of the gathered data and the resources available to the research team.
Selecting the case(s)
The decision on how to select the case(s) to study is a very important one that merits some reflection. In an intrinsic case study, the case is selected on its own merits[ 8 ]. The case is selected not because it is representative of other cases, but because of its uniqueness, which is of genuine interest to the researchers. This was, for example, the case in our study of the recruitment of minority ethnic participants into asthma research (Table 1 ) as our earlier work had demonstrated the marginalisation of minority ethnic people with asthma, despite evidence of disproportionate asthma morbidity[ 14 , 15 ]. In another example of an intrinsic case study, Hellstrom et al.[ 16 ] studied an elderly married couple living with dementia to explore how dementia had impacted on their understanding of home, their everyday life and their relationships.
For an instrumental case study, selecting a "typical" case can work well[ 8 ]. In contrast to the intrinsic case study, the particular case which is chosen is of less importance than selecting a case that allows the researcher to investigate an issue or phenomenon. For example, in order to gain an understanding of doctors' responses to health policy initiatives, Som undertook an instrumental case study interviewing clinicians who had a range of responsibilities for clinical governance in one NHS acute hospital trust[ 17 ]. Sampling a "deviant" or "atypical" case may however prove even more informative, potentially enabling the researcher to identify causal processes, generate hypotheses and develop theory.
In collective or multiple case studies, a number of cases are carefully selected. This offers the advantage of allowing comparisons to be made across several cases and/or replication. Choosing a "typical" case may enable the findings to be generalised to theory (i.e. analytical generalisation) or to test theory by replicating the findings in a second or even a third case (i.e. replication logic)[ 1 ]. Yin suggests two or three literal replications (i.e. predicting similar results) if the theory is straightforward and five or more if the theory is more subtle. However, critics might argue that selecting 'cases' in this way is insufficiently reflexive and ill-suited to the complexities of contemporary healthcare organisations.
The selected case study site(s) should allow the research team access to the group of individuals, the organisation, the processes or whatever else constitutes the chosen unit of analysis for the study. Access is therefore a central consideration; the researcher needs to come to know the case study site(s) well and to work cooperatively with them. Selected cases need to be not only interesting but also hospitable to the inquiry [ 8 ] if they are to be informative and answer the research question(s). Case study sites may also be pre-selected for the researcher, with decisions being influenced by key stakeholders. For example, our selection of case study sites in the evaluation of the implementation and adoption of electronic health record systems (see Table 3 ) was heavily influenced by NHS Connecting for Health, the government agency that was responsible for overseeing the National Programme for Information Technology (NPfIT)[ 5 ]. This prominent stakeholder had already selected the NHS sites (through a competitive bidding process) to be early adopters of the electronic health record systems and had negotiated contracts that detailed the deployment timelines.
It is also important to consider in advance the likely burden and risks associated with participation for those who (or the site(s) which) comprise the case study. Of particular importance is the obligation for the researcher to think through the ethical implications of the study (e.g. the risk of inadvertently breaching anonymity or confidentiality) and to ensure that potential participants/participating sites are provided with sufficient information to make an informed choice about joining the study. The outcome of providing this information might be that the emotive burden associated with participation, or the organisational disruption associated with supporting the fieldwork, is considered so high that the individuals or sites decide against participation.
In our example of evaluating implementations of electronic health record systems, given the restricted number of early adopter sites available to us, we sought purposively to select a diverse range of implementation cases among those that were available[ 5 ]. We chose a mixture of teaching, non-teaching and Foundation Trust hospitals, and examples of each of the three electronic health record systems procured centrally by the NPfIT. At one recruited site, it quickly became apparent that access was problematic because of competing demands on that organisation. Recognising the importance of full access and co-operative working for generating rich data, the research team decided not to pursue work at that site and instead to focus on other recruited sites.
Collecting the data
In order to develop a thorough understanding of the case, the case study approach usually involves the collection of multiple sources of evidence, using a range of quantitative (e.g. questionnaires, audits and analysis of routinely collected healthcare data) and more commonly qualitative techniques (e.g. interviews, focus groups and observations). The use of multiple sources of data (data triangulation) has been advocated as a way of increasing the internal validity of a study (i.e. the extent to which the method is appropriate to answer the research question)[ 8 , 18 – 21 ]. An underlying assumption is that data collected in different ways should lead to similar conclusions, and approaching the same issue from different angles can help develop a holistic picture of the phenomenon (Table 2 )[ 4 ].
Brazier and colleagues used a mixed-methods case study approach to investigate the impact of a cancer care programme[ 22 ]. Here, quantitative measures were collected with questionnaires before, and five months after, the start of the intervention which did not yield any statistically significant results. Qualitative interviews with patients however helped provide an insight into potentially beneficial process-related aspects of the programme, such as greater, perceived patient involvement in care. The authors reported how this case study approach provided a number of contextual factors likely to influence the effectiveness of the intervention and which were not likely to have been obtained from quantitative methods alone.
In collective or multiple case studies, data collection needs to be flexible enough to allow a detailed description of each individual case to be developed (e.g. the nature of different cancer care programmes), before considering the emerging similarities and differences in cross-case comparisons (e.g. to explore why one programme is more effective than another). It is important that data sources from different cases are, where possible, broadly comparable for this purpose even though they may vary in nature and depth.
Analysing, interpreting and reporting case studies
Making sense and offering a coherent interpretation of the typically disparate sources of data (whether qualitative alone or together with quantitative) is far from straightforward. Repeated reviewing and sorting of the voluminous and detail-rich data are integral to the process of analysis. In collective case studies, it is helpful to analyse data relating to the individual component cases first, before making comparisons across cases. Attention needs to be paid to variations within each case and, where relevant, the relationship between different causes, effects and outcomes[ 23 ]. Data will need to be organised and coded to allow the key issues, both derived from the literature and emerging from the dataset, to be easily retrieved at a later stage. An initial coding frame can help capture these issues and can be applied systematically to the whole dataset with the aid of a qualitative data analysis software package.
The Framework approach is a practical approach, comprising of five stages (familiarisation; identifying a thematic framework; indexing; charting; mapping and interpretation) , to managing and analysing large datasets particularly if time is limited, as was the case in our study of recruitment of South Asians into asthma research (Table 1 )[ 3 , 24 ]. Theoretical frameworks may also play an important role in integrating different sources of data and examining emerging themes. For example, we drew on a socio-technical framework to help explain the connections between different elements - technology; people; and the organisational settings within which they worked - in our study of the introduction of electronic health record systems (Table 3 )[ 5 ]. Our study of patient safety in undergraduate curricula drew on an evaluation-based approach to design and analysis, which emphasised the importance of the academic, organisational and practice contexts through which students learn (Table 4 )[ 6 ].
Case study findings can have implications both for theory development and theory testing. They may establish, strengthen or weaken historical explanations of a case and, in certain circumstances, allow theoretical (as opposed to statistical) generalisation beyond the particular cases studied[ 12 ]. These theoretical lenses should not, however, constitute a strait-jacket and the cases should not be "forced to fit" the particular theoretical framework that is being employed.
When reporting findings, it is important to provide the reader with enough contextual information to understand the processes that were followed and how the conclusions were reached. In a collective case study, researchers may choose to present the findings from individual cases separately before amalgamating across cases. Care must be taken to ensure the anonymity of both case sites and individual participants (if agreed in advance) by allocating appropriate codes or withholding descriptors. In the example given in Table 3 , we decided against providing detailed information on the NHS sites and individual participants in order to avoid the risk of inadvertent disclosure of identities[ 5 , 25 ].
What are the potential pitfalls and how can these be avoided?
The case study approach is, as with all research, not without its limitations. When investigating the formal and informal ways undergraduate students learn about patient safety (Table 4 ), for example, we rapidly accumulated a large quantity of data. The volume of data, together with the time restrictions in place, impacted on the depth of analysis that was possible within the available resources. This highlights a more general point of the importance of avoiding the temptation to collect as much data as possible; adequate time also needs to be set aside for data analysis and interpretation of what are often highly complex datasets.
Case study research has sometimes been criticised for lacking scientific rigour and providing little basis for generalisation (i.e. producing findings that may be transferable to other settings)[ 1 ]. There are several ways to address these concerns, including: the use of theoretical sampling (i.e. drawing on a particular conceptual framework); respondent validation (i.e. participants checking emerging findings and the researcher's interpretation, and providing an opinion as to whether they feel these are accurate); and transparency throughout the research process (see Table 8 )[ 8 , 18 – 21 , 23 , 26 ]. Transparency can be achieved by describing in detail the steps involved in case selection, data collection, the reasons for the particular methods chosen, and the researcher's background and level of involvement (i.e. being explicit about how the researcher has influenced data collection and interpretation). Seeking potential, alternative explanations, and being explicit about how interpretations and conclusions were reached, help readers to judge the trustworthiness of the case study report. Stake provides a critique checklist for a case study report (Table 9 )[ 8 ].
Conclusions
The case study approach allows, amongst other things, critical events, interventions, policy developments and programme-based service reforms to be studied in detail in a real-life context. It should therefore be considered when an experimental design is either inappropriate to answer the research questions posed or impossible to undertake. Considering the frequency with which implementations of innovations are now taking place in healthcare settings and how well the case study approach lends itself to in-depth, complex health service research, we believe this approach should be more widely considered by researchers. Though inherently challenging, the research case study can, if carefully conceptualised and thoughtfully undertaken and reported, yield powerful insights into many important aspects of health and healthcare delivery.
Yin RK: Case study research, design and method. 2009, London: Sage Publications Ltd., 4
Google Scholar
Keen J, Packwood T: Qualitative research; case study evaluation. BMJ. 1995, 311: 444-446.
Article CAS PubMed PubMed Central Google Scholar
Sheikh A, Halani L, Bhopal R, Netuveli G, Partridge M, Car J, et al: Facilitating the Recruitment of Minority Ethnic People into Research: Qualitative Case Study of South Asians and Asthma. PLoS Med. 2009, 6 (10): 1-11.
Article Google Scholar
Pinnock H, Huby G, Powell A, Kielmann T, Price D, Williams S, et al: The process of planning, development and implementation of a General Practitioner with a Special Interest service in Primary Care Organisations in England and Wales: a comparative prospective case study. Report for the National Co-ordinating Centre for NHS Service Delivery and Organisation R&D (NCCSDO). 2008, [ http://www.sdo.nihr.ac.uk/files/project/99-final-report.pdf ]
Robertson A, Cresswell K, Takian A, Petrakaki D, Crowe S, Cornford T, et al: Prospective evaluation of the implementation and adoption of NHS Connecting for Health's national electronic health record in secondary care in England: interim findings. BMJ. 2010, 41: c4564-
Pearson P, Steven A, Howe A, Sheikh A, Ashcroft D, Smith P, the Patient Safety Education Study Group: Learning about patient safety: organisational context and culture in the education of healthcare professionals. J Health Serv Res Policy. 2010, 15: 4-10. 10.1258/jhsrp.2009.009052.
Article PubMed Google Scholar
van Harten WH, Casparie TF, Fisscher OA: The evaluation of the introduction of a quality management system: a process-oriented case study in a large rehabilitation hospital. Health Policy. 2002, 60 (1): 17-37. 10.1016/S0168-8510(01)00187-7.
Stake RE: The art of case study research. 1995, London: Sage Publications Ltd.
Sheikh A, Smeeth L, Ashcroft R: Randomised controlled trials in primary care: scope and application. Br J Gen Pract. 2002, 52 (482): 746-51.
PubMed PubMed Central Google Scholar
King G, Keohane R, Verba S: Designing Social Inquiry. 1996, Princeton: Princeton University Press
Doolin B: Information technology as disciplinary technology: being critical in interpretative research on information systems. Journal of Information Technology. 1998, 13: 301-311. 10.1057/jit.1998.8.
George AL, Bennett A: Case studies and theory development in the social sciences. 2005, Cambridge, MA: MIT Press
Eccles M, the Improved Clinical Effectiveness through Behavioural Research Group (ICEBeRG): Designing theoretically-informed implementation interventions. Implementation Science. 2006, 1: 1-8. 10.1186/1748-5908-1-1.
Article PubMed Central Google Scholar
Netuveli G, Hurwitz B, Levy M, Fletcher M, Barnes G, Durham SR, Sheikh A: Ethnic variations in UK asthma frequency, morbidity, and health-service use: a systematic review and meta-analysis. Lancet. 2005, 365 (9456): 312-7.
Sheikh A, Panesar SS, Lasserson T, Netuveli G: Recruitment of ethnic minorities to asthma studies. Thorax. 2004, 59 (7): 634-
CAS PubMed PubMed Central Google Scholar
Hellström I, Nolan M, Lundh U: 'We do things together': A case study of 'couplehood' in dementia. Dementia. 2005, 4: 7-22. 10.1177/1471301205049188.
Som CV: Nothing seems to have changed, nothing seems to be changing and perhaps nothing will change in the NHS: doctors' response to clinical governance. International Journal of Public Sector Management. 2005, 18: 463-477. 10.1108/09513550510608903.
Lincoln Y, Guba E: Naturalistic inquiry. 1985, Newbury Park: Sage Publications
Barbour RS: Checklists for improving rigour in qualitative research: a case of the tail wagging the dog?. BMJ. 2001, 322: 1115-1117. 10.1136/bmj.322.7294.1115.
Mays N, Pope C: Qualitative research in health care: Assessing quality in qualitative research. BMJ. 2000, 320: 50-52. 10.1136/bmj.320.7226.50.
Mason J: Qualitative researching. 2002, London: Sage
Brazier A, Cooke K, Moravan V: Using Mixed Methods for Evaluating an Integrative Approach to Cancer Care: A Case Study. Integr Cancer Ther. 2008, 7: 5-17. 10.1177/1534735407313395.
Miles MB, Huberman M: Qualitative data analysis: an expanded sourcebook. 1994, CA: Sage Publications Inc., 2
Pope C, Ziebland S, Mays N: Analysing qualitative data. Qualitative research in health care. BMJ. 2000, 320: 114-116. 10.1136/bmj.320.7227.114.
Cresswell KM, Worth A, Sheikh A: Actor-Network Theory and its role in understanding the implementation of information technology developments in healthcare. BMC Med Inform Decis Mak. 2010, 10 (1): 67-10.1186/1472-6947-10-67.
Article PubMed PubMed Central Google Scholar
Malterud K: Qualitative research: standards, challenges, and guidelines. Lancet. 2001, 358: 483-488. 10.1016/S0140-6736(01)05627-6.
Article CAS PubMed Google Scholar
Yin R: Case study research: design and methods. 1994, Thousand Oaks, CA: Sage Publishing, 2
Yin R: Enhancing the quality of case studies in health services research. Health Serv Res. 1999, 34: 1209-1224.
Green J, Thorogood N: Qualitative methods for health research. 2009, Los Angeles: Sage, 2
Howcroft D, Trauth E: Handbook of Critical Information Systems Research, Theory and Application. 2005, Cheltenham, UK: Northampton, MA, USA: Edward Elgar
Book Google Scholar
Blakie N: Approaches to Social Enquiry. 1993, Cambridge: Polity Press
Doolin B: Power and resistance in the implementation of a medical management information system. Info Systems J. 2004, 14: 343-362. 10.1111/j.1365-2575.2004.00176.x.
Bloomfield BP, Best A: Management consultants: systems development, power and the translation of problems. Sociological Review. 1992, 40: 533-560.
Shanks G, Parr A: Positivist, single case study research in information systems: A critical analysis. Proceedings of the European Conference on Information Systems. 2003, Naples
Pre-publication history
The pre-publication history for this paper can be accessed here: http://www.biomedcentral.com/1471-2288/11/100/prepub
Download references
Acknowledgements
We are grateful to the participants and colleagues who contributed to the individual case studies that we have drawn on. This work received no direct funding, but it has been informed by projects funded by Asthma UK, the NHS Service Delivery Organisation, NHS Connecting for Health Evaluation Programme, and Patient Safety Research Portfolio. We would also like to thank the expert reviewers for their insightful and constructive feedback. Our thanks are also due to Dr. Allison Worth who commented on an earlier draft of this manuscript.
Author information
Authors and affiliations.
Division of Primary Care, The University of Nottingham, Nottingham, UK
Sarah Crowe & Anthony Avery
Centre for Population Health Sciences, The University of Edinburgh, Edinburgh, UK
Kathrin Cresswell, Ann Robertson & Aziz Sheikh
School of Health in Social Science, The University of Edinburgh, Edinburgh, UK
You can also search for this author in PubMed Google Scholar
Corresponding author
Correspondence to Sarah Crowe .
Additional information
Competing interests.
The authors declare that they have no competing interests.
Authors' contributions
AS conceived this article. SC, KC and AR wrote this paper with GH, AA and AS all commenting on various drafts. SC and AS are guarantors.
Rights and permissions
This article is published under license to BioMed Central Ltd. This is an Open Access article distributed under the terms of the Creative Commons Attribution License ( http://creativecommons.org/licenses/by/2.0 ), which permits unrestricted use, distribution, and reproduction in any medium, provided the original work is properly cited.
Reprints and permissions
About this article
Cite this article.
Crowe, S., Cresswell, K., Robertson, A. et al. The case study approach. BMC Med Res Methodol 11 , 100 (2011). https://doi.org/10.1186/1471-2288-11-100
Download citation
Received : 29 November 2010
Accepted : 27 June 2011
Published : 27 June 2011
DOI : https://doi.org/10.1186/1471-2288-11-100
Share this article
Anyone you share the following link with will be able to read this content:
Sorry, a shareable link is not currently available for this article.
Provided by the Springer Nature SharedIt content-sharing initiative
- Case Study Approach
- Electronic Health Record System
- Case Study Design
- Case Study Site
- Case Study Report
BMC Medical Research Methodology
ISSN: 1471-2288
- General enquiries: [email protected]

Case Study Research: Methods and Designs
Case study research is a type of qualitative research design. It’s often used in the social sciences because it involves…

Case study research is a type of qualitative research design. It’s often used in the social sciences because it involves observing subjects, or cases, in their natural setting, with minimal interference from the researcher.
In the case study method , researchers pose a specific question about an individual or group to test their theories or hypothesis. This can be done by gathering data from interviews with key informants.
Here’s what you need to know about case study research design .
What Is The Case Study Method?
Main approaches to data collection, case study research methods, how case studies are used, case study model.
Case study research is a great way to understand the nuances of a matter that can get lost in quantitative research methods. A case study is distinct from other qualitative studies in the following ways:
- It’s interested in the effect of a set of circumstances on an individual or group.
- It begins with a specific question about one or more cases.
- It focuses on individual accounts and experiences.
Here are the primary features of case study research:
- Case study research methods typically involve the researcher asking a few questions of one person or a small number of people—known as respondents—to test one hypothesis.
- Case study in research methodology may apply triangulation to collect data, in which the researcher uses several sources, including documents and field data. This is then analyzed and interpreted to form a hypothesis that can be tested through further research or validated by other researchers.
- The case study method requires clear concepts and theories to guide its methods. A well-defined research question is crucial when conducting a case study because the results of the study depend on it. The best approach to answering a research question is to challenge the existing theories, hypotheses or assumptions.
- Concepts are defined using objective language with no reference to preconceived notions that individuals might have about them. The researcher sets out to discover by asking specific questions on how people think or perceive things in their given situation.
They commonly use the case study method in business, management, psychology, sociology, political science and other related fields.
A fundamental requirement of qualitative research is recording observations that provide an understanding of reality. When it comes to the case study method, there are two major approaches that can be used to collect data: document review and fieldwork.
A case study in research methodology also includes literature review, the process by which the researcher collects all data available through historical documents. These might include books, newspapers, journals, videos, photographs and other written material. The researcher may also record information using video cameras to capture events as they occur. The researcher can also go through materials produced by people involved in the case study to gain an insight into their lives and experiences.
Field research involves participating in interviews and observations directly. Observation can be done during telephone interviews, events or public meetings, visits to homes or workplaces, or by shadowing someone for a period of time. The researcher can conduct one-on-one interviews with individuals or group interviews where several people are interviewed at once.
Let’s look now at case study methodology.
The case study method can be divided into three stages: formulation of objectives; collection of data; and analysis and interpretation. The researcher first makes a judgment about what should be studied based on their knowledge. Next, they gather data through observations and interviews. Here are some of the common case study research methods:
One of the most basic methods is the survey. Respondents are asked to complete a questionnaire with open-ended and predetermined questions. It usually takes place through face-to-face interviews, mailed questionnaires or telephone interviews. It can even be done by an online survey.
2. Semi-structured Interview
For case study research a more complex method is the semi-structured interview. This involves the researcher learning about the topic by listening to what others have to say. This usually occurs through one-on-one interviews with the sample. Semi-structured interviews allow for greater flexibility and can obtain information that structured questionnaires can’t.
3. Focus Group Interview
Another method is the focus group interview, where the researcher asks a few people to take part in an open-ended discussion on certain themes or topics. The typical group size is 5–15 people. This method allows researchers to delve deeper into people’s opinions, views and experiences.
4. Participant Observation
Participant observation is another method that involves the researcher gaining insight into an experience by joining in and taking part in normal events. The people involved don’t always know they’re being studied, but the researcher observes and records what happens through field notes.
Case study research design can use one or several of these methods depending on the context.
Case studies are widely used in the social sciences. To understand the impact of socio-economic forces, interpersonal dynamics and other human conditions, sometimes there’s no other way than to study one case at a time and look for patterns and data afterward.
It’s for the same reasons that case studies are used in business. Here are a few uses:
- Case studies can be used as tools to educate and give examples of situations and problems that might occur and how they were resolved. They can also be used for strategy development and implementation.
- Case studies can evaluate the success of a program or project. They can help teams improve their collaboration by identifying areas that need improvements, such as team dynamics, communication, roles and responsibilities and leadership styles.
- Case studies can explore how people’s experiences affect the working environment. Because the study involves observing and analyzing concrete details of life, they can inform theories on how an individual or group interacts with their environment.
- Case studies can evaluate the sustainability of businesses. They’re useful for social, environmental and economic impact studies because they look at all aspects of a business or organization. This gives researchers a holistic view of the dynamics within an organization.
- We can use case studies to identify problems in organizations or businesses. They can help spot problems that are invisible to customers, investors, managers and employees.
- Case studies are used in education to show students how real-world issues or events can be sorted out. This enables students to identify and deal with similar situations in their lives.
And that’s not all. Case studies are incredibly versatile, which is why they’re used so widely.
Human beings are complex and they interact with each other in their everyday life in various ways. The researcher observes a case and tries to find out how the patterns of behavior are created, including their causal relations. Case studies help understand one or more specific events that have been observed. Here are some common methods:
1. Illustrative case study
This is where the researcher observes a group of people doing something. Studying an event or phenomenon this way can show cause-and-effect relationships between various variables.
2. Cumulative case study
A cumulative case study is one that involves observing the same set of phenomena over a period. Cumulative case studies can be very helpful in understanding processes, which are things that happen over time. For example, if there are behavioral changes in people who move from one place to another, the researcher might want to know why these changes occurred.
3. Exploratory case study
An exploratory case study collects information that will answer a question. It can help researchers better understand social, economic, political or other social phenomena.
There are several other ways to categorize case studies. They may be chronological case studies, where a researcher observes events over time. In the comparative case study, the researcher compares one or more groups of people, places, or things to draw conclusions about them. In an intervention case study, the researcher intervenes to change the behavior of the subjects. The study method depends on the needs of the research team.
Deciding how to analyze the information at our disposal is an important part of effective management. An understanding of the case study model can help. With Harappa’s Thinking Critically course, managers and young professionals receive input and training on how to level up their analytic skills. Knowledge of frameworks, reading real-life examples and lived wisdom of faculty come together to create a dynamic and exciting course that helps teams leap to the next level.
Explore Harappa Diaries to learn more about topics such as Objectives Of Research , What are Qualitative Research Methods , How To Make A Problem Statement and How To Improve your Cognitive Skills to upgrade your knowledge and skills.
