
- Advanced Search
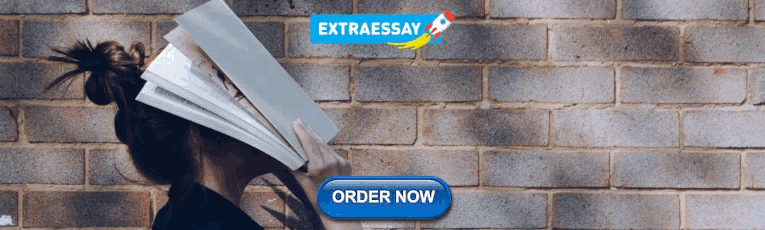
E-voting in Literature: Analyzing Nations’ Interest
New citation alert added.
This alert has been successfully added and will be sent to:
You will be notified whenever a record that you have chosen has been cited.
To manage your alert preferences, click on the button below.
New Citation Alert!
Please log in to your account
Information & Contributors
Bibliometrics & citations, index terms.
General and reference
Document types
General literature
Reference works
Social and professional topics
User characteristics
Geographic characteristics
Recommendations
Electronic voting security 10 years after the help america vote act.
Merle S. King, executive director of the Center for Election Systems at Kennesaw State University, and Brian Hancock, director of voting system testing and certification at the US Election Assistance Commission (EAC), discuss e-voting security 10 years ...
E-voting in the 2005 local elections in Estonia and the broader impact for future e-voting projects
In this project, we analyze the introduction of voting by internet (e-voting) in political elections. In particular, we focus on the Estonian municipal elections held on 16 October 2005, where the possibility of casting a vote via the internet was newly ...
New Research Results for Electronic Voting
Voting systems have become controversial in the years following the multiple election disasters that occurred in the United States during and after 2000. Of particular note were the electronic voting (e-voting) systems that were widely deployed to ...
Information
Published in.

Association for Computing Machinery
New York, NY, United States
Publication History
Permissions, check for updates, author tags.
- Electoral Systems
- Electronic Voting
- Internet Voting
- Remote Voting
- Voting Machines
- e-Democracy
- e-Participation
- Research-article
- Refereed limited
Contributors
Other metrics, bibliometrics, article metrics.
- 0 Total Citations
- 106 Total Downloads
- Downloads (Last 12 months) 106
- Downloads (Last 6 weeks) 0
View Options
Login options.
Check if you have access through your login credentials or your institution to get full access on this article.
Full Access
View options.
View or Download as a PDF file.
View online with eReader .
HTML Format
View this article in HTML Format.
Share this Publication link
Copying failed.
Share on social media
Affiliations, export citations.
- Please download or close your previous search result export first before starting a new bulk export. Preview is not available. By clicking download, a status dialog will open to start the export process. The process may take a few minutes but once it finishes a file will be downloadable from your browser. You may continue to browse the DL while the export process is in progress. Download
- Download citation
- Copy citation
We are preparing your search results for download ...
We will inform you here when the file is ready.
Your file of search results citations is now ready.
Your search export query has expired. Please try again.
- Political Science
- Political Methodology
DESIGN AND IMPLEMENTATION OF AN E-VOTING SYSTEM
- January 2019
- Affiliation: Nnamdi Azikiwe University, Awka

- Nnamdi Azikiwe University, Awka
Abstract and Figures

Discover the world's research
- 25+ million members
- 160+ million publication pages
- 2.3+ billion citations

- Madasu Hanmandlu

- Sarah P. Everett
- Kristen K. Greene

- Manuel Egele
- David Brumley
- Yanick Fratantonio
- Christopher Kruegel

- Mugurel Ionut Andreica

- Angelos Stavrou
- Recruit researchers
- Join for free
- Login Email Tip: Most researchers use their institutional email address as their ResearchGate login Password Forgot password? Keep me logged in Log in or Continue with Google Welcome back! Please log in. Email · Hint Tip: Most researchers use their institutional email address as their ResearchGate login Password Forgot password? Keep me logged in Log in or Continue with Google No account? Sign up
IEEE Account
- Change Username/Password
- Update Address
Purchase Details
- Payment Options
- Order History
- View Purchased Documents
Profile Information
- Communications Preferences
- Profession and Education
- Technical Interests
- US & Canada: +1 800 678 4333
- Worldwide: +1 732 981 0060
- Contact & Support
- About IEEE Xplore
- Accessibility
- Terms of Use
- Nondiscrimination Policy
- Privacy & Opting Out of Cookies
A not-for-profit organization, IEEE is the world's largest technical professional organization dedicated to advancing technology for the benefit of humanity. © Copyright 2024 IEEE - All rights reserved. Use of this web site signifies your agreement to the terms and conditions.
Updates to our Terms of Use
We are updating our Terms of Use. Please carefully review the updated Terms before proceeding to our website.

- Environment
- International
- Civil Rights
- Entertainment
- Personal Injury
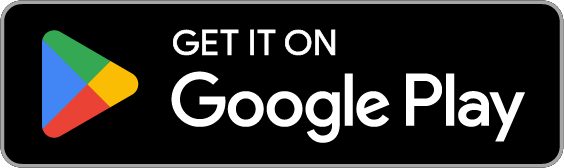
Colorado clerk urges Supreme Court to block trial over voting machine password leak
Former Mesa County clerk-recorder Tina Peters claims she has constitutional immunity from prosecution by "hostile state actors."
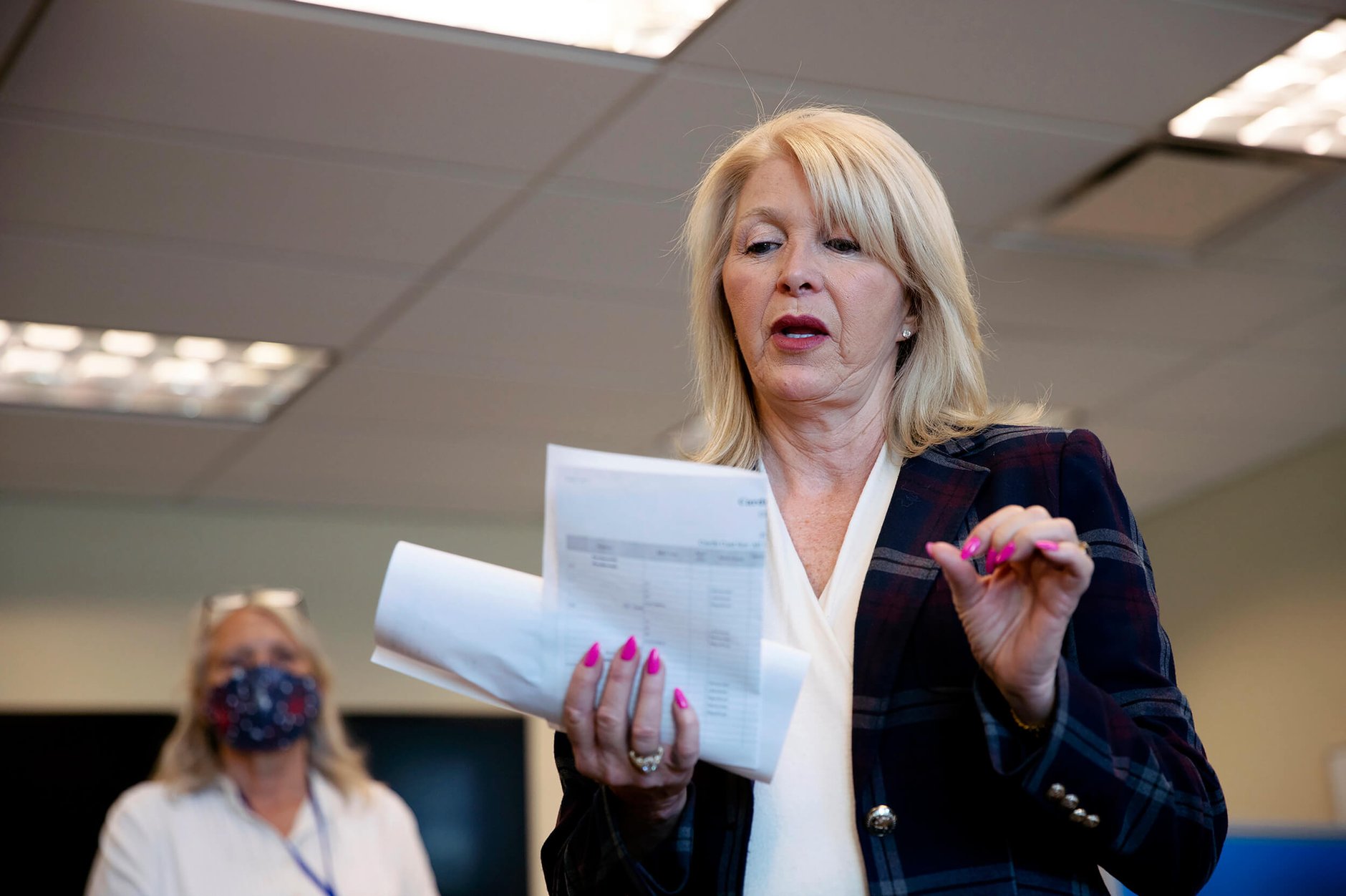
WASHINGTON (CN) — A former Colorado election official asked the Supreme Court on Friday for emergency intervention to prevent the start of her trial over leaked voting machine passwords.
Tina Peters, who served as Mesa County’s clerk and recorder during the 2020 election cycle, faces criminal charges for giving a security company unauthorized access to voting machines during a system update in 2021.
In an attempt to avert her July 29 trial, Peters filed an emergency application with the justices, claiming federal elections protections give her immunity from the state’s prosecution.
“An injunction stopping the state trial while this court considers this case is necessary to preserve the status quo and prevent an irreparable injury to the institutional interests of the federal government and to Ms. Peters’ right not to be subjected to state trial for executing her duty under federal law,” Peters wrote in her application.
Peters said prosecutors’ charges relate to her duty to preserve election records as Colorado’s chief election official. She argued her actions reflected official responsibilities under federal law, giving her immunity under the 14th Amendment.
The controversy kicked off when Colorado announced an upgrade to their election management system. Concerned that records of the 2020 presidential would be deleted, prosecutors say Peters hired Gerald Wood to help her back up Dominion voting machines.
A video was then posted on the social media site Telegram and the Gateway Pundit blog of the country’s equipment being updated including system passwords.
Peters’ claims of unusual activity and deleted records were vindicated but an investigation identified those instances as human error, not election fraud.
Colorado prosecutors charged Peters with seven felony counts related to attempting to influence a public servant, impersonation and identity theft. Peters also faces several misdemeanor charges of official misconduct, violating her duties and failing to comply with the secretary of state’s requirements.
Peters pleaded not guilty , claiming the charges were a political hitjob due to her support for former President Donald Trump.
Colorado Secretary of State Jena Griswold sued to remove Peters as clerk and recorder for the 2022 elections. Peters decided to run for secretary of state to unseat Griswold. A judge blocked Peters from overseeing county elections and she lost the Republican primary by more than 15 points.
Peters filed a federal lawsuit against a slew of state officials and U.S. Attorney General Merrick Garland, claiming she was wrongly prosecuted in violation of her First Amendment rights.
U.S. District Judge Nina Wang, a Joe Biden appointee, granted Mesa County District Attorney Daniel Rubinstein’s request to dismiss the suit in January. Wang said Peters could present her challenges in state court.
In February, the 10th Circuit refused to delay Peters’ trial while she challenged the ruling. The appeals court terminated her appeal in June.
At the Supreme Court, Peters asked the justices to postpone her trial so they could review her immunity appeal. Peters said supremacy clause immunity had been extensively litigated by the lower courts and the justices’ intervention was overdue.
“Arising as it does from the Constitution’s foundational allocation of power between the federal and state governments, it is critically important that the immunity provided by the supremacy clause for federal actors contending with hostile state officials be properly applied,” Peters wrote.
Subscribe to Closing Arguments
Sign up for new weekly newsletter Closing Arguments to get the latest about ongoing trials, major litigation and hot cases and rulings in courthouses around the U.S. and the world.
Additional Reads
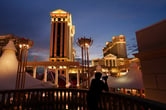

Journal of Materials Chemistry A
Leveraging machine learning in porous media †.

* Corresponding authors
a School of Engineering, Newcastle University, Newcastle Upon Tyne, UK E-mail: [email protected] , [email protected]
b School of Mathematics and Physics, University of Portsmouth, Portsmouth, UK
c Department of Mechanical Engineering, University of Kashan, Kashan, Iran
d School of Mechanical Engineering, Iran University of Science and Technology, Iran
e Department of Chemical Engineering, University of Waterloo, Waterloo, Canada
f School of Metallurgy and Materials Engineering, College of Engineering, University of Tehran, Tehran, Iran
g Department of Chemical Engineering, University of Manchester, Oxford Road, Manchester, UK E-mail: [email protected]
h School of Climate Change and Adaptation, University of Prince Edward Island, Charlottetown, PEI, Canada
i Department of Chemical Engineering & Materials Science, University of Southern California, USA
j Department of Chemistry and Bioscience, Aalborg University, Niels Bohrs Vej 8A, Esbjerg 6700, Denmark
k Department of Chemical and Petroleum Engineering, Khalifa University, Abu Dhabi, UAE
The emergence of artificial intelligence (AI) and, more particularly, machine learning (ML), has had a significant impact on engineering and the fundamental sciences, resulting in advances in various fields. The use of ML has significantly enhanced data processing and analysis, eliciting the development of new and improved technologies. Specifically, ML is projected to play an increasingly significant role in helping researchers better understand and predict the behavior of porous media. Furthermore, ML models will be able to make use of sizable datasets, such as subsurface data and experiments, to produce accurate predictions and simulations of porous media systems. This capability could help optimize the design of porous materials for specific applications and improve the effectiveness of industrial processes. To this end, this review paper attempts to provide an overview of the present status quo in this context, i.e. , the interface of ML and porous media in six different applications, namely, heat exchanger and storage, energy storage and combustion, electrochemical devices, hydrocarbon reservoirs, carbon capture and sequestration, and groundwater, stressing the advances made in the application of ML to porous media and offering insights into the challenges and opportunities for future research. Each section also entails a supplementary database of the literature as a spreadsheet, which includes the details of ML models, datasets, key findings, etc. , and mentions relevant available online datasets that can be used to train ML models. Future research trends include employing hybrid models by combining ML models with physics-based models of porous media to improve predictions concerning accuracy and interpretability.
- This article is part of the themed collection: Journal of Materials Chemistry A Recent Review Articles
Supplementary files
- Supplementary information PDF (127K)
- Supplementary information XLSX (217K)
Article information

Download Citation
Permissions.

Leveraging machine learning in porous media
M. Delpisheh, B. Ebrahimpour, A. Fattahi, M. Siavashi, H. Mir, H. Mashhadimoslem, M. A. Abdol, M. Ghorbani, J. Shokri, D. Niblett, K. Khosravi, S. Rahimi, S. M. Alirahmi, H. Yu, A. Elkamel, V. Niasar and M. Mamlouk, J. Mater. Chem. A , 2024, Advance Article , DOI: 10.1039/D4TA00251B
This article is licensed under a Creative Commons Attribution 3.0 Unported Licence . You can use material from this article in other publications without requesting further permissions from the RSC, provided that the correct acknowledgement is given.
Read more about how to correctly acknowledge RSC content .
Social activity
Search articles by author.
This article has not yet been cited.
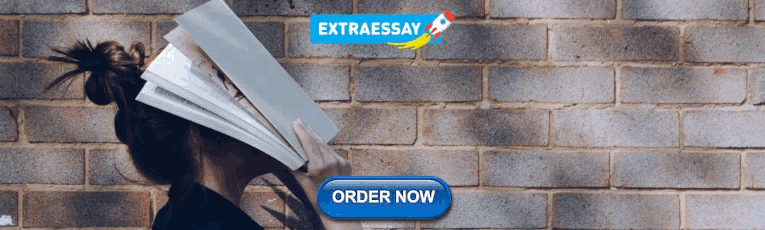
Advertisements
Information
- Author Services
Initiatives
You are accessing a machine-readable page. In order to be human-readable, please install an RSS reader.
All articles published by MDPI are made immediately available worldwide under an open access license. No special permission is required to reuse all or part of the article published by MDPI, including figures and tables. For articles published under an open access Creative Common CC BY license, any part of the article may be reused without permission provided that the original article is clearly cited. For more information, please refer to https://www.mdpi.com/openaccess .
Feature papers represent the most advanced research with significant potential for high impact in the field. A Feature Paper should be a substantial original Article that involves several techniques or approaches, provides an outlook for future research directions and describes possible research applications.
Feature papers are submitted upon individual invitation or recommendation by the scientific editors and must receive positive feedback from the reviewers.
Editor’s Choice articles are based on recommendations by the scientific editors of MDPI journals from around the world. Editors select a small number of articles recently published in the journal that they believe will be particularly interesting to readers, or important in the respective research area. The aim is to provide a snapshot of some of the most exciting work published in the various research areas of the journal.
Original Submission Date Received: .
- Active Journals
- Find a Journal
- Proceedings Series
- For Authors
- For Reviewers
- For Editors
- For Librarians
- For Publishers
- For Societies
- For Conference Organizers
- Open Access Policy
- Institutional Open Access Program
- Special Issues Guidelines
- Editorial Process
- Research and Publication Ethics
- Article Processing Charges
- Testimonials
- Preprints.org
- SciProfiles
- Encyclopedia
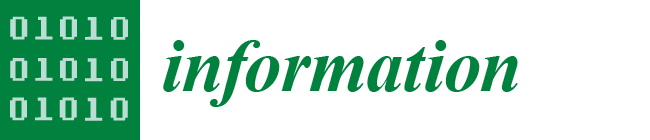
Article Menu
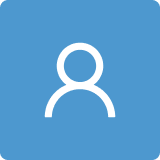
- Subscribe SciFeed
- Recommended Articles
- Author Biographies
- Google Scholar
- on Google Scholar
- Table of Contents
Find support for a specific problem in the support section of our website.
Please let us know what you think of our products and services.
Visit our dedicated information section to learn more about MDPI.
JSmol Viewer
Sinner: a reward-sensitive algorithm for imbalanced malware classification using neural networks with experience replay.
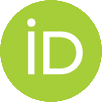
1. Introduction
- It presents SINNER, i.e., a DRL-based classifier, which leverages a reward function slightly modified compared to that proposed in [ 28 ].
- It provides an extended benchmark analysis that involves a state-of-the art DL-based malware family classifier that can deal with class skew at the algorithm level.
2. Preliminaries
2.1. malware analysis, 2.1.1. static analysis, 2.1.2. dynamic analysis, 2.2. deep reinforcement learning, 2.2.1. deep q-network, 2.2.2. double deep q-network, 2.2.3. dueling network, 2.2.4. prioritized experience replay, 3. related work, 3.1. deep learning for api-based malware classification, 3.2. imbalanced multi-class malware classification, 3.3. deep reinforcement learning for malware analysis, 3.4. motivation, 4. methodology, 4.1. environment setting.
- Training data provide the observation space S ; therefore, each training sample represents an observation s t for a specific timestep t . Note that S ∈ R m × n , with m the number of samples within the training set and n the number of features.
- The action space A consists of all known labels for classes. Therefore, given K classes, A = { 1 , 2 , . . . , K } , i.e., | A | = K .
- The reward function f R represents the main component of the proposed cost-sensitive approach according to the following formula: r = f R ( s t , a t , l t ) = λ t = m l t − 1 Λ , if a t = l t − λ t , otherwise (7) where Λ = | | m 1 − 1 , m 2 − 1 , . . . , m K − 1 | | 2 , l t refers to the true label of the observed s t , and m l t represents the number of samples in the l t -th class. In this way, the agent can adjust the learning to be more sensitive to minority classes because the higher the m l t , the lower the λ t . Furthermore, in [ 28 ], the authors found that the use of the normalization factor Λ improves the learning performance, having as the main effect the scaling of r so that it falls in [ 0 , 1 ] .
- Finally, according to the definition of S , the states-transition probability ϕ is deterministic; thus, the agent advances from s t to s t + 1 , as determined by the order of the samples within S .
4.2. Reward-Sensitive Training Analysis
4.3. modified reward function, 5. experimental setup, 5.1. approaches used for benchmark.
