
An official website of the United States government
The .gov means it’s official. Federal government websites often end in .gov or .mil. Before sharing sensitive information, make sure you’re on a federal government site.
The site is secure. The https:// ensures that you are connecting to the official website and that any information you provide is encrypted and transmitted securely.
- Publications
- Account settings
Preview improvements coming to the PMC website in October 2024. Learn More or Try it out now .
- Advanced Search
- Journal List
- Genes (Basel)

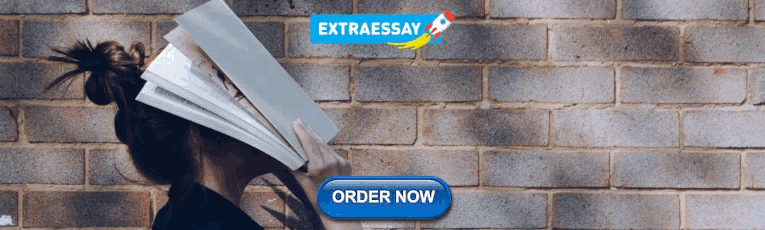
Genetic Research and Plant Breeding
Kwon-kyoo kang.
1 Division of Horticultural Biotechnology, Hankyong National University, Anseong 17579, Republic of Korea
Yong-Gu Cho
2 Department of Crop Science, Chungbuk National University, Cheongju 28644, Republic of Korea
In the past 20 years, plant genetics and breeding research using molecular biology has been greatly improved via the functional analysis of genes, species identification and transformation techniques [ 1 ]. At the same time, innovations in molecular technology have spawned a new discipline called genomics [ 2 ]. The most epochal change among them is the development of next-generation sequencing (NGS) technology, which spurred research on the composition of regulatory regions and biological functions of many genes as the entire genome was deciphered with a focus on model plants. Functional genomics has become one of the most promising scientific areas in the characterization of gene (and protein) functions and interactions using vast genetics and various omics data [ 2 ]. In addition, there are various advanced tools and methodologies that could also enable the effective and efficient validation of gene functions when used in lab settings such as transcriptome, metabolomics, overexpression, knock-out, RNAi and gene editing, and when applying advanced computational methodologies such as machine learning and deep learning [ 2 , 3 ]. Farmers of the future can identify genetic differences between individual plants in a crop to make breeding-related inferences based on genomic information. The function of individual genes or DNA fragments can be inferred through links between genetic differences and emergence variations in various plants [ 1 , 4 ]. Genetic research also helps to characterize plants based on gene networks rather than individual genes. This allows plant breeding to understand and adapt to complex traits such as yield and tolerance to biotic and abiotic stresses. Therefore, to provide more information about gene function, scientists and farmers need to examine the genome, transcriptome, proteome and metabolites based on genome sequence data [ 1 , 5 ]. Based on these results, not only traditional breeding but also new and innovative breeding methods such as genome editing can be used to regenerate new plants for the future. Therefore, this Special Issue includes an agronomic characterization and gene function analysis using both molecular and computational biology in the laboratory to accelerate plant breeding programs. This topic includes a total of 25 articles: 22 research articles and 3 review articles. The articles in this topic focus on a variety of new tools and technologies to developing knowledge and resources for breeding yielding, climate-tolerant crop varieties.
1. Abiotic Stress Tolerance
An important factor in crop production is abiotic stress. Among abiotic stresses, heat and salt stress are regarded as the most critical factors affecting yield in most crops. this Special Issue includes two articles on abiotic stress tolerance research. In the first article, Jung et al. [ 6 ] found a resistance to heat stress in rice transformants overexpressing the OsOr or OsOr-R115H gene (Arg replaced by His at position 115 of the OsOr protein) and discussed their resistance mechanism. From these results, it was suggested that the OsOr-R115H gene can be used to develop heat-resistant rice varieties. In another article, Lim et al. [ 7 ] generated transgenic rice plants with single-copy/intergenic/homozygous overexpression PsGAPDH ( PsGAPDH -OX) and investigated their responses to salinity stress. To elucidate the role of PsGAPDH -OX in the salt-stress tolerance of rice, an Illumina HiSeq 2000 platform was used to analyze transcriptome profiles of leaves under salt stress. The analysis results of sequencing data show that 1124 transcripts were differentially expressed. Using the list of differentially expressed genes (DEGs), functional enrichment analyses of DEGs, such as GO (Gene Ontology) terms and KEGG (Kyoto Encyclopedia of Genes and Genomes) pathways, were performed. KEGG pathway enrichment analysis revealed that unigenes exhibiting differential expressions were involved in starch and sucrose metabolism. Interestingly, Trehalose-6-phosphate synthase ( TPS ) genes, whose expression was enhanced by abiotic stress, showed a significant difference in PsGAPDH -OX.
2. Biotic Stress Resistance
In addition, tolerance to biotic stress is one of the most important factors of crop production. This Special Issue contains three articles on biotic stress tolerance research. In the first article, Vanlay et al. [ 8 ] generated a total of 96 lines of interspecific and intraspecies hybrid rootstocks to improve tomato soil-borne disease resistance. The results show that the new hybrid combination improves horticultural traits and soil-borne disease resistance more than the commercial rootstock, Maxifort. Additionally, gray mold disease caused by Botrytis in onions ( Allium cepa L.) during growth and storage negatively affects their yield and quality. In the second article, Lee et al. [ 9 ] searched for genes associated with gray mold resistance via a transcriptome analysis in onions. As a result, the resistant lines showed differences in expression patterns compared to the susceptible lines in jasmonate resistance 1 (JAR1), coronatine-insensitive protein 1 (COI 1), and transcription factor MYC2 (MYC2) genes. Additionally, Sclerotinia stem rot is one of the utmost important diseases in mustard, causing considerable losses in seed yield and oil quality. In the third article, Singh et al. [ 10 ] investigated the genetic pattern of Sclerotinia stem rot resistance in Indian mustard using six generations (P 1 , P 2 , F 1 , F 2 , BC 1 P 1 , and BC 1 P 2 ), developed from the crossing of one resistant (RH 1222-28) and two susceptible genotypes (EC 766300 and EC 766123). Genetic analysis revealed that resistance was governed by duplicate epistasis. The comparative proteome analysis of resistant and susceptible genotypes indicated that peptidyl-prolyl cis-trans isomerase (A0A078IDN6 PPIase) showed high expression in resistant genotypes at the early infection stage, while its expression was delayed in susceptible genotypes.
3. Genetic Research on Gene Function
The genetic analysis of various genes of interest provides scientists with important information on their functions and gives useful insights to plant breeders for their breeding programs. Jeon et al. [ 11 ] performed the identification and characterization of PTE-2 , a Stowaway -like MITE activated in transgenic Chinese cabbage lines. Transposable elements (TEs) such as PTE-2 make up a high proportion of the plant genome and contribute to genetic diversity and evolution, affecting genome structure or gene activity. Additionally, Kim et al. [ 12 ] identified and verified the genetic variation in transgenic cabbage ( Brassica rapa ssp. pekinensis) by next-generation sequencing. The variation candidates that were expected to consistently occur in the transgenic lines were selected and validated. The single-nucleotide polymorphism (SNP) and insertion and deletion (InDel) candidates were identified using the resequencing data and validated by reverse transcription (RT)-PCR analysis. Xie et al. [ 13 ] analyzed of SI-related BoGAPDH family genes in Brassica oleracea L. and the response of BoGAPC to SI signals. A total of 16 members of the BoGAPDH family were identified in B. oleracea , which were conserved, distributed unevenly on chromosomes and had tandem repeat genes. Most of the genes were down-regulated during self-pollination, and the highest expression was found in stigmas and sepals. Additionally, different transcriptome data show that BoGAPDH genes were differentially expressed under stress, which was consistent with the results of qRT-PCR. The results of yeast two-hybrid and GST pull-down studies show that the SRK kinase domain interacts with the BoGAPC protein. The above results suggest that the BoGAPDH family of B. oleracea plays an important role in the process of plant stress resistance, and the BoGAPC gene may be involved in the process of self-incompatibility in B. oleracea , which may respond to an SI by directly encoding proteins interacting with SRK. In addition, plant tissue culture is an in vitro technique used to manipulate cells, tissues, or organs, and plays an important role in genetic transformation. However, plants cultured in vitro often exhibit unintended genetic and epigenetic variations. Park et al. [ 14 ] investigated DNA methylation level changes in transgenic Chinese cabbage plants ( B. rapa ssp. pekinensis ) and their effects on corresponding gene expression patterns. Differentially methylated regions (DMRs) of DNA between non-transgenic and transgenic lines were detected by bisulfite sequencing, and ten DMRs located in exonic regions were identified. The regions with methylation variations that were inherited and consistently maintained in the next-generation lines were selected and validated. Additionally, fuzzless mutants are ideal materials to decipher the regulatory network and mechanism underlying fuzz initiation and formation. Feng et al. [ 15 ] used two Gossypium arboretum accessions differing in fuzz characteristics to explore expression pattern differences and differentiate between genes involved in fuzz development using RNA sequencing. Weighted gene co-expression network analysis discerned the ME magenta module highly associated with a fuzz/fuzzless trait, which included a total of 50 hub genes differentially expressed between two materials. This module and hub genes identified will provide new insights on fiber and fuzz formation and be useful for the molecular design breeding of cotton genetic improvement. Additionally, decapitation is an essential agricultural practice and is a typical method for analyzing shoot branching. However, it is unclear exactly how decapitation controls branching. Dong et al. [ 16 ] reported that the decapitation of sunflower plants led to the development of lateral buds, accompanied by a decrease in indole-3-acetic acid (IAA) and abscisic acid (ABA) levels and an increase in cytokinin (CK) levels. Additionally, 82 members of the HabZIP family were discovered and categorized into nine groups using phylogenetic and conservative domain analyses. Based on tissue-specific expression and expression analysis following decapitation derived from the transcriptome, several HabZIP members may be involved in controlling decapitation-induced bud outgrowth. Therefore, it is hypothesized that the dynamic variations in hormone levels, in conjunction with particular HabZIP genes, led to the development of axillary buds in sunflowers following decapitation. In addition, the LBD (lateral organ boundaries domain) family are a new group of plant-specific genes, which encode a class of transcription factors containing conserved lateral organization boundary (LOB) domains, and play an important role in regulating the adaxial–abaxial polarity of plant leaves. Lin et al. [ 17 ] isolated the BcAS2 gene from the pak choi cultivar “NHCC001”, and analyzed its expression pattern. The results show that the BcAS2 encoded a protein made up of 202 amino acid residues located in the nucleus and cytomembrane. The yeast two-hybrid system (Y2H) assay indicates that BcAS2 interacts with BcAS1-1 and BcAS1-2 (the homologous genes of AS1 gene in pak choi). In the transgenic Arabidopsis thaliana that overexpresses the BcAS2 gene, it presented an abnormal phenotype with a curly shape. Taken together, our findings not only validate the function of BcAS2 in leaf development in A. thaliana , but also contribute to unravelling the molecular regulatory mechanism of BcAS2 , which fulfills a special role by forming complexes with BcAS1-1/2 in the establishment of the adaxial–abaxial polarity of the lateral organs in pak choi. Additionally, tetraploid plants often have altered rates of vegetative growth relative to their diploid progenitors. However, the molecular basis for altered growth rates remains a mystery. Xu et al. [ 18 ] reports microRNA (miRNA) and gene expression differences in Populus tetraploids and counterpart diploids using RNA and miRNA sequencing. The results show that there was no significant difference between young leaves in the expression of vegetative growth-related miRNAs. However, as the leaves aged, the expression of auxin- and gibberellin-related miRNAs was significantly upregulated, while the expression of senescence-related miRNAs was significantly downregulated. As a result, the chloroplast degradation of tetraploid leaves was accelerated, the photosynthetic rate decreased, and the synthesis and decomposition ability of carbohydrate decreased. Additionally, root network structure plays a crucial role in growth and development processes in rice. Longer, more branched root structures help plants to assimilate water and nutrition from soil, support robust plant growth, and improve resilience to stresses, such as disease. Understanding the molecular basis of root development through the screening of root-related traits in rice germplasms is critical to future rice breeding programs. Zhang et al. [ 19 ] used a small germplasm collection of 137 rice varieties chosen from the Korean rice core set (KRICE_CORE) to identify loci linked to root development.
4. Molecular Breeding Based on SNP Markers
The development of an efficient, robust and high-throughput SNP genotyping platform is greatly significant as it plays a pivotal role in crop genetics and breeding. Lee et al. [ 20 ] studied the development and applications of a target capture-sequencing SNP-genotyping platform in rice. This platform was used in the diversity analysis of 50 rice varieties. The 2341 of 2565 SNP markers produced useful polymorphic genotype data, enabling the production of a phylogenetic tree of the 50 varieties. The platform was used for the QTL mapping of PHS (preharvest sprouting) resistance in an F 8 recombinant inbred line population derived from the cross Odae × Joun. This strategy is a powerful tool for breeding and rice genetics and facilitates gene mapping and QTL studies, germplasm diversity analysis, and marker-assisted selection. Additionally, NGS (next-generation sequencing technologies) have enabled the discovery of a lot of sequence variations among closely related crop varieties. Ji et al. [ 21 ] used a set of resequencing data from 24 Korean japonica rice varieties in a temperate region and discovered 954,233 sequence variations, including 791,121 SNPs (single nucleotide polymorphisms) and 163,112 InDels (insertions/deletions). The SNP variants were classified into four groups according to their impact: high, moderate, low, and modifier. These groups contain 3524 (0.4%), 27,656 (2.9%), 24,875 (2.6%), and 898,178 (94.1%) variants, respectively. These results are useful for developing new markers for MAS (marker-assisted selection), selecting candidate genes in map-based cloning, and producing efficient high-throughput genome-wide genotyping systems for Korean temperate japonica rice varieties. In addition, Kim et al. [ 22 ] measured the thickness of seed coat and aleurone layers using a set of 294 rice varieties. They also found candidate genes for selecting the thickness of seed coat and aleurone layers by performing GWAS analyses using whole-genome resequencing data. Two primer pairs that can be used as HRM (high-resolution melting) markers were developed using HRM markers and the genotyping of BC 2 F 2 individuals derived from a cross between Samgwang and Seolgaeng. These authors yielded reliable results to finally develop HRM markers for selecting seed coat and aleurone layer thickness. Additionally, molecular breeding that involves marker-assisted selection (MAS) addresses the limitations of conventional breeding and allows the pyramiding of multiple useful genes into a single cultivar. The utilization of DNA markers in a marker-assisted backcrossing (MABc) program significantly increases selection efficiency. Kim et al. [ 22 ] attempted to develop new breeding lines with a thinner seed coat and aleurone layer to provide a high eating quality with softer chewing characteristics and processability in rice grain. Line selection according to genotype of KASP markers was successful in the BC 1 F 1 and BC 2 F 1 generations, with the recurrent parent genome recovery ratio ranging from 91.22% to 98.65%. In BC 2 F 1 seeds of the selected lines, the thickness of the aleurone layer was found to range from 13.82 to 21.67 μm, which is much thinner than the 30.91 μm of the wild type, suggesting that selection by MABc could be used as an additional breeding material for the development of highly processed rice varieties. Additionally, Scariolo et al. [ 23 ] performed an in-depth characterization of 15 varietal clones belonging to two distinct Lavandula species by means of a restriction-site-associated DNA sequencing (RAD-Seq). These authors demonstrated that this technology screens single-nucleotide variants that can access the genetic identity of individual accessions, reconstruct genetic relationships among related breeding lines, group them into genetically distinguishable main subclusters, and assign their molecular lineages to distinct ancestors. Overall, the results highlight the presence of pure ancestries and interspecific hybrids for the analyzed Lavandula species and demonstrate that RAD-Seq analysis is very informative and highly reliable for characterizing Lavandula clones and managing plant variety protection. The review article by Yu et al. [ 24 ] addresses how breeders persistently supply farmers with the best varieties in order to exceed consumer demand through resource-intensive plant-breeding processes. In order to motivate continuous innovation in variety development, a system needs to provide incentives for plant breeders to develop superior varieties, for example, exclusive ownership to produce and market those varieties. The most common system is the acquisition of intellectual property protection through plant variety protection, also known as breeder’s right. Most countries have adopted the system established by the International Union for the Protection of New Varieties of Plants (UPOV). To be granted plant variety protection, the variety should prove to be unique by meeting three requirements: distinctness, uniformity, and stability.
5. Genetic Research on Plant Development
An important review on the interspecific hybridization of transgenic B. napus and B. rapa was compiled by Sohn et al. [ 25 ] The authors also found that intraspecific hybridization among the Brassica species is very common, especially between B. napus and B. rapa . In general, interspecific hybrids cause numerous genetic and phenotypic changes in parental lines. Consequently, their fitness and reproductive ability are also very diverse. Additionally, the plant leaf, the main organ of photosynthesis, is an important regulator of growth. Zhang et al. [ 26 ] investigated leaf length, width, thickness, area, leaf mass per area (LMA), and cell size from two genotypes and profiled the transcriptome-wide gene expression patterns through RNA sequencing. The results show that the leaf area of Pd was significantly larger than that of Ps, but the epidermal cell area was significantly smaller than that of Ps. The difference in leaf size was caused by cell numbers. A transcriptome analysis also revealed that genes related to chromosome replication and DNA repair were highly expressed in Pd, while genes such as the EXPANSIN ( EXPA ) family, which promoted cell expansion, were highly expressed in Ps. Therefore, these data provide a valuable resource for understanding leaf development in the Populus genus. Additionally, seed coat color is a crucial agronomic trait in sesame ( Sesamum indicum L.) since it is strongly linked to seed oil, proteins, and lignans contents and influences consumer preferences. In East Asia, black sesame seeds are used for the treatment and prevention of various diseases. However, in sesame, little is known about the establishment of the seed coat color, and only one gene has been reported to control black pigmentation. Wang et al. [ 27 ] provide an overview of developing seeds transcriptome of two varieties of sesame “Zhongfengzhi No.1” (white seed) and “Zhongzhi No.33” (black seed) and shed light on genes involved in black seed formation. Litvinov et al. [ 28 ] found that the development of new, more productive varieties of agricultural crops is becoming an increasingly difficult task. Modern approaches to the identification of beneficial alleles and their use in elite cultivars, such as quantitative trait loci (QTL) mapping and marker-assisted selection (MAS), are effective but insufficient for keeping pace with the improvement of wheat or other crops. Metabolomics is a powerful but underutilized approach that can assist with crop breeding. The article summarizes basic methodological information, and the current strategies of applications of metabolomics related to crop breeding are explored using recent examples.
In summary, this Special Issue comprises a diverse collection of both original research and review articles. These articles generated a large amount of genetic and genomic resources in different crops, including rice, sesame, Chinese cabbage, B. oleracea, sunflower, tomato, onion, Indian mustard and poplar, etc. The articles in this topic reported the application of many modern genomic tools for crop improvement, such as transgenic approach, GWAS, high-density marker platforms, genotype by sequencing, marker-assisted and genomic selection, single-molecule long-read sequencing technology, transcriptome analyses and candidate gene analyses, etc. We believe that the resources and knowledge generated through articles published in this Special Issue will help to stimulate crop improvements, thus playing an important role in increasing crop production for future food security.
Acknowledgments
This research was carried out with the support of ‘Basic Science Research Program through the National Research Foundation of Korea (NRF) funded by the Ministry of Education (2021R1I1A4A01057295) and (2022R1I1A3071999), Republic of Korea.
Funding Statement
This research was supported by Chungbuk National University Korea National University Development Project (2022), Republic of Korea.
Author Contributions
Writing—original draft, K.-K.K.; writing—review and editing, K.-K.K., Y.-G.C. All authors have read and agreed to the published version of the manuscript.
Conflicts of Interest
The authors declare no conflict of interest.
Disclaimer/Publisher’s Note: The statements, opinions and data contained in all publications are solely those of the individual author(s) and contributor(s) and not of MDPI and/or the editor(s). MDPI and/or the editor(s) disclaim responsibility for any injury to people or property resulting from any ideas, methods, instructions or products referred to in the content.
REVIEW article
Digitalizing breeding in plants: a new trend of next-generation breeding based on genomic prediction.
- 1 Plant Computational Genomics Laboratory, Department of Science in Smart Agriculture Systems, Chungnam National University, Daejeon, Republic of Korea
- 2 Plant Computational Genomics Laboratory, Department of Crop Science, Chungnam National University, Daejeon, Republic of Korea
- 3 Genomics Division, National Institute of Agricultural Sciences, Jeonju, Republic of Korea
As the world’s population grows and food needs diversification, the demand for cereals and horticultural crops with beneficial traits increases. In order to meet a variety of demands, suitable cultivars and innovative breeding methods need to be developed. Breeding methods have changed over time following the advance of genetics. With the advent of new sequencing technology in the early 21st century, predictive breeding, such as genomic selection (GS), emerged when large-scale genomic information became available. GS shows good predictive ability for the selection of individuals with traits of interest even for quantitative traits by using various types of the whole genome-scanning markers, breaking away from the limitations of marker-assisted selection (MAS). In the current review, we briefly describe the history of breeding techniques, each breeding method, various statistical models applied to GS and methods to increase the GS efficiency. Consequently, we intend to propose and define the term digital breeding through this review article. Digital breeding is to develop a predictive breeding methods such as GS at a higher level, aiming to minimize human intervention by automatically proceeding breeding design, propagating breeding populations, and to make selections in consideration of various environments, climates, and topography during the breeding process. We also classified the phases of digital breeding based on the technologies and methods applied to each phase. This review paper will provide an understanding and a direction for the final evolution of plant breeding in the future.
1. Introduction
By 2050, the world’s population is expected to reach 9.6 billion, which requires large agricultural production. Consequently, it will be necessary to increase agricultural production by more than 70% ( Godfray, 2014 ). In addition to direct causes, such as population growth, the indirect causes include the appearance of rapidly developing countries leading to urbanization and modernization and their population’s diet shift towards dairy and meat products. The increased demand for animal-based diets promotes higher cereal crop consumption than a vegetarian diet due to feeding cereal crops to animals. Therefore, without changes in crop production, unequal food distribution among the world’s population will deepen ( Bradshaw, 2017 ). Moreover, the world’s climate is changing rapidly due to global warming. The average temperature of the world rises every year and abnormal climate phenomena occur ( Zandalinas et al., 2021 ).. High temperatures and unpredictable precipitation patterns create challenges for crop growth. Therefore, a strategy to increase agricultural production in preparation for climate change is essential. Considerable progress has been achieved in producing crops tolerant to biotic and abiotic stress. Moreover, several attempts to increase the economically beneficial traits of crops in the 20th century were successful ( Crossa et al., 2017 ). However, despite these improvements, the recent increase in yields of major crops is not enough to meet the expectations of future agricultural production demands. In order to increase agricultural production, it is essential to develop new cultivars that are resilient to climate change and have increased yields. Moreover, economic growth in many countries increased the demand for horticultural crops. As a result, horticultural crops, particularly fruits and vegetables, which are indispensable to us, increase our interest in improving our health and quality of life. Therefore, agricultural researchers worldwide must use various breeding methods to improve varieties, increase production of all crops and horticultural crops, and develop new breeding techniques and varieties using new technologies to meet consumers’ requirements.
In the second half of the 20th century, a backcrossing breeding method was commercialized. Marker-assisted backcrossing (MABC) allows rapid introgression of key genes representing superior traits into elite cultivars or breeding lines, resulting in a cultivar containing both the transgene and the preferred alleles ( Ragot et al., 1995 ). MAS genetically enhances useful traits in crops that are difficult to phenotype. In addition, the fixation of the transgene in commercial cultivars can proceed rapidly ( Moose and Mumm, 2008 ). However, MAS is effective for a few quantitative trait loci (QTLs) with a significant effect on the trait but not for traits dominated by numerous QTLs with minor effects. Therefore, researchers try to solve these constraints. As a result, GS emerged as an alternative. GS estimates the value of breeding based on markers distributed throughout the genome and does not use just a few markers like traditional MAS ( Meuwissen et al., 2001 ). While MAS completely depends on molecular markers associated with target traits, GS resembles conventional breeding methods that depend on phenotypes and the breeder’s selection ability. In conventional breeding, breeders select plant individuals based on their preferences and experience. On the contrary, MAS objectively selects plant individuals with molecular markers. In the GS procedures, the genetic or breeding population is mechanically trained based on statistical models (training population). Then, the model is applied to the breeding population for selection (breeding population or validation population). Provided that training is the process of breeders gaining experience, the application of the model may be the process of breeder’s selection. Consequently, GS can be more reasonable for breeding complex traits to which a number of minor genes are related.
Humans have bred crops to introgress useful traits and increase yields. Breeding methods have changed from domestication to traditional phenotypic selection, molecular breeding, and phenotype prediction ( Razzaq et al., 2021 ). The recently proposed GS in plants is more difficult to predict the effect of environmental variables than animals and most of the processes are still labor-intensive. So, it is difficult to apply GS to actual breeding selection yet. In order to solve this problem, several studies have conducted GS using ML technology. Some agricultural scientists call these attempts digital breeding to distinguish them from previous GS. However, digital breeding is still in its infancy and lacks a precise definition, causing much confusion in communication among researchers. In order to solve these controversies, we would like to define a new term digital breeding. Digital breeding means that breeding design, experimental plot arrangement, growth process, and selection are all carried out automatically in the breeding process while predicting and considering various environmental variables. Digital breeding aims to automate the breeding process of plants by minimizing human intervention. Recently, biological data has been digitized and increased. In the same way, breeding technologies adopt some cutting-edge sciences such as next-generation sequencing, machine/deep learning, speed breeding, and advanced statistical models, enabling predictive breeding. This article surveys breeding technologies to date, classifies the concept, application, and research status of digital breeding, and attempts to provide clarification by borrowing the idea of the levels of driving automation. In this review article, the past, present, and future of breeding is divided into six stages according to the level of technological development. It will be helpful to understand breeding trends so far and to present the direction and achievements of breeding in the future.
2. Outline of breeding techniques
2.1. traditional breeding techniques.
Plant breeding developed through efforts to establish better-performing varieties by crossing between cultivars with the traits desired by the breeder ( Acquaah, 2009 ). Breeders select phenotypes with desired traits, such as semi-dwarf and nutrient-efficient plants. Plant breeding aims to select, identify, and expand varieties with valuable traits desired by consumers and breeders in the next generations by targeting diversity through new variations. Therefore, breeding will fail if suitable individuals cannot be selected for the next generation. So for breeding success, breeders studied various breeding methods, considering the multiple characteristics: fertilization method, breeding ability, combination ability, breeding scale, and breeding age. In addition, the breeding method was determined through the genetic structure of the cultivars’ traits and the degree of interaction between genes ( Kearsey, 1997 ). Breeding methods considering these factors can be classified into segregation breeding and cross-breeding ( Table 1 ). Segregation breeding nurtures excellent individuals or groups while selecting outstanding individuals or lines when a genetically diverse group has already been secured. The pure line selection method and the mass selection method are representative. Cross-breeding is breeding cultivars with an excellent performance by recombining or introducing beneficial or desired genes through an artificial cross between cultivars or lines. Pedigree selection, bulk population, single-seed descent (SSD), and backcross breeding are representative methods in cross-breeding.
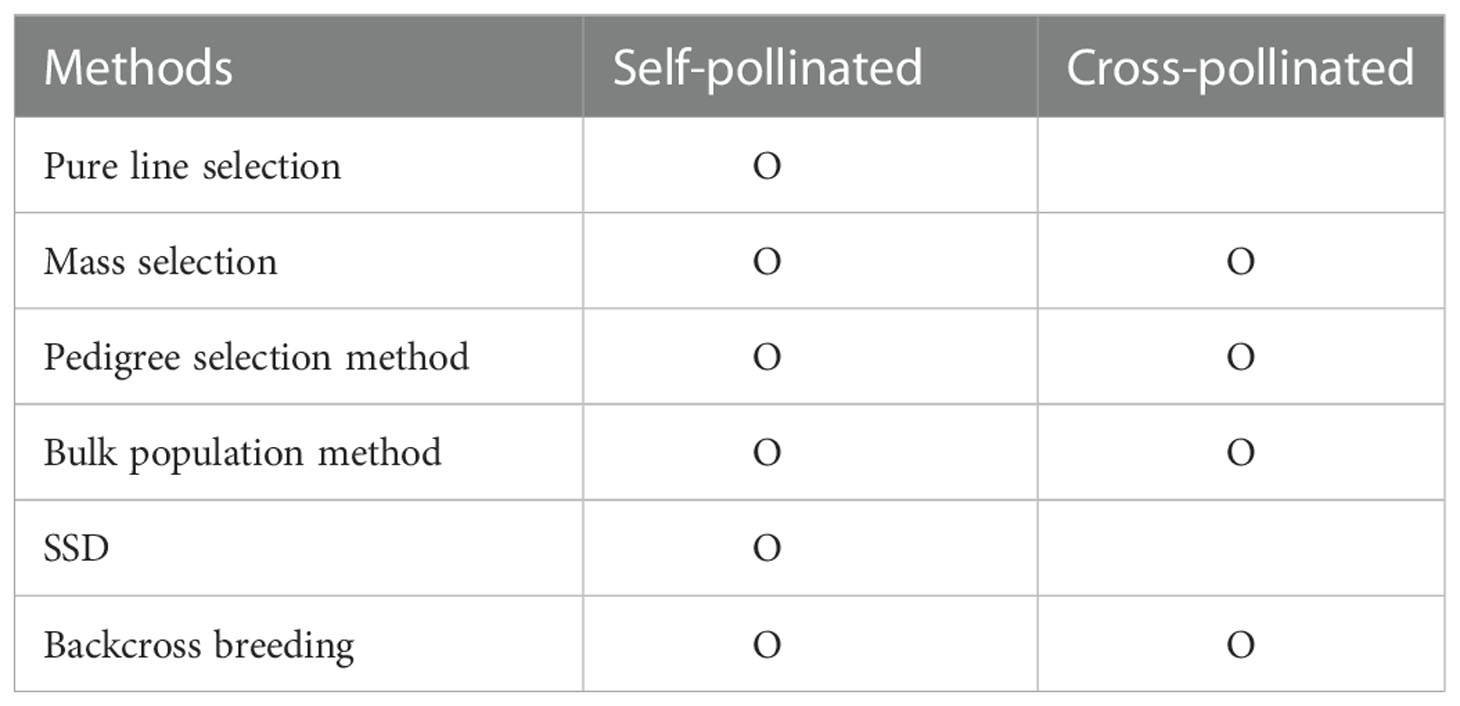
Table 1 Comparison of breeding methods according to pollination of plants.
2.1.1. Pure line selection
In 1903, Johannsen’s research confirmed that a population mixed with self-pollinated species could be classified as a genetically pure line. Pure lines are suitable for applications that require trait uniformity because of small genetic differences and similar phenotypes ( Acquaah, 2009 ). Furthermore, pure line selection is rapid, and genotype selection from a diverse population can continue to repeat selfing until there is no apparent segregation in subsequent generations ( Poehlman and Sleper, 1995 ). Based on these results, selection can eliminate variation but not create variations. Thus, the variations produced in the pure line are caused by environmental factors, meaning that selection in the pure line is meaningless. In addition, pure line cultivars are virtually challenging to produce in diverse environments due to their small genetic differences. Therefore, pure lines play an important role as a material for cross-breeding or generating genetic populations.
2.1.2. Mass selection
Mass selection applies to self-pollination and cross-pollination, but the genetic results differ ( Allard, 1960 ). The continuation of inbreeding changes the gene frequency of the population. It decreases the heterozygosity from one generation to the next. Still, there is little change in allele frequency in cross-pollination unless the allele associated with the desired trait is changed through selection ( Acquaah, 2009 ). This method is a population improvement strategy aiming to increase the average performance of the base population by increasing the gene frequency of the desired gene and acting on existing variabilities without creating new ones ( Acquaah, 2015 ). The general process of mass selection can be divided into two categories. One is the negative mass selection to remove plants with unwanted traits or off-types, and the other is the positive mass selection to select plants with desirable traits to maintain purity and to generate more plants with desired traits ( Acquaah, 2009 ). These processes can be performed based entirely on the breeder’s visual judgment, either directly by selecting the desired trait or indirectly by selecting traits related to the desired trait ( Gjedrem and Thodesen, 2005 ). Also, since the selection is based on the plant’s phenotype, it is preferable if the desired trait is highly heritable or controlled by additive genes ( Brown and Caligari, 2011 ). Although the resulting plants are relatively homogeneous, they are genotypically broad, containing different pure lines. Since only one generation is required per cycle, this method is inexpensive, simple, and fast.
