Unraveling Social Media’s Issues on Public Opinion and Activism: A Thematic Review
- First Online: 11 August 2024
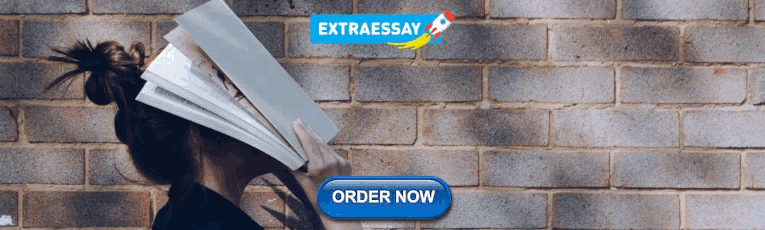
Cite this chapter
- Irma Syarlina Che Ilias ORCID: orcid.org/0000-0002-9404-4295 5 , 6 ,
- Suzaimah Ramli ORCID: orcid.org/0000-0002-6970-2663 6 ,
- Muslihah Wook ORCID: orcid.org/0000-0002-2075-4753 6 &
- Nor Asiakin Hasbullah ORCID: orcid.org/0000-0002-6948-6382 6
Part of the book series: CSR, Sustainability, Ethics & Governance ((CSEG))
37 Accesses
In the age of widespread connectivity, social media has emerged as a dynamic platform shaping public opinion and activism globally. This chapter aims to systematically review literature spanning 2013–2022, aiming to uncover prevalent social media issues influencing public opinion and activism. The objective is to discern the pivotal themes that have driven discourse, comprehend the implications, and suggest future directions for research. This research employs a thematic review approach, using research databases like Scopus and Mendeley to collect articles, resulting in a pool of 92 publications. After applying selection criteria, 30 papers form the basis of analysis. The Atlas.ti tool aids thematic analysis, enabling the identification of key patterns and categories that address the research question. Five dominant themes emerge ranging from the democratizing potential of digital literacy to the challenges stemming from misinformation, and from the role of policy in shaping public sentiment to the interplay of political viewpoints with user engagement, each theme contributes to a nuanced comprehension of the field. This research recommends future research exploring cross-cultural variations, algorithmic biases, and ethical considerations. Utilizing visual aids, such as images illustrating false information dissemination and user engagement dynamics, could enhance understanding of the challenges and potential of social media in shaping the modern socio-political landscape.
This is a preview of subscription content, log in via an institution to check access.
Access this chapter
Subscribe and save.
- Get 10 units per month
- Download Article/Chapter or eBook
- 1 Unit = 1 Article or 1 Chapter
- Cancel anytime
- Available as PDF
- Read on any device
- Instant download
- Own it forever
- Available as EPUB and PDF
- Durable hardcover edition
- Dispatched in 3 to 5 business days
- Free shipping worldwide - see info
Tax calculation will be finalised at checkout
Purchases are for personal use only
Institutional subscriptions
Abdulla, R., Poell, T., Rieder, B., Woltering, R., & Zack, L. (2018). Facebook polls as proto-democratic instruments in the Egyptian revolution: The ‘We Are All Khaled Said’ Facebook page. Global Media and Communication, 14 (1), 141–160. https://doi.org/10.1177/1742766518760085
Article Google Scholar
Al-Shamsi, Y. (2015). Freedom of expression in the Omani social media: Comparative analysis between private and government-owned media on. Citeseer, 08 (03), 483–496.
Google Scholar
Bashonga, R., & Khuzwayo, Z. (2017). “This thing of the victim has to prove that the perp intended to assault is kak!”: Social media responses to sexual violence on South African university campuses. Agenda, 31 (3–4), 35–49. https://doi.org/10.1080/10130950.2017.1394636
Bhimani, H., Mention, A. L., & Barlatier, P. J. (2019). Social media and innovation: A systematic literature review and future research directions. Technological Forecasting and Social Change, 144 , 251–269. https://doi.org/10.1016/j.techfore.2018.10.007
Bondarenko, V., Kaptiurova, O., & Orlova, V. (2021). #BLACKLIVESMATTER and struggle over national discourse on twitter: Digital activism as new public sphere. Current Issues of Ukrainian Linguistics Theory and Practice, 42 , 90–103. https://doi.org/10.17721/apultp.2021.42.90-103
Boscarino, J. E. (2022). Is seeing believing? Comparing the influence of textual and visual policy narratives on public opinion. Politics & Policy, 50 (4), 720–751. https://doi.org/10.1111/polp.12471
Bouza, L., Oleart, Á., & Tuñón, J. (2019). Framing eu trade policy online: The case of @NoALTTIP on twitter. Communications Society, 32 (4 Special Issue), 257–273. https://doi.org/10.15581/003.32.4.257-273
Braun, V., & Clarke, V. (2012). Thematic analysis. 2. https://doi.org/10.1037/13620-004
Buntoro, K., Suswanta, S., Nurmandi, A., Setiawan, A., & Saputra, H. A. (2021). Twitter media activities: Virtual activism related to China Uighur Muslim problems. Jurnal Tarbiyatuna, 12 (1), 1–18. https://doi.org/10.31603/tarbiyatuna.v12i1.4115
Cervi, L., & Marín-Lladó, C. (2022). Freepalestine on TikTok: From performative activism to (meaningful) playful activism. Journal of International and Intercultural Communication, 15 (4), 414–434. https://doi.org/10.1080/17513057.2022.2131883
Chauhan, P., & Chaddah, J. K. (2017). Role of social media in influencing sustainability practices among Indian organizations. Sansmaran Research Journal, 7 (2), 12–18. www.lib.gnims.com
Dalvi-Esfahani, M., Wai Leong, L., Ibrahim, O., & Nilashi, M. (2020). Explaining students’ continuance intention to use Mobile web 2.0 learning and their perceived learning: An integrated approach. Journal of Educational Computing Research, 57 (8), 1956. https://doi.org/10.1177/0735633118805211
Demir, S., & Koksoy, E. (2021). The impact of social media on agenda setting and public opinion formation: The black lives matter movement as an example of social activism. Social Sciences Studies Journal, 7 (82), 1858–1868. https://avesis.marmara.edu.tr/yayin/ce7c31b2-ce85-4721-b470-fd3621363f3d/the-impact-of-social-media-on-agenda-setting-and-public-opinion-formation-the-black-lives-matter-movement-as-an-example-of-social-activism
Dey, S. (2020). Let there be clamor: Exploring the emergence of a new public sphere in India and use of social media as an instrument of activism. Journal of Communication Inquiry, 44 (1), 48–68. https://doi.org/10.1177/0196859919827319
Doherty, K., & Doherty, G. (2019). Engagement in HCI: Conception, theory and measurement. ACM Computing Surveys, 51 (5), 1–39. https://doi.org/10.1145/3234149
Gull, R., Shoaib, U., Rasheed, S., Abid, W., & Zahoor, B. (2016). Pre processing of Twitter’s data for opinion Mining in Political Context. Procedia Computer Science, 96 (September), 1560–1570. https://doi.org/10.1016/j.procs.2016.08.203
Hassan, R. (2018). Egypt’s watchdogs: Citizen journalism before, during and after the 2011 revolution. Journal of Arab and Muslim Media Research, 11 (1), 45–60. https://doi.org/10.1386/jammr.11.1.45_1
Hollywood, N. B., Hill, A., & Banks, T. (2021). Early findings of a study exploring the social media, political and cultural awareness, and civic activism of gen Z students in the mid-Atlantic United States. Proceedings of the information science + information technology education conference , 011.
Hutchinson, J. (2013). The cultural impact of institutional remix: The formalisation of textual reappropriation within the ABC. M/C Journal, 16 (4), 1–6.
Korschun, D. (2021). Brand activism is here to stay: Here’s why. NIM Marketing Intelligence Review, 13 (2), 10–17. https://doi.org/10.2478/nimmir-2021-0011
Lam, V. (2022). Information and communications technologies, online activism, and implications for Vietnam’s public diplomacy. Journal of Current Southeast Asian Affairs, 41 (1), 3–33. https://doi.org/10.1177/18681034211002850
Leung, L. Y. M. (2020). Closeted love? Borders of (mediated) belonging in ‘minority radio broadcasting’. Social Identities, 26 (2), 250–269. https://doi.org/10.1080/13504630.2020.1761315
McKay, F. H., & Dunn, M. (2017). Can online participation on issues of asylum seeking lead to action? Understanding the intent to act. Australian Journal of Psychology, 69 (4), 247–255. https://doi.org/10.1111/ajpy.12147
Mialon, M., & da Gomes, F. S. (2019). Public health and the ultra-processed food and drink products industry: Corporate political activity of major transnationals in Latin America and the Caribbean. Public Health Nutrition, 22 (10), 1898–1908.
Naqvi, S. M. I. R., & Khouzani, N. K. (2017). Influence of political marketing campaigns through social media on political activism: Special emphasis on youth of Pakistan. Economic and Social Development: Book of Proceedings , 238–246. https://www.proquest.com/conference-papers-proceedings/influence-political-marketing-campaigns-through/docview/2070392019/se-2?accountid=145163
Pontoh, A. K. (2021). Computational propaganda in hashtag activism. Jurnal Komunikasi, 13 (2), 251. https://doi.org/10.24912/jk.v13i2.11086
Rogers, A., Vassilev, I., Pumar, M. J. J., Todorova, E., Portillo, M. C., Foss, C., Koetsenruijter, J., Ratsika, N., Serrano, M., Knutsen, I. A. R., Wensing, M., Roukova, P., Patelarou, E., Kennedy, A., & Lionis, C. (2015). Meso level influences on long term condition self-management: Stakeholder accounts of commonalities and differences across six European countries health policies, systems and management in high-income countries. BMC Public Health, 15 (1), 1–11. https://doi.org/10.1186/s12889-015-1957-1
She, C. (2022). Social media dissemination of counter accounts and stakeholder support–evidence from greenpeace’s “save the Arctic” campaign on Facebook. Accounting Forum, 0 (0), 1–26. https://doi.org/10.1080/01559982.2021.2019524
Sim, M. C., Lim, E. C. L., Chu, T. C., Yin, C. L., Yung, C. C., Abdul, S., & S.-. (2022). How #TaiwanCanHelp reverberates: An exploratory analysis of advocacy hashtag on twitter. Social Media and Society, 8 (3), 1–17. https://doi.org/10.1177/20563051221116847
Stevens, T. M., Aarts, N., Termeer, C. J. A. M., & Dewulf, A. (2018). Social media hypes about agro-food issues: Activism, scandals and conflicts. Food Policy, 79 (June), 23–34. https://doi.org/10.1016/j.foodpol.2018.04.009
Stone, C. B., & Wang, Q. (2019). From conversations to digital communication: The mnemonic consequences of consuming and producing information via social media. Topics in Cognitive Science, 11 (4), 774–793. https://doi.org/10.1111/tops.12369
Thakur, P. B., & De, S. (2016). Social media and social movement:Contemporary online activism in Asia. Media Watch, 7 (2), 226–243. https://doi.org/10.15655/mw/2016/v7i2/98745
Turner, J. (2021). “Someone should do something”: Exploring public sphere ideals in the audiences of UK hyperlocal media Facebook pages. Journalism Studies, 22 (16), 2236–2255. https://doi.org/10.1080/1461670X.2021.1991837
Wilcox, D. L. (2019). Dialogic communication theory in the age of corporate activism: A postmodern perspective. Communication and Media in Asia Pacific (CMAP), 2 (1), 1–10.
Zairul, M. (2020). A thematic review on student-centred learning in the studio education. Journal of Critical Reviews, 7 (2), 504–511. https://doi.org/10.31838/jcr.07.02.95
Zhu, H., & Miao, W. (2021). Should I click the “like” button for my colleague? Domesticating social media affordance in the workplace. Journal of Broadcasting and Electronic Media, 65 (5), 1–21. https://doi.org/10.1080/08838151.2021.1991350
Download references
Author information
Authors and affiliations.
Computer Engineering Technology Section, Malaysian Institute of Information Technology, Universiti Kuala Lumpur, Kuala Lumpur, Malaysia
Irma Syarlina Che Ilias
Computer Science Department, Defense Technology and Science Faculty, National Defense University of Malaysia, Kuala Lumpur, Malaysia
Irma Syarlina Che Ilias, Suzaimah Ramli, Muslihah Wook & Nor Asiakin Hasbullah
You can also search for this author in PubMed Google Scholar
Corresponding author
Correspondence to Irma Syarlina Che Ilias .
Editor information
Editors and affiliations.
Brunel University - London, Uxbridge, Middlesex, UK
Reem Khamis
Brunel University London, Uxbridge, Middlesex, UK
Amina Buallay
Rights and permissions
Reprints and permissions
Copyright information
© 2024 The Author(s), under exclusive license to Springer Nature Switzerland AG
About this chapter
Ilias, I.S.C., Ramli, S., Wook, M., Hasbullah, N.A. (2024). Unraveling Social Media’s Issues on Public Opinion and Activism: A Thematic Review. In: Khamis, R., Buallay, A. (eds) Board Diversity and Corporate Governance. CSR, Sustainability, Ethics & Governance. Springer, Cham. https://doi.org/10.1007/978-3-031-53877-3_13
Download citation
DOI : https://doi.org/10.1007/978-3-031-53877-3_13
Published : 11 August 2024
Publisher Name : Springer, Cham
Print ISBN : 978-3-031-53876-6
Online ISBN : 978-3-031-53877-3
eBook Packages : Business and Management Business and Management (R0)
Share this chapter
Anyone you share the following link with will be able to read this content:
Sorry, a shareable link is not currently available for this article.
Provided by the Springer Nature SharedIt content-sharing initiative
- Publish with us
Policies and ethics
- Find a journal
- Track your research

An official website of the United States government
The .gov means it’s official. Federal government websites often end in .gov or .mil. Before sharing sensitive information, make sure you’re on a federal government site.
The site is secure. The https:// ensures that you are connecting to the official website and that any information you provide is encrypted and transmitted securely.
- Publications
- Account settings
Preview improvements coming to the PMC website in October 2024. Learn More or Try it out now .
- Advanced Search
- Journal List
- Springer Nature - PMC COVID-19 Collection

Conceptualising and measuring social media engagement: A systematic literature review
Mariapina trunfio.
Department of Management and Quantitative Studies, University of Naples “Parthenope”, Naples, Italy
Simona Rossi
The spread of social media platforms enhanced academic and professional debate on social media engagement that attempted to better understand its theoretical foundations and measurements. This paper aims to systematically contribute to this academic debate by analysing, discussing, and synthesising social media engagement literature in the perspective of social media metrics. Adopting a systematic literature review, the research provides an overarching picture of what has already been investigated and the existing gaps that need further research. The paper confirms the polysemic and multidimensional nature of social media engagement. It identifies the behavioural dimension as the most used proxy for users' level of engagement suggesting the COBRA model as a conceptual tool to classify and interpret the construct. Four categories of metrics emerged: quantitative metrics, normalised indexes, set of indexes, qualitative metrics. It also offers insights and guidance to practitioners on modelling and managing social media engagement.
Introduction
Over the last decade, customer engagement has received increasing attention in academic and professional debate (Hollebeek, 2019 ; Kumar et al., 2019 ; Marketing Science Institute, 2020 ; Peltier et al., 2020 ; Rather et al., 2019 ; Rossmann et al., 2016 ). It can be considered a “consumer’s positively brand-related cognitive, emotional and behavioural activity during, or related to, focal consumer/brand interactions” (Hollebeek, 2014 , p.149). Engaged customers display greater brand loyalty and satisfaction (Bowden, 2009 ; Jaakkola & Alexander, 2014 ) and are more likely to contribute to new product development (Haumann et al., 2015 ), service innovation (Kumar et al., 2010 ), and viral marketing activity spread by word of mouth (Wu et al., 2018 ). Customer engagement can also be linked with important brand performance indicators, including sales growth, feedback, and referrals (Van Doorn et al., 2010 ).
Acknowledging the potential of ICTs, scholars and practitioners are experimenting with new ways to capitalise on customer engagement and adapt to the new challenges of digital platforms (Barger et al., 2016 ; Peltier et al., 2020 ). Social media platforms reshaped the dyadic interaction between customers and organisations, creating spaces for digital sharing and engagement. By enabling users to comment, review, create, and share content across online networks, social media provide direct access to brands and allow co-creation processes. As such, the pervasive character of social media with its potential for engaging with customers and building relationships generated much interest in the concept of social media engagement (Barger et al., 2016 ; Hallock et al., 2019 ; Oviedo-García et al., 2014 ; Peltier et al., 2020 ; Schivinski et al., 2016 ). Engaging with customers in real-time and managing many incoming customers’ big data interested academic investigation and opened opportunities for marketers to enhance social media marketing success (Liu et al., 2019 ).
Understanding, monitoring, and measuring social media engagement are key aspects that interest scholars and practitioners who proposed diverse conceptualisations, several indicators and KPIs. With the spread of social media analytics, social networking platforms, digital service providers, marketers, and freelancers developed their metrics to measure engagement with brand-related social media contents and advertising campaigns. At the same time, scholars have pointed out various metrics and procedures that contribute to evaluating social media engagement in different fields (Mariani et al., 2018 ; Muñoz-Expósito et al., 2017 ; Trunfio & Della Lucia, 2019 ). Nevertheless, many of these studies offer a partial perspective of analysis that does not allow the phenomenon to be represented in diverse aspects (Oviedo-García et al., 2014 ). As a result, social media engagement remains an enigma wrapped in a riddle for many executives (McKinsey, 2012 ). How communities across an ever-growing variety of platforms, new forms of customer-brand interactions, different dimensions and cultural differences impact social media engagement measurement represents one of the main challenges (Peltier et al., 2020 ).
Although social media engagement represented a key topic in marketing research (Barger et al., 2016 ; Peltier et al., 2020 ), an overarching perspective of the existing knowledge can drive the investigation of the state of the field, including the study of the research streams, and the analysis of the measurement tools. This paper aims to systematically contribute to the academic debate by analysing, discussing, and synthesising social media engagement literature from the social media metrics perspective. A systematic literature review approach provides an overarching picture of what has already been investigated and the existing gaps that need further research. It contributes towards a systematic advancement of knowledge in the field and offers insights and guidance to practitioners on modelling and managing social media engagement (Tranfield et al., 2003 ).