- According to the literature review provided in Section 3 , the following DL models are selected and combined with the cost-sensitive strategies proposed in [ 11 , 62 ], the working principle of which is shown in Table 2 : - LSTM [ 79 ]: This popular model belongs to the class of RNNs. The structure of this network consists of three gates in its hidden layers: an input gate, an output gate, and a forget gate. These entities form the so-called memory cell, which traces the data flow, i.e., remembers or forgets information over time. In such a way, LSTMs can maintain long-term dependencies on sequential data. The LSTM used in our experiments has 100 units (size of hidden cells) connected to a final layer, which is a multi-class classification layer ( K nodes, each having a softmax activation). Between these connections, there is a dropout layer to mitigate overfitting using a chance of 20 % of randomly discarding a neuron. - BiLSTM [ 80 ]: This differs from the aforementioned model regarding the adoption of a bidirectional layer, which enables the forwarding and backwarding of the input to two separate recurrent nets, both of which are connected to the same output layer (having the same properties of the LSTM last layer). - BiGRU [ 81 ]: This method uses a bidirectional approach to analyze sequences in both directions as the previous DL model described, involving as a main model the so-called GRU, which is an LSTM variant. In fact, the GRU has gating units (update and reset gates) that control the flow of information inside each unit without having separate memory cells. The update gate helps the model to determine how much of the past information (from previous time steps) must be passed to the future. In contrast, the reset gate is used by the model to determine how much of the past information is to be forgotten. In this case, the dropout rate is fixed so that a neuron can be discarded with a probability of 0.3. - TabNet [ 82 ]: This is a DL architecture specifically designed for tabular data. During each of the N s t e p s decision steps, such a model exploits a sequential attention mechanism to select N d features useful to perform a specific prediction, according to the aggregated information collected (the aggregation in N a dimension is realized by the attentive transformer component of the TabNet encoder). This property enhances the explainability of the model (because of the presence of a feature masking component, which is part of the TabNet decoder, i.e., the module delegated to reconstruct the features generated by the encoder). According to the suggestions provided by the authors of the original paper, N s t e p s = 3 , N a = N d = 16 . Lastly, all the above DL models optimize the loss function using the Adam optimization algorithm and sampling mini-batches of 128, 64, and 1024 training samples for LSTM, bidirectional models, and TabNet, respectively. A number of 50 epochs were considered for the first three models, whereas the last model was trained on 100 training epochs.
- RTF [ 16 ]: This model consists of an ensemble of ξ homogeneous (equivalent structure of base estimators) pre-trained transformer models. Each is fine-tuned to implement a sequence classification layer using a subset of ξ training data obtained as a result of a stratified (to retain the class distribution coming from the original set) bootstrap sampling technique. Each i -th model, with i = 1 , ⋯ , ξ , generates a probability employed in a majority voting schema, which leads to a traditional bagging method (exploiting the robustness of such an algorithmic procedure with respect to class skew). BERT and CANINE (including CANINE-C and CANINE-S variants) were evaluated as pre-trained models. The setting was the same as that proposed in the experimental evaluation of the original article (Table 7 in [ 16 ]).
5.2. Datasets Selected for This Study
- APIs statically extracted from the PE structure of malware samples collected from two main providers, i.e., VirusShare ( https://virusshare.com/ , accessed on 16 May 2024) and VirusSample ( https://www.virussamples.com/ , accessed on 16 May 2024), which were labeled using the VirusTotal ( https://www.virustotal.com/ , accessed on 16 May 2024) engine [ 83 ]. These two datasets differ in the number of samples and malware families within each, but they share the feature space size as in [ 16 ].
- API sequences traced by dynamically analyzing each malware sample using the Cuckoo sandbox. These are collected in two different datasets, namely Catak [ 63 ] and Oliveira [ 84 ]. Note that while Catak represents the state-of-the-art in the category of multi-class malware classification problems using APIs, the Oliveira dataset was released as suitable for binary classification problems. Therefore, only the malware contained in the latter dataset were used and labeled, so they were assigned to the malware family indicated by the VirusTotal service. In this assignment process, statistical units without associated classes and malware families with fewer than 100 samples were discarded [ 16 ].
5.3. Metrics
- The F1 score, as the harmonic mean of precision (PREC) and true positive rate (TPR), defined as PREC = TP TP + FP and TPR = TP TP + FN , i.e., F 1 score = TP TP + FP + FN 2 . Specifically, the macro-averaged metric was examined because it assumes that each class has the same impact regardless of its skew [ 86 ].
- The area under receiver operating characteristic curve (AUC) computed by identifying the surface below the graph that relates the false positive rate (FPR) to the TPR.
5.4. Setting of the Proposed Methodology
5.5. hardware settings and implementation details, 6. results and discussion, 6.1. reward influence analysis.
- The three algorithms that achieve the highest F1 score among all evaluated DRL configurations in the case of the Catak dataset are dueling DDQN, dueling DQN with PER and dueling DQN. This trio shares a key finding: the reward function used is Equation ( 11 ). With the same configuration, adopting Equation ( 7 ) results in a performance degradation that is more evident in the F1 score than in the AUC.
- Using the Oliveira dataset, among the top three performers, there are, once more, dueling DQN and dueling DDQN, followed by the dueling of DDQN with PER. As before, the best results are obtained using r s as the reward; in fact, it is remarkable that the three opposite algorithms achieve F1 scores that are half of those achieved by the algorithms using Equation ( 11 ). Similarly, using r s rather than r improves the AUC.
- Using the PER technique for the VirusSample dataset brings benefits that are reflected in the performance achieved by the dueling of DQN (which performs effectively also using the ER not prioritized) and DDQN algorithms, respectively. The dueling of DQN with PER, adopting r s for reward-sensitive training, reaches an F1 close to 80%, outperforming the same algorithm configuration trained using Equation ( 7 ) by ∼ 20 % . An improvement in F1 scores is also found for the remaining two algorithms using r s instead of r . In contrast, the opposite trend is shown by evaluating the AUC.
- The benefit achieved by introducing the revised reward formulation is confirmed for the VirusShare dataset, for which the top performers are given by the following three algorithms: dueling DDQN, dueling DQN, and dueling DDQN with PER.
Click here to enlarge figure
6.2. Performance Comparison
- Table 4 reveals that the cost-sensitive strategies proposed in [ 11 ] and [ 62 ] are uniquely beneficial to the BiLSTM model when using the VirusShare dataset. In fact, the remaining three DL algorithms do not produce satisfactory performance, with AUC values (∼0.5) indicating that the algorithms performed random classifications. However, the classification metric scores obtained by the BiLSTM algorithm do not reach the state-of-the-art performance achieved by the RTF algorithm. In addition, it appears to be extremely disadvantageous from the perspective of the required training time, which is the maximum in this list, regardless of the cost-sensitive strategy adopted. The proposed methodology outperforms all of the competitors in terms of F1 score and prediction time. Specifically, SINNER achieves a value of the F1 that is approximately 2% higher compared to the same obtained by RTF, requiring significantly less prediction time. On the other hand, RTF remains advantageous in terms of both the training period and the AUC value that it yields.
- In contrast to the previous case, Table 5 indicates that the results achieved by BiGRU are comparable to those obtained by the BiLSTM. In particular, the scores produced are comparable when adopting a specific cost-sensitive strategy; however, between the two alternatives, the one based on the use of the custom loss function proposed in [ 62 ] performs better in timing performance and F1 score. Furthermore, LSTM and TabNet combined with cost-sensitive strategies are also ineffective in this test. However, as mentioned previously, bidirectional classifiers do not achieve performance comparable to RTF, which is targeted by SINNER. In fact, the proposed methodology generated the closest F1 score to that achieved by RTF, with an inference time that is again shorter, although it is ten times longer in learning time and 10 − 1 less in AUC.
- As shown in Table 6 , SINNER appears to be underperforming when compared with bidirectional DL models combined with the pair of cost-sensitive strategies and RTF, which remains the state-of-the-art model on the Catak dataset with impressive F1 scores and AUC values. Therefore, it appears that SINNER has difficulty learning using an observation space with a large number of variables ( n ).
- According to Table 7 , five top performers are identified using the Oliveira dataset, namely LSTM (that joins the top classifiers for the first time, indicating the presence of a temporal relationship between the variables in each statistical unit, which is a likely condition since the dataset is extracted from a dynamic analysis process); BiLSTM and BiGRU leveraging the strategy proposed in [ 62 ]; RTF, and SINNER. In particular, the five algorithms obtained F1 values between 0.561 and 0.569. While RTF remains the most advantageous in terms of AUC, SINNER stands out with respect to timing performance, requiring the second-lowest training time (LSTM is the top performer for this particular metric) and the lowest prediction time, which, as in all the cases discussed above, is approximately hundredths of seconds.
7. Conclusions
Author contributions, institutional review board statement, informed consent statement, data availability statement, conflicts of interest, abbreviations.
ADASYN | Adaptive Synthetic Sampling Approach for Imbalanced Learning |
AI | Artificial Intelligence |
API | Application Programming Interface |
AUC | Area Under Receiver Operating Characteristic Curve |
AutoML | Automated Machine Learning |
AV | Anti-Virus |
BERT | Bidirectional Encoder Representations from Transformers |
BiLSTM | Bidirectional Long Short-Term Memory |
BiGRU | Bidirectional Gated Recurrent Unit |
CANINE | Character Architecture with No Tokenization In Neural Encoders |
CART | Classification and Regression Tree |
CNN | Convolutional Neural Network |
DL | Deep Learning |
DNN | Deep Neural Network |
DRL | Deep Reinforcement Learning |
DQN | Deep Q-Network |
DDQN | Double Deep Q-Network |
ER | Experience Replay |
FN | False Negative |
FP | False Positive |
FPR | False Positive Rate |
GRU | Gated Recurrent Unit |
ICMDP | Imbalanced Classification Markov Decision Process |
IoC | Indicator of Compromise |
IoT | Internet of Things |
LIME | Local Interpretable Model-Agnostic Explanations |
LSTM | Long Short-Term Memory |
MDP | Markov Decision Process |
ML | Machine Learning |
MLP | Multilayer Perceptron |
NoisyNet | Noisy Network |
NLP | Natural Language Processing |
PE | Portable Executable |
PER | Prioritized Experience Replay |
PREC | Precision |
PPO | Proximal Policy Optimization |
RELU | Rectified Linear Unit |
RL | Reinforcement Learning |
RNN | Recurrent Neural Network |
ROS | Random Oversampler |
RTF | Random Transformer Forest |
RUS | Random Undersampler |
SHAP | Shapley Additive Explanations |
TabNet | Deep Neural Network Architecture for Tabular Data |
TD | Temporal Difference |
T-link | Tomek Links |
TN | True Negative |
TP | True Positive |
TPR | True Positive Rate |
US | Unbalanced Scenario |
XAI | Explainable Artificial Intelligence |
YARA | Yet Another Recursive Acronym |
- Aboaoja, F.A.; Zainal, A.; Ghaleb, F.A.; Al-rimy, B.A.S.; Eisa, T.A.E.; Elnour, A.A.H. Malware detection issues, challenges, and future directions: A survey. Appl. Sci. 2022 , 12 , 8482. [ Google Scholar ] [ CrossRef ]
- Sibi Chakkaravarthy, S.; Sangeetha, D.; Vaidehi, V. A Survey on malware analysis and mitigation techniques. Comput. Sci. Rev. 2019 , 32 , 1–23. [ Google Scholar ] [ CrossRef ]
- Xu, L.; Qiao, M. Yara rule enhancement using Bert-based strings language model. In Proceedings of the 2022 5th International Conference on Advanced Electronic Materials, Computers and Software Engineering (AEMCSE), Wuhan, China, 22–24 April 2022; pp. 221–224. [ Google Scholar ] [ CrossRef ]
- Coscia, A.; Dentamaro, V.; Galantucci, S.; Maci, A.; Pirlo, G. YAMME: A YAra-byte-signatures Metamorphic Mutation Engine. IEEE Trans. Inf. Forensics Secur. 2023 , 18 , 4530–4545. [ Google Scholar ] [ CrossRef ]
- Or-Meir, O.; Nissim, N.; Elovici, Y.; Rokach, L. Dynamic Malware Analysis in the Modern Era—A State of the Art Survey. ACM Comput. Surv. 2019 , 52 , 1–48. [ Google Scholar ] [ CrossRef ]
- Ucci, D.; Aniello, L.; Baldoni, R. Survey of machine learning techniques for malware analysis. Comput. Secur. 2019 , 81 , 123–147. [ Google Scholar ] [ CrossRef ]
- Liu, Y.; Wang, Y. A Robust Malware Detection System Using Deep Learning on API Calls. In Proceedings of the 2019 IEEE 3rd Information Technology, Networking, Electronic and Automation Control Conference (ITNEC), Chengdu, China, 15–17 March 2019; pp. 1456–1460. [ Google Scholar ] [ CrossRef ]
- Vinayakumar, R.; Alazab, M.; Soman, K.P.; Poornachandran, P.; Venkatraman, S. Robust Intelligent Malware Detection Using Deep Learning. IEEE Access 2019 , 7 , 46717–46738. [ Google Scholar ] [ CrossRef ]
- Li, C.; Cheng, Z.; Zhu, H.; Wang, L.; Lv, Q.; Wang, Y.; Li, N.; Sun, D. DMalNet: Dynamic malware analysis based on API feature engineering and graph learning. Comput. Secur. 2022 , 122 , 102872. [ Google Scholar ] [ CrossRef ]
- Rabadi, D.; Teo, S.G. Advanced Windows Methods on Malware Detection and Classification. In Proceedings of the ACSAC ’20: 36th Annual Computer Security Applications Conference, Austin, TX, USA, 7–11 December 2020; pp. 54–68. [ Google Scholar ] [ CrossRef ]
- Alzammam, A.; Binsalleeh, H.; AsSadhan, B.; Kyriakopoulos, K.G.; Lambotharan, S. Comparative Analysis on Imbalanced Multi-class Classification for Malware Samples using CNN. In Proceedings of the 2019 International Conference on Advances in the Emerging Computing Technologies (AECT), Al Madinah Al Munawwarah, Saudi Arabia, 10 February 2020; pp. 1–6. [ Google Scholar ] [ CrossRef ]
- Lu, Y.; Shetty, S. Multi-Class Malware Classification Using Deep Residual Network with Non-SoftMax Classifier. In Proceedings of the 2021 IEEE 22nd International Conference on Information Reuse and Integration for Data Science (IRI), Las Vegas, NV, USA, 10–12 August 2021; pp. 201–207. [ Google Scholar ] [ CrossRef ]
- Kumar, K.A.; Kumar, K.; Chiluka, N.L. Deep learning models for multi-class malware classification using Windows exe API calls. Int. J. Crit. Comput.-Based Syst. 2022 , 10 , 185–201. [ Google Scholar ] [ CrossRef ]
- Oak, R.; Du, M.; Yan, D.; Takawale, H.; Amit, I. Malware Detection on Highly Imbalanced Data through Sequence Modeling. In Proceedings of the 12th ACM Workshop on Artificial Intelligence and Security, Association for Computing Machinery, London, UK, 15 November 2019; pp. 37–48. [ Google Scholar ] [ CrossRef ]
- Ding, Y.; Wang, S.; Xing, J.; Zhang, X.; Qi, Z.; Fu, G.; Qiang, Q.; Sun, H.; Zhang, J. Malware Classification on Imbalanced Data through Self-Attention. In Proceedings of the 2020 IEEE 19th International Conference on Trust, Security and Privacy in Computing and Communications (TrustCom), Guangzhou, China, 29 December 2020–1 January 2021; pp. 154–161. [ Google Scholar ] [ CrossRef ]
- Demirkıran, F.; Çayır, A.; Ünal, U.; Dağ, H. An ensemble of pre-trained transformer models for imbalanced multiclass malware classification. Comput. Secur. 2022 , 121 , 102846. [ Google Scholar ] [ CrossRef ]
- Wang, H.; Singhal, A.; Liu, P. Tackling imbalanced data in cybersecurity with transfer learning: A case with ROP payload detection. Cybersecurity 2023 , 6 , 2. [ Google Scholar ] [ CrossRef ]
- Naim, O.; Cohen, D.; Ben-Gal, I. Malicious website identification using design attribute learning. Int. J. Inf. Secur. 2023 , 22 , 1207–1217. [ Google Scholar ] [ CrossRef ]
- Sewak, M.; Sahay, S.K.; Rathore, H. Deep reinforcement learning in the advanced cybersecurity threat detection and protection. Inf. Syst. Front. 2023 , 25 , 589–611. [ Google Scholar ] [ CrossRef ]
- Nguyen, T.T.; Reddi, V.J. Deep Reinforcement Learning for Cyber Security. IEEE Trans. Neural Networks Learn. Syst. 2021 , 34 , 3779–3795. [ Google Scholar ] [ CrossRef ] [ PubMed ]
- Kamal, H.; Gautam, S.; Mehrotra, D.; Sharif, M.S. Reinforcement Learning Model for Detecting Phishing Websites. In Cybersecurity and Artificial Intelligence: Transformational Strategies and Disruptive Innovation ; Jahankhani, H., Bowen, G., Sharif, M.S., Hussien, O., Eds.; Springer: Berlin, Germany, 2024; pp. 309–326. [ Google Scholar ] [ CrossRef ]
- Shen, S.; Xie, L.; Zhang, Y.; Wu, G.; Zhang, H.; Yu, S. Joint Differential Game and Double Deep Q-Networks for Suppressing Malware Spread in Industrial Internet of Things. IEEE Trans. Inf. Forensics Secur. 2023 , 18 , 5302–5315. [ Google Scholar ] [ CrossRef ]
- Lin, E.; Chen, Q.; Qi, X. Deep Reinforcement Learning for Imbalanced Classification. Appl. Intell. 2020 , 50 , 2488–2502. [ Google Scholar ] [ CrossRef ]
- Yuan, F.; Tian, T.; Shang, Y.; Lu, Y.; Liu, Y.; Tan, J. Malicious Domain Detection on Imbalanced Data with Deep Reinforcement Learning. In Proceedings of the Neural Information Processing ; Springer International Publishing: Berlin/Heidelberg, Germany, 2021; pp. 464–476. [ Google Scholar ] [ CrossRef ]
- Maci, A.; Santorsola, A.; Coscia, A.; Iannacone, A. Unbalanced Web Phishing Classification through Deep Reinforcement Learning. Computers 2023 , 12 , 118. [ Google Scholar ] [ CrossRef ]
- Maci, A.; Tamma, N.; Coscia, A. Deep Reinforcement Learning-based Malicious URL Detection with Feature Selection. In Proceedings of the 2024 IEEE 3rd International Conference on AI in Cybersecurity (ICAIC), Houston, TX, USA, 7–9 February 2024; pp. 1–7. [ Google Scholar ] [ CrossRef ]
- Maci., A.; Urbano., G.; Coscia., A. Deep Q-Networks for Imbalanced Multi-Class Malware Classification. In Proceedings of the 10th International Conference on Information Systems Security and Privacy—ICISSP, Roma, Italy, 26–28 February 2024; pp. 342–349. [ Google Scholar ] [ CrossRef ]
- Yang, J.; El-Bouri, R.; O’Donoghue, O.; Lachapelle, A.S.; Soltan, A.A.S.; Clifton, D.A. Deep Reinforcement Learning for Multi-class Imbalanced Training. arXiv 2022 , arXiv:2205.12070. [ Google Scholar ] [ CrossRef ]
- Mnih, V.; Kavukcuoglu, K.; Silver, D.; Rusu, A.A.; Veness, J.; Bellemare, M.G.; Graves, A.; Riedmiller, M.; Fidjeland, A.K.; Ostrovski, G.; et al. Human-level control through deep reinforcement learning. Nature 2015 , 518 , 529–533. [ Google Scholar ] [ CrossRef ]
- Hasselt, H.V.; Guez, A.; Silver, D. Deep reinforcement learning with double Q-Learning. In Proceedings of the Thirtieth AAAI Conference on Artificial Intelligence ; AAAI Press: Washington, DC, USA, 2016; Volume 30, pp. 2094–2100. [ Google Scholar ] [ CrossRef ]
- Wang, Z.; Schaul, T.; Hessel, M.; van Hasselt, H.; Lanctot, M.; de Freitas, N. Dueling Network Architectures for Deep Reinforcement Learning. In Proceedings of the 33rd International Conference on Machine Learning. PMLR, New York, NY, USA, 20–22 June 2016; Volume 48, pp. 1995–2003. [ Google Scholar ] [ CrossRef ]
- Schaul, T.; Quan, J.; Antonoglou, I.; Silver, D. Prioritized Experience Replay. arXiv 2016 , arXiv:1511.05952. [ Google Scholar ] [ CrossRef ]
- Fortunato, M.; Azar, M.G.; Piot, B.; Menick, J.; Osband, I.; Graves, A.; Mnih, V.; Munos, R.; Hassabis, D.; Pietquin, O.; et al. Noisy Networks for Exploration. arXiv 2019 , arXiv:1706.10295. [ Google Scholar ] [ CrossRef ]
- Alkhateeb, E.; Ghorbani, A.; Habibi Lashkari, A. Identifying Malware Packers through Multilayer Feature Engineering in Static Analysis. Information 2024 , 15 , 102. [ Google Scholar ] [ CrossRef ]
- Gibert, D. PE Parser: A Python package for Portable Executable files processing. Softw. Impacts 2022 , 13 , 100365. [ Google Scholar ] [ CrossRef ]
- Yamany, B.; Elsayed, M.S.; Jurcut, A.D.; Abdelbaki, N.; Azer, M.A. A Holistic Approach to Ransomware Classification: Leveraging Static and Dynamic Analysis with Visualization. Information 2024 , 15 , 46. [ Google Scholar ] [ CrossRef ]
- Brescia, W.; Maci, A.; Mascolo, S.; De Cicco, L. Safe Reinforcement Learning for Autonomous Navigation of a Driveable Vertical Mast Lift. IFAC-PapersOnLine 2023 , 56 , 9068–9073. [ Google Scholar ] [ CrossRef ]
- Han, D.; Mulyana, B.; Stankovic, V.; Cheng, S. A Survey on Deep Reinforcement Learning Algorithms for Robotic Manipulation. Sensors 2023 , 23 , 3762. [ Google Scholar ] [ CrossRef ]
- Tran, M.; Pham-Hi, D.; Bui, M. Optimizing Automated Trading Systems with Deep Reinforcement Learning. Algorithms 2023 , 16 , 23. [ Google Scholar ] [ CrossRef ]
- Hu, Y.J.; Lin, S.J. Deep Reinforcement Learning for Optimizing Finance Portfolio Management. In Proceedings of the 2019 Amity International Conference on Artificial Intelligence (AICAI), Dubai, United Arab Emirates, 4–6 February 2019; pp. 14–20. [ Google Scholar ] [ CrossRef ]
- Yang, J.; El-Bouri, R.; O’Donoghue, O.; Lachapelle, A.S.; Soltan, A.A.S.; Eyre, D.W.; Lu, L.; Clifton, D.A. Deep reinforcement learning for multi-class imbalanced training: Applications in healthcare. Mach. Learn. 2023 , 113 , 2655–2674. [ Google Scholar ] [ CrossRef ]
- Chen, T.; Liu, J.; Xiang, Y.; Niu, W.; Tong, E.; Han, Z. Adversarial attack and defense in reinforcement learning-from AI security view. Cybersecurity 2019 , 2 , 11. [ Google Scholar ] [ CrossRef ]
- Sutton, R.S.; Barto, A.G. Reinforcement Learning: An Introduction ; MIT Press: Cambridge, MA, USA, 2018; Available online: https://web.stanford.edu/class/psych209/Readings/SuttonBartoIPRLBook2ndEd.pdf (accessed on 18 January 2024).
