
An official website of the United States government
The .gov means it’s official. Federal government websites often end in .gov or .mil. Before sharing sensitive information, make sure you’re on a federal government site.
The site is secure. The https:// ensures that you are connecting to the official website and that any information you provide is encrypted and transmitted securely.
- Publications
- Account settings
Preview improvements coming to the PMC website in October 2024. Learn More or Try it out now .
- Advanced Search
- Journal List
- Proc Natl Acad Sci U S A
- v.120(47); 2023 Nov 21
- PMC10666095

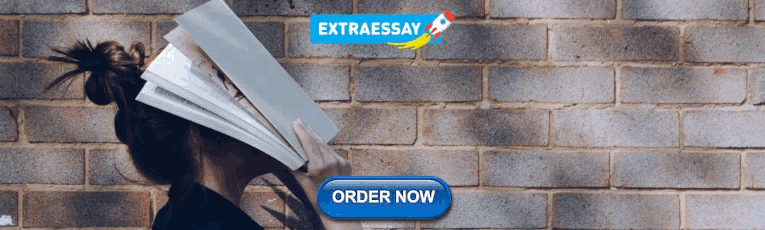
Overcoming bias in the comparison of human language and animal communication
Erica a. cartmill.
a Department of Anthropology, University of California, Los Angeles, CA 90095
b Department of Psychology, University of California, Los Angeles, CA 90095
Associated Data
There are no data underlying this work.
Human language is a powerful communicative and cognitive tool. Scholars have long sought to characterize its uniqueness, but each time a property is proposed to set human language apart (e.g., reference, syntax), some (attenuated) version of that property is found in animals. Recently, the uniqueness argument has shifted from linguistic rules to cognitive capacities underlying them. Scholars argue that human language is unique because it relies on ostension and inference, while animal communication depends on simple associations and largely hardwired signals. Such characterizations are often borne out in published data, but these empirical findings are driven by radical differences in the ways animal and human communication are studied. The field of animal communication has been dramatically shaped by the “code model,” which imagines communication as involving information packets that are encoded, transmitted, decoded, and interpreted. This framework standardized methods for studying meaning in animal signals, but it does not allow for the nuance, ambiguity, or contextual variation seen in humans. The code model is insidious. It is rarely referenced directly, but it significantly shapes how we study animals. To compare animal communication and human language, we must acknowledge biases resulting from the different theoretical models used. By incorporating new approaches that break away from searching for codes, we may find that animal communication and human language are characterized by differences of degree rather than kind.
Human communication draws on highly developed ostensive and inferential capacities ( 1 – 3 ). While much of language is codified and structured, humans can use virtually any behavior to communicate (e.g., a head tilt, a look, moving an object). The ostensive capacity to turn anything into a signal is seen as a powerful driver of human communication. The hypothesized mechanisms underlying this capacity have become one of the last bulwarks of human language’s uniqueness.
There is a long history of searching for the property that makes human language unique. Many properties once considered unique to humans (e.g., referential communication, syntax, cultural transmission, and intentionality) have subsequently been found in animal communication systems, at least in simple forms. I argue that recent claims that the cognitive foundations of human language are unique are not supported by the evidence cited, because the theories and methods used to study human language are not comparable to those used to study animal communication. These differences bias the conclusions we draw about the nature of the two systems.
Searching for Features Unique to Human Language
Human language was once thought to be a more formal, structured system, in contrast to the emotional, contextually bound communicative acts of animals. Even Darwin’s famous work comparing the expression of emotions in humans and other animals set “articulate language” to one side, considering it to be an altogether different type of system ( 4 ). These perceptions were influenced by a long tradition in Western philosophy privileging humanity’s capacity for rational thought, as well as by models of language that focused on our ability to convey meaning in predictable, decontextualized ways ( 5 ). These models were often developed by people studying written language and accordingly focused on the structural features and decontextualized, disembodied aspects of language.
For most of the 20th century, scholars argued that structural features of human language distinguished it from animal communication. The search for the structural “key” to language turned into an arms race between linguists and animal communication researchers. Every time a linguistic feature was proposed as the Rubicon separating human language and animal communication, animal researchers would look for evidence of that ability in their study species. Eventually, it would be found in one or more species (though in simpler or attenuated forms). In order to demonstrate the ability in nonhuman animals, researchers would often broaden its definition to make sense for nonlinguistic communication. This sometimes changed the definition considerably. Linguists would object. Animal researchers would defend their generalizations. This cycle was repeated many times.
Four of the primary candidates for features differentiating human language and animal communication—cultural transmission, reference, syntax, recursion—are reviewed below. Many other aspects of communication have been compared between animal and human systems (e.g., turn-taking, multimodality, intentionality), but these four illustrate how diverse aspects of language—learning, meaning, and structure—have been identified in animal systems. Note that finding a feature of human language in an animal system does not imply that language is not unique. Each feature has been found in one or more animal species, but never to the degree seen in human language. Language supercharges these features, and it is likely unique in its constellation thereof.
Cultural transmission was long assumed to be a defining feature of human language since language is acquired through convention and culture rather than biological inheritance. We now know that many other species, particularly birds, also acquire their vocalizations through learning from adult models. Some species even have a “critical period” in infancy, during which they learn from song models and rehearse their songs, and after which they cannot learn to produce these songs correctly ( 6 ). Evidence of vocal learning coupled with evidence of dialect differences across populations led scholars to conclude that there was cultural transmission of vocalizations in birds, whales, bats, and elephants ( 7 – 12 ) * .
Reference (using a signal to denote something in the external environment) was once argued to be unique to human language. Then, researchers demonstrated that the alarm calls of many types of animals differ systematically in response to different types of predators and elicit different escape behaviors in listeners ( 13 , 14 ). This predictable association with an external event seemed to meet the broad definition of reference; however, animal researchers began to use the term “functional reference,” since no one knew whether the animals intended to refer to the predators when producing alarm calls. Once animals appeared to demonstrate a capacity for (at least functional) reference, displaced reference became the new bar. Humans not only refer to things in their immediate environment but also refer to things displaced in space or time ( 5 , 15 ). In contrast, the animal alarm calls that demonstrated functional reference were always produced in the presence of a predator (the referent). Arguments for the uniqueness of displaced reference dovetailed with arguments that only humans were capable of mental time travel: the cognitive ability to remember the past or imagine the future from a first-person perspective ( 16 ). Experimental paradigms have since shown that great apes can point to things in their immediate environment to reference objects displaced in space and time ( 17 ). Other studies suggest that apes and other species are capable of mental time travel; they plan for future opportunities to retrieve food by selectively caching different types of food or storing tools where needed to retrieve future rewards ( 18 – 20 ).
Many scholars have argued that syntax is the defining feature of human language ( 21 – 24 ). Syntactic rules allow humans to communicate about an infinite number of things with a finite number of symbols. Having a set of rules that can be applied in predictable but open-ended ways to a set of symbols (e.g., words) enables humans to create sentences that they have never heard before. No other species has been found to use syntax as humans do, but evidence of syntax-like structural rules has been found in several animal communication systems. The songs produced by many bird species have “rules” that predict which sounds can be combined into motifs and which motifs can follow one another ( 25 , 26 ). The same is true for whales ( 27 ) and the call sequences of some primates ( 28 ). When perception of song sequences is tested by playing back recordings to birds, most species recognize when components are presented in orders that break the rules ( 29 – 31 ); there is little evidence, however, that rules about component order encode differences in meaning. Some argue that the “syntactic” patterns in bird and whale song are better described as phonological rules (or phonological syntax rather than lexical syntax), since they apply to sound combinations but not, as far as we can tell, to changes in meaning ( 25 ). Note that I use meaning throughout this paper to encompass both the rich, intended meanings of human language (and arguably ape gestures), and the information receivers extract from animal signals.
Animals can combine signals to amplify or modify one of the component parts ( 32 – 37 )—for example, by increasing intensity. However, there is little evidence that signal combinations produce meanings that differ from their parts. This type of combination (A + B = C) would suggest a syntax more reliant on the rule than on the identity of the components, one where the combination takes on an entirely new meaning. Such rules have been hinted at in the call sequences of a few monkey ( 38 – 40 ) and bird species ( 41 , 42 ), but not all scholars are convinced these systems require syntax to explain the observed patterns ( 43 ). Of course, animals might combine calls for reasons that have nothing to do with changing the meaning of the component parts. Song complexity has been linked to reproductive success across different taxa, suggesting that the ability to combine different sounds is itself attractive ( 44 , 45 ).
One particular type of syntactic rule—recursion—has been proposed as the feature that distinguishes both human language and human minds ( 46 ). Recursion is the ability to apply a syntactic rule to the output of that same syntactic rule, ad infinitum. This enables humans to create infinitely long utterances with multiple embeddings. For example, the utterance “I read the sentence that Cartmill wrote that illustrated the property that Chomsky described that Fitch argued made human language unique” exhibits several instances of recursion. This type of rule is complicated to employ and difficult to test, but one study found evidence that European starlings are capable of distinguishing patterns that rely on recursion ( 47 ). Others have argued that these results may have been based on numerical cognition rather than understanding of recursion ( 48 ), and so the search continues.
In recent years, the focus on structure as the defining aspect of language has relaxed. Indeed, the broad characterization of human language as structured and of animal communication as lacking structure has largely reversed. Human language is now often characterized by its flexibility, openness, and ability to deviate from its “code,” while animal systems are depicted as inflexible and reflexive, restricted to operating within set rules ( 3 , 49 , 50 ). This shift from structural features to cognitive building blocks aligns with a broader shift towards studying the minds rather than just the behavior of animals ( 51 ). However, shifting from the features of communicative acts to the often invisible motivational states and cognitive abilities behind them makes it more difficult to draw comparisons across species. As we shall see, the shift toward meaning and cognition also gives theoretical models of communication—and their methodological implications—an outsized role in shaping the empirical evidence about animal abilities.
Human Language Relies on Understanding Others’ Minds.
Human language is an exceptionally rich communicative system, even when it appears in the impoverished form studied by most linguists (written down and stripped of its nonverbal components and interactional information). Sociolinguists and linguistic anthropologists argue that human language is characterized not by the formal features seen in disembodied and decontextualized examples but by the rich social cognition and pragmatic inferences that underlie face-to-face communication. Pragmatics is the branch of linguistics that focuses on how language is used in everyday life and how meaning is conveyed in situated, interactional contexts rather than through only words and syntax. The heart of pragmatics is the idea that language meaning is inflected by its physical, social, and conversational environments.
The flexibility of human language means that each language user has the potential to modify, embellish, or expand the system, either in the long term (by inventing new words or structures) or in the short term (by using things outside the linguistic system in communicative ways). Long-term modifications allow languages to change over time. Short-term modifications allow speakers to adapt particular communicative acts to their goals, interlocutors, and environments. This might involve weaving together conventional symbols and structures with nonverbal elements like gestures, facial expressions, intonations, or prosodic emphasis. It may simply involve rich inferences about the goals or knowledge of one’s interlocutor. Take, for example, the following exchange between two friends at a party:
- Susan: Is George here?
- Lila: I saw an orange truck when I came.
To understand this exchange, you do not need to know that George drives an orange truck; you can extract the meaning from the response. This is done through inference, rather than a literal reading of the sentence. Many human conversations are intelligible only because (A) the speaker makes clear that their actions are communicative and (B) the listener makes a series of inferences about the relevance of the speaker’s actions to the ongoing conversation. The shared goals and coordinated actions of speaker and listener are encapsulated in the cooperative principle of philosopher Paul Grice ( 52 ). It is debated whether animal communication in general—and great ape gestures in particular—ever display Gricean principles of communication ( 53 – 57 ).
The cognitive abilities underlying our capacity to communicate in novel, nonliteral, and flexible ways are ostension and inference. Through ostension, a person draws attention to their actions and conveys that they are communicative (e.g., sliding their glass towards someone who is pouring wine). Through inference, others can perceive the communicative intent and respond accordingly (e.g., by filling the glass). The ostensive-inferential model of human language proposes that signalers are concerned with making their communicative intentions known (and may use a variety of behaviors to provide evidence of intention), and listeners are concerned with gathering this evidence and making inferences about the intended meaning ( 1 , 3 , 49 , 58 , 59 ). In line 2 of the example above, Lila answers Susan’s question by referring to an unusual type of car (not to George). Susan makes sense of this reply by assuming that Lila’s utterance was relevant to her question and infers that George is present. Such indirect reference seems complicated, but it is common in everyday language and demonstrates how easily humans employ ostensive-inferential abilities. Importantly, it relies heavily on understanding others’ minds and intentions: so-called theory of mind ( 2 ). There is evidence from cognitive studies that animals possess at least some aspects of theory of mind (e.g., understanding gaze), but the ways in which we typically study animal communication do not leave much room for questions about ostension and inference.
Socio-Cognitive Abilities in Animals.
Social animals have evolved sophisticated cognitive abilities that help them navigate their social worlds. These abilities include learning from others, tracking relationships across time, predicting others’ actions, making inferences about others’ goals, and responding to others’ behavior based on inferences about what they can or cannot see refs. ( 60 – 62 ).
Nonhuman primates are renowned for complex social cognition, which they use to navigate social hierarchies, form friendships, recruit allies, and avoid rivals ( 63 – 65 ). They understand not only their own relationships, but the nature of relationships between others ( 66 ). There is evidence that at least great apes understand what others can and can’t see ref. ( 60 ), as well as what others know and don’t know ( 67 , 68 ), suggesting that they possess some type of theory of mind. Adult humans can understand long recursive chains of theory of mind (e.g., I know that Matt knows that Jacob believes that Gal hopes that I want to see the movie tomorrow), but the building blocks of understanding other minds develop slowly over the first few years of life ( 69 , 70 ). Human toddlers and great apes both pass simple theory-of-mind tests; for example, by visually predicting where others will search for an item that the subject knows has been moved ( 65 , 67 , 71 , 72 ). Note, however, that young children (but not apes) have at least some knowledge of language, which may give them an advantage in propositional thinking about others’ beliefs ( 73 ).
Some argue that socio-cognitive abilities like theory of mind provide the foundation underlying human language but only enrich (rather than underlie) animal communication ( 1 , 3 , 49 , 50 ). This might seem like a semantic quibble, but the difference is significant. The claim is that human language is built on a fundamentally different type of cognition (one that requires ostension and inference), while all animal systems are exchanges of codes that do not require inferences about others (but may sometimes employ them). This model of language origins argues that language is not an animal-like system enriched with properties like syntax and complex inferences about others’ intentions. Instead, it claims that language is an entirely new type of system reliant on inferences about others’ minds at its most basic level. This claim is intriguing but it proposes that human language represents a discontinuity in the evolution of animal communication systems: Language involves sophisticated social inferences about signaler intentions and recipient knowledge purportedly lacking in animal systems. This, in turn, requires considerable evidence that rich social inferences about others’ minds are missing from nonhuman communicative acts. The difficulty is that data on human and animal communication have been heavily biased by differences in the way humans and animals are studied.
Animal Communication As Codes.
Animal communication research has been strongly shaped by the “code model” of communication, though it is rarely referenced directly ( Fig. 1 ). In this model, a message is encoded by the signaler, transmitted across a communicative channel (where it can distorted by “noise”), and then decoded by the receiver. The goal is for the decoded and encoded messages to be the same, with little information lost during transmission. This model grew out of Shannon’s information theory ( 74 ) and the conduit model of information transfer ( 75 ). The code model reflects its origins—the transmission of electronic signals in broadcasting and engineering—through the metaphors of encoding, decoding, transmission, noise, and signal interference.

A schematic illustrating the code model of communication.
The code model provides a useful recipe for breaking communication into phases, each a potential source of miscommunication. For example, a signaler could choose the wrong signal, the signal could be difficult to perceive due to interference (noise), or the receiver could misinterpret the signal and respond inappropriately. In describing potential sources of miscommunication, I have avoided code model terms (encoding, decoding, error, transmission) because they equate communication with robotic transfer. Herein lies the main problem with the code model: It dramatically restricts the ways we conceptualize (and study) animal communication.
The code model has had a pervasive effect on the design and interpretation of research on animal communication. Studies are predominantly built around searching for replicable contingencies between a signal produced by one individual and a subsequent change of behavior in another—in other words, codes ( 76 ). This search for code-like systems is mostly unavoidable; it is the primary way researchers demonstrate that animal signals have meaning (i.e., that they are communicative). If a signal does not reliably relate to a feature of the environment and/or evoke a particular response, then it’s hard to argue that it means anything—at least if your starting assumption is that animal signals are meaningless until proven otherwise.
This approach differs profoundly from how meaning is studied in language. Many human words, sentences, and gestures do not evoke an immediate reaction in others. Consider the paper you are currently reading. Unless reading this paper led to a predictable response in readers (e.g., angrily throwing it across the room or immediately designing a new study), standard methods in animal communication would conclude that the paper had no meaning. Methods for determining meaning in animal signals are not good at detecting communicative acts with delayed reactions, acts that impact internal states rather than external behavior, or acts that are embedded within ongoing interactions and are difficult to isolate.
Methodological Bias in the Search for Meaning.
To establish that animal signals have meaning, researchers focus on isolated signals that elicit predictable, immediate behavioral reactions. This makes sense; humans are not fluent in the systems they are studying, and animals cannot provide translations. However, this focus on predictable contingencies between signals and responses tightly constrains how researchers study meaning in animal systems and which behaviors they study in the first place.
The gold standard for determining that an animal signal has meaning is the “playback” design. There are many forms of meaning-making that draw on social, contextual, or environmental features outside of the signal, but the playback method looks only at the meaning transmitted to the receiver by the information encoded in the signal itself (directly borrowing metaphors from the code model). In a playback design, a signal (almost always auditory) is recorded and later played back to one or more animals to observe their reactions. It is critical to play the recorded signal back outside of its original context so that the environmental conditions that elicited the signal are not present; any responses will then be due to the information in the signal.
Playback designs are effective at measuring particular types of signals (alarms), in particular modalities (auditory), with particular responses (immediate behaviors like fleeing). With careful experimental manipulation, the playback method allows researchers to test their attributions of signal meaning. This is a major advantage. Humans bias our ascription of meaning to animal signals by the ways we parse and label our worlds. Alarm calls are typically thought to reference the type of predator that elicits them (e.g., leopard, eagle), but animals might perceive and categorize the world differently. For example, domestic chickens were thought to have separate alarm calls for foxes and eagles. When chickens heard an eagle alarm call, they hunched down. When they heard a fox alarm call, they flew up to perches. However, when chickens were shown video of foxes on screens overhead, they gave “eagle” calls. And when they saw videos of eagles on the ground, they gave “fox” calls ( 77 ). This simple manipulation revealed that chickens do not have alarm calls referencing the type of animal [something humans, including infants, would consider a “natural kind” ( 78 , 79 )]; instead, they provide information about the location of the impending attack.
Studies of dynamic visual signals (like gestures) also look for contingencies between signals and responses, though it is usually not possible to play back visual signals. In animal gesture studies, researchers typically take all cases of a particular gesture form, filter for those with markers of intentional use, categorize and count receivers’ responses, and assign meaning to gesture types based on apparently satisfactory outcomes—situations where the gesturer ceases to signal rather than persisting ( 80 – 82 ). After finding that signalers do not continue gesturing following Response X to Gesture A—but do persist following other responses to Gesture A—researchers conclude that Gesture A likely means “do X”. Such analyses of meaning focus on the signaler’s goal (what response from the receiver satisfies the signaler). This differs from playback studies, where analyses of meaning focus on how receivers perceive, interpret, and respond to the signal. This perspective shift is important because the goals of communication differ significantly between signalers and receivers ( 83 ). Analyses of gesture meaning and auditory playback designs are both successful at studying signals used in isolation with clear, immediate, behavioral responses, but this represents only a subset of all potentially communicative behaviors. What happens to other behaviors?
Animal signals not reliably associated with particular responses are often dismissed as “ambiguous” and excluded from analyses of meaning (or structure). In this way, studies present a highly curated subset of all potentially communicative behavior. This curation allows researchers to focus their efforts on frequent actions with immediate, predictable responses. Focusing on a subset of behavior has the added benefit of increasing the sample size for analyzed signals and standardizing methods across studies.
The use of playback to study alarm calls has become the paradigm for rigorously demonstrating that animal signals have meaning. Indeed, most studies of meaning in animal communication use variations of the playback design and draw directly on metaphors from the code model, like signaler and receiver. However, this limits the kinds of questions researchers can ask and the conclusions they can draw.
The Code Model Constrains Even Studies of Flexible Animal Systems.
Not all kinds of communication can be studied via playback, but methodological practices stemming from the code model skew conclusions about these systems as well. To illustrate this, I draw on examples from ape gesture. I do this for two reasons: First, because I have worked in this field for almost 20 y, so any criticisms I make necessarily include my own work; second, because ape gesture is arguably the least likely animal system to fall prey to the constraints of the code model. From the start, ape gesture has been recognized as both intentional and flexible—properties that make it more similar to human communication and significantly less code-like than other animal systems ( 84 – 89 ). This focus on intentionality and flexibility helped to distinguish ape gestures from vocalizations, which were argued to be largely inflexible and contextually bound ( 90 , 91 ). Ironically, it was only once ape gestures had been shown to be used flexibly that researchers began to systematically investigate gesture meaning by looking for tighter relationships between gestures and responses (i.e., codes). By highlighting the ways that the code model permeates study designs of even such extremely flexible and intentional animal systems as ape gesture, I hope to show how pervasive the search for codes is in studies of animal communication.
To study gesture, researchers start with video of ape groups. They cut these videos down to focus on nonfunctional movements produced in social situations, excluding movements produced when apes are alone and instrumental movements that achieve a physical goal (like picking up an infant). The remaining movements are categorized by their physical forms and contextual uses. They are often named by their similarity to human gestures (e.g., beckon, shoo, wave). Researchers may exclude gestures that do not meet criteria for intentionality, like waiting for a response or elaborating when there is no response ( 92 ). It is also common to exclude gestures that occur during play or multiparty interactions, since these are not likely to occur the same way multiple times. Most studies also exclude “rare” gestures observed only infrequently. Sample size is particularly important for analyses of meaning or function, where many examples of the same gesture are needed. In studies with smaller sample sizes, rarely observed gestures may be a sizable portion of the data. For example, Cartmill & Byrne ( 80 ) described 64 gestures in orangutans but included only 40 in analyses of meaning. Genty et al. ( 93 ) reported 102 gestures in gorillas but analyzed function for only the 10 most common. Finally, analyses of gesture typically focus on gestures that either occur alone or at the start of sequences, i.e., those that initiate interactions. This increases the likelihood that a gesture can be shown to elicit a particular response, critical for demonstrating that gestures have (code-like) meanings.
These curation and analysis practices have led to important discoveries about ape gesture. Nevertheless, it is important to recognize what these practices leave out and to recognize the ways in which they too have been shaped by assumptions of the code model. Table 1 presents examples of findings and methods from a range of ape gesture studies. This table is by no means exhaustive; it is meant to demonstrate how pervasive the search for codes is, even for a type of communication characterized by flexibility and intentionality. Some current studies of ape gesture attempt to move beyond searching for code-like contingencies by focusing on the pragmatics of interactions or socio-ecological contexts of communication ( 94 – 99 ); I discuss these below. Despite recent work to widen our lens, the study of ape gesture continues to be blinkered by the code model, in both methods and research questions.
Comparison of methods and conclusions from published studies of ape gesture meaning (emphasis mine)
Study | Species | Conclusions | Methods |
---|---|---|---|
Hobaiter & Byrne ( ) | Chimpanzee ( ) | “…found [gestures] to be used intentionally to achieve 15 purposes...” | “We examined whether different gestures were associated with a specific pattern of outcomes..” “… we therefore excluded data from play bouts to avoid masking the “real-world” meaning of gestures.” |
Roberts et al. ( ) | Chimpanzee ( ) | “...gestures were also strongly associated with specific responses and outcomes…” | “…consistent association between a given gesture type and particular behavioural change may be used to infer the meaning of different gestures” “…this necessarily excluded both actions that were not clearly directed…towards a specific recipient that could visually perceive the signaller's behaviour…and actions that could feasibly be readily explained in noncommunicative terms” |
Cartmill & Byrne ( ) | Orangutan ( ) | “…more than half of the orangutan gestures we were able to analyse had predictable intentional meanings” | “The aim is to identify gestures that are used predictably to elicit specific reactions...” “Only gestures that occurred singly or as the first gesture in a sequence were analysed for meaning. While this simplified the analysis by restricting it to a single signal and reaction in each case, it necessarily excluded some gestures from analysis.” |
Graham et al. ( ) | Bonobo ( ) | “Bonobos intentionally deploy gestures to achieve at least 14 different intended outcomes” | “We are able to deduce the meaning of great ape gestures by looking at the ‘Apparently Satisfactory Outcome’ (ASO)…” “…Fifteen gesture types were suitable for analysis, having been used by at least 3 individuals at least 3 times to achieve an ASO.” |
These studies represent a range of authors and study species and all employ methods frequently used in the field. Each study found that gestures were used to communicate specific meanings. Each also explained in the methods that the researchers were explicitly looking for code-like contingencies between signal and response and curated their data in particular ways that made these codes easier to find.
Meaning beyond Codes.
Studies of animal communication (like those in Table 1 ) typically find that animals communicate using simple codes that elicit particular responses. However, this is unsurprising: These studies are looking for codes, often discarding communications that do not fit the code model.
Human language, in contrast, is already assumed to be both communicative and meaningful. This assumption frees up the ways it is studied. Flexibility in the way words and gestures are used is assumed to reveal properties of thought or interaction, rather than evidence that the observed behaviors are not communicative. A word doesn’t need to show a predictable response in order to be considered meaningful.
Humans communicate by integrating and interweaving culturally transmitted codes (spoken or signed language) with nonverbal conventions (e.g., gestures, prosody), nonverbal nonconventions (e.g., interacting with the physical environment), and assumptions about the knowledge and goals of their interlocutor. This integration occurs for both signaler and receiver, with information exchanged in both directions. Receivers provide feedback when they encounter difficulties in understanding, and signalers revise their communicative acts in real time based on this feedback ( 100 , 101 ). Studies of human gesturing demonstrate that gesture is tightly integrated with speech, both semantically and temporally ( 102 – 104 ). When one modality is disrupted (as in the case of stuttering), the other is as well ( 105 , 106 ). Receivers also integrate information across gesture and speech when interpreting language ( 107 , 108 ). Evidence from studies of gesture production and gesture perception supports a model of human language as a single, integrated, multimodal system—rather than two systems produced at the same time ( 109 – 112 ). We have only begun to understand the full range of behavior and information humans use when communicating face-to-face. Fig. 2 gives an overview of some of the many possible sources of meaning humans draw on.