2.1.3. Pedigree selection
Pure line selection and mass selection methods focus on genetic variation, whereas pedigree selection methods create variation through hybridization. A pedigree selection method can be used for cross-pollinating species, but it is mainly used for breeding self-pollinating species. Pedigree selection uses a pedigree with accurate records of breeders to keep the ancestors by knowing who the parents of the F2 generation are in order that F2 individuals can be identified through the descendants of subsequent generations ( Poehlman and Sleper, 1995 ). As a result, a base population is established through parental hybridization, followed by selection and isolation, which progresses through generations until a desired degree of homozygosity is achieved ( Allard, 1960 ). Breeders can also influence genetic diversity and variation through these selections and processes. In this method, selecting species that are easy to observe, select, and harvest is desirable. Since lines begin to form after F4, selection should be based on progenies rather than individuals.
2.1.4. Bulk population
The bulk population method improves crops by putting off artificial selection until later generations and influencing variation through natural selection at the early generation stage ( Briggs and Knowles ). In other words, it is a breeding method in which homozygosity of the population is increased by repeating mixed breeding and group cultivation without selection in the early generation of hybrids (F2 to F4) and then fixing the line through individual selection in later generations (F4 to F5) ( Allard, 1960 ). The rationale for this method is that natural selection will eliminate individuals sensitive to various abiotic stresses in the production area for which traits are not desirable ( Acquaah, 2015 ). Varieties produced by this method will have already adapted to the place of production, and the method can be applied to creating cross-pollinated inbreeding populations. Still, breeding self-pollinating species that grow in tight spaces between individuals is best. However, the approach is that the genotype of the desired trait can be lost in early generations if it is not competitive under natural selection. In contrast, the genotype of a competitive but unwanted trait under natural selection can persist into future generations ( Acquaah, 2009 ).
SSD is a compromised breeding method between the bulk population and pedigree selection. This breeding method randomly selects ‘only one seed’ from each plant of the F2 population with the desired trait created by artificial hybridization of F1 to shorten the breeding time and reduce genotype loss in the segregated generation ( Allard, 1960 ). This advances the generation of many F2 plants through multiple generations, enabling rapid cultivation and selection of lines with high homozygosity in later generations. This method is best for plants such as soybeans that can self-pollinate and be grown densely ( Tigchelaar and Casali, 1976 ) and can be used in small spaces such as greenhouses. However, it is not suitable for traits with low heritability, polygenes, or traits associated with pleiotropic genes.
2.1.6. Backcross breeding
Backcross breeding aims to transfer one or more specific genes of interest from an ineffective breed to an excellent breeding line while preserving all other characteristics in the already excellent breeding line. It is a breeding method achieved over a relatively short time through repeated crosses and selection of superior breeding lines, i.e., recurrent parents ( Acquaah, 2009 ). Although this method is best suited for qualitative traits ( Borojevic, 1990 ), backcrossing may be difficult if the gene of interest is closely associated with unwanted genes, resulting in linkage drag ( Allard, 1960 ).
2.2. Techniques in molecular breeding
Traditional breeding, with a history of nearly 100 years, liberated humanity from starvation with groundbreaking achievements. Despite these outstanding achievements, it stagnated due to a long breeding time and the limited number of crops that could be cross-bred. Amid these difficulties, a new concept emerged that applied a molecular-based selection strategy that replaced the existing phenotype-based breeding strategy. Until then, breeders visually made selections for breeding. However, after the advent of molecular breeding, it became possible to determine whether the genes of the parent generation were passed on to the next generation through gene analysis technology ( Savadi et al., 2018 ). Next-generation sequencing (NGS) enables the discovery of molecular markers by sequencing the entire genome of a plant. Molecular breeding makes it possible to accurately select useful individuals with desired traits without being greatly affected by the environment by using molecular markers that detect the traits of each individual ( Metzker, 2010 ). Modern molecular breeding is a powerful molecular tool based on precision breeding that can reduce the time and effort required to produce new varieties and provide new and accurate in-depth research to satisfy the increasing global population and large-scale plant breeding requirements ( Figure 1 ).
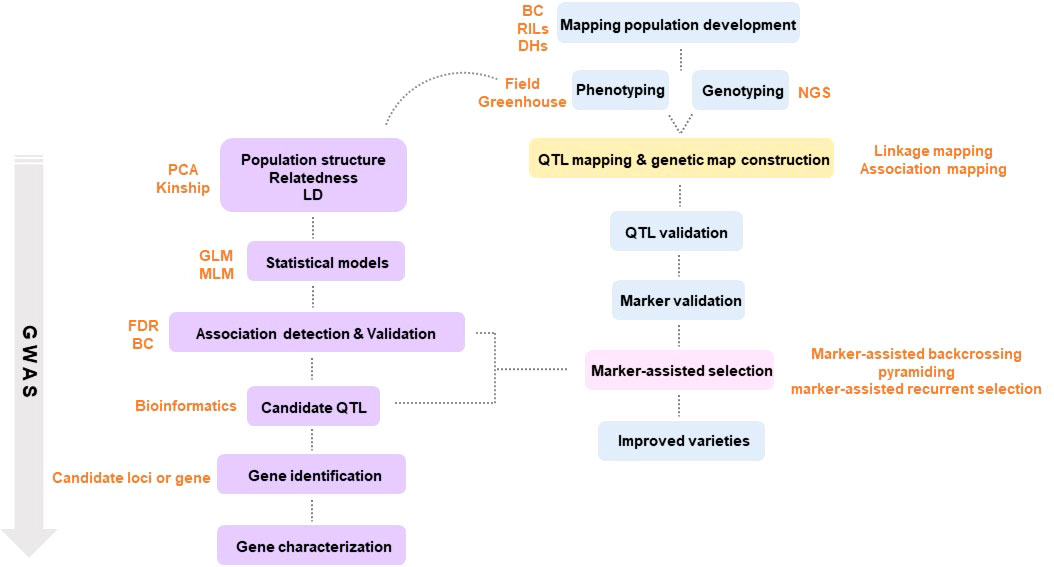
Figure 1 Schematic diagram of plant breeding using molecular markers.
2.2.1. QTL mapping
Molecular markers with DNA-based polymorphisms can be used for genetic improvement by allowing for the selection of useful traits ( Tewodros, 2016 ). In general, most of the agriculturally important traits are quantitatively inherited. Their genetic variation can be attributed to the collective response of several small effects associated with the trait. Methods utilizing traditional molecular markers include finding QTL-associated markers that regulate the expression of any trait in single or multiple parental mapping populations ( Semagn et al., 2015 ). Throughout the 1980s and 1990s, various rich molecular markers were developed, enabling QTL mapping with reasonable marker density and genome coverage ( Cooper et al., 2004 ). In plants, QTL mapping enriches biological knowledge of genetics and genetic structures across related species, providing useful markers to understand the genetic structures of complex traits ( Bernardo, 2008 ). Therefore, building linkage maps and finding correlations between genetic markers and phenotypic traits is fundamental ( Wang et al., 2016 ). Relatively simple single-marker analysis and more sophisticated interval mapping ( Haley and Knott, 1992 ), joint mapping ( Kearsey and Hyne, 1994 ), multiple regression ( Whittaker et al., 1996 ) and composite interval mapping ( Zeng, 1994 ) are used in various ways to link genotypes with many different quantitative traits. In recent decades, QTL mapping studies identified various QTLs that regulate complex phenotypic traits in crops such as rice ( Yano et al., 2000 ), maize ( Yano et al., 2000 ), arabidopsis ( El-Assal et al., 2001 ), and tomato ( Fridman et al., 2000 ).
2.2.2. Linkage disequilibrium (LD) mapping
Another method of selection to identify loci involved in the inheritance of complex traits is association mapping, also called LD mapping. This methodology is more efficient than QTL mapping, as it explores diversity using existing natural populations or germplasm collections with diverse cultivars, as opposed to QTL analysis, which uses bi-parental populations constructed using contrasting parents ( Gómez, 2011 ). Correlation between mapped genetic markers and traits can be used to detect QTLs ( Ibrahim et al., 2020 ). This can be difficult to do unless you have a well-annotated genome, as it allows for detecting more alleles with high resolution and precise mapping of quantitative traits but requires extensive knowledge of markers within the genome.
2.2.3. Genome-wide association study (GWAS)
With the development of NGS technology, computational methods using information from the genetic analysis have been improved, and GWAS search for key genes underlying important traits, contributing to the production of genetically improved plants. The first published GWAS was a study on humans in the early 2000s. Since then, several active studies in animals and plants have been conducted ( Ozaki et al., 2002 ). This methodology has emerged as an alternative approach to bi-parental QTL mapping in various crop species. Research on associations between molecular markers (e.g., SNPs) and desired phenotypic traits is key to identifying relevant genes. In addition, there is no need to develop new mapping populations because historical recombination events between accessions are used to find relevant genomic regions ( Nakano, 2020 ). Once accessions are genotyped, the data can be used for many different traits, enabling quick research of different traits in various environments ( Deng et al., 2021 ). In the case of GWAS, since the genetic variation is identified by genotyping many markers, it is important to select an optimal statistical model to detect false positives. Consequently, statistical power and computational efficiency may be indispensable for detecting truly associated markers ( Wu and Zhao, 2009 ). In GWAS, the mixed linear model (MLM) and general linear model (GLM) are the most recommended. In addition to those, there are various models such as the compressed MLM (CMLM), enriched CMLM (ECMLM), and the settlement of MLM under progressively exclusive relationship (SUPER), which are single locus analyses similar to GLM and MLM but more advanced. There are also the multiple loci mixed linear model (MLMM) and the fixed and random model circulating probability unification (FarmCPU). Among them, GLM is one of the methods with high computational efficiency, which can lower false positives by using the population structure and principal components as fixed effects. However, if the polygenic background is not sufficiently calculated, the false positives will be high, and the family structure will not be considered in the statistical analysis ( Mebratie et al., 2019 ). However, MLM (kinship or kinship + Q matrix + PCA) takes into account the population structure and is used to control for false positives by virtue of familial relatedness. These MLMs perform better than the GLM model alone and are widely used as an alternative to GLM ( Alqudah et al., 2020 ). These models were improved and developed to produce better statistical results. However, MLM can be difficult to control for false positives when it involves population structures with extensive genetic diversity. In this case, CMLM and ECMLM were developed to increase statistical power further. The SUPER model increases statistical power by inducing kinship using relevant genetic markers instead of the entire markers. MLMM and FarmCPU extend the single-loci method of MLM to a multiple-loci method. FarmCPU combines the MLMM strategy into the limited kinship matrix of the SUPER model ( Kusmec, 2018 ). Thus, it tests markers using multiple related markers as covariates in a fixed effects model and performs analysis on related covariate markers in a random effects model. FarmCPU is faster than MLMM and effectively improves problems caused by false positives ( Liu et al., 2016 ). In addition, as new models are continuously developed, the performance and statistical power of GWAS is expected to increase over time. Also, existing models need to be improved more efficiently in line with increasing data availability.
Recently, the amount of molecular markers for traits of interest in plant breeding has been gradually increasing. QTL mapping to identify genetic loci quantitatively associated with traits of interest is the basis for developing molecular markers used in MA) ( Ibrahim et al., 2020 ). MAS can be defined as the manipulation of a genomic region involved in expressing a trait of interest in a short time through the application of DNA markers. These attempts led the study of molecular breeding into a new era ( Sharma, 2020 ). It is also applied to plant breeding to improve tolerance to biotic or abiotic stress and to improve crop yield and quality. MAS has the basic idea of using LD between markers and QTLs: using a non-random association between the marker and the QTL allele ( Hospital, 2009 ). Identifying genes in the target trait and markers linked to QTLs is a prerequisite for these MAS ( Khush, 2000 ). The framework of MAS in plant breeding is divided into four groups: (i) marker-assisted backcrossing, (ii) marker-assisted pyramiding, (iii) early generation marker-assisted selection, and (iv) marker-based recurrent selection. These systems characterize genetic material in early segregating generations and strongly anchor the breeding cycle ( Nadeem et al., 2018 ). Marker-assisted backcrossing (i), first described in 1992, is a technique for the introgression of one or several major genes of a donor line into the genetic background of an elite line or recurrent line. With the help of molecular markers, it was possible to speed up the selection and genomic recovery of recurrent parents ( Muranty et al., 2014 ), and it is widely used to eliminate undesirable traits (ex., disease susceptibility) in popular varieties ( Sharma, 2020 ). Marker-assisted pyramiding (ii) is the process of combining multiple genes into one genotype. The most common strategy of pyramiding is to combine several resistance genes to biotic and abiotic stress, which is a strategy to prevent the decay of resistance to specific diseases or stresses. This method efficiently transfers genes into improved varieties by pyramiding the gene combinations. Early generation MAS (iii) has the advantage of selecting markers at an early generation, allowing attention to fewer important lineages in the next generation compared to the previous generation ( Collard and Mackill, 2008 ). In plants, a technique that helps to improve quantitative traits by repeating crosses and selection is called recurrent selection. The goal of marker-based recurrent selection (iv) with such recurrent selection is to augment favorable alleles and more QTLs in the population before inbred lines extraction ( Bankole et al., 2017 ). This method can efficiently breed complex traits because it can use minor genes/QTLs that do not significantly affect the phenotype ( Abdulmalik et al., 2017 ). However, the limitation of MAS in plant breeding is that traits composed of many minor effect alleles cannot be efficiently selected. Besides, linkage drags occur in every breeding effort, which hampers the improvement of target traits. Consequently, predictive breeding procedures using agro-bigdata and advanced statistical models embedded in some basic machine learning algorithms are being recently used for overcoming the limits of MAS, which will be discussed in the next section.
Complex quantitative traits are regulated by genome-wide minor effect alleles. Most economically useful traits, such as yield, fruit quality, and stress tolerance, are mostly complex quantitative traits ( Bhat et al., 2015 ). There are two major marker-based breeding methods—MAS and GS—to select plants with superior traits. In MAS, crops are selected based on QTLs detected through linkage mapping (LM) or GWAS ( Myles et al., 2009 ). MAS cannot identify genes with minor effects associated with complex traits, and if the associated markers constitute a small fraction of genetic variation, the results are worse than phenotypic selection ( Xu and Crouch, 2008 ). Moreover, in MAS, only a few statistically significant specific markers are used, and the rest are excluded from the analysis. Therefore, in traditional MAS, the number of specific markers per trait is generally low, and the use of MAS is limited when several genes with small effects are involved in one trait ( Bernardo, 2008 ). Therefore, MAS is optimized for qualitative traits, such as specific metabolites and disease resistance, rather than quantitative ones.
A new method called GS deals with these problems. Improvement of complex traits requires phenotypic evaluation at various locations and multi-years to confirm the correlation between environment and genotype. However, it was difficult due to the lack of cost and labor. With the development of NGS technology, sequencing costs have become cheaper, and high-resolution genome information can be easily obtained. Advances in sequencing methods have made GS possible ( Gorjanc et al., 2015 ). All available high-performance markers in the genome can be used to select GS crops ( Jannink et al., 2010 ). Given a marker set covering the entire genome, GS models consider all markers influencing a trait regardless of a specific threshold. Traditional MAS focuses on a small number of major genes or QTLs, whereas GS makes predictions by integrating all available markers in the genome into the model. Calculating all genetic effects prevents the loss of genetic variance occupied by minor genes or QTLs. Therefore, GS is more effective than MAS for traits regulated by multiple markers ( Meuwissen et al., 2001 ). The most significant advantage of GS is that it can predict the phenotype information of mature individuals based on genotype data obtained from seeds or seedlings. This process eliminates the need for comprehensive phenotypic evaluation by year or environment and increases the speed of crop varietal development ( Bhat et al., 2016 ). Previous studies showed that compared to MAS, the accuracy of genomic prediction (GP) is three times higher for maize ( Zea mays L.) and two times higher for wheat ( Triticum aestivum L.) ( Heffner et al., 2009 ). Therefore, it is expected that this genome-based predictive selection has the potential to replace phenotypic selection or marker-assisted breeding.
GS combines phenotypic and genotype data of the training population to construct a model. Then, based on the learned model, the genomic estimated breeding values (GEBVs) of individuals in the breeding population are predicted ( Figure 2 ). Therefore, with genotype data, GS selects individuals based on GEBVs from the validation (or breeding) population. In this case, phenotype information from the validation population is not required ( Meuwissen et al., 2001 ). Breeding values consist of two elements. The first is the average breeding values of the parents, and the second is the variance of the progeny from the mean breeding values of both parents due to Mendelian sampling. Since GS uses a high density of markers to quantify Mendelian sampling, large-scale phenotypes of the progeny are not required. This process reduces the breeding cycle and is more efficient than comprehensive phenotyping. GS is effective for complex traits with low heritability and simple traits with high heritability. In addition, it is possible to reduce the development cost of a hybrid or breeding line ( Crossa et al., 2017 ). The process of training models resembles that of acquiring breeding resources by breeders. Therefore, GS is closer to a conventional selection procedure. On the other hand, selection in the early stages of growth based on the trained models can be made, like MAS, combining the advantages of conventional breeding and MAS.
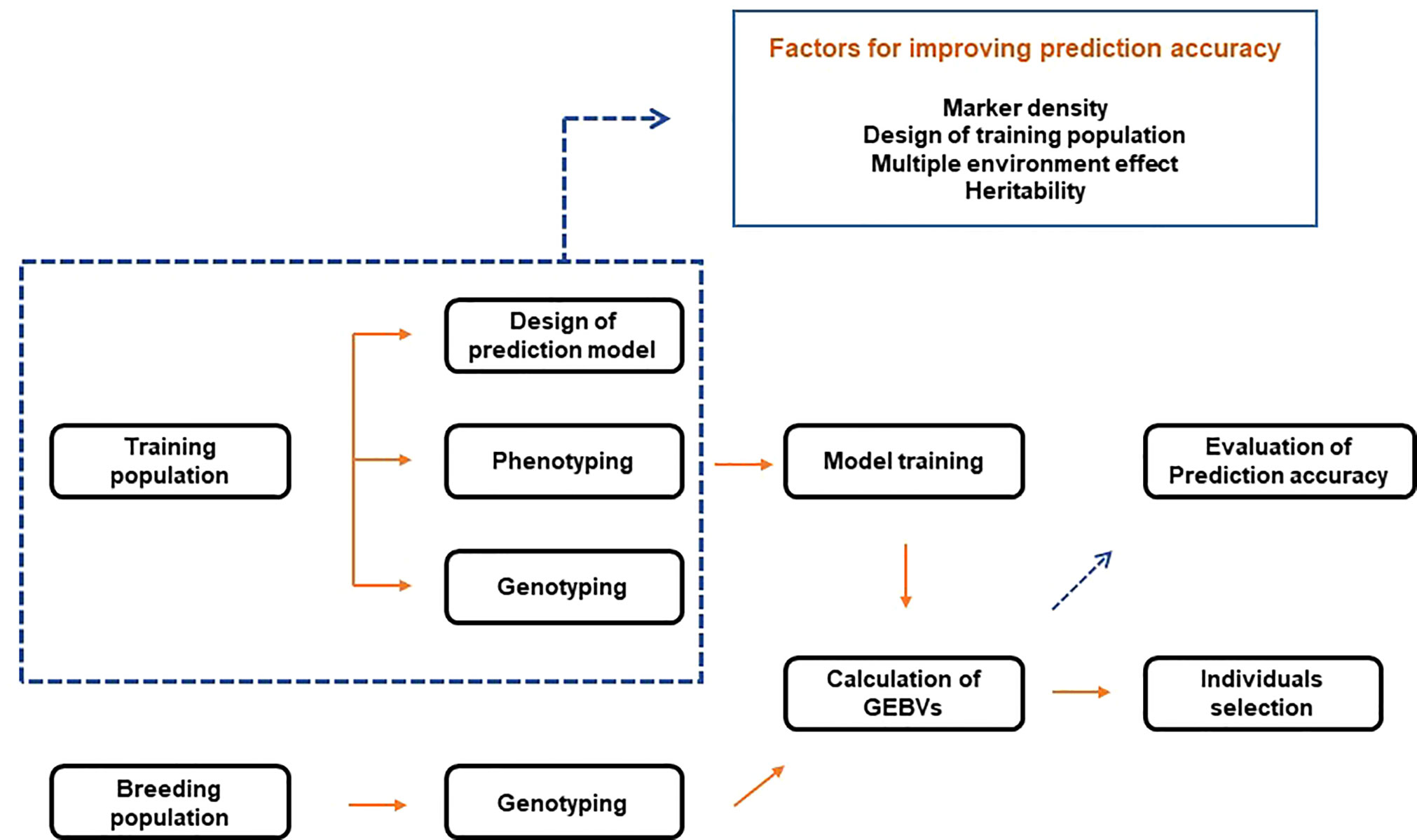
Figure 2 Schematic diagram of genomic selection.
Although GS is a useful tool in plant breeding, there is limited information on setting up an optimized statistical model. Incorrect data imputation, unexpected responses, and environmental constraints limit the performance of GP. Although attempts were made to consider and overcome these limitations in several statistical prediction models, it is still the most challenging problem in multidimensional genomic data ( Budhlakoti et al., 2020 ). The prediction accuracy difference between the respective models is determined according to the underlying statistical methods. Many GP models use a large set of markers to predict the phenotype, and each model makes different assumptions according to the distribution and difference of the markers ( Goddard, 2009 ). So far, the only solution to avoid this limitation may be repeated trials with different statistical models to find an optimized scenario that can be applied to target traits. GP models are divided into parametric and non-parametric methods according to the presence of prior information and the setting of parameters ( Budhlakoti et al., 2022 ). In parametric methods, Regularized Linear Regression (RLR) models such as least absolute shrinkage and selection operator (LASSO) ( Tibshirani, 1996 ) and ridge regression (RR) ( Meuwissen et al., 2001 ) emerged, improving the over-parameterization problem of the existing simple linear model. Currently, best linear unbiased prediction (BLUP) and Bayesian models are mainly used according to variance assumption in GS ( Meuwissen et al., 2001 ). Semi-parametric methods such as reproducing kernel Hilbert space (RKHS) and Nadraya-Watson estimator predict GEBVs taking into account epistatic genetic structure ( Gianola et al., 2006 ). Furthermore, ML-based statistical models such as SVM ( Long et al., 2011 ), artificial neural networks (ANN) ( Gianola et al., 2011 ), and random forest (RF) ( Holliday et al., 2012 ) have been applied to plant breeding ( Figure 3 ). Various studies have tried to find optimal accuracy by applying various statistical models for each crop. However, it is difficult to define an optimal statistical method due to differences in crops, cultivars, environments, populations, and markers. Therefore, it is necessary for breeders to compare and to select appropriate statistical methods for each situation when conducting GS.
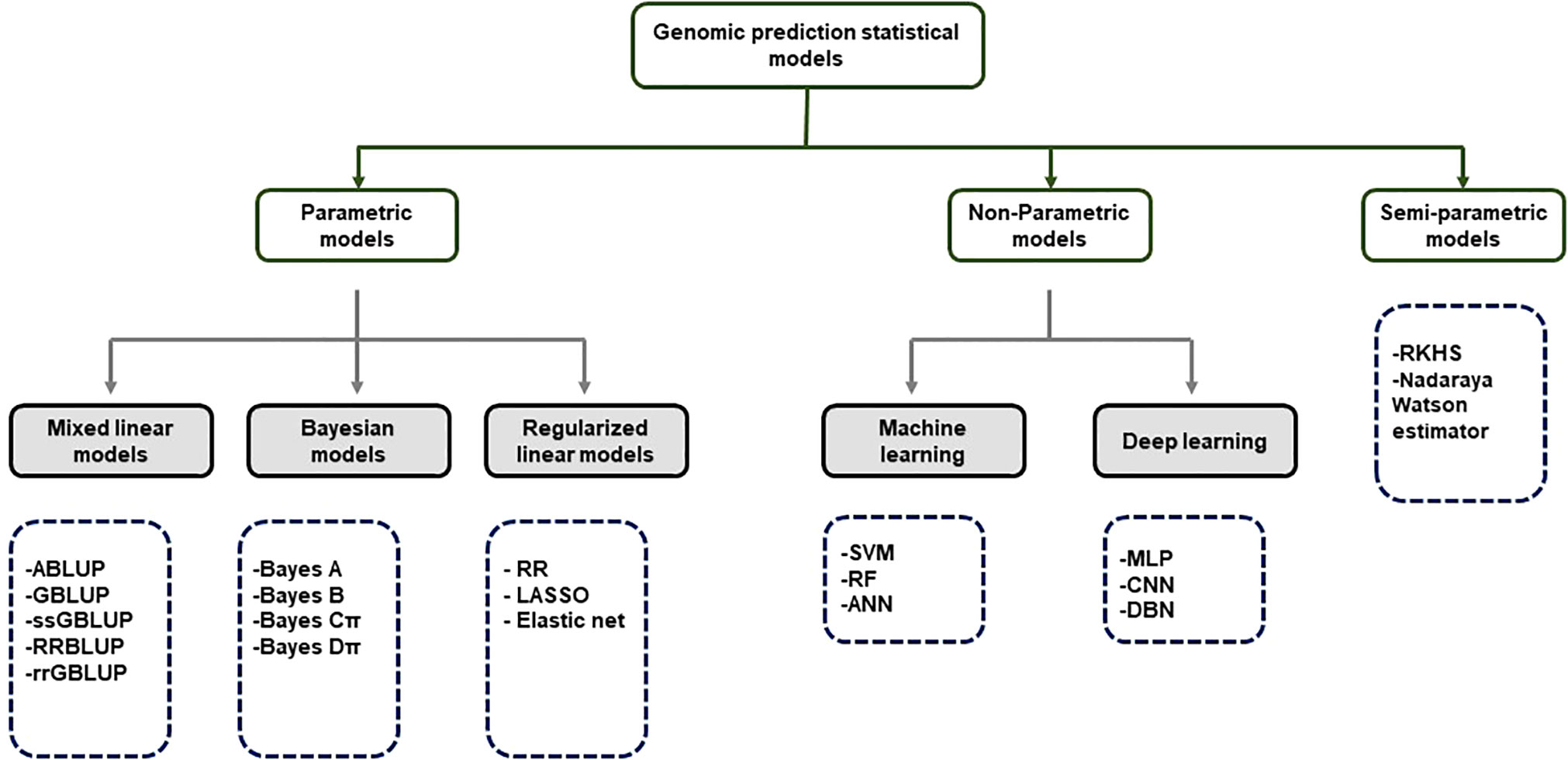
Figure 3 Classification of GS statistical models.
ABLUP- Traditional pedigree- Best Linear Unbiased Predictor; GBLUP-Genomic Best Linear Unbiased Predictor; ssGBLUP-Single-step Genomic Best Linear Unbiased Predictor; RRBLUP-Ridge Regression Best Linear Unbiased Predictor; rrGBLUP- Ridge Regression Best Linear Unbiased Predictor; Bayes-Bayesian method RF-Random Forest; RR-Ridge Regression; LASSO-Least Absolute Shrinkage and Selection Operator; SVM- Support Vector Machine; RF-Random Forest; ANN-Artificial Neural Networks; MLP-Multi-Layer Perception; CNN-Convolutional Neural Networks; DBN-Deep Belief Network; RKHS- Reproducing Kernerl Hillbert Space.
2.3.1. RLR models
GS tries to avoid biased marker prediction effects by using the entire marker. Ordinary least squares (OLS) is a simple method for measuring the influence of markers. However, OLS only makes predictions within a sample and does not allow the weighting of markers. In the case of a high density of markers, the number of markers (p) exceeds the sample size (n). Therefore, it is not appropriate to obtain an estimate of the marker effect through OLS ( Perez et al., 2010 ). In order to solve this problem, RLR models were proposed, such as RR and LASSO. The RLR model reduces the variance of regression coefficients by shrinking the regression coefficients. Through this process, only the key predictor variables are considered when making estimations in the statistical model ( Szymczak et al., 2009 ). This process can improve prediction accuracy by reducing the mean squared error ( Friedman et al., 2010 ).
RR estimates the regression coefficient using parameter shrinkage, giving the ℓ2-norm penalty. The ℓ2 penalty of RR tends to shrink the coefficient to zero. In particular, RR is advantageous for non-zero coefficients. In the case of k identical predictor variables, when the predictor variables are fitted alone, each coefficient is equally reduced to the size of 1/k. Moreover, RR is effective when many predictors have small effects, especially coefficients with many correlated variables. Therefore, RR cannot eliminate coefficients and cannot select only a relevant subset of predictors. Similar to RR, LASSO is advantageous for processing large amounts of data quickly and efficiently ( Friedman et al., 2010 ). However, LASSO has a weakness when many predictors are correlated. If only one suitable predictor is selected from k identical predictor variables, the others are ignored. That is, Lasso’s ℓ1 penalized least squares criterion excludes coefficients close to zero. The most significant difference between LASSO and RR is that ridge regression only shrinks coefficients, whereas LASSO also selects variables. In addition, LASSO shrinks coefficients with the same force for all coefficients, but RR shrinks coefficients in proportion to the size of the coefficients ( Ogutu, 2012 ). On the other hand, elastic net combines the penalties of LASSO and RR. Elastic net penalty weights LASSO and RR. When the penalty parameter is close to 0, it corresponds to RR. However, when it is close to 1, it shows a performance similar to LASSO. Elastic net solves the disadvantages of using RR or LASSO alone and shows high prediction accuracy when predictors are correlated ( Tutz, 2009 ).
Estimating the value of breeding requires optimizing the estimation of the regression coefficients but also optimizing the information in the phenotypic data. There is a method that combines these two factors. There is a method of obtaining a phylogenetic effect by simultaneously modifying the phenotype and a method of estimating the breeding value using an additional genetic relationship between plants. This method is called best linear unbiased prediction (BLUP) ( Goldberger, 1962 ). BLUP statistical method was proposed by Henderson (1975) as the coefficient matrix of mixed model equations (MME) for linear mixed models used in data analysis to estimate and predict random effects. BLUP evaluates all objects simultaneously and acts as a linear model, correcting for different effects between objects and estimating by combining objects and fixed effects. For linear models, a single genotype effect appears as an independent random variable, uncorrelated ( Piepho et al., 2008 ). These BLUP models include pedigree-based BLUP (ABLUP), genomic best linear unbiased predictor (GBLUP), and ridge regression BLUP (rrBLUP). ABLUP is a standard method for predicting breeding values through inferable associations between individuals using relevant information based on pedigree ( Crossa et al., 2010 ). ABLUP’s Ep is assumed to follow a normal distribution of random additive effects ( Rezende et al., 2014 ). GBLUP is derived from ABLUP but differs in that the matrix of the marker-based matrix Eq is replaced by G ( Myburg et al., 2007 ). GBLUP has potential advantages over ABLUP. First, the prediction of similarity computed based on pedigree is replaced by similarity feasible in GBLUP, which consists of weak assumptions. Possible similarities can also be characterized in pairs instead of based on family history in ABLUP ( de los Campos et al., 2009 ). Unlike the previous two methods, rrBLUP changes the parameter notation in Eq. It has a similar computational method to GBLUP and assumes that the same marker effects are reduced, and the variances are equally normal. The GBLUP method is the most common approach in animals and plants. GBLUP was parameterized with the ridge regression model ( Hoerl and Kennard, 1970 ), rrBLUP, to predict the genome. The reduction of SNPs can be determined either by normalizing rrBLUP or by the ratio of variance components in GBLUP ( Heslot et al., 2012 ).
2.3.3. Bayesian prediction models
Bayesian analysis is named after the British mathematician Thomas Bayes. This analysis is a statistical method that combines the information in the sample with the information on the parameters in the population in advance to explain the statistical reasoning process ( Gelman et al., 1995 ). The first step in using the model is specifying the probability distribution of the parameter of interest in advance and applying it by providing the posterior probability distribution for the parameter. These posterior distributions provide the basis for the parameters ( Mila and Ngugi, 2011 ). In this method, single nucleotide polymorphisms (SNPs) encompass the entire genome and are used in animals and plants to estimate breeding values. Training to estimate the effect of SNPs in statistical problems allows us to estimate the effect in situations where the number of individuals is much smaller than the vast amount of SNPs ( Habier et al., 2011 ). A key part of this analysis is the probability distribution of a parameter in the population. The Bayesian approach allows for subjective and objective data to pre-determine the distribution. Therefore, some argue that Bayesian analysis lacks objectivity ( Habier et al., 2011 ).