The remainder of the paper is structured as follows. Section 2 presents the theoretical background of the study on customer engagement and social media engagement. Section 3 describes the methodology used for conducting the systematic literature review (Pickering & Byrne, 2014 ; Tranfield et al., 2003 ). Section 4 presents the bibliometric analysis results, including the year in which research began, the journals that publish most research, and the most relevant authors with publications on the topic. Then, Sect. 5 classifies these studies in terms of four macro-themes, conceptualisations, platforms, measurement, and behaviours and describes the key results available in the literature. Section 6 provides a critical discussion of the findings from the literature review and highlights its key contributions. Lastly, Sect. 7 concludes the study by highlighting its limitations and proposing directions for future research.
Theoretical background
Customer engagement.
Although customer engagement research has increased theoretical and managerial relevance (Brodie et al., 2011 ; Hollebeek et al., 2016 , 2019 ; Kumar et al., 2019 ; Vivek et al., 2012 ), to date, there is still no consensus on its definition due to its multidimensional, multidisciplinary and polysemic nature.
Several customer engagement conceptualisations have been proposed in the literature, drawing on various theoretical backgrounds, particularly service-dominant logic, and relationship marketing. From a psychological perspective, one of the first definitions of customer engagement is the one of Bowden ( 2009 ) that conceptualises it as a psychological process that drives customer loyalty. Similarly, Brodie et al. ( 2011 ) define customer engagement as a psychological state that occurs by interactive, co-creative customer experiences with a focal object. Later, focusing on the behavioural aspects, it has been described as the intensity of an individual’s participation in an organisation’s offerings or organisational activities (Vivek et al., 2012 ). More recently, from a value-based perspective, customer engagement has been defined as the mechanics that customers use to add value to the firm (Kumar et al., 2019 ).
Although the perspectives may vary, common elements can be identified in various conceptualisations. Literature generally understands customer engagement as a highly experiential, subjective, and context-dependent construct (Brodie et al., 2011 ) based on customer-brand interactions (Hollebeek, 2018 ). Moreover, scholars agree on its multidimensional nature (Brodie et al., 2013 ; Hollebeek et al., 2016 ; So et al., 2016 ; Vivek et al., 2012 ) encompassing cognitive (customer focus and interest in a brand), emotional (feelings of inspiration or pride caused by a brand), and behavioural (customer effort and energy necessary for interaction with a brand) dimensions. Also, researchers have proposed that customer engagement affects different marketing constructs (Brodie et al., 2011 ; Van Doorn et al., 2010 ). For example, in Bowden’s research (2009), there is evidence to support that customer engagement is a predictor of loyalty. Brodie et al. ( 2011 ) explore its effects on customer satisfaction, empowerment, trust, and affective commitment towards the members of a community. Van Doorn et al. ( 2010 ) propose customer-based drivers, including attitudinal factors such as satisfaction, brand commitment and trust, as well as customer goals, resources, and value perceptions.
Social media engagement: The academic perspective
Social media engagement has also been investigated as brand-user interaction on social media platforms (Barger et al., 2016 ; De Vries & Carlson, 2014 ; Hallock et al., 2019 ; Oviedo-García et al., 2014 ; Peltier et al., 2020 ; Schivinski et al., 2016 ). However, while conceptual discussions appear to dominate the existing customer engagement literature, research results fragmented when moving to the online context. Scholars agree that social media engagement is a context-specific occurrence of customer engagement (Brodie et al., 2013 ) that reflects customers’ individual positive dispositions towards the community or a focal brand (Dessart, 2017 ). Social media engagement can emerge with respect to different objects: the community, representing other customers in the network, and the brand (Dessart, 2017 ). Furthermore, antecedents and consequences of social media engagement have been identified to understand why customers interact on social media and the possible outcomes (Barger et al., 2016 ), such as loyalty, satisfaction, trust, and commitment (Van Doorn et al., 2010 ).
In continuity with literature on customer engagement, also social media engagement can be traced back to affective, cognitive, and behavioural dimensions (Van Doorn et al., 2010 ). Most of the literature focuses on the behavioural dimension as it can be expressed through actions such as liking, commenting, sharing, and viewing contents from a brand (Barger et al., 2016 ; Muntinga et al., 2011 ; Oh et al., 2017 ; Oviedo-García et al., 2014 ; Peltier et al., 2020 ; Rietveld et al., 2020 ; Schivinski et al., 2016 ). It is worth pointing out that not all these actions determine the same level of engagement. Schivinski et al. ( 2016 ) in the COBRA (Consumer Online Brand Related Activities) Model differentiate between three levels of social media engagement: consumption, contribution, and creation. Consumption constitutes the minimum level of engagement and is the most common brand-related activity among customers (e.g., viewing brand-related audio, video, or pictures). Contribution denotes the response in peer-to-peer interactions related to brands (e.g., liking, sharing, commenting on brand-related contents). Creation is the most substantial level of the online brand-related activities that occur when customers spontaneously participate in customising the brand experiences (e.g., publishing brand-related content, uploading brand-related video, pictures, audio or writing brand-related articles). Starting from these social media actions, scholars attempted to measure social media engagement in several ways developing scales, indexes, and metrics (Harrigan et al., 2017 ; Oviedo-García et al., 2014 ; Schivinski et al., 2016 ; Trunfio & Della Lucia, 2019 ). Nevertheless, many of these studies offer a partial perspective of analysis that does not allow the phenomenon to be represented in its diverse aspects (Oviedo-García et al., 2014 ). Researchers have also examined emotional and cognitive dimensions (Dessart, 2017 ) as essential components of social media engagement that lead to positive brand outcomes (Loureiro et al., 2017 ).
Social media engagement: The practitioners’ perspective
In business practice, the concept of customer engagement appeared for the first time in 2006 when the Advertising Research Foundation (ARF), in conjunction with the American Association of Advertising Agencies and the Association of National Advertisers, defined it as a turning on a prospect to a brand idea enhanced by the surrounding context (ARF, 2006 ) . Later, several consulting firms tried to give their definition emphasising different aspects and perspectives. For example, in 2008, Forrester Consulting, an American market research company, defined customer engagement as a way to create ‘deep connections with customers that drive purchase decisions, interaction, and participation over time’ (Forrester Consulting, 2008 , p.4). Gallup Consulting identified four levels of customer engagement and defined it as an emotional connection between customers and companies (Gallup Consulting, 2009 ). Similarly, the famous American software provider Hubspot ( 2014 ) identified social media engagement as ‘ the ongoing interactions between company and customer, offered by the company, chosen by the customer’ (Hubspot, 2014 , p.1).
With the increasing spread of social networks and their exploitation as an important marketing tool, practitioners recognised a clear linkage between customer engagement and the metrics to assess digital strategy success. Over time, social networking platforms such as Facebook, LinkedIn, and YouTube, developed their metrics to measure engagement with brand-related social media contents and advertising campaigns (Table (Table1 1 ).
Social media engagement metrics by social networking platforms (2020)
Construct | Metric | Social Network |
---|---|---|
Engagement | ||
Engagement Rate | ||
Engagement Rate | ||
Engagement Rate | YouTube |
With the spread of social media analytics, platforms and digital service providers developed dashboards and analytical indicators to assess, measure and monitor the engagement generated by social media marketing activities (Table (Table2). 2 ). At the same time, many bloggers, marketers, and freelancers have weighed in on the topic, enriching the debate with new contributions.
Social media engagement metrics by social media management and analytics platforms (2020)
Construct | Metric | Platform |
---|---|---|
Engagement Rate | Socialbakers | |
Engagement | Fanpage Karma | |
Engagement Rate | Talkwalker | |
Engagement Rate | Hootsuite | |
Engagement Rate | Dashthis | |
Engagement Rate | Keyhole |
As a result, while scholars still have to agree upon a shared definition of social media engagement, marketers have recognised it as one of the most important online outcome companies need to deliver with social media and a key metric to assess social media strategy success . Despite the growing interest in business practice and its solid traditional theoretical roots, most of the existing literature on social media engagement offers only conceptual guidelines (Barger et al., 2016 ; Peltier et al., 2020 ). The measurement of engagement in social media and its financial impact remains an enigma wrapped in a riddle for many executives (McKinsey, 2012 ) and requires further investigations. Mainly, how new and emerging platforms, new forms of customer-brand interactions, different dimensions, and cultural differences impact social media engagement measurement remains an understudied phenomenon (Peltier et al., 2020 ).
Methodology
The literature review is one of the most appropriate research methods, which aims to map the relevant literature identifying the potential research gaps that need further research to contribute towards a systematic advancement of new knowledge in the field (Tranfield et al., 2003 ). This research is built upon the rigorous, transparent, and reproducible protocol of the systematic literature review as a scientific and transparent process that reduces the selection bias through an exhaustive literature search (Pencarelli & Mele, 2019 ; Pickering & Byrne, 2014 ; Tranfield et al., 2003 ). Building on recent studies (Inamdar et al., 2020 ; Linnenluecke et al., 2020 ; Phulwani et al., 2020 ), in addition to the systematic literature review, a bibliometric analysis (Li et al., 2017 ) was also performed to provide greater comprehensions into the field's current state and highlight the future research directions.
Database, keywords, inclusion, and exclusion criteria
To conduct a literature review, quality journals are considered the basis for selecting quality publications (Wallace & Wray, 2016 ). Therefore, the database Scopus, run by Elsevier Publishing, was considered to search for relevant literature, being the most significant abstract and citation source database used in recent reviews.
When conducting a literature review, a fundamental issue is determining the keywords that allow identifying the papers (Aveyard, 2007 ). To address it, the most frequently used keywords in peer-reviewed literature have been under investigation. As such, the following research chain was used: “Social media” “Engagement” AND “metric*”, searching under title, abstract, and keywords.
The systematic literature review protocol (Fig. 1 ) has been conducted on the 26 th of March 2020. The study considers an open starting time to trace back to the origin of social media engagement metrics research up to late March 2020. The initial search attempts identified 259 documents.

The systematic literature review protocol
After the articles’ identification, criteria for inclusion and exclusion were adopted. First, the 259 articles were screened, considering English-language articles published in peer-reviewed academic journals to safeguard the quality and effectiveness of the review. Due to variability in the peer-review process and their limited availability, book reviews, editorials, and papers from conference proceedings were excluded from this research. After the screening, a sample of 157 papers was obtained.
Afterwards, the full text of these papers was reviewed to assess eligible articles. As a result, 116 articles were excluded because their subject matter was not closely related to the topic of social media engagement metrics. In detail, papers were excluded when: 1) they mainly focused on social media engagement but superficially touched the metrics or 2) they mainly focused on metrics but superficially touched on social media engagement. In the end, 41 eligible articles were identified.
Analysis tools
The relevant data of the 41 documents in the final sample were saved and organised in a Microsoft Excel spreadsheet to include all the essential paper information such as paper title, authors’ names, and affiliations, abstract, keywords and references. Then, adopting the bibliometrics analysis method (Aria & Cuccurullo, 2017 ), the R-Tool ‘Biblioshiny for Bibliometrix’ was used to perform a comprehensive bibliometric analysis. Bibliometrix is a recent R-package that facilitates a more complete bibliometric analysis, employing specific tools for both bibliometric and scientometric quantitative research (Aria & Cuccurullo, 2017 ; Dervis, 2019 ; Jalal, 2019 ).
An overview of social media engagement metrics research.
The bibliometric analysis provided information on the 41 articles, allowing to highlight the significance of the topic.
Publication trend
The number of annual publications shows a rollercoaster trend (Fig. 2 ). Although the first relevant paper was published in 2013, only since 2016 publications begun to increase significantly with a slight decrease in 2018. This renders social media engagement metrics a relatively young research field.

Timeline of the studies (January 2013- March 2020)
It is worth pointing out that the articles extraction was done in March 2020: this explains the low number of articles published in 2020.
Most relevant sources
When looking at the Journal sources overview, the analysis revealed 34 journals covering different fields, including marketing, management, economics, tourism and hospitality, engineering, communication, and technology. As shown in Fig. 3 , only four journals have more than two publications: Internet Research , Journal of Engineering and Applied Sciences , International Journal of Sports Marketing and Sponsorship. and Online Information Review .

Seminal papers
Interesting findings emerged considering the most global cited documents that allow identifying the seminal articles in according to the timeliness, utility and quality, expressed by the scientific community (Okubo, 1997 ). The number of citations an article receives, and the studies cited in an article are two of the most popular bibliometric indicators used to determine the popularity of a publication.
Figure 4 shows the number of author citations for each article, identifying as seminal works: Malthouse’s (2013) paper ‘ Managing Customer Relationships in the Social Media Era: Introducing the Social CRM House’ with 278 global citations; Sabate’s (2014) paper ‘Factors influencing popularity of branded content in Facebook fan pages’ with 145 global citations; Mariani’s (2016) paper ‘ Facebook as a destination marketing tool: Evidence from Italian regional Destination Management Organizations ’ with 104 global citations; Oh’s (2017) paper ‘ Beyond likes and tweets: Consumer engagement behavior and movie box office in social media ’ with 54 global citations; Colicev’s (2018)’ Improving consumer mindset metrics and shareholder value through social media: The different roles of owned and earned media ’ with 39 global citations; Rossmann’s (2016) ‘ Drivers of user engagement in eWoM communication ’ with 35 global citations; Oviedo-Garcia’s (2014) ‘ Metric proposal for customer engagement in Facebook’ with 33 global citations .

Most cited articles
The analysis of the papers reviewed revealed that the theme of social media engagement metrics turns out to be a hot topic and a newly emerging stream of research.
Social media engagement: areas of investigation
In recent years social media engagement has gained relevance in academic research, and many scholars have questioned its measurement, intensifying the academic debate with ever new contributions. Following previous studies, a comprehensive analysis allows framing the following categories of broad research subjects, used to conduct the subsequent systematic literature review (Fig. 5 ): (1) conceptualisation, (2) platforms, (3) measurement and (4) behaviours. All 41 articles were analysed according to the proposed scheme.

Areas of investigation
Investigating social media engagement
What emerges from the analysis of the 41 papers is that scholars used different approaches and methodologies to conceptualise and measure engagement in the digital context of social media.
As shown in Fig. 6 , most studies (66%) employ quantitative methodologies. For instance, Yoon et al. ( 2018 ) explored the relationship between digital engagement metrics and financial performance in terms of company revenue, confirming that customer engagement on a company’s Facebook fan page can influence revenue. Colicev et al. ( 2018 ) developed three social media metrics, including engagement, to study the effects of earned social media and owned social media on brand awareness, purchase intention, and customer satisfaction. In comparison, Wang and Kubickova ( 2017 ) examined factors affecting the engagement metrics of Facebook fan pages in the Northeast America hotel industry, factors such as time-of-day, day-of-week, age, gender and distance between the hotel and users’ origin of residence. They also analysed the impact of Facebook engagement on electronic word-of-mouth (eWOM), to better understand the importance of the engagement metrics within the hospitality context.

Classification of the 41 articles based on the methodology applied
From a qualitative point of view (17% of the papers), Hallock et al. ( 2019 ) used a case study approach to understand the firm perspective on social media engagement metrics, shedding light on how companies view engagement with social media as measurable metrics of customer interactions with the platform. Conversely, Michopoulou and Moisa ( 2019 ) used the same approach to investigate the use of social media marketing metrics and practices in the U.K. hotel industry.
Only a small part of the studies analysed (10% of the papers) explores social media engagement from a purely conceptual perspective. In this sense, Oviedo-Garcìa et al. ( 2014 ) and Muñoz-Expósito et al. ( 2017 ) directly identified social media engagement metrics for Facebook and Twitter, providing fascinating insights for scholars and practitioners.
Finally, among the papers analysed, only three studies (7% of the papers) use mixed methodologies to explore the phenomenon from qualitative and quantitative perspectives.
Defining social media engagement
Researchers identified 30 unique definitions of engagement applied to the social media context. Multiple definitions used several terms when defining engagement on social media. They were not singular and straightforward but were interspersed with various key terms and overlapping concepts, as presented in Table Table3 3 .
Frequency of the terms used to define engagement in social media
Terms | Frequency | % |
---|---|---|
Social media engagement | 19 | 46% |
User engagement | 9 | 22% |
Customer engagement in social media | 6 | 15% |
Online engagement | 3 | 7% |
Virtual brand community engagement | 1 | 2.5% |
Audience engagement | 1 | 2.5% |
Viewer engagement | 1 | 2.5% |
Digital engagement | 1 | 2.5% |
Total | 41 | 100% |
The presence of synonymous terms directly addresses the lack of a standard definition and the challenges that this presents to researchers and practitioners in the field (Table (Table4 4 ).
Social media engagement main definitions
Authors | Terms | Definitions |
---|---|---|
Khan et al. ( ) | Social media engagement | |
Segijn et al. ( ) | Viewer engagement | |
Hallock et al. ( ) | Social media engagement | |
Osokin ( ) | User engagament | |
Medjani et al. ( ) | Social media engagement | |
Sitta et al. ( ) | Social media engagement | |
Le et al., ( ) | Online engagement | |
Muñoz-Expósito et al. ( ) | Customer engagement on Twitter | |
Khan et al. ( ) | Online engagement |
As a relevant result, most authors focus on its behavioural manifestation (22% of the studies) resulting from motivational drivers when defining social media engagement. It is considered as the active behavioural efforts that both existing and potential customers exert toward online brand-related content (Yoon et al., 2018 ). It involves various activities that range from consuming content, participating in discussions, and interacting with other customers to digital buying (Oh et al., 2017 ; Yoon et al., 2018 ). Similarly, in addition to the behavioural manifestations, other scholars (12%) focus on the emotional connection expressed through the intensity of interactions and their implications, toward the offers and activities of a brand, product, or firm, regardless of whether it is initiated by the individual or by the firm (Muñoz-Expósito et al., 2017 ).
Shifting the observation lens from the customers to the firms, another group of scholars (10% of the studies) define social media engagement as the non-monetary return that derives from the online marketing strategies of brands (Khan, 2017 ; Medjani et al., 2019 ; Michopoulou & Moisa, 2019 ). In this case, engagement is viewed exclusively as a non-financial metric and as a measure of the performance of social media marketing activities.
Lastly, a small percentage of studies (10% of the studies) considers engagement as the number of people who acknowledge agreement or preference for content, who participate in creating, sharing and using content (Colicev et al., 2018 ; Li et al., 2019 ; Rahman et al., 2017 ).