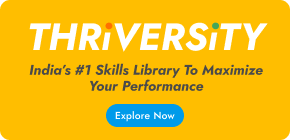

An official website of the United States government
The .gov means it’s official. Federal government websites often end in .gov or .mil. Before sharing sensitive information, make sure you’re on a federal government site.
The site is secure. The https:// ensures that you are connecting to the official website and that any information you provide is encrypted and transmitted securely.
- Publications
- Account settings
- My Bibliography
- Collections
- Citation manager
Save citation to file
Email citation, add to collections.
- Create a new collection
- Add to an existing collection
Add to My Bibliography
Your saved search, create a file for external citation management software, your rss feed.
- Search in PubMed
- Search in NLM Catalog
- Add to Search
Continuing to enhance the quality of case study methodology in health services research
Affiliations.
- 1 Faculty of Health Sciences, Western University, London, Ontario, Canada.
- 2 Department of Family Medicine, Schulich School of Medicine and Dentistry, Western University, London, Ontario, Canada.
- 3 The Schulich Interfaculty Program in Public Health, Schulich School of Medicine and Dentistry, Western University, London, Ontario, Canada.
- PMID: 34227408
- PMCID: PMC8392758
- DOI: 10.1177/08404704211028857
Case study methodology has grown in popularity within Health Services Research (HSR). However, its use and merit as a methodology are frequently criticized due to its flexible approach and inconsistent application. Nevertheless, case study methodology is well suited to HSR because it can track and examine complex relationships, contexts, and systems as they evolve. Applied appropriately, it can help generate information on how multiple forms of knowledge come together to inform decision-making within healthcare contexts. In this article, we aim to demystify case study methodology by outlining its philosophical underpinnings and three foundational approaches. We provide literature-based guidance to decision-makers, policy-makers, and health leaders on how to engage in and critically appraise case study design. We advocate that researchers work in collaboration with health leaders to detail their research process with an aim of strengthening the validity and integrity of case study for its continued and advanced use in HSR.
PubMed Disclaimer
Similar articles
- Health researchers and policy makers involvement in knowledge translation activities in Malawi. Mapulanga P, Raju J, Matingwina T. Mapulanga P, et al. J Health Organ Manag. 2019 Jun 28;33(4):380-395. doi: 10.1108/JHOM-01-2019-0008. Epub 2019 Jun 12. J Health Organ Manag. 2019. PMID: 31282816
- Decision-makers and the usefulness of research evidence in policy implementation--a case study from Lao PDR. Tomson G, Paphassarang C, Jönsson K, Houamboun K, Akkhavong K, Wahlström R. Tomson G, et al. Soc Sci Med. 2005 Sep;61(6):1291-9. doi: 10.1016/j.socscimed.2005.01.014. Epub 2005 Mar 5. Soc Sci Med. 2005. PMID: 15970238
- How is the use of research evidence in health policy perceived? A comparison between the reporting of researchers and policy-makers. Ellen ME, Lavis JN, Horowitz E, Berglas R. Ellen ME, et al. Health Res Policy Syst. 2018 Jul 20;16(1):64. doi: 10.1186/s12961-018-0345-6. Health Res Policy Syst. 2018. PMID: 30029647 Free PMC article.
- From theory to practice: improving the impact of health services research. Brazil K, Ozer E, Cloutier MM, Levine R, Stryer D. Brazil K, et al. BMC Health Serv Res. 2005 Jan 7;5:1. doi: 10.1186/1472-6963-5-1. BMC Health Serv Res. 2005. PMID: 15638931 Free PMC article. Review.
- Advancing the field of health systems research synthesis. Langlois EV, Ranson MK, Bärnighausen T, Bosch-Capblanch X, Daniels K, El-Jardali F, Ghaffar A, Grimshaw J, Haines A, Lavis JN, Lewin S, Meng Q, Oliver S, Pantoja T, Straus S, Shemilt I, Tovey D, Tugwell P, Waddington H, Wilson M, Yuan B, Røttingen JA. Langlois EV, et al. Syst Rev. 2015 Jul 10;4:90. doi: 10.1186/s13643-015-0080-9. Syst Rev. 2015. PMID: 26159806 Free PMC article. Review.
- "We were leery of going": qualitatively exploring Canadian international retirement migrants' travel-related decisions during the COVID-19 pandemic. Tate J, Crooks VA, Snyder J. Tate J, et al. Trop Dis Travel Med Vaccines. 2024 May 1;10(1):9. doi: 10.1186/s40794-024-00218-z. Trop Dis Travel Med Vaccines. 2024. PMID: 38689364 Free PMC article.
- Partnership-building considerations for implementation science in learning health systems: a case study of the Implementation Science Collaborative in Alberta, Canada. Brooks SP, Alba C, Thomson D, Davison SN, Storey K. Brooks SP, et al. Front Health Serv. 2024 Feb 16;4:1327395. doi: 10.3389/frhs.2024.1327395. eCollection 2024. Front Health Serv. 2024. PMID: 38433991 Free PMC article.
- Exploring patient safety risk in an emergency ward for substance use through a mixed-method analysis. Svensson J. Svensson J. BMC Health Serv Res. 2024 Jan 31;24(1):153. doi: 10.1186/s12913-024-10621-z. BMC Health Serv Res. 2024. PMID: 38297311 Free PMC article.
- Reconceptualizing Integrated Knowledge Translation goals: a case study on basic and clinical science investigating the causes and consequences of food allergy. Dixon J, Shantz E, Clarke AE, Elliott SJ. Dixon J, et al. Implement Sci Commun. 2023 Sep 27;4(1):120. doi: 10.1186/s43058-023-00473-9. Implement Sci Commun. 2023. PMID: 37759275 Free PMC article.
- Harrison H, Birks M, Franklin R, Mills J.Case study research: foundations and methodological orientations. Forum Qual Soc Res. 2017;18(1):19.
- Peirson L, Ciliska D, Dobbins M, Mowat D. Building capacity for evidence informed decision making in public health: a case study of organizational change. BMC Public Health. 2012;12(1):137. doi:10.1186/1471-2458-12-137 - PMC - PubMed
- Edwards N, Barker PM. The importance of context in implementation research. J Acquir Immune Defic Syndr. 2014;67(suppl 2):S157–S162. - PubMed
- Milat AJ, King L, Bauman AE, Redman S.The concept of scalability: increasing the scale and potential adoption of health promotion interventions into policy and practice. Health Prompt Int. 2013;28(3):285–298. - PubMed
- Stake RE. The Art of Case Study Research. SAGE; 1995.
- Search in MeSH
LinkOut - more resources
Full text sources.
- Europe PubMed Central
- PubMed Central
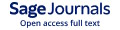
- Citation Manager
NCBI Literature Resources
MeSH PMC Bookshelf Disclaimer
The PubMed wordmark and PubMed logo are registered trademarks of the U.S. Department of Health and Human Services (HHS). Unauthorized use of these marks is strictly prohibited.

An official website of the United States government
Here's how you know
Official websites use .gov A .gov website belongs to an official government organization in the United States.
Secure .gov websites use HTTPS A lock ( Lock A locked padlock ) or https:// means you’ve safely connected to the .gov website. Share sensitive information only on official, secure websites.
- Data and Tools
- Publications
- Forestcast Podcast
- Urban Forest Connections
- First Friday All Climate Change Talks
21st Century Wildlife Monitoring – A Case Study of Spotted Owls and Artificial Intelligence
Wildlife monitoring programs, especially those with long-term datasets, are invaluable for informing forest management and conservation decisions in the face of climate change. But monitoring can be costly in terms of time, labor, and money. New technology is ushering in exciting possibilities that can help long-term monitoring programs evolve and benefit from next generation methods. Monitoring northern spotted owls provides an excellent case study.
Research wildlife biologist Damon Lesmeister ’s research integrates artificial intelligence and passive acoustic monitoring. This new approach is both effective and minimally intrusive to the owls. Tune in to hear how passive acoustic monitoring can detect spotted owls and their competitors, estimate occupancy rates, detect trends in population levels, and even distinguish between male and female birds.
This promising development in monitoring methodology could be a game changer for forest managers as they strive to track trends in spotted owls and monitor biodiversity in dynamic landscapes. It could facilitate a biodiversity monitoring program with unprecedented flexibility and scale and will likely provide insights in the future that are unimaginable today.

Damon B. Lesmeister

An official website of the United States government
The .gov means it’s official. Federal government websites often end in .gov or .mil. Before sharing sensitive information, make sure you’re on a federal government site.
The site is secure. The https:// ensures that you are connecting to the official website and that any information you provide is encrypted and transmitted securely.
- Publications
- Account settings
Preview improvements coming to the PMC website in October 2024. Learn More or Try it out now .
- Advanced Search
- Journal List
- BMC Med Res Methodol

The case study approach
Sarah crowe.
1 Division of Primary Care, The University of Nottingham, Nottingham, UK
Kathrin Cresswell
2 Centre for Population Health Sciences, The University of Edinburgh, Edinburgh, UK
Ann Robertson
3 School of Health in Social Science, The University of Edinburgh, Edinburgh, UK
Anthony Avery
Aziz sheikh.
The case study approach allows in-depth, multi-faceted explorations of complex issues in their real-life settings. The value of the case study approach is well recognised in the fields of business, law and policy, but somewhat less so in health services research. Based on our experiences of conducting several health-related case studies, we reflect on the different types of case study design, the specific research questions this approach can help answer, the data sources that tend to be used, and the particular advantages and disadvantages of employing this methodological approach. The paper concludes with key pointers to aid those designing and appraising proposals for conducting case study research, and a checklist to help readers assess the quality of case study reports.
Introduction
The case study approach is particularly useful to employ when there is a need to obtain an in-depth appreciation of an issue, event or phenomenon of interest, in its natural real-life context. Our aim in writing this piece is to provide insights into when to consider employing this approach and an overview of key methodological considerations in relation to the design, planning, analysis, interpretation and reporting of case studies.
The illustrative 'grand round', 'case report' and 'case series' have a long tradition in clinical practice and research. Presenting detailed critiques, typically of one or more patients, aims to provide insights into aspects of the clinical case and, in doing so, illustrate broader lessons that may be learnt. In research, the conceptually-related case study approach can be used, for example, to describe in detail a patient's episode of care, explore professional attitudes to and experiences of a new policy initiative or service development or more generally to 'investigate contemporary phenomena within its real-life context' [ 1 ]. Based on our experiences of conducting a range of case studies, we reflect on when to consider using this approach, discuss the key steps involved and illustrate, with examples, some of the practical challenges of attaining an in-depth understanding of a 'case' as an integrated whole. In keeping with previously published work, we acknowledge the importance of theory to underpin the design, selection, conduct and interpretation of case studies[ 2 ]. In so doing, we make passing reference to the different epistemological approaches used in case study research by key theoreticians and methodologists in this field of enquiry.
This paper is structured around the following main questions: What is a case study? What are case studies used for? How are case studies conducted? What are the potential pitfalls and how can these be avoided? We draw in particular on four of our own recently published examples of case studies (see Tables Tables1, 1 , ,2, 2 , ,3 3 and and4) 4 ) and those of others to illustrate our discussion[ 3 - 7 ].
Example of a case study investigating the reasons for differences in recruitment rates of minority ethnic people in asthma research[ 3 ]
Minority ethnic people experience considerably greater morbidity from asthma than the White majority population. Research has shown however that these minority ethnic populations are likely to be under-represented in research undertaken in the UK; there is comparatively less marginalisation in the US. |
To investigate approaches to bolster recruitment of South Asians into UK asthma studies through qualitative research with US and UK researchers, and UK community leaders. |
Single intrinsic case study |
Centred on the issue of recruitment of South Asian people with asthma. |
In-depth interviews were conducted with asthma researchers from the UK and US. A supplementary questionnaire was also provided to researchers. |
Framework approach. |
Barriers to ethnic minority recruitment were found to centre around: |
1. The attitudes of the researchers' towards inclusion: The majority of UK researchers interviewed were generally supportive of the idea of recruiting ethnically diverse participants but expressed major concerns about the practicalities of achieving this; in contrast, the US researchers appeared much more committed to the policy of inclusion. |
2. Stereotypes and prejudices: We found that some of the UK researchers' perceptions of ethnic minorities may have influenced their decisions on whether to approach individuals from particular ethnic groups. These stereotypes centred on issues to do with, amongst others, language barriers and lack of altruism. |
3. Demographic, political and socioeconomic contexts of the two countries: Researchers suggested that the demographic profile of ethnic minorities, their political engagement and the different configuration of the health services in the UK and the US may have contributed to differential rates. |
4. Above all, however, it appeared that the overriding importance of the US National Institute of Health's policy to mandate the inclusion of minority ethnic people (and women) had a major impact on shaping the attitudes and in turn the experiences of US researchers'; the absence of any similar mandate in the UK meant that UK-based researchers had not been forced to challenge their existing practices and they were hence unable to overcome any stereotypical/prejudicial attitudes through experiential learning. |
Example of a case study investigating the process of planning and implementing a service in Primary Care Organisations[ 4 ]
Health work forces globally are needing to reorganise and reconfigure in order to meet the challenges posed by the increased numbers of people living with long-term conditions in an efficient and sustainable manner. Through studying the introduction of General Practitioners with a Special Interest in respiratory disorders, this study aimed to provide insights into this important issue by focusing on community respiratory service development. |
To understand and compare the process of workforce change in respiratory services and the impact on patient experience (specifically in relation to the role of general practitioners with special interests) in a theoretically selected sample of Primary Care Organisations (PCOs), in order to derive models of good practice in planning and the implementation of a broad range of workforce issues. |
Multiple-case design of respiratory services in health regions in England and Wales. |
Four PCOs. |
Face-to-face and telephone interviews, e-mail discussions, local documents, patient diaries, news items identified from local and national websites, national workshop. |
Reading, coding and comparison progressed iteratively. |
1. In the screening phase of this study (which involved semi-structured telephone interviews with the person responsible for driving the reconfiguration of respiratory services in 30 PCOs), the barriers of financial deficit, organisational uncertainty, disengaged clinicians and contradictory policies proved insurmountable for many PCOs to developing sustainable services. A key rationale for PCO re-organisation in 2006 was to strengthen their commissioning function and those of clinicians through Practice-Based Commissioning. However, the turbulence, which surrounded reorganisation was found to have the opposite desired effect. |
2. Implementing workforce reconfiguration was strongly influenced by the negotiation and contest among local clinicians and managers about "ownership" of work and income. |
3. Despite the intention to make the commissioning system more transparent, personal relationships based on common professional interests, past work history, friendships and collegiality, remained as key drivers for sustainable innovation in service development. |
It was only possible to undertake in-depth work in a selective number of PCOs and, even within these selected PCOs, it was not possible to interview all informants of potential interest and/or obtain all relevant documents. This work was conducted in the early stages of a major NHS reorganisation in England and Wales and thus, events are likely to have continued to evolve beyond the study period; we therefore cannot claim to have seen any of the stories through to their conclusion. |
Example of a case study investigating the introduction of the electronic health records[ 5 ]
Healthcare systems globally are moving from paper-based record systems to electronic health record systems. In 2002, the NHS in England embarked on the most ambitious and expensive IT-based transformation in healthcare in history seeking to introduce electronic health records into all hospitals in England by 2010. |
To describe and evaluate the implementation and adoption of detailed electronic health records in secondary care in England and thereby provide formative feedback for local and national rollout of the NHS Care Records Service. |
A mixed methods, longitudinal, multi-site, socio-technical collective case study. |
Five NHS acute hospital and mental health Trusts that have been the focus of early implementation efforts. |
Semi-structured interviews, documentary data and field notes, observations and quantitative data. |
Qualitative data were analysed thematically using a socio-technical coding matrix, combined with additional themes that emerged from the data. |
1. Hospital electronic health record systems have developed and been implemented far more slowly than was originally envisioned. |
2. The top-down, government-led standardised approach needed to evolve to admit more variation and greater local choice for hospitals in order to support local service delivery. |
3. A range of adverse consequences were associated with the centrally negotiated contracts, which excluded the hospitals in question. |
4. The unrealistic, politically driven, timeline (implementation over 10 years) was found to be a major source of frustration for developers, implementers and healthcare managers and professionals alike. |
We were unable to access details of the contracts between government departments and the Local Service Providers responsible for delivering and implementing the software systems. This, in turn, made it difficult to develop a holistic understanding of some key issues impacting on the overall slow roll-out of the NHS Care Record Service. Early adopters may also have differed in important ways from NHS hospitals that planned to join the National Programme for Information Technology and implement the NHS Care Records Service at a later point in time. |
Example of a case study investigating the formal and informal ways students learn about patient safety[ 6 ]
There is a need to reduce the disease burden associated with iatrogenic harm and considering that healthcare education represents perhaps the most sustained patient safety initiative ever undertaken, it is important to develop a better appreciation of the ways in which undergraduate and newly qualified professionals receive and make sense of the education they receive. | |
---|---|
To investigate the formal and informal ways pre-registration students from a range of healthcare professions (medicine, nursing, physiotherapy and pharmacy) learn about patient safety in order to become safe practitioners. | |
Multi-site, mixed method collective case study. | |
: Eight case studies (two for each professional group) were carried out in educational provider sites considering different programmes, practice environments and models of teaching and learning. | |
Structured in phases relevant to the three knowledge contexts: | |
Documentary evidence (including undergraduate curricula, handbooks and module outlines), complemented with a range of views (from course leads, tutors and students) and observations in a range of academic settings. | |
Policy and management views of patient safety and influences on patient safety education and practice. NHS policies included, for example, implementation of the National Patient Safety Agency's , which encourages organisations to develop an organisational safety culture in which staff members feel comfortable identifying dangers and reporting hazards. | |
The cultures to which students are exposed i.e. patient safety in relation to day-to-day working. NHS initiatives included, for example, a hand washing initiative or introduction of infection control measures. | |
1. Practical, informal, learning opportunities were valued by students. On the whole, however, students were not exposed to nor engaged with important NHS initiatives such as risk management activities and incident reporting schemes. | |
2. NHS policy appeared to have been taken seriously by course leaders. Patient safety materials were incorporated into both formal and informal curricula, albeit largely implicit rather than explicit. | |
3. Resource issues and peer pressure were found to influence safe practice. Variations were also found to exist in students' experiences and the quality of the supervision available. | |
The curriculum and organisational documents collected differed between sites, which possibly reflected gatekeeper influences at each site. The recruitment of participants for focus group discussions proved difficult, so interviews or paired discussions were used as a substitute. |
What is a case study?
A case study is a research approach that is used to generate an in-depth, multi-faceted understanding of a complex issue in its real-life context. It is an established research design that is used extensively in a wide variety of disciplines, particularly in the social sciences. A case study can be defined in a variety of ways (Table (Table5), 5 ), the central tenet being the need to explore an event or phenomenon in depth and in its natural context. It is for this reason sometimes referred to as a "naturalistic" design; this is in contrast to an "experimental" design (such as a randomised controlled trial) in which the investigator seeks to exert control over and manipulate the variable(s) of interest.
Definitions of a case study
Author | Definition |
---|---|
Stake[ ] | (p.237) |
Yin[ , , ] | (Yin 1999 p. 1211, Yin 1994 p. 13) |
• | |
• (Yin 2009 p18) | |
Miles and Huberman[ ] | (p. 25) |
Green and Thorogood[ ] | (p. 284) |
George and Bennett[ ] | (p. 17)" |
Stake's work has been particularly influential in defining the case study approach to scientific enquiry. He has helpfully characterised three main types of case study: intrinsic , instrumental and collective [ 8 ]. An intrinsic case study is typically undertaken to learn about a unique phenomenon. The researcher should define the uniqueness of the phenomenon, which distinguishes it from all others. In contrast, the instrumental case study uses a particular case (some of which may be better than others) to gain a broader appreciation of an issue or phenomenon. The collective case study involves studying multiple cases simultaneously or sequentially in an attempt to generate a still broader appreciation of a particular issue.
These are however not necessarily mutually exclusive categories. In the first of our examples (Table (Table1), 1 ), we undertook an intrinsic case study to investigate the issue of recruitment of minority ethnic people into the specific context of asthma research studies, but it developed into a instrumental case study through seeking to understand the issue of recruitment of these marginalised populations more generally, generating a number of the findings that are potentially transferable to other disease contexts[ 3 ]. In contrast, the other three examples (see Tables Tables2, 2 , ,3 3 and and4) 4 ) employed collective case study designs to study the introduction of workforce reconfiguration in primary care, the implementation of electronic health records into hospitals, and to understand the ways in which healthcare students learn about patient safety considerations[ 4 - 6 ]. Although our study focusing on the introduction of General Practitioners with Specialist Interests (Table (Table2) 2 ) was explicitly collective in design (four contrasting primary care organisations were studied), is was also instrumental in that this particular professional group was studied as an exemplar of the more general phenomenon of workforce redesign[ 4 ].
What are case studies used for?
According to Yin, case studies can be used to explain, describe or explore events or phenomena in the everyday contexts in which they occur[ 1 ]. These can, for example, help to understand and explain causal links and pathways resulting from a new policy initiative or service development (see Tables Tables2 2 and and3, 3 , for example)[ 1 ]. In contrast to experimental designs, which seek to test a specific hypothesis through deliberately manipulating the environment (like, for example, in a randomised controlled trial giving a new drug to randomly selected individuals and then comparing outcomes with controls),[ 9 ] the case study approach lends itself well to capturing information on more explanatory ' how ', 'what' and ' why ' questions, such as ' how is the intervention being implemented and received on the ground?'. The case study approach can offer additional insights into what gaps exist in its delivery or why one implementation strategy might be chosen over another. This in turn can help develop or refine theory, as shown in our study of the teaching of patient safety in undergraduate curricula (Table (Table4 4 )[ 6 , 10 ]. Key questions to consider when selecting the most appropriate study design are whether it is desirable or indeed possible to undertake a formal experimental investigation in which individuals and/or organisations are allocated to an intervention or control arm? Or whether the wish is to obtain a more naturalistic understanding of an issue? The former is ideally studied using a controlled experimental design, whereas the latter is more appropriately studied using a case study design.
Case studies may be approached in different ways depending on the epistemological standpoint of the researcher, that is, whether they take a critical (questioning one's own and others' assumptions), interpretivist (trying to understand individual and shared social meanings) or positivist approach (orientating towards the criteria of natural sciences, such as focusing on generalisability considerations) (Table (Table6). 6 ). Whilst such a schema can be conceptually helpful, it may be appropriate to draw on more than one approach in any case study, particularly in the context of conducting health services research. Doolin has, for example, noted that in the context of undertaking interpretative case studies, researchers can usefully draw on a critical, reflective perspective which seeks to take into account the wider social and political environment that has shaped the case[ 11 ].
Example of epistemological approaches that may be used in case study research
Approach | Characteristics | Criticisms | Key references |
---|---|---|---|
Involves questioning one's own assumptions taking into account the wider political and social environment. | It can possibly neglect other factors by focussing only on power relationships and may give the researcher a position that is too privileged. | Howcroft and Trauth[ ] Blakie[ ] Doolin[ , ] | |
Interprets the limiting conditions in relation to power and control that are thought to influence behaviour. | Bloomfield and Best[ ] | ||
Involves understanding meanings/contexts and processes as perceived from different perspectives, trying to understand individual and shared social meanings. Focus is on theory building. | Often difficult to explain unintended consequences and for neglecting surrounding historical contexts | Stake[ ] Doolin[ ] | |
Involves establishing which variables one wishes to study in advance and seeing whether they fit in with the findings. Focus is often on testing and refining theory on the basis of case study findings. | It does not take into account the role of the researcher in influencing findings. | Yin[ , , ] Shanks and Parr[ ] |
How are case studies conducted?
Here, we focus on the main stages of research activity when planning and undertaking a case study; the crucial stages are: defining the case; selecting the case(s); collecting and analysing the data; interpreting data; and reporting the findings.