- Wang, X.; Wang, S.; Liang, X.; Zhao, D.; Huang, J.; Xu, X.; Dai, B.; Miao, Q. Deep Reinforcement Learning: A Survey. IEEE Trans. Neural Netw. Learn. Syst. 2022 , 35 , 5064–5078. [ Google Scholar ] [ CrossRef ] [ PubMed ]
- Jang, B.; Kim, M.; Harerimana, G.; Kim, J.W. Q-Learning Algorithms: A Comprehensive Classification and Applications. IEEE Access 2019 , 7 , 133653–133667. [ Google Scholar ] [ CrossRef ]
- Zhang, H.; Yu, T. Taxonomy of Reinforcement Learning Algorithms. In Deep Reinforcement Learning: Fundamentals, Research and Applications ; Dong, H., Ding, Z., Zhang, S., Eds.; Springer: Singapore, 2020; pp. 125–133. [ Google Scholar ] [ CrossRef ]
- Berman, D.S.; Buczak, A.L.; Chavis, J.S.; Corbett, C.L. A Survey of Deep Learning Methods for Cyber Security. Information 2019 , 10 , 122. [ Google Scholar ] [ CrossRef ]
- Kolosnjaji, B.; Zarras, A.; Webster, G.; Eckert, C. Deep Learning for Classification of Malware System Call Sequences. In Proceedings of the AI 2016: Advances in Artificial Intelligence ; Springer International Publishing: Berlin/Heidelberg, Germany, 2016; pp. 137–149. [ Google Scholar ] [ CrossRef ]
- Meng, X.; Shan, Z.; Liu, F.; Zhao, B.; Han, J.; Wang, H.; Wang, J. MCSMGS: Malware Classification Model Based on Deep Learning. In Proceedings of the 2017 International Conference on Cyber-Enabled Distributed Computing and Knowledge Discovery (CyberC), Nanjing, China, 12–14 October 2017; pp. 272–275. [ Google Scholar ] [ CrossRef ]
- Maniath, S.; Ashok, A.; Poornachandran, P.; Sujadevi, V.; A.U., P.S.; Jan, S. Deep learning LSTM based ransomware detection. In Proceedings of the 2017 Recent Developments in Control, Automation & Power Engineering (RDCAPE), Noida, India, 26–27 October 2017; pp. 442–446. [ Google Scholar ] [ CrossRef ]
- Cannarile, A.; Dentamaro, V.; Galantucci, S.; Iannacone, A.; Impedovo, D.; Pirlo, G. Comparing Deep Learning and Shallow Learning Techniques for API Calls Malware Prediction: A Study. Appl. Sci. 2022 , 12 , 1645. [ Google Scholar ] [ CrossRef ]
- Cannarile, A.; Carrera, F.; Galantucci, S.; Iannacone, A.; Pirlo, G. A Study on Malware Detection and Classification Using the Analysis of API Calls Sequences Through Shallow Learning and Recurrent Neural Networks. In Proceedings of the 6th Italian Conference on Cybersecurit (ITASEC22), CEUR Workshop Proceedings. Rome, Italy, 20–23 June 2022; Available online: https://ceur-ws.org/Vol-3260/paper9.pdf (accessed on 8 March 2024).
- Li, C.; Lv, Q.; Li, N.; Wang, Y.; Sun, D.; Qiao, Y. A novel deep framework for dynamic malware detection based on API sequence intrinsic features. Comput. Secur. 2022 , 116 , 102686. [ Google Scholar ] [ CrossRef ]
- Chanajitt, R.; Pfahringer, B.; Gomes, H.M.; Yogarajan, V. Multiclass Malware Classification Using Either Static Opcodes or Dynamic API Calls. In Proceedings of the AI 2022: Advances in Artificial Intelligence ; Springer International Publishing: Berlin/Heidelberg, Germany, 2022; Volume 13728, pp. 427–441. [ Google Scholar ] [ CrossRef ]
- Maniriho, P.; Mahmood, A.N.; Chowdhury, M.J.M. API-MalDetect: Automated malware detection framework for windows based on API calls and deep learning techniques. J. Netw. Comput. Appl. 2023 , 218 , 103704. [ Google Scholar ] [ CrossRef ]
- Bensaoud, A.; Kalita, J. CNN-LSTM and transfer learning models for malware classification based on opcodes and API calls. Knowl.-Based Syst. 2024 , 290 , 111543. [ Google Scholar ] [ CrossRef ]
- Syeda, D.Z.; Asghar, M.N. Dynamic Malware Classification and API Categorisation of Windows Portable Executable Files Using Machine Learning. Appl. Sci. 2024 , 14 , 1015. [ Google Scholar ] [ CrossRef ]
- He, X.; Zhao, K.; Chu, X. AutoML: A survey of the state-of-the-art. Knowl.-Based Syst. 2021 , 212 , 106622. [ Google Scholar ] [ CrossRef ]
- Brown, A.; Gupta, M.; Abdelsalam, M. Automated machine learning for deep learning based malware detection. Comput. Secur. 2024 , 137 , 103582. [ Google Scholar ] [ CrossRef ]
- Qian, L.; Cong, L. Channel Features and API Frequency-Based Transformer Model for Malware Identification. Sensors 2024 , 24 , 580. [ Google Scholar ] [ CrossRef ]
- Yunan, Z.; Huang, Q.; Ma, X.; Yang, Z.; Jiang, J. Using Multi-features and Ensemble Learning Method for Imbalanced Malware Classification. In Proceedings of the 2016 IEEE Trustcom/BigDataSE/ISPA, Tianjin, China, 23–26 August 2016; pp. 965–973. [ Google Scholar ] [ CrossRef ]
- Akarsh, S.; Simran, K.; Poornachandran, P.; Menon, V.K.; Soman, K. Deep Learning Framework and Visualization for Malware Classification. In Proceedings of the 2019 5th International Conference on Advanced Computing & Communication Systems (ICACCS), Coimbatore, India, 15–16 March 2019; pp. 1059–1063. [ Google Scholar ] [ CrossRef ]
- Catak, F.O.; Ahmed, J.; Sahinbas, K.; Khand, Z.H. Data augmentation based malware detection using convolutional neural networks. PeerJ Comput. Sci. 2021 , 7 , e346. [ Google Scholar ] [ CrossRef ]
- Liu, J.; Zhuge, C.; Wang, Q.; Guo, X.; Li, Z. Imbalance Malware Classification by Decoupling Representation and Classifier. In Proceedings of the Advances in Artificial Intelligence and Security ; Sun, X., Zhang, X., Xia, Z., Bertino, E., Eds.; Springer: Cham, Switzerland, 2021; pp. 85–98. [ Google Scholar ] [ CrossRef ]
- Bacevicius, M.; Paulauskaite-Taraseviciene, A. Machine Learning Algorithms for Raw and Unbalanced Intrusion Detection Data in a Multi-Class Classification Problem. Appl. Sci. 2023 , 13 , 7328. [ Google Scholar ] [ CrossRef ]
- Li, T.; Luo, Y.; Wan, X.; Li, Q.; Liu, Q.; Wang, R.; Jia, C.; Xiao, Y. A malware detection model based on imbalanced heterogeneous graph embeddings. Expert Syst. Appl. 2024 , 246 , 123109. [ Google Scholar ] [ CrossRef ]
- Xue, L.; Zhu, T. Hybrid resampling and weighted majority voting for multi-class anomaly detection on imbalanced malware and network traffic data. Eng. Appl. Artif. Intell. 2024 , 128 , 107568. [ Google Scholar ] [ CrossRef ]
- Fang, Z.; Wang, J.; Geng, J.; Kan, X. Feature Selection for Malware Detection Based on Reinforcement Learning. IEEE Access 2019 , 7 , 176177–176187. [ Google Scholar ] [ CrossRef ]
- Wu, Y.; Li, M.; Zeng, Q.; Yang, T.; Wang, J.; Fang, Z.; Cheng, L. DroidRL: Feature selection for android malware detection with reinforcement learning. Comput. Secur. 2023 , 128 , 103126. [ Google Scholar ] [ CrossRef ]
- Wang, Y.; Stokes, J.W.; Marinescu, M. Neural Malware Control with Deep Reinforcement Learning. In Proceedings of the MILCOM 2019 - 2019 IEEE Military Communications Conference (MILCOM), Norfolk, VA, USA, 12–14 November 2019; pp. 1–8. [ Google Scholar ] [ CrossRef ]
- Fang, Z.; Wang, J.; Li, B.; Wu, S.; Zhou, Y.; Huang, H. Evading Anti-Malware Engines with Deep Reinforcement Learning. IEEE Access 2019 , 7 , 48867–48879. [ Google Scholar ] [ CrossRef ]
- Wang, Y.; Stokes, J.; Marinescu, M. Actor Critic Deep Reinforcement Learning for Neural Malware Control. In Proceedings of the AAAI Conference on Artificial Intelligence. Association for the Advancement of Artificial Intelligence (AAAI), 2020, Hilton New York Midtown, New York, NY, USA, 7–12 February 2020; Volume 34, pp. 1005–1012. [ Google Scholar ] [ CrossRef ]
- Song, W.; Li, X.; Afroz, S.; Garg, D.; Kuznetsov, D.; Yin, H. MAB-Malware: A Reinforcement Learning Framework for Blackbox Generation of Adversarial Malware. In Proceedings of the 2022 ACM on Asia Conference on Computer and Communications Security. Association for Computing Machinery, Nagasaki, Japan, 30 May–3 June 2022; pp. 990–1003. [ Google Scholar ] [ CrossRef ]
- Anderson, H.S.; Kharkar, A.; Filar, B.; Evans, D.; Roth, P. Learning to Evade Static PE Machine Learning Malware Models via Reinforcement Learning. arXiv 2018 , arXiv:1801.08917. [ Google Scholar ] [ CrossRef ]
- Deng, X.; Cen, M.; Jiang, M.; Lu, M. Ransomware early detection using deep reinforcement learning on portable executable header. Cluster Computing 2023 , 27 , 1867–1881. [ Google Scholar ] [ CrossRef ]
- Birman, Y.; Hindi, S.; Katz, G.; Shabtai, A. Cost-effective ensemble models selection using deep reinforcement learning. Inf. Fusion 2022 , 77 , 133–148. [ Google Scholar ] [ CrossRef ]
- Atti, M.; Yogi, M.K. Application of Deep Reinforcement Learning (DRL) for Malware Detection. Int. J. Inf. Technol. Comput. Eng. (IJITC) 2024 , 4 , 23–35. [ Google Scholar ] [ CrossRef ]
- Al-Fawa’reh, M.; Abu-Khalaf, J.; Szewczyk, P.; Kang, J.J. MalBoT-DRL: Malware Botnet Detection Using Deep Reinforcement Learning in IoT Networks. IEEE Internet Things J. 2024 , 11 , 9610–9629. [ Google Scholar ] [ CrossRef ]
- Hochreiter, S.; Schmidhuber, J. Long Short-Term Memory. Neural Comput. 1997 , 9 , 1735–1780. [ Google Scholar ] [ CrossRef ] [ PubMed ]
- Graves, A.; Schmidhuber, J. Framewise phoneme classification with bidirectional LSTM networks. In Proceedings of the 2005 IEEE International Joint Conference on Neural Networks, Montreal, QC, Canada, 31 July–4 August 2005; Volume 4, pp. 2047–2052. [ Google Scholar ] [ CrossRef ]
- Cho, K.; van Merrienboer, B.; Gulcehre, C.; Bahdanau, D.; Bougares, F.; Schwenk, H.; Bengio, Y. Learning Phrase Representations using RNN Encoder-Decoder for Statistical Machine Translation. arXiv 2014 , arXiv:1406.1078. [ Google Scholar ] [ CrossRef ]
- Arik, S.Ö.; Pfister, T. TabNet: Attentive Interpretable Tabular Learning. In Proceedings of the AAAI Conference on Artificial Intelligence, 2021, Virtual conference, 2–9 February 2021; Volume 35, pp. 6679–6687. [ Google Scholar ] [ CrossRef ]
- Düzgün, B.; Cayir, A.; Demirkiran, F.; Kahya, C.; Gençaydın, B.; Dag, H. New Datasets for Dynamic Malware Classification. 2021. Available online: https://www.researchgate.net/publication/356664607_New_Datasets_for_Dynamic_Malware_Classification (accessed on 16 May 2024).
- De Oliveira, A.S.; Sassi, R.J. Behavioral Malware Detection Using Deep Graph Convolutional Neural Networks. TechRxiv 2019 . [ Google Scholar ] [ CrossRef ]
- Do, N.Q.; Selamat, A.; Krejcar, O.; Herrera-Viedma, E.; Fujita, H. Deep Learning for Phishing Detection: Taxonomy, Current Challenges and Future Directions. IEEE Access 2022 , 10 , 36429–36463. [ Google Scholar ] [ CrossRef ]
- Grandini, M.; Bagli, E.; Visani, G. Metrics for Multi-Class Classification: An Overview. arXiv 2020 , arXiv:2008.05756. [ Google Scholar ] [ CrossRef ]
- Harris, C.R.; Millman, K.J.; van der Walt, S.J.; Gommers, R.; Virtanen, P.; Cournapeau, D.; Wieser, E.; Taylor, J.; Berg, S.; Smith, N.J.; et al. Array programming with NumPy. Nature 2020 , 585 , 357–362. [ Google Scholar ] [ CrossRef ]
- McKinney, W. Data Structures for Statistical Computing in Python. In Proceedings of the 9th Python in Science Conference, Austin, Texas, USA, 28 June–3 July 2010; van der Walt, S., Millman, J., Eds.; pp. 56–61. [ Google Scholar ] [ CrossRef ]
- Abadi, M.; Agarwal, A.; Barham, P.; Brevdo, E.; Chen, Z.; Citro, C.; Corrado, G.S.; Davis, A.; Dean, J.; Devin, M.; et al. TensorFlow: Large-Scale Machine Learning on Heterogeneous Systems. 2015. Available online: https://www.tensorflow.org (accessed on 5 February 2024).
Malware | m | |||||
---|---|---|---|---|---|---|
Family | US-1 | US-2 | US-3 | US-1 | US-2 | US-3 |
Adware | 303 | 36 | ← | 0.689 | 0.987 | 0.942 |
Backdoor | 800 | ← | ← | 0.261 | 0.044 | 0.042 |
Downloader | 800 | ← | ← | ↑ | ↑ | ↑ |
Dropper | 713 | 446 | 179 | 0.292 | 0.079 | 0.189 |
Spyware | 665 | 398 | 131 | 0.314 | 0.089 | 0.258 |
Trojan | 800 | ← | ← | 0.261 | 0.044 | 0.042 |
Virus | 800 | ← | ← | ↑ | ↑ | ↑ |
Worms | 800 | ← | ← | ↑ | ↑ | ↑ |
Paper | Equation | Implementation Details and Description |
---|---|---|
A. Alzammam et al. [ ] | The computed class weight is passed as a parameter to the learning function of the model. Because of such a formulation, the lower , the greater . | |
S. Akarsh et al. [ ] | The developed custom categorical cross-entropy loss consists of the product between the traditional loss and the left-side equation. Note that . does not consider class skew, while , which decreases with the increase in . In our setting, . |
Dataset | Technique | |||
---|---|---|---|---|
DQN | DDQN | Dueling | PER | |
Catak | ||||
VirusSample | ||||
Oliveira | ||||
VirusShare |
Algorithm | Cost-Sensitive Strategy | Training Time | Inference Time | F1-Score | AUC |
---|---|---|---|---|---|
LSTM | Alzammam et al. [ ] | 355.892 | 2.319 | 0.009 | 0.500 |
BiLSTM | 11,166.234 | 29.632 | 0.519 | 0.857 | |
BiGRU | 8840.091 | 26.029 | 0.015 | 0.500 | |
TabNet | 1283.507 | 5.157 | 0.141 | 0.575 | |
LSTM | Akarsh et al. [ ] | 296.317 | 2.338 | 0.098 | 0.500 |
BiLSTM | 11,122.994 | 29.627 | 0.676 | 0.795 | |
BiGRU | 8745.446 | 26.149 | 0.015 | 0.500 | |
TabNet | 1208.977 | 6.005 | 0.099 | 0.498 | |
RTF | None | 682.800 | 2.755 | 0.727 | 0.951 |
SINNER | Equation ( ) | 5087.307 | 0.044 | 0.744 | 0.832 |
Algorithm | Cost-Sensitive Strategy | Training Time | Inference Time | F1-Score | AUC |
---|---|---|---|---|---|
LSTM | Alzammam et al. [ ] | 188.601 | 1.202 | 0.014 | 0.500 |
BiLSTM | 1417.669 | 3.997 | 0.661 | 0.917 | |
BiGRU | 1132.346 | 3.564 | 0.659 | 0.918 | |
TabNet | 899.666 | 4.437 | 0.230 | 0.604 | |
LSTM | Akarsh et al. [ ] | 143.860 | 1.215 | 0.129 | 0.500 |
BiLSTM | 1383.538 | 3.980 | 0.753 | 0.849 | |
BiGRU | 1112.441 | 3.529 | 0.741 | 0.852 | |
TabNet | 944.372 | 4.175 | 0.129 | 0.499 | |
RTF | None | 481.300 | 4.952 | 0.806 | 0.977 |
SINNER | Equation ( ) | 3605.696 | 0.021 | 0.791 | 0.864 |
Algorithm | Cost-Sensitive Strategy | Training Time | Inference Time | F1-Score | AUC |
---|---|---|---|---|---|
LSTM | Alzammam et al. [ ] | 92.839 | 0.798 | 0.186 | 0.556 |
BiLSTM | 362.934 | 1.764 | 0.541 | 0.737 | |
BiGRU | 345.497 | 1.703 | 0.537 | 0.736 | |
TabNet | 662.308 | 2.877 | 0.101 | 0.498 | |
LSTM | Akarsh et al. [ ] | 91.102 | 0.798 | 0.178 | 0.547 |
BiLSTM | 352.377 | 1.785 | 0.515 | 0.721 | |
BiGRU | 336.035 | 1.711 | 0.537 | 0.736 | |
TabNet | 658.649 | 2.856 | 0.091 | 0.497 | |
RTF | None | 1626.000 | 4.952 | 0.615 | 0.882 |
SINNER | Equation ( ) | 1262.938 | 0.014 | 0.427 | 0.668 |
Algorithm | Cost-Sensitive Strategy | Training Time | Inference Time | F1-Score | AUC |
---|---|---|---|---|---|
LSTM | Alzammam et al. [ ] | 380.438 | 2.764 | 0.341 | 0.780 |
BiLSTM | 1217.654 | 4.006 | 0.400 | 0.782 | |
BiGRU | 1051.595 | 4.411 | 0.410 | 0.790 | |
TabNet | 3572.845 | 15.849 | 0.047 | 0.532 | |
LSTM | Akarsh et al. [ ] | 373.986 | 2.656 | 0.561 | 0.735 |
BiLSTM | 1166.489 | 3.826 | 0.568 | 0.734 | |
BiGRU | 1023.659 | 3.601 | 0.569 | 0.731 | |
TabNet | 3428.129 | 17.121 | 0.137 | 0.516 | |
RTF | None | 8711.400 | 4.531 | 0.565 | 0.885 |
SINNER | Equation ( ) | 976.798 | 0.015 | 0.563 | 0.725 |
Imbalanced Malware Classifier | Positive | Negative |
---|---|---|
LSTM | Combined with an appropriate loss function balancing strategy, the model performs adequately with a strong time dependence among the data, i.e., a dynamic analysis-based dataset. In addition, the learning and inference times are very competitive with respect to the same measures achieved by the alternative DL solutions employed in the experiment. | Poor classification performance in 87.5% of experiments involving this algorithm. |
BiLSTM/BiGRU | The bidirectional models can benefit from the cost-sensitive strategy proposed by Akarsh et al. | Poor timing performance, and using three of the evaluated datasets, the classification scores are far from those achieved by competitors. |
TabNet | In most cases, it appears suitable in terms of training time (in 2 out of 4 tests) compared to other solutions. | This algorithm, combined with the two evaluated cost-senstive strategies, was the worst classifier in all experiments. |
RTF | State-of-the-art classification performance on three out of four datasets. | This model performs an ensemble of transformers; therefore, the high number of parameters required by the involved DL architectures can make it impracticable in several application scenarios. In addition, it achieves state-of-the-art classification performance via dataset-specific hyperparameter tuning. |
Using the same hyperparameters for each tested dataset, the proposed solution targets the RTF in terms of F1 and outperforms the top competitor using VirusShare. It achieves promising results on both static and dynamic analysis datasets. In addition, it is the fastest algorithm among the considered list of competitors in providing the prediction. | Longer training time in 50% of tests compared to RTF. Lower AUC score than that achieved by RTF. |
The statements, opinions and data contained in all publications are solely those of the individual author(s) and contributor(s) and not of MDPI and/or the editor(s). MDPI and/or the editor(s) disclaim responsibility for any injury to people or property resulting from any ideas, methods, instructions or products referred to in the content. |
Share and Cite
Coscia, A.; Iannacone, A.; Maci, A.; Stamerra, A. SINNER: A Reward-Sensitive Algorithm for Imbalanced Malware Classification Using Neural Networks with Experience Replay. Information 2024 , 15 , 425. https://doi.org/10.3390/info15080425
Coscia A, Iannacone A, Maci A, Stamerra A. SINNER: A Reward-Sensitive Algorithm for Imbalanced Malware Classification Using Neural Networks with Experience Replay. Information . 2024; 15(8):425. https://doi.org/10.3390/info15080425
Coscia, Antonio, Andrea Iannacone, Antonio Maci, and Alessandro Stamerra. 2024. "SINNER: A Reward-Sensitive Algorithm for Imbalanced Malware Classification Using Neural Networks with Experience Replay" Information 15, no. 8: 425. https://doi.org/10.3390/info15080425
Article Metrics
Further information, mdpi initiatives, follow mdpi.