Many Bayesian methods for GS have been developed. Similar sampling models have been shared, and a new concept of the Bayesian alphabet has emerged, including Bayes A and Bayes B in different analytical methods ( Gianola et al., 2009 ). In animal and plant breeding studies, hierarchical Bayesian models such as Bayes A and Bayes B were presented ( Meuwissen et al., 2001 ), as well as Bayesian LASSO ( Legarra et al., 2011 ; Li and Sillanpää, 2012 ) and Bayesian ridge regression (BRR) ( De Los Campos et al., 2013 ).
Direct approaches such as GBLUP first construct the relationship between SNPs and molecules and then utilize mixed model equations to determine genetic merit directly [89]. The Bayesian approach is more effective than other QTLs and genetic value prediction models, as many SNPs are preferentially merged into ineffective ones ( Meuwissen et al., 2001 ). In addition, the Bayesian method, which assigns higher variances to subsets of SNP effects, has been shown to achieve higher prediction accuracy than GBLUP when large effect variations contribute to complex traits ( Hayes et al., 2010 ).
Although the Bayesian model is widely used in animal and plant breeding, it has several drawbacks, including those previously described. First, SNPs treated as close to one are assigned an ineffective ratio. Second, the degree of freedom of the independent data dictionary is used for distribution. Only one degree of freedom can be added regardless of subsequent phenotypes and genotypes. In order to overcome these shortcomings, Bayes Cπ and Bayes Dπ were developed and sampled by replacing parameter π or scale parameter S with variables rather than data information ( Habier et al., 2011 ).
Bayesian methods in this context have mechanisms to combine prior probability distributions with sample data information for later probability distributions in the natural state. Because of this, it can also be used to make better decisions in posterior probabilities.
2.3.4. Semi-parametric prediction models
Parametric models such as MLM and Bayesian models, widely used in GS, use a prior effect size distribution determined by several parameters. The parameters used in predictive models limit the amount of information the model can use. Therefore, the small number of parameters determined in the predictive model limits the flexibility of the model. On the other hand, in the semi-parametric model, there is no assumption that data follows a specific distribution, and the number of parameters is determined according to the amount of training data. The semi-parametric model can be used when there is no prior information about the data ( Murphy, 2012 ). Predictive models can be applied differently depending on the genetic architecture of the trait. Parametric predictive models can take into account additive effects but are ineffective for epistasis due to the difficulty of predicting high interactions ( Howard et al., 2014 ). Semi-parametric and non-parametric models have high accuracy in genetic architectures with epistasis effects.
Conversely, semi-parametric models involve epistasis effects and have been used in several plant prediction studies. Epistasis plays a vital role in explaining the occurrence of genetic variation, and considering epistasis in predictive models can obtain good predictive accuracy of plant breeding in quantitative traits ( Cordell, 2002 ; Howard et al., 2014 ). Epistasis is the interaction between genes in which one locus affects the phenotype by altering the effects of another locus. Therefore, epistasis can occur between multiple loci, and various interactions must be included in the model to calculate GEBVs for GS. A large number of markers is used in GS, and the corresponding epistasis interactions increase even more. Therefore, it is difficult to predict genetic gain using a model using only a small number of specific parameters ( Moore and Williams, 2009 ).
For this reason, several models have been devised for genetic prediction. RKHS is designed for genetic prediction in non-linear models. It makes inferences about functions without prior information in a semi-parametric method. RKHS reflects independent variables in a finite-dimensional space into infinite-dimensional Hilbert spaces. This method assumes that distances in Euclidean space can be expressed through a kernel matrix that reflects the distances between objects in Hilbert space ( Rodriguez-Ramilo et al., 2014 ). RKHS obtains prediction results by applying ML after transforming the independent variable using a kernel function. RKHS using implicit transformations has good results for predicting non-linear patterns of data ( Gianola and van Kaam, 2008 ).
3. Digitalizing plant breeding
3.1. strategies to increase prediction accuracy in gs.
Comparison of GS methods is evaluated by prediction accuracy. The prediction accuracy is measured by the correlation between the measured GEBVs and the actually measured phenotype data. Therefore, improvement of prediction accuracy is important for GS applications.
3.1.1. Marker density and selection
Among them is the density of the marker. Since GS estimates the effects of markers using LD between quantitative loci and markers, a high density of markers is advantageous for GS ( Meuwissen et al., 2001 ). Even for low-density markers in GS, some efficiency can be guaranteed if the intervals of the markers are uniformly distributed ( Habier et al., 2009 ; Spindel et al., 2015 ). In addition, markers are selected by additive effect sizes, and when the trait of interest is oligogenic, it has high predictability compared to markers with uniform spacing ( Li et al., 2018 ). In GS, a method using high-density SNPs is widely used to increase accuracy. However, this method has a negative side when species have low importability or large populations ( Crossa et al., 2010 ). Therefore, reducing marker density could be a solution to reducing the cost of GS implementation.
To take advantage of GS while lowering the marker density, highly correlated duplicate markers can be removed from the LD block. This approach reduces multicollinearity and does not interfere with the marker effect. It can also be a good alternative because it reduces the possibility of overfitting ( Xu, 2013 ). The low density of markers reduces computational analysis time and allows for genotyping of more individuals at the same cost. Even if the number of markers is reduced, it can have high prediction accuracy, so a method to identify valid markers can be a reasonable strategy for GS in the future. Therefore, breeders should consider the appropriate marker density to fit their budget and time when conducting GS ( Gorjanc et al., 2015 ). If it is difficult to reduce the number of markers using LD, the accuracy of GP can be improved by using GWAS-related markers. The presence of irrelevant markers in the GP process can reduce prediction accuracy. GWAS predicts the effect of markers based on the entire genome and selects markers statistically linked to the target trait. Therefore, the prediction accuracy can be significantly improved if GP is performed with markers associated with the target trait ( Odilbekov et al., 2019 ). In addition, combining GWAS and GS is convenient because it does not use additional data and uses the same existing phenotypic and genotypic information ( Odilbekov et al., 2019 ). In short, selecting an appropriate marker for GS is an important factor for improving prediction accuracy. Breeders should consider the range of markers prior to selection.
3.1.2. Design of training population
The accuracy of genetic predictions is also determined by the design of the training population. Well-established training populations are important in GS. The training population consists of breeding lines with phenotype data for target traits and genotype data. After training a predictive model with markers, the prediction of GEBVs in the test population is performed using the trained model ( Akdemir and Isidro-Sanchez, 2019 ). As NGS technology advances, genotyping costs and time continue to decrease, but the progress in phenotyping is slow. High costs and wasted labor are difficulties and limitations in plant breeding. In general, increasing the size of the training population tends to improve prediction accuracy. However, breeders should choose an optimized training population that maximizes predictive accuracy while significantly reducing phenotypic costs ( Lado et al., 2013 ). Traditional optimization methods use random sampling. Random sampling does not increase prediction accuracy because of under- or over-represented genetic information ( Bustos-Korts et al., 2016 ). After comparing random sampling methods, the coefficient of determination (CD) and the prediction error variance (PEV) methods have been proposed. CD shows slightly better results than PEV because CD from random samples shows genetic diversity when selecting individuals ( Rincent et al., 2012 ). Another method of optimizing the training population is to use the genetic information of the test population when establishing the training population. The use of genetic information in the test population leads to a significant increase in prediction accuracy by applying a genetic algorithm ( Lorenz and Smith, 2015 ; Akdemir et al., 2015 ). The genetic link between the training and test populations increases GP accuracy ( Wientjes et al., 2013 ) because when the genetic distance between individuals is close, they share a common ancestry, and there is less recombination between the marker and the QTLs. Furthermore, the two groups share polymorphic loci that produce genetic variations ( Habier et al., 2010 ). In addition, if the genetic background between groups is shared, the interaction deviation between the QTLs and the genetic background is shared ( Lorenz and Cohen, 2012 ). Therefore, breeders should consider training the population composition of unrelated individuals if they want to increase the accuracy of GP.
3.1.3. Multiple environments and heritability
Difficulties in making genetic predictions should apply to GEBVs in various environments. For accurate predictions, predictive models must consider terms that interact with various environments and environment x genotype interactions. These changed prediction models are divided into two types. The first is a non-informed model, which includes the environment as the main random effect. Also, the interaction between a genotype and environment is specified as a random effect. These prediction models report improved accuracy compared to traditional prediction models that specify only a genotype and environment as the main effects ( Jarquin et al., 2014 ; Lopez-Cruz et al., 2015 ). On the other side, the informed prediction model includes measured environmental covariates in each environment. Then, informed prediction models incorporate information through variance-covariance structures using kernel-based methods. Finally, the informed prediction model calculates the interaction between each marker and environmental covariates and includes these calculations in predicting GEBVs. It is important to predict GEBVs by considering these models in various environments. Predictive models should be improved in terms of prediction results, or genetic and environmental variability should be analyzed to improve accuracy ( Basnet et al., 2019 ). In general, traits with high heritability are determined by some genes having a major effect. Because these traits are less affected by the environment, their prediction accuracy is high. However, since numerous genes determine most of the traits humans try to breed with minor effects, it is necessary to consider the environment when selecting a predictive model ( Combs and Bernardo, 2013 ).
3.1.4. High-throughput phenotyping (HTP)
With the development of NGS and large-scale marker information, the application of GS increases in plants. However, one of the challenges that breeders face in training GS models is inaccurate phenotypic data. The sophistication of phenotypic information is as important as genomic information. Inaccurate phenotypic data make the predictive ability of the GS model decline. In addition, the existing phenotype measurement method has disadvantages because it is labor-intensive and requires a lot of time and costs. Recently, high-throughput phenotyping (HTP) has been used for accurate phenotypic information measurements. HTP is a non-destructive, fast, and accurate phenotypic measurement method that accurately captures traits of interest ( Pabuayon et al., 2019 ). HTP has developed rapidly in plant breeding over the past decade. HTP processes stress tolerance, yield, and growth information through automated sensing, data acquisition, and processing. These advantages of HTP include accelerating the breeding cycle while allowing rapid screening of numerous plants at various growth stages ( Yadav, 2021 ). The HTP platforms include RGB, normalized difference vegetation index (NDVI) sensors, multispectral and hyperspectral cameras, spectrometers, and light detection and ranging (LiDAR) technology ( Yadav, 2021 ). The HTP platforms include RGB, normalized difference vegetation index (NDVI) sensors, multispectral and hyperspectral cameras, spectrometers, and light detection and ranging (LiDAR) technology ( Shabannejad et al., 2020 ). Many studies on GS in plants using HTP have been conducted. GS was performed utilizing HTP using NDVI in wheat. Data collected through HTP showed a 7 to 33% increase compared to the standard univariate model ( Crain et al., 2018 ). In addition, when HTP was performed in wheat using RGB, the prediction accuracy for days to maturity increased by 3 to 4 times ( Shabannejad et al., 2020 ). Also, in another study, the measurement of secondary traits in wheat using an unmanned aerial vehicle (UAV) remote sensing increased the genetic prediction accuracy of grain yield by an average of 146% ( Sun et al., 2019 ). These results suggest that using HTP improves model performance while enabling accurate selection. The efficient use of HTP becomes the background for ML and DL technology. The advanced combination of genotyping and phenotyping will provide breeders with opportunities for better GS.
3.2. Application of ML for GS
ML uses statistical techniques to allow systems to learn from data without explicitly programming them. ML takes a sample and then builds a model to explore algorithms that learn from current data and make predictions on new data. ML-based methods can be effective in improving prediction accuracy compared to conventional GS ( Yoosefzadeh-Najafabadi et al., 2022 ). The main difference between the traditional statistics model and ML is that ML is a non-parametric model that offers tremendous flexibility to adapt to complex associations between data and outputs. It is difficult to build informative and predictive models because of the expanding scale of genome data, inherent complexity, the unique characteristics of organisms, and various environmental variables. Accordingly, the use of ML continues to grow and can be a good alternative ( Greener et al., 2022 ). It initially adapts hidden patterns of unknown structures that cannot be incorporated into parametric models ( Gianola, 2013 ).
GS statistical methods use ML for more accurate predictions ( Table 2 ). Statistical analysis of the genetic basis of quantitative traits in plants is unsuitable for complex configurations such as pleiotropic genes, gene X gene, and gene X environment interactions. It is difficult to capture all marker effects, and problems such as the ‘large p, small n’ problem, sometimes lead to over-parameterization. ML methods improve prediction accuracy through observations of repeated experiences ( Gianola et al., 2006 ). ML can identify hidden information in large data. Therefore, it is attractive for complex genomic information, including information about gene interactions and pleiotropic genes, when performing GS. ML develops and applies data through computer algorithms and is divided into supervised and unsupervised learning. Supervised learning predicts desired trait values from input data. On the other hand, unsupervised learning checks the group and association between input variables in which output variables do not exist.
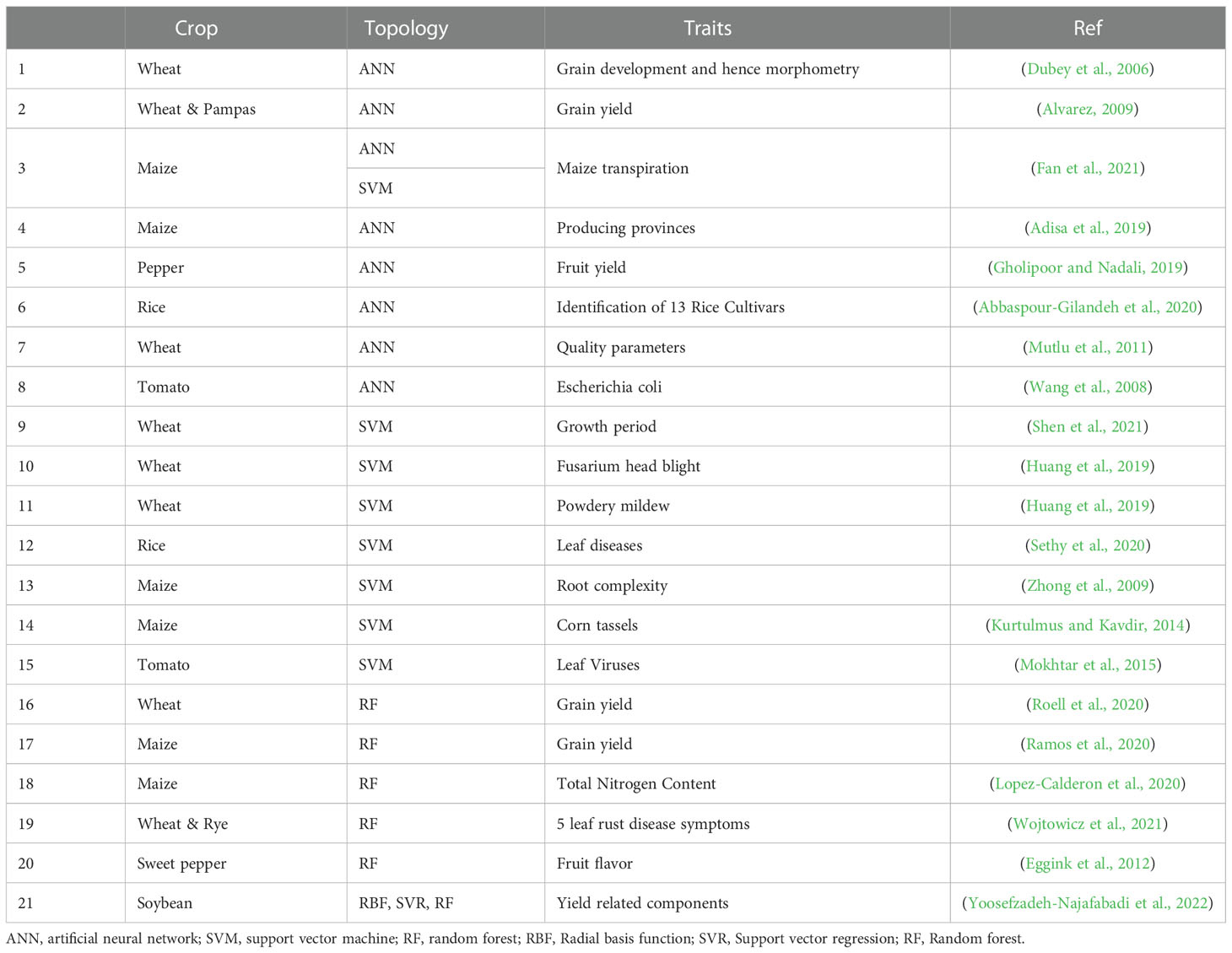
Table 2 Machine learning application to GS in plants.
Most ML involves supervised learning, such as Bayes nets, rule-based learning decision trees, naive Bayes, and nearest-neighbors, and applies to GS in the form of RF, ANN, and SVM ( Gonzalez-Camacho et al., 2018 ). RF is an attractive alternative to analyzing complex individual traits using dense genetic markers. RF has good predictive power to measure the importance of each marker. RF does not require the specification of inheritance and takes into account non-additive effects. In addition, it is fast even when dealing with many covariates and can be applied to regression and classification models ( Gonzalez-Recio et al., 2010 ).
Another ML-based model, SVM, is advantageous for classification and regression, similar to other ML models. The difference from other ML models is that SVM is specialized in identifying subtle patterns in complex and large amounts of information data. SVM makes a decision boundary with various feature vectors to achieve predictions. SVM can flexibly handle data using kernel-based functions. Furthermore, SVM can improve the non-linear form of phenotypes and genotypes using kernel functions ( Noble, 2006 ). ML models can be applied in many scientific fields, but it is not clear whether these methods are superior to other statistical models. Therefore, if you want to make a clear prediction in the GS process, data acquired by various methods should be accumulated, and the optimal method should be selected based on experience.
Recently, the ML method, a specific type of ANN, has been considered to increase the performance of GS. ANN takes into account patterns in data and makes predictions about complex functions as universal approximations ( Gianola et al., 2011 ). In GS, these functions automatically identify factors such as epistasis or dominance in genomic marker information. Moreover, it does not require any assumptions about the phenotypic distribution, and applying ANN to GS enables effective estimations of the effects of complex interactions ( Rosado et al., 2020 )
3.3. Application of DL for GS
Although studies on GS applied with DL are still lacking, several advanced studies exist ( Table 4 ). In 2018, Montesinos-López et al. ( Montesinos-Lopez et al., 2018 ). compared DL and GBLUP models using densely connected network architecture. Their study used nine published genomic data sets (three maize and six wheat data sets). When genotype x environment interactions (G x E) were ignored, DL had good predictive accuracy in 6 of 9 data sets. However, the prediction accuracy of GBLUP was excellent in 8 of 9 data sets when the G x E interaction was taken into account. In the study conducted in 2019, genomic-based prediction performance was confirmed by comparing the Bayesian threshold genomic best linear unbiased prediction (TGBLUP) model with multi-layer perceptron (MLP) and SVM methods ( Montesinos-Lopez et al., 2019 ). It was confirmed that SVM and MLP were the most efficient in terms of computation time. These studies suggest that DL is not a data science panacea but a worthwhile addition to the data science toolkit in plant breeding. So far, research on GS using DL confirming prediction accuracy through the comparison with existing statistical models is limited. Thus, more research is needed.
An artificial neural network (ANN), the most fundamental concept in DL, is a network structure created by mimicking the neuron connection structure of a human neural network. A structure in which three or more ANNs are superimposed is called a deep neural network (DNN), and ML using this is called DL ( LeCun et al., 2015 ). Popular DL topologies in GS include MLP, a convolutional neural network (CNN), and a recurrent neural network (RNN) ( Montesinos-Lopez et al., 2021 ).
In MLP, in an artificial neural network, data moves in one direction from the input node through the hidden node to the output node ( Figure 4 ). It has at least one hidden layer and is usually supervised learning. This method is the simplest to train, generally performs well in a variety of applications, and is suitable for general prediction problems where it is assumed that there is no special relationship between the inputs. However, these networks are prone to overfitting during the training process, so there is a problem in that the accuracy decreases in real data ( Abdollahi-Arpanahi et al., 2020 ).
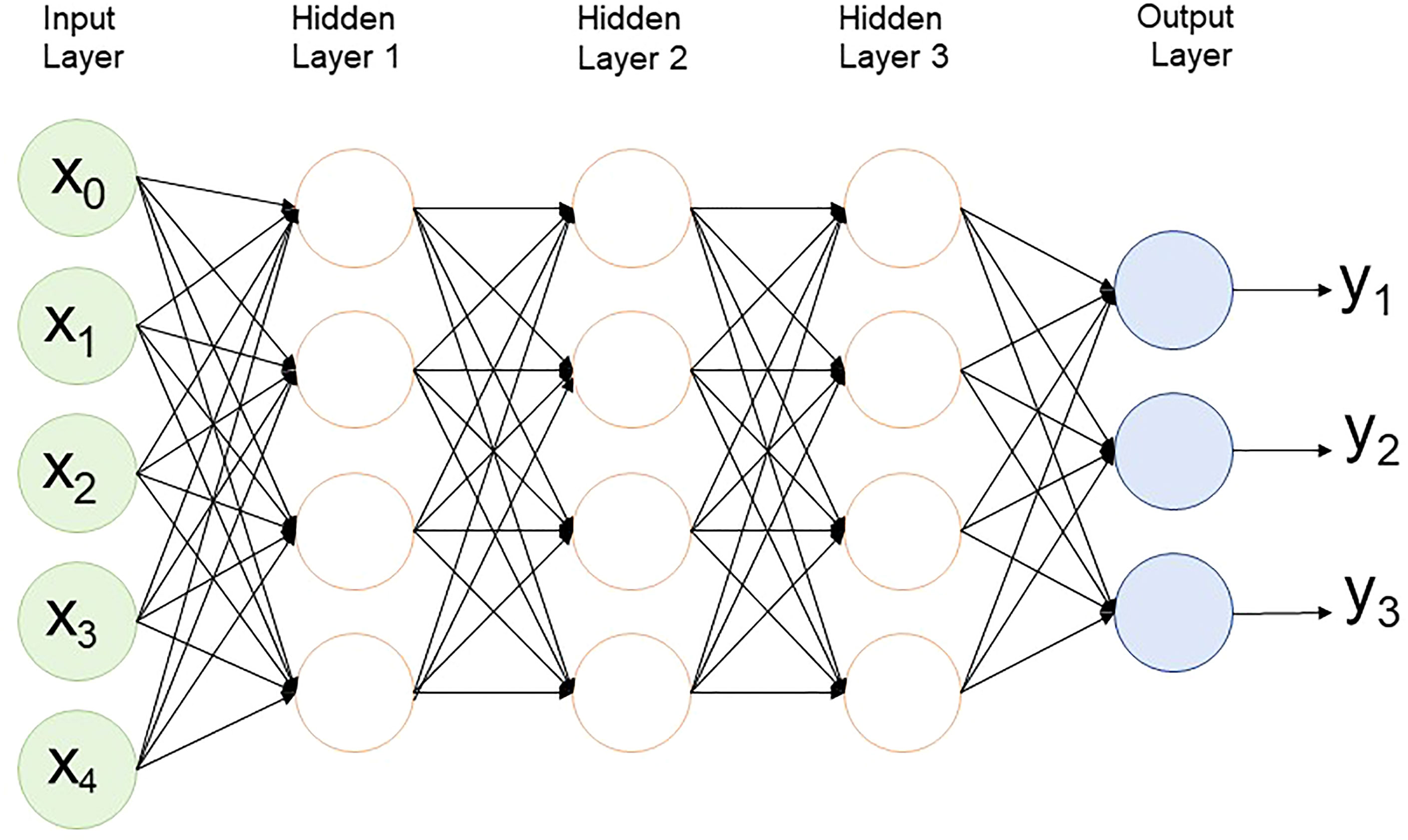
Figure 4 Multilayer perceptron structure with 3 hidden layers.
CNNs are used in visual recognition tasks involving images or video data. CNNs reduce the size of input and parameter sharing because the inputs are only partially connected to some neurons. Therefore, it is efficient because it reduces the parameters that need to be estimated. Most CNNs include three operations: convolution, non-linear transformation, and pooling ( Figure 5 ). This process allows you to reduce the input size without losing relevant information. In addition, the training time can be decreased by reducing the parameters ( Pook et al., 2020 ).
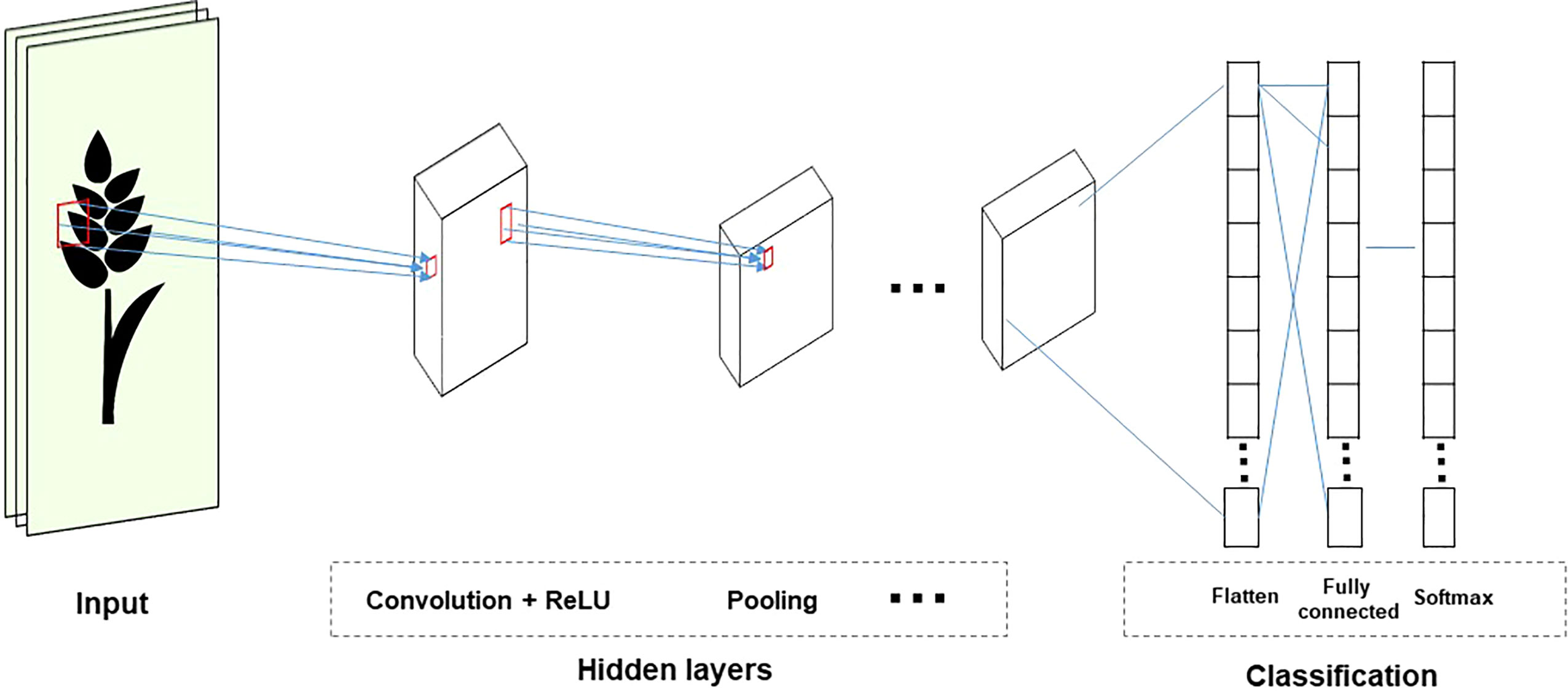
Figure 5 The structure of the CNN topology.
RNNs do not always travel in one direction, as they can be fed back to previous layers via synaptic connections. At least one feedback loop exists because the signal travels in both directions. Although the training parameters are reduced by sharing parameters across multiple steps, the short-term memory or latency of the network improves the performance, so training requires a lot of computational resources ( Montesinos-Lopez et al., 2021 ).
Although few GS programs use DL, DL is emerging as a promising tool. First, the reason is that the DL model efficiently processes the image’s raw data without any preprocessing. Second, it captures naturally genetic diversity without specifying additional terms for the predictors. This is important for non-additive effects or complex relationships and interactions that are important to capture the genetic merit of the whole. Third, topologies such as CNNs efficiently capture the LD of neighboring SNPs. Fourth, some topologies, such as CNNs, share parameters so that more parameters do not need to be estimated, reducing the number of parameters that need to be estimated. However, there are a few caveats to using DL in GS. It is more prone to overfitting than existing statistical models. Research results have reported that these problems can be solved with a Bayesian paradigm ( Neal, 1996 ). In addition, considerable knowledge is required to implement and output the DL model because it depends on the choice of many hyper-parameters and requires a very complex adjustment process.
The possibility that DLs can provide good predictive performance in GS has been suggested by several studies ( Table 3 ). However, it still shows a similar or lower level of predictive performance than the existing statistical models. More iterative and collaborative experiments are needed, and more data are needed to utilize DL in genome selection. In addition, the data should include not only the phenotype but also various types of omics data, climate data, and experience data of breeders. Then we need to design an efficient topology for the DL model ( Montesinos-Lopez et al., 2021 ).
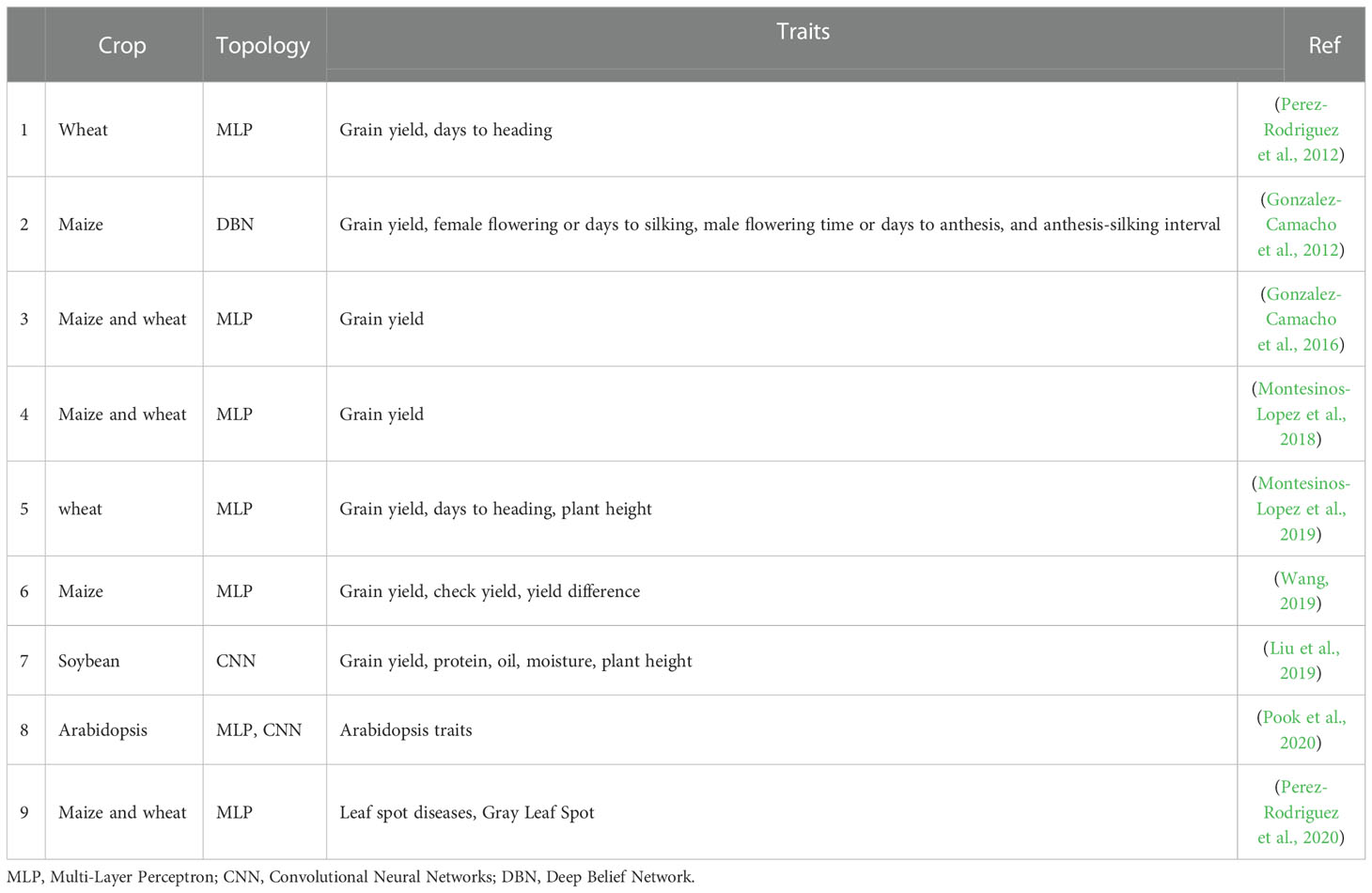
Table 3 Deep learning application to GS in plants.