Social Media Platforms
In a total of 41 articles reviewed, 85% of studies mention the platforms analysed, as shown in Table Table5. 5 . Facebook is the most popular platform analysed, followed by Twitter, YouTube, LinkedIn, and Instagram. These results were rather expected, given the fact that Facebook, with 2.6 billion monthly active users (Facebook, May 2020), is the most popular social media platform worldwide.
Platforms mentioned in the 41 articles and related frequencies
Platform | Frequency | % |
---|---|---|
28 | 52% | |
12 | 22% | |
YouTube | 6 | 11% |
1 | 2% | |
1 | 2% | |
Multiple Platforms | 6 | 11% |
Total | 54 | 100% |
An interesting finding is that there are several articles (15% of the studies) which do not refer to a specific platform or that consider all the platforms together, when measuring social media engagement (e.g., Hallock et al., 2019 ; Medjani et al., 2019 ). This is interesting, given that each social network has different features that make the engagement measurement unique and not replicable.
Measuring social media engagement
The systematic literature review confirms that there is no theoretical certainty or solid consensus among scholars about measuring engagement on social media.
As can be seen from Table Table6, 6 , studies on social media engagement metrics can be grouped and classified into four macro-categories. The first group of studies, namely ‘quantitative metrics’, which is also the most numerous (66% of the studies), attempts to propose a simplistic assessment of the impact of social media engagement, based on the number of comments, likes, shares, followers etc. (Khan et al., 2019 ; Medjani et al., 2019 ; Yoon et al., 2018 ).
Social media engagement metrics used in the 41 articles
Author | Classification | Measurement | Platform |
---|---|---|---|
Buffard and Papasava ( ) | Normalized Index | Unique click-through-rate | |
Khan et al. ( ) | Quantitative Metrics | No. of Like, No. of Click | |
Zanini et al. ( ) | Quantitative Metrics | No. of Tweet, No. of Retweet, No. of Likes, Average Tweet, Average Retweet, Average Like, Total Interactions | |
Segijn et al. ( ) | Quantitative Metrics | No. of Tweets | |
Hallock et al. ( ) | Quantitative Metrics | No. of users on a social platform and the regularity of their interactions on the platform | Not Specified |
Osokin ( ) | Quantitative Metrics | Average no. of likes per 50 posts; Average no. of comments per 50 posts; Average no. of shares per 50 posts; No. of followers | |
Kalinić and Vujičić ( ) | Quantitative Metrics | Total interactions, No. of comments, No. of shares; Average interactions, Average comments, Average shares | |
Michopoulou and Moisa ( ) | Qualitative Metrics | N\A | Not Specified |
Medjani et al. ( ) | Quantitative Metrics | No. of like, No. of comments, No. of shares | Not Specified |
Li et al. ( ) | Set of Indexes | Conversation rate: average no. of comments per post Amplification rate: average no. of retweets and shares per post Applause rate: total no. of likes per post | Not Specified |
Abuljadail and Ha, ( ) | Qualitative Metrics | Cognition, Affection, Activation | |
Vrettos and Gouscos, ( ) | Set of Indexes | Popularity, Commitment, Virality, Online User Engagement | Facebook, Twitter, Youtube |
Aggrawal and Arora ( ) | Quantitative Metrics | No. of like count, No. of comments, No. of shares, No. of Dislike | Youtube |
McCoy et al. ( ) | Quantitative Metrics | No. of primary and secondary followers | |
Mariani et al. ( ) | Set of Indexes | Generic Engagement, Brand Engagement, User Engagement, Generic Engagement for users' activity, Brand Engagement for users' activity, User Engagement for users' activity | |
Sitta et al. ( ) | Quantitative Metrics | No. of follower, Monthly Growth, People Talking about this | |
Yoon et al. ( ) | Quantitative Metrics | No. of Comments | Faceboo k |
Rogers ( ) | Qualitative Metrics | Dominant voice, Concern, Commitment, Positioning, Alignment | Not Specified |
Colicev et al. ( ) | Quantitative Metrics | People Talking about This No.of retweets, No. of video views | Youtube |
Gruner and Power ( ) | Quantitative Metrics | No.of like, No.of comments People Talking about This No. of Twitter followers No. of LinkedIn followers No. of YouTube followers | YouTube |
Le ( ) | Quantitative Metrics | No. of like, No. of comments, No. of shares | |
Aswani et al. ( ) | Set of Indexes | Number of created discussions, Author increase, Attention paid, Burstiness level | |
Rahman et al. ( ) | Quantitative Metrics | No. of like, No. of comments, No. of shares | |
Oh et al. ( ) | Quantitative Metrics | People Talking about This No. of Facebook followers No. of Tweet No. of YouTube Views No. of YouTube Comments | YouTube |
Dolan et al. ( ) | Normalized Index | The percentage of people who saw a post (post reach) and liked, shared, clicked or commented on it | |
Rahman, et al. ( ) | Quantitative Metrics | No. of like, No. of comments, No. of shares | |
Rahman, et al. ( ) | Quantitative Metrics | No. of like, No. of comments, No. of shares | |
Popp et al. ( ) | Quantitative Metrics | No. of Facebook Followers, No. of Twitter Followers | |
Muñoz-Expósito, et al. ( ) | Normalized Index | Engagement on Twitter [(Interactions\No. of tweets) \ (Average impressions)]\ (Average reach) * 100 | |
Wang and Kubickova ( ) | Normalized Index | Daily Engaged users; Lifetime Engaged Users; People Talking About This | |
Vlachvei and Kyparissis ( ) | Normalized Index | ||
Rossmann et al. ( ) | Normalized Index | ||
Mariani et al. ( ) | Set of Indexes | Generic Engagement, Brand Engagement, User Engagement, Generic Engagement for users' activity, Brand Engagement for users' activity, User Engagement for users' activity | |
Rahman et al. ( ) | Quantitative Metrics | No. of like, No. of comments, No. of shares | |
Khan et al. ( ) | Quantitative Metrics | No. of like, No. of comments, No. of shares | |
Rahman et al. ( ) | Quantitative Metrics | No. of like, No. of comments, No. of shares | |
Rahman et al. ( ) | Quantitative Metrics | No. of like, No. of comments, No. of shares | |
Sabate et al. ( ) | Quantitative Metrics | No. of like, No. of comments | |
Oviedo-García et al. ( ) | Normalized Index | Engagement on Facebook = [(Like + Comment + share\ number of post) \Average Impressions] \average reach | |
Guidry et al. ( ) | Quantitative Metrics | No. of like, No. of retweet, No. of click on hyperlink, No. tweet resulted in conversation | |
Malthouse et al. ( ) | Quantitative Metrics | Lower customer engagement No. of like, No. of comments, No. of views, No. of Click Higher customer engagement No. of Review, No. of Shares | Not Specified |
The second group of studies (17% of the studies), namely ‘normalised indexes’, provide a quantitative evaluation of the engagement a content generates in relation to the number of people to whom that content has been displayed. In this way, it is possible to obtain an average measure of the users’ engagement, dividing the total actions of interest by the total number of posts (Osokin, 2019 ; Zanini et al., 2019 ), the number of followers (Vlachvei & Kyparissi, 2017 ) or the number of people reached by a post (Muñoz-Expósito et al., 2017 ; Rossmann et al., 2016 ).
In a more complex and detailed way, studies from the third group (10% of the studies) identify social media engagement metrics developing ‘set of indexes’. For example, Li et al. ( 2019 ) use three social media metrics to measure engagement in the casual-dining restaurant setting: rates of conversation, amplification, and applause. In detail, conversation rate measures the number of comments or reviews in response to a post, amplification rate measures how much online content is shared, and applause rate measures the number of positive reactions on posts. Similarly, drawing from previous literature, Mariani et al. ( 2018 ) develop three social media metrics, namely generic engagement, brand engagement, and user engagement. Authors calculated these metrics by assessing different weights to different interaction actions, to emphasise the degree of users’ involvement implied by the underlying activities of respectively liking, sharing, or commenting.
Despite their great diffusion among academics and practitioners, some scholars (7% of the studies) argue that quantitative metrics are not enough to appreciate the real value of customer engagement on social media, and a qualitative approach is more suitable. For example, Abuljadail and Ha ( 2019 ) conducted an online survey of 576 Facebook users in Saudi Arabia to examine customer engagement on Facebook. Rogers ( 2018 ) critiques contemporary social media metrics considered ‘vanity metrics’ and repurpose alt metrics scores and other engagement measures for social research—namely dominant voice, concern, commitment, positioning, and alignment—to measure the ‘otherwise engaged’.
Social media engagement brand-related activities
When measuring social media engagement, scholars dealt with different social media actions that can be classified (Table (Table7) 7 ) according to the three dimensions of the COBRA model (Consumer Online Brand Related Activities): consumption, contribution, or creation (Schivinski et al., 2016 ).
Dimensions of the COBRA model and related frequencies
Dimensions of the COBRA model | Frequency | % |
---|---|---|
Consumption | 7 | 14% |
Contribution | 35 | 68% |
Creation | 9 | 18% |
Total | 51 | 100% |
In a total of 41 articles reviewed, the most investigated dimension by researchers is contribution, i.e. when a customer comments, shares, likes a form of pre-existing brand content (e.g., Buffard et al., 2020 ; Khan et al., 2019 ). Its popularity among the studies may be due to its interactive nature of “liking” and “commenting”, which can be said to be the most common behaviour exhibited across social media platforms and often one of the most manageable interactions to obtain data. Additionally, studies that include creation in the measurement of social media engagement consider posting/publishing brand-related content, uploading brand-related video, pictures, audio or writing brand-related articles (e.g., Zanini et al., 2019 ). Among the sampled papers, the least investigated dimension of the COBRA model is consumption, considered by only seven studies (e.g., Colicev et al., 2018 ; Oh et al., 2017 ). It considers viewing brand-related audio, video, and pictures, following threads on online brand community forums or downloading branded widgets.
Dimensions have been investigated individually, for example, just considering the number of likes or comments (Khan et al., 2019 ; Yoon et al., 2018 ), or jointly using composite indicators, as in the case of Oviedo-Oviedo-García et al., 2014 ).
This research presents fresh knowledge in the academic debate by providing an overarching picture of social media engagement, framing the phenomenon conceptually and offering a lens to interpret platforms and measuring tools. Conceptual and empirical studies tried to define, conceptualise, and measure social media engagement in diverse ways from different fields of research. They increased the gap between academia and managerial practice, where the topic of social media engagement metrics seems to be much more consolidated. The paper contributes to the academic debate on social media engagement, presenting continuity and discontinuity elements between different fields of enquiry. It also offers avenues for future research that both academics and marketers should explore. It also provides insights and guidance to practitioners on modelling and managing social media engagement.
Theoretical contribution
The article offers some theoretical contributions to this relatively young research field through the systematic literature review approach.
Firstly, the paper confirms the multidimensional and polysemic nature of engagement, even in the specific context of social media platforms, in continuity with the academic customer engagement research (Brodie et al., 2013 ; Hollebeek et al., 2016 ; So et al., 2016 ; Vivek et al., 2012 ). The concept of social media engagement can be traced back to three dimensions of analysis (Van Doorn, 2010 )—affective, cognitive, and behavioural—and some empirical studies measure it as such (Dessart, 2017 ; Vivek et al., 2014 ). However, the behavioural dimension is still the most used proxy to measure users’ level of engagement. Similarly, marketers and social media platforms have focused on behavioural interactions associated with likes, comments and sharing when reporting engagement metric (Peltier et al., 2020 ). What is worth pointing out is that emotional and cognitive dimensions are also essential components of social media engagement and should be adequately addressed by future research.
Secondly, strictly related to the first point, the paper suggests the COBRA model (Schivinski, 2016 ) as a conceptual tool to classify and interpret social media engagement from the behavioural perspective. Social media engagement can be manifested symbolically through actions (Barger et al., 2016 ; Oh et al., 2017 ; Van Doorn et al., 2010 ) that can be traced back to the three dimensions of consumption, contribution and creation (Schivinski et al., 2016 ). However, it is worth pointing out that not all these actions determine the same level of engagement. When measuring social media engagement, researchers should pay attention not only to ‘contribution’ but also to ‘consumption’ and ‘creation’, which are important indicators of the attention a post receives (Oviedo-Garcìa, 2014 ; Schivinski et al., 2016 ), giving them a different weight. It becomes even more important if considering that the same social networks provide different weights to users' actions. For example, in several countries, Instagram has tested removing the like feature on content posted by others, although users can still see the number of likes on their posts. YouTube has also decided to stop showing precise subscriber counts and Facebook is experimenting with hiding like counts, similar to Instagram.
Thirdly, the paper presents some of the key metrics used to evaluate social media engagement identifying quantitative metrics, normalised indexes, set of indexes and qualitative metrics. Although all indicators are based on the interaction between the user and the brand, as the literature suggests (Barger et al., 2016 ; Oviedo-Garcìa, 2014 ; Vivek et al., 2014 ), the paper argues that different metrics measure diverse aspects of social media engagement and should be used carefully by researchers. Despite the conceptual and qualitative research on the topic, even the most recent metrics offer measurements that do not allow engagement to be widely represented in its multidimensional and polysemic nature (Oviedo-García et al., 2014 ; Peltier et al., 2020 ). To get a deeper understanding of the construct, researchers should also consider some of the most recent advances in business practice. As an example, more and more practitioners have the chance to measure engagement by tracking the time spent on content and web pages to blend the different types of material, such as pictures, text, or even videos. Also, cursor movements, which are known to correlate with visual attention, and eye-tracking, can provide insights into the within-content engagement.
Managerial implications
Even if the topic of social media engagement seems to be more consolidated in business practice, this study also provides valuable implications for practitioners. Particularly, the findings shed light on the nature of social media engagement construct and on how metrics can be an extremely useful tool to evaluate, monitor, and interpret the effectiveness of social media strategies and campaigns.
This research offers a strategic-operational guide to the measurement of social media engagement, helping marketers understand what engagement is and choose the most effective and suitable KPIs to assess the performance and success of their marketing efforts. In this sense, marketers should accompany traditional metrics, such as likes, comments and shares, with new metrics capable of better capturing user behaviours.
Marketers also need to realise that engagement is a complex construct that goes beyond the simple behavioural dimension, encompassing cognitive and emotional traits. As a result, in some cases, the so-called “vanity metrics” could fail in fully representing all the aspects of social media engagement. In these cases, it should be accompanied by qualitative insights to analyse what users like to share or talk about and not merely look at likes, comments, and shares counts.
Limitations and future research
This research is not without limitations. First, the systematic literature review only includes English articles published in Journals. As social media engagement and engagement metrics are emerging research topics, conference proceedings and book chapters could also be included to deepen the understanding of the subject. Second, this research was conducted on the database Scopus of Elsevier for the keywords “social media engagement metrics”. Researchers could use a combination of different databases and keywords to search for new contributions and insights. Third, although the paper is based on a systematic literature review, this methodology reveals the subjectivity in the social sciences.
As this is a relatively young field of research, a further academic investigation is needed to overcome the limitations of the study and outline new scenarios and directions for future research. In addition, considering the growing importance of social media, there is value in broadening the analysis through additional studies. Future marketing research could use mixed approaches to integrate the three dimensions of social media engagement, linking qualitative and quantitative data. Advanced sentiment web mining techniques could be applied to allow researchers to analyse what users like to share or talk about and not merely look at likes, comments, and shares as the only metrics (Peltier et al., 2020 ).
Although Facebook and Twitter are the most used social network by brands, and the most significant part of the literature focuses on these two platforms, researchers should not forget that there are new and emerging social media in different countries (e.g., TikTok, Clubhouse). They already represent a hot topic for practitioners and are calling scholars to define new metrics to measure engagement. Additionally, as the use of social media increased during the COVID-19 pandemic, future research should take this into account to better understand social media engagement across different social media platforms.
Open access funding provided by Università Parthenope di Napoli within the CRUI-CARE Agreement.
Publisher's Note
Springer Nature remains neutral with regard to jurisdictional claims in published maps and institutional affiliations.
Contributor Information
Mariapina Trunfio, Email: ti.eponehtrapinu@oifnurt .
Simona Rossi, Email: [email protected] .