Schematic illustration of some of the sources of meaning humans integrate when communicating. Sources with code-like features (e.g., words) form only a small part of the semiotic landscape in natural conversation.
Fig. 2 is not a comprehensive model of human communication ( 113 ); it merely highlights the many ways humans convey meaning to one another. Note, however, how complex this simplified model of human communication is when compared to the code model used to study animal communication. Humans might ostensively signal that they are communicating by drawing on a wide range of sources: linguistic conventions, nonverbal conventions, or nonverbal nonconventions. For example, imagine that the people in Fig. 2 are at an event when the music switches to an obscure song. The signaler might smile and hold one finger up to draw attention to the song (nonverbal conventions), say “I remember digging through records at an estate sale and finding this waaay in the back,” and mime flipping through record albums (a nonverbal nonconvention). The receiver integrates information across the speaker’s words, actions, tone, expression—and the song itself—with inferences about the speaker’s intentions to interpret the communicative act.
It is unlikely that such a complex personal narrative would be found in a nonhuman animal. However, it seems possible (and even likely) that at least some animals use some of these ostensive and inferential abilities at least some of the time. This is especially true for great apes, who flexibly modify their gestures in response to the visual attention ( 114 ) and understanding of others ( 115 ), and who can (at least sometimes) make inferences based on others’ knowledge ( 67 , 68 ) and expectations ( 116 ). However, the code model and the methods commonly used to study animals leave little room for discovering complex or infrequent interactions that involve integrating information across different sources. Recognizing differences in our implicit models of human and animal communication—and the ways in which these models bias our studies—is a necessary step toward credible comparisons between language and animal communication.
A Path Forward?
It is impossible to eliminate every difference in the way communication is studied in animals and humans, but it is critical to acknowledge and account for theoretical and methodological differences when comparing abilities between species. To draw accurate comparisons between the communication systems and underlying cognitive abilities of humans and animals, animal communication studies must expand beyond searching for code-like signals with predictable behavioral responses.
Scholars studying great ape communication have recently begun to break away from the code model by introducing a range of new methods, mainly adapted from studies of human interaction. Some have introduced analyses of pragmatics, focusing on the ways context can inflect meaning ( 94 , 117 ); others allow for greater signal ambiguity, so that each signal does not need to have a single typical response to be included in analyses ( 82 ). Some have begun to apply analytic frames from the field of conversation analysis to primate gesture, shifting the focus from the signaling individual to the interacting dyad ( 95 – 97 ). Others have argued that ape gesture researchers need to be bolder in their research questions and conclusions, urging scholars to more fully explore the types of communicative inferences apes can make rather than stopping at demonstrations of intentional signaling ( 118 ). Still others have begun developing computer-vision and machine-learning methods to circumvent human biases in the categorization of gesture forms or meanings ( 119 ). Finally, a few scholars have begun to use analytical frameworks from animal communication to study human interaction [e.g., to study gestures of human infants ( 120 )]. These emerging lines of work have found much greater continuity between animal and human communication than traditional studies. However, most studies of animal communication remain constrained in both imagination and design by the limitations of the code model.
Current work on ape gesture provides some examples for how animal communication research might expand beyond the code model. This echoes an earlier shift in the field—also led by work on ape gesture—toward considering the intentionality of primate signals (e.g., refs. 121 , 122 ). However, many of the more flexible approaches to ape gesture draw directly on methods from studies of human interaction (e.g., conversation analysis and pragmatics). Some of these methods would take significant work to apply to species more distantly related to humans; modifying them to fit different social structures, body plans, perceptual systems, and environments demands added care to avoid stretching definitions and categories past the point of useful comparison. Methods adapted from conversation analysis seem to be among the most directly applicable across a wide range of species ( 96 , 123 – 125 ). This is probably because conversation analysis began as a framework to study the underlying rules of human interaction from patterns of external behavior, much as is done with animals ( 126 ). Analyses of several aspects of interaction, including turn-taking and communicative repair, have been usefully applied to both animal and human data with only minor modifications ( 96 , 127 ). The focus of conversation analysis on externally observable behavior helps it transfer between species, but its backgrounding of cognitive processes limits its utility in demonstrating abilities like ostension and inference ( 128 ). A wider sea change is needed in the ways researchers approach the communication and minds of animals. Here are two possibilities:
- Inclusion-by-association: One small but potentially impactful change would be for scholars to grant animals the same generous assumption that they do preverbal human children: that ambiguous behaviors directed toward others in the context of known communicative acts are probably also communicative. This assumption would greatly expand the types of exchanges that are included in studies of animal communication. Inclusion-by-association is common practice in interpretation of preverbal infant behavior, but it is not applied to animals. Instead, animal research typically engages in exclusion-by-association. For example, if an animal behavior occurs alongside signals or behaviors typically found in the context of play, it will often be glossed as play and excluded from further analyses of signal meaning ( 81 , 129 ). Taking a page from studies of human language development, I propose inclusion-by-association rather than exclusion-by-association.
- Communicative play: Researchers could also ask whether animals engage in communicative play. Animal play can be viewed as an opportunity for rich inference and potentially for pretense, as individuals signal to others that their actions should not be taken literally ( 130 – 132 ). Some types of play even display game-like qualities involving role reversal and hierarchical rules ( 116 , 133 ). Despite considerable interest in animal play, communicative play is not often studied. Researchers could start with known signals and ask how animals use those signals in nonstandard ways. They could also conduct qualitative analyses of rare interactions. For example, a researcher might observe animals sometimes producing signals in nonsocial contexts; pairing signals with actions that are not obviously communicative; or using signals in nontypical ways during social play. These nonstandard uses would likely be missed or excluded by current protocols for studying animal communication, but they are similar to behaviors in human children that are known to be important in communicative development. One type of communicative play—babbling—has been documented in animal species that learn to produce their vocalizations. Many species of birds, some species of bats and marine mammals, and at least one species of marmosets go through periods of vocal rehearsal early in development characterized by playful exploration of the acoustic space ( 134 – 139 ). This suggests that there may be other types of communicative play to be found outside of typical signaler-receiver information exchanges.
Opening up studies of animal communication to “messy” signaling would not require that researchers abandon the strict definitions and protocols that ensure interobserver reliability and replicability. Nor would it involve an “anything goes” approach to building a dataset. However, by systematically exploring instances where signals are used in ways that do not fit the expectations and assumptions of the code model, researchers may discover that animals can use a range of semiotic resources in indirect or novel ways.
Studies showing code-like contingencies between signals and responses have been crucial for demonstrating that animal signals have meanings and are not purely reflexes. The proposed methodological shifts toward considering animal communication outside of simple signal-response pairs would not invalidate or discount the contributions of studies following the code model. New, more flexible methods build on findings from code-model analyses, using known codes to anchor or contextualize ambiguous or rare behaviors. By acknowledging historic differences in the ways scholars study animals and humans—and incorporating new approaches that break away from primarily searching for codes—we may find that animal communication and human language are largely characterized by differences of degree rather than kind.
Acknowledgments
I am grateful to Jacob Foster for his encouragement and comments on the manuscript. I am indebted to Matt Cartmill and Kaye Brown for challenging me to think more deeply about these topics. I thank Thom Scott-Phillips, Heidi Lyn, Richard Moore, Lila Gleitman, and interlocutors from EVOLANG for spirited debates about the code model.
Author contributions
E.A.C. wrote the paper.
Competing interests
The author declares no competing interest.
This article is a PNAS Direct Submission.
* Cultural transmission does not play a substantial role in the acquisition of either vocal or gestural communication in non-human primates ( 140 – 145 ). Primates, like many other species, do develop cultural traditions in other domains ( 146 ).
Data, Materials, and Software Availability
This page has been archived and is no longer updated
An Introduction to Animal Communication

The ability to communicate effectively with other individuals plays a critical role in the lives of all animals. Whether we are examining how moths attract a mate, ground squirrels convey information about nearby predators, or chimpanzees maintain positions in a dominance hierarchy, communication systems are involved. Here, I provide a primer about the types of communication signals used by animals and the variety of functions they serve. Animal communication is classically defined as occurring when “...the action of or cue given by one organism [the sender] is perceived by and thus alters the probability pattern of behavior in another organism [the receiver] in a fashion adaptive to either one both of the participants” (Wilson 1975). While both a sender and receiver must be involved for communication to occur (Figure 1), in some cases only one player benefits from the interaction. For example, female Photuris fireflies manipulate smaller, male Photinus fireflies by mimicking the flash signals produced by Photinus females. When males investigate the signal, they are voraciously consumed by the larger firefly (Lloyd 1975; Figure 2). This is clearly a case where the sender benefits and the receiver does not. Alternatively, in the case of fringe-lipped bats, Trachops cirrhosus , and tungara frogs, Physalaemus pustulosus , the receiver is the only player that benefits from the interaction. Male tungara frogs produce advertisement calls to attract females to their location; while the signal is designed to be received by females, eavesdropping fringe-lipped bats also detect the calls, and use that information to locate and capture frogs (Ryan et al . 1982). Despite these examples, there are many cases in which both the sender and receiver benefit from exchanging information. Greater sage grouse nicely illustrate such “true communication”; during the mating season, males produce strutting displays that are energetically expensive, and females use this honest information about male quality to choose which individuals to mate with (Vehrencamp et al . 1989).
Figure 1 A model of animal communication.
Figure 2: Photinus fireflies. Courtesy of Tom Eisner.
Signal Modalities
Animals use a variety of sensory channels, or signal modalities, for communication. Visual signals are very effective for animals that are active during the day. Some visual signals are permanent advertisements; for example, the bright red epaulets of male red-winged blackbirds, Agelaius phoeniceus, which are always displayed, are important for territory defense. When researchers experimentally blackened epaulets, males were subject to much higher rates of intrusion by other males (Smith 1972). Alternatively, some visual signals are actively produced by an individual only under appropriate conditions. Male green anoles, Anolis carolinensis, bob their head and extend a brightly colored throat fan (dewlap) when signaling territory ownership. Acoustic communication is also exceedingly abundant in nature, likely because sound can be adapted to a wide variety of environmental conditions and behavioral situations. Sounds can vary substantially in amplitude, duration, and frequency structure, all of which impact how far the sound will travel in the environment and how easily the receiver can localize the position of the sender. For example, many passerine birds emit pure-tone alarm calls that make localization difficult, while the same species produce more complex, broadband mate attraction songs that allow conspecifics to easily find the sender (Marler 1955). A particularly specialized form of acoustic communication is seen in microchiropteran bats and cetaceans that use high-frequency sounds to detect and localize prey. After sound emission, the returning echo is detected and processed, ultimately allowing the animal to build a picture of their surrounding environment and make very accurate assessments of prey location. Compared to visual and acoustic modalities, chemical signals travel much more slowly through the environment since they must diffuse from the point source of production. Yet, these signals can be transmitted over long distances and fade slowly once produced. In many moth species, females produce chemical cues and males follow the trail to the female’s location. Researchers attempted to tease apart the role of visual and chemical signaling in silkmoths, Bombyx mori , by giving males the choice between a female in a transparent airtight box and a piece of filter paper soaked in chemicals produced by a sexually receptive female. Invariably, males were drawn to the source of the chemical signal and did not respond to the sight of the isolated female (Schneider 1974; Figure 3). Chemical communication also plays a critical role in the lives of other animals, some of which have a specialized vomeronasal organ that is used exclusively to detect chemical cues. For example, male Asian elephants, Elaphus maximus , use the vomeronasal organ to process chemical cues in female’s urine and detect if she is sexually receptive (Rasmussen et al . 1982).
Figure 3 Male silkmoths are more strongly attracted to the pheromones produced by females (chemical signal) than the sight of a female in an airtight box (visual signal). Tactile signals, in which physical contact occurs between the sender and the receiver, can only be transmitted over very short distances. Tactile communication is often very important in building and maintaining relationship among social animals. For example, chimpanzees that regularly groom other individuals are rewarded with greater levels of cooperation and food sharing (de Waal 1989). For aquatic animals living in murky waters, electrical signaling is an ideal mode of communication. Several species of mormyrid fish produce species-specific electrical pulses, which are primarily used for locating prey via electrolocation, but also allow individuals searching for mates to distinguish conspecifics from heterospecifics. Foraging sharks have the ability to detect electrical signals using specialized electroreceptor cells in the head region, which are used for eavesdropping on the weak bioelectric fields of prey (von der Emde 1998).
Signal Functions
Some of the most extravagant communication signals play important roles in sexual advertisement and mate attraction. Successful reproduction requires identifying a mate of the appropriate species and sex, as well as assessing indicators of mate quality. Male satin bowerbirds, Ptilonorhynchus violaceus , use visual signals to attract females by building elaborate bowers decorated with brightly colored objects. When a female approaches the bower, the male produces an elaborate dance, which may or may not end with the female allowing the male to copulate with her (Borgia 1985). Males that do not produce such visual signals have little chance of securing a mate. While females are generally the choosy sex due to greater reproductive investment, there are species in which sexual roles are reversed and females produce signals to attract males. For example, in the deep-snouted pipefish, Syngnathus typhle , females that produce a temporary striped pattern during the mating period are more attractive to males than unornamented females (Berglund et al . 1997). Communication signals also play an important role in conflict resolution, including territory defense. When males are competing for access to females, the costs of engaging in physical combat can be very high; hence natural selection has favored the evolution of communication systems that allow males to honestly assess the fighting ability of their opponents without engaging in combat. Red deer, Cervus elaphus , exhibit such a complex signaling system. During the mating season, males strongly defend a group of females, yet fighting among males is relatively uncommon. Instead, males exchange signals indicative of fighting ability, including roaring and parallel walks. An altercation between two males most often escalates to a physical fight when individuals are closely matched in size, and the exchange of visual and acoustic signals is insufficient for determining which animal is most likely to win a fight (Clutton-Brock et al . 1979). Communication signals are often critical for allowing animals to relocate and accurately identify their own young. In species that produce altricial young, adults regularly leave their offspring at refugia, such as a nest, to forage and gather resources. Upon returning, adults must identify their own offspring, which can be especially difficult in highly colonial species. Brazilian free-tailed bats, Tadarida brasiliensis , form cave colonies containing millions of bats; when females leave the cave each night to forage, they place their pup in a crèche that contains thousands of other young. When females return to the roost, they face the challenge of locating their own pups among thousands of others. Researchers originally thought that such a discriminatory task was impossible, and that females simply fed any pups that approached them, yet further work revealed that females find and nurse their own pup 83% of the time (McCracken 1984, Balcombe 1990). Females are able to make such fantastic discriminations using a combination of spatial memory, acoustic signaling, and chemical signaling. Specifically, pups produce individually-distinct “isolation calls”, which the mother can recognize and detect from a moderate distance. Upon closer inspection of a pup, females use scent to further confirm the pup’s identity. Many animals rely heavily on communication systems to convey information about the environment to conspecifics, especially close relatives. A fantastic illustration comes from vervet monkeys, Chlorocebus pygerythrus , in which adults give alarm calls to warn colony members about the presence of a specific type of predator. This is especially valuable as it conveys the information needed to take appropriate actions given the characteristics of the predator (Figure 4). For example, emitting a “cough” call indicates the presence of an aerial predator, such as an eagle; colony members respond by seeking cover amongst vegetation on the ground (Seyfarth & Cheney 1980). Such an evasive reaction would not be appropriate if a terrestrial predator, such as a leopard, were approaching.
Figure 4 Vervet monkeys. Many animals have sophisticated communication signals for facilitating integration of individuals into a group and maintaining group cohesion. In group-living species that form dominance hierarchies, communication is critical for maintaining ameliorative relationships between dominants and subordinates. In chimpanzees, lower-ranking individuals produce submissive displays toward higher-ranking individuals, such as crouching and emitting “pant-grunt” vocalizations. In turn, dominants produce reconciliatory signals that are indicative of low aggression. Communication systems also are important for coordinating group movements. Contact calls, which inform individuals about the location of groupmates that are not in visual range, are used by a wide variety of birds and mammals. Overall, studying communication not only gives us insight into the inner worlds of animals, but also allows us to better answer important evolutionary questions. As an example, when two isolated populations exhibit divergence over time in the structure of signals use to attract mates, reproductive isolation can occur. This means that even if the populations converge again in the future, the distinct differences in critical communication signals may cause individuals to only select mates from their own population. For example, three species of lacewings that are closely related and look identical are actually reproductively isolated due to differences in the low-frequency songs produced by males; females respond much more readily to songs from their own species compared to songs from other species (Martinez, Wells & Henry 1992). A thorough understanding of animal communication systems can also be critical for making effective decisions about conservation of threatened and endangered species. As an example, recent research has focused on understanding how human-generated noise (from cars, trains, etc) can impact communication in a variety of animals (Rabin et al . 2003). As the field of animal communication continues to expand, we will learn more about information exchange in a wide variety of species and better understand the fantastic variety of signals we see animals produce in nature.
Vomeronasal organ – auxiliary olfactory organ that detects chemosensory cues
Altricial – the state of being born in an immature state and relying exclusively on parental care for survival during early development
Refugia – areas that provide concealment from predators and/or protection from harsh environmental conditions
Conspecifics – organisms of the same species
References and Recommended Reading
Balcombe, J.P. Vocal recognition of pups by mother Mexican free-tailed bats, Tadarida brasiliensis mexicana . Animal Behaviour 39 , 960-966 (1990). Berglund, J., Rosenqvist G. and Bernet P. Ornamentation predicts reproductive success in female pipefish. Behavioral Ecology and Sociobiology 40 , 145-150 (1997). Clutton-Brock, T., Albon S., Gibson S. & Guinness F. The logical stag: Adaptive aspects of fighing in the red deer. Animal Behaviour 27 , 211-225 (1979). de Waal F.B.M. Food sharing and reciprocal obligations among chimpanzees. Journal of Human Evolution 18 , 433–459 (1989).
Hauser, M. 1997. The Evolution of Communication . Cambridge, MA: MIT Press. Lloyd, J.E. Aggressive mimicry in Photuris: signal repertoires by femmes fatales. Science 197 , 452-453 (1975).
Marler, P. Characteristics of some animal calls. Nature 176 , 6-8 (1955). Martinez Well, M. & Henry C.S. The role of courtship songs in reproductive isolation among populations of green lacewings of the genus Chrysoperla . Evolution 46 , 31-43 (1992). McCracken, G.F. Communal nursing in Mexican free-tailed bat maternity colonies. Science 223 , 1090-1091(1984).
Rabin, L.A., McCowan B., Hooper S.L & Owings D.H. Anthropogenic noise and its effect on animal communication: an interface between comparative psychology and conservation biology. International Journal of Comparative Psychology 16 , 172-192 (2003). Ryan M.J., Tuttle M.D., & Rand A.S. Sexual advertisement and bat predation in a neotropical frog. American Naturalist 119 , 136–139 (1982). Schneider, D. The sex attractant receptors of moths. Scientific American 231 , 28-35 (1974). Seyfarth, R.M., Cheney D.L. & Marler P. Monkey responses to three different alarm calls: Evidence for predator classification and semantic communication. Science 210 , 801-803 (1980). Smith, D. The role of the epaulets in the red-winged blackbird, ( Agelaius phoeniceus ) social system. Behaviour 41 , 251-268 (1972).
Vehrencamp, S.L., Bradbury J.W., & Gibson R.M. The energetic cost of display in male sage grouse. Animal Behaviour 38 , 885-896 (1989). von der Emde, G. Electroreception. In D. H. Evans (ed.). The Physiology of Fishes , pp. 313-343. Boca Raton, FL: CRC Press (1998). Wilson, E.O. Sociobiology: The New Synthesis . Cambridge, MA: Harvard University Press (1975).
Article History
Flag inappropriate.