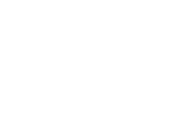
Subscribe to receive issue release notifications and newsletters from MDPI journals
Machine and deep learning techniques for the prediction of diabetics: a review
- Published: 16 July 2024
Cite this article
- Sandip Kumar Singh Modak ORCID: orcid.org/0000-0001-7985-4161 1 &
- Vijay Kumar Jha 2
33 Accesses
Explore all metrics
Diabetes has become one of the significant reasons for public sickness and death in worldwide. By 2019, diabetes had affected more than 463 million people worldwide. According to the International Diabetes Federation report, this figure is expected to rise to more than 700 million in 2040, so early screening and diagnosis of diabetes patients have great significance in detecting and treating diabetes on time. Diabetes is a multi factorial metabolic disease, its diagnostic criteria are difficult to cover all the ethology, damage degree, pathogenesis and other factors, so there is a situation for uncertainty and imprecision under various aspects of the medical diagnosis process. With the development of Data mining, researchers find that machine learning and deep learning, playing an important role in diabetes prediction research. This paper is an in-depth study on the application of machine learning and deep learning techniques in the prediction of diabetics. In addition, this paper also discusses the different methodology used in machine and deep learning for prediction of diabetics since last two decades and examines the methods used, to explore their successes and failure. This review would help researchers and practitioners understand the current state-of-the-art methods and identify gaps in the literature.
This is a preview of subscription content, log in via an institution to check access.
Access this article
Subscribe and save.
- Get 10 units per month
- Download Article/Chapter or Ebook
- 1 Unit = 1 Article or 1 Chapter
- Cancel anytime
Price includes VAT (Russian Federation)
Instant access to the full article PDF.
Rent this article via DeepDyve
Institutional subscriptions
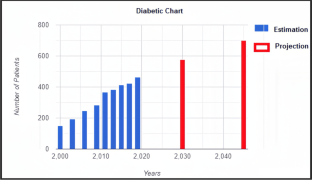
Similar content being viewed by others
A Review for Predicting the Diabetes Mellitus Using Different Techniques and Methods
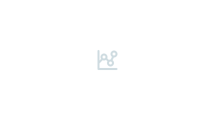
Recent applications of machine learning and deep learning models in the prediction, diagnosis, and management of diabetes: a comprehensive review
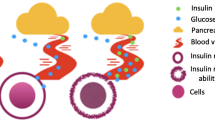
Diabetes prediction model based on an enhanced deep neural network
Data availability.
Data sharing not applicable to this article as no datasets were generated or analyzed during the current study.
Bloomgarden Z (2016) Questioning glucose measurements used in the International Diabetes Federation (IDF) Atlas. J Diab 8(6):746–747. https://doi.org/10.1111/1753-0407.12453
Article Google Scholar
Ming Z, Wang X, Zhu X (2014) Understanding diabetes from the diagnosis of diabetes mellitus. J Diagn Concept Pract 2:226–228
Google Scholar
Rajesh K, Sangeetha V (2012) Application of Data Mining Methods and Techniques for Diabetes Diagnosis. Int J Eng Innov Technol 2(3):224–229
Kaveeshwar SA, Cornwall J (2014) The current state of diabetes mellitus in India. Australas Med J 7(1):45
Wild S, Roglic G, Green A, Sicree R, King H (2004) Global prevalence of diabetes: estimates for the year 2000 and projections for 2030. Diabetes Care 27 (5):1047–1053
Shaw JE, Simpson RW (2009) Prevention of type 2 diabetes. Diabetes and Exercise. Springer, pp 55–62
IDF Diabetes Atlas - 8th Edition. Available from: http://www.diabetesatlas.org/across-the-globe.html . Accessed 31 Dec. 2017
Kaur P, Sharma M (2018) Analysis of data mining and soft computing techniques in prospecting diabetes disorder in human beings: a review. Int J Pharm Sci Res 9:2700–2719
Sun YL, Zhang DL (2019) Machine learning techniques for screening and diagnosis of diabetes: a survey. Tehnički Vjesnik 26(3):872–880
Yoo I, Alafaireet P, Marinov M, Pena-Hernandez K, Gopidi R, Chang JF, Hua L (2012) Data mining in healthcare and biomedicine: a survey of the literature. J Med Syst 36(4):2431–2448
Fatima M, Pasha M (2017) Survey of machine learning algorithms for disease diagnostic. J Intell Learn Syst Appl 9(01):1
Kaur H, Kumari V (2020) Predictive modelling and analytics for diabetes using machine learning approach. Appl Comput Inform 18(1/2):90–100
Javitt JC, Aiello LP, Chiang Y, Ferris FL, Canner JK, Greenfield S (1994) Preventive eye care in people with diabetes is cost-saving to the federal government: implications for health-care reform. Diabetes Care 17(8):909–917
Mendonca AM, Campilho AJ, Nunes JM (1999) Automatic segmentation of microaneurysms in retinal angiograms of diabetic patients. In Proceedings 10th International Conference on Image Analysis and Processing. IEEE. pp 728-733
Cree MJ, Olson JA, McHardy KC, Forrester JV, Sharp PF (1996) Automated microaneurysm detection. In Proceedings of 3rd IEEE International Conference on Image Processing. vol. 3. IEEE. pp 699-702
Zhang X, Chutatape O (2005) A SVM approach for detection of hemorrhages in background diabetic retinopathy. In Proceedings. 2005 IEEE International Joint Conference on Neural Networks, 2005. vol. 4. IEEE. pp 2435-2440
Stoean R, Stoean C, Preuss M, El-Darzi E, Dumitrescu D (2006) Evolutionary support vector machines for diabetes mellitus diagnosis. In 2006 3rd International IEEE Conference Intelligent Systems. IEEE. pp 182-187
Balakrishnan S, Narayanaswamy R, Savarimuthu N, Samikannu R (2008) SVM ranking with backward search for feature selection in type II diabetes databases. In 2008 IEEE International Conference on Systems, Man and Cybernetics. IEEE. pp 2628-2633
Polat K, Güneş S, Arslan A (2008) A cascade learning system for classification of diabetes disease: Generalized discriminant analysis and least square support vector machine. Expert Syst Appl 34(1):482–487
Wu J, Diao YB, Li ML, Fang YP, Ma DC (2009) A semi-supervised learning based method: Laplacian support vector machine used in diabetes disease diagnosis. Interdiscip Sci: Comput Life Sci 1(2):151–155
Yu W, Liu T, Valdez R, Gwinn M, Khoury MJ (2010) Application of support vector machine modeling for prediction of common diseases: the case of diabetes and pre-diabetes. BMC Med Inform Decis Mak 10(1):1–7
Barakat N, Bradley AP, Barakat MNH (2010) Intelligible support vector machines for diagnosis of diabetes mellitus. IEEE Trans Inf Technol Biomed 14(4):1114–1120
Çalişir D, Doğantekin E (2011) An automatic diabetes diagnosis system based on LDA-Wavelet Support Vector Machine Classifier. Expert Syst Appl 38(7):8311–8315
Gupta S, Kumar D, Sharma A (2011) Performance analysis of various data mining classification techniques on healthcare data. Int J Comput Sci Inform Technol 3(4):155–169
Marling C, Wiley M, Cooper T, Bunescu R, Shubrook J, Schwartz F (2011) The 4 diabetes support system: a case study in CBR research and development. In International Conference on Case-Based Reasoning. Springer, Berlin, Heidelberg. pp 137-150
Zolfaghari R (2012) Diagnosis of diabetes in female population of pima indian heritage with ensemble of bp neural network and svm. Int J Comput Eng Manag 15:2230–7893
Giveki D, Salimi H, Bahmanyar G, Khademian Y (2012) Automatic detection of diabetes diagnosis using feature weighted support vector machines based on mutual information and modified cuckoo search. arXiv preprint arXiv:1201.2173
Hashim MF, Hashim SZM (2012) Comparison of clinical and textural approach for Diabetic Retinopathy grading. In 2012 IEEE International Conference on Control System, Computing and Engineering. IEEE. pp 290-295
Karatsiolis S, Schizas CN (2012) Region based Support Vector Machine algorithm for medical diagnosis on Pima Indian Diabetes dataset. In 2012 IEEE 12th International Conference on Bioinformatics & Bioengineering (BIBE). IEEE. pp 139-144
Kumari VA, Chitra R (2013) Classification of diabetes disease using support vector machine. Int J Eng Res Appl 3(2):1797–1801
Farran B, Channanath AM, Behbehani K, Thanaraj TA (2013) Predictive models to assess risk of type 2 diabetes, hypertension and comorbidity: machine-learning algorithms and validation using national health data from Kuwait—a cohort study. BMJ Open 3(5):e002457
Mansour RF, Abdelrahim EM and Al-Johani AS (2013) Identification of diabetic retinal exudates in digital color images using support vector machine
Book Google Scholar
Tapak L, Mahjub H, Hamidi O, Poorolajal J (2013) Real-data comparison of data mining methods in prediction of diabetes in Iran. Healthc Inform Res 19(3):177
Anthimopoulos MM, Gianola L, Scarnato L, Diem P, Mougiakakou SG (2014) A food recognition system for diabetic patients based on an optimized bag-of-features model. IEEE J Biomed Health Inform 18(4):1261–1271
Choi SB, Kim WJ, Yoo TK, Park JS, Chung JW, Lee YH, Kang EU, Kim DW (2014) Screening for prediabetes using machine learning models. Comput Math Methods Med 2014(1):618976
Roychowdhury S, Koozekanani DD, Parhi KK (2014) DREAM: diabetic retinopathy analysis using machine learning. IEEE J Biomed Health Inform 18(5):1717–1728
Cai L, Wu H, Li D, Zhou K, Zou F (2015) Type 2 diabetes biomarkers of human gut microbiota selected via iterative sure independent screening method. PLoS One 10(10):e0140827
Jaya T, Dheeba J, Singh NA (2015) Detection of hard exudates in colour fundus images using fuzzy support vector machine-based expert system. J Digit Imaging 28(6):761–768
Arjun C, Anto M (2015) Diagnosis of diabetes using support vector machine and ensemble learning approach. Int J Eng Appl Sci 2(11):257790
Kang S, Kang P, Ko T, Cho S, Rhee SJ, Yu KS (2015) An efficient and effective ensemble of support vector machines for anti-diabetic drug failure prediction. Expert Syst Appl 42(9):4265–4273
Ramanathan TT, Sharma D (2015) An SVM-Fuzzy Expert System design for diabetes risk classification. Int J Comput Sci Inform Technol 6(3):2221–2226
Santhanam T, Padmavathi MS (2015) Application of K-means and genetic algorithms for dimension reduction by integrating SVM for diabetes diagnosis. Procedia Comput Sci 47:76–83
Sowjanya K, Singhal A, Choudhary C (2015) MobDBTest: A machine learning based system for predicting diabetes risk using mobile devices. In 2015 IEEE International Advance Computing Conference (IACC). IEEE. pp 397-402
Tafa Z, Pervetica N, Karahoda B (2015) An intelligent system for diabetes prediction. In 2015 4th Mediterranean Conference on Embedded Computing (MECO). IEEE. pp 378-382
Abdillah AA, Suwarno S (2016) Diagnosis of diabetes using support vector machines with radial basis function kernels. Int J Technol 7(5)
Bano S, Khan MNA (2016) A Framework to Improve Diabetes Prediction using k-NN and SVM. Int J Comput Sci Inform Sec 14(11):450
Gill NS, Mittal P (2016) A computational hybrid model with two level classification using SVM and neural network for predicting the diabetes disease. J Theor Appl Inf Technol 87(1):1–10
Huang YP, Nashrullah M (2016) SVM-based Decision Tree for medical knowledge representation. In 2016 International Conference on Fuzzy Theory and Its Applications (iFuzzy). IEEE. pp 1-6
Kose U, Guraksin GE, Deperlioglu O (2016) Cognitive development optimization algorithm based support vector machines for determining diabetes. Broad Res Artif Intell Neurosci 7(1):80–90
Malik S, Khadgawat R, Anand S, Gupta S (2016) Non-invasive detection of fasting blood glucose level via electrochemical measurement of saliva. Springerplus 5(1):1–12
Negi A, Jaiswal V (2016) A first attempt to develop a diabetes prediction method based on different global datasets. In 2016 Fourth International Conference on Parallel, Distributed and Grid Computing (PDGC). IEEE. pp 237-241
Osman AH, Aljahdali HM (2017) Diabetes disease diagnosis method based on feature extraction using K-SVM. Int J Adv Comput Sci Appl 8(1)
Carrera EV, González A, Carrera R (2017) Automated detection of diabetic retinopathy using SVM. In 2017 IEEE XXIV international conference on electronics, electrical engineering and computing (INTERCON). IEEE. pp 1-4
Khalil RM, Al-Jumaily A (2017) Machine learning based prediction of depression among type 2 diabetic patients. In 2017 12th international conference on intelligent systems and knowledge engineering (ISKE). IEEE. pp 1-5
Rathore A, Chauhan S, Gujral S (2017) Detecting and predicting diabetes using supervised learning: an approach towards better healthcare for women. Int J Adv Res Comput Sci 8(5)
Wang Y, Liu ZP (2017) Identifying biomarkers of diabetes with gene co expression networks. In 2017 Chinese Automation Congress (CAC). IEEE. pp 5283-5286
Zhang J, Xu J, Hu X, Chen Q, Tu L, Huang J, Cui J (2017) Diagnostic method of diabetes based on support vector machine and tongue images. BioMed Res Int 2017(1):7961494
Cui S, Wang D, Wang Y, Yu PW, Jin Y (2018) An improved support vector machine-based diabetic readmission prediction. Comput Methods Prog Biomed 166:123–135
Dagliati A, Marini S, Sacchi L, Cogni G, Teliti M, Tibollo V, … Bellazzi R (2018) Machine learning methods to predict diabetes complications. J Diabetes Sci Technol 12(2):295–302
Joshi TN, Chawan PM (2018) Logistic regression and svm based diabetes prediction system. Int J Technol Res Eng 5:4347–4350
Rao NM, Kannan K, Gao XZ, Roy DS (2018) Novel classifiers for intelligent disease diagnosis with multi-objective parameter evolution. Comput Electr Eng 67:483–496
Mule DB, Chowhan SS, Somwanshi DR (2018) Detection and classfication of non-proliferative diabetic retinopathy using retinal images. In International Conference on Recent Trends in Image Processing and Pattern Recognition. Springer, Singapore. pp 312-320
Abdullah AS, Gayathri N, Selvakumar S, Kumar SR (2018) Identification of the Risk Factors of Type II Diabetic Data Based Support Vector Machine Classifiers upon Varied Kernel Functions. In Computational Vision and Bio Inspired Computing. Springer, Cham. pp 496-505
Sisodia D, Sisodia DS (2018) Prediction of diabetes using classification algorithms. Procedia Comput Sci 132:1578–1585
Tsao HY, Chan PY, Su ECY (2018) Predicting diabetic retinopathy and identifying interpretable biomedical features using machine learning algorithms. BMC Bioinform 19(9):111–121
Alirezaei M, Niaki STA, Niaki SAA (2019) A bi-objective hybrid optimization algorithm to reduce noise and data dimension in diabetes diagnosis using support vector machines. Expert Syst Appl 127:47–57
Bernardini M, Romeo L, Misericordia P, Frontoni E (2019) Discovering the type 2 diabetes in electronic health records using the sparse balanced support vector machine. IEEE J Biomed Health Inform 24(1):235–246
Raj RS, Sanjay DS, Kusuma M, Sampath S (2019) Comparison of support vector machine and Naive Bayes classifiers for predicting diabetes. In 2019 1st International Conference on Advanced Technologies in Intelligent Control, Environment, Computing & Communication Engineering (ICATIECE). IEEE. pp. 41-45
He K, Huang S, Qian X (2019) Early detection and risk assessment for chronic disease with irregular longitudinal data analysis. J Biomed Inform 96:103231
Karkuzhali S, Manimegalai D (2019) Distinguising Proof of Diabetic Retinopathy Detection by Hybrid Approaches in Two Dimensional Retinal Fundus Images. J Med Syst 43(6):1–12
Abbas HT, Alic L, Erraguntla M, Ji JX, Abdul-Ghani M, Abbasi QH, Qaraqe MK (2019) Predicting long-term type 2 diabetes with support vector machine using oral glucose tolerance test. PLoS One 14(12):e0219636
Lokuarachchi D, Muthumal L, Gunarathna K, Gamage TD (2019) Detection of red lesions in retinal images using image processing and machine learning techniques. In 2019 Moratuwa Engineering Research Conference (MERCon). IEEE. pp 550-555
Aminah R, Saputro AH (2019) Application of machine learning techniques for diagnosis of diabetes based on iridology. In 2019 International Conference on Advanced Computer Science and information Systems (ICACSIS). IEEE. pp 133-138
Qomariah DUN, Tjandrasa H, Fatichah C (2019) Classification of diabetic retinopathy and normal retinal images using CNN and SVM. In 2019 12th International Conference on Information & Communication Technology and System (ICTS). IEEE. pp 152-157
Selvathi D, Suganya K (2019) Support vector machine based method for automatic detection of diabetic eye disease using thermal images. In 2019 1st International Conference on Innovations in Information and Communication Technology (ICIICT). IEEE. pp 1-6
Sneha N, Gangil T (2019) Analysis of diabetes mellitus for early prediction using optimal features selection. J Big Data 6(1):1–19
Hao Y, Cheng F, Pham M, Rein H, Patel D, Fang Y, … Wang Y (2019) A Noninvasive, Economical, and Instant-Result Method to Diagnose and Monitor Type 2 Diabetes Using Pulse Wave: Case-Control Study. JMIR MHealth UHealth 7(4):e11959
Azad C, Mehta AK, Mahto D, Yadav DK (2020) Support Vector Machine based eHealth Cloud System for Diabetes Classification. EAI Endorsed Trans Pervasive Health Technol 6(22):e3
Harimoorthy K, Thangavelu M (2020) Multi-disease prediction model using improved SVM-radial bias technique in healthcare monitoring system. J Ambient Intell Humaniz Comput 12(3):3715–3723
Jayabalan S, Pratheeksha PS, Bolar NS, Malavika NL (2020) Prediction of diabetic retinopathy using svm algorithm. J Crit Rev 7(14):1702–1711
Kazerouni F, Bayani A, Asadi F, Saeidi L, Parvizi N, Mansoori Z (2020) Type2 diabetes mellitus prediction using data mining algorithms based on the long-noncoding RNAs expression: a comparison of four data mining approaches. BMC Bioinformatics 21(1):1–13
Nnamoko N, Korkontzelos I (2020) Efficient treatment of outliers and class imbalance for diabetes prediction. Artif Intell Med 104:101815
Shuja M, Mittal S, Zaman M (2020) Effective prediction of type ii diabetes mellitus using data mining classifiers and SMOTE. In Advances in computing and intelligent systems. Springer, Singapore. pp 195-211
Mishra SK, Tiwari AK (2020) An Ensemble Approach for the Prediction of Diabetes. SAMRIDDHI 12(02):122–129
Viloria A, Herazo-Beltran Y, Cabrera D, Pineda OB (2020) Diabetes diagnostic prediction using vector support machines. Procedia Comput Sci 170:376–381
Wang X, Yang Y, Xu Y, Chen Q, Wang H, Gao H (2020) Predicting hypoglycemic drugs of type 2 diabetes based on weighted rank support vector machine. Knowl-Based Syst 197:105868
Srivastava AK, Kumar Y, Singh PK (2020) Computer aided diagnostic system based on SVM and K harmonic mean based attribute weighting method. Obes Med 19:100270
Xue J, Min F, Ma F (2020) Research on Diabetes Prediction Method Based on Machine Learning. In Journal of Physics: Conference Series. vol. 1684, no. 1. IOP Publishing. p 012062
Ahmad HF, Mukhtar H, Alaqail H, Seliaman M, Alhumam A (2021) Investigating Health-Related Features and Their Impact on the Prediction of Diabetes Using Machine Learning. Appl Sci 11(3):1173
Alabdulwahhab KM, Sami W, Mehmood T, Meo SA, Alasbali TA, Alwadani FA (2021) Automated detection of diabetic retinopathy using machine learning classifiers. Eur Rev Med Pharmacol Sci 25(2):583–590
Chaves L, Marques G (2021) Data Mining Techniques for Early Diagnosis of Diabetes: A Comparative Study. Appl Sci 11(5):2218
Dinesh MG, Prabha D (2021) Diabetes Mellitus Prediction System Using Hybrid KPCA-GA-SVM Feature Selection Techniques. J Phys Conf Ser. 1767(1):012001
Khanam JJ, Foo SY (2021) A comparison of machine learning algorithms for diabetes prediction. ICT Express
Reddy SS, Sethi N, Rajender R (2021) Discovering Optimal Algorithm to Predict Diabetic Retinopathy using Novel Assessment Methods. EAI Endorsed Trans Scalable Inf Syst 8(29):e1