- Abuljadail M, Ha L. Engagement and brand loyalty through social capital in social media. International Journal of Internet Marketing and Advertising. 2019; 13 (3):197–217. doi: 10.1504/IJIMA.2019.102557. [ CrossRef ] [ Google Scholar ]
- Advertising Research Foundation. (2006). Engagement: Definitions and Anatomy . ARF White Paper. https://thearf.org/ . Retrieved 5 May 2021
- Aggrawal N, Arora A. Behaviour of viewers: YouTube videos viewership analysis. International Journal of Business Innovation and Research. 2019; 20 (1):106–128. doi: 10.1504/IJBIR.2019.101692. [ CrossRef ] [ Google Scholar ]
- Aria M, Cuccurullo C. Bibliometrix: An R-tool for comprehensive science mapping analysis. Journal of Informetrics. 2017; 11 (4):959–975. doi: 10.1016/j.joi.2017.08.007. [ CrossRef ] [ Google Scholar ]
- Aswani R, Ghrera SP, Kar AK, Chandra S. Identifying buzz in social media: A hybrid approach using artificial bee colony and k-nearest neighbors for outlier detection. Social Network Analysis and Mining. 2017; 7 (1):1–10. doi: 10.1007/s13278-017-0461-2. [ CrossRef ] [ Google Scholar ]
- Aveyard H. Doing a Literature Review in Health and Social Care: A practical guide. Pennsylvania Plaza New York: McGrow Hill; 2007. [ Google Scholar ]
- Barger V, Peltier JW, Schultz DE. Social media and consumer engagement: A review and research agenda. Journal of Research in Interactive Marketing. 2016; 10 (4):268–287. doi: 10.1108/JRIM-06-2016-0065. [ CrossRef ] [ Google Scholar ]
- Bowden J. The process of customer engagement: A conceptual framework. Journal of Marketing Theory and Practice. 2009; 17 (1):63–74. doi: 10.2753/MTP1069-6679170105. [ CrossRef ] [ Google Scholar ]
- Brodie RJ, Hollebeek LD, Jurić B, Ilić A. Customer engagement: Conceptual domain, fundamental propositions, and implications for research. Journal of Service Research. 2011; 14 (3):252–271. doi: 10.2753/MTP1069-6679170105. [ CrossRef ] [ Google Scholar ]
- Brodie RJ, Ilic A, Juric B, Hollebeek L. Consumer engagement in a virtual brand community: An exploratory analysis. Journal of Business Research. 2013; 66 (1):105–114. doi: 10.1016/j.jbusres.2011.07.029. [ CrossRef ] [ Google Scholar ]
- Buffard J, Papasava A. A quantitative study on the impact of emotion on social media engagement and conversion. Journal of Digital and Social Media Marketing. 2020; 7 (4):355–375. [ Google Scholar ]
- Colicev A, Malshe A, Pauwels K, O’Connor P. Improving consumer mindset metrics and shareholder value through social media: The different roles of owned and earned media. Journal of Marketing. 2018; 82 (1):37–56. doi: 10.1509/jm.16.0055. [ CrossRef ] [ Google Scholar ]
- De Vries NJ, Carlson J. Examining the drivers and brand performance implications of customer engagement with brands in the social media environment. Journal of Brand Management. 2014; 21 (6):495–515. doi: 10.1057/bm.2014.18. [ CrossRef ] [ Google Scholar ]
- Dervis H. Bibliometric analysis using bibliometrix an R package. Journal of Scientometric Research. 2019; 8 (3):156–160. doi: 10.5530/jscires.8.3.32. [ CrossRef ] [ Google Scholar ]
- Dessart L. Social media engagement: A model of antecedents and relational outcomes. Journal of Marketing Management. 2017; 33 (5–6):375–399. doi: 10.1080/0267257X.2017.1302975. [ CrossRef ] [ Google Scholar ]
- Dolan R, Conduit J, Fahy J, Goodman S. Social media: Communication strategies, engagement and future research directions. International Journal of Wine Business Research. 2017; 29 (1):2–19. doi: 10.1108/IJWBR-04-2016-0013. [ CrossRef ] [ Google Scholar ]
- Forrester Consulting. (2008). How engaged are your customers? . Forrester Consuting. http://docplayer.net/9663683-How-engaged-are-your-customers.html . Retrieved 5 May 2021
- Gallup Consulting. (2009). Customer engagement: What’s your engagement ratio? . Gallup Consulting. https://strengthszone.com/wp-content/uploads/2016/01/Customer-Engagement-Ratio-Brochure.pdf . Retrieved 5 May 2021
- Gruner RL, Power D. To integrate or not to integrate? Understanding B2B social media communications. Online Information Review. 2018; 42 (1):73–92. doi: 10.1108/OIR-04-2016-0116. [ CrossRef ] [ Google Scholar ]
- Guidry JPD, Waters RD, Saxton GD. Moving social marketing beyond personal change to social change: Strategically using Twitter to mobilize supporters into vocal advocates. Journal of Social Marketing. 2014; 4 (3):240–260. doi: 10.1108/JSOCM-02-2014-0014. [ CrossRef ] [ Google Scholar ]
- Hallock W, Roggeveen AL, Crittenden V. Firm-level perspectives on social media engagement: An exploratory study. Qualitative Market Research. 2019; 22 (2):217–226. doi: 10.1108/QMR-01-2017-0025. [ CrossRef ] [ Google Scholar ]
- Harrigan P, Evers U, Miles M, Daly T. Customer engagement with tourism social media brands. Tourism Management. 2017; 59 :597–609. doi: 10.1016/j.tourman.2016.09.015. [ CrossRef ] [ Google Scholar ]
- Haumann T, Güntürkün P, Schons LM. Engaging customers in coproduction processes: How value-enhancing and intensity-reducing communication strategies mitigate the negative effects of coproduction intensity. Journal of Marketing. 2015; 79 (6):17–33. doi: 10.1509/jm.14.0357. [ CrossRef ] [ Google Scholar ]
- Hollebeek LD. Individual-level cultural consumer engagement styles: Conceptualization, propositions and implications. International Marketing Review. 2018; 35 :42–71. doi: 10.1108/IMR-07-2016-0140. [ CrossRef ] [ Google Scholar ]
- Hollebeek LD. Developing business customer engagement through social media engagement-platforms: An integrative S-D logic/RBV-informed model. Industrial Marketing Management. 2019; 81 :89–98. doi: 10.1016/j.indmarman.2017.11.016. [ CrossRef ] [ Google Scholar ]
- Hollebeek LD, Conduit J, Brodie RJ. Strategic drivers, anticipated and unanticipated outcomes of customer engagement. Journal of Marketing Management. 2016; 32 (5–6):393–398. doi: 10.1080/0267257X.2016.1144360. [ CrossRef ] [ Google Scholar ]
- Hollebeek LD, Glynn MS, Brodie RJ. Consumer brand engagement in social media: Conceptualization, scale development and validation. Journal of Interactive Marketing. 2014; 28 (2):149–165. doi: 10.1016/j.intmar.2013.12.002. [ CrossRef ] [ Google Scholar ]
- Hollebeek LD, Srivastava RK, Chen T. S-D logic–informed customer engagement: Integrative framework, revised fundamental propositions, and application to CRM. Journal of the Academy of Marketing Science. 2019; 47 (1):161–185. doi: 10.1007/s11747-016-0494-5. [ CrossRef ] [ Google Scholar ]
- Hubspot. (2014). CRM expert Paul Greenberg defines customer engagement . Hubspot. https://blog.hubspot.com/sales/paul-greenberg-defines-customer-engagement . Retrieved 5 May 2021
- Inamdar Z, Raut R, Narwane VS, Gardas B, Narkhede B, Sagnak M. A systematic literature review with bibliometric analysis of big data analytics adoption from period 2014 to 2018. Journal of Enterprise Information Management. 2020; 34 (1):101–139. doi: 10.1108/JEIM-09-2019-0267. [ CrossRef ] [ Google Scholar ]
- Jaakkola E, Alexander M. The role of customer engagement behavior in value co-creation: A service system perspective. Journal of Service Research. 2014; 17 (3):247–261. doi: 10.1177/1094670514529187. [ CrossRef ] [ Google Scholar ]
- Jalal SK. Co-authorship and co-occurrences analysis using bibliometrix r-package: A case study of india and bangladesh. Annals of Library and Information Studies. 2019; 66 (2):57–64. [ Google Scholar ]
- Kalinić Č, Vujičić M. A subnational assessment of hotel social media metrics - The case of Serbia. Geographica Pannonica. 2019; 23 (2):87–101. doi: 10.5937/gp23-19968. [ CrossRef ] [ Google Scholar ]
- Khan G, Mohaisen M, Trier M. The network ROI: Concept, metrics, and measurement of social media returns (a Facebook experiment) Internet Research. 2019; 30 (2):631–652. doi: 10.1108/INTR-07-2018-0346. [ CrossRef ] [ Google Scholar ]
- Khan I, Dongping H, Wahab A. Does culture matter in effectiveness of social media marketing strategy? An investigation of brand fan pages. Aslib Journal of Information Management. 2016; 68 (6):694–715. doi: 10.1108/AJIM-03-2016-0035. [ CrossRef ] [ Google Scholar ]
- Khan ML. Social media engagement: What motivates user participation and consumption on YouTube? Computers in Human Behavior. 2017; 66 :236–247. doi: 10.1016/j.chb.2016.09.024. [ CrossRef ] [ Google Scholar ]
- Kumar V, Aksoy L, Donkers B, Venkatesan R, Wiesel T, Tillmanns S. Undervalued or overvalued customers: Capturing total customer engagement value. Journal of Service Research. 2010; 13 (3):297–310. doi: 10.1177/1094670510375602. [ CrossRef ] [ Google Scholar ]
- Kumar V, Rajan B, Gupta S, Dalla Pozza I. Customer engagement in service. Journal of the Academy of Marketing Science. 2019; 47 (1):138–160. doi: 10.1007/s11747-017-0565-2. [ CrossRef ] [ Google Scholar ]
- Le TD. Influence of WOM and content type on online engagement in consumption communities : The information flow from discussion forums to Facebook. Online Information Review. 2018; 42 (2):161–175. doi: 10.1108/OIR-09-2016-0246. [ CrossRef ] [ Google Scholar ]
- Li J, Kim WG, Choi HM. Effectiveness of social media marketing on enhancing performance: Evidence from a casual-dining restaurant setting. Tourism Economics. 2019; 20 (10):1–20. doi: 10.1177/1354816619867807. [ CrossRef ] [ Google Scholar ]
- Li X, Wu P, Shen GQ, Wang X, Teng Y. Mapping the knowledge domains of building information modeling (BIM): A bibliometric approach. Automation in Construction. 2017; 84 :195–206. doi: 10.1016/j.autcon.2017.09.011. [ CrossRef ] [ Google Scholar ]
- Linnenluecke MK, Marrone M, Singh AK. Conducting systematic literature reviews and bibliometric analyses. Australian Journal of Management. 2020; 45 (2):175–194. doi: 10.1177/0312896219877678. [ CrossRef ] [ Google Scholar ]
- Liu X, Shin H, Burns AC. Examining the impact of luxury brand’s social media marketing on customer engagement: Using big data analytics and natural language processing. Journal of Business Research. 2019 doi: 10.1016/j.jbusres.2019.04.042. [ CrossRef ] [ Google Scholar ]
- Loureiro SMC, Gorgus T, Kaufmann HR. Antecedents and outcomes of online brand engagement: The role of brand love on enhancing electronic-word-of-mouth. Online Information Review. 2017; 41 (7):985–1005. doi: 10.1108/OIR-08-2016-0236. [ CrossRef ] [ Google Scholar ]
- Malthouse EC, Haenlein M, Skiera B, Wege E, Zhang M. Managing customer relationships in the social media era: Introducing the social CRM house. Journal of Interactive Marketing. 2013; 27 (4):270–280. doi: 10.1016/j.intmar.2013.09.008. [ CrossRef ] [ Google Scholar ]
- Mariani MM, Di Felice M, Mura M. Facebook as a destination marketing tool: Evidence from Italian regional destination management organizations. Tourism Management. 2016; 54 :321–343. doi: 10.1016/j.tourman.2015.12.008. [ CrossRef ] [ Google Scholar ]
- Mariani MM, Mura M, Di Felice M. The determinants of Facebook social engagement for national tourism organizations’ Facebook pages: A quantitative approach. Journal of Destination Marketing and Management. 2018; 8 :312–325. doi: 10.1016/j.jdmm.2017.06.003. [ CrossRef ] [ Google Scholar ]
- Marketing Science Institute. (2020). Research priorities 2020–2022 . Marketing Science Institute. https://www.msi.org/wp-content/uploads/2020/06/MSI_RP20-22.pdf . Retrieved 5 May 2021
- McCoy CG, Nelson ML, Weigle MC. Mining the Web to approximate university rankings. Information Discovery and Delivery. 2018; 46 (3):173–183. doi: 10.1108/IDD-05-2018-0014. [ CrossRef ] [ Google Scholar ]
- McKinsey. (2012). Demystifyng social media . McKinsey. https://www.mckinsey.com/business-functions/marketing-and-sales/our-insights/demystifying-social-media . Retrieved 5 May 2021
- Medjani F, Rutter R, Nadeau J. Social media management, objectification and measurement in an emerging market. Business and Emerging Markets. 2019; 11 (3):288–311. doi: 10.1504/IJBEM.2019.102654. [ CrossRef ] [ Google Scholar ]
- Michopoulou E, Moisa DG. Hotel social media metrics: The ROI dilemma. International Journal of Hospitality Management. 2019; 76 :308–315. doi: 10.1016/j.ijhm.2018.05.019. [ CrossRef ] [ Google Scholar ]
- Muñoz-Expósito M, Oviedo-García MÁ, Castellanos-Verdugo M. How to measure engagement in Twitter: Advancing a metric. Internet Research. 2017; 27 (5):1122–1148. doi: 10.1108/IntR-06-2016-0170. [ CrossRef ] [ Google Scholar ]
- Muntinga DG, Moorman M, Smit EG. Introducing COBRAs: Exploring motivations for brand-related social media use. International Journal of Advertising. 2011; 30 (1):13–46. doi: 10.2501/IJA-30-1-013-046. [ CrossRef ] [ Google Scholar ]
- Oh C, Roumani Y, Nwankpa JK, Hu HF. Beyond likes and tweets: Consumer engagement behavior and movie box office in social media. Information and Management. 2017; 54 (1):25–37. doi: 10.1016/j.im.2016.03.004. [ CrossRef ] [ Google Scholar ]
- Okubo Y. Bibliometric indicators and analysis of research systems: methods and examples. Paris: OECD Publishing; 1997. [ Google Scholar ]
- Osokin N. User engagement and gratifications of NSO supporters on Facebook: Evidence from European football. International Journal of Sports Marketing and Sponsorship. 2019; 20 (1):61–80. doi: 10.1108/IJSMS-11-2017-0115. [ CrossRef ] [ Google Scholar ]
- Oviedo-García MÁ, Muñoz-Expósito M, Castellanos-Verdugo M, Sancho-Mejías M. Metric proposal for customer engagement in Facebook. Journal of Research in Interactive Marketing. 2014; 8 (4):327–344. doi: 10.1108/JRIM-05-2014-0028. [ CrossRef ] [ Google Scholar ]
- Peltier J, Dahl AJ, VanderShee BA. Antecedent consumer factors, consequential branding outcomes and measures of online consumer engagement: Current research and future directions. Journal of Research in Interactive Marketing. 2020; 14 (2):239–268. doi: 10.1108/JRIM-01-2020-0010. [ CrossRef ] [ Google Scholar ]
- Pencarelli T, Mele G. A systematic literature review on social media metrics. Mercati & Competitività 2019; 1 :1–24. [ Google Scholar ]
- Phulwani PR, Kumar D, Goyal P. A Systematic Literature Review and Bibliometric Analysis of Recycling Behavior. Journal of Global Marketing. 2020; 33 (5):354–376. doi: 10.1080/08911762.2020.1765444. [ CrossRef ] [ Google Scholar ]
- Pickering C, Byrne J. The benefits of publishing systematic quantitative literature reviews for PhD candidates and other early-career researchers. Higher Education Research & Development. 2014; 33 (3):534–548. doi: 10.1080/07294360.2013.841651. [ CrossRef ] [ Google Scholar ]
- Popp N, McEvoy C, Watanabe N. Do college athletics marketers convert social media growth into ticket sales? International Journal of Sports Marketing and Sponsorship. 2017; 18 (2):212–227. doi: 10.1108/IJSMS-05-2017-090. [ CrossRef ] [ Google Scholar ]
- Rahman Z, Suberamanian K, Zanuddin H, Moghavvemi S, Bin MdNasir MHN. SNS metrics analysis “A study on fanpage interactive contents” International Journal of Applied Business and Economic Research. 2016; 14 (2):1405–1415. [ Google Scholar ]
- Rahman Z, Suberamanian K, Zanuddin H, Moghavvemi S, Nasir MHNM. Fanpage viral metrics analysis “study on frequently posted contents” Journal of Engineering and Applied Sciences. 2017; 12 (16):4039–4046. [ Google Scholar ]
- Rather RA, Hollebeek LD, Islam JU. Tourism-based customer engagement: The construct, antecedents, and consequences. The Service Industries Journal. 2019; 39 (7–8):519–540. doi: 10.1080/02642069.2019.1570154. [ CrossRef ] [ Google Scholar ]
- Rietveld R, Van Dolen W, Mazloom M, Worring M. What you feel, is what you like influence of message appeals on customer engagement on Instagram. Journal of Interactive Marketing. 2020; 49 :20–53. doi: 10.1016/j.intmar.2019.06.003. [ CrossRef ] [ Google Scholar ]
- Rogers R. Digital traces in context| Otherwise engaged: Social media from vanity metrics to critical analytics. International Journal of Communication. 2018; 12 (23):450–472. [ Google Scholar ]
- Rossmann A, Ranjan KR, Sugathan P. Drivers of user engagement in eWoM communication. Journal of Services Marketing. 2016; 30 (5):541–553. doi: 10.1108/JSM-01-2015-0013. [ CrossRef ] [ Google Scholar ]
- Sabate F, Berbegal-Mirabent J, Cañabate A, Lebherz PR. Factors influencing popularity of branded content in Facebook fan pages. European Management Journal. 2014; 32 (6):1001–1011. doi: 10.1016/j.emj.2014.05.001. [ CrossRef ] [ Google Scholar ]
- Schivinski B, Christodoulides G, Dabrowski D. Measuring consumers’ engagement with brand-related social-media content: Development and validation of a scale that identifies levels of social-media engagement with brands. Journal of Advertising Research. 2016; 56 (1):64–80. doi: 10.2501/JAR-2016-004. [ CrossRef ] [ Google Scholar ]
- Segijn CM, Maslowska E, Araujo T, Viswanathan V. Engaging with TV events on Twitter: The interrelations between TV consumption, engagement actors, and engagement content. Internet Research. 2019; 30 (2):381–401. doi: 10.1108/INTR-08-2018-0389. [ CrossRef ] [ Google Scholar ]
- Sitta D, Faulkner M, Stern P. What can the brand manager expect from Facebook? Australasian Marketing Journal. 2018; 26 (1):17–22. doi: 10.1016/j.ausmj.2018.01.001. [ CrossRef ] [ Google Scholar ]
- So KKF, King C, Sparks BA, Wang Y. Enhancing customer relationships with retail service brands: The role of customer engagement. Journal of Service Management. 2016; 27 (2):170–193. doi: 10.1108/JOSM-05-2015-0176. [ CrossRef ] [ Google Scholar ]
- Tranfield D, Denyer D, Smart P. Towards a methodology for developing evidence-informed management knowledge by means of systematic review. British Journal of Management. 2003; 14 (3):207–222. doi: 10.1111/1467-8551.00375. [ CrossRef ] [ Google Scholar ]
- Trunfio M, Della Lucia M. Engaging destination stakeholders in the digital era: The best practice of italian regional DMOs. Journal of Hospitality and Tourism Research. 2019; 43 (3):349–373. doi: 10.1177/1096348018807293. [ CrossRef ] [ Google Scholar ]
- Van Doorn J, Lemon KN, Mittal V, Nass S, Pick D, Pirner P, Verhoef PC. Customer engagement behavior: Theoretical foundations and research directions. Journal of Service Research. 2010; 13 (3):253–266. doi: 10.1177/1094670510375599. [ CrossRef ] [ Google Scholar ]
- Vivek SD, Beatty SE, Dalela V, Morgan RM. A generalized multidimensional scale for measuring customer engagement. Journal of Marketing Theory and Practice. 2014; 22 (4):401–420. doi: 10.2753/MTP1069-6679220404. [ CrossRef ] [ Google Scholar ]
- Vivek SD, Beatty SE, Morgan RM. Customer engagement: Exploring customer relationships beyond purchase. Journal of Marketing Theory and Practice. 2012; 20 (2):122–146. doi: 10.2753/MTP1069-6679200201. [ CrossRef ] [ Google Scholar ]
- Vlachvei A, Kyparissis A. Museums on Facebook wall: A case staudy of Thessaloniki’s museums. Tourismos. 2017; 12 (3):75–96. [ Google Scholar ]
- Vrettos K, Gouscos D. Evaluating the presence of Greek tourism-related public sector entities in online social networks. International Journal of Public Administration in the Digital Age. 2019; 6 (1):15–40. doi: 10.4018/IJPADA.2019010102. [ CrossRef ] [ Google Scholar ]
- Wallace M, Wray A. Critical reading and writing for postgraduates. Sage; 2016. [ Google Scholar ]
- Wang C, Kubickova M. The impact of engaged users on eWOM of hotel Facebook page. Journal of Hospitality and Tourism Technology. 2017; 8 (2):190–204. doi: 10.1108/JHTT-09-2016-0056. [ CrossRef ] [ Google Scholar ]
- Wu J, Fan S, Zhao JL. Community engagement and online word of mouth: An empirical investigation. Information & Management. 2018; 55 (2):258–270. doi: 10.1016/j.im.2017.07.002Get. [ CrossRef ] [ Google Scholar ]
- Yoon G, Li C, Ji Y, North M, Hong C, Liu J. Attracting comments: digital engagement metrics on facebook and financial performance. Journal of Advertising. 2018; 47 (1):24–37. doi: 10.1080/00913367.2017.1405753. [ CrossRef ] [ Google Scholar ]
- Zanini MT, Carbone de Moraes F, Lima V, Migueles C, Lourenco C, Reis Irigaray HA. Soccer and twitter: Virtual brand community engagement practices. Marketing Intelligence and Planning. 2019; 37 (7):791–805. doi: 10.1108/MIP-08-2018-0371. [ CrossRef ] [ Google Scholar ]
To read this content please select one of the options below:
Please note you do not have access to teaching notes, social media platforms and sleep problems: a systematic literature review, synthesis and framework for future research.