Email your Friend
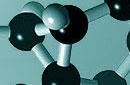
- | Lead Editor: Sara Tenney

Within this Subject (24)
- Basic (13)
- Intermediate (5)
- Advanced (6)
Other Topic Rooms
- Ecosystem Ecology
- Physiological Ecology
- Population Ecology
- Community Ecology
- Global and Regional Ecology
- Conservation and Restoration
- Animal Behavior
- Teach Ecology
- Earth's Climate: Past, Present, and Future
- Terrestrial Geosystems
- Marine Geosystems
- Scientific Underpinnings
- Paleontology and Primate Evolution
- Human Fossil Record
- The Living Primates

© 2014 Nature Education
- Press Room |
- Terms of Use |
- Privacy Notice |

Visual Browse
- A-Z Publications
Annual Review of Linguistics
Volume 9, 2023, review article, open access, animal communication in linguistic and cognitive perspective.
- Thom Scott-Phillips 1 , and Christophe Heintz 2
- View Affiliations Hide Affiliations Affiliations: 1 Institute for Logic, Cognition, Language, and Information, San Sebastian, Spain; email: [email protected] 2 Department of Cognitive Science, Central European University, Budapest, Hungary
- Vol. 9:93-111 (Volume publication date January 2023) https://doi.org/10.1146/annurev-linguistics-030421-061233
- First published as a Review in Advance on September 28, 2022
- Copyright © 2023 by the author(s). This work is licensed under a Creative Commons Attribution 4.0 International License, which permits unrestricted use, distribution, and reproduction in any medium, provided the original author and source are credited. See credit lines of images or other third-party material in this article for license information
Detailed comparative studies have revealed many surface similarities between linguistic communication and the communication of nonhumans. How should we interpret these discoveries in linguistic and cognitive perspective? We review the literature with a specific focus on analogy (similar features and function but not shared ancestry) and homology (shared ancestry). We conclude that combinatorial features of animal communication are analogous but not homologous to natural language. Homologies are found instead in cognitive capacities of attention manipulation, which are enriched in humans, making possible many distinctive forms of communication, including language use. We therefore present a new, graded taxonomy of means of attention manipulation, including a new class we call Ladyginian, which is related to but slightly broader than the more familiar class of Gricean interaction. Only in the latter do actors have the goal of revealing specifically informative intentions. Great ape interaction may be best characterized as Ladyginian but not Gricean.
Article metrics loading...
Full text loading...
Literature Cited
- Ackermann H , Hage SR , Ziegler W. 2014 . Brain mechanisms of acoustic communication in humans and nonhuman primates: an evolutionary perspective. Behav. Brain Sci. 37 : 6 529– 46 [Google Scholar]
- Anderson SR. 2006 . Doctor Dolittle's Delusion: Animals and the Uniqueness of Human Language New Haven, CT: Yale Univ. Press [Google Scholar]
- Arnold K , Zuberbühler K. 2006 . Semantic combinations in primate calls. Nature 441 : 7091 303 [Google Scholar]
- Arnold K , Zuberbühler K. 2008 . Meaningful call combinations in a non-human primate. Curr. Biol. 18 : 5 R202– 3 [Google Scholar]
- Berwick RC. 2016 . Monkey business. Theor. Linguist. 42 : 1/2 91– 95 [Google Scholar]
- Berwick RC , Chomsky N. 2016 . Why Only Us? Language and Evolution Cambridge, MA: MIT Press [Google Scholar]
- Berwick RC , Okanoya K , Beckers GJL , Bolhuis JJ 2011 . Songs to syntax: the linguistics of birdsong. Trends Cogn. Sci. 15 : 3 113– 21 [Google Scholar]
- Bohn M , Call J , Tomasello M. 2015 . Communication about absent entities in great apes and human infants. Cognition 145 : 63– 72 [Google Scholar]
- Bohn M , Frank MC. 2019 . The pervasive role of pragmatics in early language. Annu. Rev. Dev. Psychol. 1 : 223– 49 [Google Scholar]
- Bolhuis JJ , Beckers GJ , Huybregts MA , Berwick RC , Everaert MB. 2018 . Meaningful syntactic structure in songbird vocalizations?. PLOS Biol . 16 : 6 ). e2005157 Presents a skeptical analysis on combinatorial communication in songbirds, from the perspective of generative grammar. [Google Scholar]
- Bolhuis JJ , Okanoya K , Scharff C. 2010 . Twitter evolution: converging mechanisms in birdsong and human speech. Nat. Rev. Neurosci. 11 : 11 747– 59 [Google Scholar]
- Bolhuis JJ , Tattersall I , Chomsky N , Berwick RC. 2014 . How could language have evolved?. PLOS Biol . 12 : 8 e1001934 [Google Scholar]
- Bryant GA 2020 . Evolution, structure, and functions of human laughter. Handbook of Communication Science and Biology K Floyd, R Weber 63– 77 London: Routledge [Google Scholar]
- Bryant GA , Aktipis CA. 2014 . The animal nature of spontaneous human laughter. Evol. Hum. Behav. 35 : 4 327– 35 [Google Scholar]
- Bugnyar T , Reber SA , Buckner C. 2016 . Ravens attribute visual access to unseen competitors. Nat. Commun. 7 : 10506 [Google Scholar]
- Burling R. 2005 . The Talking Ape: How Language Evolved Oxford, UK: Oxford Univ. Press [Google Scholar]
- Byrne RW , Cartmill E , Genty E , Graham KE , Hobaiter C , Tanner J. 2017 . Great ape gestures: intentional communication with a rich set of innate signals. Anim. Cogn. 20 : 4 755– 69 Provides an overview of the St Andrews approach to meaning in great ape gesture. [Google Scholar]
- Cartmill EA , Byrne RW. 2010 . Semantics of primate gestures: intentional meanings of orangutan gestures. Anim. Cogn. 13 : 6 793– 804 [Google Scholar]
- Cartmill EA , Hobaiter C. 2019 . Developmental perspectives on primate gesture: 100 years in the making. Anim. Cogn. 22 : 4 453– 59 [Google Scholar]
- Chomsky N. 2017 . The Galilean challenge. Inference 3 : 1 https://doi.org/10.37282/991819.17.1 [Crossref] [Google Scholar]
- Chomsky N. 2021 . Linguistics then and now: some personal reflections. Annu. Rev. Linguist. 7 : 1– 11 [Google Scholar]
- Clark B. 2013 . Relevance Theory Cambridge, UK: Cambridge Univ. Press [Google Scholar]
- Collier K , Bickel B , van Schaik CP , Manser MB , Townsend SW. 2014 . Language evolution: syntax before phonology?. Proc. R. Soc. B 281 : 1788 20140263 [Google Scholar]
- Cornforth D , Popat R , McNally L , Gurney J , Scott-Phillips T et al. 2014 . Combinatorial quorum-sensing communication allows bacteria to resolve physical and social uncertainty. PNAS 111 : 11 4280– 84 [Google Scholar]
- Davila Ross M , Owren MJ , Zimmermann E. 2009 . Reconstructing the evolution of laughter in great apes and humans. Curr. Biol. 19 : 13 1106– 11 [Google Scholar]
- de Boer B , Sandler W , Kirby S 2012 . New perspectives on duality of patterning: introduction to the special issue. Lang. Cogn. 4 : 4 251– 59 [Google Scholar]
- Dediu D , de Boer B. 2016 . Language evolution needs its own journal. J. Lang. Evol. 1 : 1 1– 6 [Google Scholar]
- Engesser S , Crane JM , Savage JL , Russell AF , Townsend SW. 2015 . Experimental evidence for phonemic contrasts in a nonhuman vocal system. PLOS Biol . 13 : 6 e1002171 [Google Scholar]
- Engesser S , Fitch WT. 2021 . Babbler phonology and combinatorial systems. Inference 6 : 2 https://doi.org/10.37282/991819.21.33 [Crossref] [Google Scholar]
- Engesser S , Holub JL , O'Neill LG , Russell AF , Townsend SW 2019 . Chestnut-crowned babbler calls are composed of meaningless shared building blocks. PNAS 116 : 39 19579– 84 Describes experiments showing songbird vocalizations with the features commonly known as duality of patterning. [Google Scholar]
- Engesser S , Townsend SW. 2019 . Combinatoriality in the vocal systems of nonhuman animals. Wiley Interdiscip. Rev. Cogn. Sci. 10 : 4 e1493 [Google Scholar]
- Fischer J 2017 . Playback experiments. The International Encyclopedia of Primatology A Fuentes New York: Wiley https://doi.org/10.1002/9781119179313.wbprim0140 [Crossref] [Google Scholar]
- Fitch WT. 2016 . Why formal semantics and primate communication make strange bedfellows. Theor. Linguist. 42 : 1/2 97– 109 [Google Scholar]
- Fitch WT. 2018 . The biology and evolution of speech: a comparative analysis. Annu. Rev. Linguist. 4 : 255– 79 [Google Scholar]
- Fitch WT , Friederici AD. 2012 . Artificial grammar learning meets formal language theory: an overview. Philos. Trans. R. Soc. B 367 : 1598 1933– 55 [Google Scholar]
- Genty E , Zuberbühler K. 2014 . Spatial reference in a bonobo gesture. Curr. Biol. 24 : 14 1601– 5 [Google Scholar]
- Gervais M , Wilson DS. 2005 . The evolution and functions of laughter and humor: a synthetic approach. Q. Rev. Biol. 80 : 4 395– 430 [Google Scholar]
- Ghazanfar AA , Rendall D. 2008 . Evolution of human vocal production. Curr. Biol. 18 : 11 R457– 60 [Google Scholar]
- Gibbs RW Jr. , Colston HL. 2012 . Interpreting Figurative Meaning Cambridge, UK: Cambridge Univ. Press [Google Scholar]
- Graham KE , Wilke C , Lahiff NJ , Slocombe KE. 2020 . Scratching beneath the surface: intentionality in great ape signal production. Philos. Trans. R. Soc. B 375 : 1789 20180403 [Google Scholar]
- Grice HP. 1957 . Meaning. Philos. Rev. 66 : 3 377– 88 [Google Scholar]
- Grice HP. 1989 . Studies in the Way of Words Cambridge, MA: Harvard Univ. Press [Google Scholar]
- Grosse G , Behne T , Carpenter M , Tomasello M. 2010 . Infants communicate in order to be understood. Dev. Psychol. 46 : 6 1710– 22 [Google Scholar]
- Gurney J , Azimi S , Brown SP , Diggle SP. 2020 . Combinatorial quorum sensing in Pseudomonas aeruginosa allows for novel cheating strategies. Microbiology 166 : 8 777– 84 [Google Scholar]
- Hagoort P. 2019 . The neurobiology of language beyond single-word processing. Science 366 : 6461 55– 58 [Google Scholar]
- Hauser MD , Yang C , Berwick RC , Tattersall I , Ryan MJ et al. 2014 . The mystery of language evolution. Front. Psychol. 5 : 401 [Google Scholar]
- Heesen R , Hobaiter C , Ferrer-i-Cancho R , Semple S. 2019 . Linguistic laws in chimpanzee gestural communication. Proc. R. Soc. B 286 : 1896 20182900 [Google Scholar]
- Heintz C , Karabegovic M , Molnar A. 2016 . The co-evolution of honesty and strategic vigilance. Front. Psychol. 7 : 1503 [Google Scholar]
- Heintz C , Scott-Phillips T. 2023 . Expression unleashed: the evolutionary and cognitive foundations of human communication. Behav. Brain Sci. In press Describes how and why humans, and only humans, evolved capacities necessary for open-ended communication. [Google Scholar]
- Hobaiter C , Byrne RW. 2014 . The meanings of chimpanzee gestures. Curr. Biol. 24 : 14 1596– 1600 [Google Scholar]
- Huybregts MA. 2020 . Babbling birds. Inference 5 : 3 https://doi.org/10.37282/991819.20.39 [Crossref] [Google Scholar]
- Hurford JR. 2007 . The Origins of Meaning: Language in the Light of Evolution , Vol. 1 Oxford, UK: Oxford Univ. Press [Google Scholar]
- Hurford JR. 2012 . The Origins of Grammar: Language in the Light of Evolution , Vol. 2 Oxford, UK: Oxford Univ. Press [Google Scholar]
- Kirby S. 2017 . Culture and biology in the origins of linguistic structure. Psychon. Bull. Rev. 24 : 1 118– 37 [Google Scholar]
- Jäger G 2016 . Grice, Occam, Darwin. Theor. Linguist. 42 : 1/2 111– 15 [Google Scholar]
- Ladygina-Kohts NN , de Waal FBM. 2002 . Infant Chimpanzee and Human Child: A Classic 1935 Comparative Study of Ape Emotions and Intelligence Oxford, UK: Oxford Univ. Press [Google Scholar]
- Leaver LA , Hopewell L , Caldwell C , Mallarky L. 2007 . Audience effects on food caching in grey squirrels ( Sciurus carolinensis ): evidence for pilferage avoidance strategies. Anim. Cogn. 10 : 1 23– 27 [Google Scholar]
- Leroux M , Townsend SW. 2020 . Call combinations in great apes and the evolution of syntax. Anim. Behav. Cogn. 7 : 2 131– 39 [Google Scholar]
- Levinson SC 2006 . On the human ‘interactional engine. ’. In Roots of Human Sociality: Culture, Cognition and Interaction , ed. NJ Enfield, SC Levinson 39– 69 Oxford, UK: Berg [Google Scholar]
- Lewis D. 1969 . Convention Cambridge, MA: Harvard Univ. Press [Google Scholar]
- Lyn H , Russell JL , Leavens DA , Bard KA , Boysen ST et al. 2014 . Apes communicate about absent and displaced objects: Methodology matters. Anim. Cogn. 17 : 1 85– 94 [Google Scholar]
- Maynard Smith J , Harper D 2003 . Animal Signals Oxford, UK: Oxford Univ. Press [Google Scholar]
- Moore R. 2016 . Meaning and ostension in great ape gestural communication. Anim. Cogn. 19 : 1 223– 31 [Google Scholar]
- Moore R. 2017 . Convergent minds: ostension, inference and Grice's third clause. Interface Focus 7 : 20160107 [Google Scholar]
- Munz T. 2005 . The bee battles: Karl von Frisch, Adrian Wenner and the honey bee dance language controversy. J. Hist. Biol. 38 : 3 535– 70 [Google Scholar]
- Nowak MA , Plotkin JB , Jansen VAA. 2000 . The evolution of syntactic communication. Nature 404 : 6777 495– 98 [Google Scholar]
- Ouattara K , Lemasson A , Zuberbühler K. 2009 . Campbell's monkeys use affixation to alter call meaning. PLOS ONE 4 : 11 e7808 [Google Scholar]
- Paunov AM , Blank IA , Fedorenko E. 2019 . Functionally distinct language and Theory of Mind networks are synchronized at rest and during language comprehension. J. Neurophysiol. 121 : 4 1244– 65 [Google Scholar]
- Pepperberg IM. 2017 . Animal language studies: What happened?. Psychon. Bull. Rev. 24 : 1 181– 85 [Google Scholar]
- Piantadosi ST , Fedorenko E. 2017 . Infinitely productive language can arise from chance under communicative pressure. J. Lang. Evol. 2 : 2 141– 47 [Google Scholar]
- Pika S , Liebal K , Call J , Tomasello M. 2005 . Gestural communication of apes. Gesture 5 : 1/2 41– 56 [Google Scholar]
- Provine RR. 2000 . Laughter: A Scientific Investigation New York: Penguin [Google Scholar]
- Radick G. 2007 . The Simian Tongue: The Long Debate About Animal Language Berkeley: Univ. Calif. Press Presents a fascinating history of playback experiments. [Google Scholar]
- Rendall D. 2021 . Aping language: historical perspectives on the quest for semantics, syntax, and other rarefied properties of human language in the communication of primates and other animals. Front. Psychol. 12 : 675172 [Google Scholar]
- Rizzi L. 2016 . Monkey morpho-syntax and Merge-based systems. Theor. Linguist. 42 : 1/2 139– 45 [Google Scholar]
- Sandler W. 2012 . The phonological organization of sign languages. Lang. Linguist. Compass 6 : 3 162– 82 [Google Scholar]
- Scarantino A , Clay Z. 2015 . Contextually variable signals can be functionally referential. Anim. Behav. 100 : e1– 8 [Google Scholar]
- Schlenker P , Chemla E , Schel AM , Fuller J , Gautier JP et al. 2016 . Formal monkey linguistics.. Theor. Linguist. 42 : 1/2 1– 90 Provides an overview of a research program applying tools of formal semantics to nonhuman primate communication. [Google Scholar]
- Scott-Phillips T. 2015a . Meaning in animal and human communication. Anim. Cogn. 18 : 3 801– 5 [Google Scholar]
- Scott-Phillips T 2015b . Speaking Our Minds: Why Human Communication Is Different and How Language Evolved to Make It Special London: Palgrave Macmillan [Google Scholar]
- Scott-Phillips T. 2016 . Meaning in great ape communication: summarising the debate. Anim. Cogn. 19 : 1 233– 38 [Google Scholar]
- Scott-Phillips T. 2017 . Pragmatics and the aims of language evolution. Psychon. Bull. Rev. 24 : 1 186– 89 [Google Scholar]
- Scott-Phillips T , Blythe RA. 2013 . Why is combinatorial communication rare in the natural world, and why is language an exception to this trend?. J. R. Soc. Interface 10 : 88 20130520 [Google Scholar]
- Scott-Phillips T , Blythe R , Gardner A , West S 2012 . How do communication systems emerge?. Proc. R. Soc. B 279 : 1943– 49 [Google Scholar]
- Scott-Phillips T , Diggle S , Gurney J , Ivens A , Popat R. 2014 . Combinatorial communication in bacteria: implications for the origins of linguistic generativity. PLOS ONE 9 : 4 e95929 Shows experimentally that combinatorial communication systems are present even in bacteria. [Google Scholar]
- Scott-Phillips T , Kirby S 2010 . Language evolution in the laboratory. Trends Cogn. Sci. 14 : 9 411– 17 [Google Scholar]
- Scott-Phillips T , Kirby S 2013 . Information, influence and inference in language evolution. Animal Communication Theory: Information and Influence U Stegmann 421– 42 Cambridge, UK: Cambridge Univ. Press [Google Scholar]
- Searcy WA , Nowicki S. 2005 . The Evolution of Animal Communication Princeton, NJ: Princeton Univ. Press [Google Scholar]
- Seyfarth RM , Cheney DL. 2003 . Signalers and receivers in animal communication. Annu. Rev. Psychol. 54 : 145– 73 [Google Scholar]
- Seyfarth RM , Cheney DL. 2018 . The Social Origins of Language Princeton, NJ: Princeton Univ. Press [Google Scholar]
- Seyfarth RM , Cheney DL , Marler P. 1980 . Vervet monkey alarm calls: semantic communication in a free-ranging primate. Anim. Behav. 28 : 1070– 94 [Google Scholar]
- Sperber D , Origgi G 2010 . A pragmatic account of the origin of language. The Evolution of Human Language: Biolinguistic Perspectives RK Larson, V Déprez, H Yamakido 124– 31 Cambridge, UK: Cambridge Univ. Press [Google Scholar]
- Sperber D , Wilson D. 2002 . Pragmatics, modularity and mind-reading. Mind Lang . 17 : 1/2 3– 23 [Google Scholar]
- Spotorno N , Koun E , Prado J , van der Henst JB , Noveck IA. 2012 . Neural evidence that utterance-processing entails mentalizing: the case of irony. NeuroImage 63 : 1 25– 39 [Google Scholar]
- Suzuki TN , Zuberbühler K. 2019 . Animal syntax. Curr. Biol. 29 : 14 R669– 71 [Google Scholar]
- ten Cate C. 2017 . Assessing the uniqueness of language: Animal grammatical abilities take center stage. Psychon. Bull. Rev. 24 : 1 91– 96 [Google Scholar]
- Tomasello M 2003 . On the different origins of symbols and grammar. Language Evolution S Kirby, M Christiansen 94– 110 Oxford, UK: Oxford Univ. Press [Google Scholar]
- Tomasello M , Call J. 2019 . Thirty years of great ape gestures. Anim. Cogn. 22 : 4 461– 69 [Google Scholar]
- Tomasello M , Carpenter M , Liszkowski U. 2007 . A new look at infant pointing. Child Dev . 78 : 3 705– 22 [Google Scholar]
- Townsend SW , Engesser S , Stoll S , Zuberbühler K , Bickel B. 2018 . Compositionality in animals and humans. PLOS Biol . 16 : 8 e2006425 [Google Scholar]
- Truswell R. 2017 . Dendrophobia in bonobo comprehension of spoken English. Mind Lang . 32 : 4 395– 415 [Google Scholar]
- van Hooff JA. 1972 . A comparative approach to the phylogeny of laughter and smiling. Nonverbal Communication RA Hinde 209– 41 Cambridge, UK: Cambridge Univ. Press [Google Scholar]
- Vanlangendonck F , Willems RM , Hagoort P. 2018 . Taking common ground into account: specifying the role of the mentalizing network in communicative language production. PLOS ONE 13 : 10 e0202943 [Google Scholar]
- Vettin J , Todt D. 2005 . Human laughter, social play, and play vocalizations of nonhuman primates: an evolutionary approach. Behaviour 142 : 217– 40 [Google Scholar]
- Warren E , Call J. 2022 . Inferential communication: bridging the gap between intentional and ostensive communication in non-human primates. Front. Psychol. 12 : 718251 Presents an innovative cognitive analysis of nonhuman primate communication, with conclusions similar to those of this review. [Google Scholar]
- Washburn MF. 1908 . The Animal Mind: A Text-Book of Comparative Psychology London: Macmillan [Google Scholar]
- Wheeler BC , Fischer J. 2012 . Functionally referential signals: a promising paradigm whose time has passed. Evol. Anthropol. 21 : 5 195– 205 [Google Scholar]
- Wilson D. 2003 . Relevance and lexical pragmatics. Ital. J. Linguist. 15 : 273– 92 [Google Scholar]
- Wilson D , Carston R. 2007 . A unitary approach to lexical pragmatics: relevance, inference and ad hoc concepts. Pragmatics N Burton-Roberts 230– 59 London: Palgrave [Google Scholar]
- Wilson D , Sperber D. 2012 . Meaning and Relevance Cambridge, UK: Cambridge Univ. Press [Google Scholar]
- Zlatev J , Żywiczyński P , Wacewicz S. 2020 . Pantomime as the original human-specific communicative system. J. Lang. Evol. 5 : 2 156– 74 [Google Scholar]
- Zuberbühler K. 2018 . Combinatorial capacities in primates. Curr. Opin. Behav. Sci. 21 : 161– 69 [Google Scholar]
- Zuberbühler K. 2020 . Syntax and compositionality in animal communication. Philos. Trans. R. Soc. B 375 : 1789 20190062 Presents a more detailed review of combinatorial communication systems in nonhumans. [Google Scholar]
Data & Media loading...
- Article Type: Review Article
Most Read This Month
Most cited most cited rss feed, bilingualism, mind, and brain, how nature meets nurture: universal grammar and statistical learning, the indo-european homeland from linguistic and archaeological perspectives, sign language typology: the contribution of rural sign languages, correlational studies in typological and historical linguistics, advances in dialectometry, genetics and the language sciences, ditransitive constructions, diachronic semantics, vagueness and imprecision: empirical foundations.
Information
- Author Services
Initiatives
You are accessing a machine-readable page. In order to be human-readable, please install an RSS reader.
All articles published by MDPI are made immediately available worldwide under an open access license. No special permission is required to reuse all or part of the article published by MDPI, including figures and tables. For articles published under an open access Creative Common CC BY license, any part of the article may be reused without permission provided that the original article is clearly cited. For more information, please refer to https://www.mdpi.com/openaccess .
Feature papers represent the most advanced research with significant potential for high impact in the field. A Feature Paper should be a substantial original Article that involves several techniques or approaches, provides an outlook for future research directions and describes possible research applications.
Feature papers are submitted upon individual invitation or recommendation by the scientific editors and must receive positive feedback from the reviewers.
Editor’s Choice articles are based on recommendations by the scientific editors of MDPI journals from around the world. Editors select a small number of articles recently published in the journal that they believe will be particularly interesting to readers, or important in the respective research area. The aim is to provide a snapshot of some of the most exciting work published in the various research areas of the journal.
Original Submission Date Received: .
- Active Journals
- Find a Journal
- Proceedings Series
- For Authors
- For Reviewers
- For Editors
- For Librarians
- For Publishers
- For Societies
- For Conference Organizers
- Open Access Policy
- Institutional Open Access Program
- Special Issues Guidelines
- Editorial Process
- Research and Publication Ethics
- Article Processing Charges
- Testimonials
- Preprints.org
- SciProfiles
- Encyclopedia
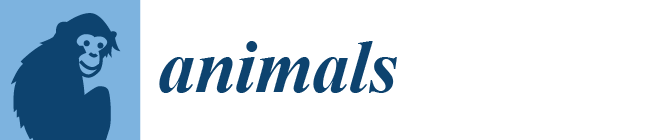
Journal Menu
- Animals Home
- Aims & Scope
- Editorial Board
- Reviewer Board
- Topical Advisory Panel
- Instructions for Authors
- Special Issues
- Sections & Collections
- Article Processing Charge
- Indexing & Archiving
- Editor’s Choice Articles
- Most Cited & Viewed
- Journal Statistics
- Journal History
- Journal Awards
- Society Collaborations
- Conferences
- Editorial Office
Journal Browser
- arrow_forward_ios Forthcoming issue arrow_forward_ios Current issue
- Vol. 14 (2024)
- Vol. 13 (2023)
- Vol. 12 (2022)
- Vol. 11 (2021)
- Vol. 10 (2020)
- Vol. 9 (2019)
- Vol. 8 (2018)
- Vol. 7 (2017)
- Vol. 6 (2016)
- Vol. 5 (2015)
- Vol. 4 (2014)
- Vol. 3 (2013)
- Vol. 2 (2012)
- Vol. 1 (2011)
Find support for a specific problem in the support section of our website.
Please let us know what you think of our products and services.
Visit our dedicated information section to learn more about MDPI.
Animal Communication
- Print Special Issue Flyer
- Special Issue Editors
Special Issue Information
- Published Papers
A special issue of Animals (ISSN 2076-2615). This special issue belongs to the section " Human-Animal Interactions, Animal Behaviour and Emotion ".
Deadline for manuscript submissions: closed (1 July 2018) | Viewed by 78230
Share This Special Issue
Special issue editor.
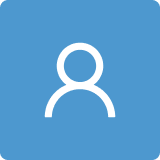
Dear Colleagues,
For decades researchers have studied the ways in which animals communicate by sending and receiving signals in the visual, auditory, tactile and chemical modalities. More recently, communication via electric signals has been studied in electric fish and communication using seismic signals has been recognised although far less studied.
Communication is essential to the development and maintenance of social systems in vertebrates and invertebrates. It is used in a variety of situations, including courtship, advertising the presence of food or territory, conveying anger and aggression, and in parent-offspring interaction. In some species, vocal communication is used to alert conspecifics to the presence of a predator, often as referential signals sent intentionally and conveying information about the type of predator. Vocal turn-taking is of recent research interest, as it either mimics, or is the precursor to, human language. Indeed, mimicry in its own right is relevant to this special issue.
Communication can be either honest or deceptive depending on context. Communication within and between species is shaped by both the social and physical environment; a recently studied example of the latter is the potential influence of background noise (e.g., that produced by wind or waves, and anthropocentric noise) on the vocalisations of birds, insects and other animals.
This special issue is interested in both reviews and research papers on all aspects of communication, signal production and processing, in vertebrate and invertebrate species, including non-verbal communication in humans. It is also interested in the neural processes involved in both producing and processing signals and in learning the meaning of signals. This includes papers on specialisations of both the central nervous system and the peripheral organs that channel the perception of, and response to, signals.
Prof. Lesley J. Rogers Guest Editor
Manuscripts should be submitted online at www.mdpi.com by registering and logging in to this website . Once you are registered, click here to go to the submission form . Manuscripts can be submitted until the deadline. All submissions that pass pre-check are peer-reviewed. Accepted papers will be published continuously in the journal (as soon as accepted) and will be listed together on the special issue website. Research articles, review articles as well as short communications are invited. For planned papers, a title and short abstract (about 100 words) can be sent to the Editorial Office for announcement on this website.
Submitted manuscripts should not have been published previously, nor be under consideration for publication elsewhere (except conference proceedings papers). All manuscripts are thoroughly refereed through a single-blind peer-review process. A guide for authors and other relevant information for submission of manuscripts is available on the Instructions for Authors page. Animals is an international peer-reviewed open access semimonthly journal published by MDPI.
Please visit the Instructions for Authors page before submitting a manuscript. The Article Processing Charge (APC) for publication in this open access journal is 2400 CHF (Swiss Francs). Submitted papers should be well formatted and use good English. Authors may use MDPI's English editing service prior to publication or during author revisions.
- perception of signals
- receptor filtering
- processing of signals
- response to signals
- social systems
- communication networks
- evolution of communication
- meaning of signals
- referential signals
- modality specificity
- multimodal communication
- flexibility
- context dependence
- neural processes involved
Published Papers (6 papers)
Jump to: Review
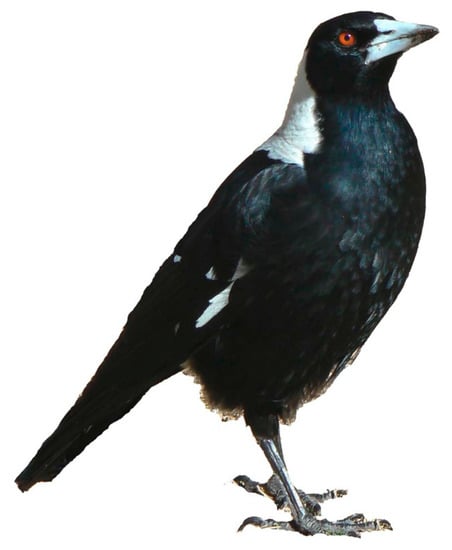
Graphical abstract
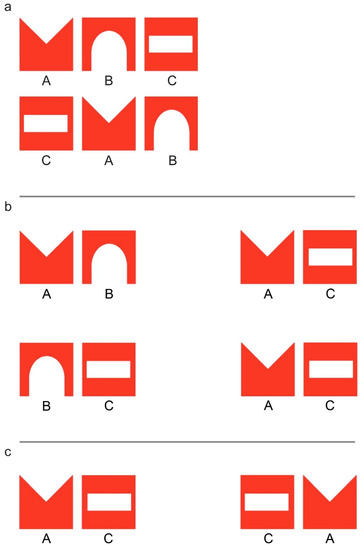
Jump to: Research
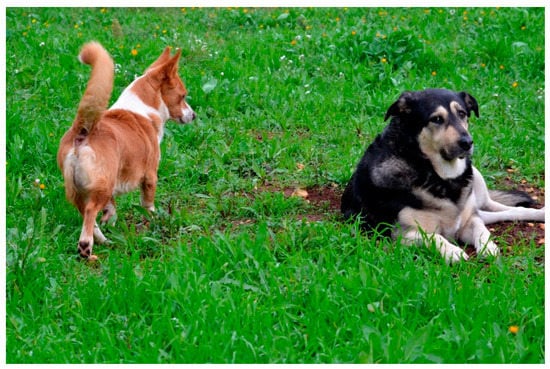
Further Information
Mdpi initiatives, follow mdpi.
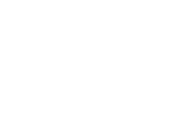
Subscribe to receive issue release notifications and newsletters from MDPI journals
Academia.edu no longer supports Internet Explorer.
To browse Academia.edu and the wider internet faster and more securely, please take a few seconds to upgrade your browser .
- We're Hiring!
- Help Center
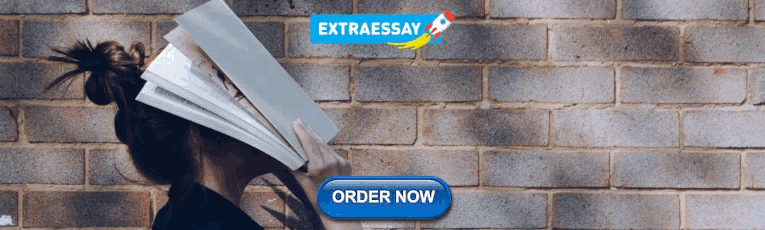
Animal communication
- Most Cited Papers
- Most Downloaded Papers
- Newest Papers
- Last »
- Animal Behavior Follow Following
- Animal Cognition Follow Following
- Bioacoustics Follow Following
- Acoustic Communication Follow Following
- Acoustic Ecology Follow Following
- Behavioral Ecology Follow Following
- Social behavior in animals Follow Following
- Cognitive Ethology Follow Following
- Animal Behaviour Follow Following
- Primatology Follow Following
Enter the email address you signed up with and we'll email you a reset link.
- Academia.edu Journals
- We're Hiring!
- Help Center
- Find new research papers in:
- Health Sciences
- Earth Sciences
- Cognitive Science
- Mathematics
- Computer Science
- Academia ©2024
The Animal Communication System (ANICOMs): Some Interesting Observations
- December 2017
- International Journal of Communication 27(2):7-47
- This person is not on ResearchGate, or hasn't claimed this research yet.