Rodríguez-Rodríguez I, Rodríguez JV, Woo WL, Wei B, Pardo-Quiles DJ (2021) A Comparison of Feature Selection and Forecasting Machine Learning Algorithms for Predicting Glycaemia in Type 1 Diabetes Mellitus. Appl Sci 11(4):1742
Tang H, Zhang Y, Xiang B, Liu M, Hu J, Liu C (2021) Risk prediction of early diabetes mellitus based on combination model. In MATEC Web of Conferences. vol. 336, EDP Sciences. p 07018
Hossain ME, Uddin S, Khan A (2021) Network analytics and machine learning for predictive risk modelling of cardiovascular disease in patients with type 2 diabetes. Expert Syst Appl 164:113918
Senthil Velmurugan N, Viveka T (2021) Performance analysis of ML algorithms on diabetes data. Int Adv Res J Sci Eng Technol 8(2):72–79
Suresh K, Obulesu O, Ramudu BV (2020) Diabetes Prediction using Machine Learning Techniques. Helix 10(02):136–142
Brisimi TS, Xu T, Wang T, Dai W, Adams WG, Paschalidis IC (2018) Predicting chronic disease hospitalizations from electronic health records: an interpretable classification approach. Proc IEEE 106(4):690–707
Baitharu TR, Pani SK, Dhal S (2015) Comparison of Kernel selection for support vector machines using diabetes dataset. J Comput Sci Appl 3(6):181–184
Breault JL, Goodall CR, Fos PJ (2002) Data mining a diabetic data warehouse. Artif Intell Med 26(1-2):37–54
Miyaki K, Takei I, Watanabe K, Nakashima H, Watanabe K, Omae K (2002) Novel statistical classification model of type 2 diabetes mellitus patients for tailormade prevention using data mining algorithm. J Epidemiol 12(3):243–248
Duhamel A, Nuttens MC, Devos P, Picavet M, Beuscart R (2003) A preprocessing method for improving data mining techniques. Appl Large Med Diab Database Stud Health Technol Inform 95:269–274
Huang Y, McCullagh P, Black N and Harper R (2004) Evaluation of outcome prediction for a clinical diabetes database. In International Symposium on Knowledge Exploration in Life Science Informatics (pp. 181-190). Springer, Berlin, Heidelberg
Harper PR (2005) A review and comparison of classification algorithms for medical decision making. Health Policy 71(3):315–331
Sigurdardottir AK, Jonsdottir H, Benediktsson R (2007) Outcomes of educational interventions in type 2 diabetes: WEKA data-mining analysis. Patient Educ Couns 67(1-2):21–31
Huang Y, McCullagh P, Black N, Harper R (2007) Feature selection and classification model construction on type 2 diabetic patients’ data. Artif Intell Med 41(3):251–262
Liou FM, Tang YC, Chen JY (2008) Detecting hospital fraud and claim abuse through diabetic outpatient services. Health Care Manag Sci 11(4):353–358
Toussi M, Lamy JB, Le Toumelin P, Venot A (2009) Using data mining techniques to explore physicians' therapeutic decisions when clinical guidelines do not provide recommendations: methods and example for type 2 diabetes. BMC Med Inform Decis Mak 9(1):1–12
Hische M, Luis-Dominguez O, Pfeiffer AF, Schwarz PE, Selbig J, Spranger J (2010) Decision trees as a simple-to-use and reliable tool to identify individuals with impaired glucose metabolism or type 2 diabetes mellitus. Eur J Endocrinol 163(4):565
Patil BM, Joshi RC, Toshniwal D (2010) Hybrid prediction model for type-2 diabetic patients. Expert Syst Appl 37(12):8102–8108
Ahmad A, Mustapha A, Zahadi ED, Masah N, Yahaya NY (2011) Comparison between neural networks against decision tree in improving prediction accuracy for diabetes mellitus. In International conference on digital information processing and communications. Springer, Berlin, Heidelberg. pp 537-545
Al Jarullah AA (2011) Decision tree discovery for the diagnosis of type II diabetes. In 2011 International conference on innovations in information technology. IEEE 303-307
Karegowda AG, Manjunath AS, Jayaram MA (2011) Application of genetic algorithm optimized neural network connection weights for medical diagnosis of pima Indians diabetes. Int J Soft Comput 2(2):15–23
Kelarev AV, Stranieri A, Yearwood JL, Jelinek HF (2012) Empirical study of decision trees and ensemble classifiers for monitoring of diabetes patients in pervasive healthcare. In 2012 15th International Conference on Network-Based Information Systems. IEEE. pp 441-446
Hemant P, Pushpavathi T (2012) A novel approach to predict diabetes by Cascading Clustering and Classification. In 2012 Third International Conference on Computing, Communication and Networking Technologies (ICCCNT'12). IEEE. pp 1-7
Hussein AS, Omar WM, Li X, Ati M (2012) Efficient chronic disease diagnosis prediction and recommendation system. In 2012 IEEE-EMBS Conference on Biomedical Engineering and Sciences. IEEE. pp 209-214
Rajesh K, Sangeetha V (2012) Application of data mining methods and techniques for diabetes diagnosis. Int J Eng Innov Technol 2(3):224–229
Li CP, Zhi XY, Jun MA, Zhuang CUI, Zhu ZL, Zhang C, Hu LP (2012) Performance comparison between logistic regression, decision trees, and multilayer perceptron in predicting peripheral neuropathy in type 2 diabetes mellitus. Chin Med J 125(5):851–857
Chen H, Tan C (2012) Prediction of type-2 diabetes based on several element levels in blood and chemometrics. Biol Trace Elem Res 147(1):67–74
Karegowda AG, Punya V, Jayaram MA, Manjunath AS (2012) Rule based classification for diabetic patients using cascaded k-means and decision tree C4. 5. Int J Comput Appl 45(12):45–50
Karthikeyani V, Begum IP, Tajudin K, Begam IS (2012) Comparative of data mining classification algorithm (CDMCA) in diabetes disease prediction. Int J Comput Appl 60(12)
Ameri H, Alizadeh S, Barzegari A (2013) Knowledge extraction of diabetics' data by decision tree method. J Healthc Adm 16(53):58–72
Meng XH, Huang YX, Rao DP, Zhang Q, Liu Q (2013) Comparison of three data mining models for predicting diabetes or prediabetes by risk factors. Kaohsiung J Med Sci 29(2):93–99
Karthikeyani V, Begum IP (2013) Comparison a performance of data mining algorithms (CPDMA) in prediction of diabetes disease. Int J Comput Sci Eng 5(3):205
Rahman RM, Afroz F (2013) Comparison of various classification techniques using different data mining tools for diabetes diagnosis. J Softw Eng Appl 6(03):85
Varma KV, Rao AA, Lakshmi TSM, Rao PN (2014) A computational intelligence approach for a better diagnosis of diabetic patients. Comput Electr Eng 40(5):1758–1765
Kaur G, Chhabra A (2014) Improved J48 classification algorithm for the prediction of diabetes. Int J Comput Appl 98(22):13–17
Seera M, Lim CP (2014) A hybrid intelligent system for medical data classification. Expert Syst Appl 41(5):2239–2249
Uppin S, Anusuya MA (2014) Expert system design to predict heart and diabetes diseases. Int J Sci EngTechnol 3(8):1054–1059
Ramezankhani A, Pournik O, Shahrabi J, Khalili D, Azizi F, Hadaegh F (2014) Applying decision tree for identification of a low risk population for type 2 diabetes. Tehran Lipid and Glucose Study. Diabetes Res Clin Pract 105(3):391–398
Bashir S, Qamar U, Khan FH, Javed MY (2014) An efficient rule-based classification of Diabetes using ID3, C4. 5, & CART ensembles. In 2014 12th International Conference on Frontiers of Information Technology. IEEE. pp 226-231
Habibi S, Ahmadi M, Alizadeh S (2015) Type 2 diabetes mellitus screening and risk factors using decision tree: results of data mining. Global J Health Sci 7(5):304
Kandhasamy JP, Balamurali SJPCS (2015) Performance analysis of classifier models to predict diabetes mellitus. Procedia Comput Sci 47:45–51
Iyer A, Jeyalatha S, Sumbaly R (2015) Diagnosis of diabetes using classification mining techniques. arXiv preprint arXiv:1502.03774
Vijayan VV, Anjali C (2015) Prediction and diagnosis of diabetes mellitus—A machine learning approach. In 2015 IEEE Recent Advances in Intelligent Computational Systems (RAICS). IEEE. pp 122-127
Thirumal PC, Nagarajan N (2015) Utilization of data mining techniques for diagnosis of diabetes mellitus-a case study. ARPN J Eng Appl Sci 10(1):8–13
Nai-arun N, Moungmai R (2015) Comparison of classifiers for the risk of diabetes prediction. Procedia Comput Sci 69:132–142
Heydari M, Teimouri M, Heshmati Z, Alavinia SM (2016) Comparison of various classification algorithms in the diagnosis of type 2 diabetes in Iran. Int J Diabetes Dev Ctries 36(2):167–173
Ahmed TM (2016) Developing a predicted model for diabetes type 2 treatment plans by using data mining. J Theor Appl Inf Technol 90(2):181
Ahmed TM (2016) Using data mining to develop model for classifying diabetic patient control level based on historical medical records. J Theor Appl Inf Technol 87(2):316
Daghistani T, Alshammari R (2016) Diagnosis of diabetes by applying data mining classification techniques. Int J Adv Comput Sci Appl 7(7):329–332
Orabi KM, Kamal YM, Rabah TM (2016) Early predictive system for diabetes mellitus disease. In Industrial Conference on Data Mining. Springer, Cham. pp 420-427
Perveen S, Shahbaz M, Guergachi A, Keshavjee K (2016) Performance analysis of data mining classification techniques to predict diabetes. Procedia Comput Sci 82:115–121
Pradeep KR, Naveen NC (2016) Predictive analysis of diabetes using J48 algorithm of classification techniques. In 2016 2nd International Conference on Contemporary Computing and Informatics (IC3I). IEEE. pp 347-352
Shetty SP, Joshi S (2016) A tool for diabetes prediction and monitoring using data mining technique. Int J Inform TechnolComput Sci 8(11):26–32
Songthung P, Sripanidkulchai K (2016) Improving type 2 diabetes mellitus risk prediction using classification. In 2016 13th International Joint Conference on Computer Science and Software Engineering (JCSSE). IEEE. pp 1-6
Srikanth P, Deverapalli D (2016) A critical study of classification algorithms using diabetes diagnosis. In 2016 IEEE 6th International Conference on Advanced Computing (IACC). IEEE. pp 245-249
Teimouri M, Farzadfar F, Alamdari MS, Hashemi-Meshkini A, Alamdari PA, Rezaei-Darzi E, … Zeynalabedini A (2016) Detecting diseases in medical prescriptions using data mining tools and combining techniques. Iranian J Pharmaceut Res 15(Suppl):113
Chen W, Chen S, Zhang H, Wu T (2017) A hybrid prediction model for type 2 diabetes using K-means and decision tree. In 2017 8th IEEE International conference on software engineering and service science (ICSESS). IEEE. pp 386-390
Kasbekar PU, Goel P, Jadhav SP (2017) A decision tree analysis of diabetic foot amputation risk in indian patients. Front Endocrinol 8:25
Sayadi M, Zibaeenezhad M, Taghi Ayatollahi SM (2017) Simple prediction of type 2 diabetes mellitus via decision tree modeling. Int Cardiovasc Res J 11(2):71–76
Yuvaraj N, SriPreethaa KR (2019) Diabetes prediction in healthcare systems using machine learning algorithms on Hadoop cluster. Clust Comput 22(1):1–9
Zou Q, Qu K, Luo Y, Yin D, Ju Y, Tang H (2018) Predicting diabetes mellitus with machine learning techniques. Front Genet 9:515
Kadhm MS, Ghindawi IW, Mhawi DE (2018) An accurate diabetes prediction system based on K-means clustering and proposed classification approach. Int J Appl Eng Res 13(6):4038–4041
Esmaily H, Tayefi M, Doosti H, Ghayour-Mobarhan M, Nezami H, Amirabadizadeh A (2018) A comparison between decision tree and random forest in determining the risk factors associated with type 2 diabetes. J Res Health Sci 18(2):412
Barhate R, Kulkarni P (2018) Analysis of classifiers for prediction of type ii diabetes mellitus. In 2018 Fourth International Conference on Computing Communication Control and Automation (ICCUBEA). IEEE. pp 1-6
Mahmud SH, Hossin MA, Ahmed MR, Noori SRH, Sarkar MNI (2018) Machine learning based unified framework for diabetes prediction. In Proceedings of the 2018 International Conference on Big Data Engineering and Technology. pp 46-50
Fiarni C, Sipayung EM, Maemunah S (2019) Analysis and prediction of diabetes complication disease using data mining algorithm. Procedia Comput Sci 161:449–457
Hebbar A, Kumar M, Sanjay HA (2019) DRAP: Decision Tree and Random Forest Based Classification Model to Predict Diabetes. In 2019 1st International Conference on Advances in Information Technology (ICAIT). IEEE. pp 271-276
Pei D, Zhang C, Quan Y, Guo Q (2019) Identification of potential type II diabetes in a Chinese population with a sensitive decision tree approach. J Diabetes Res 2019(1):4248218
Choudhury A, Gupta D (2019) A survey on medical diagnosis of diabetes using machine learning techniques. In Recent developments in machine learning and data analytics. Springer, Singapore. pp 67-78
Sun Y, Zhang D (2019) Diagnosis and analysis of diabetic retinopathy based on electronic health records. Ieee Access 7:86115–86120
Choubey DK, Kumar P, Tripathi S, Kumar S (2020) Performance evaluation of classification methods with PCA and PSO for diabetes. Netw Model Anal Health Inform Bioinform 9(1):1–30
Al-Zebari A, Sengur A (2019) Performance Comparison of Machine Learning Techniques on Diabetes Disease Detection. In 2019 1st International Informatics and Software Engineering Conference (UBMYK). IEEE. pp 1-4
Pei D, Yang T, Zhang C (2020) Estimation of Diabetes in a High-Risk Adult Chinese Population Using J48 Decision Tree Model. Diabetes Metab Syndr Obes 13:4621
Maniruzzaman M, Rahman MJ, Ahammed B, Abedin MM (2020) Classification and prediction of diabetes disease using machine learning paradigm. Health inform Sci Syst 8(1):1–14
Pranto B, Mehnaz S, Mahid EB, Sadman IM, Rahman A, Momen S (2020) Evaluating machine learning methods for predicting diabetes among female patients in bangladesh. Information 11(8):374
Tigga NP, Garg S (2020) Prediction of type 2 diabetes using machine learning classification methods. Procedia Comput Sci 167:706–716
Haq AU, Li JP, Khan J, Memon MH, Nazir S, Ahmad S, … Ali A (2020) Intelligent Machine Learning Approach for Effective Recognition of Diabetes in E-Healthcare Using Clinical Data. Sensors 20(9):2649
Taser PY (2021) Application of Bagging and Boosting Approaches Using Decision Tree-Based Algorithms in Diabetes Risk Prediction. In Multidisciplinary Digital Publishing Institute Proceedings. vol. 74, no. 1. p. 6
Chen T, Shang C, Su P, Keravnou-Papailiou E, Zhao Y, Antoniou G, Shen Q (2021) A Decision Tree-Initialised Neuro-fuzzy Approach for Clinical Decision Support. Artif Intell Med 111:101986
Emon MU, Zannat R, Khatun T, Rahman M, Keya MS (2021) Performance Analysis of Diabetic Retinopathy Prediction using Machine Learning Models. In 2021 6th International Conference on Inventive Computation Technologies (ICICT). IEEE. pp 1048-1052
Lee M, Gatton TM, Lee KK (2010) A monitoring and advisory system for diabetes patient management using a rule-based method and KNN. Sensors 10(4):3934–3953
Chikh MA, Saidi M, Settouti N (2012) Diagnosis of diabetes diseases using an artificial immune recognition system2 (AIRS2) with fuzzy k-nearest neighbor. J Med Syst 36(5):2721–2729
Aslam MW, Zhu Z, Nandi AK (2013) Feature generation using genetic programming with comparative partner selection for diabetes classification. Expert Syst Appl 40(13):5402–5412
Christobel YA, Sivaprakasam P (2013) A new classwise k nearest neighbor (CKNN) method for the classification of diabetes dataset. Int J Eng Adv Technol 2(3):396–200
NirmalaDevi M, Alias Balamurugan SA, Swathi UV (2013) An amalgam KNN to predict diabetes mellitus. In 2013 IEEE International Conference ON Emerging Trends in Computing, Communication and Nanotechnology (ICECCN). IEEE. pp 691-695
Sarwar A, Sharma V (2014) Comparative analysis of machine learning techniques in prognosis of type II diabetes. AI & Soc 29(1):123–129
Farahmandian M, Lotfi Y, Maleki I (2015) Data mining algorithms application in diabetes diseases diagnosis: A case study. Magnt Res Tech Rep 3(1):989–997
Hidalgo JI, Colmenar JM, Kronberger G, Winkler SM, Garnica O, Lanchares J (2017) Data based prediction of blood glucose concentrations using evolutionary methods. J Med Syst 41(9):1–20
Kumar PS, Pranavi S (2017) Performance analysis of machine learning algorithms on diabetes dataset using big data analytics. In 2017 International Conference on Infocom Technologies and Unmanned Systems (Trends and Future Directions)(ICTUS). IEEE. pp 508-513
Aiello EM, Toffanin C, Messori M, Cobelli C, Magni L (2018) Postprandial glucose regulation via KNN meal classification in type 1 diabetes. IEEE Control Syst Lett 3(2):230–235
Mittal K, Aggarwal G, Mahajan P (2019) Performance study of K-nearest neighbor classifier and K-means clustering for predicting the diagnostic accuracy. Int J Inf Technol 11(3):535–540
Dey SK, Hossain A, Rahman MM (2018) Implementation of a web application to predict diabetes disease: an approach using machine learning algorithm. In 2018 21st international conference of computer and information technology (ICCIT). IEEE. pp 1-5
Azrar A, Ali Y, Awais M, Zaheer K (2018) Data mining models comparison for diabetes prediction. Int J Adv Comput Sci Appl 9(8):320–323
Alehegn M, Joshi RR, Mulay P (2019) Diabetes analysis and prediction using random forest KNN Naïve Bayes and J48: An ensemble approach. Int J Sci Technol Res 8(9):1346–1354
Aminah R, Saputro AH (2019) Diabetes prediction system based on iridology using machine learning. In 2019 6th International Conference on Information Technology, Computer and Electrical Engineering (ICITACEE). IEEE. pp 1-6
Faruque MF, Sarker IH (2019) Performance analysis of machine learning techniques to predict diabetes mellitus. In 2019 International Conference on Electrical, Computer and Communication Engineering (ECCE). IEEE. pp 1-4
Dahiwade D, Patle G, Meshram E (2019) Designing disease prediction model using machine learning approach. In 2019 3rd International Conference on Computing Methodologies and Communication (ICCMC). IEEE. pp 1211-1215
El-Sappagh S, Elmogy M, Ali F, Abuhmed T, Islam SM, Kwak KS (2019) A comprehensive medical decision–support framework based on a heterogeneous ensemble classifier for diabetes prediction. Electronics 8(6):635
Ali AMEER, Alrubei MA, Hassan LFM, Al-Ja’afari MA, Abdulwahed SH (2020) Diabetes classification based on KNN. IIUM Eng J 21(1):175–181
Garcia-Carretero R, Vigil-Medina L, Mora-Jimenez I, Soguero-Ruiz C, Barquero-Perez O, Ramos-Lopez J (2020) Use of a K-nearest neighbors model to predict the development of type 2 diabetes within 2 years in an obese, hypertensive population. Med Biol Eng Comput 58(5):991–1002
Gupta SC, Goel N (2020) Performance enhancement of diabetes prediction by finding optimum K for KNN classifier with feature selection method. In 2020 Third International Conference on Smart Systems and Inventive Technology (ICSSIT). IEEE. pp 980-986
Hassan AS, Malaserene I, Leema AA (2020) Diabetes Mellitus Prediction using Classification Techniques. Int J Innov Technol Explor Eng 9(5):2080–2084
Sarker IH, Faruque F, Alqahtani H, Kalim A (2018) K-nearest neighbor learning based diabetes mellitus prediction and analysis for eHealth services. EAI Endorsed Trans Scalable Inf Syst 7(26):e4–e4
Bhardwaj C, Jain S, Sood M (2021) Hierarchical severity grade classification of non-proliferative diabetic retinopathy. J Ambient Intell Humaniz Comput 12(2):2649–2670
Mohanty S, Mishra A, Saxena A (2021) Medical Data Analysis Using Machine Learning with KNN. In International Conference on Innovative Computing and Communications. Springer, Singapore. pp 473-485
Patra R (2021) Analysis and Prediction Of Pima Indian Diabetes Dataset Using SDKNN Classifier Technique. In IOP Conference Series: Materials Science and Engineering. vol. 1070, no. 1. IOP Publishing. p 012059