Internet Research
ISSN : 1066-2243
Article publication date: 17 March 2021
Issue publication date: 12 July 2021
This study is a systematic literature review (SLR) on prior research examining the impact of the nocturnal use of social media platforms on a user's sleep, its dimensions and its perceptually allied problems. This SLR aims to curate, assimilate and critically examine the empirical research in this domain.
Design/methodology/approach
Forty-five relevant studies identified from the Scopus and Web of Science (WoS) databases were analyzed to develop a comprehensive research profile, identify gaps in the current knowledge and delineate emergent research topics.
Prior research has narrowly focused on investigating the associations between specific aspects of social media use behavior and sleep dimensions. The findings suggest that previous studies are limited by research design and sampling issues. We highlight the imperative need to expand current research boundaries through a comprehensive framework that elucidates potential issues to be addressed in future research.
Originality/value
The findings have significant implications for clinicians, family members and educators concerning promoting appropriate social media use, especially during sleep latency.
- Systematic literature review
- Sleep hygiene
- Nocturnal use of social media
- Social media platforms
Acknowledgements
This research was funded by the Deanship of Scientific Research at Princess Nourah bint Abdulrahman University through the Fast- track Research Funding Program.
Kaur, P. , Dhir, A. , Alkhalifa, A.K. and Tandon, A. (2021), "Social media platforms and sleep problems: a systematic literature review, synthesis and framework for future research", Internet Research , Vol. 31 No. 4, pp. 1121-1152. https://doi.org/10.1108/INTR-04-2020-0187
Emerald Publishing Limited
Copyright © 2021, Emerald Publishing Limited
Related articles
All feedback is valuable.
Please share your general feedback
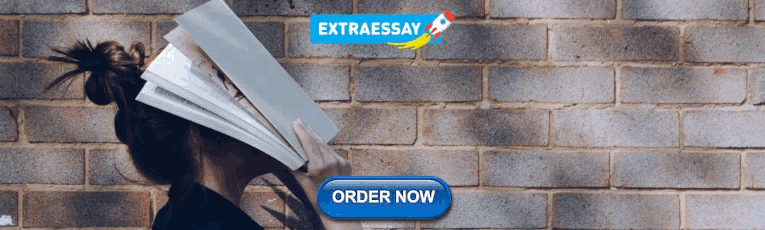
Report an issue or find answers to frequently asked questions
Contact Customer Support

An official website of the United States government
The .gov means it’s official. Federal government websites often end in .gov or .mil. Before sharing sensitive information, make sure you’re on a federal government site.
The site is secure. The https:// ensures that you are connecting to the official website and that any information you provide is encrypted and transmitted securely.
- Publications
- Account settings
- My Bibliography
- Collections
- Citation manager
Save citation to file
Email citation, add to collections.
- Create a new collection
- Add to an existing collection
Add to My Bibliography
Your saved search, create a file for external citation management software, your rss feed.
- Search in PubMed
- Search in NLM Catalog
- Add to Search
Exploring the Usage of Social Media Networking Platforms Among Medical Practitioners: Reasons, and Proposed Features for Improved Experience
Affiliations.
- 1 MBA (Hospital & Healthcare Management) Symbiosis Institute of Health Sciences, Symbiosis International (Deemed University), Pune, Maharashtra, India.
- 2 Faculty of Medical and Health Sciences, Symbiosis International (Deemed University), Pune, Maharashtra, India.
- PMID: 39162062
- DOI: 10.1080/00185868.2024.2392193
Purpose: This study aims to identify and prioritize the reasons for social media use among medical practitioners to facilitate the selection of the most appropriate social media platforms for healthcare providers. The findings of this study will help address the challenges posed by the wide variety of available social media platforms and assist healthcare providers in choosing platforms that best meet their professional needs.
Design/methodology/approach: This cross-sectional study was conducted in the period of two months, i.e., August and September 2021. This study is based on the AHP (Analytic Hierarchy Process) method of the MCDM (Multi-Criteria Decision-Making) approach. In the first phase, a four-hour brainstorming session was held to identify the medical Practitioner's reasons for social media usage; in the second phase, the identified reasons were linked with the top five social media platforms to determine the prioritized order of preference.
Findings: The top three reasons driving medical practitioners' usage of social media platforms include "Getting an update on current affairs," "Network with industry professionals," and "Healthy Lifestyle and Wellness Promotion." In contrast, the bottom three causes include "Advocacy for causes," "Get Updates about friends and family members," and "Opinions and ideas sharing." The prioritized order of social media platforms is LinkedIn, followed by Instagram, WhatsApp, Facebook, and Twitter.
Practical implications: This study helps solve the medical Practitioner's dilemma of selecting suitable social media platforms that serve their intended functions. In the future, it is expected that the usage of SM platforms will increase for causes such as Institution Branding and Healthcare Issues related Advocacy as well.
Originality/value: Previous research has not adequately identified the reasons driving social media usage by physicians. This study is one of the few studies that has attempted to prioritize reasons for social media usage by physicians and has provided a prioritized list of social media platforms.
Keywords: Analytic Hierarchy Process (AHP); Physicians; Social Media; Social Media Platform Features; Social Media Platform’s.
PubMed Disclaimer
- Citation Manager
NCBI Literature Resources
MeSH PMC Bookshelf Disclaimer
The PubMed wordmark and PubMed logo are registered trademarks of the U.S. Department of Health and Human Services (HHS). Unauthorized use of these marks is strictly prohibited.
This paper is in the following e-collection/theme issue:
Published on 19.8.2024 in Vol 26 (2024)
This is a member publication of Open University
Prevalence of Health Misinformation on Social Media—Challenges and Mitigation Before, During, and Beyond the COVID-19 Pandemic: Scoping Literature Review
Authors of this article:

- Dhouha Kbaier 1 , PhD ;
- Annemarie Kane 2 , PhD ;
- Mark McJury 3 ;
- Ian Kenny 1 , PhD
1 School of Computing and Communications, The Open University, Milton Keynes, United Kingdom
2 Faculty of Arts and Social Sciences, The Open University, Milton Keynes, United Kingdom
3 School of Physical Sciences, The Open University, Milton Keynes, United Kingdom
Corresponding Author:
Dhouha Kbaier, PhD
School of Computing and Communications
The Open University
Walton Hall
Milton Keynes, MK7 6AA
United Kingdom
Email: [email protected]
Background: This scoping review accompanies our research study “The Experience of Health Professionals With Misinformation and Its Impact on Their Job Practice: Qualitative Interview Study.” It surveys online health misinformation and is intended to provide an understanding of the communication context in which health professionals must operate.
Objective: Our objective was to illustrate the impact of social media in introducing additional sources of misinformation that impact health practitioners’ ability to communicate effectively with their patients. In addition, we considered how the level of knowledge of practitioners mitigated the effect of misinformation and additional stress factors associated with dealing with outbreaks, such as the COVID-19 pandemic, that affect communication with patients.
Methods: This study used a 5-step scoping review methodology following Arksey and O’Malley’s methodology to map relevant literature published in English between January 2012 and March 2024, focusing on health misinformation on social media platforms. We defined health misinformation as a false or misleading health-related claim that is not based on valid evidence or scientific knowledge. Electronic searches were performed on PubMed, Scopus, Web of Science, and Google Scholar. We included studies on the extent and impact of health misinformation in social media, mitigation strategies, and health practitioners’ experiences of confronting health misinformation. Our independent reviewers identified relevant articles for data extraction.
Results: Our review synthesized findings from 70 sources on online health misinformation. It revealed a consensus regarding the significant problem of health misinformation disseminated on social network platforms. While users seek trustworthy sources of health information, they often lack adequate health and digital literacies, which is exacerbated by social and economic inequalities. Cultural contexts influence the reception of such misinformation, and health practitioners may be vulnerable, too. The effectiveness of online mitigation strategies like user correction and automatic detection are complicated by malicious actors and politicization. The role of health practitioners in this context is a challenging one. Although they are still best placed to combat health misinformation, this review identified stressors that create barriers to their abilities to do this well. Investment in health information management at local and global levels could enhance their capacity for effective communication with patients.
Conclusions: This scoping review underscores the significance of addressing online health misinformation, particularly in the postpandemic era. It highlights the necessity for a collaborative global interdisciplinary effort to ensure equitable access to accurate health information, thereby empowering health practitioners to effectively combat the impact of online health misinformation. Academic research will need to be disseminated into the public domain in a way that is accessible to the public. Without equipping populations with health and digital literacies, the prevalence of online health misinformation will continue to pose a threat to global public health efforts.
Introduction
The global adoption of the internet has made health information more accessible, and the development of digital technology has enabled its rapid dissemination. However, the internet has also made possible the dissemination of false and misleading health misinformation and disinformation, with negative consequences, including the potential to exacerbate health inequalities. Health practitioners have found themselves at the forefront of communicating with patients who have taken on board health misinformation in the context of its proliferation on the web. This paper (associated with the study by Ismail et al [ 1 ]) surveyed the current literature concerning online health misinformation to establish the extent and scope of the problem, with special reference to the needs of health practitioners in their efforts to mitigate its impact. Several studies have established useful definitions of the terms misinformation and disinformation and distinctions between them. Misinformation has been defined as information that is not supported by evidence and contradicts the best-supported evidence available [ 2 , 3 ]. Wang et al [ 4 ] made a further distinction between online misinformation and disinformation, in particular on social media platforms. For Wang et al [ 4 ], misinformation is information that is not known to be false and is shared without malice. By contrast, disinformation involves the knowing and sharing of false information with the purpose of causing harm. This paper follows the distinctions of Wang et al [ 4 ] to use the terms misinformation and disinformation as appropriate.
It is important to acknowledge at the outset that digital technology in health and social contexts presents both risks and opportunities for equity among different information audiences [ 5 ]. However, there has recently been a change in the reception and assessment of the role of the internet, social media in particular, among researchers, even predating the COVID-19 pandemic. In the early days of social media, researchers largely identified prosocial and altruistic uses of social media platforms such as Facebook and Twitter by the public. However, considerable disquiet about the impact of social media and its potential for the spread of “fake news” and the amplification of conspiracy theories has displaced the more positive evaluation that was apparent when social media was in its infancy [ 6 ]. In the majority of the current research, there is a view that digital technology, particularly social media, has amplified the problem of health misinformation. The risk most frequently identified, either explicitly or implicitly, is the susceptibility of ordinary users, who may be lacking sophisticated levels of health and digital literacies, to health misinformation. Further risks noted in the literature include disinformation disseminated by organized trolling networks and bots that can be hard to distinguish from human users. The recognition of these risks underpins an emerging policy discourse about the threat of health misinformation, particularly the role of social media in its spread, in which health information and misinformation has become a politicized issue. From one policy perspective, there is an assumption that social media users are vulnerable, even passive, recipients of health misinformation rather than reflective interpreters of the available information. The corollary of this is that correcting misinformation with authoritative knowledge will solve the problem. However, a survey of the literature suggested that neither assumption fully expresses the complexity of how health misinformation is disseminated, received, and used via the internet. This may be because although there is a growing body of evidence on the extent of online health misinformation, there is much less research about what users do with health misinformation, why users consume health misinformation, and why (and which) people believe health misinformation [ 7 - 9 ].
In this scoping review, we reviewed the current state of knowledge regarding the prevalence of online misinformation before and during the COVID-19 pandemic and the impact that has on users’ understanding of health information. We considered this context with special reference to patients’ understanding, health practitioners’ practice in response to that, and policy makers’ concerns. The pressures and distractions that health professionals face in attempting to mitigate the impacts of online health misinformation are discussed in relation to patients’ health and digital literacies and the politicization of health information and misinformation.
Information Sources
We conducted a comprehensive literature search to identify relevant studies that explored health misinformation on social media platforms. The search was conducted across multiple electronic databases, including PubMed, Scopus, Web of Science, and Google Scholar.
The search terms included a combination of relevant keywords and phrases, including “health misinformation,” “social media,” “online health communities,” and “COVID-19 pandemic.” The search was not limited by publication date. Detailed search strategies are provided in Multimedia Appendix 1 .
Study Selection
Our study selection process followed a scoping approach, where we aimed to identify and include studies that provided insights into the prevalence and challenges of health misinformation on social media platforms. Initially, 2 researchers independently screened titles and abstracts of the identified articles to determine their relevance. Articles that did not meet the inclusion criteria were excluded at this stage.
Inclusion Criteria
Articles were included if they discussed health misinformation on social media, addressed the challenges posed by health misinformation, or were relevant to the period before, during, and after the COVID-19 pandemic.
Any disagreements between the 2 researchers were resolved through discussion and consensus. Full-text articles were then retrieved for the remaining studies, and a further assessment of eligibility was conducted based on the same inclusion criteria.
Data Extraction
We gathered information on (1) study objectives, (2) research methods, (3) findings, and (4) key themes related to health misinformation. This process was performed independently by 2 researchers, and any discrepancies were resolved through discussion.
Data Synthesis and Analysis
We adopted a scoping review content analysis approach to analyze the data extracted from the selected articles. The analysis process involved identifying key themes and patterns related to health misinformation on social media. The content analysis allowed us to gain a deeper understanding of the challenges posed by health misinformation and the strategies for its mitigation, both before and during the COVID-19 pandemic.
Results of Search
In our article selection process ( Figure 1 ), we initiated our search by identifying a total of 4563 articles from various databases. Following the removal of duplicates, 1295 articles were excluded, leaving us with 3268 unique articles. Subsequently, these articles underwent an initial screening, which involved evaluating their abstracts and titles, resulting in the exclusion of 2635 articles that did not align with our inclusion criteria. Further scrutiny was applied during full-text screening, which was conducted on 633 articles. Among these, 563 articles were found ineligible due to reasons such as not meeting the inclusion criteria (n=378 articles), being classified as literature reviews, editorials, or letters (n=174 articles), or the full texts being inaccessible (n=11 articles). A total of 70 articles were ultimately included in this scoping review.

Characteristics of Included Documents (n=70)
The majority (65/70, 93%) of documents were published in the last 10 years and originated predominantly in North America (42/70, 60%), Europe (19/70, 27%), and Asia (11/70, 16%). The funding sources were mainly public (61/70, 87%). The documents were classified as original research papers (38/70, 54%), subjective “opinion” articles (editorials, viewpoints, commentaries, and letters to the journal; 11/70, 16%), and knowledge syntheses or reviews (9/70, 13%) which included systematic reviews (n=6), descriptive reviews (n=2), and 1 integrated theoretic review.
Extent and Impact of Health Misinformation Disseminated Across a Range of Outlets
This section will review the literature concerning the extent and impact of the problem of health misinformation, including the spread of antivaccination discourse. In a study by Wood et al [ 10 ] among health practitioners in North Carolina, 94.2% of the respondents reported encounters with patient health misinformation within the previous year. While the sources of this misinformation were not broken down and identified by Wood et al [ 10 ], several other studies linked patient health misinformation to the prevalence of health misinformation on social media sites, identifying the latter as a significant problem [ 4 , 11 - 15 ]. There is a growing consensus among researchers, health professionals, and policy makers about the need to confront, challenge, and even prevent the online dissemination of health misinformation [ 16 ]. Since the emergence of online social networks, users have increasingly sought and shared health information on social media sites. It is estimated that around 70% of adult internet users search health matters on the web. With the emergence of social media platforms, there has been a rise in “peer-to-peer health care,” through which individuals seek and share health information, forming online health communities with others who have similar health concerns [ 3 ]. In addition, health organizations and health professionals are increasingly using social media to disseminate and promote health information and advice. The opportunities for sharing and promoting good health information via the internet are evident, and it is important to acknowledge that in online health communities, users share experiences and receive and give different kinds of support, including emotional support, to cope with specific health conditions. However, the medium has also enabled the dissemination of health misinformation, and the prosocial aspects of sharing are also likely to involve the sharing of misinformation, putting the health of users at risk [ 3 ].
Misinformation Spreads on Social Media
There is a high degree of consensus among researchers that mainly because of the increasing popularity of social media, the internet has become a space for the dissemination and amplification of “fake news,” misleading information, and rumor, including health misinformation and antivaccine conspiracy theories [ 17 ]. The COVID-19 pandemic has heightened these concerns, resulting in a proliferation of recent studies and rapid reviews focusing on the online spread of misinformation. Lee et al [ 18 ] proposed that the proliferation of health misinformation during the COVID-19 pandemic became a major public health issue. At the earliest signs of the emerging COVID-19 pandemic, the director-general of the World Health Organization, Tedros Adhanom Ghebreyesus, speaking at the February 2020 Munich Security Conference, expressed concern about the risk of an infodemic of health misinformation disseminated via social media, identifying “vaccine hesitancy” as 1 of the top 10 global health threats [ 19 ]. Bapaye and Bapaye [ 20 ] agreed that the risks of misinformation on social networking sites constitute a global issue, referring specifically to the COVID-19 infodemic.