- Indian Statistical Institute
Discover the world's research
- 25+ million members
- 160+ million publication pages
- 2.3+ billion citations

- Marcelina Kriese

- Andrzej Gugołek

- Proc Biol Sci

- Scott A. Davis
- Hubert Frings
- Karl von Frisch
- Eric H. Lenneberg
- Konrad Z. Lorenz
- Marjorie Kerr Wilson

- A. J. Premack

- Recruit researchers
- Join for free
- Login Email Tip: Most researchers use their institutional email address as their ResearchGate login Password Forgot password? Keep me logged in Log in or Continue with Google Welcome back! Please log in. Email · Hint Tip: Most researchers use their institutional email address as their ResearchGate login Password Forgot password? Keep me logged in Log in or Continue with Google No account? Sign up

An official website of the United States government
The .gov means it’s official. Federal government websites often end in .gov or .mil. Before sharing sensitive information, make sure you’re on a federal government site.
The site is secure. The https:// ensures that you are connecting to the official website and that any information you provide is encrypted and transmitted securely.
- Publications
- Account settings
- My Bibliography
- Collections
- Citation manager
Save citation to file
Email citation, add to collections.
- Create a new collection
- Add to an existing collection
Add to My Bibliography
Your saved search, create a file for external citation management software, your rss feed.
- Search in PubMed
- Search in NLM Catalog
- Add to Search
Advances in single-cell transcriptomics in animal research
Affiliations.
- 1 Institute of Dairy Science, Ministry of Education Key Laboratory of Molecular Animal Nutrition, College of Animal Sciences, Zhejiang University, Hangzhou, 310058, China.
- 2 Key Laboratory of Dairy Cow Genetic Improvement and Milk Quality Research of Zhejiang Province, Zhejiang University, Hangzhou, 310058, China.
- 3 Agency for Health and Food Safety Austria, 1220, Vienna, Austria.
- 4 Institute of Dairy Science, Ministry of Education Key Laboratory of Molecular Animal Nutrition, College of Animal Sciences, Zhejiang University, Hangzhou, 310058, China. [email protected].
- 5 Key Laboratory of Dairy Cow Genetic Improvement and Milk Quality Research of Zhejiang Province, Zhejiang University, Hangzhou, 310058, China. [email protected].
- PMID: 39090689
- PMCID: PMC11295521
- DOI: 10.1186/s40104-024-01063-y
Understanding biological mechanisms is fundamental for improving animal production and health to meet the growing demand for high-quality protein. As an emerging biotechnology, single-cell transcriptomics has been gradually applied in diverse aspects of animal research, offering an effective method to study the gene expression of high-throughput single cells of different tissues/organs in animals. In an unprecedented manner, researchers have identified cell types/subtypes and their marker genes, inferred cellular fate trajectories, and revealed cell‒cell interactions in animals using single-cell transcriptomics. In this paper, we introduce the development of single-cell technology and review the processes, advancements, and applications of single-cell transcriptomics in animal research. We summarize recent efforts using single-cell transcriptomics to obtain a more profound understanding of animal nutrition and health, reproductive performance, genetics, and disease models in different livestock species. Moreover, the practical experience accumulated based on a large number of cases is highlighted to provide a reference for determining key factors (e.g., sample size, cell clustering, and cell type annotation) in single-cell transcriptomics analysis. We also discuss the limitations and outlook of single-cell transcriptomics in the current stage. This paper describes the comprehensive progress of single-cell transcriptomics in animal research, offering novel insights and sustainable advancements in agricultural productivity and animal health.
Keywords: Disease modelling; Genetics; Health; Livestock; Nutrition; Reproductive performance; Single-cell RNA sequencing.
© 2024. The Author(s).
PubMed Disclaimer
Conflict of interest statement
The authors declare that they have no competing interests.
The workflow of single-cell/nucleus RNA…
The workflow of single-cell/nucleus RNA sequencing
Functional mechanism of rumen short-chain…
Functional mechanism of rumen short-chain fatty acids uptake
A summary of the common…
A summary of the common cell types identified in the gastrointestinal tract of…
- Otto O, Rosendahl P, Mietke A, Golfier S, Herold C, Klaue D, et al. Real-time deformability cytometry: On-the-fly cell mechanical phenotyping. Nat Methods. 2015;12(3):199–202. 10.1038/nmeth.3281. 10.1038/nmeth.3281 - DOI - PubMed
- Brady G, Barbara M, Iscove NN. Representative in vitro cDNA amplification from individual hemopoietic cells and colonies. Methods Mol Cell Biol. 1990;2:17–25. https://wwwlabs.uhnresearch.ca/labs/iscove/MMCB90.pdf .
- Maxam AM, Gilbert WA. A new method for sequencing DNA. Proc Natl Acad Sci. 1977;74(2):560–4. 10.1073/pnas.74.2.560. 10.1073/pnas.74.2.560 - DOI - PMC - PubMed
- Sanger F, Coulson AR. A rapid method for determining sequences in DNA by primed synthesis with DNA polymerase. J Mol Biol. 1975;94(3):441–8. 10.1016/0022-2836(75)90213-2. 10.1016/0022-2836(75)90213-2 - DOI - PubMed
- Stark R, Grzelak M, Hadfield J. RNA sequencing: The teenage years. Nat Rev Genet. 2019;20(11):631–56. 10.1038/s41576-019-0150-2. 10.1038/s41576-019-0150-2 - DOI - PubMed
Publication types
- Search in MeSH
Grants and funding
- LR23C170001/Natural Science Foundation of Zhejiang Province Outstanding Youth Fund Project
LinkOut - more resources
Full text sources.
- BioMed Central
- PubMed Central
Research Materials
- NCI CPTC Antibody Characterization Program
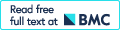
- Citation Manager
NCBI Literature Resources
MeSH PMC Bookshelf Disclaimer
The PubMed wordmark and PubMed logo are registered trademarks of the U.S. Department of Health and Human Services (HHS). Unauthorized use of these marks is strictly prohibited.
- Open access
- Published: 02 August 2024
Advances in single-cell transcriptomics in animal research
- Yunan Yan 1 ,
- Senlin Zhu 1 ,
- Minghui Jia 1 ,
- Xinyi Chen 1 ,
- Wenlingli Qi 1 ,
- Fengfei Gu 1 , 2 ,
- Teresa G. Valencak 1 , 3 ,
- Jian-Xin Liu 1 &
- Hui-Zeng Sun ORCID: orcid.org/0000-0001-5380-6030 1 , 2
Journal of Animal Science and Biotechnology volume 15 , Article number: 102 ( 2024 ) Cite this article
425 Accesses
Metrics details
Understanding biological mechanisms is fundamental for improving animal production and health to meet the growing demand for high-quality protein. As an emerging biotechnology, single-cell transcriptomics has been gradually applied in diverse aspects of animal research, offering an effective method to study the gene expression of high-throughput single cells of different tissues/organs in animals. In an unprecedented manner, researchers have identified cell types/subtypes and their marker genes, inferred cellular fate trajectories, and revealed cell‒cell interactions in animals using single-cell transcriptomics. In this paper, we introduce the development of single-cell technology and review the processes, advancements, and applications of single-cell transcriptomics in animal research. We summarize recent efforts using single-cell transcriptomics to obtain a more profound understanding of animal nutrition and health, reproductive performance, genetics, and disease models in different livestock species. Moreover, the practical experience accumulated based on a large number of cases is highlighted to provide a reference for determining key factors (e.g., sample size, cell clustering, and cell type annotation) in single-cell transcriptomics analysis. We also discuss the limitations and outlook of single-cell transcriptomics in the current stage. This paper describes the comprehensive progress of single-cell transcriptomics in animal research, offering novel insights and sustainable advancements in agricultural productivity and animal health.
Introduction
Cells, the basic unit of life, vary widely in shape, size, and gene expression. It is vital to explore the different biological properties of individual cells in complex tissues to understand the process of life activities. The invention of flow cytometry at the end of the 1960s was a major breakthrough in both qualitative and quantitative measurements of cellular characteristics, as well as cell sorting [ 1 ]. It remains a widely applied strategy for single-cell analysis and isolation to date. In 1990, polymerase chain reaction was introduced to amplify DNA or RNA in individual cells, providing even more functional information [ 2 ], which further demonstrated that transcriptomic studies on individual cells were feasible. However, this method is limited by amplification bias and low throughput. The development of first-generation sequencing techniques made significant progress in molecular sequencing technology, but the costs remained high, and the sequencing throughput was low [ 3 , 4 ]. Next-generation sequencing technologies, such as “sequencing by synthesis” and accelerated parallel sequencing, have successfully overcome the above limitations, and RNA sequencing (RNA-Seq) is the best-known and most commonly used approach to date [ 5 ]. RNA-Seq involves total RNA extracted from organs, tissues, or a group of cells, obtaining average transcriptomic data while often masking specific information of individual cells in the population. To systematically study complex biological processes at higher resolution and reveal functional heterogeneity within tissues, researchers have developed a series of technologies, including multidimensional studies at the single-cell level. In 2009, Tang’s team developed the single-cell RNA sequencing (scRNA-Seq) method, enabling large-scale access to gene expression information from individual cells [ 6 ]. Since then, the use of scRNA-Seq has undergone rapid growth and evolution. In 2018, the journal “Science” ranked scRNA-Seq at the top of its list of the year’s most noteworthy technologies. Using scRNA-seq, international research projects, such as the Human Cell Atlas ( https://www.humancellatlas.org/ ), have been launched to identify all cell types involved in human development, health, and disease. Notably, a collaborative project consortium focusing on livestock, FarmGTEx ( http://farmgtex.org/ ), joined forces in the single-cell transcriptomic research of different livestock species by bringing together researchers from around the world to discover regulatory variants, molecular targets, and phenotype predictions at the single-cell level. Single-cell technologies are now emerging at multiple molecular levels, including genomics, transcriptomics, epigenomics, proteomics, and metabolomics, at continuously enhanced resolution, accuracy, and efficiency, setting the stage for the practical application of single-cell technologies.
Single-cell RNA sequencing is a technology that can be used to comprehensively reveal the gene expression profiles of cells by sequencing transcripts in individual cells one by one [ 7 ]. It is currently the most mature technology for high-throughput functional resolution at the single-cell level. As shown in Fig. 1 , the process begins with the dissociation of fresh tissue into a single-cell suspension, followed by the selection of different single-cell capture strategies depending on the cell numbers. Manual operations (e.g., limited dilution, laser cutting, micromanipulation) are generally chosen for small cell numbers, but microfluidic and microwell techniques are used when the cell throughput is on the order of tens of thousands [ 7 ]. After single-cell capture, cDNA is obtained through reverse transcription, amplified, and then sequenced. Downstream analysis can be conducted after completion of the single-cell sequencing run. The analysis process typically involves 3 stages: primary analysis (base detection), secondary analysis (multiple isolation, alignment, and genetic identification), and tertiary analysis (data visualization and interpretation) [ 8 ]. As scRNA-Seq involves a sufficient number of active cells from fresh samples, it is impossible to perform it efficiently from frozen or indigestible samples. The emergence of modern single-nucleus RNA sequencing has solved this problem by inserting an extraction procedure to extract the nuclei of single cells before isolating and labelling the nuclei, making it possible to detect nuclear gene expression at the single-cell level [ 9 , 10 ]. However, this method is currently not applicable for immune cells, and sequencing sensitivity may be low due to the low abundance of mRNA in the nuclei of some cells. Overall, single-cell transcriptomics has allowed us to fully decipher cell types/subtypes and functions in almost all species by constructing a reference catalogue of gene expression encompassing cells throughout the body. In addition, single-cell transcriptomic data can be visualized in multiple dimensions via bioinformatics analyses, such as differential enrichment and proposed time-series analyses. These strategies enable the reconstruction and simulation of biological system operations.
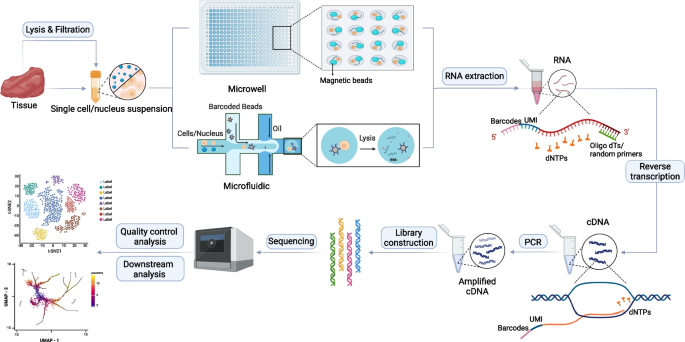
The workflow of single-cell/nucleus RNA sequencing
Livestock produces more than 15% of the world’s high-quality protein. The Food and Agriculture Organization of the United Nations predicts that by 2050, up to 50% of animal food will be required to feed 10 billion people. Rapidly emerging bioscience biotechnologies have assisted animal science experts in producing even more animal products with limited resources. However, when facing new challenges, such as studying the biological factors determining economic traits, product quality, and animal health, it is crucial to use high-throughput methods, especially single-cell transcriptomics, to better investigate cellular functions and interactions. Relevant research has already been conducted in animal studies worldwide using scRNA-Seq (see Additional file 1 for details). For example, identifying and annotating various cell types in livestock can reveal their specific functions and interactions, which is crucial for obtaining an even better understanding of the cellular composition, differentiation status, and intercellular regulatory networks in animal tissues and organs. This technology provides high-resolution information on gene expression in individual cells, facilitating the discovery and comparison of expression differences between cells and allowing for the identification of key genes related to economically important characteristics, nutrient metabolism, and disease resistance. In addition, scRNA-Seq can enable the discovery of new genes and regulatory elements, particularly those that are poorly expressed or cell-specific. Thus, our understanding of livestock animal genomes is enhanced, and a new approach to functional studies is provided. The transcriptomic changes in cells can be tracked at different time points via scRNA-Seq, revealing the dynamic processes of cell development, differentiation, and functional transformation. This information is important for understanding tissue growth, development, regeneration, and immune responses in livestock.
Our paper provides an overview of the use of scRNA-Seq in livestock research in recent years. It also offers insights and references on the single-cell data analysis process, which can serve to make scRNA-Seq a more robust tool for research on animal husbandry and breed optimization.
Applications of single-cell transcriptomics for livestock husbandry
Exploration of nutrient metabolism and immune responses.
The gastrointestinal tract (GIT) serves as a vital organ for nutrient absorption, and the mucosal immune system in animals largely affects production performance, animal welfare, and the safety of livestock products. Through single-cell sequencing of the livestock GIT, we will be able to investigate the composition and distinct metabolic patterns of various cell types, and ultimately, we may provide precise targets for nutritional manipulation and enhance nutrient absorption in these animals. Among the GIT in ruminants, the rumen holds undeniable significance for nutrient digestion and absorption, particularly for short-chain fatty acids (SCFAs), which are absorbed by the rumen epithelium and can meet 70%–80% of the body’s energy requirements [ 11 ]. However, the rumen epithelium is a complex structure consisting of four layers of cells of different types and functions. This complexity has hindered mechanistic explorations of rumen epithelial absorption and turnover of specific nutrients. Earlier scRNA-Seq work has shown that spiny cells of the rumen epithelium play a crucial role in SCFAs [ 12 ], but more detailed cell subtypes were not identified due to factors such as the lack of identified marker genes. The application of the newly developed rumen single-cell suspension preparation method for scRNA-Seq has given rise to even more comprehensive insights into rumen epithelial cell types. Wu et al. [ 13 ] integrated and expanded specific marker genes for bovine cell lineages based on previous studies; 20,728 rumen epithelial cells were clustered into 18 rumen epithelial cell types, and the specific metabolic characteristics of each cell subtype were characterized. The ability of different epithelial cells to absorb SCFAs was explored by analysing the expression of genes encoding transporter proteins and scoring the related functional pathways. The findings revealed that channel-gap-like (Cg-like) spinous cell regulated by IL-17 was the preferred subtype for SCFA absorption in dairy cows [ 13 ]. The microbiota is essential for nutrient absorption in the GIT, and further joint mining of rumen metagenomic data characterized the interactions between Cg-like cells and fibre-degrading bacteria via structural domains of the secreted proteins (Fig. 2 ) [ 14 ]. Unfortunately, the study did not address ruminal nutrient uptake during different developmental stages. Studies on intestinal nutrient absorption in monogastric animals based on single-cell transcriptomics have primarily concentrated on cross-species comparisons of distinct cell types and functional analyses. Specifically, studies have examined significant interspecies differences and regional characteristics in hormone-secreting enteroendocrine cell [ 15 , 16 ]. For example, there is a gradual decrease in the number of these cells after the birth of piglets [ 17 ].
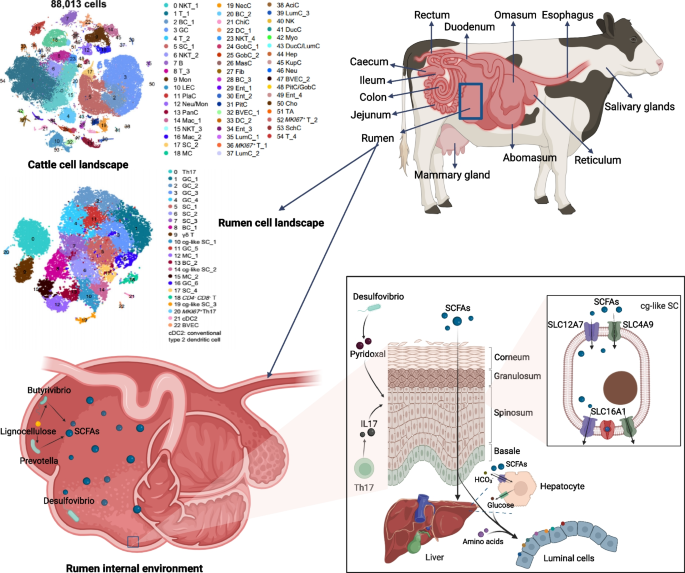
Functional mechanism of rumen short-chain fatty acids uptake
The immune system is vital for animal health and plays a pivotal role in defending against external hazards by maintaining physiological equilibrium. The application of scRNA-Seq for investigating livestock immunity has generated valuable insights. The advent of scRNA-Seq has contributed to an in-depth understanding of functional heterogeneity, molecular regulatory mechanisms, and infection responses among cellular subsets within central immune organs (e.g., the bursa and thymus) and peripheral immune organs (e.g., Pyle’s collecting lymph node) [ 18 , 19 , 20 ]. However, investigations on the bone marrow of domestic animals remain a major issue. Single-cell transcriptional profiling of various immune cells, such as peripheral blood monocytes and lymphocytes of domestic animals, underlines the homology and species specificity of immune gene expression, providing a molecular basis for understanding the immune response process in livestock [ 21 , 22 , 23 , 24 , 25 , 26 , 27 , 28 , 29 , 30 , 31 ]. For example, single-cell transcriptomic data of peripheral blood mononuclear cells (PBMCs) following disease in domestic animals have been used to identify pathogenic immune cell subpopulations and transcriptional modules driving pathogenesis [ 32 ]. This approach has helped with identifying subpopulations and molecular markers of PBMCs in chickens infected with avian influenza and avian leukemia virus [ 33 , 34 , 35 ], unravelling key signalling factors associated with lipopolysaccharide-induced glycolipid metabolism abnormalities in cattle [ 22 ], and uncovering cell types and genes linked to periparturient immunosuppression in dairy cows [ 36 ]. In recent years, single-cell sequencing research has shifted towards porcine immune cells during piglet weaning stress, weaning diarrhoea, and intestinal inflammation, as it enables the tracking of immune cell heterogeneity during pathogenic microbial infections and host responses during diseases. For example, scRNA-Seq analysis revealed that tumor necrosis factor-α secreted by different immune cells could contribute to disease, as observed in studies investigating African swine fever and porcine intestinal inflammation [ 37 , 38 ]. These observations have opened new avenues for the development of novel vaccines and targeted therapies. In summary, scRNA-Seq studies allow for the precise identification and analysis of cell types and their interactions involved in the immune processes of domestic animals. They also allow for the examination of regulated genes, signalling pathways, transcription factors, and immune cell subpopulations with potential pathogenic functions. Current studies primarily focus on exploring immune mechanisms, however the potential of scRNA-Seq could be harnessed to evaluate the efficacy and response to immunotherapy. This involves concentrating on T cells, which are important players in the immune response and play key roles in animal health.