Shinde VD, Raut JR, Sharma Y (2021) Performance evaluation of various supervised machine learning algorithms for diabetes prediction. Eur J Mol Clin Med 7(8):4921–4925
Sopharak A, Dailey MN, Uyyanonvara B, Barman S, Williamson T, Nwe KT, Moe YA (2010) Machine learning approach to automatic exudate detection in retinal images from diabetic patients. J Mod Opt 57(2):124–135
Tama BA (2011) An early detection method of type-2 diabetes mellitus in public hospital. Telkomnika 9(2):287–294
Guo Y, Bai G, Hu Y (2012) Using bayes network for prediction of type-2 diabetes. In 2012 International Conference for Internet Technology and Secured Transactions. IEEE. pp 471-472
Leung RK, Wang Y, Ma RC, Luk AO, Lam V, Ng M, … Chan JC (2013) Using a multi-staged strategy based on machine learning and mathematical modeling to predict genotype-phenotype risk patterns in diabetic kidney disease: a prospective case–control cohort analysis. BMC Nephrol 14(1):1–9
Lee BJ, Ku B, Nam J, Pham DD, Kim JY (2013) Prediction of fasting plasma glucose status using anthropometric measures for diagnosing type 2 diabetes. IEEE J Biomed Health Inform 18(2):555–561
Huang GM, Huang KY, Lee TY, Weng JTY (2015) An interpretable rule-based diagnostic classification of diabetic nephropathy among type 2 diabetes patients. BMC Bioinform 16(1):1–10
Singh DAAG, Leavline EJ, Baig BS (2017) Diabetes prediction using medical data. J Comput Intell Bioinforma 10(1):1–8
Das H, Naik B, Behera HS (2018) Classification of diabetes mellitus disease (DMD): a data mining (DM) approach. In Progress in computing, analytics and networking. Springer, Singapore. pp 539-549
Insani MI, Alamsyah A, Putra AT (2018) Implementation of Expert System for Diabetes Diseases using Naïve Bayes and Certainty Factor Methods. Sci J Inform 5(2):185–193
Uddin S, Khan A, Hossain ME, Moni MA (2019) Comparing different supervised machine learning algorithms for disease prediction. BMC Med Inform Decis Mak 19(1):1–16
Birjais R, Mourya AK, Chauhan R, Kaur H (2019) Prediction and diagnosis of future diabetes risk: a machine learning approach. SN Appl Sci 1(9):1–8
Khan NS, Muaz MH, Kabir A, Islam MN (2019) A Machine Learning-Based Intelligent System for Predicting Diabetes. Int J Big Data Analytics Healthcare 4(2):1–20
Sonar P, JayaMalini K (2019) Diabetes prediction using different machine learning approaches. In 2019 3rd International Conference on Computing Methodologies and Communication (ICCMC). IEEE. pp 367-371
Nakra A, Duhan M (2019) Comparative Analysis of Bayes Net Classifier, Naive Bayes Classifier and Combination of both Classifiers using WEKA. IJ Inf Technol Comput Sci 11:38–45
Jackins V, Vimal S, Kaliappan M, Lee MY (2021) AI-based smart prediction of clinical disease using random forest classifier and Naive Bayes. J Supercomput 77(5):5198–5219
Priya KL, Kypa MSCR, Reddy MMS, Reddy GRM (2020) A Novel Approach to Predict Diabetes by Using Naive Bayes Classifier. In 2020 4th International Conference on Trends in Electronics and Informatics (ICOEI)(48184). IEEE. pp 603-607
Rghioui A, Lloret J, Harane M, Oumnad A (2020) A Smart Glucose Monitoring System for Diabetic Patient. Electronics 9(4):678
Khalilia M, Chakraborty S, Popescu M (2011) Predicting disease risks from highly imbalanced data using random forest. BMC Med Inform Decis Mak 11(1):1–13
Casanova R, Saldana S, Chew EY, Danis RP, Greven CM, Ambrosius WT (2014) Application of random forests methods to diabetic retinopathy classification analyses. PLoS One 9(6):e98587
Sabariah MMK, Hanifa SA and Sa'adah MS (2014) Early detection of type II Diabetes Mellitus with random forest and classification and regression tree (CART). In 2014 International Conference of Advanced Informatics: Concept, Theory and Application (ICAICTA). IEEE 238-242
Butwall M, Kumar S (2015) A data mining approach for the diagnosis of diabetes mellitus using random forest classifier. Int J Comput Appl 120(8)
Xu W, Zhang J, Zhang Q, Wei X (2017) Risk prediction of type II diabetes based on random forest model. In 2017 Third International Conference on Advances in Electrical, Electronics, Information, Communication and Bio-Informatics (AEEICB). IEEE. pp 382-386
Kumar NK, Vigneswari D, Krishna MV, Reddy GP (2019) An optimized random forest classifier for diabetes mellitus. In Emerging Technologies in Data Mining and Information Security. Springer, Singapore. pp 765-773
VijiyaKumar K, Lavanya B, Nirmala I, Caroline SS (2019) Random Forest Algorithm for the Prediction of Diabetes. In 2019 IEEE International Conference on System, Computation, Automation and Networking (ICSCAN). IEEE. pp 1-5
Kaur M, Gianey HK, Singh D, Sabharwal M (2019) Multi-objective differential evolution based random forest for e-health applications. Modern Phys Lett B 33(05):1950022
Alam MZ, Rahman MS, Rahman MS (2019) A Random Forest based predictor for medical data classification using feature ranking. Inform Med Unlocked 15:100180
Kaur P, Kumar R, Kumar M (2019) A healthcare monitoring system using random forest and internet of things (IoT). Multimed Tools Appl 78(14):19905–19916
Benbelkacem S, Atmani B (2019) Random forests for diabetes diagnosis. In 2019 International Conference on Computer and Information Sciences (ICCIS). IEEE. pp 1-4
Wang J, Shi L (2020) Prediction of medical expenditures of diagnosed diabetics and the assessment of its related factors using a random forest model, MEPS 2000–2015. Int J Qual Health Care 32(2):99–112
Wang X, Zhai M, Ren Z, Ren H, Li M, Quan D, … Qiu L (2021) Exploratory study on classification of diabetes mellitus through a combined Random Forest Classifier. BMC Med Inform Decis Mak 21(1):1–14
Ooka T, Johno H, Nakamoto K, Yoda Y, Yokomichi H, Yamagata Z (2021) Random forest approach for determining risk prediction and predictive factors of type 2 diabetes: large-scale health check-up data in Japan. BMJ Nutrition, Prevention & Health 4(1):140
Padmaja P, Vikkurty S, Siddiqui NI, Dasari P, Ambica B, Rao VV, … Rudraraju VR (2008) Characteristic evaluation of diabetes data using clustering techniques. IJCSNS 8(11):244
Khanna S, Agarwal S (2013) An Integrated Approach towards the prediction of Likelihood of Diabetes. In 2013 International Conference on Machine Intelligence and Research Advancement. IEEE. pp 294-298
Paul R, Hoque ASML (2010) Clustering medical data to predict the likelihood of diseases. In 2010 fifth international conference on digital information management (ICDIM). IEEE. pp 44-49
Al Hazemi F, Youn CH, Al-Rubeaan KA (2011) Grid-based interactive diabetes system. In 2011 IEEE First International Conference on Healthcare Informatics, Imaging and Systems Biology. IEEE. pp 258-263
Antonelli D, Baralis E, Bruno G, Cerquitelli T, Chiusano S, Mahoto N (2013) Analysis of diabetic patients through their examination history. Expert Syst Appl 40(11):4672–4678
Al-Hazemi F (2014) Grid-based Workflow System for Chronic Disease Study. Life Sci J 11(7):1–3
Jeong S, Youn CH, Kim YW, Shim SO (2014) Temporal progress model of metabolic syndrome for clinical decision support system. IRBM 35(6):310–320
Kim E, Oh W, Pieczkiewicz DS, Castro MR, Caraballo PJ, Simon GJ (2014) Divisive hierarchical clustering towards identifying clinically significant pre-diabetes subpopulations. In AMIA Annual Symposium Proceedings, vol. 2014. American Medical Informatics Association. p 1815
Vijayarani DS, Jothi MP (2014) Hierarchical and partitioning clustering algorithms for detecting outliers in data streams. International Journal of Advanced Research in Computer and Communication Engineering, ISSN, pp 2278–1021
Sanakal R, Jayakumari T (2014) Prognosis of diabetes using data mining approach-fuzzy C means clustering and support vector machine. Int J Comput Trends Technol 11(2):94–98
Flynt A, Daepp MI (2015) Diet-related chronic disease in the northeastern United States: a model-based clustering approach. Int J Health Geogr 14(1):1–14
Barale MS, Shirke DT (2016) Cascaded modeling for PIMA Indian diabetes data. Int J Comput Appl 139(11):1–4
Bhatia K, Syal R (2017) Predictive analysis using hybrid clustering in diabetes diagnosis. In 2017 Recent Developments in Control, Automation & Power Engineering (RDCAPE). IEEE. pp 447-452
Cheruku R, Edla DR, Kuppili V (2017) Diabetes classification using radial basis function network by combining cluster validity index and bat optimization with novel fitness function. Int J Comput Intell Syst 10(1):247–265
Ahlqvist E, Storm P, Käräjämäki A, Martinell M, Dorkhan M, Carlsson A, … Groop L (2018) Novel subgroups of adult-onset diabetes and their association with outcomes: a data-driven cluster analysis of six variables. Lancet Diabetes Endocrinol 6(5):361–369
Rani S, Kautish S (2018) Association Clustering and Time Series Based Data Mining in Continuous Data for Diabetes Prediction. In 2018 Second International Conference on Intelligent Computing and Control Systems (ICICCS). IEEE. pp 1209-1214
Derevitskii IV, Kovalchuk SV (2019) Analysis course of the disease of type 2 diabetes patients using Markov chains and clustering methods. Procedia Comput Sci 156:114–122
Lasek P, Mei Z (2019) Clustering and visualization of a high-dimensional diabetes dataset. Procedia Comput Sci 159:2179–2188
Raihan M, Islam MT, Farzana F, Raju MGM, Mondal HS (2019) An Empirical Study to Predict Diabetes Mellitus using K-Means and Hierarchical Clustering Techniques. In 2019 10th International Conference on Computing, Communication and Networking Technologies (ICCCNT). IEEE. pp 1-6
Nguyen HT, Phan NYK, Luong HH, Cao NH, Huynh HX (2020) Binning approach based on classical clustering for type 2 diabetes diagnosis. Int J Adv Comput Sci Appl 11(3)
Syafaah L, Azizah DF, Sofiani IR, Lestandy M, Faruq A (2020) Self-Monitoring and Detection of Diabetes with art Toilet based on Image Processing and K-Means Technique. In 2020 IEEE International Conference on Automatic Control and Intelligent Systems (I2CACIS). IEEE. pp 87-91
Anwar S, Alqarni A, Alafnan A, Alamri A, Mathew S, Ricciardi E, Mathew S, Alamri A, Alafnan A, Alqarni A, Anwar S (2021) Cluster identification of diabetic risk factors among Saudi population. J Pharma Res Int 3(8)45–58
Takahashi K, Uchiyama H, Yanagisawa S, Kamae I (2006) The logistic regression and ROC analysis of group-based screening for predicting diabetes incidence in four years. Kobe J Med Sci 52(6):171
Sparacino G, Zanderigo F, Corazza S, Maran A, Facchinetti A, Cobelli C (2007) Glucose concentration can be predicted ahead in time from continuous glucose monitoring sensor time-series. IEEE Trans Biomed Eng 54(5):931–937
Eren-Oruklu M, Cinar A, Quinn L, Smith D (2009) Estimation of future glucose concentrations with subject-specific recursive linear models. Diabetes Technol Ther 11(4):243–253
Eren-Oruklu M, Cinar A, Quinn L, Smith D (2009) Adaptive control strategy for regulation of blood glucose levels in patients with type 1 diabetes. J Process Control 19(8):1333–1346
Gani A, Gribok AV, Rajaraman S, Ward WK, Reifman J (2008) Predicting subcutaneous glucose concentration in humans: data-driven glucose modeling. IEEE Trans Biomed Eng 56(2):246–254
Estrada GC, Kirchsteiger H, del Re L, Renard E (2010) Innovative approach for online prediction of blood glucose profile in type 1 diabetes patients. In Proceedings of the 2010 American Control Conference. IEEE. pp 2015-2020
Gani A, Gribok AV, Lu Y, Ward WK, Vigersky RA, Reifman J (2009) Universal glucose models for predicting subcutaneous glucose concentration in humans. IEEE Trans Inf Technol Biomed 14(1):157–165
Lu Y, Rajaraman S, Ward WK, Vigersky RA, Reifman J (2011) Predicting human subcutaneous glucose concentration in real time: a universal data-driven approach. In 2011 Annual International Conference of the IEEE Engineering in Medicine and Biology Society. IEEE. pp 7945-7948
Zhao C, Dassau E, Zisser HC, Jovanovič L, Doyle FJ III, Seborg DE (2014) Online prediction of subcutaneous glucose concentration for type 1 diabetes using empirical models and frequency-band separation. AICHE J 60(2):574–584
Georga EI, Protopappas VC, Ardigo D, Marina M, Zavaroni I, Polyzos D, Fotiadis DI (2012) Multivariate prediction of subcutaneous glucose concentration in type 1 diabetes patients based on support vector regression. IEEE J Biomed Health Inform 17(1):71–81
Bayrak ES, Turksoy K, Cinar A, Quinn L, Littlejohn E, Rollins D (2013) Hypoglycemia early alarm systems based on recursive autoregressive partial least squares models. J Diabetes Sci Technol 7(1):206–214
Yu C, Zhao C (2014) Rapid model identification for online glucose prediction of new subjects with type 1 diabetes using model migration method. IFAC Proc Volumes 47(3):2094–2099
Zhao C, Yu C (2015) Rapid model identification for online subcutaneous glucose concentration prediction for new subjects with type I diabetes. IEEE Trans Biomed Eng 62(5):1333–1344
Paul SK, Samanta M (2015) Predicting upcoming glucose levels in patients with type 1 diabetes using a generalized autoregressive conditional heteroscedasticity modelling approach. Int J Stat Med Res 4(2):188–198
Bagherzadeh-Khiabani F, Ramezankhani A, Azizi F, Hadaegh F, Steyerberg EW, Khalili D (2016) A tutorial on variable selection for clinical prediction models: feature selection methods in data mining could improve the results. J Clin Epidemiol 71:76–85
Agarwal V, Podchiyska T, Banda JM, Goel V, Leung TI, Minty EP, … Shah NH (2016) Learning statistical models of phenotypes using noisy labeled training data. J Am Med Inform Assoc 23(6):1166–1173
Lee BJ, Kim JY (2016) Identification of type 2 diabetes risk factors using phenotypes consisting of anthropometry and triglycerides based on machine learning. IEEE J Biomed Health Inform 20(1):39–46
Rahimloo P, Jafarian A (2016) Prediction of diabetes by using artificial neural network, logistic regression statistical model and combination of them. Bull Soc R Sci Liège 85:1148–1164
Article MathSciNet Google Scholar
Rau HH, Hsu CY, Lin YA, Atique S, Fuad A, Wei LM, Hsu MH (2016) Development of a web-based liver cancer prediction model for type II diabetes patients by using an artificial neural network. Comput Methods Prog Biomed 125:58–65
Usman S, Reaz MBI, Ali MAM (2016) Risk prediction of having increased arterial stiffness among diabetic patients using logistic regression. In 2016 IEEE EMBS Conference on Biomedical Engineering and Sciences (IECBES). IEEE. pp 699-701
Zhao LP, Bolouri H, Zhao M, Geraghty DE, Lernmark Å, Better Diabetes Diagnosis Study Group (2016) An object-oriented regression for building disease predictive models with multiallelic HLA genes. Genet Epidemiol 40(4):315–332
Bajestani NS, Kamyad AV, Esfahani EN, Zare A (2018) Prediction of retinopathy in diabetic patients using type-2 fuzzy regression model. Eur J Oper Res 264(3):859–869
Hassan M, Butt MA, Baba MZ (2017) Logistic regression versus neural networks: the best accuracy in prediction of diabetes disease. Asi J Comp Sci Tech 6:33–42
Zheng T, Xie W, Xu L, He X, Zhang Y, You M, … Chen Y (2017) A machine learning-based framework to identify type 2 diabetes through electronic health records. Int J Med Inform 97:120–127
Wu H, Yang S, Huang Z, He J, Wang X (2018) Type 2 diabetes mellitus prediction model based on data mining. Inform Med Unlocked 10:100–107
Qiu S, Li J, Chen B, Wang P, Gao X (2019) An improved prediction method for diabetes based on a feature-based least angle regression algorithm. In Proceedings of the 3rd International Conference on Machine Learning and Soft Computing. pp 232-238
Yao L, Zhong Y, Wu J, Zhang G, Chen L, Guan P, … Liu L (2019) Multivariable logistic regression and back propagation artificial neural network to predict diabetic retinopathy. Diabetes Metab Syndr Obes 12:1943
Alshamlan H, Taleb HB, Al Sahow A (2020) A Gene Prediction Function for Type 2 Diabetes Mellitus using Logistic Regression. In 2020 11th International Conference on Information and Communication Systems (ICICS). IEEE. pp 1-4
Kopitar L, Kocbek P, Cilar L, Sheikh A, Stiglic G (2020) Early detection of type 2 diabetes mellitus using machine learning-based prediction models. Sci Rep 10(1):1–12
Hsu W, Lee ML, Liu B, Ling TW (2000) Exploration mining in diabetic patients databases: findings and conclusions. In Proceedings of the sixth ACM SIGKDD international conference on Knowledge discovery and data mining. pp 430-436
Stilou S, Bamidis PD, Maglaveras N, Pappas C (2001) Mining association rules from clinical databases: an intelligent diagnostic process in healthcare. Stud Health Technol Inform 2:1399–1403
Zorman M, Masuda G, Kokol P, Yamamoto R, Stiglic B (2002) Mining diabetes database with decision trees and association rules. In Proceedings of 15th IEEE Symposium on Computer-Based Medical Systems (CBMS 2002). IEEE. pp 134-139
Duru N (2005) An application of apriori algorithm on a diabetic database. In International Conference on Knowledge-Based and Intelligent Information and Engineering Systems (pp. 398-404). Springer, Berlin, Heidelberg
Mao W and Mao J (2009) The application of apriori-gen algorithm in the association study in type 2 diabetes. In 2009 3rd International Conference on Bioinformatics and Biomedical Engineering. IEEE 1-4
Patil B, Joshi R, Toshniwal D (2010) Association rule for classification of type -2 diabetic patients. In 2010 Second International Conference on Machine Learning and Computing. IEEE 330-334
Patil BM, Joshi RC, Toshniwal D (2011) Classification of type-2 diabetic patients by using Apriori and predictive Apriori. Int J Comput Vis Robotics 2(3):254–265
Kasemthaweesab P and Kurutach W (2012) Association analysis of diabetes mellitus (DM) with complication states based on association rules. In 2012 7th IEEE Conference on Industrial Electronics and Applications (ICIEA). IEEE 1453-1457
Kim HS, Shin AM, Kim MK, Kim YN (2012) Comorbidity study on type 2 diabetes mellitus using data mining. Korean J Int Med 27(2):197
Simon GJ, Schrom J, Castro MR, Li PW and Caraballo P J (2013) Survival association rule mining towards type 2 diabetes risk assessment. In AMIA annual symposium proceedings. Am Med Inform Assoc 2013:1293
Schrom JR, Caraballo PJ, Castro MR and Simon GJ (2013) Quantifying the effect of statin use in pre-diabetic phenotypes discovered through association rule mining. In AMIA Annual Symposium Proceedings. Am Med Inform Assoc 2013:1249
Lakshmi KS and Kumar GS (2014) Association rule extraction from medical transcripts of diabetic patients. In The Fifth International Conference on the Applications of Digital Information and Web Technologies (ICADIWT 2014). IEEE 201-206
Karthikeyan T, Vembandasamy K (2015) A novel algorithm to diagnosis type II diabetes mellitus based on association rule mining using MPSO-LSSVM with outlier detection method. Indian J Sci Technol 8(S8):310–320
Ramezankhani A, Pournik O, Shahrabi J, Azizi F, Hadaegh F (2015) An application of association rule mining to extract risk pattern for type 2 diabetes using tehran lipid and glucose study database. Int J Endocrinol Metabol 13(2)
Simon GJ, Caraballo PJ, Therneau TM, Cha SS, Castro MR, Li PW (2013) Extending association rule summarization techniques to assess risk of diabetes mellitus. IEEE Trans Knowl Data Eng 27(1):130–141
Kamalesh MD, Prasanna KH, Bharathi B, Dhanalakshmi R and Canessane RA (2016) Predicting the risk of diabetes mellitus to subpopulations using association rule mining. In proceedings of the international conference on soft computing systems (pp. 59-65). Springer, New Delhi
Alam TM, Iqbal MA, Ali Y, Wahab A, Ijaz S, Baig TI, … Abbas Z (2019) A model for early prediction of diabetes. Inform Med Unlocked 16:100204