Defining the case
Carefully formulated research question(s), informed by the existing literature and a prior appreciation of the theoretical issues and setting(s), are all important in appropriately and succinctly defining the case[ 8 , 12 ]. Crucially, each case should have a pre-defined boundary which clarifies the nature and time period covered by the case study (i.e. its scope, beginning and end), the relevant social group, organisation or geographical area of interest to the investigator, the types of evidence to be collected, and the priorities for data collection and analysis (see Table Table7 7 )[ 1 ]. A theory driven approach to defining the case may help generate knowledge that is potentially transferable to a range of clinical contexts and behaviours; using theory is also likely to result in a more informed appreciation of, for example, how and why interventions have succeeded or failed[ 13 ].
Example of a checklist for rating a case study proposal[ 8 ]
Clarity: Does the proposal read well? | |
Integrity: Do its pieces fit together? | |
Attractiveness: Does it pique the reader's interest? | |
The case: Is the case adequately defined? | |
The issues: Are major research questions identified? | |
Data Resource: Are sufficient data sources identified? | |
Case Selection: Is the selection plan reasonable? | |
Data Gathering: Are data-gathering activities outlined? | |
Validation: Is the need and opportunity for triangulation indicated? | |
Access: Are arrangements for start-up anticipated? | |
Confidentiality: Is there sensitivity to the protection of people? | |
Cost: Are time and resource estimates reasonable? |
For example, in our evaluation of the introduction of electronic health records in English hospitals (Table (Table3), 3 ), we defined our cases as the NHS Trusts that were receiving the new technology[ 5 ]. Our focus was on how the technology was being implemented. However, if the primary research interest had been on the social and organisational dimensions of implementation, we might have defined our case differently as a grouping of healthcare professionals (e.g. doctors and/or nurses). The precise beginning and end of the case may however prove difficult to define. Pursuing this same example, when does the process of implementation and adoption of an electronic health record system really begin or end? Such judgements will inevitably be influenced by a range of factors, including the research question, theory of interest, the scope and richness of the gathered data and the resources available to the research team.
Selecting the case(s)
The decision on how to select the case(s) to study is a very important one that merits some reflection. In an intrinsic case study, the case is selected on its own merits[ 8 ]. The case is selected not because it is representative of other cases, but because of its uniqueness, which is of genuine interest to the researchers. This was, for example, the case in our study of the recruitment of minority ethnic participants into asthma research (Table (Table1) 1 ) as our earlier work had demonstrated the marginalisation of minority ethnic people with asthma, despite evidence of disproportionate asthma morbidity[ 14 , 15 ]. In another example of an intrinsic case study, Hellstrom et al.[ 16 ] studied an elderly married couple living with dementia to explore how dementia had impacted on their understanding of home, their everyday life and their relationships.
For an instrumental case study, selecting a "typical" case can work well[ 8 ]. In contrast to the intrinsic case study, the particular case which is chosen is of less importance than selecting a case that allows the researcher to investigate an issue or phenomenon. For example, in order to gain an understanding of doctors' responses to health policy initiatives, Som undertook an instrumental case study interviewing clinicians who had a range of responsibilities for clinical governance in one NHS acute hospital trust[ 17 ]. Sampling a "deviant" or "atypical" case may however prove even more informative, potentially enabling the researcher to identify causal processes, generate hypotheses and develop theory.
In collective or multiple case studies, a number of cases are carefully selected. This offers the advantage of allowing comparisons to be made across several cases and/or replication. Choosing a "typical" case may enable the findings to be generalised to theory (i.e. analytical generalisation) or to test theory by replicating the findings in a second or even a third case (i.e. replication logic)[ 1 ]. Yin suggests two or three literal replications (i.e. predicting similar results) if the theory is straightforward and five or more if the theory is more subtle. However, critics might argue that selecting 'cases' in this way is insufficiently reflexive and ill-suited to the complexities of contemporary healthcare organisations.
The selected case study site(s) should allow the research team access to the group of individuals, the organisation, the processes or whatever else constitutes the chosen unit of analysis for the study. Access is therefore a central consideration; the researcher needs to come to know the case study site(s) well and to work cooperatively with them. Selected cases need to be not only interesting but also hospitable to the inquiry [ 8 ] if they are to be informative and answer the research question(s). Case study sites may also be pre-selected for the researcher, with decisions being influenced by key stakeholders. For example, our selection of case study sites in the evaluation of the implementation and adoption of electronic health record systems (see Table Table3) 3 ) was heavily influenced by NHS Connecting for Health, the government agency that was responsible for overseeing the National Programme for Information Technology (NPfIT)[ 5 ]. This prominent stakeholder had already selected the NHS sites (through a competitive bidding process) to be early adopters of the electronic health record systems and had negotiated contracts that detailed the deployment timelines.
It is also important to consider in advance the likely burden and risks associated with participation for those who (or the site(s) which) comprise the case study. Of particular importance is the obligation for the researcher to think through the ethical implications of the study (e.g. the risk of inadvertently breaching anonymity or confidentiality) and to ensure that potential participants/participating sites are provided with sufficient information to make an informed choice about joining the study. The outcome of providing this information might be that the emotive burden associated with participation, or the organisational disruption associated with supporting the fieldwork, is considered so high that the individuals or sites decide against participation.
In our example of evaluating implementations of electronic health record systems, given the restricted number of early adopter sites available to us, we sought purposively to select a diverse range of implementation cases among those that were available[ 5 ]. We chose a mixture of teaching, non-teaching and Foundation Trust hospitals, and examples of each of the three electronic health record systems procured centrally by the NPfIT. At one recruited site, it quickly became apparent that access was problematic because of competing demands on that organisation. Recognising the importance of full access and co-operative working for generating rich data, the research team decided not to pursue work at that site and instead to focus on other recruited sites.
Collecting the data
In order to develop a thorough understanding of the case, the case study approach usually involves the collection of multiple sources of evidence, using a range of quantitative (e.g. questionnaires, audits and analysis of routinely collected healthcare data) and more commonly qualitative techniques (e.g. interviews, focus groups and observations). The use of multiple sources of data (data triangulation) has been advocated as a way of increasing the internal validity of a study (i.e. the extent to which the method is appropriate to answer the research question)[ 8 , 18 - 21 ]. An underlying assumption is that data collected in different ways should lead to similar conclusions, and approaching the same issue from different angles can help develop a holistic picture of the phenomenon (Table (Table2 2 )[ 4 ].
Brazier and colleagues used a mixed-methods case study approach to investigate the impact of a cancer care programme[ 22 ]. Here, quantitative measures were collected with questionnaires before, and five months after, the start of the intervention which did not yield any statistically significant results. Qualitative interviews with patients however helped provide an insight into potentially beneficial process-related aspects of the programme, such as greater, perceived patient involvement in care. The authors reported how this case study approach provided a number of contextual factors likely to influence the effectiveness of the intervention and which were not likely to have been obtained from quantitative methods alone.
In collective or multiple case studies, data collection needs to be flexible enough to allow a detailed description of each individual case to be developed (e.g. the nature of different cancer care programmes), before considering the emerging similarities and differences in cross-case comparisons (e.g. to explore why one programme is more effective than another). It is important that data sources from different cases are, where possible, broadly comparable for this purpose even though they may vary in nature and depth.
Analysing, interpreting and reporting case studies
Making sense and offering a coherent interpretation of the typically disparate sources of data (whether qualitative alone or together with quantitative) is far from straightforward. Repeated reviewing and sorting of the voluminous and detail-rich data are integral to the process of analysis. In collective case studies, it is helpful to analyse data relating to the individual component cases first, before making comparisons across cases. Attention needs to be paid to variations within each case and, where relevant, the relationship between different causes, effects and outcomes[ 23 ]. Data will need to be organised and coded to allow the key issues, both derived from the literature and emerging from the dataset, to be easily retrieved at a later stage. An initial coding frame can help capture these issues and can be applied systematically to the whole dataset with the aid of a qualitative data analysis software package.
The Framework approach is a practical approach, comprising of five stages (familiarisation; identifying a thematic framework; indexing; charting; mapping and interpretation) , to managing and analysing large datasets particularly if time is limited, as was the case in our study of recruitment of South Asians into asthma research (Table (Table1 1 )[ 3 , 24 ]. Theoretical frameworks may also play an important role in integrating different sources of data and examining emerging themes. For example, we drew on a socio-technical framework to help explain the connections between different elements - technology; people; and the organisational settings within which they worked - in our study of the introduction of electronic health record systems (Table (Table3 3 )[ 5 ]. Our study of patient safety in undergraduate curricula drew on an evaluation-based approach to design and analysis, which emphasised the importance of the academic, organisational and practice contexts through which students learn (Table (Table4 4 )[ 6 ].
Case study findings can have implications both for theory development and theory testing. They may establish, strengthen or weaken historical explanations of a case and, in certain circumstances, allow theoretical (as opposed to statistical) generalisation beyond the particular cases studied[ 12 ]. These theoretical lenses should not, however, constitute a strait-jacket and the cases should not be "forced to fit" the particular theoretical framework that is being employed.
When reporting findings, it is important to provide the reader with enough contextual information to understand the processes that were followed and how the conclusions were reached. In a collective case study, researchers may choose to present the findings from individual cases separately before amalgamating across cases. Care must be taken to ensure the anonymity of both case sites and individual participants (if agreed in advance) by allocating appropriate codes or withholding descriptors. In the example given in Table Table3, 3 , we decided against providing detailed information on the NHS sites and individual participants in order to avoid the risk of inadvertent disclosure of identities[ 5 , 25 ].
What are the potential pitfalls and how can these be avoided?
The case study approach is, as with all research, not without its limitations. When investigating the formal and informal ways undergraduate students learn about patient safety (Table (Table4), 4 ), for example, we rapidly accumulated a large quantity of data. The volume of data, together with the time restrictions in place, impacted on the depth of analysis that was possible within the available resources. This highlights a more general point of the importance of avoiding the temptation to collect as much data as possible; adequate time also needs to be set aside for data analysis and interpretation of what are often highly complex datasets.
Case study research has sometimes been criticised for lacking scientific rigour and providing little basis for generalisation (i.e. producing findings that may be transferable to other settings)[ 1 ]. There are several ways to address these concerns, including: the use of theoretical sampling (i.e. drawing on a particular conceptual framework); respondent validation (i.e. participants checking emerging findings and the researcher's interpretation, and providing an opinion as to whether they feel these are accurate); and transparency throughout the research process (see Table Table8 8 )[ 8 , 18 - 21 , 23 , 26 ]. Transparency can be achieved by describing in detail the steps involved in case selection, data collection, the reasons for the particular methods chosen, and the researcher's background and level of involvement (i.e. being explicit about how the researcher has influenced data collection and interpretation). Seeking potential, alternative explanations, and being explicit about how interpretations and conclusions were reached, help readers to judge the trustworthiness of the case study report. Stake provides a critique checklist for a case study report (Table (Table9 9 )[ 8 ].
Potential pitfalls and mitigating actions when undertaking case study research
Potential pitfall | Mitigating action |
---|---|
Selecting/conceptualising the wrong case(s) resulting in lack of theoretical generalisations | Developing in-depth knowledge of theoretical and empirical literature, justifying choices made |
Collecting large volumes of data that are not relevant to the case or too little to be of any value | Focus data collection in line with research questions, whilst being flexible and allowing different paths to be explored |
Defining/bounding the case | Focus on related components (either by time and/or space), be clear what is outside the scope of the case |
Lack of rigour | Triangulation, respondent validation, the use of theoretical sampling, transparency throughout the research process |
Ethical issues | Anonymise appropriately as cases are often easily identifiable to insiders, informed consent of participants |
Integration with theoretical framework | Allow for unexpected issues to emerge and do not force fit, test out preliminary explanations, be clear about epistemological positions in advance |
Stake's checklist for assessing the quality of a case study report[ 8 ]
1. Is this report easy to read? |
2. Does it fit together, each sentence contributing to the whole? |
3. Does this report have a conceptual structure (i.e. themes or issues)? |
4. Are its issues developed in a series and scholarly way? |
5. Is the case adequately defined? |
6. Is there a sense of story to the presentation? |
7. Is the reader provided some vicarious experience? |
8. Have quotations been used effectively? |
9. Are headings, figures, artefacts, appendices, indexes effectively used? |
10. Was it edited well, then again with a last minute polish? |
11. Has the writer made sound assertions, neither over- or under-interpreting? |
12. Has adequate attention been paid to various contexts? |
13. Were sufficient raw data presented? |
14. Were data sources well chosen and in sufficient number? |
15. Do observations and interpretations appear to have been triangulated? |
16. Is the role and point of view of the researcher nicely apparent? |
17. Is the nature of the intended audience apparent? |
18. Is empathy shown for all sides? |
19. Are personal intentions examined? |
20. Does it appear individuals were put at risk? |
Conclusions
The case study approach allows, amongst other things, critical events, interventions, policy developments and programme-based service reforms to be studied in detail in a real-life context. It should therefore be considered when an experimental design is either inappropriate to answer the research questions posed or impossible to undertake. Considering the frequency with which implementations of innovations are now taking place in healthcare settings and how well the case study approach lends itself to in-depth, complex health service research, we believe this approach should be more widely considered by researchers. Though inherently challenging, the research case study can, if carefully conceptualised and thoughtfully undertaken and reported, yield powerful insights into many important aspects of health and healthcare delivery.
Competing interests
The authors declare that they have no competing interests.
Authors' contributions
AS conceived this article. SC, KC and AR wrote this paper with GH, AA and AS all commenting on various drafts. SC and AS are guarantors.
Pre-publication history
The pre-publication history for this paper can be accessed here:
http://www.biomedcentral.com/1471-2288/11/100/prepub
Acknowledgements
We are grateful to the participants and colleagues who contributed to the individual case studies that we have drawn on. This work received no direct funding, but it has been informed by projects funded by Asthma UK, the NHS Service Delivery Organisation, NHS Connecting for Health Evaluation Programme, and Patient Safety Research Portfolio. We would also like to thank the expert reviewers for their insightful and constructive feedback. Our thanks are also due to Dr. Allison Worth who commented on an earlier draft of this manuscript.
- Yin RK. Case study research, design and method. 4. London: Sage Publications Ltd.; 2009. [ Google Scholar ]
- Keen J, Packwood T. Qualitative research; case study evaluation. BMJ. 1995; 311 :444–446. [ PMC free article ] [ PubMed ] [ Google Scholar ]
- Sheikh A, Halani L, Bhopal R, Netuveli G, Partridge M, Car J. et al. Facilitating the Recruitment of Minority Ethnic People into Research: Qualitative Case Study of South Asians and Asthma. PLoS Med. 2009; 6 (10):1–11. [ PMC free article ] [ PubMed ] [ Google Scholar ]
- Pinnock H, Huby G, Powell A, Kielmann T, Price D, Williams S, The process of planning, development and implementation of a General Practitioner with a Special Interest service in Primary Care Organisations in England and Wales: a comparative prospective case study. Report for the National Co-ordinating Centre for NHS Service Delivery and Organisation R&D (NCCSDO) 2008. http://www.sdo.nihr.ac.uk/files/project/99-final-report.pdf
- Robertson A, Cresswell K, Takian A, Petrakaki D, Crowe S, Cornford T. et al. Prospective evaluation of the implementation and adoption of NHS Connecting for Health's national electronic health record in secondary care in England: interim findings. BMJ. 2010; 41 :c4564. [ PMC free article ] [ PubMed ] [ Google Scholar ]
- Pearson P, Steven A, Howe A, Sheikh A, Ashcroft D, Smith P. the Patient Safety Education Study Group. Learning about patient safety: organisational context and culture in the education of healthcare professionals. J Health Serv Res Policy. 2010; 15 :4–10. doi: 10.1258/jhsrp.2009.009052. [ PubMed ] [ CrossRef ] [ Google Scholar ]
- van Harten WH, Casparie TF, Fisscher OA. The evaluation of the introduction of a quality management system: a process-oriented case study in a large rehabilitation hospital. Health Policy. 2002; 60 (1):17–37. doi: 10.1016/S0168-8510(01)00187-7. [ PubMed ] [ CrossRef ] [ Google Scholar ]
- Stake RE. The art of case study research. London: Sage Publications Ltd.; 1995. [ Google Scholar ]
- Sheikh A, Smeeth L, Ashcroft R. Randomised controlled trials in primary care: scope and application. Br J Gen Pract. 2002; 52 (482):746–51. [ PMC free article ] [ PubMed ] [ Google Scholar ]
- King G, Keohane R, Verba S. Designing Social Inquiry. Princeton: Princeton University Press; 1996. [ Google Scholar ]
- Doolin B. Information technology as disciplinary technology: being critical in interpretative research on information systems. Journal of Information Technology. 1998; 13 :301–311. doi: 10.1057/jit.1998.8. [ CrossRef ] [ Google Scholar ]
- George AL, Bennett A. Case studies and theory development in the social sciences. Cambridge, MA: MIT Press; 2005. [ Google Scholar ]
- Eccles M. the Improved Clinical Effectiveness through Behavioural Research Group (ICEBeRG) Designing theoretically-informed implementation interventions. Implementation Science. 2006; 1 :1–8. doi: 10.1186/1748-5908-1-1. [ PMC free article ] [ PubMed ] [ CrossRef ] [ Google Scholar ]
- Netuveli G, Hurwitz B, Levy M, Fletcher M, Barnes G, Durham SR, Sheikh A. Ethnic variations in UK asthma frequency, morbidity, and health-service use: a systematic review and meta-analysis. Lancet. 2005; 365 (9456):312–7. [ PubMed ] [ Google Scholar ]
- Sheikh A, Panesar SS, Lasserson T, Netuveli G. Recruitment of ethnic minorities to asthma studies. Thorax. 2004; 59 (7):634. [ PMC free article ] [ PubMed ] [ Google Scholar ]
- Hellström I, Nolan M, Lundh U. 'We do things together': A case study of 'couplehood' in dementia. Dementia. 2005; 4 :7–22. doi: 10.1177/1471301205049188. [ CrossRef ] [ Google Scholar ]
- Som CV. Nothing seems to have changed, nothing seems to be changing and perhaps nothing will change in the NHS: doctors' response to clinical governance. International Journal of Public Sector Management. 2005; 18 :463–477. doi: 10.1108/09513550510608903. [ CrossRef ] [ Google Scholar ]
- Lincoln Y, Guba E. Naturalistic inquiry. Newbury Park: Sage Publications; 1985. [ Google Scholar ]
- Barbour RS. Checklists for improving rigour in qualitative research: a case of the tail wagging the dog? BMJ. 2001; 322 :1115–1117. doi: 10.1136/bmj.322.7294.1115. [ PMC free article ] [ PubMed ] [ CrossRef ] [ Google Scholar ]
- Mays N, Pope C. Qualitative research in health care: Assessing quality in qualitative research. BMJ. 2000; 320 :50–52. doi: 10.1136/bmj.320.7226.50. [ PMC free article ] [ PubMed ] [ CrossRef ] [ Google Scholar ]
- Mason J. Qualitative researching. London: Sage; 2002. [ Google Scholar ]
- Brazier A, Cooke K, Moravan V. Using Mixed Methods for Evaluating an Integrative Approach to Cancer Care: A Case Study. Integr Cancer Ther. 2008; 7 :5–17. doi: 10.1177/1534735407313395. [ PubMed ] [ CrossRef ] [ Google Scholar ]
- Miles MB, Huberman M. Qualitative data analysis: an expanded sourcebook. 2. CA: Sage Publications Inc.; 1994. [ Google Scholar ]
- Pope C, Ziebland S, Mays N. Analysing qualitative data. Qualitative research in health care. BMJ. 2000; 320 :114–116. doi: 10.1136/bmj.320.7227.114. [ PMC free article ] [ PubMed ] [ CrossRef ] [ Google Scholar ]
- Cresswell KM, Worth A, Sheikh A. Actor-Network Theory and its role in understanding the implementation of information technology developments in healthcare. BMC Med Inform Decis Mak. 2010; 10 (1):67. doi: 10.1186/1472-6947-10-67. [ PMC free article ] [ PubMed ] [ CrossRef ] [ Google Scholar ]
- Malterud K. Qualitative research: standards, challenges, and guidelines. Lancet. 2001; 358 :483–488. doi: 10.1016/S0140-6736(01)05627-6. [ PubMed ] [ CrossRef ] [ Google Scholar ]
- Yin R. Case study research: design and methods. 2. Thousand Oaks, CA: Sage Publishing; 1994. [ Google Scholar ]
- Yin R. Enhancing the quality of case studies in health services research. Health Serv Res. 1999; 34 :1209–1224. [ PMC free article ] [ PubMed ] [ Google Scholar ]
- Green J, Thorogood N. Qualitative methods for health research. 2. Los Angeles: Sage; 2009. [ Google Scholar ]
- Howcroft D, Trauth E. Handbook of Critical Information Systems Research, Theory and Application. Cheltenham, UK: Northampton, MA, USA: Edward Elgar; 2005. [ Google Scholar ]
- Blakie N. Approaches to Social Enquiry. Cambridge: Polity Press; 1993. [ Google Scholar ]
- Doolin B. Power and resistance in the implementation of a medical management information system. Info Systems J. 2004; 14 :343–362. doi: 10.1111/j.1365-2575.2004.00176.x. [ CrossRef ] [ Google Scholar ]
- Bloomfield BP, Best A. Management consultants: systems development, power and the translation of problems. Sociological Review. 1992; 40 :533–560. [ Google Scholar ]
- Shanks G, Parr A. Proceedings of the European Conference on Information Systems. Naples; 2003. Positivist, single case study research in information systems: A critical analysis. [ Google Scholar ]
Constructing a Novel Network Structure Weighting Technique into the ANP Decision Support System for Optimal Alternative Evaluation: A Case Study on Crowdfunding Tokenization for Startup Financing
- Research Article
- Open access
- Published: 26 August 2024
- Volume 17 , article number 222 , ( 2024 )
Cite this article
You have full access to this open access article
- Chun-Yueh Lin 1
This study constructed a novel decision-making framework for startup companies to evaluate token financing options. A Network structure weighting (NSW) technique was developed and integrated with the analytic network process (ANP) to create a comprehensive assessment model. This innovative approach addressed the limitations of traditional multi-criteria decision-making methods by effectively capturing the complex interdependencies between factors influencing token financing decisions. The proposed model comprises three main steps: (1) utilizing a modified Delphi method to identify key factors affecting token financing, (2) developing the NSW technique to determine the network structure of these factors, and (3) integrating the NSW results into the ANP model to evaluate and rank the critical factors and alternatives. This study applied this framework to assess three token financing alternatives: Initial Coin Offerings (ICO), Initial Exchange Offerings (IEO), and Security Token Offerings (STO). The results indicate that STO is the optimal financing alternative for the analyzed startup scenario in token financing, followed by Initial Exchange Offerings and Initial Coin Offerings. The model identified platform fees, issuance costs, and financing success rate as the three most critical factors influencing the decision. This study contributes to both methodology and practice in FinTech decision-making. The NSW-ANP framework offers a more robust approach to modeling complex financial decisions, while the application to token financing provides valuable insights for startup companies navigating this emerging funding landscape. The proposed framework lays the groundwork for more informed and structured decision-making in the rapidly evolving field of cryptocurrency-based financing.
Explore related subjects
- Artificial Intelligence
Avoid common mistakes on your manuscript.
1 Introduction
Due to the rise and development of Financial Technology (FinTech), as well as the enactment of the Jumpstart Our Business Startups (JOBS) in the U.S. [ 1 ], crowdfunding has become the newest financing means for enterprises in need of external funds [ 2 , 3 ]. In 2014, the total amount of funds raised through crowdfunding reached USD 16.2 billion, which was 167% higher than that of 2013 [ 4 ]. In addition, according to the statistical results of Statista Inc. (2020) [ 5 ], the total amount of alternative financing in 2020 was USD 6.1 billion, among which crowdfunding accounted for the largest market share. For this reason, it could be said that the development scale of crowdfunding in the global financial market has been rocketing.