4. Conclusion
Plant breeding has steadily increased crop productivity by developing superior varieties to support a growing population. Due to recent global environmental changes such as global warming, resource depletion, outbreaks of pests and diseases, and diversification of consumer demands, the role of plant breeding attracts much attention. Traditional breeding has developed dramatically and contributed to increased crop production, yield, and improved nutrition. Traditional breeding has created modern cultivars since the 20th century and has achieved great success in productivity. However, it is insufficient to meet the demand for crop production accompanied by exponential population growth. Breeding methods based on the traditional phenotypic selection are ineffective for low heritability and multi-genic quantitative traits (biological and abiotic stress, yield, and quality) because these traits are greatly influenced by the interaction between genes and the environment. Moreover, traditional breeding methods are time-consuming, laborious, and ineffective for cost and land use. In addition, low reliability and accuracy make these breeding methods less efficient. Accordingly, a new breeding method is required to quickly and accurately breed crops with high yield, good quality, and climate resilience. Researchers successfully established biotechnology-based molecular breeding technology to overcome the limitations of traditional breeding technology. They are now putting genome breeding technology into practice, also called predictive breeding. In summary, the history of plant breeding developed from traditional to molecular breeding. Predictive breeding will be available in the near future.
Rapid development in advanced technologies such as biotechnology, genomics, and phenomics improves progress in plant breeding. Breeders in the 21st century create mutations that directly correct target genes and expand the limits of genetic resources indefinitely by transcending the category of sexual reproduction with transformation technology. Selection in a population or lineage can rapidly and accurately fix the desired genotype using genomic information.
Integrating novel digital tools will be valuable and helpful in enhancing breeding progress, particularly for difficult-to-breed, quantitative traits. Vast genetic, genomic, phenotypic, and environmental data must be integrated and handled based on digital technology to fulfill the breeding technologies. In the current article, we first defined the term digital breeding, including breeding technologies from molecular to predictive breeding. For digital-based breeding technology to be commercialized, gene and genome information related to traits for each crop must be identified. It is expected that digital breeding can make it possible to reflect complex climates, geography, quantitative traits, and multiple traits in the breeding process. In a broad sense, therefore, digital breeding is not a single but a convergence technology. It can be defined as various attempts to actively utilize advanced technologies such as big data and artificial intelligence for agricultural breeding. However, as common confusion with new concepts in the early days of establishment, each researcher may have different ideas about digital breeding depending on their research field, research experience, and breeding resources. Particularly, some researchers think that DL-based techniques in breeding can become a game changer based on the results that DL has shown in various fields so far, so only DL-based techniques may be considered digital breeding. The different perceptions of digital breeding by researchers may cause confusion in the planning and promotion of related R&D. To address the problem, we first listened to the opinions of many researchers working with various breeding technologies. Consequently, we could classify the breeding level based on the intensity of digitalization. As a solution, we suggest that if digital breeding is divided like the autonomous driving levels in automobiles, it will be possible to easily organize the differences in thinking about digital breeding. Accordingly, we divided digital breeding into six phases. Phase 0 is traditional breeding that does not use digital technology and includes cross-breeding and mutation breeding. Phase 1 refers to identifying and processing substantial marker data generated by the development of genome sequencing technology. In Phase 2, markers are developed using bioinformatics tools. It is advantageous to select markers associated with qualitative traits using GWAS. Marker selection using GWAS has been actively carried out, but it is difficult to apply it to breeding directly. On the other hand, Phase 3 shows the potential to be practically applied to breeding. In this phase, the basis for practical application to breeding was laid by calculating the GEBVs using genomic information. Research based on GS started digital breeding. Although GS research has been actively conducted on livestock, it is still lacking in plants. Predictive statistical models for GS have been used in BLUP, LASSO, Bayesian, and ML-based models have also been applied. In Phase 4, breeding mainly uses DL to predict the phenotype by considering factors that affect plants, such as the environment. Some studies have been carried out by applying DL to plants, but it is still incomplete due to accuracy and technical problems. It looks like digital breeding, in the narrow sense, refers to this phase. In Phase 5, all processes from breeding design to phenotype prediction are automatically performed using DL. It has not yet been studied and is the ultimate goal for digital breeding techniques to evolve ( Table 4 ). More phases can be added if new technologies emerge in the future.
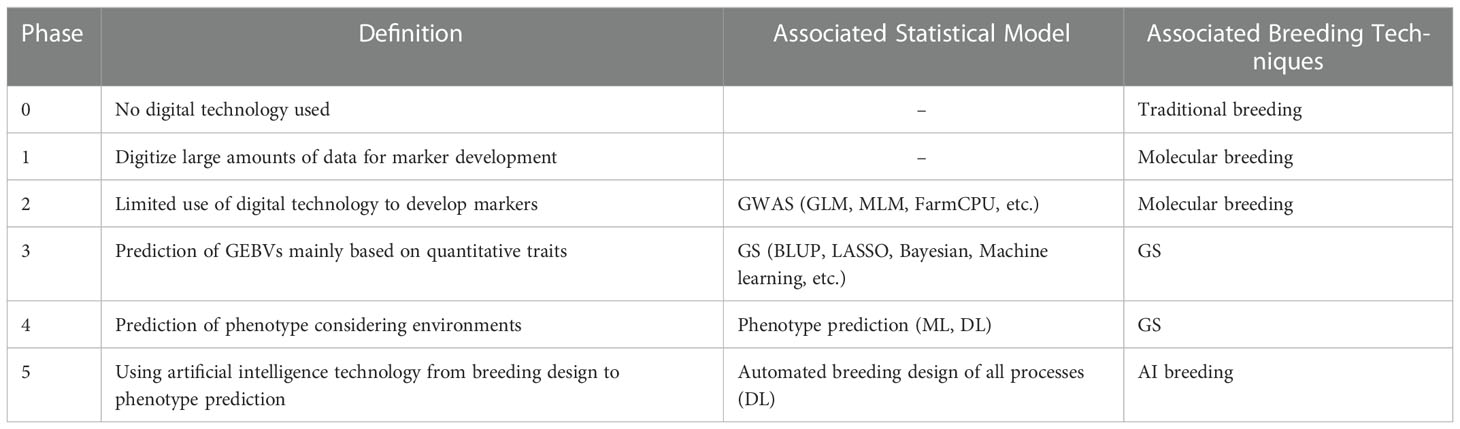
Table 4 Phases of digital breeding defined in the current review.
Author contributions
CK, T-HL, and DJ designed and structured the review, DJ, YK, SL, SC, and YS collected the information, organized the figures and tables, and wrote the original draft. CK and T-HL reviewed the manuscript. DJ edited and wrote the manuscript. All authors contributed to the article and approved the submitted version.
This work was carried out with the support of “Cooperative Research Program for Agriculture Science and Technology Development (Project No. PJ01427602)” Rural Development Administration, Republic of Korea.
Conflict of interest
The authors declare that the research was conducted in the absence of any commercial or financial relationships that could be construed as a potential conflict of interest.
Publisher’s note
All claims expressed in this article are solely those of the authors and do not necessarily represent those of their affiliated organizations, or those of the publisher, the editors and the reviewers. Any product that may be evaluated in this article, or claim that may be made by its manufacturer, is not guaranteed or endorsed by the publisher.
Abbaspour-Gilandeh, Y., Molaee, A., Sabzi, S., Nabipur, N., Shamshirband, S., Mosavi, A. (2020). A combined method of image processing and artificial neural network for the identification of 13 Iranian rice cultivars. Agronomy-Basel 10 (1). doi: 10.3390/agronomy10010117
PubMed Abstract | CrossRef Full Text | Google Scholar
Abdollahi-Arpanahi, L. R., Gianola, D., Penagaricano, F. (2020). Deep learning versus parametric and ensemble methods for genomic prediction of complex phenotypes. Genet. Sel Evol. 52 (1). doi: 10.1186/s12711-020-00531-z
CrossRef Full Text | Google Scholar
Abdulmalik, R. O., Menkir, A., Meseka, S. K., Unachukwu, N., Ado, S. G., Olarewaju, J. D., et al. (2017). Genetic gains in grain yield of a maize population improved through marker assisted recurrent selection under stress and non-stress conditions in West Africa. Front. Plant Sci. 8. doi: 10.3389/fpls.2017.00841
Acquaah, G. (2009). Principles of plant genetics and breeding (John Wiley & Sons), 455–458.
Google Scholar
Acquaah, G. (2015). Conventional plant breeding principles and techniques. advances in plant breeding strategies: Breeding, biotechnology and molecular tools (Springer), 115–158.
Adisa, O. M., Botai, J. O., Adeola, A. M., Hassen, A., Botai, C. M., Darkey, D., et al. (2019). Application of artificial neural network for predicting maize production in south Africa. Sustainability-Basel 11 (4). doi: 10.3390/su11041145
Akdemir, D., Isidro-Sanchez, J. (2019). Design of training populations for selective phenotyping in genomic prediction. Sci. Rep-Uk. 9, 1446. doi: 10.1038/s41598-018-38081-6
Akdemir, D., Sanchez, J. I., Jannink, J. L. (2015). Optimization of genomic selection training populations with a genetic algorithm. Genet. Sel Evol. 47. doi: 10.1186/s12711-015-0116-6
Allard, R. (1960). Principles of plant breeding Vol. 485 (John Willey and Sons).
Alqudah, A. M., Sallam, A., Baenziger, P. S., Borner, A. (2020). GWAS: Fast-forwarding gene identification and characterization in temperate cereals: lessons from barley - a review. J. Adv. Res. 22, 119–135. doi: 10.1016/j.jare.2019.10.013
Alvarez, R. (2009). Predicting average regional yield and production of wheat in the Argentine pampas by an artificial neural network approach. Eur. J. Agron. 30 (2), 70–77. doi: 10.1016/j.eja.2008.07.005
Bankole, F., Menkir, A., Olaoye, G., Crossa, J., Hearne, S., Unachukwu, N., et al. (2017). Genetic gains in yield and yield related traits under drought stress and favorable environments in a maize population improved using marker assisted recurrent selection. Front. Plant Sci. 8. doi: 10.3389/fpls.2017.00808
Basnet, B. R., Crossa, J., Dreisigacker, S., Perez-Rodriguez, P., Manes, Y., Singh, R. P., et al. (2019). Hybrid wheat prediction using genomic, pedigree, and environmental covariables interaction models. Plant Genome-Us 12 (1). doi: 10.3835/plantgenome2018.07.0051
Bernardo, R. (2008). Molecular markers and selection for complex traits in plants: Learning from the last 20 years. Crop Sci. 48 (5), 1649–1664. doi: 10.2135/cropsci2008.03.0131
Bhat, J. A., Ali, S., Salgotra, R. K., Mir, Z. A., Dutta, S., Jadon, V., et al. (2016). Genomic selection in the era of next generation sequencing for complex traits in plant breeding. Front. Genet. 7. doi: 10.3389/fgene.2016.00221
Bhat, J. A., Salgotra, R. K., Dar, Y. (2015). Phenomics: A challenge for crop improvement in genomic era. Mol. Plant Breeding. 6 (20), 1–11. doi: 10.5376/mpb.2015.06.0022
Borojevic, S. (1990). Principles and methods of plant breeding (Elsevier) 251–287.
Bradshaw, J. E. (2017). Plant breeding: past, present and future. Euphytica 213 (3). doi: 10.1007/s10681-016-1815-y
Briggs, F., Knowles, P. (1967). Introduction to plant breeding (New York: Reinhold Publishing Corporation).
Brown, J., Caligari, P. (2011). An introduction to plant breeding (John Wiley & Sons) 120–142.
Budhlakoti, N., Kushwaha, A. K., Rai, A., Chaturvedi, K. K., Kumar, A., Pradhan, A. K., et al. (2022). Genomic selection: A tool for accelerating the efficiency of molecular breeding for development of climate-resilient crops. Front. Genet. 13. doi: 10.3389/fgene.2022.832153
Budhlakoti, N., Rai, A., Mishra, D. C. (2020). Statistical approach for improving genomic prediction accuracy through efficient diagnostic measure of influential observation. Sci. Rep-Uk. 10 (1). doi: 10.1038/s41598-020-65323-3
Bustos-Korts, D., Malosetti, M., Chapman, S., Biddulph, B., van Eeuwijk, F. (2016). Improvement of predictive ability by uniform coverage of the target genetic space. G3-Genes Genom Genet. 6 (11), 3733–3747. doi: 10.1534/g3.116.035410
Collard, B. C. Y., Mackill, D. J. (2008). Marker-assisted selection: an approach for precision plant breeding in the twenty-first century. Philos. T R Soc. B. 363 (1491), 557–572. doi: 10.1098/rstb.2007.2170
Combs, E., Bernardo, R. (2013). Accuracy of genomewide selection for different traits with constant population size, heritability, and number of markers. Plant Genome-Us. 6 (1). doi: 10.3835/plantgenome2012.11.0030
Cooper, M., Smith, O. S., Graham, G., Arthur, L., Feng, L. Z., Podlich, D. W. (2004). Genomics, genetics, and plant breeding: A private sector perspective. Crop Sci. 44 (6), 1907–1913. doi: 10.2135/cropsci2004.1907
Cordell, H. J. (2002). Epistasis: what it means, what it doesn't mean, and statistical methods to detect it in humans. Hum. Mol. Genet. 11 (20), 2463–2468. doi: 10.1093/hmg/11.20.2463
Crain, J., Mondal, S., Rutkoski, J., Singh, R. P., Poland, J. (2018). Combining high-throughput phenotyping and genomic information to increase prediction and selection accuracy in wheat breeding. Plant Genome-Us. 11 (1). doi: 10.3835/plantgenome2017.05.0043
Crossa, J., de los Campos, G., Perez, P., Gianola, D., Burgueno, J., Araus, J. L., et al. (2010). Prediction of genetic values of quantitative traits in plant breeding using pedigree and molecular markers. Genetics. 186 (2), 713–U406. doi: 10.1534/genetics.110.118521
Crossa, J., Perez-Rodriguez, P., Cuevas, J., Montesinos-Lopez, O., Jarquin, D., de los Campos, G., et al. (2017). Genomic selection in plant breeding: Methods, models, and perspectives. Trends Plant Sci. 22 (11), 961–975. doi: 10.1016/j.tplants.2017.08.011
de los Campos, G., Gianola, D., Rosa, G. J. (2009). Reproducing kernel Hilbert spaces regression: a general framework for genetic evaluation. J. Anim. Sci. 87 (6), 1883–1887. doi: 10.2527/jas.2008-1259
De Los Campos, G., Vazquez, A. I., Fernando, R., Klimentidis, Y. C., Sorensen, D. (2013). Prediction of complex human traits using the genomic best linear unbiased predictor. PLoS Genet. 9 (7), e1003608. doi: 10.1371/journal.pgen.1003608
Deng, S. W., Caddell, D. F., Xu, G., Dahlen, L., Washington, L., Yang, J. L., et al. (2021). Genome wide association study reveals plant loci controlling heritability of the rhizosphere microbiome. Isme J. 15 (11), 3181–3194. doi: 10.1038/s41396-021-00993-z
Dubey, B. P., Bhagwat, S. G., Shouche, S. P., Sainis, J. K. (2006). Potential of artificial neural networks in varietal identification using morphometry of wheat grains. Biosyst. Eng. 95 (1), 61–67. doi: 10.1016/j.biosystemseng.2006.06.001
Eggink, P. M., Maliepaard, C., Tikunov, Y., Haanstra, J. P. W., Pohu-Flament, L. M. M., de Wit-Maljaars, S. C., et al. (2012). Prediction of sweet pepper (Capsicum annuum) flavor over different harvests. Euphytica. 187 (1), 117–131. doi: 10.1007/s10681-012-0761-6
El-Assal, S. E. D., Alonso-Blanco, C., Peeters, A. J. M., Raz, V., Koornneef, M. (2001). A QTL for flowering time in arabidopsis reveals a novel allele of CRY2. Nat. Genet. 29 (4), 435–440. doi: 10.1038/ng767
Fan, J. L., Zheng, J., Wu, L. F., Zhang, F. C. (2021). Estimation of daily maize transpiration using support vector machines, extreme gradient boosting, artificial and deep neural networks models. Agr Water Manage. 245. doi: 10.1016/j.agwat.2020.106547
Fridman, E., Pleban, T., Zamir, D. (2000). A recombination hotspot delimits a wild-species quantitative trait locus for tomato sugar content to 484 bp within an invertase gene. P Natl. Acad. Sci. U.S.A. 97 (9), 4718–4723. doi: 10.1073/pnas.97.9.4718
Friedman, J., Hastie, T., Tibshirani, R. (2010). Regularization paths for generalized linear models via coordinate descent. J. Stat. Software 33 (1), 1. doi: 10.18637/jss.v033.i01
Gelman, A., Carlin, J. B., Stern, H. S., Rubin, D. B. (1995). Bayesian Data analysis (Chapman and Hall/CRC) 20–29.
Gholipoor, M., Nadali, F. (2019). Fruit yield prediction of pepper using artificial neural network. Sci. Hortic-Amsterdam. 250, 249–253. doi: 10.1016/j.scienta.2019.02.040
Gianola, D. (2013). Priors in whole-genome regression: the Bayesian alphabet returns. Genetics. 194 (3), 573–596. doi: 10.1534/genetics.113.151753
Gianola, D., de Los Campos, G., Hill, W. G., Manfredi, E., Fernando, R. (2009). Additive genetic variability and the Bayesian alphabet. Genetics. 183 (1), 347–363. doi: 10.1534/genetics.109.103952
Gianola, D., Fernando, R. L., Stella, A. (2006). Genomic-assisted prediction of genetic value with semiparametric procedures. Genetics. 173 (3), 1761–1776. doi: 10.1534/genetics.105.049510
Gianola, D., Okut, H., Weigel, K. A., Rosa, G. J. M. (2011). Predicting complex quantitative traits with Bayesian neural networks: a case study with Jersey cows and wheat. BMC Genet. 12. doi: 10.1186/1471-2156-12-87
Gianola, D., van Kaam, JBCHM. (2008). Reproducing kernel Hilbert spaces regression methods for genomic assisted prediction of quantitative traits. Genetics. 178 (4), 2289–2303. doi: 10.1534/genetics.107.084285
Gjedrem, T., Thodesen, J. (2005). Selection and breeding programs in aquaculture (Springer), 89–111.
Goddard, M. (2009). Genomic selection: prediction of accuracy and maximisation of long term response. Genetica. 136 (2), 245–257. doi: 10.1007/s10709-008-9308-0
Godfray, H. C. J. (2014). “How can 9–10 billion people be fed sustainably and equitably by 2050?,” in Goldin, I (ed.), Is the Planet Full? (Oxford, 2014; online edn, Oxford Academic).
Goldberger, A. S. (1962). Best linear unbiased prediction in the generalized linear regression model. J. Am. Stat. Assoc. 57 (298), 369–375. doi: 10.1080/01621459.1962.10480665
Gómez, G. (2011). Association mapping, a method to detect quantitative trait loci: statistical bases. Agronomía Colombiana. 29 (3), 367–376.
Gonzalez-Camacho, J. M., Crossa, J., Perez-Rodriguez, P., Ornella, L., Gianola, D. (2016). Genome-enabled prediction using probabilistic neural network classifiers. BMC Genomics 17. doi: 10.1186/s12864-016-2553-1
Gonzalez-Camacho, J. M., de los Campos, G., Perez, P., Gianola, D., Cairns, J. E., Mahuku, G., et al. (2012). Genome-enabled prediction of genetic values using radial basis function neural networks. Theor. Appl. Genet. 125 (4), 759–771. doi: 10.1007/s00122-012-1868-9
Gonzalez-Camacho, J. M., Ornella, L., Perez-Rodriguez, P., Gianola, D., Dreisigacker, S., Crossa, J. (2018). Applications of machine learning methods to genomic selection in breeding wheat for rust resistance. Plant Genome-Us. 11 (2). doi: 10.3835/plantgenome2017.11.0104
Gonzalez-Recio, O., Weigel, K. A., Gianola, D., Naya, H., Rosa, G. J. M. (2010). L-2-Boosting algorithm applied to high-dimensional problems in genomic selection. Genet. Res. 92 (3), 227–237. doi: 10.1017/S0016672310000261
Gorjanc, G., Cleveland, M. A., Houston, R. D., Hickey, J. M. (2015). Potential of genotyping-by-sequencing for genomic selection in livestock populations. Genet. Sel Evol. 47. doi: 10.1186/s12711-015-0102-z
Greener, J. G., Kandathil, S. M., Moffat, L., Jones, D. T. (2022). A guide to machine learning for biologists. Nat. Rev. Mol. Cell Bio. 23 (1), 40–55. doi: 10.1038/s41580-021-00407-0
Habier, D., Fernando, R. L., Dekkers, J. C. M. (2009). Genomic selection using low-density marker panels. Genetics. 182 (1), 343–353. doi: 10.1534/genetics.108.100289
Habier, D., Fernando, R. L., Kizilkaya, K., Garrick, D. J. (2011). Extension of the bayesian alphabet for genomic selection. BMC Bioinf. 12 (1), 186. doi: 10.1186/1471-2105-12-186
Habier, D., Tetens, J., Seefried, F. R., Lichtner, P., Thaller, G. (2010). The impact of genetic relationship information on genomic breeding values in German Holstein cattle. Genet. Sel Evol. 42. doi: 10.1186/1297-9686-42-5
Haley, C. S., Knott, S. A. (1992). A simple regression method for mapping quantitative trait loci in line crosses using flanking markers. Heredity. 69, 315–324. doi: 10.1038/hdy.1992.131
Hayes, B. J., Pryce, J., Chamberlain, A. J., Bowman, P. J., Goddard, M. E. (2010). Genetic architecture of complex traits and accuracy of genomic prediction: Coat colour, milk-fat percentage, and type in Holstein cattle as contrasting model traits. PLoS Genet. 6 (9), e1001139. doi: 10.1371/journal.pgen.1001139
Heffner, E. L., Sorrells, M. E., Jannink, J. L. (2009). Genomic selection for crop improvement. Crop Sci. 49 (1), 1–12. doi: 10.2135/cropsci2008.08.0512
Henderson, C. R. (1975). Best linear unbiased estimation and prediction under a selection model. Biometrics. 31 (2), 423–447. doi: 10.2307/2529430
Heslot, N., Yang, H. P., Sorrells, M. E., Jannink, J. L. (2012). Genomic selection in plant breeding: a comparison of models. Crop Sci. 52 (1), 146–160. doi: 10.2135/cropsci2011.06.0297
Hoerl, A. E., Kennard, R. W. (1970). Ridge regression: applications to nonorthogonal problems. Technometrics. 12 (1), 69–82. doi: 10.1080/00401706.1970.10488635
Holliday, J. A., Wang, T. L., Aitken, S. (2012). Predicting adaptive phenotypes from multilocus genotypes in sitka spruce (Picea sitchensis) using random forest. G3-Genes Genom Genet. 2 (9), 1085–1093. doi: 10.1534/g3.112.002733
Hospital, F. (2009). Challenges for effective marker-assisted selection in plants. Genetica. 136 (2), 303–310. doi: 10.1007/s10709-008-9307-1
Howard, R., Carriquiry, A. L., Beavis, W. D. (2014). Parametric and nonparametric statistical methods for genomic selection of traits with additive and epistatic genetic architectures. G3-Genes Genom Genet. 4 (6), 1027–1046. doi: 10.1534/g3.114.010298
Huang, L. S., Ding, W. J., Liu, W. J., Zhao, J. L., Huang, W. J., Xu, C., et al. (2019). Identification of wheat powdery mildew using in-situ hyperspectral data and linear regression and support vector machines. J. Plant Pathol. 101 (4), 1035–1045. doi: 10.1007/s42161-019-00334-2
Huang, L. S., Wu, Z. C., Huang, W. J., Ma, H. Q., Zhao, J. L. (2019). Identification of fusarium head blight in winter wheat ears based on fisher's linear discriminant analysis and a support vector machine. Appl. Sci-Basel. 9 (18). doi: 10.3390/app9183894
Ibrahim, A. K., Zhang, L. W., Niyitanga, S., Afzal, M. Z., Xu, Y., Zhang, L. L., et al. (2020). Principles and approaches of association mapping in plant breeding. Trop. Plant Biol. 13 (3), 212–224. doi: 10.1007/s12042-020-09261-4
Jannink, J. L., Lorenz, A. J., Iwata, H. (2010). Genomic selection in plant breeding: from theory to practice. Brief Funct. Genomics 9 (2), 166–177. doi: 10.1093/bfgp/elq001
Jarquin, D., Crossa, J., Lacaze, X., Du Cheyron, P., Daucourt, J., Lorgeou, J., et al. (2014). A reaction norm model for genomic selection using high-dimensional genomic and environmental data. Theor. Appl. Genet. 127 (3), 595–607. doi: 10.1007/s00122-013-2243-1
Kearsey, M. J. B. (1997). Genetic resources and plant breeding (Biotechnology and plant genetic resources), 175, 77–100.
Kearsey, M. J., Hyne, V. (1994). Qtl analysis - a simple marker-regression approach. Theor. Appl. Genet. 89 (6), 698–702. doi: 10.1007/BF00223708
Khush, G. S. (2000). Sequence tagged site marker-assisted selection for three bacterial blight resistance genes in rice. Crop Sci. 40 (3), 792–797. doi: 10.2135/cropsci2000.403792x
Kurtulmus, F., Kavdir, I. (2014). Detecting corn tassels using computer vision and support vector machines. Expert Syst. Appl. 41 (16), 7390–7397. doi: 10.1016/j.eswa.2014.06.013
Kusmec, A. P. S. S. (2018). Farm CPU pp: efficient large-scale genomewide association studies. Plant Direct. 2 (4), e00053. doi: 10.1002/pld3.53
Lado, B., Matus, I., Rodriguez, A., Inostroza, L., Poland, J., Belzile, F., et al. (2013). Increased genomic prediction accuracy in wheat breeding through spatial adjustment of field trial data. G3-Genes Genom Genet. 3 (12), 2105–2114. doi: 10.1534/g3.113.007807
LeCun, Y., Bengio, Y., Hinton, G. (2015). Deep learning. Nature. 521 (7553), 436–444. doi: 10.1038/nature14539
Legarra, A., Robert-Granié, C., Croiseau, P., Guillaume, F., Fritz, S. (2011). Improved lasso for genomic selection. Genet. Res. 93 (1), 77–87. doi: 10.1017/S0016672310000534
Li, Z., Sillanpää, M. J. (2012). Overview of LASSO-related penalized regression methods for quantitative trait mapping and genomic selection. Theor. Appl. Genet. 125 (3), 419–435. doi: 10.1007/s00122-012-1892-9
Liu, X., Huang, M., Fan, B., Buckler, E. S., Zhang, Z. (2016). Iterative usage of fixed and random effect models for powerful and efficient genome-wide association studies (vol 12, e1005767, 2016). PLoS One 11 (3). doi: 10.1371/journal.pgen.1005767
Liu, Y., Wang, D. L., He, F., Wang, J. X., Joshi, T., Xu, D. (2019). Phenotype prediction and genome-wide association study using deep convolutional neural network of soybean. Front. Genet. 10. doi: 10.3389/fgene.2019.01091
Li, B., Zhang, N. X., Wang, Y. G., George, A. W., Reverter, A., Li, Y. T. (2018). Genomic prediction of breeding values using a subset of SNPs identified by three machine learning methods. Front. Genet. 9. doi: 10.3389/fgene.2018.00237
Long, N., Gianola, D., Rosa, G. J. M., Weigel, K. A. (2011). Application of support vector regression to genome-assisted prediction of quantitative traits. Theor. Appl. Genet. 123 (7), 1065–1074. doi: 10.1007/s00122-011-1648-y
Lopez-Calderon, M. J., Estrada-Avalos, J., Rodriguez-Moreno, V. M., Mauricio-Ruvalcaba, J. E., Martinez-Sifuentes, A. R., Delgado-Ramirez, G., et al. (2020). Estimation of total nitrogen content in forage maize (Zea mays l.) using spectral indices: Analysis by random forest. Agriculture-Basel 10 (10). doi: 10.3390/agriculture10100451
Lopez-Cruz, M., Crossa, J., Bonnett, D., Dreisigacker, S., Poland, J., Jannink, J. L., et al. (2015). Increased prediction accuracy in wheat breeding trials using a marker x environment interaction genomic selection model. G3-Genes Genom Genet. 5 (4), 569–582. doi: 10.1534/g3.114.016097
Lorenz, K., Cohen, B. A. (2012). Small- and Large-effect quantitative trait locus interactions underlie variation in yeast sporulation efficiency. Genetics. 192 (3), 1123–1132. doi: 10.1534/genetics.112.143107
Lorenz, A. J., Smith, K. P. (2015). Adding genetically distant individuals to training populations reduces genomic prediction accuracy in barley. Crop Sci. 55 (6), 2657–2667. doi: 10.2135/cropsci2014.12.0827
Mebratie, W., Reyer, H., Wimmers, K., Bovenhuis, H., Jensen, J. (2019). Genome wide association study of body weight and feed efficiency traits in a commercial broiler chicken population, a re-visitation. Sci. Rep-Uk. 9. doi: 10.1038/s41598-018-37216-z
Metzker, M. L. (2010). APPLICATIONS OF NEXT-GENERATION SEQUENCING sequencing technologies - the next generation. Nat. Rev. Genet. 11 (1), 31–46. doi: 10.1038/nrg2626
Meuwissen, T. H. E., Hayes, B. J., Goddard, M. E. (2001). Prediction of total genetic value using genome-wide dense marker maps. Genetics. 157 (4), 1819–1829. doi: 10.1093/genetics/157.4.1819
Mila, A. L., Ngugi, H. K. (2011). A Bayesian approach to meta-analysis of plant pathology studies. Phytopathology 101 (1), 42–51. doi: 10.1094/PHYTO-03-10-0070
Mokhtar, U., Ali, M. A. S., Hassanien, A. E., Hefny, H. (2015). Identifying two of tomatoes leaf viruses using support vector machine. Adv. Intell. Syst. 339, 771–782. doi: 10.1007/978-81-322-2250-7_77
Montesinos-Lopez, O. A., Martin-Vallejo, J., Crossa, J., Gianola, D., Hernandez-Suarez, C. M., Montesinos-Lopez, A., et al. (2019). A benchmarking between deep learning, support vector machine and Bayesian threshold best linear unbiased prediction for predicting ordinal traits in plant breeding. G3-Genes Genom Genet. 9 (2), 601–618. doi: 10.1534/g3.118.200998
Montesinos-Lopez, A., Montesinos-Lopez, O. A., Gianola, D., Crossa, J., Hernandez-Suarez, C. M. (2018). Multi-environment genomic prediction of plant traits using deep learners with dense architecture. G3-Genes Genom Genet. 8 (12), 3813–3828. doi: 10.1534/g3.118.200740
Montesinos-Lopez, O. A., Montesinos-Lopez, A., Perez-Rodriguez, P., Barron-Lopez, J. A., Martini, J. W. R., Fajardo-Flores, S. B., et al. (2021). A review of deep learning applications for genomic selection. BMC Genomics 22 (1). doi: 10.1186/s12864-020-07319-x
Moore, J. H., Williams, S. M. (2009). Epistasis and its implications for personal genetics. Am. J. Hum. Genet. 85 (3), 309–320. doi: 10.1016/j.ajhg.2009.08.006
Moose, S. P., Mumm, R. H. (2008). Molecular plant breeding as the foundation for 21st century crop improvement. Plant Physiol. 147 (3), 969–977. doi: 10.1104/pp.108.118232
Muranty, H., Jorge, V., Bastien, C., Lepoittevin, C., Bouffier, L., Sanchez, L. (2014). Potential for marker-assisted selection for forest tree breeding: lessons from 20 years of MAS in crops. Tree Genet. Genomes. 10 (6), 1491–1510. doi: 10.1007/s11295-014-0790-5
Murphy, K. P. (2012). Machine learning: A probabilistic perspective (The MIT Press), 516–523.