Investigation of cell factors for animal reproductive performance
The level of reproductive performance in livestock, which can directly affect productivity, depends on the ability to produce high-quality male and female gametes. The use of scRNA-Seq combined with proposed time-series analysis has allowed for the careful investigation of the dynamic mapping of cell fate transitions and gene expression changes during spermatogenesis in dairy goats, sheep, yaks, and premature piglets [ 39 , 40 , 41 , 42 , 43 , 44 , 45 ]. Through comprehensive analyses, researchers have identified specific marker genes and key signalling pathways related to germ cells within the testes of farm animals. Furthermore, studies have explored the homology and differences in male germ lines across different species, expanding our understanding of testicular development and spermatogenesis [ 40 , 46 , 47 ]. It has become evident that spermatogenesis relies on an ecological niche composed of testicular somatic cells. Consequently, studies have increasingly focused even more on single-cell sequencing analyses of these cells over the past few decades. However, due to variations in study samples and resolution, there are significant knowledge gaps related to the classification of testicular somatic cells, necessitating further investigation [ 40 , 42 , 43 , 44 , 46 ]. The growth and development of ovarian follicles are primarily regulated by granulosa cells. Through single-cell transcriptomics, the heterogeneity and differentiation pathways of follicular cells and granulosa cells in the ovaries of livestock animals have been characterized [ 48 , 49 , 50 , 51 , 52 ]. However, intercellular interactions within the ovary remain poorly understood. Recent studies in yaks, goats, and domesticated pigs have attempted to fill this gap [ 53 , 54 , 55 , 56 ]. For instance, Chen et al. discovered that porcine ovarian mural granulosa cells primarily engage in intercellular communication with cells of the same type, whereas ovarian theca granulosa cells predominantly emit signalling cues to different cell types [ 53 ]. Nevertheless, these studies are based on relatively small sample sizes, and more in-depth work is needed to corroborate their findings. High-precision mapping of germ cell genesis in livestock animals has enabled researchers to conduct in-depth studies on the screening, diagnosis, and treatment of reproduction-related disorders, such as sperm damage, abnormal oocyte development, and male infertility, in the progeny of interspecifically crossbred individuals [ 57 , 58 , 59 ]. The regulatory characteristics and interspecific differences of various types of cells in the gonads of livestock animals obtained at specific time points represent another research focus that can be assessed by single-cell transcriptomics [ 60 , 61 , 62 ]. To date, continuous developmental differentiation in livestock gonads is poorly understood. Only the gonadal differentiation of the chicken embryo has been analysed, and the discovery that the supporting cells during gonadal differentiation in the chicken embryo are derived from mesenchymal stem cells, in contrast to other vertebrates, has revolutionized our previous understanding of gonadal cell types [ 63 ]. Notably, noncoding RNAs significantly affect the regulation of germ cell proliferation and differentiation in livestock [ 40 , 64 ]. However, capturing these noncoding RNAs remains challenging, necessitating advancements in the application of this technology to enhance our understanding of livestock reproductive performance [ 65 ].
Single-cell transcriptomics has also been widely accepted as an efficient tool for investigating cell fate and transcriptional regulation during embryonic development. Studies employing scRNA-Seq have examined embryos at various life stages in livestock, such as cattle, pigs, chickens, sheep, and rabbits, thus making dynamic transcriptional profiles and cellular differentiation trajectories accessible within each germ layer of early-stage embryos [ 66 , 67 , 68 , 69 , 70 , 71 , 72 , 73 , 74 ]. For example, these analyses have uncovered species-specific features of early embryonic development (E5-E13) in pigs, revealing the differentiation of bovine trophoblast mononuclear cells into binucleated cells and the changes in differential gene expression and associated signalling pathways from the 8-cell to the mulberry embryo stage in sheep [ 67 , 68 , 71 ]. By leveraging scRNA-Seq, researchers can also analyse embryos with developmental abnormalities or those transferred from in vitro cultures. These investigations contribute to identifying potential causes of developmental abnormalities, offering valuable guidance for improving reproductive techniques such as in vitro fertilization and embryo transfer. It has been observed that incomplete activation of certain metabolic pathways leads to metabolic abnormalities. Epigenetic modification may be responsible for the significant effects on subsequent pregnancy and calving rates in females, and comparing the developmental transcriptional profiles of embryos transferred in vitro from different states [ 75 ] may provide new ideas for the treatment of embryonic developmental abnormalities.
Elucidation of genetics and developmental biology in livestock
Throughout the ontogeny of an organism, the transcriptome of certain cells undergoes substantial transformation. scRNA-Seq represents a novel approach for elucidating the dynamic patterns of gene expression during livestock genetic development and for revealing the regulatory networks governing developmental and evolutionary processes. The ultimate goal is to depict the trajectory of cellular fate transformations. Developmental mapping has been performed on the nervous systems and skeletal limbs of monogastric animals [ 74 , 76 , 77 , 78 ] while focusing on the nodes and key cell types involved in the developmental differentiation of organs or tissues. It is worth mentioning that aided by single-cell transcriptomics of embryonic limbs in poultry, studies have pinpointed pivotal regulators and signalling pathways driving limb differentiation formation along with their regionalization for the first time [ 79 , 80 ]. Among ruminants, rumen development has received significant attention. Scientists have constructed a comprehensive developmental landscape of thirteen metabolic tissues in ruminants [ 81 , 82 ], which encompasses a holistic comparison of heterogeneity within rumen epithelial cell types, cell functions and interacting microbiota between calves and adults. These findings suggested that calf epithelial progenitor cells exhibit greater differentiation potential and display greater activity in cell proliferation, differentiation, and innate immune responses [ 81 , 82 ]. Conversely, adult bovine cells show prominence in immune cells and have increased activity during antioxidant, adaptive immune, and fatty acid metabolism processes [ 81 , 82 ]. Similarly, studies have shown that the keratinization process during rumen epithelial cell development is associated with the cessation of keratinocyte differentiation at specific stages [ 12 , 83 ]. Previous studies have successfully identified key genes associated with rumen growth and development by scRNA-Seq of the rumen epithelium [ 84 , 85 ]. Overall, scRNA-Seq is assisting in the construction of a developmental evolutionary tree of livestock from the embryo to the mature individual. In single-cell transcriptional studies on animal genetic development, previously unidentified cell types are discovered, such as a specific class of endothelial cell clusters found in yak lungs, were revealed as potential factors in plateau acclimatization, and it was shown that calf-specific STOML3 + cells have the potential to maintain the internal environment of the liver [ 82 , 86 ]. In addition, the exploration of unexpected functionalities harboured by common cell types has become possible, e.g., luminal epithelial cells could be involved in both lactation and immune responses [ 13 ]. It is worth mentioning that whole-body single-cell atlases have been generated for several mammalian species [ 87 , 88 , 89 , 90 , 91 ], with those in humans and mice covering the entirety of life stages [ 92 ]. However, to date, comprehensive whole-body single-cell atlases are still lacking for livestock animals.
The comparison of single-cell transcriptomic data from high- and low-productivity livestock has become an approach to identify genes and key cell types related to economic traits and has led to the selection and breeding of highly productive livestock breeds. Taking meat production traits as an example, the quantity and quality of meat production largely depend on the development of skeletal muscle. Analyses of the single-cell transcriptomics of skeletal muscle from fat and lean pigs could effectively identify key genes for adipocyte differentiation and epigenetic modifications [ 93 , 94 , 95 ]. By analysing the developmental trajectories of myogenic progenitor cells, it was observed that the skeletal muscles of lean livestock were more closely related to myogenic progenitor cells and more responsible for muscle development than the skeletal muscles of fat pigs, suggesting that mechanistic explorations of myogenesis can lead to the study of differences in genealogical cell differentiation [ 93 , 96 ]. The identification of specific liver cell clusters between laying and non-egg-laying populations in egg-laying birds may also provide new insights for improving egg production in the future [ 97 ]. More information can also be acquired from single-cell transcriptomic studies of niche economic traits such as fleece- and silk-producing traits. The former is limited by the asynchronous development of the wool bursa [ 98 ], and the latter is limited by the understudied organ of the silk gland; neither the wool bursa nor silk glands have been accurately analysed previously for specific cell types. The emergence of scRNA-Seq has enabled in-depth knowledge of the distribution of the composition of each cell type and the trajectories of key cells during the different developmental periods of the wool bursa and silk glands [ 99 , 100 , 101 , 102 ]. Additionally, new marker genes involved in the synthesis of velvet and silk proteins have been identified [ 100 ], which can improve the quality of cashmere and silk. For instance, the ACTA2 , COL1A1 , and CLCL6 genes may regulate cashmere fineness [ 103 ]. Although single-cell transcriptomic analyses have shown promise for improving genetic breeding, current research results are difficult to apply in animal production or have limited impact. Future research concentrating on diseases that are commonly associated with production should follow. For example, particular cell subpopulations associated with mastitis in dairy cows can be identified.
Emergence of disease models
The utilization of scRNA-Seq in model animals is highly important, especially in pigs, an animal commonly used as a medical/disease model [ 104 , 105 , 106 ]. By employing scRNA-Seq, researchers have gained profound insights into the composition of organelle types between pigs and men, thereby revealing the heterogeneity and conservatism of gene expression and regulatory mechanisms within the biological processes of interest and promoting in-depth biomedical research and widespread use of the domestic pig as an animal model. Previous studies have focused on individual tissues or organs (e.g., immune cells [ 15 , 18 , 23 , 24 , 27 , 60 ], lungs [ 107 ], liver, embryos [ 108 ], the reproductive system [ 46 , 47 , 109 ], and the digestive tract [ 16 , 110 ]) in pigs. These studies utilized cross-species analyses to identify conserved or specific gene modules in tissues or screened for cellular subpopulations and risk genes associated with disease. Moreover, they have revealed gene–trait associations through modelling or induction. Pig lung tissue data were characterized for both similarities and differences in cellular communication and expression patterns of respiratory virus receptors in each cell type of the lung compared with human lung tissue data [ 107 ]. A comprehensive porcine brain atlas has facilitated the identification of cell types and risk genes linked to eight neurological disorders, e.g., attention deficiency [ 111 ]. Data from the pancreas have suggested that TXNIP , a stress gene in acinar cells, could become a potential target for the treatment of diabetes [ 112 ]. The investigation of the gallbladder in neonatal piglets has shed light on the mechanisms of cystic fibrosis-related hepatobiliary disease [ 113 ]. For the first time, a pioneering study constructed a porcine single-cell atlas database that comprehensively describes the heterogeneity of cells among 20 tissues/organs in pigs [ 114 ], providing a global view of tissue differences between domestic pigs and humans. Rather than focusing on specific cell clusters, Wang et al. [ 114 ] emphasized distinct functions and typical markers of endothelial cells commonly involved in different tissues and suggested that the endothelium may interact with cells through the VEGF, PDGF, TGF-β, and BMP pathways. Microglia have also been noted to be highly conserved across species during evolution [ 114 ], strongly supporting the view of pigs as an invaluable data resource for research on human diseases. In the context of xenotransplantation, pigs are considered the most suitable donors for human organ transplantation. However, the occurrence of rejection has restricted the application of this technique [ 115 ]. The expression patterns of ten genes associated with human immunobiological incompatibility and dysregulation of coagulation have been obtained across different cell types in pigs [ 107 ]. This discovery holds the potential to enhance the immunocompatibility of porcine xenotransplantation in the future through targeted genetic engineering, thereby improving survival after organ transplantation [ 116 ]. However, is important to note that the current study does not adequately address the influence of physiological states and manipulation on the samples. These factors may affect the results by leading to differing numbers of captured cells and altered cell typing. Future research should address this crucial aspect.
In addition to pigs, chickens and rabbits serve as valuable disease models for constructing single-cell reference maps. Differential cellular components can be identified by comparing “disease maps” with “normal maps”, which may in turn predict molecular disease mechanisms. For example, rabbits have been used to study mammalian cardiac contraction, proto-gut embryonic development, proliferative vitreoretinopathy, and hyperlipidaemia-induced spongiosis [ 72 , 117 , 118 , 119 ]. Similarly, chickens have proven valuable for investigating retinal development, hearing damage, and the mechanism underlying melatonin-related weight loss [ 120 , 121 , 122 ]. Among wild animals, antlers, a unique mammalian appendage capable of complete natural regeneration, have been demonstrated to grow similarly to long bones in humans. Leveraging the potential of scRNA-Seq, scientists have revealed key cell types and differentiation trajectories involved in antler regeneration [ 123 , 124 , 125 ], opening new avenues for exploring mammalian organ regeneration and organ damage repair. Future research should address the current limitations in genome annotations for reindeer [ 123 , 124 , 125 ].
Experience in single-cell transcriptomic data analysis
The optimal sample size for single-cell transcriptomic research.
Increases in sample size and sequencing depth could result in the discovery of new and rare cell types, encompassing both previously unrecognized entities and those present in tissues where they have not been detected before [ 126 ]. For instance, among 42,182 cells from three forestomach samples, T helper 17 and epithelial stem and progenitor cells were first identified in dairy cows [ 13 ]. A total of 29,231 individual cells were obtained from three samples of porcine adipose tissue, from which subtypes of cells transformed from endothelial to mesenchymal cells were distinguished [ 114 ]. Compared to studies in livestock, there are more instances of new cell type identification in humans and model organisms, such as a new specialized uroepithelial cell type discovered among 25,307 cells across three bladder samples in humans and mice [ 127 ] and four clusters of nonsensory epithelial cells of the ampulla identified from four stages of mouse crista ampulla samples [ 128 ]. Based on the aforementioned examples of novel cell discoveries, it is evident that employing three or more biological replicates and analysing over 20,000 cells significantly enhances the likelihood of uncovering new cell types. Considering the spatial positioning of tissue sampling and individual differences in cell dissociation, a larger number of biological samples further provides advantages in elucidating the comprehensive distribution of cell types. However, due to the high cost of reagents and sequencing (a total of 10–15 K RMB per sample), excessive sampling may cause more significant input and divert focus from validation experiments. As an illustration, in cell atlas studies, larger biological sample numbers are preferable for identifying cell types at higher resolution [ 21 , 44 , 102 ]. However, if the focus is solely on identifying which cell type is responsive to an experimental treatment, one biological replicate may be effective, as that one cell type typically consists of more than 20 cells/transcriptomes [ 129 , 130 ]. In addition, time-series designs generally involve fewer biological replicates than two-group comparisons [ 36 , 129 ].
With the development of single-cell sequencing technologies, an eight-channel microfluidic system that can capture up to 10,000 cells per channel has been developed, allowing for simultaneous detection of sample cell counts ranging from 50,000 to 800,000 cells in a single run [ 131 ], making a single sample cover a large number of cell/transcriptomic replicates. Bioinformatics tools allow us to enhance and refine the power of single-cell data analysis through the interpretation of transcriptome data. Deconvolution algorithms are now being employed to dissect bulk transcriptomic data to the single-cell level [ 132 ], increasing the statistical power of bulk transcriptomics in single-cell experiments. In addition to deconvolution algorithms, a framework for integrating single-cell RNA sequencing, epigenomic SNP-to-gene maps and genome-wide data enables the identification of target cell types based on the strong statistical power of GWAS data. Currently, emerging large-scale single-cell pre-trained models with tens of millions of cells, such as scGPT, can empower the tasks of our small datasets, including cell classification, network inference, and transcription factor perturbation analysis [ 133 ]. Taken together, researchers need to determine the amount of biological replication that is sufficient to capture biological variability and provide statistically significant results while considering the cost of experiments and the complexity of data analysis. Bulk sequence data and large-scale pre-trained models could be used to enhance the statistical power of single cells.
The number of cell clusters that optimally match the real situation