Crowdfunding involves a number of different forms. The first form is donation-based crowdfunding, which mainly means to raise charity funds for the implementation of programs and projects. The second form is rewards-based crowdfunding, in which the investor can receive non-monetary rewards because of capital contributions. The third form is debt-based crowdfunding, in which the relevant interest arrangements between the investor and the fundraiser are determined in line with credit contracts. The fourth form is equity-based crowdfunding, in which the fundraiser uses the equities of the target company to exchange funds from the investor, while the investor receives such equities and therefore is entitled to that company’s revenues or dividends [ 6 , 7 , 8 ]. Estrin et al. [ 9 ] pointed out that equity-based crowdfunding depends mainly on the Internet or social network platforms. This fund-raising method not only reduces the transaction cost but also stands for a new business pattern under which startup companies can establish their own goodwill and provide investors with opportunities for investment. Although crowdfunding has many advantages for startup companies, risks do exist, including uncertainty of equity ownership, lack of liquidity, and damage to stockholder equity [ 10 , 11 , 12 ]. For this reason, past studies suggested that startup companies might obtain funds by offering tokens on the basis of distributed ledger technology and the immutability of blockchains. This not only could reduce the potential risks of traditional fundraising platforms but also could promote the transparency level of the relevant transactions [ 12 , 13 , 14 ]. Howell et al. [ 15 ] indicated that token financing has become one of the important sources for enterprises to raise funds through digital platforms. Presently, the development of crowdfunding tokenization mainly involves three patterns: (1) initial coin offerings (ICO), (2) initial exchange offerings (IEO), and (3) security token offerings (STO). ICO has the advantages of low cost and high speed. However, the risks of theft and fraud exist [ 15 , 16 , 17 ]. The advantages of IEO include having the business reputation of a third-party platform as a guarantee and handling the relevant transactions directly on the transaction platform. However, the possibility of the token price being manipulated cannot be ruled out [ 17 , 18 ]. The last pattern, STO, has the advantages of the highest level of safety and of being protected by the rules and regulations of regional governments. However, the high complexity of examination and verification as well as excessively low liquidity are problems that cannot be avoided [ 17 , 19 ]. The research results of past literature also show that for startup companies, the efficiency of token financing is higher than that of equity financing [ 20 ]. Furthermore, Chod et al. [ 14 ] pointed out that enterprises may take advantage of the decentralization features of token financing to make it more convenient for token investors in their project investments and reduce the cost of encouraging token investors to join the investment platforms. In this way, it is easier for entrepreneurs in raising funds.
For this reason, the utilization of token financing for the purpose of raising operation efficiency has become an important business strategy. The aforesaid three patterns of crowdfunding tokenization have their respective advantages and disadvantages, as well as potential risks. If startup companies intend to raise funds through virtual currencies, the alternatives of financing in cryptocurrency will affect the financing efficiency and lead to the capital turnover issue. Previous studies on token financing focused more on risk-return analysis [ 21 , 22 , 23 , 24 ], token rules and regulations [ 25 , 26 , 27 ], hedging of tokens [ 28 , 29 , 30 , 31 ], and prediction of price in tokens [ 32 , 33 , 34 , 35 ]. However, there is scarce evidence and a lack of applicable measurement tools in regard to assessing the optimal solution for the token financing of startup companies. Hence, algorithms for multiple criteria decision-making can be utilized for the construction of assessment models, so that the optimal solution for assessment can be reached [ 36 , 37 , 38 ]. Past studies also suggested that the optimal solution can be solved using the analytic hierarchy process (AHP) [ 38 , 39 , 40 , 41 , 42 ]. Although AHP can be used to assess the optimal solutions in different fields, it is unsuitable to use traditional AHP methods for decision-making problems in real situations. AHP is characterized by a hierarchical structure and based upon the presumption that the variables or criteria are independent from each other. Numerous problems relating to the assessment of optimal solutions and the relevant variables are correlated to or dependent on each other; as a result, complicated internal relationships cannot be solved through hierarchical or independent methods [ 43 , 44 ]. To solve this problem, Saaty [ 45 ] proposed the analytic network process (ANP), which added a feedback mechanism and interdependency to the AHP method to solve the problems of a lack of correlation and interdependency. ANP does not require the linear relationship of traditional AHP methods, which is top-down, and can establish an assessment pattern of networked relationships. Past literature has applied ANP models in the assessment of different industries, such as traffic problems [ 46 , 47 ], environment and energy assessment [ 48 , 49 , 50 ], filtration and selection of suppliers [ 51 , 52 , 53 ], and assessment of risk factors [ 54 , 55 , 56 ]. Thus, it can be seen that the problem of correlation or interdependency between criteria or variables cannot be solved effectively through AHP during decision-making, while ANP can effectively solve this problem. Although ANP can overcome the difficulties related to the presumption of independence in AHP, the ANP algorithm cannot ascertain the strength of the dependence and relationships between variables needed to generate a network structure. Previous studies addressing the network structure issue have applied deep machine learning concepts, as demonstrated by Moghaddasi et al., Gharehchopogh et al., and subsequent works by Moghaddasi et al. [ 57 , 58 , 59 , 60 , 61 ]. However, these studies primarily focused on the relationship in the Internet of Things, implicitly highlighting the challenges in applying such approaches to multi-criteria decision-making (MCDM) problems. Additionally, several studies employed the Decision-Making Trial and Evaluation Laboratory (DEMATEL) method to resolve network structures among criteria [ 62 , 63 , 64 , 65 , 66 ]. This approach offers an alternative perspective on capturing complex interrelationships within decision-making frameworks. However, the DEMATEL method has several limitations. First, the relationships derived through DEMATEL may be biased or misleading [ 67 , 68 ]. Additionally, the method faces convergence issues, as it cannot determine relationships between criteria when the data fail to converge [ 69 ]. As evident from Table 1 , there are two primary gaps in the existing literature. First, in terms of network structure methodology, while ANP, DEMATEL, and other decision-making frameworks have been proposed, they each have limitations. Second, regarding the research problem, while many studies have examined different aspects of token financing, there is a notable absence of comprehensive, quantitative decision-making frameworks specifically designed for startup companies evaluating token financing alternatives. In view of the above, this study developed a new network structure weighting (NSW) model, and then integrated NSW into ANP to remedy ANP’s shortcoming of being unable to determine the network structure. Finally, case studies were carried out to assess the optimal solution for startup companies engaging in token financing.
For the proposed NSW-ANP model, the modified Delphi method was utilized to determine the clusters and factors influencing startup companies engaging in token financing. Then, the network structure of these clusters and factors was determined based on the NSW method. Finally, the ANP model was utilized to calculate the weights of various factors and financing schemes for startup companies engaging in token financing and then sequence them to determine the optimal token financing schemes and their key factors. While ANP has been applied in various fields, this study proposed the first application of an enhanced ANP approach (integrated with NSW) to evaluate the token financing options for startups. This novel application demonstrates the versatility and effectiveness of our integrated approach in addressing complex FinTech decision-making scenarios.
This study makes significant contributions to the existing literature in both methodological innovations and novel applications. In terms of methodological advancements, we introduce a novel NSW technique that quantifies the strength of relationships between decision factors in a network structure. Furthermore, we develop an integrated NSW-ANP framework that enhances the capabilities of the traditional ANP by incorporating a more robust method for determining network relationships. With regard to novel applications, this study breaks new ground in two key areas. Firstly, we apply this integrated NSW-ANP framework to evaluate token financing options for startup companies, an area that has not been addressed using such a comprehensive decision-making approach. Secondly, this study provides the first systematic evaluation of ICO, IEO, and STO using a multi-criteria decision-making framework. This framework resolves the complex interdependencies between various factors, offering a more nuanced understanding of these emerging financing mechanisms. By combining methodological innovation with practical application in an emerging field, this study not only advances the theoretical understanding of multi-criteria decision-making processes but also provides valuable insights for practitioners in cryptocurrency-based startup financing. Academically, the new NSW-ANP model put forward in this study could be used for determining the network relationship of a research structure, and be integrated into the ANP to remedy the ANP’s shortcomings. The new integrated decision-making pattern put forward in this study also could provide valuable references for the measurement of the interdependency and correlation among variables in the assessment of the optimal solution of token financing for startup companies. Practically, the proposed framework could provide startup companies with a measurement tool containing a network structure and is valuable, so as to determine the optimal solution of token financing for startup companies introducing token financing to their businesses.
The remainder of this paper is organized as follows: Sect. 1 is the introduction, Sect. 2 describes the research method, Sect. 3 presents the case study, and Sect. 4 offers the conclusions.
2 Methodology
In this study, the clusters and factors were acquired through collecting experts’ opinions and literature reviews via modified Delphi method (MDM) as a first step. Next, the network structure of the clusters and factors was determined on the basis of the network structure weighting (NSW) method. Finally, the analytic network process (ANP) model was utilized to calculate and sequence the weightings of the various factors and financing schemes of startup companies engaging in token financing so that the most suitable token financing scheme and the key factors could be determined. The research method is presented in the following sections.
The Delphi method is an anonymous technique of decision-making by a group of experts. To solve a certain problem or find a solution for a particular future event, these experts are treated as the appraisal targets. For the final goal of reaching a stable group consensus among the experts, the group members are anonymous to each other, and particular procedures and repetitive steps are employed. The Delphi method attempts to combine the knowledge, opinions, and speculative abilities of experts in the field in an interruption-free environment. The Delphi method can be used to deduce what will happen in the future, effectively predict future trends, or reach a consensus over a certain issue [ 70 , 71 ]. This method is based upon the judgment of experts, and multiple rounds of opinion feedback are utilized to solve complicated decision-making problems. The traditional Delphi method emphasizes the following five basic principles [ 72 , 73 ]:
The principle of anonymity: All experts voice their opinions as individuals, and they remain anonymous when doing so.
Iteration: The questionnaire issuer gathers up the experts’ opinions and sends them to other experts. This step is carried out repeatedly.
Controlled feedback: In each round, the experts are required to answer pre-designed questionnaires, and the results are served as references for the next appraisal.
Statistical group responses: Comprehensive judgments are made only after the statistics of all the experts’ opinions are conducted.
Expert consensus: The ultimate goal is to reach a consensus after the experts’ opinions are consolidated.
The procedures of the Delphi method are as follows [ 74 ]:
Select the anonymous experts.
Carry out the first round of the questionnaire survey.
Carry out the second round of the questionnaire survey.
Carry out the third round of the questionnaire survey.
Consolidate the experts’ opinions and reach a consensus.
According to the modified Delphi method, Steps C and D are carried out repeatedly until a consensus is reached among the experts, and the number of experts should be between five and nine [ 75 , 76 ].
In this study, the experts’ opinions were gathered through the Delphi method and the relevant literature was discussed, so that the clusters and factors influencing startup companies engaging in token financing could be obtained.
2.2 NSW Model
This study utilized the Delphi method to collect the clusters and factors that could influence startup companies engaging in token financing schemes. In order to effectively carry out the calculation and assessment of ANP, the network structure of these clusters and factors need to be determined as a prerequisite for the subsequent filtration and selection of the optimal token financing scheme. Therefore, this study put forward the NSW method in order to acquire the relationships and the structure chart between clusters and factors. The NSW procedure is as follows:
Step 1: Collect and confirm the decision factors
The collection and confirmation of the decision factors can be realized through common tools such as literature reviews, the Delphi method, focus group interviews, and brainstorming. When decision-makers or experts need to determine n assessment factors that are consistent with the decision-making issues, the n assessment factors may be defined as \(\{ C_{1} ,C_{2} , \ldots ,C_{n} \}\) .
Step 2: Design the questionnaire
As far as the n factors determined by the decision makers or experts in Step 1 are concerned, a nine-point Likert scale can be utilized to ascertain the correlation and correlation strength between the factors. In the event of n factors, n ( n − 1) comparisons in line with the scale need to be carried out.
Step 3: Calculate the weight of the network structure
Each expert compares and scores the decision factors. After that, all the comparison scores of the experts are used in the matrix construction and weighted calculation. The procedure is as follows:
2.2.1 Establish the Matrix of the Network Correlation and the Correlation Diagram
The correlation matrix is established as M , while \(\{ C_{1} ,C_{2} , \ldots ,C_{n} \}\) are the decision factors. If C i is influenced by C j , \(m_{ij}\) will be the scores of a quantitative judgment given by experts. On the contrary, if \(m_{ij} = 0\) , C i is not influenced by C j . The results can be shown in matrix M ( n × n ) as follows:
The column aggregation and row aggregation of matrix M are:
\({\text{Column}}_{j}\) and \({\text{row}}_{i}\) , respectively, give the scores of factor j , which affects other factors, or factor j , which is influenced by other factors.
2.2.2 Define the Transition Probability Matrix
If transition matrix A is defined by the features of the Markov chain, A = ( a ij ), as shown in Eq. ( 2 ). A is a regular Markov matrix, and the existence of stationary distribution \(x = \left( {x_{1} ,x_{2} , \ldots ,x_{N} } \right)^{T}\) satisfies Ax = x and \(\sum\nolimits_{i} {x_{i} = 1}\) . The characteristic value of 1 can be acquired through the characteristic vector corresponding to the characteristic value of Matrix A , or through the iteration method \(x^{0}\) , where \(x^{k + 1} = Ax^{k}\) , to obtain the characteristic value. x stands for the distribution of probabilities of the various factors being influenced when the transition number approaches infinity, and \(x_{i}\) stands for the network node score of the i th factor.
2.2.3 Calculate the Weightings of the Network Structure
According to the results described in II above, the network node score of each factor is distributed to the correlation diagram of each expert ( n experts have n correlation diagrams). Afterwards, based on the node score of factor i , the strength score of each expert’s factor i influencing other factor j goes through a standardized distribution using the correlation diagram to obtain each expert’s weighted value of the network structure, R, as shown in Eq. ( 3 ). In the end, the \(R(C_{i} ,C_{j} )\) of n experts is averaged and standardized, as shown in Eq. ( 4 ) and Eq. ( 5 ). The standardized results can then be integrated into the ANP model to assess the optimal token financing scheme for startup companies.
Saaty put forward ANP in 1996. This method is rendered through a network structure and derived from an ANP. Practically, there are many questions about decision-making assessment that are not limited to expressing their complex interrelated properties in a hierarchical and independent manner, and they are not of purely linear relationships either. Rather, these questions have a network-like structure [ 45 , 77 , 78 , 79 ]. Based on the original presumption and prerequisite of the analytic hierarchy process (AHP), Saaty [ 45 ] integrated relationship and feedback mechanisms into the AHP model to solve the problem of correlation between different principles.
Saaty pointed out that the relationships of interactive influence between clusters and elements can be analyzed in a graphic manner. Such relationships and interactive influence can be demonstrated through arrow lines [ 45 , 80 ], as shown in Fig. 1 . This network structure is crucial for understanding the fundamental difference between hierarchical and network-based decision-making models. Unlike traditional hierarchical structures, this network allows for complex interdependencies between different elements of the decision-making process. In Fig. 1 , the bidirectional arrows indicate that influence can flow both ways between clusters, reflecting real-world complexities where factors can mutually affect each other.
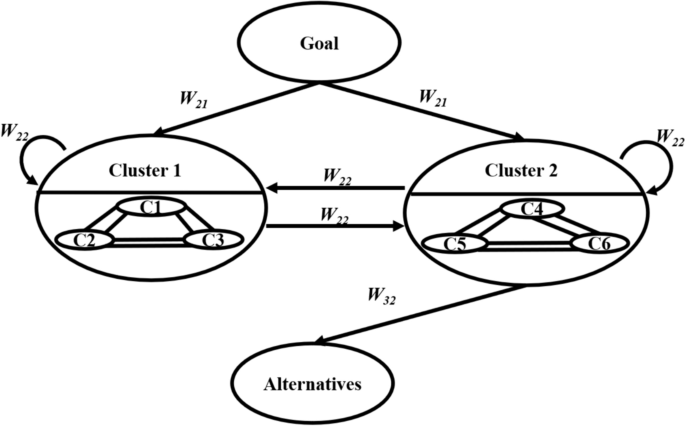
Source : Ref. [ 45 ]
The network structure.
According to the relationships and strengths of different factors in the aforesaid models and structure charts of ANP, a supermatrix is utilized for demonstration, as shown in Fig. 2 . This matrix is a critical component of the ANP, allowing for the quantification of relationships between all elements in the network. It is formed when the various clusters and respective factors contained in such clusters are listed on the left side and upper part of the matrix in an orderly manner. The supermatrix consists of a number of sub-matrices, which are formulated based on the eigenvectors after the comparison of different factors. In Fig. 2 , \(W_{11} ,W_{kk} , \ldots ,W_{nn}\) are the values of the eigenvectors after the comparisons and calculations.
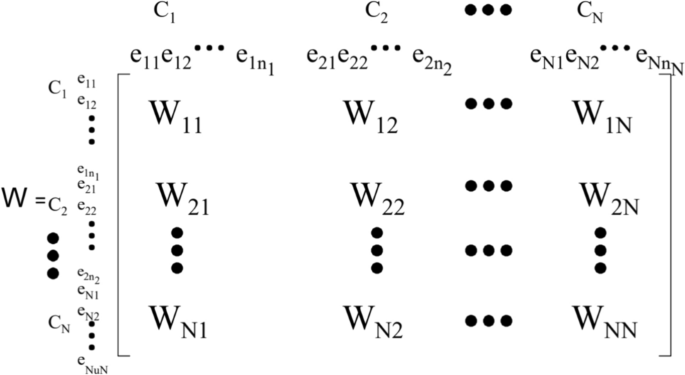
Source : Refs. [ 45 ] [ 80 ]
The supermatrix of a network.
ANP is an algorithm based on AHP and can be divided into four steps. In Step 1, the structures are formed step by step. In Step 2, the questions are raised. In Step 3, comparisons of interdependent clusters are made in pairs and a supermatrix is formed. In Step 4, the ultimate choice and optimal scheme are selected [ 45 , 79 ].
This study apples the ANP as the foundation of our approach due to several key advantages it offers in the context of complex decision-making scenarios. First, it is well-suited for this application because it allows for the consideration of interdependencies and feedback relationships between decision factors, which is crucial in the dynamic and interconnected world of FinTech and token financing. Furthermore, it provides a structured approach to incorporating both qualitative and quantitative factors into the decision-making process. This is particularly beneficial when evaluating token financing options, as it allows us to consider both qualitative and quantitative data. Finally, it is able to prioritize alternatives based on a comprehensive set of criteria and sub-criteria. This is especially valuable when comparing different alternatives, each of which has its own unique set of characteristics and implications. ANP allows for a more comprehensive comparison than simpler decision-making tools. Among various MCDM techniques, the ANP has a superior capacity to model complex systems with intricate interdependencies. While other MCDM techniques, such as the Technique for Order of Preference by Similarity to Ideal Solution (TOPSIS) and the VIseKriterijumska Optimizacija I Kompromisno Resenje (VIKOR) method, offer effective means for ranking alternatives, they exhibit limitations in accounting for the multifaceted interrelationships among criteria.
Consequently, this study employs the ANP method as the foundation for constructing an integrated decision-making model. A brief introduction of the construction program of the network process pattern is as follows:
Step 1: Confirm the research problems and network structure
Determine the targets according to features of the problems and search for decision-making clusters, as well as the factors contained in the various clusters by employing the proposed NSW method to acquire the influencing strength of the various factors; finally, draw the network structure models of the decision-making problems according to the results of NSW.
Step 2: Create pair-wise comparison matrices and priority vectors
Compare the factors in pairs. This step has two parts: the comparison of clusters (in pairs) and the comparison of factors within clusters (in pairs). The comparison of factors within clusters (in pairs) can be divided into the comparison within a particular group and comparisons among different clusters. The assessment scale of the comparison is similar to that of AHP. In addition, the eigenvectors, which are reached through the various comparison matrices, serve as the values of the supermatrix, which can be used to illustrate the interdependency and relative significance among the clusters. Equation ( 6 ) can be utilized to calculate the scores of relative significance in regard to the various clusters and factors. As for the strength of the interdependency among the clusters and among the factors, NSW can be utilized to determine the network structure (as described in Sect. 2.2 .)
Step 3: Construct the supermatrix
The supermatrix can effectively solve problems related to the interdependency among the various clusters and factors within the system (as shown in Fig. 2 ). The values of the supermatrix consist of small matrices, which include the comparison of different factors (in pairs) and the comparison of interdependent factors (in pairs). The numerical values of clusters or factors without the influence of feedback are 0, as shown in Eq. ( 7 ). In this study, it was suggested that the overall network structure could be confirmed by NSW. For this reason, the NSW results were integrated into the supermatrix for subsequent assessment and to determine the strength of the interdependency in the supermatrix, as shown in Eq. ( 8 ).
The ANP calculation process includes three matrices: the unweighted supermatrix, the weighted supermatrix, and the limit supermatrix. The unweighted supermatrix stands for the weightings of the original results of the comparison in pairs. In the weighted supermatrix, the weighted values of a particular element within an unweighted matrix are multiplied by the weighted values of the relevant clusters. In the limit supermatrix, the weighted matrix multiplies itself repeatedly until a stable state is attained. According to ANP, if supermatrix W is in an irreducible state of stability, all columns in the supermatrix will have similar vectors, indicating convergence can be attained. The ultimate weighted values of each cluster, factor, and scheme can be calculated through Eq. ( 9 ) during the convergence process.
Step 4: Evaluate the optimal alternative
Through the ANP framework and the calculations of the unweighted supermatrix, weighted supermatrix, and limit supermatrix, all the alternative schemes, as well as the ultimate values of the groups and factors, can be attained in the limit supermatrix. The ultimate results of the weighted values are then ranked to determine the optimal scheme.
3 Case Study
This study aimed to establish the network structure weighting (NSW) model by integrating NSW into the analytic network process (ANP) and establishing an assessment pattern to analyze the optimal scheme of token financing for startup companies, as well as the weighted values of clusters and factors. The consolidation-type diagram of the analytical process is shown in Fig. 3 . This integrated framework is a key innovation, that employs the Modified Delphi Method to identify relevant factors, and applies the NSW technique to determine the network structure. The results are then integrated into the ANP model for final calculations and analysis. This integrated approach addresses the limitations of traditional ANP by providing a more robust and objective method for determining network relationships. It combines the strengths of expert knowledge (through the Delphi method), systematic relationship quantification (via NSW), and comprehensive decision analysis (through ANP), resulting in a more reliable and nuanced decision-making tool for token financing. First, the modified Delphi method was utilized to calculate the clusters and factors influencing startup companies engaging in token financing. Second, the network structure of the clusters and factors was determined on the basis of the NSW method put forward in this study. Finally, the weighted values of the network structure of NSW were integrated into the ANP model to calculate the weighted values for the various factors and various financing schemes of startup companies engaging in token financing. These weighted values were then sequenced to obtain the optimal scheme and key factors of token financing. Figure 4 presents the integrated framework for evaluating token financing options. This model incorporates five main clusters: Finance, Laws and Regulations, Risk, Investor, and Online Community, each containing several specific factors. The model also includes three token financing alternatives: ICO, IEO, and STO. This structure allows for a comprehensive evaluation of token financing alternatives, considering a wide range of relevant factors. By inclusion of diverse clusters including financial considerations, as well as legal, risk-related, investor-focused, and community aspects, the proposed framework allows startup companies to make well-informed decisions based on a thorough analysis of all relevant factors.