However, this is not in itself a new problem; longstanding concerns about “fake news” and misinformation in traditional media have been evident since the early decades of the 20th century [ 21 ], and the prevalence of misinformation on internet platforms certainly predates the COVID-19 pandemic. Therefore, because the COVID-19 pandemic has only intensified the concern regarding health misinformation, it might be more appropriate to see the pandemic as symptomatic of, and crystallizing, the challenges of countering health misinformation in the digital age, as the development of digital technology and the internet have brought about profound changes in the capacity of both misinformation and disinformation to spread globally and amplify rapidly [ 4 ].
Suarez-Lledo and Alvarez-Galvez [ 16 ] undertook a review of 69 studies of health misinformation on social media to identify the main health misinformation topics and their frequency on different social media platforms. The studies surveyed used a variety of research methods, including social network analysis (28%), evaluation of content (26%), evaluation of quality (24%), content/text analysis (16%), and sentiment analysis (6%). Suarez-Lledo and Alvarez-Galvez [ 16 ] concluded that the incidence of health misinformation was highest on Twitter, in particular, regarding the use of tobacco and other drugs, with some studies citing 87% of such posts containing misinformation. However, health misinformation about vaccines was also prevalent, with around 43% of posts containing misinformation, with the human papillomavirus vaccine being the most affected. This review by Suarez-Lledo and Alvarez-Galvez [ 16 ] confirmed many of the findings from earlier surveys. For example, in their survey of 57 articles, Wang et al [ 4 ] found that the most frequently discussed topics were regarding vaccination and infectious diseases, including Ebola and the Zika virus. Other topics such as nutrition, cancer, water fluoridation, and smoking were also prevalent. The studies they surveyed had tended to find that a high degree of misinformation on these topics was being shared and liked on social media.
Lee et al [ 18 ] conducted a cross-sectional online survey in South Korea to examine the prevalence of COVID-19 misinformation and the impact of exposure to COVID-19 misinformation on beliefs and behaviors. They found that exposure to COVID-19 misinformation was associated with misinformation belief, which then resulted in fewer preventive behaviors. Therefore, they highlighted the potential of misinformation to undermine global efforts in disease control and argued that public health strategies are needed to combat the proliferation of misinformation. Bapaye and Bapaye [ 20 ] conducted a cross-sectional online questionnaire survey of 1137 WhatsApp users in India. They noted that most research on the prevalence of misinformation in social media has focused on Twitter and Facebook and on the Global North. Measured by age, researchers found that users aged >65 years were the most vulnerable to accepting the veracity of messages containing health misinformation (K=0.38, 95% CI 0.341-0.419) Respondents aged 19 to 25 years displayed much lower vulnerability (K=0.31, 95% CI 0.301-0.319) than those aged >25 years ( P <.05). Measured by occupational category, users employed in nonprofessional occupations had the highest vulnerability (K=0.38, 95% CI 0.356-0.404); this was significantly higher than those of professionals and students ( P <.05). Notably, the vulnerability of health professionals was not significantly different from those of other occupation groups ( P >.05).
The authors concluded that in a developing country, WhatsApp users aged >65 years and those involved in nonprofessional occupations are the most vulnerable to false information disseminated via WhatsApp. Crucially, they noted that health care workers, who might be expected by laypersons to have expert knowledge, were as likely to be vulnerable to health misinformation as other occupation groups.
Antivaxxer Spread Before, During, and Beyond the COVID-19 Pandemic
Much of the current unease from researchers, understandably, centers on health misinformation about vaccines in the wake of the COVID-19 pandemic. In particular, there is concern about the growth and spread of so-called antivaxxer misinformation and beliefs. In 2019, the United States had its biggest measles outbreak in 30 years, with most cases involving people who had not been vaccinated. Hotez [ 22 ] claimed that much of the reason for the growth of antivaccine beliefs is because of a campaign of misinformation. He argued that social media sites are meeting places for the sharing of antivaccine views. To evade social media platforms’ automated moderation tools, which tend to focus on words, several antivaxxer groups, including one with around 250,000 members, began using visual codes, such as the carrot emoji, to hide antivaxxer content.
However, some of the misinformation has gained credibility because it has come from sources that laypersons would expect to be trustworthy. For example, in 1998, the British medical journal The Lancet published a paper by Dr Andrew Wakefield claiming a link between the measles, mumps, rubella vaccine and the onset of autism spectrum disorder. Wakefield’s paper was later rebutted, and an overwhelming body of evidence now refutes its conclusions [ 23 ]. However, despite long being discredited, Wakefield’s claims have remained a part of the antivaccine discourse. The persistence of the antivaccination narrative demonstrates the power of such discourses even in the face of evidence to challenge them.
Although strong antivaccine beliefs, and the more ambivalent attitude of vaccine hesitancy, have been around as long as there have been vaccines, until recent decades, they were on the margins. However, evidence supports the claim that they have been gaining momentum in the United States and Europe.
A survey by Skafle et al [ 24 ] aimed to synthesize the results from 19 studies in which the effect of social media misinformation on vaccine hesitancy was measured or discussed. The authors noted that the “vast majority” of studies were from industrialized Western countries. Only 1 study contained misinformation about autism as a side effect of COVID-19 vaccines. Nevertheless, the studies implied that information spread on social media had a negative effect on vaccine hesitancy and uptake. The conclusions from Skafle et al [ 24 ] were supported by data from online polling agencies. For example, a US YouGov poll from May 2020 found that only 55% of respondents would definitely take a COVID-19 vaccine if one were to become available, whereas 19% of respondents said that they would refuse and 26% were still undecided [ 25 ].
While much of the research about online vaccine discourse comes from the United States, there is also evidence that vaccine hesitancy has risen elsewhere. For example, in an Ipsos-MORI survey taken in December 2020, only 40% of respondents in France said they would take a COVID-19 vaccine, a figure symptomatic of a steep and swift decline in vaccine confidence in France [ 26 ]. However, interestingly, the same Ipsos-MORI poll indicated a rise in vaccine confidence among respondents in the United States since the earlier YouGov poll, cited earlier, by approximately 10% to 65%, and respondents in the United Kingdom expressed a still higher willingness to take a COVID-19 vaccine at approximately 77%. It is notable that in the United States and United Kingdom, the Ipsos-MORI results came after a period of intermittent lockdowns. The contrast with the results from France is, nevertheless, striking.
Understanding the Challenges Surrounding Health Misinformation
Here, we consider the challenges created by health misinformation on the web: (1) the role played by malicious actors on social media in spreading vaccine disinformation and misinformation and (2) how contextual and cultural issues have different effects on patients’ understanding of what is considered genuine, valid, and authentic health information.
Spread of Health Misinformation on Social Media by Malicious Actors
One strand of research presents the issue of health misinformation as a contest between trolls and bots on the one hand and the voices of trustworthy public health agencies on the other [ 6 ]. This view was supported by Hotez [ 22 ] and Broniatowski et al [ 11 ]. The latter investigated the role of bots and trolls as malicious actors mobilizing vaccination discourse on the web. Their study focused specifically on vaccine-related health messaging on Twitter. Comparing the rates of vaccine-related messages, they found that sophisticated bots and Russian trolls tweeted at higher rates than “average users.” However, the respective content from bots and trolls differed. Whereas bots communicated antivaccine messages, Russian troll accounts provided a seemingly balanced discussion of both provaccination and antivaccination arguments, implying an equivalence between them. The authors argued that amplifying and normalizing a debate is done with the purpose of sowing discord and may lead to undermining public confidence in scientific consensus about the effectiveness of vaccines. Wang et al [ 4 ] acknowledged that it is a challenge to readily distinguish between misinformation and disinformation on the web. They noted that disinformation, such as antivaccine propaganda, can unknowingly be spread by users with genuine concerns [ 4 ], as individuals increasingly seek health and healthy lifestyle information via the internet.
Contextual Factors Influencing the Reception of and Responses to Misinformation: Politicization of the Problem of Health Misinformation
The identification of online trolls, bots, and orchestrated networks as major contributors to the spread of health disinformation and misinformation is now part of mainstream political discourse in the United States. On July 16, 2021, a quarrel broke out between the president of the United States, Joe Biden, and Facebook over the spread of health misinformation on the company’s social media platforms. Speaking to journalists, Biden blamed social media companies for a rise in the number of deaths from COVID-19 among the unvaccinated in the United States. Referring explicitly to Facebook, the president claimed that by allowing the proliferation of health misinformation on its platforms, the company was “killing people” [ 27 ]. Discursive interventions from politicians are never neutral; nevertheless, Biden’s claim about the impact of health misinformation on social media is backed up by many of the studies surveyed for this paper. Facebook immediately rebutted Biden’s accusation by citing their rules, introduced in February 2021, which banned posts that make identifiably false claims about vaccines. Furthermore, Facebook challenged Biden’s claim by asserting that not only has Facebook provided more authoritative information about COVID-19 and vaccines than any other internet site, reaching 2 billion people with such posts, but also that the platform’s vaccine finder tool had been used by more than 3 million Americans.
These figures suggest that although antivaxxer groups find ways to evade detection, their reach may be countered by that of information grounded in current science. A spokesperson for the company said that, far from killing people, “The facts show that Facebook is helping save lives. Period” [ 27 ]. The argument between Biden and Facebook may indeed signal more lay awareness of the problem and echo the concerns of the recent academic research about the dissemination of health misinformation by organized bot and troll networks. Framed as it is, in terms of apportioning the blame for the spread of health misinformation, Biden’s intervention mirrors much of the academic discourse in the United States on the subject. However, it is also symptomatic of the politicization of health misinformation, arguably accelerated by the COVID-19 pandemic, which may thwart evidence-based decision-making. This point was emphasized strongly by Kyabaggu et al [ 5 ]. They framed the problem of pervasive misinformation and disinformation in terms of prime movers and beneficiaries who use it to advance sociopolitical agendas and entrench asymmetrical power, especially in times of uncertainty and threat, such as the COVID-19 pandemic.
Kyabaggu et al [ 5 ] identified government failures to adopt evidence-informed decision-making. They noted that such failures have costs that not only are economic but, crucially, result in poorer health outcomes. They cited as an example the United Kingdom government’s initial prevaccine herd immunity strategy. The intention of this strategy was to allow SARS-CoV-2 to indiscriminately spread to a critical mass to build up population immunity. The authors noted that this was “a particularly concerning example of evidence framing by a government.” Kyabaggu et al [ 5 ] argued that public acceptance of health risk messages and adoption of health-protecting behaviors is highly contingent on the degree to which governments engage in evidence-informed decision-making and communicate this basis effectively. The authors cited several instances of government actors failing to recognize misinformation, disseminating inconsistent or inaccurate information, and not using evidence- and information-based decision-making processes. In recent years, the public policy discourse in the United Kingdom has been veering away from evidence- and information-based decision-making, as politicians have denounced “experts” and their “influence” on policy [ 28 , 29 ].
Finally, Gruzd et al [ 30 ] reported on the impact of coordinated link-sharing behavior to spread and amplify conspiracy-related misinformation. They found a coalition of Facebook accounts that engaged in coordinated link sharing behavior to promote COVID-19 related misinformation. This coalition included US-based pro-Trump, QAnon, and antivaccination accounts.
Contextual Factors Influencing the Reception of and Responses to Misinformation: Health Literacies and Inequality
While the approach of Broniatowski et al [ 11 ], for example, provided a persuasive account of ways in which online health misinformation can be disseminated, there are limitations to this approach, as it did not provide an account of how users respond to the misinformation they encounter. The responses of ordinary users were assumed rather than investigated. Research by Vosoughi et al [ 31 ] provided a caveat to the claim that it is bots that accelerate the spread of misinformation. Their work supported that of Broniatowski et al [ 11 ] in suggesting that bots spread accurate and false information at the same rate. However, Vosoughi et al [ 31 ] also explained that misinformation spreads more rapidly than accurate information because humans, rather than bots, are more likely to spread misinformation [ 31 ]. This claim was further supported by Wang [ 32 ], who suggested that in democracies, where ideas compete for attention in a marketplace, accurate scientific information, which, for the layperson, may be boring or difficult to understand, is easily crowded out by information that is more easily grasped or sensational. Mokhtari and Mirzaei [ 12 ] located this problem specifically in the context of the COVID-19 pandemic. They considered that high mortality from COVID-19, its complexity, and its unknown features resulted in fear, anxiety, and mental pressure among people worldwide. To allay anxiety, people needed health information literacy, defined by the American Library Association as a set of abilities individuals require to recognize when information is needed and to locate, evaluate, and use it effectively [ 33 ]. In addition, Wang [ 32 ] noted that individuals are differentially vulnerable to health misinformation depending on their level of health literacy and that models need to account for this. Mokhtari and Mirzaei [ 12 ] argued that not only information and health literacies but also media literacy are needed. However, studies in the field of health literacy suggest that significant inequalities in health and digital literacies exist.
Researchers have argued that “vastly undervalued and unrecognized” health literacy ought to be considered the best “social vaccine” for preventing COVID-19 in populations [ 5 ]. However, inequalities in health literacy persist. Kyabaggu et al [ 5 ] defined health literacy as encompassing cognitive and social skills that determine individuals’ motivation and ability to access, understand, and use information, including quantitative health risk information, in ways that promote and maintain good health across the life course. They asserted that health literacy is an essential self-management skill and community resource for health, noting that health literacy is positively associated with patients’ involvement in clinical decision-making, willingness to express health concerns, and compliance with clinical guidance. However, despite research demonstrating the importance of health literacy, evidence, even from high-income countries, suggested relatively low levels of health literacy.
Kyabaggu et al [ 5 ] drew a link between health literacy and digital literacy. They suggested that the latter can be understood as health literacy in digital information and technology spaces. They argued that inequalities in health outcomes are exacerbated by a widening digital divide. While digital technology in health and social contexts presents both new risks and opportunities for equity in different information audiences, the ways in which power and privilege operated in the COVID-19 misinformation discourse have not been sufficiently examined. Although socially and economically disadvantaged groups were at a greater risk of exposure to COVID-19, their voices and experiences were often marginalized. In addition, inequalities in access to accurate information are not only related to issues of digital access and literacy but are also situational. For example, disadvantaged individuals may have fewer social connections, and low pay may necessitate longer working hours, militating against individuals having the resources of time and energy to seek out accurate health information and enhance their level of health literacy.
The experiences of specific groups may also go unreported. Quraishi [ 34 ] addressed the impact of misinformation on South Asian students—a fast-growing group in the United States, but one that often receives little media attention. Quraishi [ 34 ] concluded that there is a relationship between the COVID-19 pandemic and students’ academic performance and mental health, as well as an increase in the spread of misinformation regarding COVID-19 public safety guidelines.
Older adults can be a vulnerable group in relation to their comparatively poor digital literacy. Zhou et al [ 35 ] reported on the accuracy of older adults in judging health information credibility. They found that on average, participants only successfully judged 41.38% of health articles. Attractive headlines increased participant credibility judgments on the content, and of the articles shared with others, 62.5% contained falsehoods.
Contextual Factors Influencing the Reception of and Responses to Misinformation: Cultures and Values
Larson and Broniatowski [ 19 ] argued that developing the kinds of literacy advocated by Mokhtari and Mirzaei [ 12 ] and Tully et al [ 2 ] will not address the deep-seated problems they identified. The work by Kyabaggu et al [ 5 ] supported this, and noted that the infodemic crisis is not merely a health and digital literacy issue. Some demographics may be more vulnerable to persuasive communication from broader sociocultural forces. Kyabaggu et al [ 5 ] argued that in considering the social determinants of health, attention must be paid not only to digital and health literacies but also to the ways in which these literacies coexist and interact with other influences. Larson and Broniatowski [ 19 ] suggested that one of the strongest determinants of vaccine confidence or vaccine hesitancy is the level of trust or distrust in the institutions that produce vaccines. A higher level of trust encourages the willingness to accept a high level of risk for a greater benefit. A lower level of trust militates against the acceptance of even a low level of perceived risk. For Larson and Broniatowski [ 19 ], it is not simply the presence of misinformation on social media networks but the social and cultural context of users’ reception of that information that influences responses. Health information operates in a complex and contentious social world. Individuals and communities respond to new information in terms of already developed political, cultural, and social values that influence whether they trust or distrust authority. Populations may be characterized by trust or mistrust of scientific institutions and government. Trust has been eroded through the exposure of fraud, research scandals, and misconduct by major multinational pharmaceutical companies, for example. Communities may be predisposed to distrust the government and its agents depending on their own status or identity. According to Goldenberg [ 36 ], these contexts can make misinformation and health conspiracy theories compelling.
Strategies to Correct Online Misinformation
We address the additional pressures on health professionals in communicating accurate information to mitigate the effects of misinformation, particularly with regard to the additional requirements imposed as a result of the precautions being taken during the pandemic. One area of disagreement in the literature concerns the usefulness of user correction response.
Research Into User Correction Strategies
There is some disagreement as to whether engagement with misinformation by users spreads and reinforces it or even whether extended debates over health misinformation cause users to doubt the possibility of knowable facts. For example, Broniatowski et al [ 11 ] argued that when ordinary users directly confront vaccine-skeptic messages from bots, it only serves to legitimize the “debate.” By contrast, Tully et al [ 2 ] argued that social media users have a role to play in either spreading or stopping the spread of misinformation across platforms. Their research aimed to uncover what factors influenced users’ responses. Tully et al [ 2 ] acknowledged that a range of factors can influence the spread or prevention of misinformation, including the behavior of malicious actors such as bots and trolls; the platform’s terms of service; and content moderation policies. As already noted, while most users are not creators of misinformation, they may spread and amplify it by liking, sharing, or replying. In opposition to the work of Broniatowski et al [ 11 ], Tully et al [ 2 ] argued that the content of engagement is particularly important, as their research suggested that multiple corrections by social media users may be required to reduce misperceptions. However, they claimed that most people simply ignore misinformation when they see it on social media.
Tully et al [ 2 ] noted the promise in mobilizing users to engage in such correction, given the vast numbers of users on these sites, in comparison with professional fact-checkers and health authorities.