Mutlu, A. C., Boyaci, I. H., Genis, H. E., Ozturk, R., Basaran-Akgul, N., Sanal, T., et al. (2011). Prediction of wheat quality parameters using near-infrared spectroscopy and artificial neural networks. Eur. Food Res. Technol. 233 (2), 267–274. doi: 10.1007/s00217-011-1515-8
Myburg, A., Potts, B., Marques, C., Kirst, M., Gion, J., Grattapaglia, D., et al. (2007). Genome mapping and molecular breeding in plants Vol. 7. Ed. Kole, C. R. (New York: Springer, Forest trees).
Myles, S., Peiffer, J., Brown, P. J., Ersoz, E. S., Zhang, Z. W., Costich, D. E., et al. (2009). Association mapping: Critical considerations shift from genotyping to experimental design. Plant Cell. 21 (8), 2194–2202. doi: 10.1105/tpc.109.068437
Nadeem, M. A., Nawaz, M. A., Shahid, M. Q., Dogan, Y., Comertpay, G., Yildiz, M., et al. (2018). DNA Molecular markers in plant breeding: current status and recent advancements in genomic selection and genome editing. Biotechnol. Biotec Eq. 32 (2), 261–285. doi: 10.1080/13102818.2017.1400401
Nakano, Y. Y. K. (2020). Genome-wide association studies of agronomic traits consisting of field-and molecular-based phenotypes. Rev. Agric. Science. 8, 28–45. doi: 10.7831/ras.8.0_28
Neal, R. M. (1996). Bayesian Learning for neural networks (Springer) 99–143.
Noble, W. S. (2006). What is a support vector machine? Nat. Biotechnol. 24 (12), 1565–1567. doi: 10.1038/nbt1206-1565
Odilbekov, F., Armoniene, R., Koc, A., Svensson, J., Chawade, A. (2019). GWAS-assisted genomic prediction to predict resistance to septoria tritici blotch in Nordic winter wheat at seedling stage. Front. Genet. 10. doi: 10.3389/fgene.2019.01224
Ogutu, J. O. (2012). Genomic selection using regularized linear regression models: ridge regression, lasso, elastic net and their extensions. BMC Proc. 6, 10. doi: 10.1186/1753-6561-6-S2-S10
Ozaki, K., Ohnishi, Y., Iida, A., Sekine, A., Yamada, R., Tsunoda, T., et al. (2002). Functional SNPs in the lymphotoxin-alpha gene that are associated with susceptibility to myocardial infarction. Nat. Genet. 32 (4), 650–654. doi: 10.1038/ng1047
Pabuayon, I. L. B., Sun, Y. Z., Guo, W. X., Ritchie, G. L. (2019). High-throughput phenotyping in cotton: a review. J. Cotton Res. 2. doi: 10.1186/s42397-019-0035-0
Perez, P., de los Campos, G., Crossa, J., Gianola, D. (2010). Genomic-enabled prediction based on molecular markers and pedigree using the Bayesian linear regression package in r. Plant Genome-Us. 3 (2), 106–116. doi: 10.3835/plantgenome2010.04.0005
Perez-Rodriguez, P., Flores-Galarza, S., Vaquera-Huerta, H., del Valle-Paniagua, D. H., Montesinos-Lopez, O. A., Crossa, J. (2020). Genome-based prediction of Bayesian linear and non-linear regression models for ordinal data. Plant Genome-Us. 13 (2). doi: 10.1002/tpg2.20021
Perez-Rodriguez, P., Gianola, D., Gonzalez-Camacho, J. M., Crossa, J., Manes, Y., Dreisigacker, S. (2012). Comparison between linear and non-parametric regression models for genome-enabled prediction in wheat. G3-Genes Genom Genet. 2 (12), 1595–1605. doi: 10.1534/g3.112.003665
Piepho, H. P., Möhring, J., Melchinger, A. E., Büchse, A. (2008). BLUP for phenotypic selection in plant breeding and variety testing. Euphytica. 161 (1-2), 209–228. doi: 10.1007/s10681-007-9449-8
Poehlman, J., Sleper, DJBFC. (1995). Methods in plant breeding (Methods in Plant Breeding in Breeding Field Crops). 172–174.
Pook, T., Freudenthal, J., Korte, A., Simianer, H. (2020). Using local convolutional neural networks for genomic prediction. Front. Genet. 11. doi: 10.3389/fgene.2020.561497
Ragot, M., Biasiolli, M., Delbut, M. F., Dellorco, A., Malgarini, L., Thevenin, P., et al. (1995). Marker-assisted backcrossing: A practical example. Colloq Inra. , 45–56.
Ramos, A. P. M., Osco, L. P., Furuya, D. E. G., Goncalves, W. N., Santana, D. C., Teodoro, L. P. R., et al. (2020). A random forest ranking approach to predict yield in maize with uav-based vegetation spectral indices. Comput. Electron Agr 178, 105791. doi: 10.1016/j.compag.2020.105791
Razzaq, A., Kaur, P., Akhter, N., Wani, S. H., Saleem, F. (2021). Next-generation breeding strategies for climate-ready crops. Front. Plant Sci. 12. doi: 10.3389/fpls.2021.620420
Rezende, G., Resende, M., Assis, T., Fenning, T. (2014).
Rincent, R., Laloe, D., Nicolas, S., Altmann, T., Brunel, D., Revilla, P., et al. (2012). Maximizing the reliability of genomic selection by optimizing the calibration set of reference individuals: Comparison of methods in two diverse groups of maize inbreds (Zea mays l.). Genetics. 192 (2), 715–728. doi: 10.1534/genetics.112.141473
Rodriguez-Ramilo, S. T., Garcia-Cortes, L. A., Gonzalez-Recio, O. (2014). Combining genomic and genealogical information in a reproducing kernel Hilbert spaces regression model for genome-enabled predictions in dairy cattle. PLoS One 9 (3). doi: 10.1371/journal.pone.0093424
Roell, Y. E., Beucher, A., Moller, P. G., Greve, M. B., Greve, M. H. (2020). Comparing a random forest based prediction of winter wheat yield to historical yield potential. Agronomy-Basel. 10 (3). doi: 10.3390/agronomy10030395
Rosado, R. D. S., Cruz, C. D., Barili, L. D., Carneiro, J. E. D., Carneiro, P. C. S., Carneiro, V. Q., et al. (2020). Artificial neural networks in the prediction of genetic merit to flowering traits in bean cultivars. Agriculture-Basel 10 (12). doi: 10.3390/agriculture10120638
Savadi, S., Prasad, P., Kashyap, P. L., Bhardwaj, S. C. (2018). Molecular breeding technologies and strategies for rust resistance in wheat (Triticum aestivum) for sustained food security. Plant Pathol. 67 (4), 771–791. doi: 10.1111/ppa.12802
Semagn, K., Beyene, Y., Babu, R., Nair, S., Gowda, M., Das, B., et al. (2015). Quantitative trait loci mapping and molecular breeding for developing stress resilient maize for Sub-Saharan Africa. Crop Sci. 55 (4), 1449–1459. doi: 10.2135/cropsci2014.09.0646
Sethy, P. K., Barpanda, N. K., Rath, A. K., Behera, S. K. (2020). Deep feature based rice leaf disease identification using support vector machine. Comput. Electron Agr. 175. doi: 10.1016/j.compag.2020.105527
Shabannejad, M., Bihamta, M. R., Majidi-Hervan, E., Alipour, H., Ebrahimi, A. (2020). A simple, cost-effective high-throughput image analysis pipeline improves genomic prediction accuracy for days to maturity in wheat. Plant Methods 16 (1). doi: 10.1186/s13007-020-00686-2
Sharma, R. (2020). “Insights into marker assisted selection and its applications,” in Plant breeding : Current and future views (London: IntechOpen).
Shen, H. Z., Jiang, K. T., Sun, W. Q., Xu, Y., Ma, X. Y. (2021). Irrigation decision method for winter wheat growth period in a supplementary irrigation area based on a support vector machine algorithm. Comput. Electron Agr. 182. doi: 10.1016/j.compag.2021.106032
Spindel, J., Begum, H., Akdemir, D., Virk, P., Collard, B., Redona, E., et al. (2015). Genomic selection and association mapping in rice (Oryza sativa): Effect of trait genetic architecture, training population composition, marker number and statistical model on accuracy of rice genomic selection in elite, tropical rice breeding lines. PLoS Genet. 11 (2). doi: 10.1371/journal.pgen.1004982
Sun, J., Poland, J. A., Mondal, S., Crossa, J., Juliana, P., Singh, R. P., et al. (2019). High-throughput phenotyping platforms enhance genomic selection for wheat grain yield across populations and cycles in early stage. Theor. Appl. Genet. 132 (6), 1705–1720. doi: 10.1007/s00122-019-03309-0
Szymczak, S., Biernacka, J. M., Cordell, H. J., Gonzalez-Recio, O., Konig, I. R., Zhang, H. P., et al. (2009). Machine learning in genome-wide association studies. Genet. Epidemiol. 33, S51–SS7. doi: 10.1002/gepi.20473
Tewodros, M. B. Z. (2016). Advances in quantitative trait loci, mapping and importance of markers assisted selection in plant breeding. Int. J. Plant Breed. Genet. 10 (2), 58–68.
Tibshirani, R. (1996). Regression shrinkage and selection via the lasso. J. Roy Stat. Soc. B Met. 58 (1), 267–288. doi: 10.1111/j.2517-6161.1996.tb02080.x
Tigchelaar, E., Casali, V. (1976). “Single seed descent: applications and merits in breeding self-pollinated crops,” in I International Symposium on Floriculture Plant Breeding and Genetics, Vol. 63.
Tutz, G. (2009). Penalized regression with correlation-based penalty. Stat Computing 19 (3), 239–253. doi: 10.1007/s11222-008-9088-5
Wang, S. K. L. (2019). Crop Yield Prediction Using Deep Neural Networks. Front. Plant Sci. 10, 621. doi: 10.3389/fpls.2019.00621
Wang, Y. J., Xu, J., Deng, D. X., Ding, H. D., Bian, Y. L., Yin, Z. T., et al. (2016). A comprehensive meta-analysis of plant morphology, yield, stay-green, and virus disease resistance QTL in maize (Zea mays l.). Planta 243 (2), 459–471. doi: 10.1007/s00425-015-2419-9
Wang, X., Zhang, M., Zhu, J., Geng, S. (2008). Spectral prediction of phytophthora infestans infection on tomatoes using artificial neural network (ANN). Int. J. Remote Sens. 29 (6), 1693–1706. doi: 10.1080/01431160701281007
Whittaker, J. C., Thompson, R., Visscher, P. M. (1996). On the mapping of QTL by regression of phenotype on marker-type. Heredity. 77, 23–32. doi: 10.1038/hdy.1996.104
Wientjes, Y. C. J., Veerkamp, R. F., Calus, M. P. L. (2013). The effect of linkage disequilibrium and family relationships on the reliability of genomic prediction. Genetics. 193 (2), 621–631. doi: 10.1534/genetics.112.146290
Wojtowicz, A., Piekarczyk, J., Czernecki, B., Ratajkiewicz, H. (2021). A random forest model for the classification of wheat and rye leaf rust symptoms based on pure spectra at leaf scale. J. Photoch Photobio B. 223. doi: 10.1016/j.jphotobiol.2021.112278
Wu, Z. Y., Zhao, H. Y. (2009). Statistical power of model selection strategies for genome-wide association studies. PLoS Genet. 5 (7). doi: 10.1371/journal.pgen.1000582
Xu, S. Z. (2013). Genetic mapping and genomic selection using recombination breakpoint data. Genetics. 195 (3), 1103–1115. doi: 10.1534/genetics.113.155309
Xu, Y. B., Crouch, J. H. (2008). Marker-assisted selection in plant breeding: From publications to practice. Crop Sci. 48 (2), 391–407. doi: 10.2135/cropsci2007.04.0191
Yadav, N. R. (2021). High-throughput phenotyping: A platform to accelerate crop improvement. Phenomics volume 1, 31–53. doi: 10.1007/s43657-020-00007-6
Yano, M., Katayose, Y., Ashikari, M., Yamanouchi, U., Monna, L., Fuse, T., et al. (2000). Hd1, a major photoperiod sensitivity quantitative trait locus in rice, is closely related to the arabidopsis flowering time gene CONSTANS. Plant Cell. 12 (12), 2473–2483. doi: 10.1105/tpc.12.12.2473
Yoosefzadeh-Najafabadi, M., Rajcan, I., Eskandari, M. (2022). Optimizing genomic selection in soybean: An important improvement in agricultural genomics. Heliyon. 8 (11), e11873. doi: 10.1016/j.heliyon.2022.e11873
Zandalinas, S. I., Fritschi, F. B., Mittler, R. (2021). Global warming, climate change, and environmental pollution: Recipe for a multifactorial stress combination disaster. Trends Plant Sci. 26 (6), 588–599. doi: 10.1016/j.tplants.2021.02.011
Zeng, Z. B. (1994). Precision mapping of quantitative trait loci. Genetics. 136 (4), 1457–1468. doi: 10.1093/genetics/136.4.1457
Zhong, D., Novais, J., Grift, T. E., Bohn, M., Han, J. (2009). Maize root complexity analysis using a support vector machine method. Comput. Electron Agr. 69 (1), 46–50. doi: 10.1016/j.compag.2009.06.013
Keywords: QTLs, GWAS, MAS, genomic prediction, machine learning, deep learning, high throughput phenotyping, AI breeding
Citation: Jeon D, Kang Y, Lee S, Choi S, Sung Y, Lee T-H and Kim C (2023) Digitalizing breeding in plants: A new trend of next-generation breeding based on genomic prediction. Front. Plant Sci. 14:1092584. doi: 10.3389/fpls.2023.1092584
Received: 08 November 2022; Accepted: 05 January 2023; Published: 19 January 2023.
Reviewed by:
Copyright © 2023 Jeon, Kang, Lee, Choi, Sung, Lee and Kim. This is an open-access article distributed under the terms of the Creative Commons Attribution License (CC BY) . The use, distribution or reproduction in other forums is permitted, provided the original author(s) and the copyright owner(s) are credited and that the original publication in this journal is cited, in accordance with accepted academic practice. No use, distribution or reproduction is permitted which does not comply with these terms.
*Correspondence: Tae-Ho Lee, [email protected] ; Changsoo Kim, [email protected]
Disclaimer: All claims expressed in this article are solely those of the authors and do not necessarily represent those of their affiliated organizations, or those of the publisher, the editors and the reviewers. Any product that may be evaluated in this article or claim that may be made by its manufacturer is not guaranteed or endorsed by the publisher.

- Other Journals
- For Readers
- For Authors
- For Librarians
Announcements
- Thesis Abstracts
Journal of Plant Breeding and Genetics
Journal of Plant Breeding and Genetics is an open-access, peer-reviewed, international journal devoted to providing opportunities for original as well as extended versions of published researches. Journal of Plant Breeding and Genetics publishes original and review articles by internationally recognized researchers, covering all areas of plant genetics, genetics and related interface. The journal focuses on crop plants and is therefore of interest to seed companies and the plant breeding industry as well as to researchers and teachers in this field. Journal publishes original research covering all areas of theoretical and applied plant breeding and genetics. Journal is a periodical for the publication of records of original research in all branches of genetics, plant breeding and cytology, including molecular biology, biotechnology and other related sciences of sufficient importance and of such a character as to be of the primary interest to the geneticist and plant breeders.

No announcements have been published. | |
Vol 7, No 3 (2019): J. Plant Breed. Genet.
Table of contents.
Milletomics: a metabolomics centered integrated omics approach toward genetic progression
- Published: 02 September 2024
- Volume 24 , article number 149 , ( 2024 )
Cite this article
- Saikat Mazumder 1 , 2 ,
- Debasmita Bhattacharya 3 ,
- Dibyajit Lahiri 1 &
- Moupriya Nag 1
Producing alternative staple foods like millet will be essential to feeding ten billion people by 2050. The increased demand for millet is driving researchers to improve its genetic variation. Millets include protein, dietary fiber, phenolic substances, and flavonoid components. Its climate resilience makes millet an appealing crop for agronomic sustainability. Integrative omics technologies could potentially identify and develop millets with desirable phenotypes that may have high agronomic value. Millets’ salinity and drought tolerance have been enhanced using transcriptomics. In foxtail, finger, and pearl millet, proteomics has discovered salt-tolerant protein, phytohormone-focused protein, and drought tolerance. Metabolomics studies have revealed that certain metabolic pathways including those involving lignin, flavonoids, phenylpropanoid, and lysophospholipids are critical for many processes, including seed germination, photosynthesis, energy metabolism, and the synthesis of bioactive chemicals necessary for drought tolerance. Metabolomics integration with other omics revealed metabolome engineering and trait-specific metabolite creation. Integrated metabolomics and ionomics are still in the development stage, but they could potentially assist in comprehending the pathway of ionomers to control nutrient levels and biofortify millet. Epigenomic analysis has shown alterations in DNA methylation patterns and chromatin structure in foxtail and pearl millets in response to abiotic stress. Whole-genome sequencing utilizing next-generation sequencing is the most proficient method for finding stress-induced phytoconstituent genes. New genome sequencing enables novel biotechnological interventions including genome-wide association, mutation-based research, and other omics approaches. Millets can breed more effectively by employing next-generation sequencing and genotyping by sequencing, which may mitigate climate change. Millet marker-assisted breeding has advanced with high-throughput markers and combined genotyping technologies.
This is a preview of subscription content, log in via an institution to check access.
Access this article
Subscribe and save.
- Get 10 units per month
- Download Article/Chapter or eBook
- 1 Unit = 1 Article or 1 Chapter
- Cancel anytime
Price includes VAT (Russian Federation)
Instant access to the full article PDF.
Rent this article via DeepDyve
Institutional subscriptions
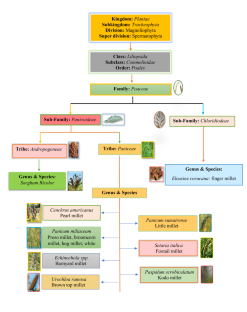
Similar content being viewed by others
Multiomics for Crop Improvement
Omics-Aided Crop Improvement in Foxtail Millet
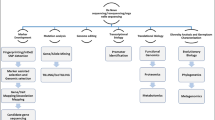
The potentialities of omics resources for millet improvement
Data availability.
No datasets were generated or analysed during the current study.
Agarwal G, Kudapa H, Ramalingam A et al (2020) Epigenetics and epigenomics: underlying mechanisms, relevance, and implications in crop improvement. Funct Integr Genomics 20:739–761. https://doi.org/10.1007/s10142-020-00756-7
Article PubMed CAS Google Scholar
Agtuca BJ, Stopka SA, Tuleski TR et al (2020) In-Situ metabolomic analysis of Setaria viridis roots colonized by beneficial endophytic Bacteria. Mol Plant-Micro Interact 33:272–283. https://doi.org/10.1094/MPMI-06-19-0174-R
Article CAS Google Scholar
Ahuja KG, Nath P, Swamy KRM (2010) Foods and Nutrition, 1st edn. Studium, New Delhi, India
Google Scholar
Ajeesh Krishna TP, Maharajan T, Ceasar SA (2022) Improvement of millets in the post-genomic era. Physio Mol Bio Plants 28:669–685. https://doi.org/10.1007/s12298-022-01158-8
Ambavane AR, Sawardekar SV, Sawantdesai SA, Gokhale NB (2015) Studies on mutagenic effectiveness and efficiency of gamma rays and its effect on quantitative traits in finger millet (Eleusine coracana L. Gaertn). J Radiat Res Appl Sci 8:120–125. https://doi.org/10.1016/j.jrras.2014.12.004
Article Google Scholar
Antony Ceasar S, Ignacimuthu S (2011) Agrobacterium-mediated transformation of finger millet (Eleusine coracana (L.) Gaertn.) Using shoot apex explants. Plant Cell Rep 30:1759–1770. https://doi.org/10.1007/s00299-011-1084-0
Ashraf MF, Hou D, Hussain Q et al (2022) Entailing the Next-Generation sequencing and metabolome for sustainable agriculture by improving Plant Tolerance. Int J Mol Sci 23:1–33. https://doi.org/10.3390/ijms23020651
Babu BK, Dinesh P, Agrawal PK et al (2014) Comparative Genomics and Association Mapping Approaches for Blast Resistant Genes in Finger Millet using SSRs. PLoS ONE 9:1–12. https://doi.org/10.1371/journal.pone.0099182
Baggett JP, Tillett RL, Cooper EA, Yerka MK (2021) De novo identification and targeted sequencing of SSRs efficiently fingerprints Sorghum bicolor sub-population identity. PLoS ONE 16:1–18. https://doi.org/10.1371/journal.pone.0248213
Banerjee S (2012) Finger millet (Eleusine coracana) polyphenols: investigation of their antioxidant capacity and antimicrobial activity. Afric J Food Sci 6:362–374. https://doi.org/10.5897/ajfs12.031
Banshidhar PS, Singh A et al (2023) The potentialities of omics resources for millet improvement. Funct Integr Genomics 23:210. https://doi.org/10.1007/s10142-023-01149-2
Bayer GYa, Yemets AI, Blume YB (2014) Obtaining the transgenic lines of finger millet Eleusine coracana (L.). With dinitroaniline resistance. Cytol Genet 48:139–144. https://doi.org/10.3103/S0095452714030025
Bekkering CS, Tian L (2019) Thinking outside of the cereal box: breeding underutilized (Pseudo)Cereals for Improved Human Nutrition. Front Genet 10:1–7. https://doi.org/10.3389/fgene.2019.01289
Bellato S, Ciccoritti R, Del Frate V et al (2013) Influence of genotype and environment on the content of 5-n alkylresorcinols, total phenols and on the antiradical activity of whole durum wheat grains. J Cereal Sci 57:162–169. https://doi.org/10.1016/j.jcs.2012.11.003
Bennetzen JL, Schmutz J, Wang H et al (2012) Reference genome sequence of the model plant Setaria. Nat Biotechnol 30:555–561. https://doi.org/10.1038/nbt.2196
Bhave KG, Dalvi VV, Thaware BL et al (2016) Mutagenesis in proso millet (Panicum miliaceum L). Int J Chem Stud 5:1635–1638
Bidinger FR, Serraj R, Rizvi SMH et al (2005) Field evaluation of drought tolerance QTL effects on phenotype and adaptation in pearl millet [Pennisetum glaucum (L.) R. Br.] Topcross hybrids. Field Crops Res 94:14–32. https://doi.org/10.1016/j.fcr.2004.11.006
Boutrot F, Zipfel C (2017) Function, Discovery, and Exploitation of Plant Pattern Recognition receptors for Broad-Spectrum Disease Resistance. Annu Rev Phytopathol 55:257–286. https://doi.org/10.1146/annurev-phyto-080614-120106
Burton GW, Powell J (1966) Morphological and cytological response of Pear Millet, Pennisetum typhoides to Thermal Neutron and Ethyl Methane Sulfonate seed treatments. Crop Sci 6:180–182. https://doi.org/10.2135/cropsci1966.0011183X000600020021x
Burton GW, PowellResearch Geneticist JB, HannaResearch Geneticist WW (1974) Effect of recurrent mutagen seed treatments on mutation frequency and combining ability for forage yield in pearl millet (Pennisetum americanum (L.) K. Schum). Radiation Bot 14:323–335. https://doi.org/10.1016/S0033-7560(74)80024-4
Cannarozzi G, Plaza-Wüthrich S, Esfeld K et al (2014) Genome and transcriptome sequencing identifies breeding targets in the orphan crop tef (Eragrostis tef). BMC Genomics 15:581. https://doi.org/10.1186/1471-2164-15-581
Article PubMed PubMed Central Google Scholar
Cao X, Hu Y, Song J et al (2022) Transcriptome Sequencing and Metabolome Analysis reveals the molecular mechanism of Drought stress in Millet. Int J Mol Sci 23:10792. https://doi.org/10.3390/ijms231810792
Article PubMed PubMed Central CAS Google Scholar
Ceasar A (2022) Genome-editing in millets: current knowledge and future perspectives. Mol Biol Rep 49:773–781. https://doi.org/10.1007/s11033-021-06975-w
Chakrabarti S, Jahandideh F, Davidge ST, Wu J (2018) Milk-derived tripeptides IPP (Ile-Pro-Pro) and VPP (Val-Pro-Pro) enhance insulin sensitivity and prevent insulin resistance in 3T3-F442A preadipocytes. J Agric Food Chem 66:10179–10187. https://doi.org/10.1021/acs.jafc.8b02051
Chandrasekara A, Shahidi F (2010) Content of insoluble bound phenolics in millets and their contribution to antioxidant capacity. J Agric Food Chem 58:6706–6714. https://doi.org/10.1021/jf100868b
Chandrasekara A, Shahidi F (2011) Determination of antioxidant activity in free and hydrolyzed fractions of millet grains and characterization of their phenolic profiles by HPLC-DAD-ESI-MSn. J Funct Foods 3:144–158. https://doi.org/10.1016/j.jff.2011.03.007
Chanwala J, Satpati S, Dixit A et al (2020) Genome-wide identification and expression analysis of WRKY transcription factors in pearl millet (Pennisetum glaucum) under dehydration and salinity stress. BMC Genomics 21:231. https://doi.org/10.1186/s12864-020-6622-0
Cheng Z, Sun Y, Yang S et al (2021) Establishing in planta haploid inducer line by edited SiMTL in foxtail millet ( Setaria italica ). Plant Biotechnol J 19:1089–1091. https://doi.org/10.1111/pbi.13584
CHETHAN S, MALLESHI N (2007) Finger millet polyphenols: optimization of extraction and the effect of pH on their stability. Food Chem 105:862–870. https://doi.org/10.1016/j.foodchem.2007.02.012
Das IK, Rakshit S (2016) Millets, their importance, and production constraints. Biotic Stress Resistance in millets. Elsevier, pp 3–19
de Dal’Molin O, Orellana CG, Gebbie C L, et al (2016) Metabolic Reconstruction of Setaria italica: A systems Biology Approach for integrating tissue-specific omics and Pathway Analysis of Bioenergy Grasses. Front Plant Sci 7:1–20. https://doi.org/10.3389/fpls.2016.01138
de Souza LP, Borghi M, Fernie A (2020) Plant single-cell Metabolomics—challenges and perspectives. Int J Mol Sci 21:1–17. https://doi.org/10.3390/ijms21238987
Dudhate A, Shinde H, Tsugama D et al (2018) Transcriptomic analysis reveals the differentially expressed genes and pathways involved in drought tolerance in pearl millet [Pennisetum glaucum (L.) R. Br]. PLoS ONE 13:1–14. https://doi.org/10.1371/journal.pone.0195908
Efremova M, Teichmann SA (2020) Computational methods for single-cell omics across modalities. Nat Methods 17:14–17. https://doi.org/10.1038/s41592-019-0692-4
Elango D, Xue W, Chopra S (2020) Genome wide association mapping of epi-cuticular wax genes in Sorghum bicolor. Physiol Mol Biology Plants 26:1727–1737. https://doi.org/10.1007/s12298-020-00848-5
Fiehn O, Kopka J, Dörmann P et al (2000) Metabolite profiling for plant functional genomics. Nat Biotechnol 18:1157–1161. https://doi.org/10.1038/81137
Fukunaga K, Abe A, Mukainari Y et al (2022) Recombinant inbred lines and next-generation sequencing enable rapid identification of candidate genes involved in morphological and agronomic traits in foxtail millet. Sci Rep 12:218. https://doi.org/10.1038/s41598-021-04012-1
Ghatak A, Chaturvedi P, Nagler M et al (2016) Comprehensive tissue-specific proteome analysis of drought stress responses in Pennisetum glaucum (L.) R. Br. (Pearl millet). J Proteom 143:122–135. https://doi.org/10.1016/j.jprot.2016.02.032
Gienapp P, Laine VN, Mateman AC et al (2017) Environment-Dependent genotype-phenotype associations in avian breeding time. Front Genet 8:1–9. https://doi.org/10.3389/fgene.2017.00102
Gimode D, Odeny DA, de Villiers EP et al (2016) Identification of SNP and SSR markers in Finger Millet using next generation sequencing technologies. PLoS ONE 11:1–23. https://doi.org/10.1371/journal.pone.0159437
Girma G, Nida H, Seyoum A et al (2019) A Large-Scale Genome-Wide Association Analyses of Ethiopian Sorghum Landrace Collection Reveal Loci Associated with important traits. Front Plant Sci 10:1–15. https://doi.org/10.3389/fpls.2019.00691
Guijas C, Montenegro-Burke JR, Warth B et al (2018) Metabolomics activity screening for identifying metabolites that modulate phenotype. Nat Biotechnol 36:316–320. https://doi.org/10.1038/nbt.4101
Guo L, Gao Z, Qian Q (2014) Application of resequencing to rice genomics, functional genomics and evolutionary analysis. Rice 7:2–10. https://doi.org/10.1186/s12284-014-0004-7
Guo L, Qiu J, Ye C et al (2017) Echinochloa crus-galli genome analysis provides insight into its adaptation and invasiveness as a weed. Nat Commun 8:1–10. https://doi.org/10.1038/s41467-017-01067-5
Guo H, Guo H, Zhang L et al (2019) Metabolome and Transcriptome Association Analysis Reveals Dynamic Regulation of Purine Metabolism and Flavonoid synthesis in transdifferentiation during somatic embryogenesis in cotton. Int J Mol Sci 20:1–23. https://doi.org/10.3390/ijms20092070
Hall RD (2006) Plant metabolomics: from holistic hope, to hype, to hot topic. New Phytol 169:453–468. https://doi.org/10.1111/j.1469-8137.2005.01632.x
Hatakeyama M, Aluri S, Balachadran MT et al (2018) Multiple hybrid de novo genome assembly of finger millet, an orphan allotetraploid crop. DNA Res 25:39–47. https://doi.org/10.1093/dnares/dsx036