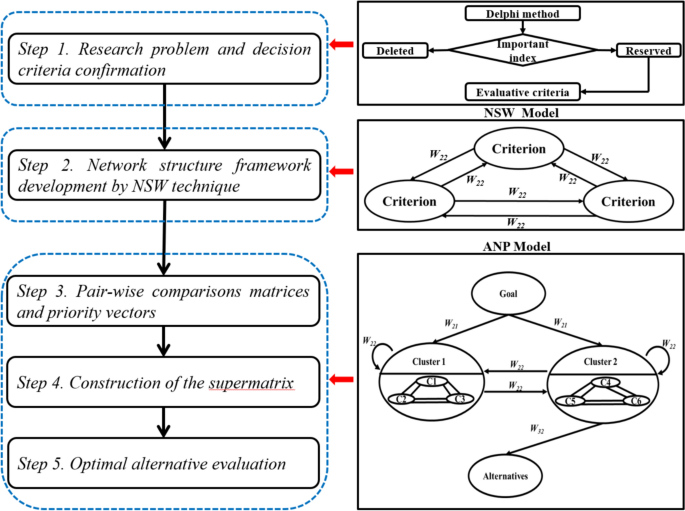
The integration processes
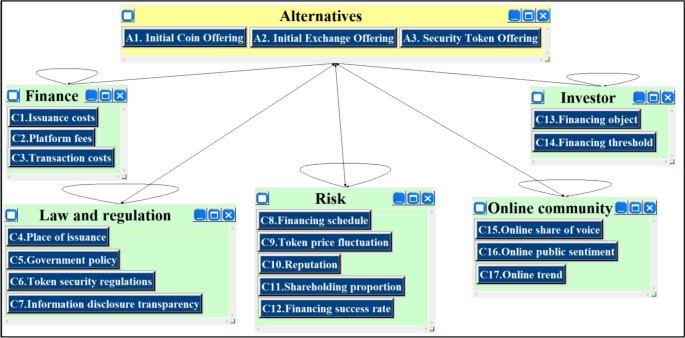
The research model
Step 1: Research the problem and confirm the decision factors
Past literature has pointed out that a research framework can be established only after experts reach a consensus on the factors [ 81 , 82 ]. Regarding the assessment of multiple principals, the number of selected experts should be between five and nine [ 76 ]. Therefore, this study included three scholars and four business starters, totaling seven experts. The goal of this study was to construct a consolidation-type pattern for the optimal scheme of token financing. Taking startup companies as examples, through a literature review and utilization of the Delphi method, a total of 17 factors, five clusters, and three token financing schemes were obtained, as shown in Fig. 4 . Relevant materials of each cluster and factors are shown as follows:
The definitions and illustrations of the clusters, factors, and token financing schemes in this study are as follows:
Finance: This includes issuance costs, platform fees, and transaction costs.
Issuance costs (C1) [ 83 , 84 ]: The costs of issuing tokens in different token financing schemes (for instance, Mint), which can vary.
Platform fees (C2) [ 83 ]: The costs for different token financing schemes to be launched on platforms (for instance, the costs for the schemes to be launched in Finance).
Transaction costs (C3) [ 83 ]: The transaction costs of different token financing schemes, which can vary (for instance, service charges).
Laws and regulations: This includes the place of issuance, government policy, token security regulations, and information disclosure transparency.
Place of issuance (C4): The laws, regulations, and rules of different countries and regions, as far as the issuance of tokens is concerned.
Policies (C5): The degree of support from government authorities on token financing.
Token security regulations (C6) [ 84 ]: The relevant policies on token security.
Information disclosure transparency (C7) [ 85 ]: Policies regarding the information disclosure of enterprises that issue tokens.
Risk: This includes financing schedules, token price fluctuations, reputation, shareholding proportion, and financing success rates.
Financing schedule (C8): The length of the financing scheme. For instance, Initial Coin Offerings (ICO) take a relatively long time, while Security Token Offerings (STO) take a relatively short time.
Token price fluctuations (C9) [ 83 ]: The price fluctuations of token transactions are obvious and influence relevant financing efficiency.
Reputation (C10) [ 86 ]: The degree of the token financing scheme’s requirements for the business reputation of the enterprises. For instance, ICO requires relatively less on the business reputation of the enterprises.
Shareholding proportion (C11): The proportion of shares corresponding to the tokens, which are held by the investors.
Financing success rates (C12) [ 87 ]: The success rates of different token financing schemes for enterprises.
The investor aspect: This includes the financing objects and financing thresholds.
Financing objects (C13): The investors being sought out by enterprises engaging in token financing. For instance, ICO and Initial Exchange Offerings (IEO) focus more on private investors, while STO focuses more on professional investors.
Financing thresholds (C14): The thresholds for enterprises to engage in token financing. For instance, the threshold of STO is relatively high.
The online community aspect: This includes the online sharing of voice, online public sentiment, and online trends.
Online sharing of voice (C15) [ 88 ]: The degree of influence of investors’ preferences of network volume in different financing platforms.
Online public sentiment (C16): The degree of influence of investor sentiment in the social network platforms of different financing platforms.
Online trends (C17): The degree of influence of the tendencies on the investors in the overall environment of token financing.
Token financing schemes: These include ICO, IEO, and STO.
ICO: The development, maintenance, and exchange for the purpose of financing, using blockchain technologies and virtual tokens.
IEO: The issuance and sales of tokens through the endorsement of exchanges. It also refers to the rules under which the exchanges are responsible for knowing your customer (KYC) compliance and anti-money laundering (AML).
STO: ICO is supervised by the government. It refers to the practice of linking the assets of enterprises to tokens through securitization, as well as the sales of such assets.
Step 2: Develop the network structure models through NSW
The results acquired in Step 1 were integrated into the NSW models suggested by this study, so as to determine the network structure. The relevant procedures are as follows:
Step 2.1: Design the questionnaire
In regards to the five clusters and 17 factors obtained by the experts in Step 1, a nine-point Likert scale was utilized to determine the strength of correlation between different factors. In the event of n factors, n ( n − 1) comparisons of the scale were carried out. Because this study referred to seven experts for the development of the network structure model, the data involved were quite complicated. The NSW procedures were illustrated in accordance with the finance clusters, as well as the three factors of issuance costs, platform fees, and transaction costs. The questionnaire design for the finance clusters is shown in Table 2 , in which 0 indicates no influence was observed, while 9 indicates the influence was of the highest level. The strength of correlation among the three factors of finance obtained through the questionnaires of the seven experts is shown in Fig. 5 . Each expert’s assessment is represented in a separate diagram, allowing for a comparison of individual perspectives. The differences in experts’ opinions highlight the subjective nature of these assessments and underscore the importance of aggregating their opinions. The generally strong correlations between factors, particularly between issuance costs and platform fees, suggest that these financial aspects are closely interrelated in token financing decisions. This visualization is crucial for understanding the foundation of our network structure, as it forms the basis for our NSW calculations.
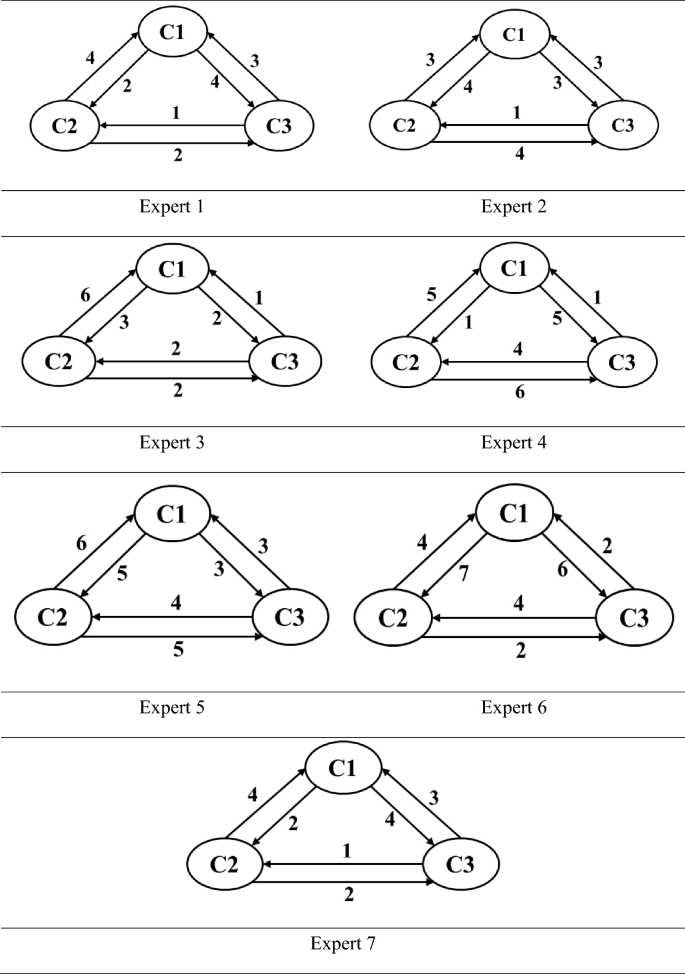
The strength of correlation among the three factors of finance obtained through the questionnaires of the seven experts
Step 2.2: Calculate the weight of the network structure
Each expert compared the factors and scored them in terms of strength. After that, the comparison scores provided by the experts were used in the construction of the matrices and weighted calculations. First, the correlation matrices of the finance clusters, M 1 to M 7 , were established on the basis of Eq. ( 1 ) and the scores of the strength given by the seven experts, as shown below. Second, correlation matrix M was transformed into probability matrices A 1 to A 7 through Eq. ( 2 ), as shown below, and the iteration method was used n times to obtain the characteristic values (eigenvalues) of each questionnaire and factor. Third, this study calculated the weighted values of the correlation among C 1 , C 2 , and C 3 , as well as R ( C i , C j ) 1 to R ( C i , C j ) 7 , through Eq. ( 3 ), as shown in Fig. 6 . This visualization is crucial for understanding how individual expert opinions contribute to the overall network structure. The variation in weights across experts highlights the subjective nature of these assessments and the necessity to aggregate multiple expert opinions. Notably, most experts consistently assign higher weights to the relationships between issuance costs ( C 1 ) and platform fees ( C 2 ), indicating a strong perceived connection between these two factors. In the end, the ultimate weighted values of the network structure (the scores of the correlation degree) were calculated using Eq. ( 4 ) and Eq. ( 5 ). The weighted values of the network structure of the various clusters and factors are shown in Fig. 7 . Figure 7 illustrates the final network structure weights for all five clusters and their respective factors, which is the foundation for our subsequent ANP analysis. These network structure weights provide a comprehensive understanding of the relative importance and interconnectedness of various factors in token financing decisions. They serve as a crucial input for our ANP model, ensuring that the final decision-making process accurately reflects the complex realities of token financing.
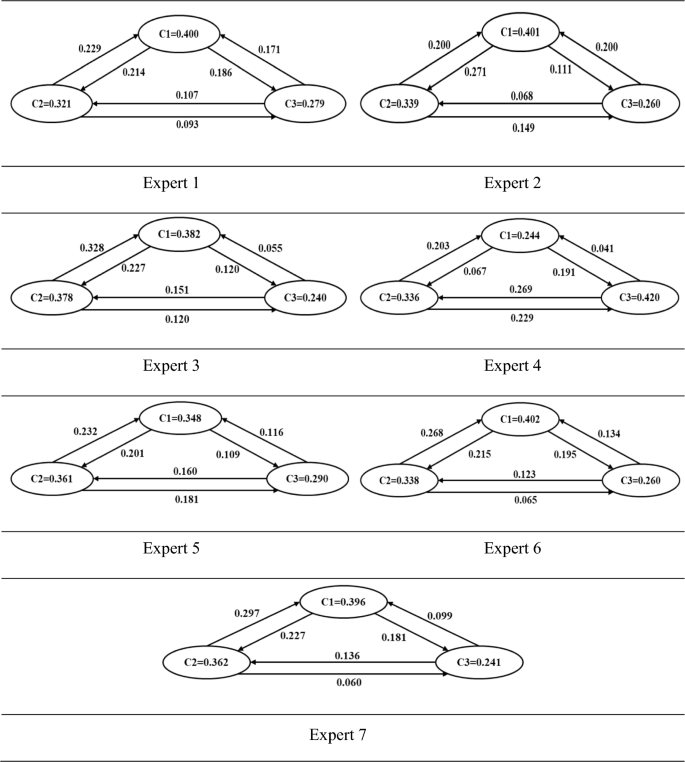
The network structure weights of finance cluster’s factors by 7 experts
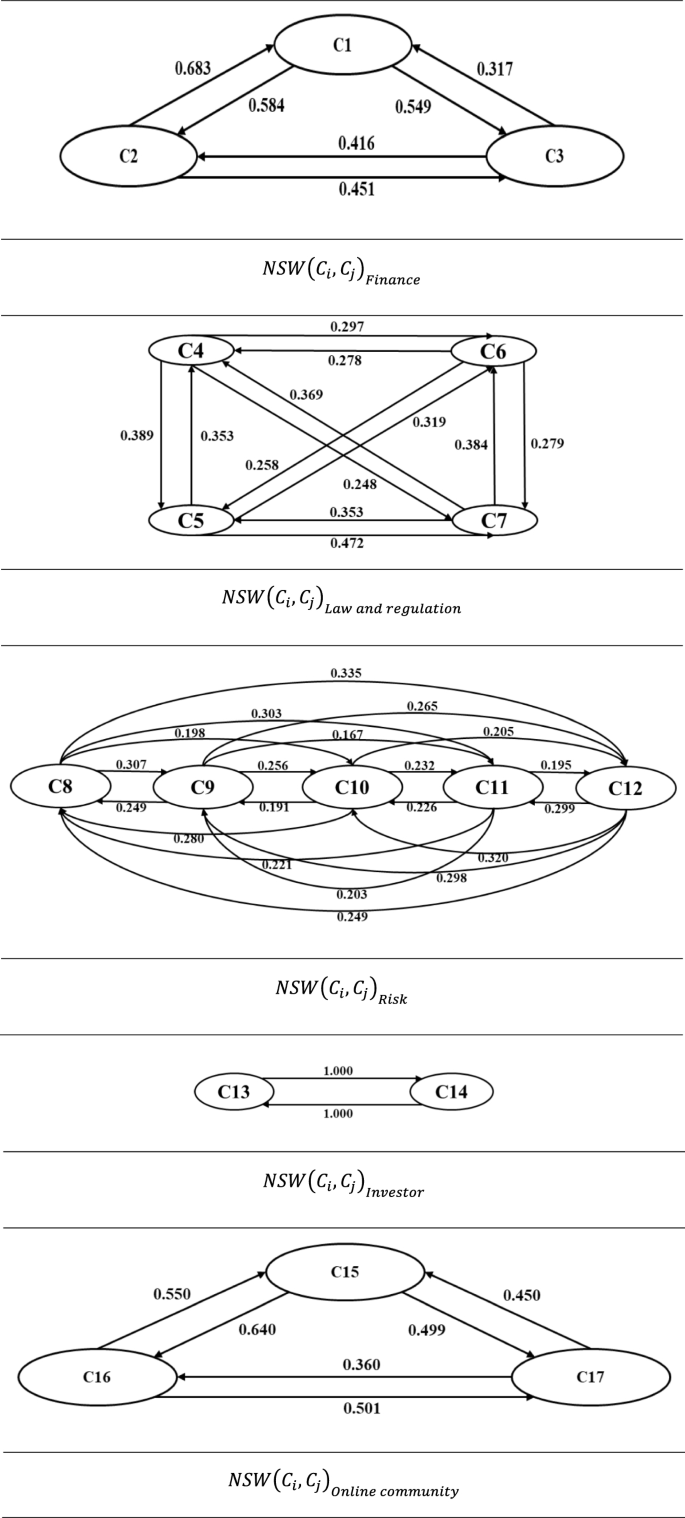
The network structure weights of five cluster’s factors
Upon completing the calculations, the results of the weighted values for the network structure were integrated into the ANP models to establish the comparison matrices and calculate the eigenvectors.
Step 3: Perform pair-wise comparisons of the matrices and priority vectors
The eigenvectors of the clusters and factors were calculated through the AHP processes and pairwise comparison of features of matrices. The eigenvectors of the degree of correlation between different clusters and factors were calculated through NSW. The cases in this study involved five clusters (finance, laws and regulations, risk, investor, and online community), 17 factors (issuance costs, platform fees, transaction costs, place of issuance, government policy, token security regulations, information disclosure transparency, financing schedules, token price fluctuations, reputation, shareholding proportion, financing success rates, financing objects, financing thresholds, online share of voice, online public sentiment, and online trends), as well as three schemes.
The comparison matrices (in pairs) and the geometric method were utilized to calculate the eigenvectors, while the eigenvectors for the network structure of the correlation strength scores were obtained on the basis of NSW. The eigenvectors obtained for the various comparison matrices, as well as the eigenvectors related to the correlation strength of the factors, served as the values of the supermatrix, which was used to illustrate the correlation strength and the relative importance of different clusters. The clusters might confirm the eigenvectors of the network structure through NSW, and the scores of the relative importance were calculated using Eq. ( 6 ). The results of the eigenvectors for the network structure of the various factors are shown in Step 2.2, and the comparison matrices (in pairs) and the weighted values of the five clusters are shown in Table 3 . Table 4 contains the scores for the relative importance of the various factors against the alternative schemes. In this study, Super Decision V2.0 (software) was utilized for the subsequent assessment of the ANP models. The eigenvectors of the network structure obtained through the NSW were inputted into Super Decision V2.0 to integrate NSW and ANP and assess the optimal scheme and the key factors.
Step 4: Construct the supermatrix
The eigenvectors of the relationships among the factors, as well as the eigenvectors regarding the weights of the factors to the schemes, were determined according to the results of Step 3. In Step 4, a supermatrix is established on the basis of the eigenvectors obtained in Step 3, so that the optimal scheme for startup companies engaging in token financing could be measured. During the ANP process, the ultimate weighted values of the various factors and schemes were calculated through the unweighted supermatrix, the weighted supermatrix, and the limit supermatrix. First, the calculated eigenvectors of the NSW model for the factors and pair-wise comparison matrices were utilized to establish the unweighted supermatrix. Second, the unweighted supermatrix was multiplied by the reciprocals of the weighted values of the relevant clusters to generate the weighted supermatrix. Finally, the results of the weighted supermatrix were multiplied by themselves repeatedly until a stable probability distribution was realized. This probability distribution reflected the ultimate weighted values to be reached. The various supermatrices are shown in Tables 5 , 6 , and 7 .
Step 5: Evaluate the optimal alternative
Through the supermatrix mentioned in Step 4, as well as the operation of Super Decision, the ultimate weighted values of the various factors and schemes under the consolidated NSW network structure could be obtained, as shown in Table 8 .
This study suggested the establishment of a set of network assessment procedures integrating the new NSW technique with the ANP model, in order to analyze the optimal scheme for startup companies engaging in token financing. The findings indicated a number of results. The sequence of the weighted values for the five clusters was as follows: finance (0.307) > risk (0.294) > laws and regulations (0.211) > investors (0.106) > online community (0.082). In addition, the sequence of the weighted values for the factors was as follows: platform fees (0.083) > issuance costs (0.078) > financing success rate (0.053) > government policy (0.0049) = financing schedule (0.049) > transaction costs (0.044) > financing threshold (0.040) > information disclosure transparency (0.039) > token price fluctuations (0.032) = shareholding proportion (0.032) > financing object (0.031) > reputation (0.030) > place of issuance (0.027) > token security regulations (0.026) > online share of voice (0.022) > online public sentiment (0.019) > online trend (0.014). Finally, the sequence of the optimal scheme for startup companies engaging in token financing is as follows: ICO (0.057) > IEO (0.101) > STO (0.175). STO is the optimal scheme for startup companies to engage in token financing.
4 Conclusion and Future Work
4.1 conclusion.
The rapid development of FinTech has become one of the goals of inclusive financing. Fintech, which depends on information technology to find solutions in the financial field, is becoming the mainstream future trend in the financial industry, especially in the development of new business patterns. Startup companies might find it difficult to borrow money from traditional financial institutions due to their business operation features and financial structures. For this reason, alternative financing has gradually become an important channel for startup companies to acquire financing. Token financing is a relatively new business pattern in the field of alternative financing, and it can avoid the shortcomings and problems of crowdfunding.
However, the development history of token financing is diversified and complicated. Previous studies in this field focused more on the analysis of the values of virtual currencies. Generally speaking, when startup companies are faced with the option of token financing, which is a new business pattern, they have relatively little information available for business assessments and decision making. When startup companies assess the optimal scheme for token financing, they often use multi-principle decision-making models, which can solve the problems of filtration and selection in token financing. However, multi-principle decision-making models depend heavily on the presumption that the variables (or criteria) are independent from each other. Therefore, such models might not be suitable for the assessment of decision-making problems in the real world.
ANP can be used to solve the problem of independence assumption in traditional multi-principle decision-making models. Although ANP can overcome the problem of independence assumption, it is still unable to ascertain the strength of the dependence and relationships between variables before producing a network structure. In this study, a new model, NSW, was put forward. This new model could be used to calculate the correlation between variables and generate the network structure. In addition, NSW could be integrated into ANP to generate the network structure. In the end, the assessment of the optimal scheme for startup companies engaging in token financing served as the case study. The results of this study show that finance is the most critical cluster in the assessment aspect. In other words, when startup companies intend to engage in token financing, financial issue is the first aspect to be considered. Token financing is the most up-to-date financing method in the era of FinTech, and capital turnover and financial structure are key issues during the development of startup companies. The sequence of key factors are platform fees, issuance costs, and financing success rate. Moreover, this sequence suggests that when startup companies intend to engage in token financing, the key factors are the aspect of costs and the success rate of financing. Finally, the optimal scheme for startup companies engaging in token financing is STO. After considering financial issues, costs, and relevant risks, startup companies should, based on the cost assessment and the success rate of financing, adopt STO for token financing to promote the financial efficiency of such companies.
This study proposed the NSW technique as a novel tool for validating network structures in decision-making processes and integrated NSW into the ANP model to develop a comprehensive framework for evaluating optimal token financing strategies. The contributions of this study in token-based financing include both methodological advancement and practical application. In terms of methodology, this study integrated the NSW technique with the ANP to enhance the robustness of existing frameworks in capturing complex interrelationships within decision-making processes. This innovative approach addresses limitations in traditional methods by providing a more comprehensive quantification of the strength and directionality of relationships between decision factors. As for practical application, this study presents the first comprehensive evaluation of token financing options for startup companies utilizing this advanced decision-making approach. The integrated NSW-ANP framework can be applied to ICO, IEO, and STO, thus offering valuable options for cryptocurrency-based startup financing. This systematic evaluation considers the intricate interdependencies among various factors influencing the selection of optimal financing strategies. By bridging the gap between theoretical innovation and practical implementation, this study not only advances the field of multi-criteria decision-making but also provides startup entrepreneurs and investors with a sophisticated tool for token-based financing options. Academically, this study provided a new NSW technique, as well as the application procedures to integrate NSW into ANP. This study also presented a case study of the assessment of the optimal scheme for startup companies engaging in token financing. Practically, this new framework could provide entrepreneurs of startup companies with valuable measurement tools for promoting their company’s capital turnover rate through token financing under the rapid development of FinTech.
4.2 Limitation and Future Research
While acknowledging the substantial advantages offered by our integrated framework, it is imperative to recognize its inherent limitations. The following constraints warrant further investigation and potential mitigation in future research:
The potential complexity and mathematical technique of the proposed model, which might make it challenging to implement for organizations.
The static nature of the model, which may not fully capture the decision risks of uncertainty in the cryptocurrency and token financing landscape.
At the current stage of development, the model may not comprehensively capture the effects of factor weight variations on the rankings of alternatives.
After discussing these limitations, we will outline potential directions for future research. This section will propose several avenues for extending and refining our work:
Expanding the application of the NSW-ANP method to other areas of FinTech decision-making beyond token financing.
Integration of fuzzy set theory into the NSW-ANP model to address decision uncertainty risks.
A sensitivity analysis was conducted to ascertain the effects of factor weight variations on the rankings of alternatives.
Data Availability
Not applicable.