Lu PH, Keng JL, Tsai FM, Lu PH, Kuo CY (2021) An apriori algorithm-based association rule analysis to identify acupoint combinations for treating diabetic gastroparesis. Evid-Based Complement Altern Med 2021(1):6649331
Cheng Y, Wang F, Zhang P and Hu J (2016). Risk prediction with electronic health records: A deep learning approach. In Proceedings of the 2016 SIAM International Conference on Data Mining. Soc Industrial App Mathematics 432-440
Pratt H, Coenen F, Broadbent DM, Harding SP, Zheng Y (2016) Convolutional neural networks for diabetic retinopathy. Procedia Comput Sci 90:200–205
Shi X, Hu Y, Zhang Y, Li W, Hao Y, Alelaiwi A, … Hossain MS (2016) Multiple disease risk assessment with uniform model based on medical clinical notes. IEEE Access 4:7074–7083
Zhu Z, Yin C, Qian B, Cheng Y, Wei J and Wang F (2016) Measuring patient similarities via a deep architecture with medical concept embedding. In 2016 IEEE 16th International Conference on Data Mining (ICDM). IEEE 749-758
Lekha S, Suchetha M (2017) Real-time non-invasive detection and classification of diabetes using modified convolution neural network. IEEE J Biomed Health Inform 22(5):1630–1636
Mohebbi A, Aradóttir TB, Johansen AR, Bengtsson H, Fraccaro M and Mørup M (2017) A deep learning approach to adherence detection for type 2 diabetics. In 2017 39th Annual International Conference of the IEEE Engineering in Medicine and Biology Society (EMBC). IEEE 2896-2899
Kwasigroch A, Jarzembinski B and Grochowski M (2018) Deep CNN based decision support system for detection and assessing the stage of diabetic retinopathy. In 2018 International Interdisciplinary PhD Workshop (IIPhDW). IEEE 111-116
Swapna G, Kp S, Vinayakumar R (2018) Automated detection of diabetes using CNN and CNN-LSTM network and heart rate signals. Procedia Comput Sci 132:1253–1262
Swapna G, Vinayakumar R, Soman KP (2018) Diabetes detection using deep learning algorithms. ICT Express 4(4):243–246
Butt MM, Latif G, Iskandar DA, Alghazo J, Khan AH (2019) Multi-channel Convolutions Neural Network Based Diabetic Retinopathy Detection from Fundus Images. Procedia Comput Sci 163:283–291
Khan SH, Abbas Z and Rizvi SD (2019) Classification of diabetic retinopathy images based on customised CNN architecture. In 2019 Amity International Conference on Artificial Intelligence (AICAI). IEEE 244-248
Sun Y (2019) The neural network of one-dimensional convolution-an example of the diagnosis of diabetic retinopathy. IEEE Access 7:69657–69666
Raj MAH, Al Mamun M and Faruk MF (2020) CNN Based Diabetic Retinopathy Status Prediction Using Fundus Images. In 2020 IEEE Region 10 Symposium (TENSYMP). IEEE 190-193
Rahman M, Islam D, Mukti RJ, Saha I (2020) A deep learning approach based on convolutional LSTM for detecting diabetes. Comput Biol Chem 88:107329
Ismail WN, Hassan MM, Alsalamah HA, Fortino G (2020) CNN-based health model for regular health factors analysis in Internet-of-medical things environment. IEEE Access 8:52541–52549
Islam MT, Al-Absi HR, Ruagh EA, Alam T (2021) DiaNet: A deep learning based architecture to diagnose diabetes using retinal images only. IEEE Access 9:15686–15695
Allam F, Nossai Z, Gomma H, Ibrahim I, Abdelsalam M (2011) A recurrent neural network approach for predicting glucose concentration in type-1 diabetic patients, In Engineering Applications of Neural Networks (pp. 254-259). Springer, Berlin, Heidelberg
Chu J, Dong W, He K, Duan H, Huang Z (2018) Using neural attention networks to detect adverse medical events from electronic health records. J Biomed Inform 87:118–130
Wang WW, Li H, Cui L, Hong X and Yan Z (2018) Predicting clinical visits using recurrent neural networks and demographic information. In 2018 IEEE 22nd International Conference on Computer Supported Cooperative Work in Design ((CSCWD)). IEEE 353-358
Wu S, Liu S, Sohn S, Moon S, Wi CI, Juhn Y, Liu H (2018) Modeling asynchronous event sequences with RNNs. J Biomed Inform 83:167–177
Dong Y, Wen R, Zhang K and Zhang L (2019) A Novel RNN-Based Blood Glucose Prediction Approach Using Population and Individual Characteristics. In 2019 IEEE 7th International Conference on Bioinformatics and Computational Biology (ICBCB). IEEE 145-149
Dong Y, Wen R, Li Z, Zhang K and Zhang L (2019) Clu-RNN: a new RNN based approach to diabetic blood glucose prediction. In 2019 IEEE 7th International Conference on Bioinformatics and Computational Biology (ICBCB). IEEE 50-55
Jang JS, Lee MJ, Lee TR (2019) Development of T2DM Prediction Model Using RNN. J Digit Converg 17(8):249–255
Munoz-Organero M (2020) Deep Physiological Model for Blood Glucose Prediction in T1DM Patients. Sensors 20(14):3896
Zhou H, Myrzashova R, Zheng R (2020) Diabetes prediction model based on an enhanced deep neural network. EURASIP J Wirel Commun Netw 2020(1):1–13
Zhu T, Li K, Chen J, Herrero P, Georgiou P (2020) Dilated recurrent neural networks for glucose forecasting in type 1 diabetes. J Healthc Inform Res 4(3):308–324
Rabby MF, Tu Y, Hossen MI, Lee I, Maida AS, Hei X (2021) Stacked LSTM based deep recurrent neural network with kalman smoothing for blood glucose prediction. BMC Med Inform Decis Mak 21(1):1–15
Martinsson J, Schliep A, Eliasson B, Meijner C, Persson S and Mogren O (2018) Automatic blood glucose prediction with confidence using recurrent neural networks. In KHD@ IJCAI
Chen J, Li K, Herrero P, Zhu T and Georgiou P (2018) Dilated Recurrent Neural Network for Short-time Prediction of Glucose Concentration. In KHD@ IJCAI (pp. 69-73)
Jaafar SFB and Ali DM (2005) Diabetes mellitus forecast using artificial neural network (ANN). In 2005 Asian Conference on Sensors and the International Conference on New Techniques in Pharmaceutical and Biomedical Research. IEEE 135-139
Mougiakakou SG, Prountzou A, Iliopoulou D, Nikita KS, Vazeou A and Bartsocas CS (2006) Neural network based glucose-insulin metabolism models for children with type 1 diabetes. In 2006 International Conference of the IEEE Engineering in Medicine and Biology Society. IEEE 3545-3548
Dey R, Bajpai V, Gandhi G and Dey B (2008) Application of artificial neural network (ANN) technique for diagnosing diabetes mellitus. In 2008 IEEE Region 10 and the Third international Conference on Industrial and Information Systems. IEEE 1-4
Pappada SM, Cameron BD, Rosman PM (2008) Development of a neural network for prediction of glucose concentration in type 1 diabetes patients. J Diabetes Sci Technol 2(5):792–801
Zainuddin Z, Pauline O, Ardil C (2009) A neural network approach in predicting the blood glucose level for diabetic patients. Int J Comput Intell 5(1):72–79
Pérez-Gandía C, Facchinetti A, Sparacino G, Cobelli C, Gómez EJ, Rigla M, … Hernando ME (2010) Artificial neural network algorithm for online glucose prediction from continuous glucose monitoring. Diabetes Technol Ther 12(1):81–88
Pappada SM, Borst MJ, Cameron BD, Bourey RE, Lather JD, Shipp D, … Papadimos TJ (2010) Development of a neural network model for predicting glucose levels in a surgical critical care setting. Patient Safety Surg 4(1):1–5
Chakraborty M and Tudu B (2010) Comparison of ANN models to predict LDL level in Diabetes Mellitus type 2. In 2010 International Conference on Systems in Medicine and Biology. IEEE 392-396
Allam F, Nossair Z, Gomma H, Ibrahim I and Abd-el Salam M (2011) Prediction of subcutaneous glucose concentration for type-1 diabetic patients using a feed forward neural network. In The 2011 International Conference on Computer Engineering and Systems. IEEE 129-133
Pappada SM, Cameron BD, Rosman PM, Bourey RE, Papadimos TJ, Olorunto W, Borst MJ (2011) Neural network-based real-time prediction of glucose in patients with insulin-dependent diabetes. Diabetes Technol Ther 13(2):135–141
Robertson G, Lehmann ED, Sandham W, Hamilton D (2011) Blood glucose prediction using artificial neural networks trained with the AIDA diabetes simulator: a proof-of-concept pilot study. J Electr Comput Eng 2011(1):681786
Ali JB, Hamdi T, Fnaiech N, Di Costanzo V, Fnaiech F, Ginoux JM (2018) Continuous blood glucose level prediction of Type 1 Diabetes based on Artificial Neural Network. Biocybern Biomed Eng 38(4):828–840
Kathiroli R, RajaKumari R and Gokulprasanth P (2018) Diagnosis Of Diabetes Using Cascade Correlation And Artificial Neural Network. In 2018 Tenth International Conference on Advanced Computing (ICoAC). IEEE 299-306
Senturk Z (2020) Artificial Neural Networks based decision support system for the detection of diabetic retinopathy. Sakarya Univ Fen Bilim Enst Derg 24(2):424–431
Sun Q, Jankovic MV, Bally L and Mougiakakou SG (2018) Predicting blood glucose with an LSTM and Bi-LSTM based deep neural network. In 2018 14th Symposium on Neural Networks and Applications (NEUREL). IEEE 1-5
Farías AFS, Mendizabal A, González-Garrido AA, Romo-Vázquez R and Morales A (2018) Long Short-Term Memory Neural Networks for Identifying Type 1 Diabetes Patients with Functional Magnetic Resonance Imaging. In 2018 IEEE Latin American Conference on Computational Intelligence (LA-CCI). IEEE 1-4
Bahadur EH, Masum AKM, Barua A, Alam MGR, Chowdhury MAUZ and Alam MR (2019) LSTM Based Approach for Diabetic Symptomatic Activity Recognition Using Smartphone Sensors. In 2019 22nd International Conference on Computer and Information Technology (ICCIT). IEEE 1-6
De Bois M, El Yacoubi MA and Ammi M (2019) Prediction-coherent LSTM-based recurrent neural network for safer glucose predictions in diabetic people. In International Conference on Neural Information Processing (pp. 510-521). Springer, Cham
De Bois M, El Yacoubi MA and Ammi M (2019) Study of short-term personalized glucose predictive models on type-1 diabetic children. In 2019 International Joint Conference on Neural Networks (IJCNN). IEEE 1-8
Massaro A, Maritati V, Giannone D, Convertini D, Galiano A (2019) LSTM DSS automatism and dataset optimization for diabetes prediction. Appl Sci 9(17):3532
Padmapritha T (2019) Prediction of Blood Glucose Level by using an LSTM based Recurrent Neural networks. In 2019 IEEE International Conference on Clean Energy and Energy Efficient Electronics Circuit for Sustainable Development (INCCES). IEEE 1-4
Carrillo-Moreno J, Pérez-Gandía C, Sendra-Arranz R, García-Sáez G, Hernando ME, Gutiérrez A (2020) Long short-term memory neural network for glucose prediction. Neural Comput Applic 33:4191–4203
Amalia R, Bustamam A, Sarwinda D (2021) Detection and description generation of diabetic retinopathy using convolutional neural network and long short-term memory. J Phys Conf Ser 1722(1):012010
El Idrissi T and Idri A (2020) Deep Learning for Blood Glucose Prediction: CNN vs LSTM. In International Conference on Computational Science and Its Applications (pp. 379-393). Springer, Cham
El Idriss T, Idri A, Abnane I and Bakkoury Z (2019) Predicting blood glucose using an LSTM neural network. In 2019 Federated Conference on Computer Science and Information Systems (FedCSIS). IEEE 35-41
Wang W, Tong M, Yu M (2020) Blood Glucose Prediction With VMD and LSTM Optimized by Improved Particle Swarm Optimization. IEEE Access 8:217908–217916
Beaulieu-Jones BK, Moore JH and POOLED RESOURCE OPEN-ACCESS ALS CLINICAL TRIALS CONSORTIUM (2017) Missing data imputation in the electronic health record using deeply learned autoencoders. In Pacific Symposium on Biocomputing 2017 (pp. 207-218)
Hwang U, Choi S, Lee HB and Yoon S (2018) Adversarial training for disease prediction from electronic health records with missing data. arXiv preprint arXiv:1711.04126
Babu SB, Suneetha A, Babu GC, Kumar YJN, Karuna G (2018) Medical disease prediction using grey wolf optimization and auto encoder based recurrent neural network. Period Eng Nat Sci 6(1):229–240
Kannadasan K, Edla DR, Kuppili V (2019) Type 2 diabetes data classification using stacked autoencoders in deep neural networks. Clin Epidemiol Glob Health 7(4):530–535
Kumar VB, Vijayalakshmi K and Padmavathamma M (2019) A hybrid data mining approach for diabetes prediction and classification. In 2019 World Congress on Engineering and Computer Science, WCECS (Vol. 22, pp. 298-303)
Sahoo AK, Pradhan C and Das H (2020) Performance evaluation of different machine learning methods and deep-learning based convolutional neural network for health decision making. In Nature inspired computing for data science (pp. 201-212). Springer, Cham
Zhang Q, Zhou J and Zhang B (2020) A noninvasive method to detect diabetes mellitus and lung cancer using the stacked sparse autoencoder. In ICASSP 2020-2020 IEEE International Conference on Acoustics, Speech and Signal Processing (ICASSP). IEEE 1409-1413
García-Ordás MT, Benavides C, Benítez-Andrades JA, Alaiz-Moretón H, García-Rodríguez I (2021) Diabetes detection using deep learning techniques with oversampling and feature augmentation. Comput Methods Prog Biomed 202:105968
Kayaer K and Yildirim T (2003) Medical diagnosis on Pima Indian diabetes using general regression neural networks. In Proceedings of the international conference on artificial neural networks and neural information processing (ICANN/ICONIP) (Vol. 181, p. 184).
Ergün U, Barýþçý N, Ozan AT, Serhatlýoðlu S, Oğur E, Hardalaç F, Güler İ (2004) Classification of MCA stenosis in diabetes by MLP and RBF neural network. J Med Syst 28(5):475–487
Quchani SA, Tahami E (2007) Comparison of MLP and Elman neural network for blood glucose level prediction in type 1 diabetics, In 3rd Kuala Lumpur International Conference on Biomedical Engineering 2006 (pp. 54-58). Springer, Berlin, Heidelberg
Bhatkar AP and Kharat GU (2015) Detection of diabetic retinopathy in retinal images using MLP classifier. In 2015 IEEE international symposium on nanoelectronic and information systems. IEEE 331-335
Ambilwade RP and Manza RR (2016) Prognosis of diabetes using fuzzy inference system and multilayer perceptron. In 2016 2nd International Conference on Contemporary Computing and Informatics (IC3I). IEEE 248-252
Choubey DK, Paul S (2016) GA_MLP NN: a hybrid intelligent system for diabetes disease diagnosis. Int J Intell Syst Appl 8(1):49
Alfian G, Syafrudin M, Ijaz MF, Syaekhoni MA, Fitriyani NL, Rhee J (2018) A personalized healthcare monitoring system for diabetic patients by utilizing BLE-based sensors and real-time data processing. Sensors 18(7):2183
Mohapatra SK, Swain JK, Mohanty MN (2019) Detection of diabetes using multilayer perceptron, In International conference on intelligent computing and applications (pp. 109-116). Springer, Singapore
Bani-Salameh H, Alkhatib SM, Abdalla M, Banat R, Zyod H, Alkhatib AJ (2020) Prediction of diabetes and hypertension using multi-layer perceptron neural networks. Int J Model Simul Sci Comput 12(02):2150012
Güldoğan E, Zeynep TUNÇ, Ayça ACET, Çolak C (2020) Performance Evaluation of Different Artificial Neural Network Models in the Classification of Type 2 Diabetes Mellitus. J Cogn Syst 5(1):23–32
Mishra S, Tripathy HK, Mallick PK, Bhoi AK, Barsocchi P (2020) EAGA-MLP—An Enhanced and Adaptive Hybrid Classification Model for Diabetes Diagnosis. Sensors 20(14):4036
Om KS, Kim HC, Min BG, Shin CS, Lee HK (1998) Statistical RBF Network with Applications to an Expert System for Characterizing Diabetes Mellitus. J Electr Eng Inf Sci 3(3):355–365
Nabney IT (2004) Efficient training of RBF networks for classification. Int J Neural Syst 14(03):201–208
Venkatesan P, Anitha S (2006) Application of a radial basis function neural network for diagnosis of diabetes mellitus. Curr Sci 91(9):1195–1199
Sa’di S, Maleki A, Hashemi R, Panbechi Z, Chalabi K (2015) Comparison of data mining algorithms in the diagnosis of type II diabetes. Int J Comput Sci Appl 5(5):1–12
Ashiquzzaman A, Tushar AK, Islam MR, Shon D, Im K, Park JH, … and Kim J (2018) Reduction of overfitting in diabetes prediction using deep learning neural network. In IT convergence and security 2017 (pp. 35-43). Springer, Singapore
Chetoui M, Akhloufi MA and Kardouchi M (2018) Diabetic retinopathy detection using machine learning and texture features. In 2018 IEEE Canadian Conference on Electrical & Computer Engineering (CCECE). IEEE 1-4
Adegoke V, Chen D, Banissi E (2019) Improving prediction accuracy of breast cancer survivability and diabetes diagnosis via RBF networks trained with EKF models. Int J Comput Inf Syst Ind Manag 11:19–19
Hosseini H, Bardsiri AK (2019) Improving Diagnosis Accuracy of Diabetic Disease Using Radial Basis Function Network and Fuzzy Clustering. Front Health Inform 8(1):24
Kamble VV, Kokate RD (2020) Automated diabetic retinopathy detection using radial basis function. Procedia Comput Sci 167:799–808
Dwivedi AK (2018) Analysis of computational intelligence techniques for diabetes mellitus prediction. Neural Comput Applic 30(12):3837–3845
Thyde DN, Mohebbi A, Bengtsson H, Jensen ML, Mørup M (2021) Machine learning-based adherence detection of type 2 diabetes patients on once-daily basal insulin injections. J Diabetes Sci Technol 15(1):98–108
Pham T, Tran T, Phung D and Venkatesh S (2016) Deepcare: a deep dynamic memory model for predictive medicine. In Pacific-Asia conference on knowledge discovery and data mining (pp. 30-41). Springer, Cham
Choi E, Bahadori MT, Schuetz A, Stewart WF and Sun J (2016) Doctor ai: Predicting clinical events via recurrent neural networks. In Machine learning for healthcare conference. PMLR 301-318
Miotto R, Li L, Kidd BA, Dudley JT (2016) Deep patient: an unsupervised representation to predict the future of patients from the electronic health records. Sci Rep 6(1):1–10
Miotto R, Li L and Dudley JT (2016) Deep learning to predict patient future diseases from the electronic health records. In European Conference on Information Retrieval (pp. 768-774). Springer, Cham
Liang Z, Zhang G, Huang JX and Hu QV (2014) Deep learning for healthcare decision making with EMRs. In 2014 IEEE International Conference on Bioinformatics and Biomedicine (BIBM). IEEE 556-559
Lipton ZC, Kale DC, Elkan C and Wetzel R (2015) Learning to diagnose with LSTM recurrent neural networks. arXiv preprint arXiv:1511.03677
Che Z, Kale D, Li W, Bahadori MT and Liu Y (2015) Deep computational phenotyping. In Proceedings of the 21th ACM SIGKDD International Conference on Knowledge Discovery and Data Mining (pp. 507-516)
Lasko TA, Denny JC, Levy MA (2013) Computational phenotype discovery using unsupervised feature learning over noisy, sparse, and irregular clinical data. PLoS One 8(6):e66341
Razavian N, Marcus J and Sontag D (2016) Multi-task prediction of disease onsets from longitudinal laboratory tests. In Machine learning for healthcare conference. PMLR 73-100
Choi Y, Chiu CYI, Sontag D (2016) Learning low-dimensional representations of medical concepts. AMIA Summits Transl Sci Proc 2016:41
Tran T, Nguyen TD, Phung D, Venkatesh S (2015) Learning vector representation of medical objects via EMR-driven nonnegative restricted Boltzmann machines (eNRBM). J Biomed Inform 54:96–105
Dernoncourt F, Lee JY, Uzuner O, Szolovits P (2017) De-identification of patient notes with recurrent neural networks. J Am Med Inform Assoc 24(3):596–606
Nguyen P, Tran T, Wickramasinghe N et al (2017) Deepr: a Convolutional Net for Medical Records. IEEE J Biomed Health Inform 21:22–30
Kumar Dewangan A, Agrawal P (2015) Classification of diabetes mellitus using machine learning techniques. Int J Eng Appl Sci 2(5):257905
Deperlioğlu, O, Köse, U. (2018). Diagnosis of Diabetes by Using Deep Neural Network. 2018 2nd International Symposium on Multidisciplinary Studies and Innovative Technologies (ISMSIT).IEEE.