He J, Zhao X, Laroche A et al (2014) Genotyping-by-sequencing (GBS), an ultimate marker-assisted selection (MAS) tool to accelerate plant breeding. Front Plant Sci 5:1–8. https://doi.org/10.3389/fpls.2014.00484
Hein JA, Sherrard ME, Manfredi KP, Abebe T (2016) The fifth leaf and spike organs of barley (Hordeum vulgare L.) display different physiological and metabolic responses to drought stress. BMC Plant Biol 16:248. https://doi.org/10.1186/s12870-016-0922-1
Hema R, Vemanna RS, Sreeramulu S et al (2014) Stable expression of mtlD Gene imparts multiple stress tolerance in Finger Millet. PLoS ONE 9:1–13. https://doi.org/10.1371/journal.pone.0099110
Hittalmani S, Mahesh HB, Shirke MD et al (2017) Genome and transcriptome sequence of Finger millet (Eleusine coracana (L.) Gaertn.) Provides insights into drought tolerance and nutraceutical properties. BMC Genomics 18:465. https://doi.org/10.1186/s12864-017-3850-z
Hu Z, Mbacké B, Perumal R et al (2015) Population genomics of pearl millet (Pennisetum glaucum (L.) R. Br.): comparative analysis of global accessions and Senegalese landraces. BMC Genomics 16:1048. https://doi.org/10.1186/s12864-015-2255-0
Hu Z, Olatoye MO, Marla S, Morris GP (2019) An Integrated genotyping-by‐sequencing polymorphism map for over 10,000 Sorghum genotypes. Plant Genome 12:1–15. https://doi.org/10.3835/plantgenome2018.06.0044
Hwang S-L, Shih P-H, Yen G-C (2012) Neuroprotective effects of Citrus flavonoids. J Agric Food Chem 60:877–885. https://doi.org/10.1021/jf204452y
Jaiswal V, Bandyopadhyay T, Gahlaut V et al (2019) Genome-wide association study (GWAS) delineates genomic loci for ten nutritional elements in foxtail millet (Setaria italica L). J Cereal Sci 85:48–55. https://doi.org/10.1016/j.jcs.2018.11.006
Jayakodi M, Madheswaran M, Adhimoolam K et al (2019) Transcriptomes of Indian barnyard millet and barnyardgrass reveal putative genes involved in drought adaptation and micronutrient accumulation. Acta Physiol Plant 41:1–11. https://doi.org/10.1007/s11738-019-2855-4
Jayasudha BG, Sushma AM, Hanjagi PS, Sashidhar VR (2014) An efficient in-vitro Agrobacterium-mediated transformation protocol for raising salinity tolerant transgenic plants in finger millet [Eleusine coracana (L). Plang Archives 14:823–829
Jency JP, Ravikesavan R, Sumathi P, Raveendran M (2016) Determination of lethal dose and efect of physical mutagen on germination percentage and seedling parameters in kodomillet variety CO 3. Electron J Plant Breed 7:1122–1126
Jendoubi T (2021) Approaches to integrating metabolomics and Multi-omics Data: a primer. Metabolites 11:184. https://doi.org/10.3390/metabo11030184
Kanfany G, Serba DD, Rhodes D et al (2020) Genomic diversity in pearl millet inbred lines derived from landraces and improved varieties. BMC Genomics 21:469. https://doi.org/10.1186/s12864-020-06796-4
Kover PX, Valdar W, Trakalo J et al (2009) A Multiparent Advanced Generation Inter-cross to Fine-Map quantitative traits in Arabidopsis thaliana. PLoS Genet 5:1–15. https://doi.org/10.1371/journal.pgen.1000551
Kumar A, Gaur VS, Goel A, Gupta AK (2015) De Novo Assembly and characterization of developing spikes transcriptome of Finger Millet (Eleusine coracana): a minor crop having Nutraceutical properties. Plant Mol Biol Rep 33:905–922. https://doi.org/10.1007/s11105-014-0802-5
Kumar A, Tripathi MK, Joshi D, Kumar V (2021) Millets and Millet Technology. Springer Singapore
Larrainzar E, Wienkoop S, Scherling C et al (2009) Carbon Metabolism and Bacteroid Functioning are involved in the regulation of Nitrogen fixation in Medicago truncatula under Drought and Recovery. Mol Plant-Micro Int 22:1565–1576. https://doi.org/10.1094/MPMI-22-12-1565
Latha AM, Rao KV, Reddy VD (2005) Production of transgenic plants resistant to leaf blast disease in finger millet (Eleusine coracana (L.) Gaertn). Plant Sci 169:657–667. https://doi.org/10.1016/j.plantsci.2005.05.009
Lestienne I, Buisson M, Lullien-Pellerin V et al (2007) Losses of nutrients and anti-nutritional factors during abrasive decortication of two pearl millet cultivars (Pennisetum glaucum). Food Chem 100:1316–1323. https://doi.org/10.1016/j.foodchem.2005.11.027
Levy E, Slavov N (2018) Single cell protein analysis for systems biology. Essays Biochem 62:595–605. https://doi.org/10.1042/EBC20180014
Li C, Yue J, Wu X et al (2014) An ABA-responsive DRE-binding protein gene from Setaria italica, SiARDP, the target gene of SiAREB, plays a critical role under drought stress. J Exp Bot 65:5415–5427. https://doi.org/10.1093/jxb/eru302
Li S, Dong X, Fan G et al (2018) Comprehensive profiling and inheritance patterns of metabolites in Foxtail Millet. Front Plant Sci 9:1–16. https://doi.org/10.3389/fpls.2018.01716
Li J, Li X, Yang Q et al (2019a) Proteomic changes in the grains of foxtail millet (Setaria italica (L.) Beau) under drought stress. Span J Agricultural Res 17:1–12. https://doi.org/10.5424/sjar/2019172-14300
Li J, Li X, Yang Q et al (2019b) Proteomic changes in the grains of foxtail millet (Setaria italica (L.) Beau) under drought stress. Span J Agricultural Res 17:1–12. https://doi.org/10.5424/sjar/2019172-14300
Li C, Wang G, Li H et al (2021a) High-depth resequencing of 312 accessions reveals the local adaptation of foxtail millet. Theor Appl Genet 134:1303–1317. https://doi.org/10.1007/s00122-020-03760-4
Li J, Wang Y, Wang L et al (2021b) Integration of transcriptomic and proteomic analyses for finger millet [Eleusine coracana (L.) Gaertn.] In response to drought stress. PLoS ONE 16:1–16. https://doi.org/10.1371/journal.pone.0247181
Li W, Wen L, Chen Z et al (2021c) Study on metabolic variation in whole grains of four proso millet varieties reveals metabolites important for antioxidant properties and quality traits. Food Chem 357:1–11. https://doi.org/10.1016/j.foodchem.2021.129791
Li Z, Zhao X, Zhang X, Liu H (2021d) Bioactive compounds and Biological activities of Sorghum grains. Foods 10:1–20. https://doi.org/10.3390/foods10112868
Li X, Gao J, Song J et al (2022) Multi-omics analyses of 398 foxtail millet accessions reveal genomic regions associated with domestication, metabolite traits, and anti-inflammatory effects. Mol Plant 15:1367–1383. https://doi.org/10.1016/j.molp.2022.07.003
Libault M, Pingault L, Zogli P, Schiefelbein J (2017) Plant Systems Biology at the single-cell level. Trends Plant Sci 22:949–960. https://doi.org/10.1016/j.tplants.2017.08.006
Lin C, Hsu C, Yang L et al (2018) Application of protoplast technology to CRISPR/Cas9 mutagenesis: from single-cell mutation detection to mutant plant regeneration. Plant Biotechnol J 16:1295–1310. https://doi.org/10.1111/pbi.12870
Liu Y, Feng X, Xu Y et al (2009) Overexpression of millet ZIP-like gene (SiPf40) affects lateral bud outgrowth in tobacco and millet. Plant Physiol Biochem 47:1051–1060. https://doi.org/10.1016/j.plaphy.2009.08.007
Luo J (2015) Metabolite-based genome-wide association studies in plants. Curr Opin Plant Biol 24:31–38. https://doi.org/10.1016/j.pbi.2015.01.006
Mace ES, Tai S, Gilding EK et al (2013) Whole-genome sequencing reveals untapped genetic potential in Africa’s indigenous cereal crop sorghum. Nat Commun 4:1–9. https://doi.org/10.1038/ncomms3320
Mahalakshmi S, Christopher GSB, Reddy TP et al (2006) Isolation of a cDNA clone (PcSrp) encoding serine-rich-protein from Porteresia coarctata T. and its expression in yeast and finger millet (Eleusine coracana L.) affording salt tolerance. Planta 224:347–359. https://doi.org/10.1007/s00425-005-0218-4
Matsuda F, Yonekura-Sakakibara K, Niida R et al (2009) MS/MS spectral tag‐based annotation of non‐targeted profile of plant secondary metabolites. Plant J 57:555–577. https://doi.org/10.1111/j.1365-313X.2008.03705.x
Mohapatra D, Patel AS, Kar A et al (2019) Effect of different processing conditions on proximate composition, anti-oxidants, anti-nutrients and amino acid profile of grain sorghum. Food Chem 271:129–135. https://doi.org/10.1016/j.foodchem.2018.07.196
Moreira TB, Shaw R, Luo X et al (2019) A genome-scale metabolic model of soybean ( Glycine max ) highlights metabolic fluxes in Seedlings. Plant Physiol 180:1912–1929. https://doi.org/10.1104/pp.19.00122
Muthamilarasan M, Prasad M (2021) Small millets for Enduring Food Security amidst pandemics. Trends Plant Sci 26:33–40. https://doi.org/10.1016/j.tplants.2020.08.008
N’Dri D, Mazzeo T, Zaupa M et al (2013) Effect of cooking on the total antioxidant capacity and phenolic profile of some whole-meal African cereals. J Sci Food Agric 93:29–36. https://doi.org/10.1002/jsfa.5837
Nah G, Im J-H, Kim J-W et al (2015) Uncovering the Differential molecular basis of adaptive diversity in three Echinochloa Leaf transcriptomes. PLoS ONE 10:1–20. https://doi.org/10.1371/journal.pone.0134419
Ngom B, Sarr I, Kimatu J et al (2017) Genome-wide analysis of cytosine DNA methylation revealed salicylic acid promotes defense pathways over seedling development in pearl millet. Plant Signal Behav 12:1–7. https://doi.org/10.1080/15592324.2017.1356967
Nithiyanantham S, Kalaiselvi P, Mahomoodally MF et al (2019) Nutritional and functional roles of millets—A review. J Food Biochem 43:1–10. https://doi.org/10.1111/jfbc.12859
Nunes-Nesi A, Alseekh S, de Oliveira Silva FM et al (2019) Identification and characterization of metabolite quantitative trait loci in tomato leaves and comparison with those reported for fruits and seeds. Metabolomics 15:1–13. https://doi.org/10.1007/s11306-019-1503-8
Okazaki Y, Saito K (2012) Recent advances of metabolomics in plant biotechnology. Plant Biotechnol Rep 6:1–15. https://doi.org/10.1007/s11816-011-0191-2
Article PubMed Google Scholar
Pan J, Li Z, Dai S et al (2020) Integrative analyses of transcriptomics and metabolomics upon seed germination of foxtail millet in response to salinity. Sci Rep 10:13660. https://doi.org/10.1038/s41598-020-70520-1
Pandey S, Singh A, Parida SK, Prasad M (2022) Combining speed breeding with traditional and genomics-assisted breeding for crop improvement. Plant Breeding 141:301–313. https://doi.org/10.1111/pbr.13012
Paterson AH, Bowers JE, Bruggmann R et al (2009) The Sorghum bicolor genome and the diversification of grasses. Nature 457:551–556. https://doi.org/10.1038/nature07723
Pathak RK, Gupta A, Shukla R, Baunthiyal M (2018) Identification of new drug-like compounds from millets as Xanthine oxidoreductase inhibitors for treatment of Hyperuricemia: a molecular docking and simulation study. Comput Biol Chem 76:32–41. https://doi.org/10.1016/j.compbiolchem.2018.05.015
Pattnaik S (2023) Next-generation sequencing (NGS) for Metabolomics Study in Medicinal plants under stress Condition. Stress-responsive factors and molecular Farming in Medicinal plants. Springer Nature Singapore, Singapore, pp 323–343
Chapter Google Scholar
Peng R, Zhang B (2021) Foxtail Millet: a New Model for C4 plants. Trends Plant Sci 26:199–201. https://doi.org/10.1016/j.tplants.2020.12.003
Poland JA, Brown PJ, Sorrells ME, Jannink J-L (2012) Development of high-density genetic maps for Barley and Wheat using a Novel two-enzyme genotyping-by-sequencing Approach. PLoS ONE 7:1–8. https://doi.org/10.1371/journal.pone.0032253
Pradeep SR, Guha M (2011) Effect of processing methods on the nutraceutical and antioxidant properties of little millet (Panicum sumatrense) extracts. Food Chem 126:1643–1647. https://doi.org/10.1016/j.foodchem.2010.12.047
Pradeep PM, Sreerama YN (2017) Soluble and bound phenolics of two different millet genera and their milled fractions: comparative evaluation of antioxidant properties and inhibitory effects on starch hydrolysing enzyme activities. J Funct Foods 35:682–693. https://doi.org/10.1016/j.jff.2017.06.033
Prakadan SM, Shalek AK, Weitz DA (2017) Scaling by shrinking: empowering single-cell omics with microfluidic devices. Nat Rev Genet 18:345–361. https://doi.org/10.1038/nrg.2017.15
Priyanka V, Kumar R, Dhaliwal I, Kaushik P (2021) Germplasm Conservation: Instrumental in Agricultural Biodiversity—A. Rev Sustain 13:1–18. https://doi.org/10.3390/su13126743
Pujari SN, Hoskeri JH (2022) Minor Millet Phytochemicals and their pharmacological potentials. Pharmacogn Rev 16:100–106. https://doi.org/10.5530/phrev.2022.16.15
Puranik S, Sahu PP, Beynon S et al (2020) Genome-wide association mapping and comparative genomics identifies genomic regions governing grain nutritional traits in finger millet ( Eleusine coracana L. Gaertn.). PLANTS, PEOPLE. PLANET 2:649–662. https://doi.org/10.1002/ppp3.10120
Rahman H, Jagadeeshselvam N, Valarmathi R et al (2014) Transcriptome analysis of salinity responsiveness in contrasting genotypes of finger millet (Eleusine coracana L.) through RNA-sequencing. Plant Mol Biol 85:485–503. https://doi.org/10.1007/s11103-014-0199-4
Rajput SG, Santra DK, Schnable J (2016) Mapping QTLs for morpho-agronomic traits in proso millet (Panicum miliaceum L). Mol Breeding 36:37. https://doi.org/10.1007/s11032-016-0460-4
Ramegowda Y, Venkategowda R, Jagadish P et al (2013) Expression of a rice zn transporter, OsZIP1, increases Zn concentration in tobacco and finger millet transgenic plants. Plant Biotechnol Rep 7:309–319. https://doi.org/10.1007/s11816-012-0264-x
Reichert RD (1979) The pH-sensitive pigments in pearl millet. Cereal Chem 56:291–294
CAS Google Scholar
Renganathan VG, Vanniarajan C, Karthikeyan A, Ramalingam J (2020) Barnyard Millet for Food and Nutritional Security: current status and Future Research Direction. Front Genet 11:1–21. https://doi.org/10.3389/fgene.2020.00500
Rhoné B, Defrance D, Berthouly-Salazar C et al (2020) Pearl millet genomic vulnerability to climate change in West Africa highlights the need for regional collaboration. Nat Commun 11:1–9. https://doi.org/10.1038/s41467-020-19066-4
Robinson SW, Fernandes M, Husi H (2014) Current advances in systems and integrative biology. Comput Struct Biotechnol J 11:35–46. https://doi.org/10.1016/j.csbj.2014.08.007
Ruiz-Giralt A, Biagetti S, Madella M, Lancelotti C (2023) Small-scale farming in drylands: New models for resilient practices of millet and sorghum cultivation. PLoS ONE 18:1–17. https://doi.org/10.1371/journal.pone.0268120
Saito K, Matsuda F (2010) Metabolomics for Functional Genomics, Systems Biology, and Biotechnology. Annu Rev Plant Biol 61:463–489. https://doi.org/10.1146/annurev.arplant.043008.092035
Sarita ES (2016) Potential functional implications of finger millet (Eleusine coracana) in nutritional benefits, processing, health and diseases: a review. Int J Home Sci 2:151–155
Satyavathi CT, Tomar RS, Ambawat S et al (2022) Stage specific comparative transcriptomic analysis to reveal gene networks regulating iron and zinc content in pearl millet [Pennisetum glaucum (L.) R. Br]. Sci Rep 12:276. https://doi.org/10.1038/s41598-021-04388-0
Scheben A, Batley J, Edwards D (2017) Genotyping-by‐sequencing approaches to characterize crop genomes: choosing the right tool for the right application. Plant Biotechnol J 15:149–161. https://doi.org/10.1111/pbi.12645
Sehgal D, Rajaram V, Armstead IP et al (2012) Integration of gene-based markers in a pearl millet genetic map for identification of candidate genes underlying drought tolerance quantitative trait loci. BMC Plant Biol 12:1–13. https://doi.org/10.1186/1471-2229-12-9
Sen S, Kundu S, Dutta SK (2016) Proteomic analysis of JAZ interacting proteins under methyl jasmonate treatment in finger millet. Plant Physiol Biochem 108:79–89. https://doi.org/10.1016/j.plaphy.2016.05.033
Serba DD, Yadav RS (2016) Genomic tools in Pearl Millet Breeding for Drought Tolerance: Status and prospects. Front Plant Sci 7:1–10. https://doi.org/10.3389/fpls.2016.01724
Severin AJ, Woody JL, Bolon Y-T et al (2010) RNA-Seq atlas of Glycine max: a guide to the soybean transcriptome. BMC Plant Biol 10:160. https://doi.org/10.1186/1471-2229-10-160
Sharma D, Tiwari A, Sood S et al (2018) Genome wide association mapping of agro-morphological traits among a diverse collection of finger millet (Eleusine coracana L.) genotypes using SNP markers. PLoS ONE 13:1–21. https://doi.org/10.1371/journal.pone.0199444
Shi J, Ma X, Zhang J et al (2019) Chromosome conformation capture resolved near complete genome assembly of broomcorn millet. Nat Commun 10:1–9. https://doi.org/10.1038/s41467-018-07876-6
Shi W, Yue L, Guo J et al (2020) Identification and evolution of C4 photosynthetic pathway genes in plants. BMC Plant Biol 20:132. https://doi.org/10.1186/s12870-020-02339-x
Shulse CN, Cole BJ, Ciobanu D et al (2019) High-throughput single-cell transcriptome profiling of plant cell types. Cell Rep 27:2241–2247. https://doi.org/10.1016/j.celrep.2019.04.054
Siddhuraju P, Becker K (2007) The antioxidant and free radical scavenging activities of processed cowpea (Vigna unguiculata (L.) Walp.) Seed extracts. Food Chem 101:10–19. https://doi.org/10.1016/j.foodchem.2006.01.004
Singh UM, Sareen P, Sengar RS, Kumar A (2013) Plant ionomics: a newer approach to study mineral transport and its regulation. Acta Physiol Plant 35:2641–2653. https://doi.org/10.1007/s11738-013-1316-8
Singh M, Metwal M, Kumar VA, Kumar A (2016) Identification and molecular characterization of 48 < scp > kDa calcium binding protein as calreticulin from finger millet ( Eleusine coracana ) using peptide mass fingerprinting and transcript profiling. J Sci Food Agric 96:672–679. https://doi.org/10.1002/jsfa.7139
Siwela M, Taylor JRN, de Milliano WAJ, Duodu KG (2010) Influence of phenolics in finger millet on grain and malt fungal load, and malt quality. Food Chem 121:443–449. https://doi.org/10.1016/j.foodchem.2009.12.062
Sokół-Łętowska A, Oszmiański J, Wojdyło A (2007) Antioxidant activity of the phenolic compounds of hawthorn, pine and skullcap. Food Chem 103:853–859. https://doi.org/10.1016/j.foodchem.2006.09.036
Sood P, Pandey S, Prasad M (2021) Biotechnological advances and advanced mutation breeding techniques for crop plants. Crop Improvement. ISBN9781003099079
Subba Rao MVSST, Muralikrishna G (2002) Evaluation of the antioxidant properties of free and bound phenolic acids from native and Malted Finger Millet (Ragi, Eleusine coracana Indaf-15). J Agric Food Chem 50:889–892. https://doi.org/10.1021/jf011210d
Sumner LW, Mendes P, Dixon RA (2003) Plant metabolomics: large-scale phytochemistry in the functional genomics era. Phytochemistry 62:817–836. https://doi.org/10.1016/S0031-9422(02)00708-2
Tiwari A, Sharma D, Sood S et al (2020) Genome-wide association mapping for seed protein content in finger millet (Eleusine coracana) global collection through genotyping by sequencing. J Cereal Sci 91:1–35. https://doi.org/10.1016/j.jcs.2019.102888
Tripathi MK, Mohapatra D, Jadam RS et al (2021) Nutritional composition of millets. Millets and Millet Technology. Springer Singapore, Singapore, pp 101–119
Tuberosa R (2012) Phenotyping for drought tolerance of crops in the genomics era. Front Physiol 3:1–26. https://doi.org/10.3389/fphys.2012.00347
Upadhyaya HD, Ravishankar CR, Narasimhudu Y et al (2011) Identification of trait-specific germplasm and developing a mini core collection for efficient use of foxtail millet genetic resources in crop improvement. Field Crops Res 124:459–467. https://doi.org/10.1016/j.fcr.2011.08.004
Varshney RK, Shi C, Thudi M et al (2017) Pearl millet genome sequence provides a resource to improve agronomic traits in arid environments. Nat Biotechnol 35:969–976. https://doi.org/10.1038/nbt.3943
Veeranagamallaiah G, Jyothsnakumari G, Thippeswamy M et al (2008) Proteomic analysis of salt stress responses in foxtail millet (Setaria italica L. Cv. Prasad) seedlings. Plant Sci 175:631–641. https://doi.org/10.1016/j.plantsci.2008.06.017
Vetriventhan M, Upadhyaya HD (2019) Variability for Productivity and Nutritional traits in Germplasm of Kodo Millet, an underutilized nutrient-Rich Climate Smart Crop. Crop Sci 59:1095–1106. https://doi.org/10.2135/cropsci2018.07.0450
Vetriventhan M, Azevedo VCR, Upadhyaya HD et al (2020) Genetic and genomic resources, and breeding for accelerating improvement of small millets: current status and future interventions. Nucleus 63:217–239. https://doi.org/10.1007/s13237-020-00322-3
Viswanath V, Urooj A, Malleshi NG (2009) Evaluation of antioxidant and antimicrobial properties of finger millet polyphenols (Eleusine coracana). Food Chem 114:340–346. https://doi.org/10.1016/j.foodchem.2008.09.053
Wang Z, Gerstein M, Snyder M (2009) RNA-Seq: a revolutionary tool for transcriptomics. Nat Rev Genet 10:57–63. https://doi.org/10.1038/nrg2484
Wang T, Xing L, Song H et al (2023) Large-scale metabolome analysis reveals dynamic changes of metabolites during foxtail millet grain filling. Food Res Int 165:112516. https://doi.org/10.1016/j.foodres.2023.112516
Watanabe M (1999) Antioxidative phenolic compounds from Japanese Barnyard Millet ( Echinochloa utilis ) grains. J Agric Food Chem 47:4500–4505. https://doi.org/10.1021/jf990498s
Weckwerth W (2003) Metabolomics in Systems Biology. Annu Rev Plant Biol 54:669–689. https://doi.org/10.1146/annurev.arplant.54.031902.135014
Wei W, Li S, Wang Y et al (2021) Metabolome-based genome-wide Association Study provides genetic insights into the natural variation of Foxtail Millet. Front Plant Sci 12:1–13. https://doi.org/10.3389/fpls.2021.665530
Wilson ML, VanBuren R (2022) Leveraging millets for developing climate resilient agriculture. Curr Opin Biotechnol 75:102683. https://doi.org/10.1016/j.copbio.2022.102683
Yadav CB, Pandey G, Muthamilarasan M, Prasad M (2018) Epigenetics and Epigenomics of Plants. pp 237–261
Yadav CB, Srivastava RK, Gangashetty PI et al (2021) Metabolite Diversity and Metabolic Genome-Wide Marker Association Studies (Mgwas) for Health benefiting nutritional traits in Pearl Millet Grains. Cells 10:1–13. https://doi.org/10.3390/cells10113076
Yadav B, Pandit DL, Banjade D et al (2024) Insights into the germplasm conservation and utilization: implications for sustainable agriculture and future crop improvement. Archives Agric Environ Sci 9:180–193. https://doi.org/10.26832/24566632.2024.0901026
Yang Z, Zhang H, Li X et al (2020) A mini foxtail millet with an Arabidopsis-like life cycle as a C4 model system. Nat Plants 6:1167–1178. https://doi.org/10.1038/s41477-020-0747-7
Yu J, Holland JB, McMullen MD, Buckler ES (2008) Genetic design and Statistical Power of Nested Association Mapping in Maize. Genetics 178:539–551. https://doi.org/10.1534/genetics.107.074245
Zhang G, Liu X, Quan Z et al (2012) Genome sequence of foxtail millet (Setaria italica) provides insights into grass evolution and biofuel potential. Nat Biotechnol 30:549–554. https://doi.org/10.1038/nbt.2195
Zou C, Li L, Miki D et al (2019) The genome of broomcorn millet. Nat Commun 10:1–11. https://doi.org/10.1038/s41467-019-08409-5
Download references
Not applicable.
Author information
Authors and affiliations.
Department of Biotechnology, Institute of Engineering and Management, University of Engineering and Management, Kolkata, West Bengal, India
Saikat Mazumder, Dibyajit Lahiri & Moupriya Nag
Department of Food Technology, Guru Nanak Institute of Technology, Kolkata, West Bengal, India
Saikat Mazumder
Department of Basic Science and Humanities, Institute of Engineering and Management, Kolkata University of Engineering and Management, Kolkata, West Bengal, India
Debasmita Bhattacharya
You can also search for this author in PubMed Google Scholar
Contributions
SM drafted the manuscript. DB & DL edited the manuscript. MN critically reviewed it.
Corresponding author
Correspondence to Moupriya Nag .
Ethics declarations
Competing interests.
The authors declare no competing interests.
Additional information
Publisher’s note.
Springer Nature remains neutral with regard to jurisdictional claims in published maps and institutional affiliations.
Rights and permissions
Springer Nature or its licensor (e.g. a society or other partner) holds exclusive rights to this article under a publishing agreement with the author(s) or other rightsholder(s); author self-archiving of the accepted manuscript version of this article is solely governed by the terms of such publishing agreement and applicable law.
Reprints and permissions
About this article
Mazumder, S., Bhattacharya, D., Lahiri, D. et al. Milletomics: a metabolomics centered integrated omics approach toward genetic progression. Funct Integr Genomics 24 , 149 (2024). https://doi.org/10.1007/s10142-024-01430-y
Download citation
Received : 05 July 2024
Revised : 25 July 2024
Accepted : 20 August 2024
Published : 02 September 2024
DOI : https://doi.org/10.1007/s10142-024-01430-y
Share this article
Anyone you share the following link with will be able to read this content:
Sorry, a shareable link is not currently available for this article.
Provided by the Springer Nature SharedIt content-sharing initiative
- Metabolomics
- Omics technology
- Next-generation sequencing
Advertisement
- Find a journal
- Publish with us
- Track your research
World-renowned, cutting-edge, interdisciplinary plant breeding and genetics program at the University of Georgia.
CARROT BREEDING TO REDUCE INPUTS FOR GROWERS AND INCREASE OUTPUTS FOR CONSUMERS
Dr. Phil Simon USDA-ARS Vegetable Crops Research Unit Department of Plant & Agroecosystem Sciences, University of Wisconsin-Madison
When: Friday, September 13 at 3:30 p.m. Where: NESPAL Seminar Room (Room 126) on the UGA Tifton Campus
Register Today
Connect with IPBGG
Currently a full professor at Alparslan Turkeş Science and Technology University in Adana/Turkiye, Sakiroglu performs research on forage genetics and genomics. He also teaches several graduate and undergraduate courses and mentors graduate students.
Apply to IPBGG
We're proud of our awesome graduate students and want to recognize their excellence!
The Institute for Plant Breeding, Genetics and Genomics has a vibrant graduate program that offers both a Master's degree and a Doctorate of Philosophy in Plant Breeding, Genetics and Genomics.
IPBGG Awards & Honors
Did you know that Georgia is responsible for about half of the United States’ peanut production? This significant contribution stems from the concentration of peanut farming in the southeastern region of the U.S., where it holds immense importance for local farmers. However, peanuts are not just a regional staple; they serve as a vital food source for many developing countries in Asia and Africa. The ability to store peanut seeds for long periods makes them crucial during crop shortages, providing a reliable source of nutrition.
PBGG Events
Thank you for visiting nature.com. You are using a browser version with limited support for CSS. To obtain the best experience, we recommend you use a more up to date browser (or turn off compatibility mode in Internet Explorer). In the meantime, to ensure continued support, we are displaying the site without styles and JavaScript.
- View all journals
- Explore content
- About the journal
- Publish with us
- Sign up for alerts
Plant breeding articles within Scientific Reports
Article 31 August 2024 | Open Access
Leveraging genetic resource diversity and identification of trait-enriched superior genotypes for accelerated improvement in linseed ( Linum usitatissimum L.)