Ivanov, V., Knyazeva, A.: US securities-based crowdfunding under Title III of the JOBS Act. In: DERA White Paper (2017). Accessed 15 Jan 2022
Rossi, M.: The new ways to raise capital: an exploratory study of crowdfunding. Int. J. Financ. Res. 5 (2), 8–18 (2014)
Article Google Scholar
Walthoff-Borm, X., Schwienbacher, A., Vanacker, T.: Equity crowdfunding: first resort or last resort? J. Bus. Ventur. 33 (4), 513–533 (2018)
Massolution. (2015). 2015CF: The Crowdfunding Industry Report. Massolution. https://www.smv.gob.pe/Biblioteca/temp/catalogacion/C8789.pdf . Accessed 20 Jan 2022
Statista Inc.: Alternative Financing Report 2021 (2021). https://www.statista.com/study/47352/fintech-report-alternative-financing/ . Accessed 10 Jan 2022
Lu, Y., Chang, R., Lim, S.: Crowdfunding for solar photovoltaics development: a review and forecast. Renew. Sustain. Energy Rev. 93 , 439–450 (2018)
Bagheri, A., Chitsazan, H., Ebrahimi, A.: Crowdfunding motivations: a focus on donors’ perspectives. Technol. Forecast. Soc. Chang. 146 , 218–232 (2019)
Petruzzelli, A.M., Natalicchio, A., Panniello, U., Roma, P.: Understanding the crowdfunding phenomenon and its implications for sustainability. Technol. Forecast. Soc. Chang. 141 , 138–148 (2019)
Estrin, S., Gozman, D., Khavul, S.: Case study of the equity crowdfunding landscape in London: an entrepreneurial and regulatory perspective. FIRES Case Study 5 (2), 1–62 (2016)
Google Scholar
Kuti, M., Madarász, G.: Crowdfunding. Public Financ. Q. 59 (3), 355 (2014)
Agrawal, A., Catalini, C., Goldfarb, A.: Some simple economics of crowdfunding. Innov. Policy Econ. 14 (1), 63–97 (2014)
Zhu, H., Zhou, Z.Z.: Analysis and outlook of applications of blockchain technology to equity crowdfunding in China. Financ. Innov. 2 (1), 29 (2016)
Baber, H.: Blockchain-based crowdfunding. In: Blockchain Technology for Industry 4.0, pp. 117–130. Springer, Singapore (2020)
Chapter Google Scholar
Chod, J., Trichakis, N., Yang, S.A.: Platform tokenization: financing, governance, and moral hazard. Manage. Sci. 68 (9), 6411–6433 (2021)
Howell, S.T., Niessner, M., Yermack, D.: Initial coin offerings: financing growth with cryptocurrency token sales. Rev. Financ. Stud. 33 (9), 3925–3974 (2020)
Amsden, R., Schweizer, D.: Are blockchain crowdsales the new ‘gold rush’? Success determinants of initial coin offerings (April 16, 2018) (2018)
Ante, L., Fiedler, I.: Cheap signals in security token offerings. Quant. Financ. Econ. 4 (4), 608–639 (2020)
Miglo, A.: Choice between IEO and ICO: speed vs. liquidity vs. risk. FinTech 1 (3), 276–293 (2022)
Kondova, G., Simonella, G.: Blockchain in startup financing: ICOs and STOs in Switzerland. J. Strateg. Innov. Sustain. 14 (6), 43–48 (2019)
Gryglewicz, S., Mayer, S., Morellec, E.: Optimal financing with tokens. J. Financ. Econ. 142 (3), 1038–1067 (2021)
Canh, N.P., Wongchoti, U., Thanh, S.D., Thong, N.T.: Systematic risk in cryptocurrency market: evidence from DCC-MGARCH model. Financ. Res. Lett. 29 , 90–100 (2019)
Borri, N.: Conditional tail-risk in cryptocurrency markets. J. Empir. Financ. 50 , 1–19 (2019)
Liu, Y., Tsyvinski, A.: Risks and returns of cryptocurrency. Rev. Financ. Stud. 34 (6), 2689–2727 (2021)
Zhang, W., Li, Y., Xiong, X., Wang, P.: Downside risk and the cross-section of cryptocurrency returns. J. Bank. Finance 133 , 106246 (2021)
Borri, N., Shakhnov, K.: Regulation spillovers across cryptocurrency markets. Financ. Res. Lett. 36 , 101333 (2020)
Feinstein, B.D., Werbach, K.: The impact of cryptocurrency regulation on trading markets. J. Financ. Regul. 7 (1), 48–99 (2021)
Chokor, A., Alfieri, E.: Long and short-term impacts of regulation in the cryptocurrency market. Q. Rev. Econ. Finance 81 , 157–173 (2021)
Beneki, C., Koulis, A., Kyriazis, N.A., Papadamou, S.: Investigating volatility transmission and hedging properties between Bitcoin and Ethereum. Res. Int. Bus. Financ. 48 , 219–227 (2019)
Okorie, D.I., Lin, B.: Crude oil price and cryptocurrencies: evidence of volatility connectedness and hedging strategy. Energy Econ. 87 , 104703 (2020)
Thampanya, N., Nasir, M.A., Huynh, T.L.D.: Asymmetric correlation and hedging effectiveness of gold & cryptocurrencies: from pre-industrial to the 4th industrial revolution✰. Technol. Forecast. Soc. Chang. 159 , 120195 (2020)
Sebastião, H., Godinho, P.: Bitcoin futures: an effective tool for hedging cryptocurrencies. Financ. Res. Lett. 33 , 101230 (2020)
Walther, T., Klein, T., Bouri, E.: Exogenous drivers of bitcoin and cryptocurrency volatility—a mixed data sampling approach to forecasting. J. Int. Finan. Markets. Inst. Money 63 , 101133 (2019)
Ma, F., Liang, C., Ma, Y., Wahab, M.I.M.: Cryptocurrency volatility forecasting: a Markov regime-switching MIDAS approach. J. Forecast. 39 (8), 1277–1290 (2020)
Article MathSciNet Google Scholar
Köchling, G., Schmidtke, P., Posch, P.N.: Volatility forecasting accuracy for Bitcoin. Econ. Lett. 191 , 108836 (2020)
Yen, K.C., Cheng, H.P.: Economic policy uncertainty and cryptocurrency volatility. Financ. Res. Lett. 38 , 101428 (2021)
Hamdan, S., Cheaitou, A.: Supplier selection and order allocation with green criteria: an MCDM and multi-objective optimization approach. Comput. Oper. Res. 81 , 282–304 (2017)
Lin, S.W.: Identifying the critical success factors and an optimal solution for mobile technology adoption in travel agencies. Int. J. Tour. Res. 19 (2), 127–144 (2017)
Lin, C.Y.: Optimal core operation in supply chain finance ecosystem by integrating the fuzzy algorithm and hierarchical framework. Int. J. Comput. Intell. Syst. 13 (1), 259–274 (2020)
Kilic, B., Ucler, C.: Stress among ab-initio pilots: a model of contributing factors by AHP. J. Air Transp. Manag. 80 , 101706 (2019)
Achu, A.L., Thomas, J., Reghunath, R.: Multi-criteria decision analysis for delineation of groundwater potential zones in a tropical river basin using remote sensing, GIS and analytical hierarchy process (AHP). Groundw. Sustain. Dev. 10 , 100365 (2020)
Gündoğdu, F.K., Duleba, S., Moslem, S., Aydın, S.: Evaluating public transport service quality using picture fuzzy analytic hierarchy process and linear assignment model. Appl. Soft Comput. 100 , 106920 (2021)
Awad, J., Jung, C.: Extracting the planning elements for sustainable urban regeneration in Dubai with AHP (analytic hierarchy process). Sustain. Cities Soc. 76 , 103496 (2022)
Jorge-García, D., Estruch-Guitart, V.: Comparative analysis between AHP and ANP in prioritization of ecosystem services—a case study in a rice field area raised in the Guadalquivir marshes (Spain). Eco. Inform. 70 , 101739 (2022)
Tsai, W.H., Chou, W.C.: Selecting management systems for sustainable development in SMEs: a novel hybrid model based on DEMATEL, ANP, and ZOGP. Expert Syst. Appl. 36 (2), 1444–1458 (2009)
Saaty, T.L.: Decision Making with Dependence and Feedback: The Analytic Network Process, vol. 4922. RWS publications, Pittsburgh (1996)
Munim, Z.H., Duru, O., Ng, A.K.: Transhipment port’s competitiveness forecasting using analytic network process modelling. Transp. Policy 124 , 70–82 (2022)
Eshtiaghi, K., Aliyannezhadi, M., Najafian, A.: Identification and prioritization of factors affecting the adoption of electric vehicles using analytic network process. Int. J. Hum. Cap. Urban Manag 6 , 323–336 (2021)
Pang, N., Nan, M., Meng, Q., Zhao, S.: Selection of wind turbine based on fuzzy analytic network process: a case study in China. Sustainability 13 (4), 1792 (2021)
Asadi, A., Moghaddam Nia, A., Bakhtiari Enayat, B., Alilou, H., Ahmadisharaf, E., Kimutai Kanda, E., Chessum Kipkorir, E.: An integrated approach for prioritization of river water quality sampling points using modified Sanders, analytic network process, and hydrodynamic modeling. Environ. Monit. Assess. 193 (8), 1–15 (2021)
Toth, W., Vacik, H., Pülzl, H., Carlsen, H.: Deepening our understanding of which policy advice to expect from prioritizing SDG targets: introducing the analytic network process in a multi-method setting. Sustain. Sci. 17 (4), 1473–1488 (2022)
Fallahpour, A., Nayeri, S., Sheikhalishahi, M., Wong, K.Y., Tian, G., Fathollahi-Fard, A.M.: A hyper-hybrid fuzzy decision-making framework for the sustainable-resilient supplier selection problem: a case study of Malaysian palm oil industry. Environ. Sci. Pollut. Res. (2021). https://doi.org/10.1007/s11356-021-12491-y
Mabrouk, N.: Green supplier selection using fuzzy Delphi method for developing sustainable supply chain. Decis. Sci. Lett. 10 (1), 63–70 (2021)
Thanh, N.V., Lan, N.T.K.: A new hybrid triple bottom line metrics and fuzzy MCDM model: sustainable supplier selection in the food-processing industry. Axioms 11 (2), 57 (2022)
Erol, H., Dikmen, I., Atasoy, G., Birgonul, M.T.: An analytic network process model for risk quantification of mega construction projects. Expert Syst. Appl. 191 , 116215 (2022)
Tabatabaee, S., Mahdiyar, A., Mohandes, S.R., Ismail, S.: Towards the development of a comprehensive lifecycle risk assessment model for green roof implementation. Sustain. Cities Soc. 76 , 103404 (2022)
Rehman, O., Ali, Y., Sabir, M.: Risk assessment and mitigation for electric power sectors: a developing country’s perspective. Int. J. Crit. Infrastruct. Prot. 36 , 100507 (2022)
Moghaddasi, K., Rajabi, S., Soleimanian Gharehchopogh, F., Hosseinzadeh, M.: An energy-efficient data offloading strategy for 5G-enabled vehicular edge computing networks using double deep Q-network. Wireless Pers. Commun. 133 (3), 2019–2064 (2023)
Gharehchopogh, F.S., Abdollahzadeh, B., Barshandeh, S., Arasteh, B.: A multi-objective mutation-based dynamic Harris Hawks optimization for botnet detection in IoT. Internet of Things 24 , 100952 (2023)
Moghaddasi, K., Rajabi, S., Gharehchopogh, F.S.: Multi-objective secure task offloading strategy for blockchain-enabled IoV-MEC systems: a double deep Q-network approach. IEEE Access 12 , 3437–3463 (2024)
Moghaddasi, K., Rajabi, S., Gharehchopogh, F.S.: An enhanced asynchronous advantage actor-critic-based algorithm for performance optimization in mobile edge computing-enabled internet of vehicles networks. Peer-to-Peer Netw. Appl. 17 , 1169–1189 (2024)
Moghaddasi, K., Rajabi, S., Gharehchopogh, F.S., Ghaffari, A.: An advanced deep reinforcement learning algorithm for three-layer D2D-edge-cloud computing architecture for efficient task offloading in the internet of things. Sustain. Comput.: Inf. Syst. 43 , 100992 (2024)
Kashyap, A., Kumar, C., Shukla, O.J.: A DEMATEL model for identifying the impediments to the implementation of circularity in the aluminum industry. Decis. Anal. J. 5 , 100134 (2022)
Kamranfar, S., Azimi, Y., Gheibi, M., Fathollahi-Fard, A.M., Hajiaghaei-Keshteli, M.: Analyzing green construction development barriers by a hybrid decision-making method based on DEMATEL and the ANP. Buildings 12 (10), 1641 (2022)
Karasan, A., Ilbahar, E., Cebi, S., Kahraman, C.: Customer-oriented product design using an integrated neutrosophic AHP & DEMATEL & QFD methodology. Appl. Soft Comput. 118 , 108445 (2022)
Nezhad, M.Z., Nazarian-Jashnabadi, J., Rezazadeh, J., Mehraeen, M., Bagheri, R.: Assessing dimensions influencing IoT implementation readiness in industries: a fuzzy DEMATEL and fuzzy AHP analysis. J. Soft Comput. Decis. Anal. 1 (1), 102–123 (2023)
Du, Y.W., Shen, X.L.: Group hierarchical DEMATEL method for reaching consensus. Comput. Ind. Eng. 175 , 108842 (2023)
Si, S.L., You, X.Y., Liu, H.C., Zhang, P.: DEMATEL technique: a systematic review of the state-of-the-art literature on methodologies and applications. Math. Probl. Eng. 2018 , 1–33 (2018)
Sun, Y., Zhang, S., Huang, Z., Miao, B.: Probabilistic linguistic-based group DEMATEL method with both positive and negative influences. Complexity 2021 , 1–20 (2021)
Šmidovnik, T., Grošelj, P.: Solution for convergence problem in DEMATEL method: DEMATEL of finite sum of influences. Symmetry 15 (7), 1357 (2023)
Linstone, H.A., Turoff, M. (eds.): The Delphi method, pp. 3–12. Addison-Wesley, Reading, MA (1975)
Murry, J.W., Jr., Hammons, J.O.: Delphi: a versatile methodology for conducting qualitative research. Rev. High. Educ. 18 (4), 423–436 (1995)
Skulmoski, G.J., Hartman, F.T., Krahn, J.: The Delphi method for graduate research. J. Inf. Technol. Educ.: Res. 6 (1), 1–21 (2007)
Okoli, C., Pawlowski, S.D.: The Delphi method as a research tool: an example, design considerations and applications. Information and Management 42 (1), 15–29 (2004)
Wu, C.R., Lin, C.T., Chen, H.C.: Evaluating competitive advantage of the location for Taiwanese hospitals. J. Inf. Optim. Sci. 28 (5), 841–868 (2007)
Sung, W.C.: Application of Delphi method, a qualitative and quantitative analysis, to the healthcare management. J. Healthc. Manag. 2 (2), 11–19 (2001)
Hasson, F., Keeney, S.: Enhancing rigour in the Delphi technique research. Technol. Forecast. Soc. Chang. 78 (9), 1695–1704 (2011)
Atmaca, E., Basar, H.B.: Evaluation of power plants in Turkey using analytic network process (ANP). Energy 44 (1), 555–563 (2012)
Keramati, A., Salehi, M.: Website success comparison in the context of e-recruitment: an analytic network process (ANP) approach. Appl. Soft Comput. 13 (1), 173–180 (2013)
Meade, L., Sarkis, J.: Strategic analysis of logistics and supply chain management systems using the analytical network process. Transp. Res. E: Logist. Transp. Rev. 34 (3), 201–215 (1998)
Saaty, T.L., Vargas, L.G.: Decision making with the analytic network process, vol. 282. Springer, Berlin (2006)
Ali-Yrkko, J., Rouvinen, P., Seppala, T., Yla-Anttila, P.: Who captures value in global supply chains? Case Nokia N95 smartphone. J. Ind. Compet. Trade 11 (3), 63–278 (2011)
Linden, G., Kraemer, K.L., Dedrick, J.: Who captures value in a global innovation network? The case of Apple’s iPod. Commun. ACM 52 (3), 140–144 (2009)
Cong, L.W., Li, Y., Wang, N.: Tokenomics: dynamic adoption and valuation. Rev. Financ. Stud. 34 (3), 1105–1155 (2021)
Myalo, A.S.: Comparative analysis of ICO, DAOICO, IEO and STO. Case study. Financ. Theory Pract. 23 (6), 6–25 (2019)
Chod, J., Lyandres, E.: A theory of ICOS: diversification, agency, and information asymmetry. Manage. Sci. 67 (10), 5969–5989 (2021)
Momtaz, P.P.: Entrepreneurial finance and moral hazard: evidence from token offerings. J. Bus. Ventur. 36 (5), 106001 (2021)
Giudici, G., Adhami, S.: The impact of governance signals on ICO fundraising success. J. Ind. Bus. Econ. 46 (2), 283–312 (2019)
Fisch, C., Masiak, C., Vismara, S., Block, J.: Motives and profiles of ICO investors. J. Bus. Res. 125 , 564–576 (2021)
Download references
This research received no external funding.
Author information
Authors and affiliations.
Department of Public Finance and Tax Administration, National Taipei University of Business, 321, Sec. 1, Jinan Rd., Zhongzheng District, Taipei, 100, Taiwan
Chun-Yueh Lin
You can also search for this author in PubMed Google Scholar
Contributions
Research design, literature review, data collection, data analysis, and manuscript writing are all conducted by Chun Yueh Lin.
Corresponding author
Correspondence to Chun-Yueh Lin .
Ethics declarations
Conflict of interest.
The authors declare no conflict of interest.
Additional information
Publisher's note.
Springer Nature remains neutral with regard to jurisdictional claims in published maps and institutional affiliations.
Rights and permissions
Open Access This article is licensed under a Creative Commons Attribution-NonCommercial-NoDerivatives 4.0 International License, which permits any non-commercial use, sharing, distribution and reproduction in any medium or format, as long as you give appropriate credit to the original author(s) and the source, provide a link to the Creative Commons licence, and indicate if you modified the licensed material. You do not have permission under this licence to share adapted material derived from this article or parts of it. The images or other third party material in this article are included in the article’s Creative Commons licence, unless indicated otherwise in a credit line to the material. If material is not included in the article’s Creative Commons licence and your intended use is not permitted by statutory regulation or exceeds the permitted use, you will need to obtain permission directly from the copyright holder. To view a copy of this licence, visit http://creativecommons.org/licenses/by-nc-nd/4.0/ .
Reprints and permissions
About this article
Lin, CY. Constructing a Novel Network Structure Weighting Technique into the ANP Decision Support System for Optimal Alternative Evaluation: A Case Study on Crowdfunding Tokenization for Startup Financing. Int J Comput Intell Syst 17 , 222 (2024). https://doi.org/10.1007/s44196-024-00643-0
Download citation
Received : 19 March 2024
Accepted : 19 August 2024
Published : 26 August 2024
DOI : https://doi.org/10.1007/s44196-024-00643-0
Share this article
Anyone you share the following link with will be able to read this content:
Sorry, a shareable link is not currently available for this article.
Provided by the Springer Nature SharedIt content-sharing initiative
- Crowdfunding
- Token financing
- Network structure weighting (NSW)
- Analytic network process (ANP)
- Find a journal
- Publish with us
- Track your research
This paper is in the following e-collection/theme issue:
Published on 26.8.2024 in Vol 26 (2024)
Evaluation of a Natural Language Processing Approach to Identify Diagnostic Errors and Analysis of Safety Learning System Case Review Data: Retrospective Cohort Study
Authors of this article:

Original Paper
- Azade Tabaie 1, 2 , PhD ;
- Alberta Tran 3 , RN, CCRN, PhD ;
- Tony Calabria 3 , MA, CPHQ, CSSBB ;
- Sonita S Bennett 1 , MSc ;
- Arianna Milicia 4 , BSc ;
- William Weintraub 5, 6 , MACC, MD ;
- William James Gallagher 6, 7 , MD ;
- John Yosaitis 6, 8 , MD ;
- Laura C Schubel 4 , MPH ;
- Mary A Hill 9, 10 , MS ;
- Kelly Michelle Smith 9, 10 , PhD ;
- Kristen Miller 4, 6 , MSPH, MSL, CPPS, DrPH
1 Center for Biostatistics, Informatics, and Data Science, MedStar Health Research Institute, Washington, DC, United States
2 Department of Emergency Medicine, Georgetown University School of Medicine, Washington, DC, United States
3 Department of Quality and Safety, MedStar Health Research Institute, Washington, DC, United States
4 National Center for Human Factors in Healthcare, MedStar Health Research Institute, Washington, DC, United States
5 Population Health, MedStar Health Research Institute, Washington, DC, United States
6 Georgetown University School of Medicine, Washington, DC, United States
7 Family Medicine Residency Program, MedStar Health Georgetown-Washington Hospital Center, Washington, DC, United States
8 MedStar Simulation Training & Education Lab (SiTEL), MedStar Institute for Innovation, Washington, DC, United States
9 Institute of Health Policy, Management & Evaluation, University of Toronto, Toronto, ON, Canada
10 Michael Garron Hospital, Toronto, ON, Canada
Corresponding Author:
Azade Tabaie, PhD
Center for Biostatistics, Informatics, and Data Science
MedStar Health Research Institute
3007 Tilden Street NW
Washington, DC, 20008
United States
Phone: 1 202 244 9810
Email: [email protected]
Background: Diagnostic errors are an underappreciated cause of preventable mortality in hospitals and pose a risk for severe patient harm and increase hospital length of stay.
Objective: This study aims to explore the potential of machine learning and natural language processing techniques in improving diagnostic safety surveillance. We conducted a rigorous evaluation of the feasibility and potential to use electronic health records clinical notes and existing case review data.
Methods: Safety Learning System case review data from 1 large health system composed of 10 hospitals in the mid-Atlantic region of the United States from February 2016 to September 2021 were analyzed. The case review outcome included opportunities for improvement including diagnostic opportunities for improvement. To supplement case review data, electronic health record clinical notes were extracted and analyzed. A simple logistic regression model along with 3 forms of logistic regression models (ie, Least Absolute Shrinkage and Selection Operator, Ridge, and Elastic Net) with regularization functions was trained on this data to compare classification performances in classifying patients who experienced diagnostic errors during hospitalization. Further, statistical tests were conducted to find significant differences between female and male patients who experienced diagnostic errors.
Results: In total, 126 (7.4%) patients (of 1704) had been identified by case reviewers as having experienced at least 1 diagnostic error. Patients who had experienced diagnostic error were grouped by sex: 59 (7.1%) of the 830 women and 67 (7.7%) of the 874 men. Among the patients who experienced a diagnostic error, female patients were older (median 72, IQR 66-80 vs median 67, IQR 57-76; P =.02), had higher rates of being admitted through general or internal medicine (69.5% vs 47.8%; P =.01), lower rates of cardiovascular-related admitted diagnosis (11.9% vs 28.4%; P =.02), and lower rates of being admitted through neurology department (2.3% vs 13.4%; P =.04). The Ridge model achieved the highest area under the receiver operating characteristic curve (0.885), specificity (0.797), positive predictive value (PPV; 0.24), and F 1 -score (0.369) in classifying patients who were at higher risk of diagnostic errors among hospitalized patients.
Conclusions: Our findings demonstrate that natural language processing can be a potential solution to more effectively identifying and selecting potential diagnostic error cases for review and therefore reducing the case review burden.