In brief, the number of cell types is determined by clustering algorithms; specifically, after obtaining single-cell datasets, the cells can be categorized into 2–5 major clusters based on automatic annotation and positional variance in the dimensionality reduction results. With major biological classifications, multiple higher resolutions could be used to identify the more specific cell types corresponding to the basic biological knowledge of a given tissue (Fig. 3 ). As an example, the large intestine comprises diverse epithelial cell types arranged in distinct configurations. Absorptive enterocytes predominate, lining the villi and crypts and specializing in the uptake of water, electrolytes, and nutrients from the luminal contents [ 134 ]. Goblet cells interspersed throughout secrete protective mucus, creating a mucosal barrier against pathogens and toxins [ 135 ]. Enteroendocrine cells release hormones to regulate digestive functions, while Paneth cells, predominantly found in the small intestine but also present in smaller numbers in the large intestine, contribute to innate immunity through the secretion of antimicrobial peptides [ 136 , 137 ]. Mesenchymal cells, immune cells, endothelial cells and neural cells are present in the large intestine. However, the epithelium of the intestine is usually composed of a single layer of columnar epithelial cells, such as those with 1 or 2 clusters, and some cuboidal cells, such as goblet cells, with 2 or 3 clusters [ 21 ]. Different resolution values should be tested to provide the finest demonstration of the known cell types within each tissue. Furthermore, based on the objectives and experimental design, the resolution and number of cell clusters may vary while maintaining biological features. A greater number of clusters is essential for key subcluster identification. For instance, specific macrophage and conventional dendritic cell subsets were identified as key mediators of cellular cross-talk in the colon tumour microenvironment from 54,285 cells divided into 40 clusters [ 138 ].
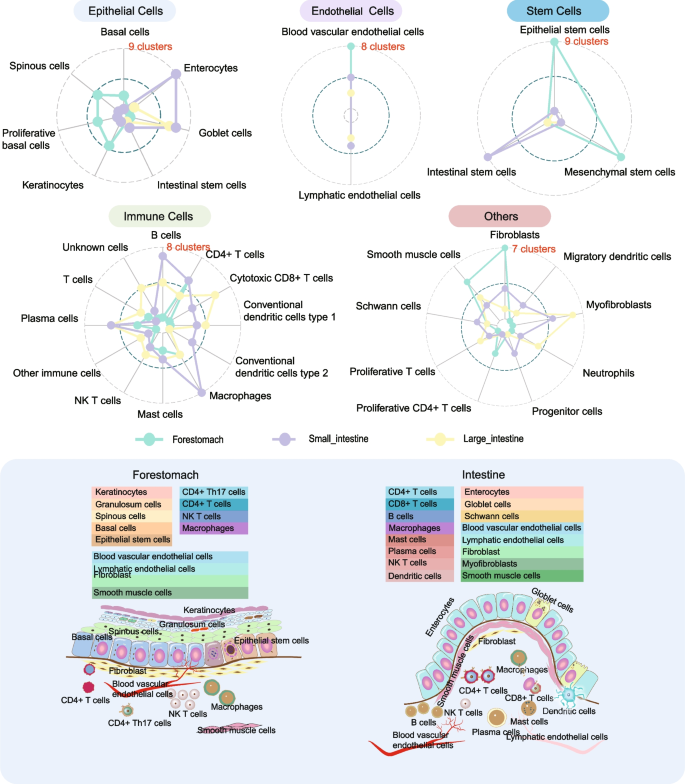
A summary of the common cell types identified in the gastrointestinal tract of livestock
Moreover, clustering algorithms should also be essential for generating the number of cell clusters. First, suitable algorithms should be chosen based on the cell numbers. The K-nearest neighbours algorithm is typically applied to datasets with small cell numbers, with the number of clusters predetermined before clustering. Moreover, the Louvain and Leiden algorithms are normally used for single-cell datasets with more than 100,000 cells, with the number of clusters determined by the resolution set by the analyser [ 139 , 140 ]. The number of clusters can be determined by intra- and intercluster similarity, community detection, eigenvector-based metrics, and stability [ 141 ]. However, these four predominant clustering methods lack systematic evaluation strategies for estimating the number of cell types. Therefore, stability-based approaches for estimating the number of cell types, such as scCCESS, have been proposed [ 141 ], enabling the estimation of the number of cell clusters without the need for researcher observation. Some bioinformatic visualization tools also help us estimate the number of clusters. The Clustree algorithm [ 142 ] employs a bottom-up hierarchical clustering approach for cluster information at different resolutions, which facilitates our selection of a suitable resolution by incorporating biological insights.
A well-formulated strategy for cell type identification
The identification of cell types is a fundamental and essential step in the analysis of single-cell transcriptome data and is accompanied by dimension reduction and clustering [ 143 ]. Generally, the number of cell types and the annotation results should be consistent with the corresponding physiological characteristics.
The common cell types identified in the GIT of livestock are summarized in Fig. 3 . The clusters can be classified into five main cell groups: epithelial cells, endothelial cells, stem cells, immune cells and others. We noticed that the number of clusters for immune cell types in the forestomach is generally lower than that in the intestine, while fibroblasts and muscle cells were more diverse. In the intestine, specifically in the small intestine, B cells and macrophages are more abundant. The cluster numbers correspond to substantial peristalsis and mechanical wear in the foregut, as well as the clear ability of the small intestine to eliminate exogenous invading microorganisms [ 144 ]. Collectively, these cells form a complex biological system responsible for digestion, nutrient absorption, protection against pathogens, and tissue repair and regeneration. Based on the previous cell group summary, we propose three approaches that are beneficial for identifying cell types: (1) collecting biological background information in a given situation; (2) referencing standard cell type names and databases; and (3) assisting in cell type identification with the help of bioinformatic software. It is necessary to summarize basic knowledge in the process of annotating cell types, including biological knowledge and standards/universal naming rules. Regarding how many types of cells should be classified, an effective method is to summarize the physiological characteristics of animal tissues through the accumulation of relevant experimental data and scientific research results [ 143 ]. For example, according to basic knowledge, the cell types present in the epithelial tissue of the rumen of dairy cows consist of four layers of epithelial cells (basal, spiny, granular, and stratum corneum; stratum corneum cells are dead cells and will not be captured), immune cells residing in the tissue, endothelial cells in the connective tissue, smooth muscle cells, and fibroblasts [ 13 ]. Therefore, the presence of these cell types can be used as a reference when determining whether the number of cell types is accurate and whether the current clustering parameters should be adjusted. In animal science research, genes related to animal production or health traits may have corresponding biological significance to cell type. For example, Wu et al. [ 13 ] found a new subcluster of rumen epithelial cells called channel gap-like spinous cells in dairy cows via an in-depth analysis of solute carrier gene expression. Additionally, Wang et al. identified EndMT cells as critical for endothelial-to-mesenchymal transition (EndMT) based on gene expression in stromal and endothelial cells [ 114 ].
To make the cell type identification results more biologically meaningful, the integration of public databases and bioinformatics analysis software should be considered. Public databases of single-cell transcriptome data, such as the Human Cell Atlas, incorporate marker genes for cell type identification and standard or universal cell type nomenclature rules [ 145 ]. In animal science research, the cattle cell atlas constructed by Wu et al. [ 82 ] and the pig cell atlas constructed by Wang et al. [ 114 ] are also of high reference value, although they do not cover all tissue types. A unified system will improve efficiency and applicability for other studies [ 146 ]. Clustree software can be used for determining whether the number of cell types obtained from clustering is appropriate [ 142 ]. Software that automatically annotates cell types can also assist in identifying cell types, e.g., Cellhint [ 146 ], CellTypist [ 147 ], and SingleR [ 148 ]. Software programs for the automated annotation of cell types have been developed based on mathematical models and are specific for annotating certain cell types. For example, Cellhint is used to annotate human cells, and CellTypist is only used to annotate human immune cells. Notably, bioinformatics analysis should be performed manually to ensure the biological significance of the annotated results.
Currently, single-cell transcriptome sequencing technology is cumbersome and costly in terms of experimental steps. Professional experimentation and specialized software support are required for all aspects, from sample preparation to data analysis. Moreover, the bias of cell capture during the sequencing process may lead to inaccurate results, which is mainly attributed to the possibility of missing or detecting specific types of cells at low frequencies. Moreover, bioinformatics tools and algorithms are still suboptimal, making it challenging to extract meaningful information from the massive amount of data. In the future, it will be necessary to simplify and optimize the process of single-cell technology to reduce its cost and operational difficulty and to make it easier to apply in different fields. The accuracy and efficiency of cell capture techniques should be improved to reflect the diversity of cellular communities. In the meantime, techniques for cell capture and mRNA enrichment in prokaryotes are still under development. The implementation of microbiome single-cell transcriptome technology will substantially broaden the application landscape of single-cell technology [ 149 ].
One of the greatest limitations of scRNA-Seq is the loss of spatial information due to tissue dissociation. Spatial transcriptomics is a more recently developed methodology that allows for the localization and construction of a cellular expression map with a spatial dimension, which is not achievable with scRNA-Seq. Coupled with scRNA-Seq, this approach can stereoscopically demonstrate the heterogeneous distribution and functional localization of individual cells and reveal spatial differences in cells during evolutionary development or disease onset. However, the application of this approach to domestic animals is still relatively rare. Only studies on the spatiotemporal transcriptional profiling of chicken heart development have been reported, but have revealed the pathways through which cardiac cell differentiation and morphological changes occur at the same time as spatially restricted regulatory programmes [ 150 ]. The complexity of life activities is difficult to determine by single-modality omics methods; therefore, single-cell multi-omics technologies, including single-cell transcriptome as the core analysis combined with genome, proteome and metabolome analyses, are inevitable [ 151 ] and will be able to deepen the understanding of cell type and state in greater dimension. For example, the dynamic changes of single-cell RNA/ATAC sequencing in porcine embryonic skeletal muscle were consistent with the activity of different cell type-specific transcription factors, which helped identify key regulators of muscle formation after integrative analysis [ 152 ].
Sequencing of certain animals or organs can be challenging due to their limited availability, difficulty in manipulation, or ethical concerns. Organoids, 3D cultures developed from stem cells that closely resemble the source tissue [ 153 ], are valuable in vitro tools for organoid single-cell transcriptomics in such cases. Integrating organoids with scRNA-Seq can allow more precise comparisons between organoids and source tissues in terms of cell types and gene expression patterns; moreover, through real-time monitoring of transcriptome changes in single cells of organoids cultured under different treatments, it is possible to provide potential cellular and molecular phenotypic information on complex traits, such as feed efficiency and disease resistance. Organoid models have been constructed for various livestock species [ 154 , 155 , 156 ] and are mainly used to study organ development, host–microbe interactions, cellular nutrient metabolism mechanisms, and drug toxicity. Zhang et al. [ 83 ] utilized a significant quantity of butyric acid to stimulate rumen organoids, and the organoids showed noticeable keratinization and significant increases in the expression levels of related keratinization genes. These findings were confirmed by single-cell sequencing, demonstrating the potential for combining single-cell transcriptomes and organoids for the study of biological mechanisms.
Single-cell transcriptomics reveals the role of different cells in organisms. However, it is important to recognize that in multicellular organisms, the functionality of the organism is dependent on synergistic interactions between different cells. Thus, single-cell transcriptome-based research should consider the relationships between cell lineages and the interactions between cells. This might also prevent an overemphasis on the functions of individual cells and might rather ensure that the influence of the hostile environment on cell development and function is not ignored. Using a multidimensional approach to single-cell transcriptome studies, such as cell communication analysis, the interaction network between cells can be reconstructed and predicted, revealing signalling and regulatory mechanisms and providing a more accurate reference for studying overall organism and tissue function. It is also possible to validate the accuracy of single-cell sequencing data through various biological experimental means, ensuring a more reliable assessment of research results.
Conclusions
Single-cell transcriptomics has enabled the analysis of heterogeneity in gene expression in tissues and organs at the single-cell level. This advancement has provided a robust framework for identifying cell types, discovering rare cell populations, screening marker genes, exploring cellular developmental trajectories, and analysing cellular functions. ScRNA-Seq has also been extensively applied in animal science, particularly in studies involving domestic species of high economic importance. Using scRNA-Seq, animal researchers have investigated topics such as nutritional regulation, metabolic mechanisms, spermatogenesis, embryonic development, genetic breeding, and disease mechanisms in livestock. Through the construction of single-cell atlases for different animal organs, researchers have revealed the intricate cellular heterogeneity and gene expression variability present at the individual, organ, and tissue levels. This approach has facilitated deeper mechanistic investigations at the molecular level, shedding light on the underlying genetic basis of important traits and disease pathogenesis. Consequently, scRNA-Seq has made substantial and significant contributions to advancing our understanding of animal biology and is ultimately poised to enhance the quality of livestock products to meet increasing consumer demands. When analysing single-cell transcriptomic data, the optimal sample size should be determined based on the biological variation, statistical significance, cost and complexity of the data analysis. Accurate cell clustering and cell type annotation should consider background knowledge, as well as appropriate and stable algorithms. Currently, scRNA-Seq still faces several great challenges, ranging from sample processing to data analysis, and the integration of novel experimental methodologies and sequencing technologies is needed to probe and elucidate the intricate regulatory networks and causal relationships among diverse biomolecules in a multidimensional manner. Through continued innovation and interdisciplinary collaboration, single-cell transcriptomics holds the promise of unlocking new frontiers in animal research and fostering sustainable advancements in agricultural productivity and animal health.
Availability of data and materials
Not applicable.
Abbreviations
Channel-gap-like
Endothelial-to-mesenchymal transition
Gastrointestinal tract
Peripheral blood mononuclear cells
RNA-Sequencing
Short-chain fatty acid
- Single-cell RNA sequencing
Otto O, Rosendahl P, Mietke A, Golfier S, Herold C, Klaue D, et al. Real-time deformability cytometry: On-the-fly cell mechanical phenotyping. Nat Methods. 2015;12(3):199–202. https://doi.org/10.1038/nmeth.3281 .
Article CAS PubMed Google Scholar
Brady G, Barbara M, Iscove NN. Representative in vitro cDNA amplification from individual hemopoietic cells and colonies. Methods Mol Cell Biol. 1990;2:17–25. https://wwwlabs.uhnresearch.ca/labs/iscove/MMCB90.pdf .
Maxam AM, Gilbert WA. A new method for sequencing DNA. Proc Natl Acad Sci. 1977;74(2):560–4. https://doi.org/10.1073/pnas.74.2.560 .
Article CAS PubMed PubMed Central Google Scholar
Sanger F, Coulson AR. A rapid method for determining sequences in DNA by primed synthesis with DNA polymerase. J Mol Biol. 1975;94(3):441–8. https://doi.org/10.1016/0022-2836(75)90213-2 .
Stark R, Grzelak M, Hadfield J. RNA sequencing: The teenage years. Nat Rev Genet. 2019;20(11):631–56. https://doi.org/10.1038/s41576-019-0150-2 .
Tang F, Barbacioru C, Wang Y, Nordman E, Lee C, Xu N, et al. mRNA-Seq whole-transcriptome analysis of a single cell. Nat Methods. 2009;6(5):377–82. https://doi.org/10.1038/nmeth.1315 .
Potter SS. Single-cell RNA sequencing for the study of development, physiology and disease. Nat Rev Nephrol. 2018;14(8):479–92. https://doi.org/10.1038/s41581-018-0021-7 .
Luecken MD, Theis FJ. Current best practices in single-cell RNA-seq analysis: a tutorial. Mol Syst Biol. 2019;15(6):e8746. https://doi.org/10.15252/msb.20188746 .
Article PubMed PubMed Central Google Scholar
Bakken TE, Hodge RD, Miller JA, Yao Z, Nguyen TN, Aevermann B, et al. Single-nucleus and single-cell transcriptomes compared in matched cortical cell types. PLoS ONE. 2018;13(12):e209648. https://doi.org/10.1371/journal.pone.0209648 .
Article Google Scholar
Wu H, Kirita Y, Donnelly EL, Humphreys BD. Advantages of single-nucleus over single-cell RNA sequencing of adult kidney: Rare cell types and novel cell states revealed in fibrosis. J Am Soc Nephrol. 2019;30(1):23–32. https://doi.org/10.1681/ASN.2018090912 .
Flint HJ, Bayer EA. Plant cell wall breakdown by anaerobic microorganisms from the mammalian digestive tract. Ann N Y Acad Sci. 2008;1125:280–8. https://doi.org/10.1196/annals.1419.022 .
Yuan Y, Sun DM, Qin T, Mao SY, Zhu WY, Yin YY, et al. Single-cell transcriptomic landscape of the sheep rumen provides insights into physiological programming development and adaptation of digestive strategies. Zool Res. 2022;43(4):634–47. https://doi.org/10.24272/j.issn.2095-8137.2022.086 .
Wu JJ, Zhu S, Gu F, Valencak TG, Liu JX, Sun HZ. Cross-tissue single-cell transcriptomic landscape reveals the key cell subtypes and their potential roles in the nutrient absorption and metabolism in dairy cattle. J Adv Res. 2021;37:1–18. https://doi.org/10.1016/j.jare.2021.11.009 .
Xue MY, Wu JJ, Xie YY, Zhu SL, Zhong YF, Liu JX, et al. Investigation of fiber utilization in the rumen of dairy cows based on metagenome-assembled genomes and single-cell RNA sequencing. Microbiome. 2022;10:11. https://doi.org/10.1186/s40168-021-01211-w .
Li H, Wang X, Wang Y, Zhang M, Hong F, Wang H, et al. Cross-species single-cell transcriptomic analysis reveals divergence of cell composition and functions in mammalian ileum epithelium. Cell Regen. 2022;11:19. https://doi.org/10.1186/s13619-022-00118-7 .
Wiarda JE, Becker SR, Sivasankaran SK, Loving CL. Regional epithelial cell diversity in the small intestine of pigs. J Anim Sci. 2023;101:skac318. https://doi.org/10.1093/jas/skac318 .
Article PubMed Google Scholar
Meng Q, Chen L, Xiong B, Kang B, Zhang P, Tang S, et al. Single-cell transcriptome sequencing and proteomics reveal neonatal ileum dynamic developmental potentials. mSystems. 2021;6(5):e0072521. https://doi.org/10.1128/mSystems.00725-21 .
Gu W, Madrid DMC, Joyce S, Driver JP. A single-cell analysis of thymopoiesis and thymic iNKT cell development in pigs. Cell Rep. 2022;40:111050. https://doi.org/10.1016/j.celrep.2022.111050 .
Shah AU, Li Y, Ouyang W, Wang Z, Zuo J, Shi S, et al. From nasal to basal: Single-cell sequencing of the bursa of Fabricius highlights the IBDV infection mechanism in chickens. Cell Biosci. 2021;11:212. https://doi.org/10.1186/s13578-021-00728-9 .
Wiarda JE, Trachsel JM, Sivasankaran SK, Tuggle CK, Loving CL. Intestinal single-cell atlas reveals novel lymphocytes in pigs with similarities to human cells. Life Sci Alliance. 2022;5(10):e202201442. https://doi.org/10.26508/lsa.202201442 .
Chen D, Sun J, Zhu J, Ding X, Lan T, Wang X, et al. Single cell atlas for 11 non-model mammals, reptiles and birds. Nat Commun. 2021;12:7083. https://doi.org/10.1038/s41467-021-27162-2 .
Gao Y, Li J, Cai G, Wang Y, Yang W, Li Y, et al. Single-cell transcriptomic and chromatin accessibility analyses of dairy cattle peripheral blood mononuclear cells and their responses to lipopolysaccharide. BMC Genomics. 2022;23:338. https://doi.org/10.1186/s12864-022-08562-0 .
Herrera-Uribe J, Wiarda JE, Sivasankaran SK, Daharsh L, Liu H, Byrne KA, et al. Reference transcriptomes of porcine peripheral immune cells created through bulk and single-cell RNA sequencing. Front Genet. 2021;12:689406. https://doi.org/10.3389/fgene.2021.689406 .
Jiao A, Zhang C, Wang X, Sun L, Liu H, Su Y, et al. Single-cell sequencing reveals the evolution of immune molecules across multiple vertebrate species. J Adv Res. 2024;55:73–87. https://doi.org/10.1016/j.jare.2023.02.017 .
Kotliar D, Lin AE, Logue J, Hughes TK, Khoury NM, Raju SS, et al. Single-Cell profiling of ebola virus disease in vivo reveals viral and host dynamics. Cell. 2020;183(5):1383–401. https://doi.org/10.1016/j.cell.2020.10.002 .
Li Z, Sun C, Wang F, Wang X, Zhu J, Luo L, et al. Molecular mechanisms governing circulating immune cell heterogeneity across different species revealed by single-cell sequencing. Clin Transl Med. 2022;12:e689. https://doi.org/10.1002/ctm2.689 .
Li J, Xu Y, Zhang J, Zhang Z, Guo H, Wei D, et al. Single-cell transcriptomic analysis reveals transcriptional and cell subpopulation differences between human and pig immune cells. Genes Genomics. 2024;46(3):303–22. https://doi.org/10.1007/s13258-023-01456-9 .
Patel RS, Tomlinson JE, Divers TJ, Van de Walle GR, Rosenberg BR. Single-cell resolution landscape of equine peripheral blood mononuclear cells reveals diverse cell types including T-bet + B cells. BMC Biol. 2021;19:13. https://doi.org/10.1186/s12915-020-00947-5 .