Katsuki T, Ono M, Koseki A, Kudo M, Haida K, Kuroda J, … and Suzuki A (2018) Risk Prediction of Diabetic Nephropathy via Interpretable Feature Extraction from EHR Using Convolutional Autoencoder. In MIE (pp. 106-110)
Makino M, Yoshimoto R, Ono M, Itoko T, Katsuki T, Koseki A, … Suzuki A (2019) Artificial intelligence predicts the progression of diabetic kidney disease using big data machine learning. Sci Rep 9(1):1–9
Deepthi K, Jereesh AS (2020) An ensemble approach for CircRNA-disease association prediction based on autoencoder and deep neural network. Gene 762:145040
Tran D, Nguyen H, Tran B, La Vecchia C, Luu HN, Nguyen T (2021) Fast and precise single-cell data analysis using a hierarchical autoencoder. Nat Commun 12(1):1–10
Li K, Daniels J, Liu C, Herrero P, Georgiou P (2019) Convolutional recurrent neural networks for glucose prediction. IEEE J Biomed Health Inform 24(2):603–613
Sistla S (2022) Predicting Diabetes using SVM Implemented by Machine Learning. International Journal of Soft Computing and Engineering 12(2):2231–2307
Li J, Ding J, Zhi DU, Gu K, Wang H (2022) Identification of type 2 diabetes based on a ten-gene biomarker prediction model constructed using a support vector machine algorithm. BioMed Res Int 2022(1):1230761
Rastogi R, Bansal M (2023) Diabetes prediction model using data mining techniques. Meas Sens 25:100605
Aslan MF, Sabanci K (2023) A novel proposal for deep learning-based diabetes prediction: Converting clinical data to image data. Diagnostics 13 (4):796
Ahamed BS, Arya MS, Nancy VAO (2022) Prediction of type-2 diabetes mellitus disease using machine learning classifiers and techniques. Front Comput Sci 4:835242
Özge ŞEN, Keser SB, Keskin K (2023) Early stage diabetes prediction using decision tree-based ensemble learning model. Int Adv Res Eng J 7(1):62–71
Suyanto S, Meliana S, Wahyuningrum T, Khomsah S (2022) A new nearest neighbor-based framework for diabetes detection. Expert Syst Appl 199:116857
Prasad BS, Gupta S, Borah N, Dineshkumar R, Lautre HK, Mouleswararao B (2023) Predicting diabetes with multivariate analysis an innovative KNN-based classifier approach. Prev Med 174:107619
Khanam JJ, Foo SY (2021) A comparison of machine learning algorithms for diabetes prediction. Ict Express 7(4):432–439
Hasan MK, Saeed RA, Alsuhibany SA, Abdel-Khalek S (2022) An empirical model to predict the diabetic positive using stacked ensemble approach. Front Public Health 9:792124
Okikiola FM, Adewale OS, Obe OO (2023) A diabetes prediction classifier model using naive bayes algorithm. Fudma J Sci 7 (1):253–260
Mondal S, Banik A, Roy S, Das J, Banerjee S and Navin H (2022) Random Forest Based Diabetic Prediction Model on Highly Unbalanced Dataset. In 2022 IEEE 2nd Mysore Sub Section International Conference (MysuruCon). IEEE 1-6
Gündoğdu S (2023) Efficient prediction of early-stage diabetes using XGBoost classifier with random forest feature selection technique. Multimed Tools Appl 82(22):34163–34181
Hassan MM, Mollick S, Yasmin F (2022) An unsupervised cluster-based feature grouping model for early diabetes detection. Healthc Analyt 2:100112
Alghamdi T (2023) Prediction of Diabetes Complications Using Computational Intelligence Techniques. Appl Sci 13(5):3030
Khafaga DS, Alharbi AH, Mohamed I, Hosny KM (2022) An integrated classification and association rule technique for early-stage diabetes risk prediction. Healthcare 10(10):2070
Madan P, Singh V, Chaudhari V, Albagory Y, Dumka A, Singh R, … AlGhamdi AS (2022) An optimization-based diabetes prediction model using CNN and Bi-directional LSTM in real-time environment. Appl Sci 12(8):3989
Srinivasu PN, Shafi J, Krishna TB, Sujatha CN, Praveen SP, Ijaz MF (2022) Using recurrent neural networks for predicting type-2 diabetes from genomic and tabular data. Diagnostics 12(12):3067
Kiruthiga G, Shakkeera L, Asha A, Dhiyanesh B, Saraswathi P, Murali M (2023) Deep Learning-Based Continuous Glucose Monitoring with Diabetic Prediction Using Deep Spectral Recurrent Neural Network. In: International Conference on Information, Communication and Computing Technology. Springer Nature Singapore, Singapore, pp 485–497
Bukhari MM, Alkhamees BF, Hussain S, Gumaei A, Assiri A, Ullah SS (2021) An improved artificial neural network model for effective diabetes prediction. Complexity 2021:1–10
Prakash EP, Srihari K, Karthik S, Kamal MV, Dileep P, Bharath Reddy S, Mukunthan MA, Somasundaram K, Jaikumar R, Gayathri N, Sahile K (2022) Implementation of artificial neural network to predict diabetes with high-quality health system. Comput Intell Neurosci 2022(1):1174173
Al Sadi K, Balachandran W (2023) Prediction Model of Type 2 Diabetes Mellitus for Oman Prediabetes Patients Using Artificial Neural Network and Six Machine Learning Classifiers. Appl Sci 13(4):2344
Alex SA, Jhanjhi NZ, Humayun M, Ibrahim AO, Abulfaraj AW (2022) Deep LSTM Model for Diabetes Prediction with Class Balancing by SMOTE. Electronics 11(17):2737
Prendin F, Pavan J, Cappon G, Del Favero S, Sparacino G, Facchinetti A (2023) The importance of interpreting machine learning models for blood glucose prediction in diabetes: an analysis using SHAP. Sci Rep 13(1):16865
Bani-Salameh H, Alkhatib SM, Abdalla M, Al-Hami MT, Banat R, Zyod H, Alkhatib AJ (2021) Prediction of diabetes and hypertension using multi-layer perceptron neural networks. Int J Model Simul Sci Comput 12(02):2150012
Sivasankari SS, Surendiran J, Yuvaraj N, Ramkumar M, Ravi CN and Vidhya RG (2022)Classification of diabetes using multilayer perceptron. In 2022 IEEE International Conference on Distributed Computing and Electrical Circuits and Electronics (ICDCECE). IEEE 1-5
Ali R, Hussain J, Lee SW (2023) Multilayer perceptron-based self-care early prediction of children with disabilities. Digital Health 9:20552076231184054
Bodapati JD (2022) Stacked convolutional auto-encoder representations with spatial attention for efficient diabetic retinopathy diagnosis. Multimed Tools Appl 81(22):32033–32056
Ismael HA, Al-A’araji NH, Shukur BK (2023) Enhanced the prediction approach of diabetes using an autoencoder with regularization and deep neural network. Period Eng Nat Sci 10(6):156–167
Rashmi K, Rao NK, Bala MM, Lahari M, Fathima N, Prudhvi V (2021) Prediction of diabetes mellitus using rbf neural model and genetic algorithm. Turkish Journal of Physiotherapy and Rehabilitation 32:3
Sivaraman M and Sumitha J (2023) An efficiency of DCKSVM and HRBFNN techniques for diabetic prediction. In AIP Conference Proceedings (Vol. 2831, No. 1). AIP Publishing
Zhang C, Hu C, Wu T, Zhu L, Liu X (2022) Achieving efficient and privacy-preserving neural network training and prediction in cloud environments. IEEE Trans Dependable Secure Comput 20(5):4245–4257
Zhang C, Zhu L, Xu C, Lu R (2018) PPDP: An efficient and privacy-preserving disease prediction scheme in cloud-based e-Healthcare system. Futur Gener Comput Syst 79:16–25
Lei D, Liang J, Zhang C, Liu X, He D, Zhu L, Guo S (2023) Publicly verifiable and secure SVM classification for cloud-based health monitoring services. IEEE Internet Things J
Mathew TE (2019) A comparative study of the performance of different Support Vector machine Kernels in Breast Cancer Diagnosis. Int J Inf Comput Sci 6(6):432–441
Podgorelec V, Kokol P, Stiglic B, Rozman I (2002) Decision trees: an overview and their use in medicine. J Med Syst 26:445–463
Dey L, Chakraborty S, Biswas A, Bose B and Tiwari S (2016) Sentiment analysis of review datasets using naive bayes and k-nn classifier. arXiv preprint arXiv:1610.09982
Khamis HS (2014) Application of k-Nearest Neighbour classification in medical data mining in the context of kenya. In Scientific Conference Proceedings 2022(1):5416722
Breiman L (2001) Random forests. Mach Learn 45:5–32
Cutler DR, Edwards TC Jr, Beard KH, Cutler A, Hess KT, Gibson J, Lawler JJ (2007) Random forests for classification in ecology. Ecology 88(11):2783–2792
Montgomery DC, Peck EA, Vining GG (2021) Introduction to linear regression analysis. John Wiley & Sons
Nathiya G, Punitha SC, Punithavalli M (2010) An analytical study on behavior of clusters using k means, em and k* means algorithm. arXiv preprint arXiv:1004.1743.
Agrawal R, Imieliński T, Swami A (1993) Mining association rules between sets of items in large databases. In Proceedings of the 1993 ACM SIGMOD international conference on Management of data. pp 207-216
Zhu X, Ghahramani Z, Lafferty JD (2003) Semi-supervised learning using gaussian fields and harmonic functions. In Proceedings of the 20th International conference on Machine learning (ICML-03). pp 912-919
Mnih V, Badia AP, Mirza M, Graves A, Lillicrap T, Harley T, … Kavukcuoglu K (2016) Asynchronous methods for deep reinforcement learning. In International conference on machine learning. PMLR. pp 1928-1937
Eiben AE, Smith JE (2015) Introduction to evolutionary computing. Springer-Verlag, Berlin Heidelberg
He K, Zhang X, Ren S, Sun J (2016) Deep residual learning for image recognition. In Proceedings of the IEEE conference on computer vision and pattern recognition. pp 770-778
Madhiarasan M, Louzazni M (2022) Analysis of artificial neural network: architecture, types, and forecasting applications. J Electr Comput Eng 2022(1):5416722
Alzubaidi L, Zhang J, Humaidi AJ, Al-Dujaili A, Duan Y, Al-Shamma O, … Farhan L (2021) Review of deep learning: Concepts, CNN architectures, challenges, applications, future directions. J Big Data 8:1–74
Sherstinsky A (2020) Fundamentals of recurrent neural network (RNN) and long short-term memory (LSTM) network. Phys D: Nonlinear Phenom 404:132306
Download references
Author information
Authors and affiliations.
Department of Computer Science & Engineering, Sarala Birla University, Ranchi, Jharkhand, India
Sandip Kumar Singh Modak
Department of Computer Science & Engineering, Birla Institute of Technology, Mesra, Ranchi, India
Vijay Kumar Jha
You can also search for this author in PubMed Google Scholar
Corresponding author
Correspondence to Sandip Kumar Singh Modak .
Ethics declarations
Conflict of interest.
There is no conflict of interest in the current research.
Additional information
Publisher’s note.
Springer Nature remains neutral with regard to jurisdictional claims in published maps and institutional affiliations.
Rights and permissions
Springer Nature or its licensor (e.g. a society or other partner) holds exclusive rights to this article under a publishing agreement with the author(s) or other rightsholder(s); author self-archiving of the accepted manuscript version of this article is solely governed by the terms of such publishing agreement and applicable law.
Reprints and permissions
About this article
Modak, S.K.S., Jha, V.K. Machine and deep learning techniques for the prediction of diabetics: a review. Multimed Tools Appl (2024). https://doi.org/10.1007/s11042-024-19766-9
Download citation
Received : 25 October 2023
Revised : 27 February 2024
Accepted : 20 June 2024
Published : 16 July 2024
DOI : https://doi.org/10.1007/s11042-024-19766-9
Share this article
Anyone you share the following link with will be able to read this content:
Sorry, a shareable link is not currently available for this article.
Provided by the Springer Nature SharedIt content-sharing initiative
- Data Mining
- Machine learning
- Deep learning
- Find a journal
- Publish with us
- Track your research
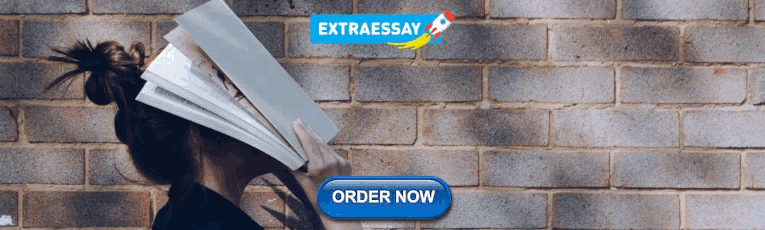
IMAGES
VIDEO
COMMENTS
Electronic voting, based on cryptographic protocols and blockchain technology, represents an advanced method for ensuring the security, transparency, and reliability of electoral processes. The scientific research presented in this article is devoted to the analysis of trends and advanced technologies in the field of electronic voting. The authors propose a systematic mapping of this area ...
Cauchan S, Jaiswal M, Kar AK (2018) The acceptance of electronic voting machines in India: A UTAUT approach. Electronic Government, an International Journal 14(3): 255-275. Crossref. Google Scholar. Charles A (2009) The electronic state: Estonia's new media revolution. ... Snyder H (2019) Literature review as a research methodology: An ...
Electronic Voting Literature Review Computer scientists who have done work in, or are interested in, electronic voting all seem to agree on two things: Internet voting does not meet the requirements for public elections ... voting machines and their makers who insist that we should fijust trust [them].fl ...
Electronic voting (e-voting) is the use of electronic systems and technologies in elections to cast and count votes. There are several types of electronic voting systems, including Direct Recording Electronic (DRE) systems, Optical Scan Systems, Internet Voting, and...
Electronic Voting Literature Review National Academies of Sciences, Engineering, and Medicine,Division on Engineering and ... Until now. The electronic voting machines being used in 37 states are vulnerable to tampering, and because the manufacturers are not required to reveal—even to the government—how they operate, voters
I-voting systems are called in the literature mobile voting, remote electronic voting, or online voting systems in which ballots are transmitted and recorded over the Internet. The blockchain-based electronic voting system is a type of i-voting, which is based on the Internet and uses a network that uses blockchain to vote and count votes in a ...
The employment of blockchain technology in electronic voting (e-voting) systems is attracting significant attention due to its ability to enhance transparency, security, and integrity in digital voting. This study presents an extensive review of the existing research on e-voting systems that rely on blockchain technology. The study investigates a range of key research concerns, including the ...
However, electronic voting (e-voting) machines are still not widely accepted. This paper analyzes research papers published on electronic voting in the Scopus database between 2000 and 2022. ... Ikhsan Darmawan. 2021. E-voting adoption in many countries: A literature review. Asian Journal of Comparative Politics, 6(4), 482-504. https://doi.org ...
This article presents a study on the evolution of research in electronic voting systems, following a systematic literature review methodology and a chronological evolution from the first systems that employed public cryptographic concepts up to blockchain-based proposals, with the objective of detailing the evolution of the technology as a ...
for remote electronic voting? First, observe that electronic banking is not perfectly secure: most electronic banking and e-commerce systems suffer a significant rate of fraud, despite the opportunity to verify the process. Furthermore, voting is harder. The key issue that is unique to elec-tronic voting is the interaction between those ...
The literature review for this field of study and other related experiments may be seen as a good path for making voting more efficient in terms of administration and participation. However, the idea of using blockchain offered a new model for electronic voting. ... Many security flaws still exist in the internet and polling machines ...
Electronic voting machine (EVM) is fundamental electronic devices used to record projects a polling form as opposed to votes papers and boxes as of late used in the standard way projecting a voting form system. ... A Review of Smart Electronic Voting Machine. In: Poonia, R.C., Singh, V., Singh Jat, D., Diván, M.J., Khan, M.S. (eds) Proceedings ...
Literature review: Systems for electronic voting (or "e-voting") have drawn interest as a way to improve and modernize the political process. In recent literature, several aspects of artificial intelligence's potential and difficulties have been discussed in relation to the integration of distant e-voting machines.
Electronic Voting Machine (EVM) is a simple electronic device used to record votes in place of ballot papers and boxes which were used earlier in conventional voting system.
as in electronic poll-site voting. Electronic voting refers to the use of computers or computerized voting equipment to cast ballots in an election. Sometimes, this term is used more specifically to refer to voting that takes place over the Internet. Electronic systems can be used to register voters, tally ballots, and record votes [2].
This project work on e-voting system is made up of five chapters: introduction, literature review, methodology and system design, systems implementation and result analysis, conclusion and ...
The Roadmap to the Electronic Voting System Development: A Literature Review M. Mesbahuddin Sarker, Tajim Md. Niamat Ullah Akhund Jahangirnagar University, Dhaka Bangladesh Abstract— Since the start of the use of the electronic voting system, it has gone through numerous updates and upgrades. These upgrades and updates include changes
LITERATURE REVIEW Raspberry Pi and image processing based on Electronic Voting Machine (EVM) [1], provides a small computer capable of image processing and controls the entire voting system. A photo of the national ID card of citizens is taken with the help of a camera which indicates a valid voter of ...
Electronic Voting Machine (EVM) is a simple electronic device used to record votes in place of ballot papers and boxes which were used earlier in conventional voting system. Fundamental right to vote or simply voting in elections forms the basis of democracy. All earlier elections be it state elections or centre elections a voter used to cast his/her favorite candidate by putting the stamp ...
In this paper, we present a Novel Electronic Voting System which is based on biometric authentication and distributed servers approach which provide high security for voting process. Whole voting system is divided into two parts one is a voting machine and another is server system. Raspberry pi 3 model B is a heart of the voting machine.
Debojyoti Ghosh [9] conducted a thorough review of fingerprint-based electronic voting machines (EVMs), with a primary focus on enhancing voting security. The comprehensive literature survey explored various aspects, including biometrics, integration with the Aadhar database, GSM alert systems, and online e-voting.
WASHINGTON (CN) — A former Colorado election official asked the Supreme Court on Friday for emergency intervention to prevent the start of her trial over leaked voting machine passwords. Tina Peters, who served as Mesa County's clerk and recorder during the 2020 election cycle, faces criminal charges for giving a security company ...
CrowdStrike produces a suite of security software products for businesses, designed to protect computers from cyberattacks.The Falcon Sensor product, CrowdStrike's vulnerability scanner, installs an endpoint sensor at the operating system kernel level on individual computers to detect and prevent threats. Patches are routinely distributed by CrowdStrike to its clients to enable their computers ...
The emergence of artificial intelligence (AI) and, more particularly, machine learning (ML), has had a significant impact on engineering and the fundamental sciences, resulting in advances in various fields. The use of ML has significantly enhanced data processing and analysis, eliciting the development of new and Journal of Materials Chemistry A Recent Review Articles
Reports produced by popular malware analysis services showed a disparity in samples available for different malware families. The unequal distribution between such classes can be attributed to several factors, such as technological advances and the application domain that seeks to infect a computer virus. Recent studies have demonstrated the effectiveness of deep learning (DL) algorithms when ...
Diabetes has become one of the significant reasons for public sickness and death in worldwide. By 2019, diabetes had affected more than 463 million people worldwide. According to the International Diabetes Federation report, this figure is expected to rise to more than 700 million in 2040, so early screening and diagnosis of diabetes patients have great significance in detecting and treating ...