Introduction
Diagnostic errors are an underappreciated cause of preventable mortality in hospitals, estimated to affect a quarter million hospital inpatients, and account for an estimated 40,000-80,000 deaths annually in the United States [ 1 ]. These errors pose a risk for severe patient harm [ 2 , 3 ], increase hospital length of stay [ 4 ], and made up 22% and accounted for US $5.7 billion of paid malpractice claims in hospitalized patients throughout a nearly 13-year period [ 5 ]. In their analysis of malpractice claims occurring in the US National Practitioner Database from 1999 to 2011, Gupta et al [ 5 ] found that diagnosis-related paid claims were most likely to be associated with death and cost (following surgery); among diagnosis-related paid claims, failure to diagnose was the most common subtype and was more likely than other types to be associated with mortality. Several factors have been proposed as contributors to inpatient diagnostic errors including time constraints related to the concurrent care of multiple patients, unpredictable workflows, distractions, and competing priorities for trainees. From their systematic review and meta-analysis, Gunderson et al [ 2 ] estimate that 250,000 diagnostic adverse events occur annually among hospitalized patients in the United States, and this is likely an underestimation of the problem due to several challenges in diagnostic error measurement [ 6 ].
Challenges in identifying and measuring diagnostic errors occur due to the evolving and iterative nature of the diagnostic process, making it difficult to determine when, if at all, a correct or more specific diagnosis could have been established by clinicians to start the appropriate treatment [ 6 ]. Since its landmark report, Improving Diagnosis in Health Care , the National Academies of Science, Engineering, and Medicine (NASEM) has produced a common understanding of diagnostic error that includes accuracy, timeliness, and communication of the explanation to the patient or patient’s family member [ 3 ]. Diagnostic errors often involve missed opportunities related to various aspects of the diagnostic process [ 7 - 9 ] and diagnostic adverse events resulting in harm [ 10 ]. However, many hospitals currently do not capture or include surveillance for diagnostic errors, despite having robust systems in place to report and analyze patient safety issues [ 6 , 11 , 12 ].
A crucial first step to improving diagnosis in hospitals is the creation of programs to identify, analyze, and learn from diagnostic errors. Ongoing efforts by the Agency for Health Care Research and Quality have supported pragmatic measurement approaches for health organizations to build a diagnostic safety program and identify and learn from diagnostic errors such as those described in the Measure Dx resource [ 9 ]. One proposed and promising solution for hospitals to improve diagnostic surveillance is to build on existing efforts to collect patient safety data, root cause analyses, or other forms of case reviews for quality improvement purposes. Cases that have already been reviewed or investigated in the organization for general patient safety and quality purposes may be able to inform or be rereviewed for information and learning opportunities specific to diagnostic safety. Widely used case-based learning methodologies in particular, such as the “Learning From Every Death” initiative developed at Mayo Clinic [ 13 ] used both nationally and worldwide, offer an excellent opportunity for hospitals to augment their existing quality and safety efforts and support diagnostic safety.
Clinical notes in electronic health records (EHRs) written by health providers in free-text format are rich sources of a patient’s diagnoses and care trajectory through hospitalization time. Approaches to processing free text, such as through natural language processing (NLP) and machine learning (ML), have demonstrated significant opportunities to improve quality and safety within health care organizations in diverse applications [ 14 - 16 ] such as cancer research [ 17 , 18 ] and infection prediction [ 19 ] to sleep issues [ 20 ] and neurological outcome prediction [ 21 ]. Besides its use in the diagnostic process, ML models proved to have added benefits when used in diagnostic error identification [ 22 , 23 ]. However, despite significant progress and evidence about the use of these ML and NLP approaches to improve patient safety, the use of ML and NLP approaches to diagnostic safety and surveillance has largely remained untapped. A 2022 study demonstrates how an academic medical center’s implementation of an NLP-based algorithm to flag safety event reports for manual review enabled early detection of emerging diagnostic risks from large volumes of safety reports, and was among the first to apply an NLP approach to safety event reports to facilitate identification of COVID-19 related diagnostic errors [ 24 ]. Meanwhile, progress in the use of data mining approaches to develop electronic trigger tools offers promising methods to detect potential diagnostic events, promote organizational learning, and support the monitoring of data prospectively to identify patients at high risk for future adverse events [ 25 ]. To our knowledge, however, NLP has not yet been applied to case review data to facilitate the identification of diagnostic errors and understand its features and sources.
While free-text formatted clinical notes provide unique opportunities to incorporate ML models, the lack of reliable labels to represent diagnostic errors often limits the use of clinical notes for diagnostic safety surveillance efforts. The opportunity to train ML and NLP algorithms to identify diagnostic errors and opportunities depends on the collation of EHR data with existing efforts to identify diagnostic errors such as through case review findings from the Safety Learning System (SLS). To further explore the potential for this approach to be used to improve diagnostic safety surveillance, a rigorous evaluation of the feasibility and potential of using EHR and existing case review data is needed.
We hypothesized that ML and NLP methods can be applied to train models based on available case review data to examine content potentially related to diagnostic errors within EHR clinical notes. These approaches automatically identify features or information from free text using controlled vocabularies, rule sets, reference dictionaries, or lexicons.
Data Sets and Case Review Approach
We analyzed SLS data from 1 large health system comprised of 10 hospitals in the mid-Atlantic region of the United States. The SLS is one example of a holistic case review methodology delivered by health care organizations in the United States and globally. Established in 2015, the SLS builds upon the Mayo Clinic Mortality Review System of Huddleston et al [ 13 ] to review and analyze EHR data from patient mortality cases to find safety issues that could be found and mitigated. This approach was designed to enhance current quality improvement projects done within health organizations, providing a perspective and strategy based on the Safety II lens and rooted in the belief that every death provides an opportunity to improve care. With a Safety II lens, participating organizations use a holistic case review methodology designed to identify vulnerabilities in systems and processes of care delivery. Reviewers identify and translate these into different categories and labels to (1) define and quantify types of process of care and system failures contributing to adverse outcomes (errors) and (2) identify the components of the process of care and system failures that when fixed will improve performance (opportunities for improvement [OFIs]).
To ensure a sufficient cross-sampling of patients across different specialties and areas, patients are selected for case reviews at this health system based on their primary provider service line category (eg, medicine, surgery, etc) and hospital length of stay; patients in primary and ambulatory care settings are not included for case review selection. The case review process occurs according to the standardized SLS methodology and recommendations [ 13 , 26 ], and between at least 1 physician and 1 nurse within the health system who have both received training in the SLS approach. The case review outcome and identification of OFIs, including diagnostic OFIs, relies on the reviewer’s consensus of any findings and through multiple multidisciplinary and multispecialty meetings that may involve a committee Chair member, clinical department leader, or escalation to other leadership.
We obtained SLS data from February 2016 to September 2021; data in later years were available but not included because of key changes to the case selection process made during and in response to the COVID-19 pandemic. All hospitalized adult patients older than 18 years were included for analysis, regardless of their hospitalization outcome (eg, mortality or discharge location). Pediatric and neonatal patients were excluded.
Ethical Considerations
The original data collection and study protocol was approved by the institutional review board (00001245) at MedStar Health Research Institute on August 26, 2019.
Data Extraction
Medical record number, encounter number, length of stay, age, date of birth, sex, diagnosis at the time of admission (ie, ICD-10 [ International Statistical Classification of Diseases, Tenth Revision ] diagnosis codes), mortality, OFI categories (eg, delayed or missed diagnosis and diagnostic opportunities), number of identified OFIs and diagnosis issues (eg, the accuracy of diagnosis and confirmation or fixation bias) were the features and patient identifiers which were extracted from SLS data [ 13 , 26 ].
Because chart reviews generally occur at a single point in time within the patient’s care trajectory, they often do not contain information or details of the patient’s full hospital course. However, clinical notes written by health care providers are rich sources of patient’s health status throughout their hospitalization period [ 27 - 29 ]. Therefore, to supplement these chart review data, we additionally extracted and included all clinical notes from the EHR for patients who could be matched by patient identifiers (eg, encounter number and date of birth).
Coding Diagnostic Errors
Case reviewers can select any number of labels to describe a diagnosis issue or an OFI identified and agreed upon by consensus. For this study, diagnostic errors were defined by the available features from chart review pertaining to diagnosis and impacting the timeliness, accuracy, or communication of a diagnosis. Our definition of diagnostic errors was limited to the categories identified during chart reviews and recorded within the SLS data set; therefore, our diagnostic error definition does not include all aspects of the definition developed by the NASEM report [ 3 ]. Table 1 describes the SLS categories and values that were labeled as diagnostic errors and used to train our classification models. Patients were coded as having experienced a diagnostic error if one or more of the conditions listed in Table 1 were identified in their SLS case review.
Feature from chart reviews | Value to indicate diagnostic error |
OFI category | Delayed or missed diagnosis |
OFI category | Diagnostic opportunities |
Diagnosis issues | accuracy of diagnosis |
Diagnosis issues | Accuracy of interpretation of laboratory or test results |
Diagnosis issues | Squirrel (red herring lab or test results) |
Diagnosis issues | Confirmation or fixation bias |
Diagnosis issues | Appropriateness of chosen tests or equipment given the patient’s differential diagnosis |
a OFI: opportunity for improvement.
NLP Approach
We used an NLP approach on critical incident reporting system data to explore the features and risk of diagnostic error among hospitalized patients.
Features From Free-Text Data
Descriptive statistical analyses were performed to identify any differences among age, length of stay, and mortality between the female and male patients who had experienced diagnostic errors.
All EHR clinical notes were transformed to lowercase. Extra white spaces, numbers, punctuations, and stop words were removed and words were stemmed. The term frequency-inverse document frequency (TF-IDF) matrix was calculated for each clinical note using the bag-of-words from the preprocessed EHR clinical notes [ 30 ]. TF-IDF is a statistical measure that evaluates how relevant a word is to a document in a collection of documents and is a popular method to translate free text to numerical features in training ML models. The TF-IDF of a word in a document is calculated by multiplying 2 metrics: the number of times a word appeared in a document and the inverse document frequency of the word across a set of documents. TF-IDF is computationally efficient and easy to interpret. We excluded the most frequent words that had appeared in more than 95% of the EHR clinical notes, as these frequent words do not provide information to help with the classification. Moreover, we excluded the rare words that appeared in less than 5% of the EHR clinical notes [ 31 ].
In a TF-IDF matrix, the number of rows corresponds to the unique patients, and the number of columns represents the unique words found in EHR clinical notes. There are numerous unique words used in EHR clinical notes; therefore, the TF-IDF approach provides a high-dimensional input matrix for the classification task. The high-dimensional input matrix can lead to training inaccurate classifiers. To overcome that issue, we used the chi-square statistical test to select the most relevant words to identify diagnostic errors; therefore, if P values associated with a word (also called a feature) are less than .05, that word is selected and included in the feature matrix to train ML classification models.
Classification Models
In lieu of an existing model with the same objective in the literature, a simple logistic regression model was trained as the baseline classifier to identify patients within SLS data who were at higher risk of diagnostic error. Moreover, 3 forms of logistic regression models with regularization functions were trained on this data to compare classification performances and identify the best-performing model [ 32 ]: Least Absolute Shrinkage and Selection Operator (LASSO), Ridge, and Elastic Net.
- LASSO: for a more accurate prediction, LASSO regularization is used with a logistic regression model. The LASSO procedure encourages simple, sparse models which has fewer parameters in a way that the estimated coefficient of features with less effect will be set to zero. This characteristic makes LASSO well-suited for models showing high levels of multicollinearity or variable selection and parameter elimination is needed. LASSO is also called L1 regularization.
- Ridge: also called L2 regularization, Ridge is a regularization method used for models suffering from multicollinearity or high-dimensional feature space. Ridge regularization keeps all the features regardless of their effect on the model. However, it pushes the estimated coefficient of features with less effect toward zero to minimize their effect on the classification outcome. This characteristic of Ridge makes it well-suited when most features impact the outcome variable.
- Elastic Net: a logistic regression model with Elastic Net regularization is a weighted combination of LASSO (L1) and Ridge (L2) regularizations [ 33 ]. Elastic Net can remove the effect of the insignificant features by setting their estimated coefficient to zero and lower the effect of the less significant features by pushing their estimated coefficient toward zero while adding more weights to the more important features. From implementation and interpretation aspects, the Elastic Net model is simple to use. Such characteristics make this model an accepted baseline in ML-based studies [ 34 ].
The hyperparameters of the 3 classification models were optimized through cross-validation. All the analyses were conducted using Python 3 (Python Software Foundation).
Classification Performance Metrics
We calculated 7 common performance metrics reported for binary classifiers to compare the performance of the 4 classification models: area under receiver operating characteristics curve (AUROC), sensitivity or recall or true positive rate, specificity or true negative rate, positive predictive value (PPV) or precision, negative predictive value (NPV), F 1 -score, and area under precision-recall curve (AUPRC). The 7 metrics take values between 0 and 1. Values closer to 1 indicate a well-performing classifier. Multimedia Appendix 1 presents the definition of the performance metrics used in this study. Figure 1 presents the summary of the methods used in this analysis.

Descriptive Summary
In total, there were 2184 unique patient records within SLS data from February 2016 to September 2021. EHR clinical notes were cross-matched, extracted, and included in analyses for 1704 (78%) of these SLS patient records. Of those patients with cross-matched EHR data, 126 (7.4%) patients had been identified by case reviewers as having experienced at least 1 diagnostic error. A total number of 20,848 EHR clinical notes associated with the 1704 unique patients were used in this study.
Patients who had experienced diagnostic errors were grouped by sex: 59 (7.1%) of the 830 women and 67 (7.7%) of the 874 men in the larger cross-matched sample had been found to have a diagnostic error. Table 2 presents the descriptive statistics between female and male patient groups. We applied the Wilcoxon rank sum test for numerical features (ie, age and length of stay), and the chi-square test for mortality rate, admission diagnosis, and admission department or specialty. Patients in the female group were older than the male group by a median of 72 (IQR 66-80) versus a median of 67 (IQR 57-76; P =.02). Compared to the male group, female patients who experienced diagnostic error had higher rates of being admitted through general or internal medicine (69.5% vs 47.8%; P =.01), lower rates of cardiovascular-related admitted diagnosis (11.9% vs 28.4%; P =.02), and lower rates of being admitted through neurology department (2.3% vs 13.4%; P =.04). We observed no differences between groups in mortality rates and length of stay.
Patients who experienced diagnostic error | All patients | |||||||||
Female group (n=59) | Male group (n=67) | Female group (n=830) | Male group (n=874) | |||||||
Age (in years), median (IQR) | 72 (66-80) | 67 (57-76) | 72 (62-83) | 69 (59-79) | ||||||
African American | 38 (64) | 42 (62) | 429 (51.7) | 429 (51.7) | ||||||
Asian | 0 (0) | 0 (0) | 12 (1.4) | 12 (1.4) | ||||||
Multiple | 0 (0) | 0 (0) | 2 (0.2) | 2 (0.2) | ||||||
Not recorded | 4 (6) | 2 (2.9) | 30 (3.6) | 30 (3.6) | ||||||
White | 11 (18) | 21 (31.3) | 310 (37.3) | 310 (37.3) | ||||||
Other | 6 (10) | 2 (2.9) | 47 (5.7) | 47 (5.7) | ||||||
Length of stay in days, median (IQR) | 4 (6-10) | 4 (8-14) | 7 (4-12) | 8 (4-12) | ||||||
Count | 25 (42) | 29 (43) | 456 (54.9) | 459 (52.5) | ||||||
General or internal medicine or hospitalist | 41 (69) | 32 (47) | 427 (51.4) | 389 (44.5) | ||||||
Cardiology | 5 (8) | 12 (17) | 99 (11.9) | 131 (14.9) | ||||||
Critical care | 6 (10) | 6 (8) | 117 (14.1) | 142 (16.2) | ||||||
Neurology | 2 (3) | 9 (13) | 75 (9) | 90 (10.3) | ||||||
Pulmonary | 1 (1) | 1 (1) | 22 (2.6) | 31 (3.5) | ||||||
Other | 4 (6) | 7 (10) | 90 (10.8) | 91 (10.4) | ||||||
Cardiovascular | 7 (11) | 19 (28) | 154 (18.6) | 167 (19.1) | ||||||
Respiratory | 7 (11) | 5 (7) | 88 (10.6) | 69 (7.9) | ||||||
Sepsis | 7 (11) | 4 (5) | 65 (7.8) | 63 (7.2) | ||||||
Altered mental status | 1 (1) | 2 (2) | 36 (4.3) | 28 (3.2) | ||||||
Diabetes | 1 (1) | 1 (1) | 6 (0.7) | 3 (0.3) | ||||||
Other | 23 (38) | 21 (31) | 244 (29.4) | 270 (30.9) | ||||||
General care | 54 (91) | 60 (89) | 144 (17.3) | 179 (20.5) | ||||||
Critical care | 5 (8.5) | 7 (10) | 686 (82.7) | 695 (79.5) | ||||||
categories, n (%) | ||||||||||
Delayed or missed diagnosis | 43 (72) | 46 (68) | 43 (5.2) | 46 (5.3) | ||||||
Diagnostic opportunities | 15 (25) | 16 (23) | 15 (1.8) | 16 (1.8) | ||||||
Accuracy of diagnosis | 1 (1) | 4 (6) | 1 (0.1) | 4 (0.5) | ||||||
Accuracy of interpretation of laboratory or test results | 0 (0) | 0 (0) | 0 (0) | 0 (0) | ||||||
Squirrel (red herring lab or test results) | 0 (0) | 1 (1) | 0 (0) | 1 (0.1) | ||||||
Confirmation or fixation bias | 0 (0) | 0 (0) | 0 (0) | 0 (0) | ||||||
Appropriateness of chosen tests or equipment given patient’s differential diagnosis | 1 (1) | 0 (0) | 1 (0.1) | 0 (0) | ||||||
Critical care | 15 (25) | 22 (32) | 273 (32.9) | 318 (36.4) | ||||||
Emergency department | 17 (28) | 18 (26) | 81 (9.8) | 76 (8.7) | ||||||
General care | 27 (45) | 27 (40) | 290 (34.9) | 285 (32.6) |
Classification Models’ Performance
Clinical notes were preprocessed for TF-IDF feature calculation. The bag-of-words included 2227 words, and each word was considered a feature (see Table S1 in Multimedia Appendix 2 for the top 100 words). We found that abscess, ascend, abnormality, scant, pair, and prefer were the top 5 features with the highest positive estimated coefficient (0.42 to 0.28); post, select, gave, muscl, hours, and unrespons were the top 5 features with the highest negative coefficients (–0.35 to –0.26). After applying the chi-square test, 250 features with a P value less than .05 were selected for the modeling process. All 4 ML classifiers were trained using the 250 selected features.
Table 3 presents the performances of the simple logistic regression and 3 regularized logistic regression models (LASSO, Ridge, and Elastic Net). The Ridge model achieved the highest AUROC (0.885), specificity (0.797), PPV (0.24), NPV (0.981), and F 1 -score (0.369) in classifying patients who were at higher risk of diagnostic errors among hospitalized patients in SLS system. The simple logistic regression model obtained the highest AUPRC (0.537). The simple logistic regression model classified all patients as the ones with diagnostic errors; therefore, it achieved a sensitivity of 1, and specificity and NPV of 0.
Figures 2 and 3 present the receiver operating characteristics curves and precision-recall curves for the 4 classifiers in this study. Models that give ROC curves closer to the top-left corner indicate a better performance. The AUROC values represent the probability that a patient who experienced a diagnostic error, chosen at random, is ranked higher by the Ridge model than a randomly chosen patient who did not experience a diagnostic error. The higher value of AUPRC indicates that the Ridge model can identify patients who experienced diagnostic errors more precisely with fewer false positives compared to LASSO and Elastic Net models.
Simple logistic regression | LASSO | Ridge | Elastic Net | |
AUROC | 0.5 | 0.846 | 0.885 | 0.859 |
Sensitivity | 1.0 | 0.802 | 0.802 | 0.802 |
Specificity | 0 | 0.733 | 0.797 | 0.742 |
Positive predictive value | 0.074 | 0.193 | 0.24 | 0.199 |
Negative predictive value | 0 | 0.979 | 0.981 | 0.979 |
-score | 0.138 | 0.312 | 0.369 | 0.319 |
AUPRC | 0.537 | 0.361 | 0.491 | 0.411 |
a LASSO: Least Absolute Shrinkage and Selection Operator.
b AUROC: area under receiver operating characteristics curve.
c AUPRC: area under precision-recall curve.

Principal Findings
Our contribution is 2-fold; first, we integrated 2 data sources that are currently used by and available to many organizations across the United States, SLS and EHR data, to explore the use of ML and NLP algorithms to help identify diagnostic errors among hospitalized patients. Although case review methodologies offer rich insights into systems errors and OFIs, the predefined pull-down menus and structured data labels typically do not capture all the necessary clinical and contextual details that are considered by reviewers. Therefore, a large portion of these case review data are stored in free-text narratives that typically record key information and judgments decided upon by the multidisciplinary reviewers. However, given persistent issues of staff shortage and lack of time in health care settings, it is becoming increasingly important to lower the burden of systematic EHR data reviews for health care providers while maintaining the review systems in place. Second, any developed ML and NLP approaches can potentially be incorporated to generate a diagnostic error risk score for each patient. The predicted risk score can be used in identifying and prioritizing patients for focused chart reviews, thus lowering the burden of systematic EHR data reviews for health care providers while maintaining the review systems in place.
To our knowledge, this study is the first attempt to apply and test several different ML classification models to identify diagnostic errors within routinely collected organizational case review data. Despite a substantial body of literature about the prevalence of diagnostic errors in hospital settings, current efforts to identify diagnostic errors generally rely on reviews of patient cases and data by clinical or quality teams that often are resource-intensive. ML classification models and NLP techniques offer an opportunity to generate diagnostic error risk scores to sort through large data sets and identify signals of potential diagnostic errors that can be flagged for further review. However, these classification models require a high number of observations (and identified diagnostic errors) to perform well, which might not be feasible for health organizations that are just beginning to identify diagnostic errors or may have limited personnel and efforts to perform high numbers of case reviews. In this study, we accessed nearly 2000 patient records (and of those, only 126 cases of diagnostic errors), which is considered to be a limited data sample size in the field of ML. However, techniques, such as feature selection and n-fold cross-validations, can potentially be approaches to address small sample size challenges [ 35 ].
Using the results of the simple logistic regression model as the baseline performance, we found that 3 regularization functions, namely LASSO, Ridge, and Elastic Net, boosted the performance of the baseline model. The Ridge model outperformed the rest of the models in terms of multiple performance metrics: AUROC of 0.885, specificity of 0.797, PPV of 0.24, NPV of 0.981, and F 1 -score of 0.369. The Ridge algorithm tries to keep all features in the model even the features with a slight effect on the classification outcome. Since the patterns pointing at a diagnostic error were subtle in the clinical notes, even a small effect of a feature on the model’s classification outcome could be important for the classification model to learn. On the other hand, the LASSO algorithm rigorously removes features that have a small effect on the classification outcome. The Elastic Net model is a weighted combination of LASSO and Ridge. The performance results presented in Table 3 show that the values achieved by the Elastic Net model lie between those of the LASSO and Ridge models.
Insights From Diagnostic Errors Within Free-Text Clinical Notes
We did not find the free text formatted clinical notes in the EHR to reflect any sort of direct language around diagnostic errors. Our analysis identified no use of the terms misdiagnosis, missed diagnosis, or diagnostic error within clinical notes, finding instead more subtle signals pointing at diagnostic errors such as “there may be a chance of misreading the test,” or “insufficient data to make a diagnosis.” Our findings demonstrate that NLP algorithms can be used to identify such patterns and find the associations between diagnostic errors and the subtle signals in the clinical notes. A natural extension of this work can focus on using other feature extraction methods, such as Bidirectional Encoder Representations from Transformers contextualized word embeddings, and explore the use of the pretrained language models for this objective.
We found that the presence of terms, such as abscess, abnormality, “cp” (chest pain) , and dialysis in a patient’s EHR clinical note were associated with reviewer-identified diagnostic errors ( Multimedia Appendix 2 ). Misinterpretation of chest pain, specifically among female patients, has the potential to cause a cardiovascular-related diagnosis error [ 36 ]. Patients with chronic kidney disease are at higher risk of cardiovascular complications [ 37 ]. Missing such risk for a patient who is on dialysis, adds to the risk of diagnostic error.