Tang Q, Iyer S, Lobbardi R, Moore JC, Chen H, Lareau C, et al. Dissecting hematopoietic and renal cell heterogeneity in adult zebrafish at single-cell resolution using RNA sequencing. J Exp Med. 2017;214(10):2875–87. https://doi.org/10.1084/jem.20170976 .
Winkler ES, Shrihari S, Hykes BJ, Handley SA, Andhey PS, Huang YS, et al. The intestinal microbiome restricts alphavirus infection and dissemination through a bile acid-type I IFN signaling axis. Cell. 2020;182(4):901–18. https://doi.org/10.1016/j.cell.2020.06.029 .
Zhu L, Yang P, Zhao Y, Zhuang Z, Wang Z, Song R, et al. Single-cell sequencing of peripheral mononuclear cells reveals distinct immune response landscapes of COVID-19 and influenza patients. Immunity. 2020;53(3):685–96. https://doi.org/10.1016/j.immuni.2020.07.009 .
Stubbington M, Rozenblatt-Rosen O, Regev A, Teichmann SA. Single-cell transcriptomics to explore the immune system in health and disease. Science. 2017;358(6359):58–63. https://doi.org/10.1126/science.aan6828 .
Dai M, Zhu S, An Z, You B, Li Z, Yao Y, et al. Dissection of key factors correlating with H5N1 avian influenza virus driven inflammatory lung injury of chicken identified by single-cell analysis. PLoS Pathog. 2023;19(10):e1011685. https://doi.org/10.1371/journal.ppat.1011685 .
Dai M, Zhao L, Li Z, Li X, You B, Zhu S, et al. The transcriptional differences of avian CD4 + CD8 + double-positive T cells and CD8 + T cells from peripheral blood of ALV-J infected chickens revealed by Smart-Seq2. Front Cell Infect Microbiol. 2021;11:747094. https://doi.org/10.3389/fcimb.2021.747094 .
Qu X, Li X, Li Z, Liao M, Dai M. Chicken peripheral blood mononuclear cells response to avian leukosis virus subgroup j infection assessed by single-cell RNA sequencing. Front Microbiol. 2022;13:800618. https://doi.org/10.3389/fmicb.2022.800618 .
Gu F, Zhu S, Tang Y, Liu X, Jia M, Malmuthuge N, et al. Gut microbiome is linked to functions of peripheral immune cells in transition cows during excessive lipolysis. Microbiome. 2023;11:40. https://doi.org/10.1186/s40168-023-01492-3 .
Tang W, Zhong Y, Wei Y, Deng Z, Mao J, Liu J, et al. Ileum tissue single-cell mRNA sequencing elucidates the cellular architecture of pathophysiological changes associated with weaning in piglets. BMC Biol. 2022;20:123. https://doi.org/10.1186/s12915-022-01321-3 .
Zheng Y, Li S, Li SH, Yu S, Wang Q, Zhang K, et al. Transcriptome profiling in swine macrophages infected with African swine fever virus at single-cell resolution. Proc Natl Acad Sci U S A. 2022;119(19):e2201288119. https://doi.org/10.1073/pnas.2201288119 .
Huang L, Zhang J, Zhang P, Huang X, Yang W, Liu R, et al. Single-cell RNA sequencing uncovers dynamic roadmap and cell-cell communication during buffalo spermatogenesis. iScience. 2022;26(1):105733. https://doi.org/10.1016/j.isci.2022.105733 .
Tian Y, Sun P, Liu WX, Shan LY, Hu YT, Fan HT, et al. Single-cell RNA sequencing of the Mongolia sheep testis reveals a conserved and divergent transcriptome landscape of mammalian spermatogenesis. FASEB J. 2022;36(6):e22348. https://doi.org/10.1096/fj.202200152R .
Lingkai Z, Ming G, Zidong L, Ruifang L, Yi Z, Taiyong Y, et al. Single-cell RNA-seq analysis of testicular somatic cell development in pigs. J Genet Genomics. 2022;49(11):1016–28. https://doi.org/10.1016/j.jgg.2022.03.014 .
Article CAS Google Scholar
Wang X, Pei J, Xiong L, Guo S, Cao M, Kang Y, et al. Single-cell RNA sequencing reveals atlas of yak testis cells. Int J Mol Sci. 2023;24(9):7982. https://doi.org/10.3390/ijms24097982 .
Wu Y, Guo T, Li J, Niu C, Sun W, Zhu S, et al. The transcriptional cell atlas of testis development in sheep at pre-sexual maturity. Curr Issues Mol Biol. 2022;44(2):483–97. https://doi.org/10.3390/cimb44020033 .
Yang H, Ma J, Wan Z, Wang Q, Wang Z, Zhao J, et al. Characterization of sheep spermatogenesis through single-cell RNA sequencing. FASEB J. 2021;35(2):e21187. https://doi.org/10.1096/fj.202001035RRR .
Yu XW, Li TT, Du XM, Shen QY, Zhang MF, Wei YD, et al. Single-cell RNA sequencing reveals atlas of dairy goat testis cells. Zool Res. 2021;42(4):401–5. https://doi.org/10.24272/j.issn.2095-8137.2020.373 .
Zhao Y, Yang C, Du Z. Integrated single cell transcriptome sequencing analysis reveals species-specific genes and molecular pathways for pig spermiogenesis. Reprod Domest Anim. 2023;58(12):1745–55. https://doi.org/10.1111/rda.14493 .
Zhang LK, Ma HD, Guo M, Wang L, Zheng Y, Wu XD, et al. Dynamic transcriptional atlas of male germ cells during porcine puberty. Zool Res. 2022;43(4):600–3. https://doi.org/10.24272/j.issn.2095-8137.2022.037 .
Li D, Ning C, Zhang J, Wang Y, Tang Q, Kui H, et al. Dynamic transcriptome and chromatin architecture in granulosa cells during chicken folliculogenesis. Nat Commun. 2022;13:131. https://doi.org/10.1038/s41467-021-27800-9 .
Ge T, Wen Y, Li B, Huang X, Jiang S, Zhang E. Single-cell sequencing reveals the reproductive variations between primiparous and multiparous Hu ewes. J Anim Sci Biotechnol. 2023;14:144. https://doi.org/10.1186/s40104-023-00941-1 .
Li Z, Wang J, Zhao Y, Ma D, Zhao M, Li N, et al. ScRNA-seq of ovarian follicle granulosa cells from different fertility goats reveals distinct expression patterns. Reprod Domest Anim. 2021;56(5):801–11. https://doi.org/10.1111/rda.13920 .
Li Z, Song X, Yin S, Yan J, Lv P, Shan H, et al. Single-cell RNA-Seq revealed the gene expression pattern during the in vitro maturation of donkey oocytes. Genes (Basel). 2021;12(10):1640. https://doi.org/10.3390/genes12101640 .
Liu Z, Zhang G, Deng M, Yang H, Pang J, Cai Y, et al. Inhibition of lysine-specific histone demethylase 1A results in meiotic aberration during oocyte maturation in vitro in goats. Theriogenology. 2020;143:168–78. https://doi.org/10.1016/j.theriogenology.2019.12.011 .
Chen N, Zhang Y, Tian Y, Wu S, Gao F, Yuan X. Deciphering cellular heterogeneity and communication patterns in porcine antral follicles by single-cell RNA sequencing. Animals (Basel). 2023;13(19):3019. https://doi.org/10.3390/ani13193019 .
Ghafouri F, Sadeghi M, Bahrami A, Naserkheil M, Dehghanian RV, Javanmard A, et al. Construction of a circRNA- lincRNA-lncRNA-miRNA-mRNA ceRNA regulatory network identifies genes and pathways linked to goat fertility. Front Genet. 2023;14:1195480. https://doi.org/10.3389/fgene.2023.1195480 .
Pei J, Xiong L, Guo S, Wang X, La Y, Chu M, et al. Single-cell transcriptomics analysis reveals a cell atlas and cell communication in yak ovary. Int J Mol Sci. 2023;24(3):1839. https://doi.org/10.3390/ijms24031839 .
Pei J, Xiong L, Guo S, Wang X, Bao P, Wu X, et al. A single-cell transcriptomic atlas characterizes cell types and their molecular features in yak ovarian cortex. FASEB J. 2023;37(1):e22718. https://doi.org/10.1096/fj.202201176RR .
Yang CX, Song ZQ, Pei S, Yu XX, Miao JK, Liang H, et al. Single cell RNA-seq reveals molecular pathways altered by 7, 12-dimethylbenz[a]anthracene treatment on pig oocytes. Theriogenology. 2020;157:449–57. https://doi.org/10.1016/j.theriogenology.2020.08.020 .
Mipam T, Chen X, Zhao W, Zhang P, Chai Z, Yue B, et al. Single-cell transcriptome analysis and in vitro differentiation of testicular cells reveal novel insights into male sterility of the interspecific hybrid cattle-yak. BMC Genomics. 2023;24:149. https://doi.org/10.1186/s12864-023-09251-2 .
Zhang T, Sun P, Geng Q, Fan H, Gong Y, Hu Y, et al. Disrupted spermatogenesis in a metabolic syndrome model: The role of vitamin A metabolism in the gut-testis axis. Gut. 2022;71(1):78–87. https://doi.org/10.1136/gutjnl-2020-323347 .
Chen M, Long X, Hao F, Kang J, Wang N, Wang Y, et al. Integration of single-cell transcriptome and chromatin accessibility of early gonads development among goats, pigs, macaques, and humans. Cell Rep. 2022;41(5):111587. https://doi.org/10.1016/j.celrep.2022.111587 .
Soto DA, Ross PJ. Similarities between bovine and human germline development revealed by single-cell RNA sequencing. Reproduction. 2021;161(3):239–53. https://doi.org/10.1530/REP-20-0313 .
Jung KM, Seo M, Han JY. Comparative single-cell transcriptomic analysis reveals differences in signaling pathways in gonadal primordial germ cells between chicken ( Gallus gallus ) and zebra finch ( Taeniopygia guttata ). FASEB J. 2023;37(1):e22706. https://doi.org/10.1096/fj.202201569R .
Estermann MA, Williams S, Hirst CE, Roly ZY, Serralbo O, Adhikari D, et al. Insights into gonadal sex differentiation provided by Single-Cell transcriptomics in the chicken embryo. Cell Rep. 2020;31:107491. https://doi.org/10.1016/j.celrep.2020.03.055 .
Tesfaye D, Gebremedhn S, Salilew-Wondim D, Hailay T, Hoelker M, Grosse-Brinkhaus C, et al. MicroRNAs: tiny molecules with a significant role in mammalian follicular and oocyte development. Reproduction. 2018;155(3):R121–35. https://doi.org/10.1530/REP-17-0428 .
Gong X, Zhang Y, Ai J, Li K. Application of Single-Cell RNA sequencing in ovarian development. Biomolecules. 2022;13(1):47. https://doi.org/10.3390/biom13010047 .
Davenport KM, O’Neil EV, Ortega MS, Patterson A, Kelleher AM, Warren WC, et al. Single cell insights into development of the bovine placenta. Biol Reprod. 2024;110(1):169–84. https://doi.org/10.1093/biolre/ioad123 .
Davenport KM, Ortega MS, Liu H, O’Neil EV, Kelleher AM, Warren WC, et al. Single-nuclei RNA sequencing (snRNA-seq) uncovers trophoblast cell types and lineages in the mature bovine placenta. Proc Natl Acad Sci U S A. 2023;120(12):e2221526120. https://doi.org/10.1073/pnas.2221526120 .
Di Fang, Tao W, Wang J, Huang F, Gao Q. Transcriptome analysis of sheep embryos in vivo based on single cell rna-seq. Acta Medica Mediterr. 2022;38(2):1263–72. https://doi.org/10.19193/0393-6384_2022_2_192 .
Guillot C, Djeffal Y, Michaut A, Rabe B, Pourquie O. Dynamics of primitive streak regression controls the fate of neuromesodermal progenitors in the chicken embryo. Elife. 2021;10:e64819. https://doi.org/10.7554/eLife.64819 .
Jia GX, Ma WJ, Wu ZB, Li S, Zhang XQ, He Z, et al. Single-cell transcriptomic characterization of sheep conceptus elongation and implantation. Cell Rep. 2023;42(8):112860. https://doi.org/10.1016/j.celrep.2023.112860 .
Liu T, Li J, Yu L, Sun HX, Li J, Dong G, et al. Cross-species single-cell transcriptomic analysis reveals pre-gastrulation developmental differences among pigs, monkeys, and humans. Cell Discov. 2021;7:8. https://doi.org/10.1038/s41421-020-00238-x .
Ton MN, Keitley D, Theeuwes B, Guibentif C, Ahnfelt-Ronne J, Andreassen TK, et al. An atlas of rabbit development as a model for single-cell comparative genomics. Nat Cell Biol. 2023;25(7):1061–72. https://doi.org/10.1038/s41556-023-01174-0 .
Vermillion KL, Bacher R, Tannenbaum AP, Swanson S, Jiang P, Chu LF, et al. Spatial patterns of gene expression are unveiled in the chick primitive streak by ordering single-cell transcriptomes. Dev Biol. 2018;439(1):30–41. https://doi.org/10.1016/j.ydbio.2018.04.007 .
Williams RM, Lukoseviciute M, Sauka-Spengler T, Bronner ME. Single-cell atlas of early chick development reveals gradual segregation of neural crest lineage from the neural plate border during neurulation. Elife. 2022;11:e74464. https://doi.org/10.7554/eLife.74464 .
Zhao L, Long C, Zhao G, Su J, Ren J, Sun W, et al. Reprogramming barriers in bovine cells nuclear transfer revealed by single-cell RNA-seq analysis. J Cell Mol Med. 2022;26(18):4792–804. https://doi.org/10.1111/jcmm.17505 .
Li J, Wang L, Yu D, Hao J, Zhang L, Adeola AC, et al. Single-cell RNA sequencing reveals thoracolumbar vertebra heterogeneity and rib-genesis in pigs. Genomics Proteomics Bioinformatics. 2021;19(3):423–36. https://doi.org/10.1016/j.gpb.2021.09.008 .
Kim DW, Place E, Chinnaiya K, Manning E, Sun C, Dai W, et al. Single-cell analysis of early chick hypothalamic development reveals that hypothalamic cells are induced from prethalamic-like progenitors. Cell Rep. 2022;38(3):110251. https://doi.org/10.1016/j.celrep.2021.110251 .
Zeller R, Lopez-Rios J, Zuniga A. Vertebrate limb bud development: Moving towards integrative analysis of organogenesis. Nat Rev Genet. 2009;10(12):845–58. https://doi.org/10.1038/nrg2681 .
Esteves DLJ, Blavet C, Bonnin MA, Hirsinger E, Havis E, Relaix F, et al. TMEM8C-mediated fusion is regionalized and regulated by NOTCH signalling during foetal myogenesis. Development. 2022;149(2):dev199928. https://doi.org/10.1242/dev.199928 .
Esteves DLJ, Blavet C, Bonnin MA, Hirsinger E, Comai G, Yvernogeau L, et al. Unexpected contribution of fibroblasts to muscle lineage as a mechanism for limb muscle patterning. Nat Commun. 2021;12(1):3851. https://doi.org/10.1038/s41467-021-24157-x .
Wu JJ, Zhu S, Tang YF, Gu F, Liu JX, Sun HZ. Microbiota-host crosstalk in the newborn and adult rumen at single-cell resolution. BMC Biol. 2022;20:280. https://doi.org/10.1186/s12915-022-01490-1 .
Wu J, Zhu S, Tang Y, Gu F, Valencak TG, Liu J, et al. Age- and microbiota-dependent cell stemness plasticity revealed by cattle cell landscape. Research (Washington). 2023;6:0025. https://doi.org/10.34133/research.0025 .
Zhang K, Zhang Y, Qin J, Zhu H, Liu N, Sun D, et al. Early concentrate starter introduction induces rumen epithelial parakeratosis by blocking keratinocyte differentiation with excessive ruminal butyrate accumulation. J Adv Res. 2023;S2090–1232(23):00401. https://doi.org/10.1016/j.jare.2023.12.016 .
Lin S, Fang L, Kang X, Liu S, Liu M, Connor EE, et al. Establishment and transcriptomic analyses of a cattle rumen epithelial primary cells (REPC) culture by bulk and single-cell RNA sequencing to elucidate interactions of butyrate and rumen development. Heliyon. 2020;6(6):e04112. https://doi.org/10.1016/j.heliyon.2020.e04112 .
Gao Y, Fang L, Baldwin RT, Connor EE, Cole JB, Van Tassell CP, et al. Single-cell transcriptomic analyses of dairy cattle ruminal epithelial cells during weaning. Genomics. 2021;113(4):2045–55. https://doi.org/10.1016/j.ygeno.2021.04.039 .
Gao X, Wang S, Wang Y, Li S, Wu S, Yan R, et al. Long read genome assemblies complemented by single cell RNA-sequencing reveal genetic and cellular mechanisms underlying the adaptive evolution of yak. Nat Commun. 2022;13:4887. https://doi.org/10.1038/s41467-022-32164-9 .
Han X, Zhou Z, Fei L, Sun H, Wang R, Chen Y, et al. Construction of a human cell landscape at single-cell level. Nature. 2020;581(7808):303–9. https://doi.org/10.1038/s41586-020-2157-4 .
Han L, Wei X, Liu C, Volpe G, Zhuang Z, Zou X, et al. Cell transcriptomic atlas of the non-human primate Macaca fascicularis. Nature. 2022;604(7907):723–31. https://doi.org/10.1038/s41586-022-04587-3 .
Han X, Wang R, Zhou Y, Fei L, Sun H, Lai S, et al. Mapping the mouse cell atlas by Microwell-seq. Cell. 2018;172(5):1307. https://doi.org/10.1016/j.cell.2018.05.012 .
Liao Y, Ma L, Guo Q, Weigao E, Fang X, Yang L, et al. Cell landscape of larval and adult Xenopus laevis at single-cell resolution. Nat Commun. 2022;13:4306. https://doi.org/10.1038/s41467-022-31949-2 .
Li H, Janssens J, De Waegeneer M, Kolluru SS, Davie K, Gardeux V, et al. Fly Cell Atlas : a single-nucleus transcriptomic atlas of the adult fruit fly. Science. 2022;375(6584):eabk2432. https://doi.org/10.1126/science.abk2432 .
Ye F, Wang J, Li J, Mei Y, Guo G. Mapping cell atlases at the single-cell level. Adv Sci (Weinh). 2024;11(8):e2305449. https://doi.org/10.1002/advs.202305449 .
Lyu P, Qi Y, Tu ZJ, Jiang H. Single-cell RNA sequencing reveals heterogeneity of cultured bovine satellite cells. Front Genet. 2021;12:742077. https://doi.org/10.3389/fgene.2021.742077 .
Li J, Xing S, Zhao G, Zheng M, Yang X, Sun J, et al. Identification of diverse cell populations in skeletal muscles and biomarkers for intramuscular fat of chicken by single-cell RNA sequencing. BMC Genomics. 2020;21:752. https://doi.org/10.1186/s12864-020-07136-2 .
Zhang X, Yao Y, Han J, Yang Y, Chen Y, Tang Z, et al. Longitudinal epitranscriptome profiling reveals the crucial role of N 6 -methyladenosine methylation in porcine prenatal skeletal muscle development. J Genet Genomics. 2020;47(8):466–76. https://doi.org/10.1016/j.jgg.2020.07.003 .
Qiu K, Xu D, Wang L, Zhang X, Jiao N, Gong L, et al. Association analysis of single-cell RNA sequencing and proteomics reveals a vital role of Ca 2+ signaling in the determination of skeletal muscle development potential. Cells. 2020;9(4):1045. https://doi.org/10.3390/cells9041045 .
Du X, Lai S, Zhao W, Xu X, Xu W, Zeng T, et al. Single-cell RNA sequencing revealed the liver heterogeneity between egg-laying duck and ceased-laying duck. BMC Genomics. 2022;23:857. https://doi.org/10.1186/s12864-022-09089-0 .
Schlake T. Determination of hair structure and shape. Semin Cell Dev Biol. 2007;18(2):267–73. https://doi.org/10.1016/j.semcdb.2007.01.005 .
Ge W, Zhang W, Zhang Y, Zheng Y, Li F, Wang S, et al. A single-cell transcriptome atlas of cashmere goat hair follicle morphogenesis. Genomics Proteomics Bioinformatics. 2021;19(3):437–51. https://doi.org/10.1016/j.gpb.2021.07.003 .
Ma Y, Zeng W, Ba Y, Luo Q, Ou Y, Liu R, et al. A single-cell transcriptomic atlas characterizes the silk-producing organ in the silkworm. Nat Commun. 2022;13:3316. https://doi.org/10.1038/s41467-022-31003-1 .
Zheng Q, Ye N, Bao P, Zhang X, Wang F, Ma L, et al. Construction of transcriptome atlas of white yak hair follicle during anagen and catagen using single-cell RNA sequencing. BMC Genomics. 2022;23:813. https://doi.org/10.1186/s12864-022-09003-8 .
Yang F, Li R, Zhao C, Che T, Guo J, Xie Y, et al. Single-cell sequencing reveals the new existence form of dermal papilla cells in the hair follicle regeneration of cashmere goats. Genomics. 2022;114(2):110316. https://doi.org/10.1016/j.ygeno.2022.110316 .
Wang Z, Wang Y, Hui T, Chen R, Xu Y, Zhang Y, et al. Single-cell sequencing reveals differential cell types in skin tissues of liaoning cashmere goats and key genes related potentially to the fineness of cashmere fiber. Front Genet. 2021;12:726670. https://doi.org/10.3389/fgene.2021.726670 .
Chiappalupi S, Salvadori L, Luca G, Riuzzi F, Calafiore R, Donato R, et al. Do porcine Sertoli cells represent an opportunity for Duchenne muscular dystrophy? Cell Prolif. 2019;52(3):e12599. https://doi.org/10.1111/cpr.12599 .
Lunney JK, Van Goor A, Walker KE, Hailstock T, Franklin J, Dai C. Importance of the pig as a human biomedical model. Sci Transl Med. 2021;13(621):eabd5758. https://doi.org/10.1126/scitranslmed.abd5758 .
Ekser B, Ezzelarab M, Hara H, van der Windt DJ, Wijkstrom M, Bottino R, et al. Clinical xenotransplantation: the next medical revolution? Lancet. 2012;379(9816):672–83. https://doi.org/10.1016/S0140-6736(11)61091-X .
Zhang L, Zhu J, Wang H, Xia J, Liu P, Chen F, et al. A high-resolution cell atlas of the domestic pig lung and an online platform for exploring lung single-cell data. J Genet Genomics. 2021;48(5):411–25. https://doi.org/10.1016/j.jgg.2021.03.012 .
Shetty A, Lim S, Strell P, Steer CJ, Rivera-Mulia JC, Low WC. In Silico stage-matching of human, marmoset, mouse, and pig embryos to enhance organ development through interspecies chimerism. Cell Transplant. 2023;32:9636897231158728. https://doi.org/10.1177/09636897231158728 .
Voigt AL, Dardari R, Su L, Lara NLM, Sinha S, Jaffer A, et al. Metabolic transitions define spermatogonial stem cell maturation. Hum Reprod. 2022;37(9):2095–112. https://doi.org/10.1093/humrep/deac157 .
Wang X, Hong F, Li H, Wang Y, Zhang M, Lin S, et al. Cross-species single-cell transcriptomic analysis of animal gastric antrum reveals intense porcine mucosal immunity. Cell Regen. 2023;12:27. https://doi.org/10.1186/s13619-023-00171-w .
Zhu J, Chen F, Luo L, Wu W, Dai J, Zhong J, et al. Single-cell atlas of domestic pig cerebral cortex and hypothalamus. Sci Bull (Beijing). 2021;66(14):1448–61. https://doi.org/10.1016/j.scib.2021.04.002 .
Peng H, Zhang K, Miao J, Yang Y, Xu S, Wu T, et al. SnRNA-Seq of pancreas revealed the dysfunction of endocrine and exocrine cells in transgenic pigs with prediabetes. Int J Mol Sci. 2023;24(9):7701. https://doi.org/10.3390/ijms24097701 .
Zarei K, Stroik MR, Gansemer ND, Thurman AL, Ostedgaard LS, Ernst SE, et al. Early pathogenesis of cystic fibrosis gallbladder disease in a porcine model. Lab Invest. 2020;100(11):1388–99. https://doi.org/10.1038/s41374-020-0474-8 .
Wang F, Ding P, Liang X, Ding X, Brandt CB, Sjostedt E, et al. Endothelial cell heterogeneity and microglia regulons revealed by a pig cell landscape at single-cell level. Nat Commun. 2022;13:3620. https://doi.org/10.1038/s41467-022-31388-z .
Shin JS, Kim JS, Kim JM, Jang JY, Kim YH, Kim HJ, et al. Minimizing immunosuppression in islet xenotransplantation. Immunotherapy. 2014;6(4):419–30. https://doi.org/10.2217/imt.14.14 .
Adams AB, Kim SC, Martens GR, Ladowski JM, Estrada JL, Reyes LM, et al. Xenoantigen deletion and chemical immunosuppression can prolong renal xenograft survival. Ann Surg. 2018;268(4):564–73. https://doi.org/10.1097/SLA.0000000000002977 .
Santiago CP, Gimmen MY, Lu Y, McNally MM, Duncan LH, Creamer TJ, et al. Comparative analysis of single-cell and single-nucleus RNA-sequencing in a rabbit model of retinal detachment-related proliferative vitreoretinopathy. Ophthalmol Sci. 2023;3(4):100335. https://doi.org/10.1016/j.xops.2023.100335 .