They considered whether the tone of a correction would influence perceptions of the credibility of the message. However, despite some mixed evidence, they concluded that overall, the tone was not a significant factor and that neutral, affirmative, and uncivil corrections were all effective at reducing misperceptions. They found that participants were generally unlikely to reply to the misinformation tweet. However, their content analysis of hypothetical replies suggested that when users did reply, they mainly provided correct information, particularly after seeing other corrections. Tully et al [ 2 ] concluded that user corrections offer “untapped potential” in responding to misinformation on social media, but further work is needed to consider how users can be mobilized to provide corrections, given their overall unwillingness to reply. However, a limitation of the experimental approach of Tully et al [ 2 ], acknowledged by the researchers, is that in asking individuals what they would hypothetically do, this may not reflect what they actually do in a real social media setting, especially in relation to an issue they care more strongly about. Although the experiment gauged attitudes, it did not delve into how strongly these attitudes were held. It is also not clear to what degree corrections were effective at reducing misperceptions and how reductions were measured.
By contrast, the results of experimental studies by Ittefaq [ 37 ] and Mourali and Drake [ 38 ] suggested that correcting misinformation is by no means a straightforward proposition. They noted the previous research on rebuttal, which suggested that properly designed corrections can mitigate the effects of misinformation. However, such studies have tended to compare responses to misinformation followed by correction with responses of a control group that receives no correction or receives an alternative correction. Mourali and Drake [ 38 ] argued that this static approach misses the dynamic nature of social media debate. They noted that the correction of misinformation is generally followed up with a rebuke by the original poster, inciting further correction and prolonged back-and-forth debate. Mourali and Drake [ 38 ] cited previous studies showing that exposure to conflicting information about health topics, including mammography, nutrition, and the human papillomavirus vaccine, may increase confusion and negative attitudes toward that particular health topic. The researchers found that initial exposure to misinformation had a negative impact on attitudes and intentions toward masking, consistent with previous studies that concluded that exposure to misinformation negatively impacts attitudes and intentions toward behaviors favored by science. Also consistent with previous research, they found that the first correction of the false claim improved attitudes and intentions toward masking. The authors suggested that this effect is partially explained by a decrease in the perceived strength of the argument underlying the false claim. However, this initial improvement diminished on further exposure to false claims and refutation attempts. This finding confirmed their hypothesis that extended exposure to false claims and refutation attempts appears to weaken belief in the possibility of objective knowledge, leading to less positive reactions toward masking as a science-based behavior. They concluded that the level of exposure to contradictory information needs to reach a certain threshold before it affects perceived truth objectivity. However, although people are more likely to share misinformation when its content is consistent with their existing beliefs or when its message is simple, direct, or sensational, correcting misinformation does reduce its likelihood of being shared on social media, an effect that persists even after multiple exposures.
Mourali and Drake [ 38 ] noted that each social media platform exhibits particular interaction norms, which may impact how users interpret the conversation. As their study was limited to a single platform, Reddit, and the debate was restricted to 4 exchanges between only 2 protagonists, the researchers acknowledged that these aspects limit the generalizability of the results. They suggested that future research could attempt to replicate their findings on different social media platforms, and to include more than 2 protagonists and more than 4 exchanges. They noted further that although extended debates are common on social media, it is not known how frequently they occur, echoing the comments by Suarez-Lledo and Alvarez-Galvez [ 16 ] that the extent of misinformation is not clear.
In contrast to the fairly sanguine view of Tully et al [ 2 ] about the potential of users to spread corrective information, Mourali and Drake [ 38 ] problematized the position, pointing to the potential for more complex and uncertain outcomes, whereas Larson and Broniatowski [ 19 ] argued that although the importance of correcting misinformation, item by item, should not be diminished, only if underlying issues driving misinformation are addressed can, for example, long-term vaccine confidence in populations be sustained. They argue that simply responding to misinformation with factual corrections is not likely to reverse the dissent that has been evident among antivaxxers or to necessarily persuade the more ambivalent vaccine-hesitant individuals. They identified deeper social and cultural issues at play, which have been discussed in this paper in the previous sections.
Research Into Effective Models to Accomplish the Automatic Detection of Health Misinformation in Online Health Communities
Here, we consider examples of research into the automatic detection of health misinformation in online health communities. Zhao et al [ 3 ] began from the premise that there is a vast amount of health misinformation, creating a challenge for health communities in identifying misinformation. Rather than relying on users’ ability to correct misinformation, they proposed that there is a need for an effective model to achieve automatic detection of health misinformation in online health communities. This view was also put forward by Weinzierl and Harabagiu [ 39 ]. Focusing specifically on COVID-19 vaccine misinformation, they argued that automatic detection of misinformation on social media is an essential first step in delivering interventions designed to address vaccine hesitancy.
Zhao et al [ 3 ] identified much of the existing analysis as concentrating on the linguistic features of communications only. They wanted to examine the underresearched area of whether integrating user behavioral features with linguistic features, sentiment features, and topic features could effectively distinguish misinformation from accurate information in online health communities. Their study combined the aforementioned features to build a detection model targeting misinformation in online health communities’ contexts. The behavioral features targeted were discussion initiation, interaction engagement, influential scope, relational mediation, and informational independence. Descriptions of these behavioral features are reproduced in Table 1 .
Behavioral feature | Measurement | Description |
Discussion initiation | The number of threads a user created | To reflect the activity of a user in terms of initiating new discussions |
Interaction engagement | The number of replies and the number of replies to a reply a user created | To reflect the activity of a user in terms of interacting with other users |
Influential scope | Degree centrality | To reflect the potential communication ability of a user |
Relational mediation | Betweenness centrality | To assess the potential of a user for the control of communication in the community |
Informational independence | Closeness centrality | To assess the ability of a user to instantly communicate with others without going through many intermediaries |
The authors tested their detection model on a data set collected from a real online health community, selecting as their data source Zibizheng Ba, an autism forum on the Baidu Tieba online health community site hosted by the Chinese web service Baidu. Baidu Tieba claims to be one of the largest interest-based discussion platforms in China. Users can generate topic-based discussion forums on the platform, share information, and make friends with other users. Posts on Baidu Tieba are indexed by Baidu, China’s most popular search engine, so users can readily find misinformation when searching for health-related information through the search engine. The authors developed a python-based web crawler to collect data from the forum. To train the health misinformation detection model, 5000 records were sampled from the whole data set by stratification according to 3 types of records (ie, thread, reply, and reply to reply) using stratified sampling methods. Therefore, the constituent types of the records (ie, thread, reply, and reply to reply) in the sample data set were consistent with the composition of the whole data set.
The researchers applied the elaboration likelihood model (ELM). The model, originally developed by Petty and Cacioppo [ 40 ] to explain attitude change, has been used extensively in advertising to try to influence consumers.
Overall, 4 types of misinformation were identified through their coding analysis, and the model correctly detected about 85% of the health misinformation. Their results also indicated that behavioral features were more informative than linguistic features in detecting misinformation. The authors concluded that their results not only demonstrated the efficacy of behavioral features in health misinformation detection but also offered both methodological and theoretical contributions to misinformation detection by integrating the features of messages as well as the features of message creators. Others have also highlighted the problems posed by misleading visual information [ 41 ].
It is worth noting that during the pandemic, the UK National Health Service (NHS) began using Twitter to promote provaccine messaging, which closely follows a combination of the features suggested by Zhao et al [ 3 ]. When users searched for the term “vaccine” or related terms, the top post was a message prominently displaying the NHS logo, identifying it as reputable and trustworthy. The tweets contained links to NHS websites providing information about vaccines and COVID-19. The posts differed in linguistic content and visual design. For example, one featured only written text on a white background and stated in bold, “Know the facts.” Another featured a large image of a happy minority ethnic family, washing dishes together, with the message that the COVID-19 vaccine decreases household transmission by up to half. The contrasting designs suggest that the message was targeted specifically to users’ timelines. It was also apparent that elements of ELM were being applied, combining the features identified by Zhao et al [ 3 ] in different ways.
Weinzierl and Harabagiu [ 39 ] adopted a different method than Zhao et al [ 3 ], reversing the more commonly used classification approach. The authors of each study claimed strong results in identifying health misinformation on social media platforms. However, Nabożny et al [ 42 ] argued that the current automatic systems for assessing the credibility of health information are not sufficiently precise to be used without supervision by human medical expert annotators.
Barve and Saini [ 43 ] have reported on their use of automated fact-checking using a coded content similarity measure (CSM). In this approach, the CSM showed improved accuracy (91.06%) compared to the accuracy of the Jaccard similarity measure (74.26%). Further, the algorithmic approach outperformed the feature-based method.
Neither Zhao et al [ 3 ] nor Weinzierl and Harabagiu [ 39 ] recorded what happens when misinformation is detected. Research from Broniatowksi et al [ 44 ] suggested that once detected, steps taken by social media platforms such as content removal or deplatforming may not be effective in stemming the spread of misinformation and may even be counterproductive. Social media platforms use a combination of “hard” and “soft” content remedies to reduce the spread of health misinformation. Soft remedies include warning labels attached to content and downranking of some content in web searches, whereas hard remedies include content removal and deplatforming of accounts. Hard remedies are controversial and have given rise to accusations of censorship. For the authors, short-term evidence for the effectiveness of hard remedies is in any case mixed, and long-term evidence is yet to be examined. Their study focused on Facebook and found that while hard remedies did reduce the number of antivaccine posts, they also produced unintended consequences. Provaccine content was removed, and engagement with the remaining antivaccine content repeatedly recovered to prepolicy levels. Worryingly, this content became more misinformative, more politically polarized, and more likely to be seen in users’ news feeds. The authors explain these results as a product of Facebook’s architecture, which is designed to promote community formation. Members of communities dedicated to vaccine refusal seek out misinformation. To meet this demand, and to circumvent content moderation efforts, antivaccine content producers post links to external sources of misinformative content, such as Bitchute, Rumble, Gab, and Telegram, in lieu of more mainstream platforms that had implemented similar content removal policies (eg, YouTube and Twitter). Broniatowski et al [ 44 ] argued that Facebook’s policy reduced the number of posts in antivaccine venues but was not successful in inducing a sustained reduction in engagement with antivaccine content, including misinformation. The authors noted that alternative platforms often host politically extreme right-wing content. Therefore, they argued that Facebook’s content removal policies may have the unintended consequence of radicalizing their audiences, and their findings suggested the need to address how social media platform architecture enables community formation and mobilization around misinformative topics when managing the spread of online content.
These studies advocate for the automatic detection of health misinformation. However, work that calls into question the ability of automatic detection to operate without human intervention has also been discussed. In addition, there are questions raised in the literature about what should be done when misinformation is detected and concerns about whether content removal or deplatforming of accounts are the most effective ways to reduce the spread of health misinformation or may even be counterproductive.
The Roles of Health Practitioners
The discussion so far has highlighted the complex and multifaceted dimensions of the context of online health misinformation in which health practitioners must operate. As noted in our introduction, a study of health practitioners in North Carolina found that nearly 95% had encountered patient health misinformation within the previous year [ 10 ]. There is very little research on the amount or effectiveness of training received by health professionals to prepare them for engaging with patients about health misinformation. Wood et al [ 10 ] found that most respondents had not received relevant training despite overwhelmingly reporting encountering health misinformation.
Nevertheless, within the literature, there is no shortage of advice from researchers and health professionals addressed to health practitioners on how to approach and correct health misinformation. This advice stems from both original research studies and reviews of best practices featured in peer-reviewed medical and health journals. Such advice centers on the need for health practitioners to understand misinformation and how to address it. Health practitioners are advised of the need to be aware of health myths and urged to dismantle them in providing accurate health guidance [ 45 , 46 ]. Practitioners are further advised that misinformation and pseudoscience are appealing to those seeking certainty because they present information in absolutes, whereas medical science is often ambiguous and contingent. Health practitioners are also encouraged to learn how to message more clearly and to mimic the strategies of misinformation [ 45 ]. One study recommends that “practitioners familiarize themselves with the tools of scientific enquiry and consider the pros and cons of various conspiracy evaluation guidelines” [ 47 ]. Thompson [ 48 ] reports on the activity of health professional influencers and pedagogues in combating misinformation. However, the effectiveness of such social media influencers who are also health professionals remains unclear. At the same time, there is some acknowledgment in this body of literature that misinformation cannot simply be offset with facts, confirming the challenges, discussed earlier, of simply engaging in online refutation. Addressing misinformation also depends on meeting patients’ emotional needs [ 45 , 49 ].
In this context, the one-to-one patient-provider relationship in the practice setting is perceived as paramount [ 45 ]. As suggested by much of the research, source credibility, or trust, is understood to be the strongest driver of effective correction strategies [ 50 ]. It is argued that health care practitioners have the unique opportunity to guide patients toward high-quality, evidence-based medical information [ 10 ]. However, it is also noted that practitioners will need patience in their efforts to persuade patients to abandon strongly held self-beliefs, however harmful. Doing so may mean patients relinquishing membership of online communities that have become integral in their lives and even their identities. As noted earlier, belief in misinformation is often persistent in the face of evidence. Success is more likely when individuals are encouraged to reexamine their information sources, alongside new information providing additional context, rather than simply characterizing the individual’s beliefs as wrong [ 51 ]. Kyabaggu et al [ 5 ] commented that good health communication needs to be tailored to the underlying cause of the misinformation problem, and efforts should be made to take on board inequalities within populations to create accurate, low-barrier, targeted health risk messaging. Skafle et al [ 24 ] contended that to challenge misconceptions, false claims need to be openly addressed and discussed with both cultural and religious awareness in mind. Guidance for practitioners noted that while responding to patient questions about alternative or unproven therapies may become laborious, a strong bond of trust between health practitioner and patient gives a patient a feeling of being supported and increases their adherence to treatment [ 52 ]. Rather than waiting for patients to raise misinformation issues, health care practitioners are advised to anticipate and proactively address potential misinformation and myths with patients. For example, the mortality rate for pediatric cancer has risen during the COVID-19 pandemic because of delayed access to medical care, but misinformation related to COVID-19 may also be a contributing factor [ 53 ]. The literature highlights the challenge of navigating the information and misinformation and the need for health practitioners to communicate with their patients more effectively. However, such efforts are not always successful. Some of the factors that may prevent effective communication of good health information have already been raised in this paper. They are revisited and discussed in the next section, along with other stressors for health practitioners.
Stressors for Health Practitioners
Challenges for health practitioners include time pressures and the additional burdens placed on them during the COVID-19 pandemic. These additional pressures add to the issues health practitioners face in trying to mitigate the impact of misinformation. The following is a brief overview of these issues.
On the one hand, administrative burdens placed on practitioners frequently deny them time for dialogue with their patients [ 52 ]. On the other, in different contexts, practitioners may be coping with a lack of proper facilities; poor infrastructure for patient care; insufficient or ineffective personal protective equipment; lack of awareness among the general population; poor compliance with preventive methods; and the fear of being infected with the virus, as they too are exposed to misinformation. During the COVID-19 pandemic, health practitioners were considered more vulnerable than other workers to developing psychological problems and other stress-related disorders, as they treated patients confirmed with COVID-19 while also dealing with misinformation [ 54 ].
As noted above, practitioners are recommended to invest in developing high levels of patient trust and to proactively correct health misinformation. However, recommendations presuppose that health practitioners necessarily have the resources to do these things well. Some of the materials produced to educate patients are not always reliable or evidence based, resulting ultimately in a loss of trust on the part of patients [ 52 ]. In addition, as noted previously, health practitioners themselves are not necessarily immune from accepting health misinformation as credible. Evidence about the level of knowledge and understanding of COVID-19 among practitioners reveals its unevenness. A study of dentists and oral health practitioners’ knowledge about COVID-19 suggested that their knowledge was at a relatively high level [ 55 ]. By contrast, a study of 310 eye care professionals in Nepal revealed some knowledge but also some acceptance of misinformation. Symptoms of COVID-19 were known to 94% of participants, but only 49% of participants were aware of how the disease is transmitted. More significantly, 41% of participants believed that the consumption of hot drinks helps to destroy the virus, in contradiction to World Health Organization information. The mean overall “knowledge” performance score, as measured by the benchmarks set by the researchers, was 69.65% [ 56 ].
A qualitative study to investigate primary health care practitioners’ perceptions and understanding of the COVID-19 pandemic was conducted in KwaZulu-Natal, South Africa. The study collected data from 15 participants at 2 different clinics situated in rural KwaZulu-Natal. Participants comprised nurses, physiotherapists, pharmacists, community caregivers, social workers, and clinical associates. Data were collected through individual, in-depth face-to-face interviews using a semistructured interview guide. The participants reported prepandemic and pandemic experiences of fear or denial. There was a perception of poor preparation for the COVID-19 outbreak. The findings also revealed participants’ misperceptions regarding the nature of the COVID-19 pandemic. Researchers concluded that respondents’ misunderstandings regarding the pandemic were primarily a result of misinformation found on social media [ 57 ].
The discussion in this section so far has highlighted the significant potential of health practitioners in mitigating the impact of online health misinformation. However, it has also underlined factors that may militate against health practitioners’ ability to do so effectively. Not least of these is the issue of health practitioners’ own knowledge, which coexists with other stressors for health practitioners in combating misinformation. The discussion will now consider health information management (HIM) as a tool for supporting health practitioners’ knowledge base as one element in a multifaceted strategy for combating misinformation on the web.
HIM as a Mitigation Strategy
We have seen there is a need for health practitioners to be supported with evidence-based knowledge that they can share with patients. Kyabaggu et al [ 5 ] argued that the COVID-19 pandemic has demonstrated that in an infectious health crisis, the gathering of accurate and reliable data to assist with the public health response is essential. They highlighted the importance of HIM professionals in supporting contact tracing and syndromic surveillance, as well as in mapping and forecasting health data. They noted that the generation of health information supports the continuum of care and the setting of targets and indicators and aids the planning, monitoring, and evaluation of health programs locally and globally. The health information produced also underpins the development of equitable, efficient, and accessible health care systems, contributing to improving public health initiatives and outcomes. Kyabaggu et al [ 5 ] emphasized the importance of an area of HIM, currently in its early stages, that deals with gathering and identifying evidence about the structural inequalities that underlie the disparities in vulnerability to health misinformation discussed in this paper. The collection of rich, high-quality information, including patient-reported experience, outcome measures, and culturally appropriate identity data, can enable health practitioners and public health advisers serving the most disadvantaged and underrepresented communities to use more tools of advocacy for patients.