Clinical and System Implications Around Diagnostic Inequity
Diagnostic inequity is defined as “the presence of preventable unwarranted variations in diagnostic process among population groups that are socially, economically, demographically, or geographically disadvantaged” [ 38 ]. Despite persistent and well-documented disparities in health care access and outcomes across different population groups, few studies have examined the association between diagnostic errors and health care disparities [ 39 ]. Recent evidence supports the notion that variation in diagnostic error rates across demographic groups may exist, particularly across sex. A systematic review of diagnostic errors in the emergency department, for example, found that female sex and non-White race were often associated with increased risk for diagnostic errors across several clinical conditions in emergency settings [ 40 ]. In cardiovascular medicine, a national cohort study of acute myocardial infarctions found that women were nearly twice as likely as men to receive the wrong initial diagnosis following signs of a heart attack [ 41 ]. Despite efforts to understand and reduce disparities in diagnosis and treatment, women not only continue to be understudied, underdiagnosed, and undertreated in cardiovascular medicine [ 42 ] but also may experience longer lengths of time to diagnosis than men in most patterns of disease diagnosis [ 43 ].
The analysis of case review data and other system-based data (eg, patient safety events or incident reporting) by subsets offer an opportunity to identify events in vulnerable patient populations and help sensitize clinicians to potential biases within the diagnostic process. To explore sex differences in diagnostic errors within our case review data, we statistically compared demographic and clinical differences between female and male patients who had been identified in case reviews as having experienced diagnostic error or errors. We found that of those patients who had experienced diagnostic error or errors, the female group of patients were older, had higher rates of being admitted through general or internal medicine or hospitalist (vs specialty) departments, and had lower rates of having a cardiovascular diagnosis on admission. These preliminary results of this study revealed unexpected differences between male and female diagnostic error groups, offering novel insights that warrant further investigation to fully understand the mechanisms underlying these relationships and their implications for clinical decision-making and practice. Future uses of NLP can potentially support clinical and system-based approaches to capture and increase the evidence around structural biases or disparities in diagnoses. Individual cases from these types of data sources could be used as example narratives to engage clinicians and improve clinician learning, contributing to the development of tailored clinician and systemic interventions that can improve quality and equity throughout the diagnostic process.
Limitations
This study has several limitations. Our definition of diagnostic errors was limited to the categories and labels used within the SLS data set, reviewer interpretations of cases (subject to reviewer bias), and does not include all aspects of the definition developed by the NASEM report [ 3 ]. Despite several continued differences in definitions of diagnostic error in the peer-reviewed literature [ 8 ], we recommend that quality and safety teams within health systems use the NASEM definition for diagnostic error—including errors in communicating the diagnosis to the patient—to develop any definitions, categories, or labels used in their case review and surveillance initiatives. Although a time-consuming task, future studies could consider EHR data chart reviews to have the ground truth for the diagnostic error cases and add to the accuracy of the data set used for training the ML classifiers. Additionally, due to staffing challenges and shifting organizational priorities, case review selection varies by hospital and has changed over time, resulting in a relatively small sample size and also introducing the potential for bias. Our data came from a single health system and may reflect the specific language, culture, and practices occurring within the system and therefore may not be similar to that of other health systems. To enhance the external validity and generalizability of results, future efforts and research studies should consider the random selection of cases to evaluate both diagnostic and general quality issues within the organization; studies with larger sample sizes can build on our preliminary findings and test differences between clinical subgroups. Finally, our classification models were developed and evaluated based on a retrospective cohort from EHR; therefore, the performance may deteriorate when the method is applied to real-time data. Further work or future studies should be conducted to prospectively validate the models.
Conclusions
We performed an NLP approach and compared 4 techniques to classify patients who were at a higher risk of experiencing diagnostic error during hospitalization. Our findings demonstrate that NLP can be a potential solution to more effectively identifying and selecting potential diagnostic error cases for review, and therefore, reducing the case review burden.
Acknowledgments
This work was supported by the Agency for Health Care Research and Quality (grant 5R18HS027280-02).
Conflicts of Interest
None declared.
Binary classification performance metrics.
The Estimated Coefficient from the Ridge Model.
- Newman-Toker DE, Wang Z, Zhu Y, Nassery N, Saber Tehrani AS, Schaffer AC, et al. Rate of diagnostic errors and serious misdiagnosis-related harms for major vascular events, infections, and cancers: toward a national incidence estimate using the "Big Three". Diagnosis (Berl). Feb 23, 2021;8(1):67-84. [ FREE Full text ] [ CrossRef ] [ Medline ]
- Gunderson CG, Bilan VP, Holleck JL, Nickerson P, Cherry BM, Chui P, et al. Prevalence of harmful diagnostic errors in hospitalised adults: a systematic review and meta-analysis. BMJ Qual Saf. Dec 2020;29(12):1008-1018. [ CrossRef ] [ Medline ]
- Ball JR, Miller BT, Balogh EP. Improving Diagnosis in Health Care. Washington, DC. National Academies Press; 2015:472.
- Hautz WE, Kämmer JE, Hautz SC, Sauter TC, Zwaan L, Exadaktylos AK, et al. Diagnostic error increases mortality and length of hospital stay in patients presenting through the emergency room. Scand J Trauma Resusc Emerg Med. May 08, 2019;27(1):54. [ FREE Full text ] [ CrossRef ] [ Medline ]
- Gupta A, Snyder A, Kachalia A, Flanders S, Saint S, Chopra V. Malpractice claims related to diagnostic errors in the hospital. BMJ Qual Saf. Aug 09, 2017;27(1):53-60. [ CrossRef ] [ Medline ]
- Zwaan L, Singh H. Diagnostic error in hospitals: finding forests not just the big trees. BMJ Qual Saf. 2020;29(12):961-964. [ CrossRef ] [ Medline ]
- Singh H. Editorial: helping health care organizations to define diagnostic errors as missed opportunities in diagnosis. Jt Comm J Qual Patient Saf. 2014;40(3):99-101. [ CrossRef ] [ Medline ]
- Giardina TD, Hunte H, Hill MA, Heimlich SL, Singh H, Smith KM. Defining diagnostic error: a scoping review to assess the impact of the national academies' report improving diagnosis in health care. J Patient Saf. 2022;18(8):770-778. [ FREE Full text ] [ CrossRef ] [ Medline ]
- Measure Dx: a resource to identify, analyze, and learn from diagnostic safety events. AHRQ: Agency for Healthcare Research and Quality. URL: https://www.ahrq.gov/patient-safety/settings/multiple/measure-dx.html [accessed 2023-06-22]
- Zwaan L, de Bruijne M, Wagner C, Thijs A, Smits M, van der Wal G, et al. Patient record review of the incidence, consequences, and causes of diagnostic adverse events. Arch Intern Med. 2010;170(12):1015-1021. [ CrossRef ] [ Medline ]
- Singh H. Diagnostic errors: moving beyond 'no respect' and getting ready for prime time. BMJ Qual Saf. 2013;22(10):789-792. [ FREE Full text ] [ CrossRef ] [ Medline ]
- Zwaan L, El-Kareh R, Meyer AND, Hooftman J, Singh H. Advancing diagnostic safety research: results of a systematic research priority setting exercise. J Gen Intern Med. 2021;36(10):2943-2951. [ FREE Full text ] [ CrossRef ] [ Medline ]
- Huddleston JM, Diedrich DA, Kinsey GC, Enzler MJ, Manning DM. Learning from every death. J Patient Saf. 2014;10(1):6-12. [ CrossRef ] [ Medline ]
- Arnaud E, Elbattah M, Gignon M, Dequen G. NLP-based prediction of medical specialties at hospital admission using triage notes. 2021. Presented at: IEEE 9th International Conference on Healthcare Informatics (ICHI); August 09-12, 2021; Victoria, British Columbia, Canada. [ CrossRef ]
- Bansal A, Saraswat B, Sharma B, Nayan S, Kathuria K. Data extraction and integration from unstructured electronic health records. 2023. Presented at: 2023 3rd International Conference on Technological Advancements in Computational Sciences (ICTACS); November 01-03, 2023; Tashkent, Uzbekistan. [ CrossRef ]
- Sezgin E, Hussain S, Rust S, Huang Y. Extracting medical information from free-text and unstructured patient-generated health data using natural language processing methods: feasibility study with real-world data. JMIR Form Res. 2023;7:e43014. [ FREE Full text ] [ CrossRef ] [ Medline ]
- Asri H, Mousannif H, Moatassime HA, Noel T. Using machine learning algorithms for breast cancer risk prediction and diagnosis. Procedia Comput Sci. 2016;83:1064-1069. [ CrossRef ]
- Goldenberg SL, Nir G, Salcudean SE. A new era: artificial intelligence and machine learning in prostate cancer. Nat Rev Urol. 2019;16(7):391-403. [ CrossRef ] [ Medline ]
- Henry KE, Adams R, Parent C, Soleimani H, Sridharan A, Johnson L, et al. Factors driving provider adoption of the TREWS machine learning-based early warning system and its effects on sepsis treatment timing. Nat Med. 2022;28(7):1447-1454. [ CrossRef ] [ Medline ]
- Nasiri S, Gari D. Attentive adversarial network for large-scale sleep staging. 2020. Presented at: Proceedings of the 5th Machine Learning for Healthcare Conference, PMLR; September, 2020:457-478; Atlanta, GA, USA. URL: https://proceedings.mlr.press/v126/nasiri20a.html
- Tabaie A, Nemati S, Allen JW, Chung C, Queiroga F, Kuk W, et al. Assessing contribution of higher order clinical risk factors to prediction of outcome in aneurysmal subarachnoid hemorrhage patients. AMIA Annu Symp Proc. 2019;2019:848-856. [ FREE Full text ] [ Medline ]
- Cheheltani R, King N, Lee S, North B, Kovarik D, Evans-Molina C, et al. Predicting misdiagnosed adult-onset type 1 diabetes using machine learning. Diabetes Res Clin Pract. 2022;191:110029. [ FREE Full text ] [ CrossRef ] [ Medline ]
- Hercus C, Hudaib A. Delirium misdiagnosis risk in psychiatry: a machine learning-logistic regression predictive algorithm. BMC Health Serv Res. 2020;20(1):151. [ FREE Full text ] [ CrossRef ] [ Medline ]
- Shen L, Levie A, Singh H, Murray K, Desai S. Harnessing event report data to identify diagnostic error during the COVID-19 pandemic. Jt Comm J Qual Patient Saf. 2022;48(2):71-80. [ FREE Full text ] [ CrossRef ] [ Medline ]
- Murphy DR, Meyer AN, Sittig DF, Meeks DW, Thomas EJ, Singh H. Application of electronic trigger tools to identify targets for improving diagnostic safety. BMJ Qual Saf. 2019;28(2):151-159. [ FREE Full text ] [ CrossRef ] [ Medline ]
- The safety learning system®. Discover the Hidden Causes of Patient Harm. URL: http://hbhealthcaresafety.org/safety-learning-system/ [accessed 2023-06-22]
- Alsentzer E, Murphy J, Boag W, Weng W, Jindi D, Naumann T, et al. Publicly available clinical BERT embeddings. 2019. Presented at: Proceedings of the 2nd Clinical Natural Language Processing Workshop; June, 2019; Minneapolis, Minnesota, USA. [ CrossRef ]
- Huang K, Altosaar A, Ranganath R. ClinicalBERT: modeling clinical notes and predicting hospital readmission. ArXiv. Preprint posted online on April 10, 2019. 2019:190405342. [ CrossRef ]
- Zhang D, Thadajarassiri J, Sen C, Rundensteiner E. Time-aware transformer-based network for clinical notes series prediction. 2020. Presented at: Proceedings of the 5th Machine Learning for Healthcare Conference; September, 2020; Worcester, MA, USA. URL: https://proceedings.mlr.press/v126/zhang20c/zhang20c.pdf
- Jones KS. A statistical interpretation of term specificity and its application in retrieval. J Doc. 1972;28(1):11-21. [ CrossRef ]
- Tabaie A, Sengupta S, Pruitt ZM, Fong A. A natural language processing approach to categorise contributing factors from patient safety event reports. BMJ Health Care Inform. 2023;30(1):e100731. [ FREE Full text ] [ CrossRef ] [ Medline ]
- Aheto JMK, Duah HO, Agbadi P, Nakua EK. A predictive model, and predictors of under-five child malaria prevalence in Ghana: how do LASSO, Ridge and Elastic Net regression approaches compare? Prev Med Rep. 2021;23:101475. [ FREE Full text ] [ CrossRef ] [ Medline ]
- Zou H, Trevor H. Regularization and variable selection via the Elastic Net. J R Stat Soc Series B Stat Methodol. 2005;67(2):301-320. [ CrossRef ]
- Lee SJ, Weinberg BD, Gore A, Banerjee I. A scalable natural language processing for inferring BT-RADS categorization from unstructured brain magnetic resonance reports. J Digit Imaging. 2020;33(6):1393-1400. [ FREE Full text ] [ CrossRef ] [ Medline ]
- Masino AJ, Harris MC, Forsyth D, Ostapenko S, Srinivasan L, Bonafide CP, et al. Machine learning models for early sepsis recognition in the neonatal intensive care unit using readily available electronic health record data. PLoS One. 2019;14(2):e0212665. [ FREE Full text ] [ CrossRef ] [ Medline ]
- Mehta PK, Bess C, Elias-Smale S, Vaccarino V, Quyyumi A, Pepine CJ, et al. Gender in cardiovascular medicine: chest pain and coronary artery disease. Eur Heart J. 2019;40(47):3819-3826. [ FREE Full text ] [ CrossRef ] [ Medline ]
- Jankowski J, Floege J, Fliser D, Böhm M, Marx N. Cardiovascular disease in chronic kidney disease: pathophysiological insights and therapeutic options. Circulation. 2021;143(11):1157-1172. [ FREE Full text ] [ CrossRef ] [ Medline ]
- Giardina TD, Woodard LD, Singh H. Advancing diagnostic equity through clinician engagement, community partnerships, and connected care. J Gen Intern Med. 2023;38(5):1293-1295. [ FREE Full text ] [ CrossRef ] [ Medline ]
- Ibrahim SA, Pronovost PJ. Diagnostic errors, health disparities, and artificial intelligence: a combination for health or harm? JAMA Health Forum. 2021;2(9):e212430. [ FREE Full text ] [ CrossRef ] [ Medline ]
- Newman-Toker DE, Peterson SM, Badihian S, Hassoon A, Nassery N, Parizadeh D, et al. Diagnostic Errors in the Emergency Department: A Systematic Review [internet]. Rockville (MD). Agency for Healthcare Research and Quality; 2022.
- Wu J, Gale CP, Hall M, Dondo TB, Metcalfe E, Oliver G, et al. Editor's choice—impact of initial hospital diagnosis on mortality for acute myocardial infarction: a national cohort study. Eur Heart J Acute Cardiovasc Care. 2018;7(2):139-148. [ FREE Full text ] [ CrossRef ] [ Medline ]
- Burgess S. Understudied, under-recognized, underdiagnosed, and undertreated: sex-based disparities in cardiovascular medicine. Circ Cardiovasc Interv. 2022;15(2):e011714. [ CrossRef ] [ Medline ]
- Sun TY, Hardin J, Nieva HR, Natarajan K, Cheng R, Ryan P, et al. Large-scale characterization of gender differences in diagnosis prevalence and time to diagnosis. MedRxiv. Preprint posted online on October 16, 2023. 2023. [ FREE Full text ] [ CrossRef ] [ Medline ]
Abbreviations
area under precision-recall curve |
area under receiver operating characteristic curve |
electronic health record |
International Statistical Classification of Diseases, Tenth Revision |
Least Absolute Shrinkage and Selection Operator |
machine learning |
National Academies of Science, Engineering, and Medicine |
natural language processing |
negative predictive value |
opportunity for improvement |
positive predictive value |
Safety Learning System |
term frequency-inverse document frequency |
Edited by S Ma, T Leung; submitted 17.07.23; peer-reviewed by D Chrimes, M Elbattah; comments to author 18.01.24; revised version received 21.03.24; accepted 20.06.24; published 26.08.24.
©Azade Tabaie, Alberta Tran, Tony Calabria, Sonita S Bennett, Arianna Milicia, William Weintraub, William James Gallagher, John Yosaitis, Laura C Schubel, Mary A Hill, Kelly Michelle Smith, Kristen Miller. Originally published in the Journal of Medical Internet Research (https://www.jmir.org), 26.08.2024.
This is an open-access article distributed under the terms of the Creative Commons Attribution License (https://creativecommons.org/licenses/by/4.0/), which permits unrestricted use, distribution, and reproduction in any medium, provided the original work, first published in the Journal of Medical Internet Research (ISSN 1438-8871), is properly cited. The complete bibliographic information, a link to the original publication on https://www.jmir.org/, as well as this copyright and license information must be included.
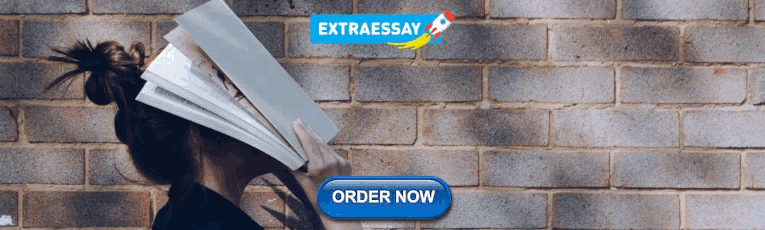
IMAGES
COMMENTS
A case study is a research method that involves an in-depth examination and analysis of a particular phenomenon or case, such as an individual, organization, community, event, or situation.
A case study is a detailed study of a specific subject, such as a person, group, place, event, organization, or phenomenon. Case studies are commonly used in social, educational, clinical, and business research. A case study research design usually involves qualitative methods, but quantitative methods are sometimes also used.
Case Study Methodology Case studies in research are both unique and uniquely confusing. The term case study is confusing because the same term is used multiple ways. The term can refer to the methodology, that is, a system of frameworks used to design a study, or the methods used to conduct it. Or, case study can refer to a type of academic writing that typically delves into a problem, process ...
Abstract A case study is one of the most commonly used methodologies of social research. This article attempts to look into the various dimensions of a case study research strategy, the different epistemological strands which determine the particular case study type and approach adopted in the field, discusses the factors which can enhance the effectiveness of a case study research, and the ...
Definition, Methods, and Examples. Case study methodology offers researchers an exciting opportunity to explore intricate phenomena within specific contexts using a wide range of data sources and collection methods. It is highly pertinent in health and social sciences, environmental studies, social work, education, and business studies.
What is a case study? Whereas quantitative methods look at phenomena at scale, case study research looks at a concept or phenomenon in considerable detail. While analyzing a single case can help understand one perspective regarding the object of research inquiry, analyzing multiple cases can help obtain a more holistic sense of the topic or issue.
Case study research involves an in-depth, detailed examination of a single case, such as a person, group, event, organization, or location, to explore causation in order to find underlying principles and gain insight for further research.
Abstract Qualitative case study methodology enables researchers to conduct an in-depth exploration of intricate phenomena within some specific context. By keeping in mind research students, this article presents a systematic step-by-step guide to conduct a case study in the business discipline. Research students belonging to said discipline face issues in terms of clarity, selection, and ...
A case study is a detailed study of a specific subject, such as a person, group, place, event, organisation, or phenomenon. Case studies are commonly used in social, educational, clinical, and business research. A case study research design usually involves qualitative methods, but quantitative methods are sometimes also used.
To facilitate application of these principles, clear examples of research questions, study propositions and the different types of case study designs are provided.
This study employed a qualitative case study methodology. The case study method is a research strategy that aims to gain an in-depth understanding of a specific phenomenon by collecting and ...
Abstract This chapter explores case study as a major approach to research and evaluation. After first noting various contexts in which case studies are commonly used, the chapter focuses on case study research directly Strengths and potential problematic issues are outlined and then key phases of the process. The chapter emphasizes how important it is to design the case, to collect and ...
Definition of case study. Case study method enables a researcher to closely examine the data within a specific context. In most cases, a case study method selects a small geograph ical area or a ...
Résumé. Case study is a common methodology in the social sciences (management, psychology, science of education, political science, sociology). A lot of methodological papers have been dedicated to case study but, paradoxically, the question "what is a case?" has been less studied.
Definitions of qualitative case study research. Case study research is an investigation and analysis of a single or collective case, intended to capture the complexity of the object of study (Stake, 1995 ). Qualitative case study research, as described by Stake ( 1995 ), draws together "naturalistic, holistic, ethnographic, phenomenological ...
The case study approach allows in-depth, multi-faceted explorations of complex issues in their real-life settings. The value of the case study approach is well recognised in the fields of business, law and policy, but somewhat less so in health services research. Based on our experiences of conducting several health-related case studies, we reflect on the different types of case study design ...
This book aims to provide case‐study researchers with a step‐by‐step practical guide to "help them conduct the study with the required degree of rigour" (p. xi). It seeks to "demonstrate that the case study is indeed a scientific method" (p. 104) and to show "the usefulness of the case method as one tool in the researcher's ...
This chapter reviews the strengths and limitations of case study as a research method in social sciences. It provides an account of an evidence base to justify why a case study is best suitable for some research questions and why not for some other research...
Case study research is a type of qualitative research design. It's often used in the social sciences because it involves observing subjects, or cases, in their natural setting, with minimal interference from the researcher. In the case study method, researchers pose a specific question about an individual or group to test their theories or ...
Learn how to conduct and analyze a case study as a qualitative research method. Download the PDF article from ResearchGate and explore related topics.
Abstract For students and novice researchers, the choice of qualitative approach and subsequent alignment among problems, research questions, data collection, and data analysis can be particularly tricky. Therefore, the purpose of this paper is to provide a concise explanation of four common qualitative approaches, case study, ethnography, narrative, and phenomenology, demonstrating how each ...
Case study methodology has grown in popularity within Health Services Research (HSR). However, its use and merit as a methodology are frequently criticized due to its flexible approach and inconsistent application. Nevertheless, case study methodology is well suited to HSR because it can track and e …
New technology is ushering in exciting possibilities that can help long-term monitoring programs evolve and benefit from next generation methods. Monitoring northern spotted owls provides an excellent case study. Research wildlife biologist Damon Lesmeister's research integrates artificial intelligence and passive acoustic monitoring. This ...
A case study is a research approach that is used to generate an in-depth, multi-faceted understanding of a complex issue in its real-life context. It is an established research design that is used extensively in a wide variety of disciplines, particularly in the social sciences. A case study can be defined in a variety of ways (Table 5 ), the ...
The remainder of this paper is organized as follows: Sect. 1 is the introduction, Sect. 2 describes the research method, Sect. 3 presents the case study, ... This study also presented a case study of the assessment of the optimal scheme for startup companies engaging in token financing. Practically, this new framework could provide ...
We conducted a rigorous evaluation of the feasibility and potential to use electronic health records clinical notes and existing case review data. Methods: Safety Learning System case review data from 1 large health system composed of 10 hospitals in the mid-Atlantic region of the United States from February 2016 to September 2021 were analyzed.
Abstract This article reviews the use of case study research for both practical and theoretical issues especially in management field with the emphasis on management of technology and innovation. Many researchers commented on the methodological issues of the case study research from their point of view thus, presenting a comprehensive framework was missing. We try representing a general ...
Therefore, further research involving a large-scale multi-center study is needed. In conclusion, TAC is rarely used for the treatment of CI in Japan. However, based on previous reports and our data, TAC appears to be a safe and effective method for preventing second-trimester loss and preterm delivery in high-risk patients.
The region under study is divided into areas of homogeneous rain hazard. For each homogeneous area, a probability model is fitted using state-of-the-art statistical methods, for each accumulation ...