Rao WT, Jiang S, Shen YH, Wang YH, Liu SN, Tang JD, et al. Myofibroblasts: A new factor affecting the Hyperlipidemia-Induced elastic abnormality of corpus cavernosum in rabbits detected by 2-D shear wave elastography. Ultrasound Med Biol. 2023;49(11):2336–45. https://doi.org/10.1016/j.ultrasmedbio.2023.07.010 .
Liang D, Xue J, Geng L, Zhou L, Lv B, Zeng Q, et al. Cellular and molecular landscape of mammalian sinoatrial node revealed by single-cell RNA sequencing. Nat Commun. 2021;12:287. https://doi.org/10.1038/s41467-020-20448-x .
El-Hodiri HM, Campbell WA, Kelly LE, Hawthorn EC, Schwartz M, Jalligampala A, et al. Nuclear Factor I in neurons, glia and during the formation of Muller glia-derived progenitor cells in avian, porcine and primate retinas. J Comp Neurol. 2022;530(8):1213–30. https://doi.org/10.1002/cne.25270 .
Li Z, Zheng M, Mo J, Li K, Yang X, Guo L, et al. Single-cell RNA sequencing of preadipocytes reveals the cell fate heterogeneity induced by melatonin. J Pineal Res. 2021;70(3):e12725. https://doi.org/10.1111/jpi.12725 .
Ghinia TM, Buenaventura DF, Kim DY, Thakurdin C, Gonzalez KC, Emerson MM. OTX2 represses sister cell fate choices in the developing retina to promote photoreceptor specification. Elife. 2020;9:e54279. https://doi.org/10.7554/eLife.54279 .
Ba H, Wang X, Wang D, Ren J, Wang Z, Sun H, et al. Single-cell transcriptome reveals core cell populations and androgen - RXFP2 axis involved in deer antler full regeneration. Cell Regen. 2022;11:43. https://doi.org/10.1186/s13619-022-00153-4 .
Qin T, Zhang G, Zheng Y, Li S, Yuan Y, Li Q, et al. A population of stem cells with strong regenerative potential discovered in deer antlers. Science. 2023;379(6634):840–7. https://doi.org/10.1126/science.add0488 .
Sinha S, Sparks HD, Labit E, Robbins HN, Gowing K, Jaffer A, et al. Fibroblast inflammatory priming determines regenerative versus fibrotic skin repair in reindeer. Cell. 2022;185(25):4717–36. https://doi.org/10.1016/j.cell.2022.11.004 .
Kashima Y, Sakamoto Y, Kaneko K, Seki M, Suzuki Y, Suzuki A. Single-cell sequencing techniques from individual to multiomics analyses. Exp Mol Med. 2020;52(9):1419–27. https://doi.org/10.1038/s12276-020-00499-2 .
Yu Z, Liao J, Chen Y, Zou C, Zhang H, Cheng J, et al. Single-Cell transcriptomic map of the human and mouse bladders. J Am Soc Nephrol. 2019;30(11):2159–76. https://doi.org/10.1681/ASN.2019040335 .
Wilkerson BA, Zebroski HL, Finkbeiner CR, Chitsazan AD, Beach KE, Sen N, et al. Novel cell types and developmental lineages revealed by single-cell RNA-seq analysis of the mouse crista ampullaris. Elife. 2021;10:e60108. https://doi.org/10.7554/eLife.60108 .
Harman RM, Patel RS, Fan JC, Park JE, Rosenberg BR, Van de Walle GR. Single-cell RNA sequencing of equine mesenchymal stromal cells from primary donor-matched tissue sources reveals functional heterogeneity in immune modulation and cell motility. Stem Cell Res Ther. 2020;11:524. https://doi.org/10.1186/s13287-020-02043-5 .
Xie L, Zhang S, Huang L, Peng Z, Lu H, He Q, et al. Single-cell RNA sequencing of peripheral blood reveals that monocytes with high cathepsin S expression aggravate cerebral ischemia-reperfusion injury. Brain Behav Immun. 2023;107:330–44. https://doi.org/10.1016/j.bbi.2022.11.001 .
Zolfaghari A, Djakiew D. Inhibition of chemomigration of a human prostatic carcinoma cell (TSU-pr1) line by inhibition of epidermal growth factor receptor function. Prostate. 1996;28(4):232–8. https://doi.org/10.1002/(SICI)1097-0045(199604)28:4%3c232::AID-PROS4%3e3.0.CO;2-F .
Chu T, Wang Z, Pe’Er D, Danko CG. Cell type and gene expression deconvolution with BayesPrism enables Bayesian integrative analysis across bulk and single-cell RNA sequencing in oncology. Nat Cancer. 2022;3(4):505–17. https://doi.org/10.1038/s43018-022-00356-3 .
Cui H, Wang C, Maan H, Pang K, Luo F, Duan N, et al. ScGPT: Toward building a foundation model for single-cell multi-omics using generative AI. Nat Methods. 2024. https://doi.org/10.1038/s41592-024-02201-0 . Epub ahead of print.
Goulet O, Pigneur B, Charbit-Henrion F. Congenital enteropathies involving defects in enterocyte structure or differentiation. Best Pract Res Clin Gastroenterol. 2022;56–57:101784. https://doi.org/10.1016/j.bpg.2021.101784 .
Gustafsson JK, Johansson M. The role of goblet cells and mucus in intestinal homeostasis. Nat Rev Gastroenterol Hepatol. 2022;19(12):785–803. https://doi.org/10.1038/s41575-022-00675-x .
He GW, Lin L, DeMartino J, Zheng X, Staliarova N, Dayton T, et al. Optimized human intestinal organoid model reveals interleukin-22-dependency of paneth cell formation. Cell Stem Cell. 2022;29(9):1333–45. https://doi.org/10.1016/j.stem.2022.08.002 .
Sanchez JG, Enriquez JR, Wells JM. Enteroendocrine cell differentiation and function in the intestine. Curr Opin Endocrinol Diabetes Obes. 2022;29(2):169–76. https://doi.org/10.1097/MED.0000000000000709 .
Zhang L, Li Z, Skrzypczynska KM, Fang Q, Zhang W, O’Brien SA, et al. Single-cell analyses inform mechanisms of myeloid-targeted therapies in colon cancer. Cell. 2020;181(2):442-59 e29. https://doi.org/10.1016/j.cell.2020.03.048 .
Traag VA, Waltman L, van Eck NJ. From Louvain to Leiden: Guaranteeing well-connected communities. Sci Rep. 2019;9:5233. https://doi.org/10.1038/s41598-019-41695-z .
Blondel VD, Guillaume JL, Lambiotte R, Lefebvre E. Fast unfolding of communities in large networks. J Stat Mech-Theory E. 2008;2008:P10008. https://doi.org/10.1088/1742-5468/2008/10/P10008 .
Yu L, Cao Y, Yang J, Yang P. Benchmarking clustering algorithms on estimating the number of cell types from single-cell RNA-sequencing data. Genome Biol. 2022;23:49. https://doi.org/10.1186/s13059-022-02622-0 .
Zappia L, Oshlack A. Clustering trees : A visualization for evaluating clusterings at multiple resolutions. Gigascience. 2018;7(7):giy083. https://doi.org/10.1093/gigascience/giy083 .
Zhang S, Li X, Lin J, Lin Q, Wong KC. Review of single-cell RNA-seq data clustering for cell-type identification and characterization. RNA. 2023;29(5):517–30. https://doi.org/10.1261/rna.078965.121 .
Steele MA, Penner GB, Chaucheyras-Durand F, Guan LL. Development and physiology of the rumen and the lower gut: Targets for improving gut health. J Dairy Sci. 2016;99(6):4955–66. https://doi.org/10.3168/jds.2015-10351 .
Jones RC, Karkanias J, Krasnow MA, Pisco AO, Quake SR, Salzman J, et al. The Tabula Sapiens: a multiple-organ, single-cell transcriptomic atlas of humans. Science. 2022;376(6594):eabl4896. https://doi.org/10.1126/science.abl4896 .
Xu C, Prete M, Webb S, Jardine L, Stewart BJ, Hoo R, et al. Automatic cell-type harmonization and integration across Human Cell Atlas datasets. Cell. 2023;186(26):5876–91. https://doi.org/10.1016/j.cell.2023.11.026 .
Dominguez CC, Xu C, Jarvis LB, Rainbow DB, Wells SB, Gomes T, et al. Cross-tissue immune cell analysis reveals tissue-specific features in humans. Science. 2022;376(6594):eabl5197. https://doi.org/10.1126/science.abl5197 .
Aran D, Looney AP, Liu L, Wu E, Fong V, Hsu A, et al. Reference-based analysis of lung single-cell sequencing reveals a transitional profibrotic macrophage. Nat Immunol. 2019;20(2):163–72. https://doi.org/10.1038/s41590-018-0276-y .
Jia M, Zhu S, Xue M, Chen H, Xu J, Song M, et al. Single-cell transcriptomics across 2,534 microbial species reveals functional heterogeneity in the rumen microbiome. Nat Microbiol. 2024. https://doi.org/10.1038/s41564-024-01723-9 .
Mantri M, Scuderi GJ, Abedini-Nassab R, Wang M, McKellar D, Shi H, et al. Spatiotemporal single-cell RNA sequencing of developing chicken hearts identifies interplay between cellular differentiation and morphogenesis. Nat Commun. 2021;12:1771. https://doi.org/10.1038/s41467-021-21892-z .
Baysoy A, Bai Z, Satija R, Fan R. The technological landscape and applications of single-cell multi-omics. Nat Rev Mol Cell Biol. 2023;24(10):695–713. https://doi.org/10.1038/s41580-023-00615-w .
Cai S, Hu B, Wang X, Liu T, Lin Z, Tong X, et al. Integrative single-cell RNA-seq and ATAC-seq analysis of myogenic differentiation in pig. BMC Biol. 2023;21:19. https://doi.org/10.1186/s12915-023-01519-z .
Lancaster MA, Knoblich JA. Organogenesis in a dish: Modeling development and disease using organoid technologies. Science. 2014;345(6194):1247125. https://doi.org/10.1126/science.1247125 .
Beaumont M, Blanc F, Cherbuy C, Egidy G, Giuffra E, Lacroix-Lamande S, et al. Intestinal organoids in farm animals. Vet Res. 2021;52:33. https://doi.org/10.1186/s13567-021-00909-x .
Kar SK, Wells JM, Ellen ED, Te PM, Madsen O, Groenen M, et al. Organoids: a promising new in vitro platform in livestock and veterinary research. Vet Res. 2021;52:43. https://doi.org/10.1186/s13567-021-00904-2 .
Xu Z, Xu X, Yang B, Mi Y, Wang J. 3D sheep rumen epithelial structures driven from single cells in vitro. Vet Res. 2023;54:104. https://doi.org/10.1186/s13567-023-01234-1 .
Download references
Acknowledgements
We thank all the members in the Institute of Dairy Science, College of Animal Sciences, Zhejiang University.
This study was supported by the Natural Science Foundation of Zhejiang Province Outstanding Youth Fund Project (LR23C170001).
Author information
Authors and affiliations.
Institute of Dairy Science, Ministry of Education Key Laboratory of Molecular Animal Nutrition, College of Animal Sciences, Zhejiang University, Hangzhou, 310058, China
Yunan Yan, Senlin Zhu, Minghui Jia, Xinyi Chen, Wenlingli Qi, Fengfei Gu, Teresa G. Valencak, Jian-Xin Liu & Hui-Zeng Sun
Key Laboratory of Dairy Cow Genetic Improvement and Milk Quality Research of Zhejiang Province, Zhejiang University, Hangzhou, 310058, China
Fengfei Gu & Hui-Zeng Sun
Agency for Health and Food Safety Austria, 1220, Vienna, Austria
Teresa G. Valencak
You can also search for this author in PubMed Google Scholar
Contributions
YNY: Conceptualization, Writing–Original Draft, Visualization. SLZ: Conceptualization, Visualization. MHJ: Writing–Original Draft. XYC: Visualization. WLLQ: Visualization. FFG: Conceptualization, Writing–Review & Editing, Supervision. TGV: writing, reviewing, revising. JXL: Conceptualization, Writing–Review & Editing, Supervision. HZS: Conceptualization, Writing–Original Draft, Writing–Review & Editing, Supervision.
Corresponding author
Correspondence to Hui-Zeng Sun .
Ethics declarations
Ethics approval and consent to participate, consent for publication, competing interests.
The authors declare that they have no competing interests.
Supplementary Information
Additional file 1.
. Applications of single-cell transcriptomics in animal science.
Rights and permissions
Open Access This article is licensed under a Creative Commons Attribution 4.0 International License, which permits use, sharing, adaptation, distribution and reproduction in any medium or format, as long as you give appropriate credit to the original author(s) and the source, provide a link to the Creative Commons licence, and indicate if changes were made. The images or other third party material in this article are included in the article's Creative Commons licence, unless indicated otherwise in a credit line to the material. If material is not included in the article's Creative Commons licence and your intended use is not permitted by statutory regulation or exceeds the permitted use, you will need to obtain permission directly from the copyright holder. To view a copy of this licence, visit http://creativecommons.org/licenses/by/4.0/ . The Creative Commons Public Domain Dedication waiver ( http://creativecommons.org/publicdomain/zero/1.0/ ) applies to the data made available in this article, unless otherwise stated in a credit line to the data.
Reprints and permissions
About this article
Cite this article.
Yan, Y., Zhu, S., Jia, M. et al. Advances in single-cell transcriptomics in animal research. J Animal Sci Biotechnol 15 , 102 (2024). https://doi.org/10.1186/s40104-024-01063-y
Download citation
Received : 30 March 2024
Accepted : 12 June 2024
Published : 02 August 2024
DOI : https://doi.org/10.1186/s40104-024-01063-y
Share this article
Anyone you share the following link with will be able to read this content:
Sorry, a shareable link is not currently available for this article.
Provided by the Springer Nature SharedIt content-sharing initiative
- Disease modelling
- Reproductive performance
Journal of Animal Science and Biotechnology
ISSN: 2049-1891
- Submission enquiries: Access here and click Contact Us
- General enquiries: [email protected]
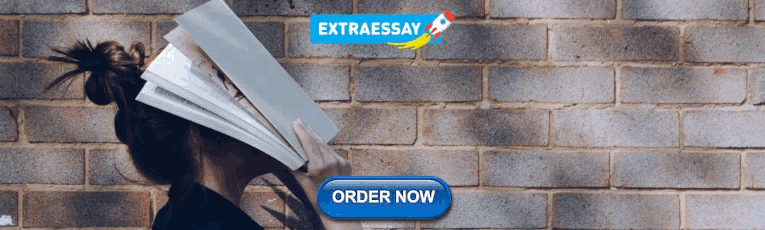
IMAGES
VIDEO
COMMENTS
1. Introduction. This theme issue is dedicated to the memory of Dorothy Cheney—an extraordinary and insightful primatologist who, with her husband Robert Seyfarth, studied vervet and baboon vocal communication and illuminated the importance of social cognition in primate evolution and language origins [1,2].For centuries, scientists have been interested in the biological origins of human ...
The evolution of animal communication. Marc Naguib a,∗and J. Jordan Price b. a Wageningen University, Department of Animal Sciences, Behavioural Ecology Group, De Elst 1, 6708 WD Wageningen, The ...
The past few years have seen a surge of interest in using machine learning (ML) methods for studying the behavior of nonhuman animals (hereafter "animals") ( 1 ). A topic that has attracted particular attention is the decoding of animal communication systems using deep learning and other approaches ( 2 ). Now is the time to tackle ...
A vocal display in which multiple types of syllables and phrases are delivered in sustained performances; usually learned (Mitani and Marler, 1989;Bohn et al., 2008; Barón Birchenall, 2016 ...
2. Words ≠ concepts. Before discussing animals, it is important to first clarify some basic issues about the nature of human concepts, and to at least dip our toes into the philosophical quagmire surrounding the term 'concept' (for a concise introduction see []).My take on concepts in this essay will be essentially that of mainstream cognitive (neuro)science today, where a concept is ...
1. Introduction. Research on the origins and evolution of language origins has a troubled history of being viewed as unscientific (see e.g. Whitney 1873[1872], Doerfer 1973, Fisiak 1985; for a discussion, see Kaplan 2021).However, as also stressed in a number of recent introductions to this field (e.g. Fitch 2010, 2017; Gong et al. 2014; Dediu and de Boer 2016; Progovac 2019; Boeckx 2021), the ...
There is a caveat to the comparisons made throughout this paper between animal communication systems and language. ... but the vocal-auditory channel continues to be the main modality explored in animal communication research today as unintentionally highlighted by a Royal Society theme issue introduction (Fishbein et al., 2019).
The findings on both the underlying neurobiological systems underpinning communicative capacity, and the complexity and diversity of the communication system itself often mirror our ability with which to work with a given species, or the existence of prominent long-term field research programs. Animal communication researchers have conducted ...
Full-text available. Sep 2023. Patrice Adret. Dena J. Clink. Sofya Dolotovskaya. Request PDF | Using machine learning to decode animal communication | New methods promise transformative insights ...
The present paper draws attention to this promising research avenue by providing an overview of the state of the art of turn-taking in four animal taxa—birds, mammals, insects and anurans. ... Although temporal coordination in animal communication has attracted interest over several decades, no clear picture has yet emerged as to why ...
Note that I use meaning throughout this paper to encompass both the rich, intended meanings of human language (and arguably ape gestures), and the information receivers extract from animal signals. ... Animal communication research has been strongly shaped by the "code model" of communication, though it is rarely referenced directly (Fig. 1 ...
As an example, recent research has focused on understanding how human-generated noise (from cars, trains, etc) can impact communication in a variety of animals (Rabin et al. 2003). As the field of ...
Motivated by Understanding Animal Communication Shafi Goldwasser ∗ UC Berkeley & Project CETI [email protected] David F. Gruber City University of New York & Project CETI [email protected] Adam Tauman Kalai ∗ Microsoft Research & Project CETI [email protected] Orr Paradise UC Berkeley & Project CETI [email protected] Abstract
(a) Modelling multiple signals and functions. The study of animal communication has largely moved past the early univariate models that analysed scenarios with one signaller, one receiver, and one signal serving one function [12,13].It has importantly expanded its focus beyond selection for signal 'content', or information transfer, to a more inclusive view that acknowledges the importance ...
This Special Issue is interested in both reviews and research papers on all aspects of human-animal communication, from both human and animals (farmed and pet) point of view. It is also interested in understanding how empathy can affect the ability of humans to interpret communication cues. Papers can also present results on automatic systems ...
Detailed comparative studies have revealed many surface similarities between linguistic communication and the communication of nonhumans. How should we interpret these discoveries in linguistic and cognitive perspective? We review the literature with a specific focus on analogy (similar features and function but not shared ancestry) and homology (shared ancestry). We conclude that ...
Comparative research has proven to be a fruitful field of study on the ontogenetic and phylogenetic evolution of language, and on the cognitive capacities unique to humans or shared with other animals. The degree of continuity between components of human language and non-human animal communication systems, as well as the existence of a core factor of language, are polemic subjects at present.
Research into the semantics of animal signals began in 1980, with evidence that alarm calls of a non-human primate designated predators as external referents. These studies have challenged the historical assumption that such referential signaling is a unique feature of human language and produced a paradigm shift in animal communication research.
Animals is an international peer-reviewed open access semimonthly journal published by MDPI. Please visit the Instructions for Authors page before submitting a manuscript. The Article Processing Charge (APC) for publication in this open access journal is 2400 CHF (Swiss Francs). Submitted papers should be well formatted and use good English.
The "Animal" in the Humanities Research Group was founded in 2017 with the support of the Humanities Center at Texas Tech in order to foster interdisciplinary, collaborative inquiry into the role played by both "the animal" and real animals in human intellectual landscapes, historical and contemporary.
communication in animals. ANIMAL PERCEPTIONS UNDER THE MICROSCOPE Nevertheless, the road to a very active and highly successful research !eld of animal communication was not at all smooth. The Descartian view of ani-mals was anything but conducive for any signi!cant probing into animal communication since animals ©2014JohnWiley&Sons, Ltd.
impact research involving animal communication. Every paper at PNAS is handled by a member of the National Academy of Sciences, a nonprofit organization comprising nearly 2,400 active members and 500 international members, of whom more than 200 are Nobel laureates. PNAS makes research on animal communication accessible
The animal communication system, (henceforth written as. ANICOMS) refers to the methods and systems animals use to. communicate with each other belonging to the same species or to. transmit ...
In Meet the Neighbors: Animal Minds and Life in a More-Than- Human World, nature and science journalist Brandon Keim offers an empathetic, scientifically convincing counternarrative to a hyped-up discourse about coyotes.We can aim not to kill, he says, but to bring about "a culture of informed coexistence" based on "understanding enough about them to live peacefully together."
In this paper, we introduce the development of single-cell technology and review the processes, advancements, and applications of single-cell transcriptomics in animal research. We summarize recent efforts using single-cell transcriptomics to obtain a more profound understanding of animal nutrition and health, reproductive performance, genetics ...
Understanding biological mechanisms is fundamental for improving animal production and health to meet the growing demand for high-quality protein. As an emerging biotechnology, single-cell transcriptomics has been gradually applied in diverse aspects of animal research, offering an effective method to study the gene expression of high-throughput single cells of different tissues/organs in ...