- Vikender Kaur
- , Sunil S. Gomashe
- & Gyanendra Pratap Singh
Article 28 August 2024 | Open Access
Functional characterization of maize phytochrome-interacting factor 3 ( ZmPIF3 ) in enhancing salt tolerance in arabidopsis
- Zanping Han
- , Haohao Liu
- & Lei Tian
Article 09 August 2024 | Open Access
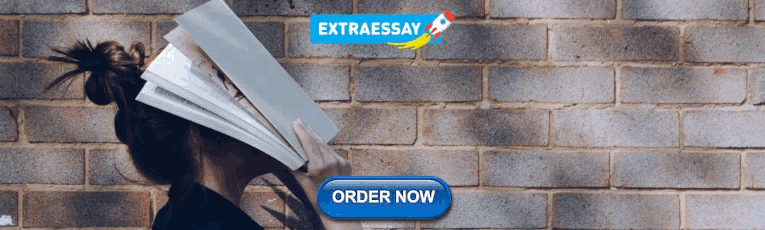
Phenotypic diversity in qualitative and quantitative traits for selection of high yield potential field pea genotypes
- Mohammad Golam Azam
- , Umakanta Sarker
- & Saud Alamri
Article 08 August 2024 | Open Access
Factor analytic selection tools and environmental feature-integration enable holistic decision-making in Eucalyptus breeding
- Saulo F. S. Chaves
- , Michelle B. Damacena
- & Leonardo L. Bhering
Article 16 July 2024 | Open Access
Discovering novel genomic regions explaining adaptation of bread wheat to conservation agriculture through GWAS
- Amit Kumar Mazumder
- , Rajbir Yadav
- & Kiran B. Gaikwad
Across-environment seed protein stability and genetic architecture of seed components in soybean
- Chengjun Wu
- , Andrea Acuña
- & Caio Canella Vieira
Article 15 July 2024 | Open Access
Assessing the yield and nutrient potential of horse gram mutants ( Macrotyloma uniflorum Lam. Verdc.) an underutilized legume through a multi-environment-based experiment
- Sumaiya Sulthana Jafarullakhan
- , Vaishnavi Vijayakumar
- & Sudhagar Rajaprakasam
Article 13 July 2024 | Open Access
Identification of candidate single-nucleotide polymorphisms (SNPs) and genes associated with sugarcane leaf scald disease
- , Pingping Lin
- & Muqing Zhang
Article 11 July 2024 | Open Access
Genotype by environment interaction and stability analysis for harvest date in sugar beet cultivars
- Saeed Sadeghzadeh Hemayati
- , Farahnaz Hamdi
- & Hamze Hamze
Article 10 July 2024 | Open Access
Transcriptome sequencing of garlic reveals key genes related to the heat stress response
- Qing-Qing Yang
- , Feng Yang
- & Ji-De Fan
Article 06 July 2024 | Open Access
Advancing common bean ( Phaseolus vulgaris L.) disease detection with YOLO driven deep learning to enhance agricultural AI
- Daniela Gomez
- , Michael Gomez Selvaraj
- & Ernesto Espitia
Article 03 July 2024 | Open Access
Optimal conditions of algal breeding using neutral beam and applying it to breed Euglena gracilis strains with improved lipid accumulation
- Sousuke Imamura
- , Koji Yamada
- & Kazuhiro Takaya
Article 02 July 2024 | Open Access
Genetic background influences mineral accumulation in rice straw and grains under different soil pH conditions
- Toshio Yamamoto
- , Kazunari Kashihara
- & Jian Feng Ma
Article 01 July 2024 | Open Access
Benefits and limits of biological nitrification inhibitors for plant nitrogen uptake and the environment
- Christian W. Kuppe
- & Johannes A. Postma
Article 25 June 2024 | Open Access
Resynthesizing Brassica napus with race specific resistance genes and race non-specific QTLs to multiple races of Plasmodiophora brassicae
- Md. Masud Karim
- & Fengqun Yu
Novel Bacillus and Prestia isolates from Dwarf century plant enhance crop yield and salinity tolerance
- Sanjoy Kumar Dhar
- , Jaspreet Kaur
- & Gholamreza Abdi
Article 21 June 2024 | Open Access
Genetic basis of an elite wheat cultivar Guinong 29 with harmonious improvement between multiple diseases resistance and other comprehensive traits
- , Yanmin Qie
- & Pengtao Ma
Article 19 June 2024 | Open Access
Conversion of superior bread wheat genotype HD3209 carrying Lr19/Sr25 into CMS line for development of rust-resistant wheat hybrids
- Abhimanyu Singh Malik
- , Nand Kishore Sharma
- & Vinod
Stability assessment of selected chrysanthemum ( Dendranthema grandiflora Tzvelev) hybrids over the years through AMMI and GGE biplot in the mid hills of North-Western Himalayas
- Shilpa Kamal
- , Amit Rana
- & Ashok Kumar
Article 17 June 2024 | Open Access
Implementation of theoretical non-photochemical quenching (NPQ (T) ) to investigate NPQ of chickpea under drought stress with High-throughput Phenotyping
- Madita Lauterberg
- , Henning Tschiersch
- & Kerstin Neumann
Insights into structure, codon usage, repeats, and RNA editing of the complete mitochondrial genome of Perilla frutescens (Lamiaceae)
- , Yongjian Luo
- & Daoshou Qiu
Article 15 June 2024 | Open Access
Climate-smart rice ( Oryza sativa L.) genotypes identification using stability analysis, multi-trait selection index, and genotype-environment interaction at different irrigation regimes with adaptation to universal warming
- Muhammad Ashraful Habib
- , Mohammad Golam Azam
- & Umakanta Sarker
Article 14 June 2024 | Open Access
The fertility research of "Huajin 6", a new variety of honeysuckle
- , Hui Chang
- & Gaobin Pu
Article 03 June 2024 | Open Access
A method for screening salt stress tolerance in Indian mustard ( Brassica juncea ) (L.) Czern & Coss at seedling stage
- Garima Aggarwal
- , Premnath Edhigalla
- & Sanjeet Singh Sandal
Article 21 May 2024 | Open Access
Establishment of an efficient regeneration system of ‘ZiKui’ tea with hypocotyl as explants
- Jiongyi Jin
- , Yulu Chen
- & Yan Li
Article 14 May 2024 | Open Access
Enhanced radiation use efficiency and grain filling rate as the main drivers of grain yield genetic gains in the CIMMYT elite spring wheat yield trial
- Guillermo Gerard
- , Suchismita Mondal
- & Leonardo Crespo-Herrera
Article 10 May 2024 | Open Access
On-farm crop diversity, conservation, importance and value: a case study of landraces from Western Ghats of Karnataka, India
- G. M. Puneeth
- , Ravi Gowthami
- & Sunil Archak
Article 02 May 2024 | Open Access
A new bacterial consortia for management of Fusarium head blight in wheat
- Vishnukiran Thuraga
- , Farideh Ghadamgahi
- & Aakash Chawade
Article 29 April 2024 | Open Access
Effects of trimer repeats on Psidium guajava L. gene expression and prospection of functional microsatellite markers
- Giovanna Pinto Pires
- , Vinicius Sartori Fioresi
- & Marcia Flores da Silva Ferreira
Article 22 April 2024 | Open Access
Assessing agronomic performance, chocolate spot resistance, and heat tolerance for diverse Vicia faba genotypes under varying environmental conditions
- Mostafa G. El-Abssi
- , Hassan A. Awaad
- & Elsayed Mansour
Article 21 April 2024 | Open Access
Testcross performance and combining ability of early-medium maturing quality protein maize inbred lines in Eastern and Southern Africa
- Addisalem Mebratu
- , Dagne Wegary
- & Amsal Tarekegne
Article 12 April 2024 | Open Access
The complete Chloroplast genome of Stachys geobombycis and comparative analysis with related Stachys species
- , Zheng Lan
- & Zhijun Deng
Article 31 March 2024 | Open Access
European soybean to benefit people and the environment
- Jose L. Rotundo
- , Rachel Marshall
- & Mariana C. Rufino
Article 27 March 2024 | Open Access
Study on the effect of different high-voltage electric field polarization process parameters on the vitality of dried chili pepper seeds
- & Hanjun Liu
Article 26 March 2024 | Open Access
Flower opening dynamics, pollen-ovule ratio, stigma receptivity and stigmatic pollen germination (in-vivo) in Chaenomeles speciosa (Sweet) Nakai
- Xianqin Wan
- , Dongchan Sun
- & Chao Gao
Article 19 March 2024 | Open Access
Uncovering the molecular mechanisms of russet skin formation in Niagara grapevine ( Vitis vinifera × Vitis labrusca )
- Guilherme Francio Niederauer
- , Geovani Luciano de Oliveira
- & Anete Pereira de Souza
Article 16 March 2024 | Open Access
Multi-trait selection for nutritional and physiological quality of cacao genotypes in irrigated and non-irrigated environments
- Maurício S. Araújo
- , Saulo F. S. Chaves
- & Marco A. G. Aguilar
Article 12 March 2024 | Open Access
Feature-specific nutrient management of onion ( Allium cepa ) using machine learning and compositional methods
- Leandro Hahn
- , Claudinei Kurtz
- & Léon-Étienne Parent
Association study of morpho-phenological traits in quinoa ( Chenopodium quinoa Willd.) using SSR markers
- Ebrahim Souri Laki
- , Babak Rabiei
- & Andreas Börner
Article 11 March 2024 | Open Access
Artificial neural networks optimize the establishment of a Brazilian germplasm core collection of winter squash ( Cucurbita moschata D.)
- Ronaldo Silva Gomes
- , Ronaldo Machado Júnior
- & Derly José Henriques da Silva
Article 04 March 2024 | Open Access
Evolutionary and synteny analysis of HIS1 , BADH2 , GBSS1 , and GBSS2 in rice: insights for effective introgression breeding strategies
- , Yong-Jin Park
- & Jungmin Ha
Investigating the genetic basis of salt-tolerance in common bean: a genome-wide association study at the early vegetative stage
- Lorenzo Raggi
- , Leonardo Caproni
- & Valeria Negri
Article 29 February 2024 | Open Access
The relation between apomictic seed production and morpho-physiological characteristics in a world collection of castor bean ( Ricinus communis L.)
- Maedeh Setayeshnasab
- , Mohammad R. Sabzalian
- & Mehdi Rahimmalek
Article 05 February 2024 | Open Access
AMMI and GGE biplot analysis of genotype by environment interaction for yield and yield contributing traits in confectionery groundnut
- Praveen Kona
- , B. C. Ajay
- & K. D. Solanki
Article 29 January 2024 | Open Access
Commercial suitability and characterization of newly developed Erucastrum canariense ( Can ) sterile cytoplasm based cytoplasmic male sterile (CMS) lines in Indian cauliflower
- K. S. Manjunath
- , Shrawan Singh
- & Bhoopal Singh Tomar
Exploring the genotype-environment interaction of bread wheat in ambient and high-temperature planting conditions: a rigorous investigation
- Vikrant Khare
- , Rama Shankar Shukla
- & Charan Singh
Article 20 January 2024 | Open Access
Root system architecture associated zinc variability in wheat ( Triticum aestivum L.)
- Mehwish Noor
- , Aysha Kiran
- & Abdul Wakeel
Article 19 January 2024 | Open Access
Unravelling the dynamics of genotype and environment interactions on chilli ( Capsicum annuum L.) yield-related attributes in soilless planting systems
- Siti Sahmsiah Sahmat
- , Mohd Yusop Rafii
- & Hasmah Mohidin
Article 11 January 2024 | Open Access
Genomic prediction in multi-environment trials in maize using statistical and machine learning methods
- Cynthia Aparecida Valiati Barreto
- , Kaio Olimpio das Graças Dias
- & Moysés Nascimento
Article 09 January 2024 | Open Access
A method for phenotypic evaluation of grapevine resistance in relation to phenological development
- Stefan Schumacher
- , Caroline Mertes
- & René Fuchs
Browse broader subjects
- Plant sciences
Quick links
- Explore articles by subject
- Guide to authors
- Editorial policies

The society was founded 60 years ago and has been keeping itself abreast with the latest research in the area of Genetics and Plant Breeding by encouraging active integration among its members through topical seminar/symposia to bring about the awareness among its members and the public at large. The Indian Journal of Genetics and Plant Breeding is a periodical for the publication of records of original research in all branches of genetics, plant breeding and cytology, including human genetics, molecular biology and biotechnology, and other cognate sciences of sufficient importance and of such a character as to be of primary interest to the geneticist and plant breeders.
Current Issue
Vol. 84 No. 03 (2024): Indian Journal of Genetics and Plant Breeding
Published: 2024-08-29
Review Articles
Developing synthetics as a byproduct of hybrid maize breeding, research article, marker-assisted introgression of leaf rust resistance from triticum turgidum cv. trinakria to bread wheat variety hd3086, marker-trait association of the major pyruvate pathway genes regulating yield related traits in rice (oryza sativa l.), a combination of analytical methods dissects genotype × environment interaction precisely and facilitates the selection of potential new field corn (zea mays l.) hybrids., genotypic variation in diverse bread wheat (triticum aestivum l.) for photosynthesis related traits, biomass and yield in response to low phosphorus stress, allelic distribution and association of key gene specific markers with rice yield under acidic soil., genetic and phyto-morphological diversity analysis in the advanced breeding lines of chickpea for pod borer (helicoverpa armigera) resistance traits genetic variability for pod borer resistance in chickpea, assessing genetic variability and identifying stable soybean genotypes across rainfed and irrigated planting conditions using multi-trait stability index (mtsi), diversity of heading date 1 (hd1) gene that conditions flowering time in traditional tropical japonica and traditional indica rice from thailand, morphological characterization and dna barcoding of farmers’ varieties of rice (oryza sativa l.) of central india, heterotic grouping methods reveal differential breeding efficiencies and season-specific categorization of inbreds in tropical maize (zea mays l.), response of chickpea (cicer arietinum l.) genotypes to high temperature under late sown condition., discerning of ahu rice (oryza sativa l) landraces of assam for phosphorus deficiency tolerance using molecular and morphological approaches, identification of fodder cowpea genotypes by leveraging gge biplot under multi-environment introduction, material and methods, results and discussion, conclusion, reference, understanding the number of genes governing fertility restoration and isolation of potential restorers on maldandi source of male sterility in rabi sorghum [sorghum bicolor (l.) moench], unveiling genotype × environment dynamics for grain yield in qpm hybrids through ammi, gge biplot, and mtsi approach, enhancing tobacco (nicotiana tabaccum l.) breeding efficiency utilizing gblup through ssr markers for superior parental selection based on leaf quality traits, pathogenic and genetic variation among fusarium graminearum isolates causing head scab of wheat in india variation among fusarium graminearum isolates causing head scab, characterization of vegetable soybean (edamame) germplasm and assessment of optimal food quality traits, an approach to identify stable genotypes based on mtsi and mgdii indexes in okra [abelmoschus esculentus (l.) moench], notification and germplasm registration.
Academia.edu no longer supports Internet Explorer.
To browse Academia.edu and the wider internet faster and more securely, please take a few seconds to upgrade your browser .
- We're Hiring!
- Help Center
Plant breeding and genetics
- Most Cited Papers
- Most Downloaded Papers
- Newest Papers
- Last »
- Plant Breeding Follow Following
- Plant biotechnology Follow Following
- Genetics and Plant Breeding Follow Following
- Genetics Follow Following
- Plant Molecular Biology Follow Following
- Marker assisted plant breeding Follow Following
- Molecular Biology Follow Following
- Molecular Markers Follow Following
- Biotechnology Follow Following
- Crop improvements Follow Following
Enter the email address you signed up with and we'll email you a reset link.
- Academia.edu Journals
- We're Hiring!
- Help Center
- Find new research papers in:
- Health Sciences
- Earth Sciences
- Cognitive Science
- Mathematics
- Computer Science
- Academia ©2024
Information
- Author Services
Initiatives
You are accessing a machine-readable page. In order to be human-readable, please install an RSS reader.
All articles published by MDPI are made immediately available worldwide under an open access license. No special permission is required to reuse all or part of the article published by MDPI, including figures and tables. For articles published under an open access Creative Common CC BY license, any part of the article may be reused without permission provided that the original article is clearly cited. For more information, please refer to https://www.mdpi.com/openaccess .
Feature papers represent the most advanced research with significant potential for high impact in the field. A Feature Paper should be a substantial original Article that involves several techniques or approaches, provides an outlook for future research directions and describes possible research applications.
Feature papers are submitted upon individual invitation or recommendation by the scientific editors and must receive positive feedback from the reviewers.
Editor’s Choice articles are based on recommendations by the scientific editors of MDPI journals from around the world. Editors select a small number of articles recently published in the journal that they believe will be particularly interesting to readers, or important in the respective research area. The aim is to provide a snapshot of some of the most exciting work published in the various research areas of the journal.
Original Submission Date Received: .
- Active Journals
- Find a Journal
- Proceedings Series
- For Authors
- For Reviewers
- For Editors
- For Librarians
- For Publishers
- For Societies
- For Conference Organizers
- Open Access Policy
- Institutional Open Access Program
- Special Issues Guidelines
- Editorial Process
- Research and Publication Ethics
- Article Processing Charges
- Testimonials
- Preprints.org
- SciProfiles
- Encyclopedia
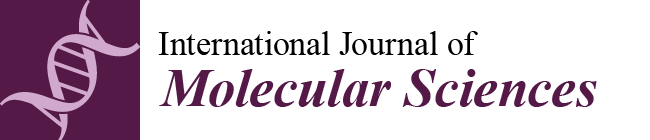
Article Menu
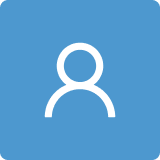
- Subscribe SciFeed
- Recommended Articles
- Google Scholar
- on Google Scholar
- Table of Contents
Find support for a specific problem in the support section of our website.
Please let us know what you think of our products and services.
Visit our dedicated information section to learn more about MDPI.
JSmol Viewer
Phenotypic, metabolic and genetic adaptations of the ficus species to abiotic stress response: a comprehensive review.
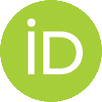
1. Introduction
Click here to enlarge figure
2. Adaption of the Ficus Species to Tropical Environment
3. the responses of ficus to abiotic stress, 3.1. the responses of ficus to heat stress, 3.2. the responses of ficus to drought stress, 3.3. the responses of ficus to flood stress, 3.4. the responses of ficus to oxidative stress, 3.5. the responses of ficus to saline-alkali stress, 4. the adaptive genes and molecular mechanism of ficus relating to abiotic stress, 5. potential application of ficus genes in genetic breeding, 6. conclusions, author contributions, conflicts of interest.
- Zhang, X.; Wang, G.; Zhang, S.; Cheng, S.; Wang, Y.; Wen, P.; Ma, X.; Shi, Y.; Qi, R.; Yang, Y.; et al. Genomes of the Banyan Tree and Pollinator Wasp Provide Insights into Fig-Wasp Coevolution. Cell 2020 , 183 , 875–889. [ Google Scholar ] [ CrossRef ] [ PubMed ]
- Jianfeng, H.; Rui, X.; Yanqiong, P. Research progress of interspecific hybridization in genus Ficus . Biodivers. Sci. 2019 , 27 , 457. [ Google Scholar ] [ CrossRef ]
- Singh, B.; Sharma, R.A. Updated review on Indian Ficus species. Arab. J. Chem. 2023 , 16 , 104976. [ Google Scholar ] [ CrossRef ]
- Cheng, J.-X.; Zhang, B.-D.; Zhu, W.-F.; Zhang, C.-F.; Qin, Y.-M.; Abe, M.; Akihisa, T.; Liu, W.-Y.; Feng, F.; Zhang, J. Traditional uses, phytochemistry, and pharmacology of Ficus hispida L.f.: A review. J. Ethnopharmacol. 2020 , 248 , 112204. [ Google Scholar ] [ CrossRef ] [ PubMed ]
- Zhang, Z.-R.; Yang, X.; Li, W.-Y.; Peng, Y.-Q.; Gao, J. Comparative chloroplast genome analysis of Ficus (Moraceae): Insight into adaptive evolution and mutational hotspot regions. Front. Plant Sci. 2022 , 13 , 965335. [ Google Scholar ] [ CrossRef ] [ PubMed ]
- Debbarma, S.; Banik, B.; Mili, M.; Bora, D.; Das, S.; Datta, B.K.; Baijnath, H. Cryptovivipary: A rare phenomenon in monoecious species of Ficus L. (Moraceae). S. Afr. J. Bot. 2024 , 167 , 391–398. [ Google Scholar ] [ CrossRef ]
- Hao, G.Y.; Goldstein, G.; Sack, L.; Holbrook, N.M.; Liu, Z.H.; Wang, A.Y.; Harrison, R.D.; Su, Z.H.; Cao, K.F. Ecology of hemiepiphytism in fig species is based on evolutionary correlation of hydraulics and carbon economy. Ecology 2011 , 92 , 2117–2130. [ Google Scholar ] [ CrossRef ]
- Mellal, M.K.; Khelifa, R.; Chelli, A.; Djouadi, N.; Madani, K. Combined Effects of Climate and Pests on Fig ( Ficus carica L.) Yield in a Mediterranean Region: Implications for Sustainable Agricultural Strategies. Sustainability 2023 , 15 , 5820. [ Google Scholar ] [ CrossRef ]
- Wijaya, I.M.S.; Defiani, M.R. Diversity and distribution of figs ( Ficus : Moraceae) in Gianyar District, Bali, Indonesia. Biodiversitas J. Biol. Divers. 2021 , 22 , 233–246. [ Google Scholar ] [ CrossRef ]
- Raji, I.A.; Downs, C.T. Tree visitation and potential seed dispersal of keystone Ficus species by vertebrates in an urban mosaic landscape in eastern South Africa. Acta Oecologica 2022 , 117 , 103865. [ Google Scholar ] [ CrossRef ]
- Raji, I.A.; Downs, C.T. Ficus -frugivore interactions, especially in areas of land-use change, in Africa: A systematic review. Acta Oecologica 2021 , 113 , 103774. [ Google Scholar ] [ CrossRef ]
- Shanahan, M.; So, S.; Compton, S.G.; Corlett, R. Fig-eating by vertebrate frugivores: A global review. Biol. Rev. 2001 , 76 , 529–572. [ Google Scholar ] [ CrossRef ]
- Rønsted, N.; Salvo, G.; Savolainen, V. Biogeographical and phylogenetic origins of African fig species ( Ficus section Galoglychia). Mol. Phylogenetics Evol. 2007 , 43 , 190–201. [ Google Scholar ] [ CrossRef ] [ PubMed ]
- Foster, M.S. Can fruit pulp meet the calcium needs of tropical frugivorous passerines during reproduction? J. Trop. Ecol. 2014 , 30 , 79–88. [ Google Scholar ] [ CrossRef ]
- Sinu, P.A.; Unni, A.P.; Jose, T. Biotic Seed Dispersal Mechanisms of Tropical Rain Forests—Bats, Fishes, and Migratory Birds. In Reproductive Ecology of Flowering Plants: Patterns and Processes ; Tandon, R., Shivanna, K.R., Koul, M., Eds.; Springer: Singapore, 2020; pp. 299–334. [ Google Scholar ] [ CrossRef ]
- Isa, M.; Jaafar, M.; Kasim, K.; Mutalib, M. Cultivation of fig ( Ficus carica L.) as an alternative high value crop in Malaysia: A brief review. IOP Conf. Ser. Mater. Sci. Eng. 2020 , 864 , 012134. [ Google Scholar ] [ CrossRef ]
- Devi, R.; Manjula, B.; Kumar, M.; Manjula, B. Food and medicinal values of some Ficus species. Med. Bio-Wealth India 2022 , 6 , 21–26. [ Google Scholar ] [ CrossRef ]
- Mascellani, A.; Natali, L.; Cavallini, A.; Mascagni, F.; Caruso, G.; Gucci, R.; Havlik, J.; Bernardi, R. Moderate Salinity Stress Affects Expression of Main Sugar Metabolism and Transport Genes and Soluble Carbohydrate Content in Ripe Fig Fruits ( Ficus carica L. cv. Dottato). Plants 2021 , 10 , 1861. [ Google Scholar ] [ CrossRef ] [ PubMed ]
- Mazzeo, A.; Magarelli, A.; Ferrara, G. The fig ( Ficus carica L.): Varietal evolution from Asia to Puglia region, southeastern Italy. CABI Agric. Biosci. 2024 , 5 , 57. [ Google Scholar ] [ CrossRef ]
- Ammar, A.; Aissa, I.B.E.N.; Mars, M.; Gouiaa, M. Seasonal variation of fig tree ( Ficus carica L.) physiological characteristics reveals its adaptation performance. S. Afr. J. Bot. 2020 , 132 , 30–37. [ Google Scholar ] [ CrossRef ]
- Ashraf, K.; Haque, M.R.; Amir, M.; Amir, M.; Ahmad, N.; Ahmad, W.; Sultan, S.; Ali Shah, S.A.; Alafeefy, A.M.; Mujeeb, M.; et al. An Overview of Phytochemical and Biological Activities: Ficus deltoidea Jack and Other Ficus spp. J. Pharm. Bioallied Sci. 2021 , 13 , 11–25. [ Google Scholar ] [ CrossRef ]
- Mohammad, N.; Wei, Y.K.; Bakar, N.F.A. Determination of Mineral Content in The Ficus Deltoidea Leaves. J. Sains Kesihat. Malays. 2012 , 10 , 25–29. [ Google Scholar ] [ CrossRef ]
- Lansky, E.P.; Paavilainen, H.M.; Pawlus, A.D.; Newman, R.A. Ficus spp. (fig): Ethnobotany and potential as anticancer and anti-inflammatory agents. J. Ethnopharmacol. 2008 , 119 , 195–213. [ Google Scholar ] [ CrossRef ] [ PubMed ]
- Zhou, P.; Qian, J.; Yuan, W.; Yang, X.; Di, B.; Meng, Y.; Shao, J. Effects of Interval Flooding Stress on Physiological Characteristics of Apple Leaves. Horticulturae 2021 , 7 , 331. [ Google Scholar ] [ CrossRef ]
- Xu, Y.; Hu, W.; Liu, J.; Song, S.; Hou, X.; Jia, C.; Li, J.; Miao, H.; Wang, Z.; Tie, W.; et al. An aquaporin gene MaPIP2-7 is involved in tolerance to drought, cold and salt stresses in transgenic banana ( Musa acuminata L.). Plant Physiol. Biochem. 2020 , 147 , 66–76. [ Google Scholar ] [ CrossRef ]
- Wang, X.; Gao, Y.; Wang, Q.; Chen, M.; Ye, X.; Li, D.; Chen, X.; Li, L.; Gao, D. 24-Epibrassinolide-alleviated drought stress damage influences antioxidant enzymes and autophagy changes in peach ( Prunus persicae L.) leaves. Plant Physiol. Biochem. 2019 , 135 , 30–40. [ Google Scholar ] [ CrossRef ] [ PubMed ]
- Mahmoud, L.M.; Shalan, A.M.; El-Boray, M.S.; Vincent, C.I.; El-Kady, M.E.; Grosser, J.W.; Dutt, M. Application of silicon nanoparticles enhances oxidative stress tolerance in salt stressed ‘Valencia’ sweet orange plants. Sci. Hortic. 2022 , 295 , 110856. [ Google Scholar ] [ CrossRef ]
- Xu, Y.; Wang, Y.; Xu, J.; Lv, Z.; Manzoor, M.A.; Mao, J.; Zhang, X.; Liu, R.; Wang, S.; Whiting, M.D.; et al. Strigolactone and salicylic acid regulate the expression of multiple stress-related genes and enhance the drought resistance of cherry rootstocks. Sci. Hortic. 2023 , 313 , 111827. [ Google Scholar ] [ CrossRef ]
- Ahmad, M.; Alabd, A.; Gao, Y.; Yu, W.; Jamil, W.; Wang, X.; Wei, J.; Ni, J.; Teng, Y.; Bai, S. Three stress-responsive NAC transcription factors, Pp-SNACs, differentially and synergistically regulate abiotic stress in pear. Sci. Hortic. 2022 , 305 , 111393. [ Google Scholar ] [ CrossRef ]
- Samarasinghe, C.R.K.; Meegahakumbura, M.K.; Dissanayaka, H.D.M.A.C.; Kumarathunge, D.; Perera, L. Variation in yield and yield components of different coconut cultivars in response to within year rainfall and temperature variation. Sci. Hortic. 2018 , 238 , 51–57. [ Google Scholar ] [ CrossRef ]
- Liang, C. Research Progress of Fig Cultivation and Postharvest Technology. Chin. Fruits Veg. 2022 , 42 , 78–84. [ Google Scholar ] [ CrossRef ]
- Krause, G.H.; Winter, K.; Krause, B.; Jahns, P.; García, M.; Aranda, J.; Virgo, A. High-temperature tolerance of a tropical tree, Ficus insipida : Methodological reassessment and climate change considerations. Funct. Plant Biol. 2010 , 37 , 890–900. [ Google Scholar ] [ CrossRef ]
- Sadder, M.T.; Alshomali, I.; Ateyyeh, A.; Musallam, A. Physiological and molecular responses for long term salinity stress in common fig ( Ficus carica L.). Physiol. Mol. Biol. Plants 2021 , 27 , 107–117. [ Google Scholar ] [ CrossRef ] [ PubMed ]
- Ammar, A.; Aissa, I.B.; Gouiaa, M.; Mars, M. Fig ( Ficus carica L.) vulnerability to climate change: Combined effects of water stress and high temperature on ecophysiological behaviour of different cultivars. S. Afr. J. Bot. 2022 , 147 , 482–492. [ Google Scholar ] [ CrossRef ]
- Ammar, A.; Ben Aissa, I.; Zaouay, F.; Gouiaa, M.; Mars, M. Physiological behaviour of fig tree ( Ficus carica L.) under different climatic conditions. In Fig (Ficus carica): Production, Processing, and Properties ; Springer International Publishing: Cham, Switzerland, 2023; pp. 247–257. [ Google Scholar ] [ CrossRef ]
- Richter, S.; Anders, N.; Wolters, H.; Beckmann, H.; Thomann, A.; Heinrich, R.; Schrader, J.; Singh, M.K.; Geldner, N.; Mayer, U.; et al. Role of the GNOM gene in Arabidopsis apical-basal patterning—From mutant phenotype to cellular mechanism of protein action. Eur. J. Cell Biol. 2010 , 89 , 138–144. [ Google Scholar ] [ CrossRef ]
- Jia, W.; Ma, M.; Chen, J.; Wu, S. Plant Morphological, Physiological and Anatomical Adaption to Flooding Stress and the Underlying Molecular Mechanisms. Int. J. Mol. Sci. 2021 , 22 , 1088. [ Google Scholar ] [ CrossRef ] [ PubMed ]
- Liu, N.; Lin, Z. Reactive Oxygen Species and Relative Enzyme Activities in the Development of Aerial Roots of Chinese Banyan ( Ficus microcarpa ). J. Plant Growth Regul. 2014 , 33 , 160–168. [ Google Scholar ] [ CrossRef ]
- Gautam, R.; Meena, R.K.; Rampuria, S.; Shukla, P.; Kirti, P.B. Ectopic expression of DnaJ type-I protein homolog of Vigna aconitifolia (VaDJI) confers ABA insensitivity and multiple stress tolerance in transgenic tobacco plants. Front. Plant Sci. 2023 , 14 , 1135552. [ Google Scholar ] [ CrossRef ]
- He, J.; Li, P.; Huo, H.; Liu, L.; Tang, T.; He, M.; Huang, J.; Liu, L. Heterologous expression of HpBHY and CrBKT increases heat tolerance in Physcomitrella patens. Plant Divers. 2019 , 41 , 266–274. [ Google Scholar ] [ CrossRef ]
- Ramkumar, T.R.; Lenka, S.K.; Arya, S.S.; Bansal, K.C. A short history and perspectives on plant genetic transformation. In Biolistic DNA Delivery in Plants: Methods and Protocols ; Springer: New York, NY, USA, 2020; pp. 39–68. [ Google Scholar ] [ CrossRef ]
- Kumar, S.; Suleski, M.; Craig, J.M.; Kasprowicz, A.E.; Sanderford, M.; Li, M.; Stecher, G.; Hedges, S.B. TimeTree 5: An Expanded Resource for Species Divergence Times. Mol. Biol. Evol. 2022 , 39 , msac174. [ Google Scholar ] [ CrossRef ]
- Harrison, R.D. Figs and the diversity of tropical rainforests. Bioscience 2005 , 55 , 1053–1064. [ Google Scholar ] [ CrossRef ]
- Harrison, R.D.; Shanahan, M. Seventy-seven ways to be a fig: Overview of a diverse plant assemblage. In Pollination Ecology and the Rain Forest: Sarawak Studies ; Springer: New York, NY, USA, 2005; pp. 111–127. [ Google Scholar ] [ CrossRef ]
- Bao, Y.; He, M.; Zhang, C.; Jiang, S.; Zhao, L.; Ye, Z.; Sun, Q.; Xia, Z.; Zou, M. Advancing understanding of Ficus carica : A comprehensive genomic analysis reveals evolutionary patterns and metabolic pathway insights. Front. Plant Sci. 2023 , 14 , 1298417. [ Google Scholar ] [ CrossRef ] [ PubMed ]
- Usai, G.; Mascagni, F.; Giordani, T.; Vangelisti, A.; Bosi, E.; Zuccolo, A.; Ceccarelli, M.; King, R.; Hassani-Pak, K.; Zambrano, L.S.; et al. Epigenetic patterns within the haplotype phased fig ( Ficus carica L.) genome. Plant J. 2020 , 102 , 600–614. [ Google Scholar ] [ CrossRef ]
- Ashalatha, K.L.; Arunkumar, K.P.; Gowda, M. Genomic and transcriptomic analysis of sacred fig ( Ficus religiosa ). BMC Genom. 2023 , 24 , 197. [ Google Scholar ] [ CrossRef ] [ PubMed ]
- Shirasawa, K.; Yakushiji, H.; Nishimura, R.; Morita, T.; Jikumaru, S.; Ikegami, H.; Toyoda, A.; Hirakawa, H.; Isobe, S. The Ficus erecta genome aids Ceratocystis canker resistance breeding in common fig ( F. carica ). Plant J. 2020 , 102 , 1313–1322. [ Google Scholar ] [ CrossRef ] [ PubMed ]
- Brodersen, C.R. Finding support for theoretical tradeoffs in xylem structure and function. New Phytol. 2015 , 209 , 8–10. [ Google Scholar ] [ CrossRef ]
- Fan, Z.-X.; Sterck, F.; Zhang, S.-B.; Fu, P.-L.; Hao, G.-Y. Tradeoff between Stem Hydraulic Efficiency and Mechanical Strength Affects Leaf–Stem Allometry in 28 Ficus Tree Species. Front. Plant Sci. 2017 , 8 , 1619. [ Google Scholar ] [ CrossRef ]
- Liu, J.-Y.; Fu, P.-L.; Wang, Y.-J.; Cao, K.-F. Different Drought-adaptation Strategies as Characterized by Hydraulic and Water-relations Traits of Evergreen and Deciduous Figs in a Tropical Karst Forest. Plant Sci. J. 2012 , 30 , 484. [ Google Scholar ] [ CrossRef ]
- Wisniewska, J.; Xu, J.; Seifertová, D.; Brewer, P.B.; Ruzicka, K.; Blilou, I.; Rouquié, D.; Benková, E.; Scheres, B.; Friml, J. Polar PIN localization directs auxin flow in plants. Science 2006 , 312 , 883. [ Google Scholar ] [ CrossRef ]
- Marhava, P. Recent developments in the understanding of PIN polarity. New Phytol. 2022 , 233 , 624–630. [ Google Scholar ] [ CrossRef ]
- Zhang, H.; Zhu, J.; Gong, Z.; Zhu, J. Abiotic stress responses in plants. Nat. Rev. Genet. 2022 , 23 , 104–119. [ Google Scholar ] [ CrossRef ]
- Zhang, H.; Liu, S.; Ren, T.; Niu, M.; Liu, X.; Liu, C.; Wang, H.; Yin, W.; Xia, X. Crucial Abiotic Stress Regulatory Network of NF-Y Transcription Factor in Plants. Int. J. Mol. Sci. 2023 , 24 , 4426. [ Google Scholar ] [ CrossRef ] [ PubMed ]
- Junaid, M.; Gokce, A. Global agricultural losses and their causes. Bull. Biol. Allied Sci. Res. 2024 , 2024 , 66. [ Google Scholar ] [ CrossRef ]
- Fang, C.; Chen, H.; Castillo-Díaz, D.; Wen, B.; Cao, K.; Goodale, U. Regeneration and Endogenous Phytohormone Responses to High-Temperature Stress Drive Recruitment Success in Hemiepiphytic Fig Species. Front. Plant Sci. 2021 , 12 , 754207. [ Google Scholar ] [ CrossRef ]
- Zhang, K.; Liu, X.; Shen, J.; Li, Z.; Sang, Y.; Wu, X.; Zha, Y.; Liang, W.; Wang, C.; Wang, K.; et al. Clinically Applicable AI System for Accurate Diagnosis, Quantitative Measurements, and Prognosis of COVID-19 Pneumonia Using Computed Tomography. Cell 2020 , 182 , 1360. [ Google Scholar ] [ CrossRef ]
- Hao, G.-Y.; Wang, A.-Y.; Liu, Z.-H.; Franco, A.C.; Goldstein, G.; Cao, K.-F. Differentiation in light energy dissipation between hemiepiphytic and non-hemiepiphytic Ficus species with contrasting xylem hydraulic conductivity. Tree Physiol. 2011 , 31 , 626–636. [ Google Scholar ] [ CrossRef ]
- Ammar, A.; Ben Aissa, I.; Mars, M.; Gouiaa, M. Comparative physiological behavior of fig ( Ficus carica L.) cultivars in response to water stress and recovery. Sci. Hortic. 2020 , 260 , 108881. [ Google Scholar ] [ CrossRef ]
- Zafer Can, H.; Aksoy, U. Seasonal and diurnal photosynthetic behaviour of fig ( Ficus carica L.) under semi-arid climatic conditions. Acta Agric. Scand. Sect. B Soil Plant Sci. 2007 , 57 , 297–306. [ Google Scholar ] [ CrossRef ]
- González-Rodríguez, A.M.; Peters, J. Strategies of leaf expansion in Ficus carica under semiarid con ditions. Plant Biol. 2010 , 12 , 469–474. [ Google Scholar ] [ CrossRef ] [ PubMed ]
- Vangelisti, A.; Zambrano, L.S.; Caruso, G.; Macheda, D.; Bernardi, R.; Usai, G.; Mascagni, F.; Giordani, T.; Gucci, R.; Cavallini, A.; et al. How an ancient, salt-tolerant fruit crop, Ficus carica L., copes with salinity: A transcriptome analysis. Sci. Rep. 2019 , 9 , 2561. [ Google Scholar ] [ CrossRef ]
- Jin, S.H.; Li, X.Q.; Wang, J.G. Effects of high temperature stress on photosynthesis in Ficus concinna var. subsessilis. Chin. Agric. Sci. Bull. 2009 , 25 , 83–87. [ Google Scholar ] [ CrossRef ]
- Zhang, S.; Huang, W.; Zhang, J.; Cao, K. Differential responses of photosystems I and II to seasonal drought in two Ficus species. Acta Oecologica-Int. J. Ecol. 2016 , 73 , 53–60. [ Google Scholar ] [ CrossRef ]
- Khandaker, M.M.; Jamaludin, R.; Majrashi, A.; Rashid, Z.M.; Karim, S.M.R.; Al-Yasi, H.M.; Badaluddin, N.A.; Alenazi, M.M.; Mohd, K.S. Enhancing Rubisco gene expression and metabolites accumulation for better plant growth in Ficus deltoidea under drought stress using hydrogen peroxide. Front. Plant Sci. 2022 , 13 , 965765. [ Google Scholar ] [ CrossRef ] [ PubMed ]
- Parveen, S.; Rashid, M.H.-U.; Inafuku, M.; Oku, H. Molecular regulatory mechanism of isoprene emission under short-term drought stress in the tropical tree Ficus septica . Tree Physiol. 2018 , 39 , 440–453. [ Google Scholar ] [ CrossRef ]
- Johnson, C.R.; Nell, T.A.; Rosenbaum, S.E.; Lauritis, J.A. Influence of light intensity and drought stress on Ficus benjamina L. J. Am. Soc. Hortic. Sci. 1982 , 107 , 252–255. [ Google Scholar ] [ CrossRef ]
- Yamasaki, H.; Takahashi, S.; Heshiki, R. The tropical fig Ficus microcarpa L. f. cv. golden leaves lacks heat-stable dehydroascorbate reductase activity. Plant Cell Physiol. 1999 , 40 , 640–646. [ Google Scholar ] [ CrossRef ]
- Liu, N.; Cao, C.; Sun, Z.; Lin, Z.; Deng, R. Pollutant-induced cell death and reactive oxygen species accumulation in the aerial roots of Chinese banyan ( Ficus microcarpa ). Sci. Rep. 2016 , 6 , 36276. [ Google Scholar ] [ CrossRef ] [ PubMed ]
- Jin, S.-h.; Li, X.-q.; Hu, J.-y.; Wang, J.-g. Cyclic electron flow around photosystem I is required for adaptation to high temperature in a subtropical forest tree, Ficus concinna . J. Zhejiang Univ. Sci. B 2009 , 10 , 784–790. [ Google Scholar ] [ CrossRef ]
- Voesenek, L.A.; Bailey-Serres, J. Flood adaptive traits and processes: An overview. New Phytol. 2015 , 206 , 57–73. [ Google Scholar ] [ CrossRef ]
- Yang, F.; Wang, Y.; Chan, Z. Perspectives on screening winter-flood-tolerant woody species in the riparian protection forests of the Three Gorges Reservoir. PLoS ONE 2014 , 9 , e108725. [ Google Scholar ] [ CrossRef ]
- Pothasin, P.; Compton, S.G.; Wangpakapattanawong, P. Riparian Ficus tree communities: The distribution and abundance of riparian fig trees in northern Thailand. PLoS ONE 2014 , 9 , e108945. [ Google Scholar ] [ CrossRef ]
- Pothasin, P.; Compton, S.G.; Wangpakapattanawong, P. Seasonality of leaf and fig production in Ficus squamosa , a fig tree with seeds dispersed by water. PLoS ONE 2016 , 11 , e0152380. [ Google Scholar ] [ CrossRef ]
- Smitha, R.B.; Bennans, T.; Mohankumar, C.; Benjamin, S. Oxidative stress enzymes in Ficus religiosa L.: Biochemical, histochemical and anatomical evidences. J. Photochem. Photobiol. B Biol. 2009 , 95 , 17–25. [ Google Scholar ] [ CrossRef ]
- Sato, H.; Mizoi, J.; Shinozaki, K.; Yamaguchi-Shinozaki, K. Complex plant responses to drought and heat stress under climate change. Plant J. 2024 , 117 , 1873–1892. [ Google Scholar ] [ CrossRef ] [ PubMed ]
- Moore, C.E.; Meacham-Hensold, K.; Lemonnier, P.; Slattery, R.A.; Benjamin, C.; Bernacchi, C.J.; Lawson, T.; Cavanagh, A.P. The effect of increasing temperature on crop photosynthesis: From enzymes to ecosystems. J. Exp. Bot. 2021 , 72 , 2822–2844. [ Google Scholar ] [ CrossRef ] [ PubMed ]
- Guilioni, L.; Wery, J.; Tardieu, F. Heat Stress-induced Abortion of Buds and Flowers in Pea: Is Sensitivity Linked to Organ Age or to Relations between Reproductive Organs? Ann. Bot. 1997 , 80 , 159–168. [ Google Scholar ] [ CrossRef ]
- Srivalli, B.; Khanna-Chopra, R. The developing reproductive ‘sink’ induces oxidative stress to mediate nitrogen mobilization during monocarpic senescence in wheat. Biochem. Biophys. Res. Commun. 2004 , 325 , 198–202. [ Google Scholar ] [ CrossRef ] [ PubMed ]
- Pandey, V.; Singh, S. Plant Adaptation and Tolerance to Heat Stress: Advance Approaches and Future Aspects. CCHTS 2024 , 27 , 1701–1715. [ Google Scholar ] [ CrossRef ]
- Hu, S.; Ding, Y.; Zhu, C. Sensitivity and Responses of Chloroplasts to Heat Stress in Plants. Front. Plant Sci. 2020 , 11 , 375. [ Google Scholar ] [ CrossRef ]
- Wu, X.; Yao, X.; Chen, J.; Zhu, Z.; Zhang, H.; Zha, D. Brassinosteroids protect photosynthesis and antioxidant system of eggplant seedlings from high-temperature stress. Acta Physiol. Plant. 2013 , 36 , 251–261. [ Google Scholar ] [ CrossRef ]
- Guo, M.; Liu, J.-H.; Ma, X.; De-Xu Luo, D.-X.; Gong, Z.-H.; Lu, M.-H. The Plant Heat Stress Transcription Factors (HSFs): Structure, Regulation, and Function in Response to Abiotic Stresses. Front. Plant Sci. 2016 , 7 , 114. [ Google Scholar ] [ CrossRef ]
- Goyal, M.; Banerjee, C.; Nag, S.; Bandyopadhyay, U. The Alba protein family: Structure and function. Biochim. Et Biophys. Acta (BBA)-Proteins Proteom. 2016 , 1864 , 570–583. [ Google Scholar ] [ CrossRef ]
- Tong, J.; Ren, Z.; Sun, L.; Zhou, S.; Yuan, W.; Hui, Y.; Ci, D.; Wang, W.; Fan, L.-M.; Wu, Z.; et al. ALBA proteins confer thermotolerance through stabilizing HSF messenger RNAs in cytoplasmic granules. Nat. Plants 2022 , 8 , 778–791. [ Google Scholar ] [ CrossRef ] [ PubMed ]
- Ling, Y.; Mahfouz, M.M.; Zhou, S. Pre-mRNA alternative splicing as a modulator for heat stress response in plants. Trends Plant Sci. 2021 , 26 , 1153–1170. [ Google Scholar ] [ CrossRef ]
- Diatta, A.A.; Fike, J.H.; Battaglia, M.L.; Galbraith, J.M.; Baig, M.B. Effects of biochar on soil fertility and crop productivity in arid regions: A review. Arab. J. Geosci. 2020 , 13 , 595. [ Google Scholar ] [ CrossRef ]
- Seleiman, M.F.; Al-Suhaibani, N.; Ali, N.; Akmal, M.; Alotaibi, M.; Refay, Y.; Dindaroglu, T.; Abdul-Wajid, H.H.; Battaglia, M.L. Drought Stress Impacts on Plants and Different Approaches to Alleviate Its Adverse Effects. Plants 2021 , 10 , 259. [ Google Scholar ] [ CrossRef ] [ PubMed ]
- O’Connell, E. Towards adaptation of water resource systems to climatic and socio-economic change. Water Resour. Manag. 2017 , 31 , 2965–2984. [ Google Scholar ] [ CrossRef ]
- Sharma, P.; Kumar, S.N. The global governance of water, energy, and food nexus: Allocation and access for competing demands. Int. Environ. Agreem. Politics Law Econ. 2020 , 20 , 377–391. [ Google Scholar ] [ CrossRef ]
- Ahmad, H.M.; Fiaz, S.; Hafeez, S.; Zahra, S.; Shah, A.N.; Gul, B.; Aziz, O.; Fakhar, A.; Rafique, M.; Chen, Y.; et al. Plant Growth-Promoting Rhizobacteria Eliminate the Effect of Drought Stress in Plants: A Review. Front. Plant Sci. 2022 , 13 , 875774. [ Google Scholar ] [ CrossRef ] [ PubMed ]
- Trabelsi, L.; Gargouri, K.; Ben Hassena, A.; Mbadra, C.; Ghrab, M.; Ncube, B.; Van Staden, J.; Gargouri, R. Impact of drought and salinity on olive water status and physiological performance in an arid climate. Agric. Water Manag. 2019 , 213 , 749–759. [ Google Scholar ] [ CrossRef ]
- Pirasteh-Anosheh, H.; Saed-Moucheshi, A.; Pakniyat, H.; Pessarakli, M. Stomatal responses to drought stress. In Water Stress and Crop Plants: A Sustainable Approach ; John Wiley & Sons, Ltd.: Hoboken, NJ, USA, 2016; pp. 24–40. [ Google Scholar ] [ CrossRef ]
- Abdolinejad, R.; Shekafandeh, A. Tetraploidy Confers Superior in vitro Water-Stress Tolerance to the Fig Tree ( Ficus carica ) by Reinforcing Hormonal, Physiological, and Bioc hemical Defensive Systems. Front. Plant Sci. 2022 , 12 , 796215. [ Google Scholar ] [ CrossRef ]
- Knipfer, T.; Bambach, N.; Hernandez, M.I.; Bartlett, M.K.; Sinclair, G.; Duong, F.; Kluepfel, D.A.; McElrone, A.J. Predicting stomatal closure and turgor loss in woody plants using predawn and midday water potential. Plant Physiol. 2020 , 184 , 881–894. [ Google Scholar ] [ CrossRef ] [ PubMed ]
- Xu, D.H.; Fang, X.W.; Su, P.X.; Wang, G. Ecophysiological responses of Caragana korshinskii Kom. under extreme drought stress: Leaf abscission and stem survives. Photosynthetica 2012 , 50 , 541–548. [ Google Scholar ] [ CrossRef ]
- Jackson, M.B.; Colmer, T.D. Response and adaptation by plants to flooding stress. Ann. Bot. 2005 , 96 , 501–505. [ Google Scholar ] [ CrossRef ]
- Fukao, T.; Barrera-Figueroa, B.E.; Juntawong, P.; Peña-Castro, J.M. Submergence and Waterlogging Stress in Plants: A Review Highlighting Research Opportunities and Understudied Aspects. Front. Plant Sci. 2019 , 10 , 340. [ Google Scholar ] [ CrossRef ]
- Lee, S.C.; Mustroph, A.; Sasidharan, R.; Vashisht, D.; Pedersen, O.; Oosumi, T.; Voesenek, L.A.C.J.; Bailey-Serres, J. Molecular characterization of the submergence response of the Arabidopsis thaliana ecotype Columbia. New Phytol. 2011 , 190 , 457–471. [ Google Scholar ] [ CrossRef ] [ PubMed ]
- Hsu, F.-C.; Chou, M.-Y.; Chou, S.-J.; Li, Y.R.; Peng, H.-P.; Shih, M.-C. Submergence confers immunity mediated by the WRKY22 transcription factor in Arabidopsis. Plant Cell 2013 , 25 , 2699–2713. [ Google Scholar ] [ CrossRef ]
- Rivera-Contreras, I.K.; Zamora-Hernández, T.; Huerta-Heredia, A.A.; Capataz-Tafur, J.; Barrera-Figueroa, B.E.; Juntawong, P.; Peña-Castro, J.M. Transcriptomic analysis of submergence-tolerant and sensitive Brachypodium distachyon ecotypes reveals oxidative stress as a major tolerance factor. Sci. Rep. 2016 , 6 , 27686. [ Google Scholar ] [ CrossRef ]
- Furness, H.; Breen, C. The vegetation of seasonally flooded areas of the Pongolo River Floodplain. Bothalia 1980 , 13 , 217–231. [ Google Scholar ] [ CrossRef ]
- Hasanuzzaman, M.; Al Mahmud, J.; Nahar, K.; Anee, T.I.; Inafuku, M.; Oku, H.; Fujita, M. Adaptation, and ROS Metabolism in Plants Exposed to Waterlogging Stress. In Reactive Oxygen Species and Antioxidant Systems in Plants: Role and Regulation under Abiotic Stress ; Khan, M.I.R., Khan, N.A., Eds.; Springer: Singapore, 2017; pp. 257–281. [ Google Scholar ] [ CrossRef ]
- Hasanuzzaman, M.; Hossain, M.A.; da Silva, J.A.T.; Fujita, M. Plant Response and Tolerance to Abiotic Oxidative Stress: Antioxidant Defense Is a Key Factor. In Crop Stress and Its Management: Perspectives and Strategies ; Venkateswarlu, B., Shanker, A., Shanker, C., Maheswari, M., Eds.; Springer: Dordrecht, The Netherlands, 2012; pp. 261–315. [ Google Scholar ] [ CrossRef ]
- Liu, N.; Lin, Z.; Mo, H. Metal (Pb, Cd, and Cu)-induced reactive oxygen species accumulations in aerial root cells of the Chinese banyan ( Ficus microcarpa ). Ecotoxicology 2012 , 21 , 2004–2011. [ Google Scholar ] [ CrossRef ]
- Prăvălie, R.; Patriche, C.; Borrelli, P.; Panagos, P.; Roșca, B.; Dumitraşcu, M.; Nita, I.-A.; Săvulescu, I.; Birsan, M.-V.; Bandoc, G. Arable lands under the pressure of multiple land degradation processes. A global perspective. Environ. Res. 2021 , 194 , 110697. [ Google Scholar ] [ CrossRef ]
- Negacz, K.; Malek, Ž.; de Vos, A.; Vellinga, P. Saline soils worldwide: Identifying the most promising areas for saline agriculture. J. Arid Environ. 2022 , 203 , 104775. [ Google Scholar ] [ CrossRef ]
- Tarolli, P.; Luo, J.; Park, E.; Barcaccia, G.; Masin, R. Soil salinization in agriculture: Mitigation and adaptation strategies combining nature-based solutions and bioengineering. iScience 2024 , 27 , 108830. [ Google Scholar ] [ CrossRef ] [ PubMed ]
- Mustafa, G.; Akhtar, M.S.; Abdullah, R. Global concern for salinity on various agro-ecosystems. In Salt Stress, Microbes, and Plant Interactions: Causes and Solution: Volume 1 ; Akhtar, M.S., Ed.; Springer: Singapore, 2019; pp. 1–19. [ Google Scholar ] [ CrossRef ]
- Hassani, A.; Azapagic, A.; Shokri, N. Global predictions of primary soil salinization under changing climate in the 21st century. Nat. Commun. 2021 , 12 , 6663. [ Google Scholar ] [ CrossRef ]
- Pradhan, C.; Mohanty, M. Submergence Stress: Responses and adaptations in crop plants. In Molecular Stress Physiology of Plants ; Rout, G.R., Das, A.B., Eds.; Springer: New Delhi, India, 2013; pp. 331–357. [ Google Scholar ] [ CrossRef ]
- Yang, Y.; Guo, Y. Elucidating the molecular mechanisms mediating plant salt-stress responses. New Phytol. 2018 , 217 , 523–539. [ Google Scholar ] [ CrossRef ]
- Liu, L.; Zheng, S.; Yang, D.; Zheng, J. Genome-wide in silico identification of glutathione S-transferase (GST) gene family members in fig ( Ficus carica L.) and expression characteristics during fruit color development. Peerj 2023 , 11 , e14406. [ Google Scholar ] [ CrossRef ] [ PubMed ]
- Zhai, Y.; Cui, Y.; Song, M.; Vainstein, A.; Chen, S.; Ma, H. Papain-Like Cysteine Protease Gene Family in Fig ( Ficus carica L.): Genome-Wide Analysis and Expression Patterns. Front. Plant Sci. 2021 , 12 , 681801. [ Google Scholar ] [ CrossRef ]
- Song, M.; Wang, H.; Wang, Z.; Huang, H.; Chen, S.; Ma, H. Genome-Wide Characterization and Analysis of bHLH Transcription Factors Related to Anthocyanin Biosynthesis in Fig ( Ficus carica L.). Front. Plant Sci. 2021 , 12 , 730692. [ Google Scholar ] [ CrossRef ]
- Kim, A.S.; Kim, Y.O.; Ryu, H.J.; Kwak, Y.S.; Lee, J.Y.; Kang, H. Isolation of stress-related genes of rubber particles and latex in fig tree ( Ficus carica ) and their expressions by abiotic stress or plant hormone treatments. Plant Cell Physiol. 2003 , 44 , 412–419. [ Google Scholar ] [ CrossRef ] [ PubMed ]
- Chen, X.X.; Ding, Y.L.; Yang, Y.Q.; Song, C.P.; Wang, B.S.; Yang, S.H.; Guo, Y.; Gong, Z.Z. Protein kinases in plant responses to drought, salt, and cold stress. J. Integr. Plant Biol. 2021 , 63 , 53–78. [ Google Scholar ] [ CrossRef ]
- Gong, Z. Plant abiotic stress: New insights into the factors that activate and modulate plant responses. J. Integr. Plant Biol. 2021 , 63 , 429–430. [ Google Scholar ] [ CrossRef ]
Latin Name | Level | GenBank | Release Date | Genome Size | References |
---|---|---|---|---|---|
Ficus carica | Chromosome | GCA_009761775.1 | December 2019 | 333.4 Mb | [ ] |
Ficus microcarpa | Chromosome | GCA_025413485.1 | September 2022 | 426.6 Mb | [ ] |
Ficus hispida | Chromosome | GCA_025413025.1 | September 2022 | 369.8 Mb | [ ] |
Ficus religiosa | Scaffold | GCA_024759925.1 | August 2022 | 406.1 Mb | [ ] |
Ficus erecta | Contig | GCA_008635985.1 | September 2019 | 595.8 Mb | [ ] |
Species | Response | Stress | References |
---|---|---|---|
Hemiepiphytic Ficus | Morphology, behavior, physiology | Heat, drought | [ , , ] |
Ficus carica L. | Morphology, behavior, physiology | Heat, drought, salt | [ , , , , , ] |
Ficus chartacea var. torulosa | Physiology | Heat | [ ] |
Ficus tinctoria | Physiology | Drought | [ ] |
Ficus racemosa | Physiology | Drought | [ ] |
Ficus deltoidea | Physiology | Drought | [ ] |
Ficus septica | Physiology | Drought | [ ] |
Ficus benjamina L. | Physiology, cytology | Drought | [ ] |
Ficus orthoneura | Morphology | Drought | [ ] |
Ficus microcarpa | Physiology, morphology | Drought, oxidative | [ , ] |
Ficus concinna | Molecular mechanisms | Drought | [ ] |
Ficus ssp. | Morphology | Flood | [ ] |
Ficus tikoua | Viability | Flood | [ ] |
Ficus crytophylla | Behavior | Flood | [ ] |
Ficus squamosa | Behavior | Flood | [ ] |
Ficus religiosa L. | Physiology | oxidative | [ ] |
Hemiepiphytic Ficus | Morphology, behavior, physiology | Heat, drought | [ , , ] |
Stress | Responses | |
---|---|---|
Heat stress | Morphology | Stomatal closure and leaf abscission. |
Physiology | Decreased photosystem activity; reduced photosynthetic rate; increased transpiration rate; elevated levels of IAA, ROS, MG, and lipid peroxidation. | |
Cytology | Reduced chlorophyll synthesis. | |
Molecular | Inactivation of heat-sensitive proteins; synthesis of heat shock proteins. | |
Drought stress | Morphology | Regulated leaf temperature, increased leaf abscission, reduced stomatal conductance; decreased root hydraulic conductance (Lp); and unchanged leaf turgor pressure. |
Physiology | Decreased photosynthetic and transpiration rates; accumulation of dry matter; reduced glutamine; enhanced non-photochemical quenching (NPQ); activation of cyclic electron flow (CEF) and increased isoprene emission rate. | |
Cytology | Reduced chlorophyll synthesis and damaged thylakoid structure. | |
Molecular | Increased transcription of POD2, POD4, Cn-ZnSOD2, and Mn-SOD1; decreased transcription of APX1. | |
Flood stress | Morphology | Seed dispersal via water; reduced stomatal conductance; formation of aerial prop roots. |
Physiology | Nutrient imbalance; accumulation of ROS; decreased photosynthetic rate; increased ethylene production. | |
Cytology | Damaged membrane integrity. | |
Molecular | Increased ADH activity and proline content. | |
Oxidative stress | Morphology | Decreased antioxidant capacity during senescence. |
Physiology | Increased hydrogen peroxide and malondialdehyde levels, increased POX activity; and lipid peroxidation. | |
Cytology | Decreased cell viability in adventitious roots; damage to the cell wall and plasma membrane. | |
Molecular | Ascorbate-glutathione (AsA-GSH) pathway. | |
Salt stress | Morphology | Reduced stomatal conductance. |
Physiology | Decreased photosynthetic rate; increased sucrose and d-sorbitol; downregulated glycolytic metabolism. | |
Cytology | Decreased chlorophyll content; altered cell wall composition. | |
Molecular | Increased transcription of carbohydrate transport genes; overexpression of ROS signaling proteins and proline synthesis coding genes. |
The statements, opinions and data contained in all publications are solely those of the individual author(s) and contributor(s) and not of MDPI and/or the editor(s). MDPI and/or the editor(s) disclaim responsibility for any injury to people or property resulting from any ideas, methods, instructions or products referred to in the content. |
Share and Cite
Yuan, S.; Yin, T.; He, H.; Liu, X.; Long, X.; Dong, P.; Zhu, Z. Phenotypic, Metabolic and Genetic Adaptations of the Ficus Species to Abiotic Stress Response: A Comprehensive Review. Int. J. Mol. Sci. 2024 , 25 , 9520. https://doi.org/10.3390/ijms25179520
Yuan S, Yin T, He H, Liu X, Long X, Dong P, Zhu Z. Phenotypic, Metabolic and Genetic Adaptations of the Ficus Species to Abiotic Stress Response: A Comprehensive Review. International Journal of Molecular Sciences . 2024; 25(17):9520. https://doi.org/10.3390/ijms25179520
Yuan, Shengyun, Tianxiang Yin, Hourong He, Xinyi Liu, Xueyan Long, Pan Dong, and Zhenglin Zhu. 2024. "Phenotypic, Metabolic and Genetic Adaptations of the Ficus Species to Abiotic Stress Response: A Comprehensive Review" International Journal of Molecular Sciences 25, no. 17: 9520. https://doi.org/10.3390/ijms25179520
Article Metrics
Article access statistics, further information, mdpi initiatives, follow mdpi.
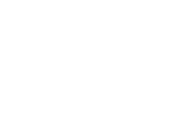
Subscribe to receive issue release notifications and newsletters from MDPI journals
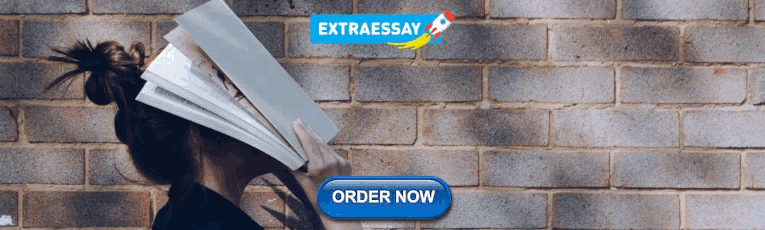
IMAGES
VIDEO
COMMENTS
Find the latest research and news on plant breeding from Nature Portfolio, covering topics such as genetic engineering, crop improvement and disease resistance.
c Department of Genetics and Plant Breeding, Faculty of Agricultural Sciences, Siksha 'O' Anusandhan (Deemed to be University), Bhubaneswar , Odisha-751029 , India.
RSS Feed. Plant genetics deals with heredity in plants, specifically mechanisms of hereditary transmission and variation of inherited characteristics. Plant genetics differs from animal genetics ...
Plant Breeding is an international agronomy journal devoted to the advancement of plant breeding. Our papers span plant genetics, plant physiology, plant pathology, and plant development. Our wide scope covers all aspects of crop plants and crop improvement of interest to both industry and academia. We provide readers with cutting-edge ...
The marriage between quantitative genetics and plant breeding, albeit nonexclusive, has reaped benefits for both during the last 100 years. Estimates of genetic variances and heritability in many ...
Therefore, crop developmental biology has been an important research area not only for curiosity-driven biological science but also for plant breeding and agriculture. In the history of plant developmental biology, the promotion of molecular genetics in the 1990s and the genome decoding of higher plants beginning in 2000 had a great impact on ...
Plant Breeding Reviews - 2022. Plant Breeding Reviews presents state-of-the-art reviews on plant genetics and the breeding of all types of crops by both traditional means and molecular methods. Many of the crops widely grown today stem from a very narrow genetic base; understanding and preserving crop genetic resources is vital to the security ...
Genetic Research and Plant Breeding. In the past 20 years, plant genetics and breeding research using molecular biology has been greatly improved via the functional analysis of genes, species identification and transformation techniques [ 1 ]. At the same time, innovations in molecular technology have spawned a new discipline called genomics [ 2 ].
In the past 20 years, plant genetics and breeding research using molecular biology has been greatly improved via the functional analysis of genes, species identification and transformation techniques [].At the same time, innovations in molecular technology have spawned a new discipline called genomics [].The most epochal change among them is the development of next-generation sequencing (NGS ...
Abstract. Advancements in molecular approaches have been utilized to breed crops with a wide range of economically valuable traits to develop superior cultivars. This review provides a concise overview of modern breakthroughs in molecular plant production. Genotyping and high-throughput phenotyping methods for predictive plant breeding are ...
The objective of this paper is to discuss plant breeding methods as an evolving technology, considering the increasing levels of knowledge of the underlying mechanisms and the control of the process of generating and selecting superior plant types. ... This study provides a fundamental resource for rice genetics research and breeding, and ...
Plant breeding, Plant breeders, Crops, Crop Improvement, Genetics | Explore the latest full-text research PDFs, articles, conference papers, preprints and more on PLANT BREEDING. Find methods ...
The foundation for molecular plant breeding is an interdisciplinary science that has revolutionized the 21st century with incorporation of genomic research, biotechnology and molecular marker ...
Plant Breeding is an international agronomy journal devoted to the advancement of plant breeding. Our papers span plant genetics, plant physiology, plant pathology, and plant development. Our wide scope covers all aspects of crop plants and crop improvement of interest to both industry and academia. We provide readers with cutting-edge ...
Quantitative genetics is a long-established field of research and application, which has always and will continue to contribute a fundamental underpinning for plant breeding.
This review paper will provide an understanding and a direction for the final evolution of plant breeding in the future. ... classifies the concept, application, and research status of digital breeding, and attempts to provide clarification by borrowing the idea of the levels of driving automation. ... Principles of plant genetics and breeding ...
A paper in the series on The Need for Agricultural Innovation to Sustainably Feed the World by 2050 Plant Breeding and Genetics 1 Italicized terms (except genus/species names and published material titles) are defined in the Glossary. CAST Issue Paper 57 revision 4 windows.indd 1 2/10/2017 4:43:19 PM
Journal publishes original research covering all areas of theoretical and applied plant breeding and genetics. Journal is a periodical for the publication of records of original research in all branches of genetics, plant breeding and cytology, including molecular biology, biotechnology and other related sciences of sufficient importance and of ...
Breeding for adaptive metabolic features will depend on the identification of uncommon substances, such as byproducts of stress metabolism, signal transduction molecules, and molecules involved in plant acclimation (Larrainzar et al. 2009).The identification of metabolic substances may be investigated further by association with expression patterns found in the transcriptome and proteome.
Introduction to Genetics and Plant Breeding. February 2019. February 2019. ISBN: 978-93-87387-22-5. Authors: Jiban Shrestha. Nepal Agricultural Research Council. Amit Chaudhary.
World-renowned, cutting-edge, interdisciplinary plant breeding and genetics program at the University of Georgia. Our researchers are developing improved plant cultivars and training the next generation of students in the latest plant breeding and genetics methods. The research being done at IPBGG contributes to food security and agricultural ...
Conversion of superior bread wheat genotype HD3209 carrying Lr19/Sr25 into CMS line for development of rust-resistant wheat hybrids. Abhimanyu Singh Malik. , Nand Kishore Sharma. & Vinod. Article ...
The Indian Journal of Genetics and Plant Breeding is a periodical for the publication of records of original research in all branches of genetics, plant breeding and cytology, including human genetics, molecular biology and biotechnology, and other cognate sciences of sufficient importance and of such a character as to be of primary interest to ...
2. Plant breeding and genetics , Abiotic and Biotic Stresses in Plants. Potential of Gamma Rays to Improve Grain Yield and Nutritional Quality of Pearl Millet (Pennisetum Glaucum L. ): A Review. Introduction Induced mutation is one of the best alternatives for the improvement of crops as it can help to regenerate and restore the variability ...
The Ficus genus, having radiated from the tropics and subtropics to the temperate zone worldwide, is the largest genus among woody plants, comprising over 800 species. Evolution of the Ficus species results in genetic diversity, global radiation and geographical differentiations, suggesting adaption to diverse environments and coping with stresses. Apart from familiar physiological changes ...