The authors noted that advances in technology, including artificial intelligence, have the potential to relieve some of the pressures and constraints on health practitioners working on the front line during crises such as the COVID-19 pandemic, allowing more time for one-to-one engagement with patients. Kyabaggu et al [ 5 ] advocated for the content expertise of health information managers to serve health practitioners by delivering patient-facing information triaging services; constructing user-friendly knowledge representations, such as data visualizations; and developing information interpretation tools, such as decision aids, plain language summaries, and supplementary explanatory information and metadata. Kyabaggu et al [ 5 ] identified the interdisciplinary underpinnings of HIM as essential in contributing to the educational, informational, and decision-making support for addressing current and future infodemic management crises.
Summary of Results
Within the literature, there is a consensus that there exists a significant problem of online health misinformation disseminated via the internet on social network platforms, often by online health communities. It is apparent that while users seek trustworthy sources of health information, they are unequally equipped to assess its credibility. This is partly because some groups lack sufficient levels of health and digital literacies, which may be exacerbated by concomitant social and economic inequalities. Reception of, and response to, online health misinformation is also shaped by users’ cultural contexts, values, and experiences, which may hinder trust in scientific institutions and governments. Evidence suggests that some demographics are more vulnerable to accepting health misinformation as credible and that health practitioners are unevenly prepared in the context of new global health crises, such as the COVID-19 pandemic. Furthermore, the evidence of disparities in positive and negative attitudes toward vaccination highlights a need to pay specific attention to regional and national settings, even in the current global context. Preexisting levels of local trust in vaccine providers may be a significant factor to consider. While the validity and reliability of YouGov polls are limited, nevertheless, the data from an admittedly narrow range of sources suggests that vaccine confidence may have become more fluctuating and potentially vulnerable to destabilization in the digital era.
While online mitigation strategies such as user correction and automatic detection may have their uses, their effectiveness is contested, and some studies suggest they may even be counterproductive. Our analysis of the available literature indicates that the effectiveness of these strategies varies and needs further evaluation [ 42 , 58 ]. The issue of online health misinformation is further complicated by the operation of malicious actors and politicization of the issue, particularly during the COVID-19 pandemic, militating against the equitable and trusted dissemination of evidence-based knowledge. The role of health practitioners in this context is a challenging one. Research suggests that on the one hand, they are still best placed, at the front line of care, to combat health misinformation with science-based knowledge and advice. On the other hand, the stressors identified in this review create barriers to their abilities to do this well. Constraints of time and lack of supporting infrastructure add to the knowledge deficit noted earlier. Our review underlines the complexity of the environment in which health practitioners operate and calls for greater support and resources to enable effective mitigation of health misinformation [ 59 ]. Investment in HIM at local and global levels could address all 3 deficits, creating the potential for health practitioners to enhance their capacity to build trust via knowledgeable one-to-one communication with patients.
Limitations
The limitations of this study are the following: First, the constraints of time and space have necessarily limited the scale and scope of the survey. Second, the study of online health misinformation is a growing field, and inevitably, the nature of the issue means that new evidence is emerging at a rapid rate. In particular, new knowledge and further reflection in the wake of the COVID-19 pandemic will continue to shed new light on the subject. Our study acknowledges these limitations and emphasizes the dynamic nature of the field.
Conclusions
Our survey of the literature on online health misinformation has revealed a complex and multifaceted context in which health practitioners must operate. As the world renormalizes following the pandemic, a collaborative global interdisciplinary effort to provide equitable access to timely, accurate, and complete health information will be needed to support health practitioners in combating the impact of online health misinformation. Academic research will need to be disseminated into the public domain in a way that is accessible to the public to counter misinformation and educate populations concerning how science is carried out. Our conclusions drawn from this review stress the urgency of effective strategies and collaborative efforts to mitigate the prevalence and impact of health misinformation on a global scale. Without strategies for equipping populations with the health and digital literacies required to interpret and use information appropriately, the prevalence of online health misinformation will continue to pose a threat to global public health efforts, disproportionately affecting vulnerable and resource-limited populations. Although social media platforms have a responsibility to correct misinformation, governments will need to engage in evidence-informed decision-making and invest in HIM to support frontline health practitioners in their work, enhance population health literacy, and strengthen evidence-informed decision-making at all levels.
Several issues for further investigation arise from the findings of this review. These include the following:
- The long-term impact of COVID-19 vaccine hesitancy
- Whether the COVID-19 pandemic has intensified or diminished information literacy, and the related question of whether the pandemic will incentivize health information literacy
- The effects of social and cultural differences on the long-term traction of future health misinformation
- Whether social and economic inequalities will become less or more pronounced in the face of a global pandemic
- The comparative effectiveness of strategies to enhance populations’ media and digital literacies to facilitate the mitigation of health misinformation and its effects
- The influence of state actors on the propagation of health misinformation on the web
- The extent to which academic research has been disseminated into the public domain in a way that is accessible to the public, and the effectiveness of strategies to do so to counter misinformation and educate populations concerning how science is carried out
Acknowledgments
This research was funded by the School of Computing and Communications at the Open University. It allowed researchers across several faculties to collaborate and build a research team that focused on the experience of health practitioners with misinformation and its impact on their job practice. The authors would also like to thank Tracie Farrell and Nashwa Ismail for their invaluable suggestions and recommendations, as well as their assistance in the article screening process.
Data Availability
The data analyzed in this study are derived from published articles available on Google Scholar. All articles included in the review are cited in the reference list. No additional data or code were collected or generated as part of this study.
Authors' Contributions
The study was conceptualized by DK; funding acquisition was managed by DK; data were curated by DK, AK, MM, and IK; formal analysis was conducted by DK and MM; the investigation was carried out by AK and MM; the methodology was designed by DK and MM; project administration was overseen by DK; resources were provided by DK; supervision was carried out by DK; validation was conducted by DK, AK, MM, and IK; visualization was handled by DK and MM; writing (original draft preparation) was done by DK; and writing (review and editing) was carried out by DK, AK, and MM. All authors reviewed and approved the final version.
Conflicts of Interest
None declared.
Detailed search strategy.
- Ismail N, Kbaier D, Farrell T, Kane A. The experience of health professionals with misinformation and its impact on their job practice: qualitative interview study. JMIR Form Res. Nov 02, 2022;6(11):e38794. [ FREE Full text ] [ CrossRef ] [ Medline ]
- Tully M, Bode L, Vraga EK. Mobilizing users: does exposure to misinformation and its correction affect users’ responses to a health misinformation post? Soc Media Soc. Dec 10, 2020;6(4):205630512097837. [ CrossRef ]
- Zhao Y, Da J, Yan J. Detecting health misinformation in online health communities: Incorporating behavioral features into machine learning based approaches. Inf Process Manage. Jan 2021;58(1):102390. [ CrossRef ]
- Wang Y, McKee M, Torbica A, Stuckler D. Systematic literature review on the spread of health-related misinformation on social media. Soc Sci Med. Nov 2019;240:112552. [ FREE Full text ] [ CrossRef ] [ Medline ]
- Kyabaggu R, Marshall D, Ebuwei P, Ikenyei U. Health literacy, equity, and communication in the COVID-19 era of misinformation: emergence of health information professionals in infodemic management. JMIR Infodemiology. 2022;2(1):e35014. [ FREE Full text ] [ CrossRef ] [ Medline ]
- Sutton J. Health communication trolls and bots versus public health agencies' trusted voices. Am J Public Health. Oct 2018;108(10):1281-1282. [ CrossRef ] [ Medline ]
- Kim S, Capasso A, Ali SH, Headley T, DiClemente RJ, Tozan Y. What predicts people's belief in COVID-19 misinformation? A retrospective study using a nationwide online survey among adults residing in the United States. BMC Public Health. Nov 18, 2022;22(1):2114. [ FREE Full text ] [ CrossRef ] [ Medline ]
- Morgan JC, Cappella JN. The effect of repetition on the perceived truth of tobacco-related health misinformation among U.S. adults. J Health Commun. Mar 04, 2023;28(3):182-189. [ CrossRef ] [ Medline ]
- Nan X, Wang Y, Thier K. Why do people believe health misinformation and who is at risk? A systematic review of individual differences in susceptibility to health misinformation. Soc Sci Med. Dec 2022;314:115398. [ CrossRef ] [ Medline ]
- Wood JL, Lee GY, Stinnett SS, Southwell BG. A pilot study of medical misinformation perceptions and training among practitioners in North Carolina (USA). Inquiry. 2021;58:469580211035742. [ FREE Full text ] [ CrossRef ] [ Medline ]
- Broniatowski DA, Jamison AM, Qi S, AlKulaib L, Chen T, Benton A, et al. Weaponized health communication: Twitter bots and Russian trolls amplify the vaccine debate. Am J Public Health. Oct 2018;108(10):1378-1384. [ CrossRef ] [ Medline ]
- Mokhtari H, Mirzaei A. The tsunami of misinformation on COVID-19 challenged the health information literacy of the general public and the readability of educational material: a commentary. Public Health. Oct 2020;187:109-110. [ FREE Full text ] [ CrossRef ] [ Medline ]
- Schmid P, Altay S, Scherer LD. The psychological impacts and message features of health misinformation - a systematic review of randomized controlled trials. Eur Psychol. Jul 2023;28(3):162-172. [ CrossRef ]
- Southwell BG, Otero Machuca J, Cherry ST, Burnside M, Barrett NJ. Health misinformation exposure and health disparities: observations and opportunities. Annu Rev Public Health. Apr 03, 2023;44(1):113-130. [ FREE Full text ] [ CrossRef ] [ Medline ]
- Westberry C, Palmer XL, Potter L. Social media and health misinformation: a literature review. In: Arai K, editor. Proceedings of the Future Technologies Conference (FTC) 2023, Volume 3. FTC 2023. Lecture Notes in Networks and Systems, vol 815. Cham, Switzerland. Springer; 2023.
- Suarez-Lledo V, Alvarez-Galvez J. Prevalence of health misinformation on social media: systematic review. J Med Internet Res. Jan 20, 2021;23(1):e17187. [ FREE Full text ] [ CrossRef ] [ Medline ]
- Borges do Nascimento IJ, Pizarro AB, Almeida J, Azzopardi-Muscat N, Gonçalves MA, Björklund M, et al. Infodemics and health misinformation: a systematic review of reviews. Bull World Health Organ. Sep 01, 2022;100(9):544-561. [ FREE Full text ] [ CrossRef ] [ Medline ]
- Lee JJ, Kang K, Wang MP, Zhao SZ, Wong JYH, O'Connor S, et al. Associations between COVID-19 misinformation exposure and belief with COVID-19 knowledge and preventive behaviors: cross-sectional online study. J Med Internet Res. Nov 13, 2020;22(11):e22205. [ FREE Full text ] [ CrossRef ] [ Medline ]
- Larson HJ, Broniatowski DA. Why debunking misinformation is not enough to change people's minds about vaccines. Am J Public Health. Jun 2021;111(6):1058-1060. [ CrossRef ] [ Medline ]
- Bapaye JA, Bapaye HA. Demographic factors influencing the impact of coronavirus-related misinformation on WhatsApp: cross-sectional questionnaire study. JMIR Public Health Surveill. Jan 30, 2021;7(1):e19858. [ FREE Full text ] [ CrossRef ] [ Medline ]
- McKernon E. Fake news and the public. Harper's. Oct 1925. URL: https://harpers.org/archive/1925/10/fake-news-and-the-public/ [accessed 2024-07-30]
- Hotez P. The physician-scientist: defending vaccines and combating antiscience. J Clin Invest. Apr 29, 2019;129(6):2169-2171. [ FREE Full text ] [ CrossRef ] [ Medline ]
- Dyer C. Lancet retracts Wakefield's MMR paper. BMJ. Mar 02, 2010;340:c696. [ CrossRef ] [ Medline ]
- Skafle I, Nordahl-Hansen A, Quintana DS, Wynn R, Gabarron E. Misinformation about COVID-19 vaccines on social media: rapid review. J Med Internet Res. Aug 04, 2022;24(8):e37367. [ FREE Full text ] [ CrossRef ] [ Medline ]
- New Yahoo News/YouGov coronavirus poll: almost 1 in 5 say they won't get vaccinated. Yahoo News. URL: https://www.yahoo.com/news/new-yahoo-news-you-gov-coronavirus-poll-almost-one-in-five-say-they-wont-get-vaccinated-143852222.html [accessed 2024-08-06]
- Peretti-Watel P, Verger P, Raude J, Constant A, Gautier A, Jestin C, et al. Dramatic change in public attitudes towards vaccination during the 2009 influenza A(H1N1) pandemic in France. Euro Surveill. Oct 31, 2013;18(44):20623. [ FREE Full text ] [ CrossRef ] [ Medline ]
- Reuters. ‘They’re killing people’: Biden slams Facebook for Covid disinformation. The Guardian. Jul 21, 2021. URL: https://www.theguardian.com/media/2021/jul/17/theyre-killing-people-biden-slams-facebook-for-covid-misinformation [accessed 2024-07-30]
- Britain has had enough of experts, says Gove. Financial Times. URL: https://www.ft.com/content/3be49734-29cb-11e6-83e4-abc22d5d108c [accessed 2024-07-30]
- US news roundup: 19-25 August. Research Professional News. Aug 25, 2022. URL: https://www.researchprofessionalnews.com/rr-news-usa-2022-8-us-news-roundup-19-25-august/ [accessed 2024-07-30]
- Gruzd A, Mai P, Soares FB. How coordinated link sharing behavior and partisans' narrative framing fan the spread of COVID-19 misinformation and conspiracy theories. Soc Netw Anal Min. 2022;12(1):118. [ FREE Full text ] [ CrossRef ] [ Medline ]
- Vosoughi S, Roy D, Aral S. The spread of true and false news online. Science. Mar 09, 2018;359(6380):1146-1151. [ CrossRef ] [ Medline ]
- Wang Y. Systematic review on the social mechanism of health misinformation dissemination in the internet era. Eur J Public Health. Nov 2018;28(suppl_4):cky213.194. [ CrossRef ]
- Framework for information literacy for higher education. American Library Association. 2016. URL: http://www.ala.org/acrl/sites/ala.org.acrl/files/content/issues/infolit/framework1.pdf [accessed 2024-07-30]
- Quraishi Z. Addressing mental health, misinformation, and religious tensions among South Asian students across California higher education during the COVID-19 pandemic: A qualitative research study. Heliyon. Jun 2023;9(6):e16396. [ FREE Full text ] [ CrossRef ] [ Medline ]
- Zhou J, Xiang H, Xie B. Better safe than sorry: a study on older adults' credibility judgments and spreading of health misinformation. Univers Access Inf Soc. Aug 04, 2022;22(3):1-10. [ FREE Full text ] [ CrossRef ] [ Medline ]
- Goldenberg MJ. Vaccine Hesitancy: Public Trust, Expertise, and the War on Science. Pittsburgh, PA. University of Pittsburgh Press; 2021.
- Ittefaq M. "It frustrates me beyond words that I can't fix that": health misinformation correction on Facebook during COVID-19. Health Commun. Nov 12, 2023;12:1-11. [ CrossRef ] [ Medline ]
- Mourali M, Drake C. The challenge of debunking health misinformation in dynamic social media conversations: online randomized study of public masking during COVID-19. J Med Internet Res. Mar 02, 2022;24(3):e34831. [ FREE Full text ] [ CrossRef ] [ Medline ]
- Weinzierl MA, Harabagiu SM. Automatic detection of COVID-19 vaccine misinformation with graph link prediction. J Biomed Inform. Dec 2021;124:103955. [ FREE Full text ] [ CrossRef ] [ Medline ]
- Petty RE, Cacioppo JT. Elaboration likelihood model of persuasion. In: Communication and Persuasion. Springer Series in Social Psychology. New York, NY. Springer; 1986.
- Cowles K, Miller R, Suppok R. When seeing isn't believing: navigating visual health misinformation through library instruction. Med Ref Serv Q. 2024;43(1):44-58. [ CrossRef ] [ Medline ]
- Nabożny A, Balcerzak B, Morzy M, Wierzbicki A, Savov P, Warpechowski K. Improving medical experts' efficiency of misinformation detection: an exploratory study. World Wide Web. 2023;26(2):773-798. [ FREE Full text ] [ CrossRef ] [ Medline ]
- Barve Y, Saini JR. Detecting and classifying online health misinformation with 'Content Similarity Measure (CSM)' algorithm: an automated fact-checking-based approach. J Supercomput. 2023;79(8):9127-9156. [ FREE Full text ] [ CrossRef ] [ Medline ]
- Broniatowski D, Gu J, Jamison AM, Simons JR. Facebook's architecture undermines vaccine misinformation removal efforts. arXiv. Preprint posted online Feb 4, 2022. [ FREE Full text ] [ CrossRef ]
- Russell N. Misinformation during COVID: how should nurse practitioners respond? J Nurse Pract. Jun 2021;17(6):763-764. [ FREE Full text ] [ CrossRef ] [ Medline ]
- Wilner T, Holton A. Breast cancer prevention and treatment: misinformation on Pinterest, 2018. Am J Public Health. Oct 2020;110(S3):S300-S304. [ CrossRef ] [ Medline ]
- MacFarlane D, Hurlstone MJ, Ecker UK. Protecting consumers from fraudulent health claims: A taxonomy of psychological drivers, interventions, barriers, and treatments. Soc Sci Med. Aug 2020;259:112790. [ CrossRef ] [ Medline ]
- Thompson JD. Public health pedagogy and digital misinformation: health professional influencers and the politics of expertise. J Sociol. Sep 28, 2022;59(3):646-663. [ CrossRef ]
- Gunter J. Medical misinformation and the internet: a call to arms. Lancet. Jun 08, 2019;393(10188):2294-2295. [ CrossRef ] [ Medline ]
- Sui Y, Zhang B. Determinants of the perceived credibility of rebuttals concerning health misinformation. Int J Environ Res Public Health. Mar 02, 2021;18(3):1345. [ FREE Full text ] [ CrossRef ] [ Medline ]
- Collier R. Containing health myths in the age of viral misinformation. CMAJ. May 14, 2018;190(19):E578. [ FREE Full text ] [ CrossRef ] [ Medline ]
- Peterson JS, Swire-Thompson B, Johnson SB. What is the alternative? Responding strategically to cancer misinformation. Future Oncol. Sep 2020;16(25):1883-1888. [ CrossRef ] [ Medline ]
- Guidry JP, Miller CA, Ksinan AJ, Rohan JM, Winter MA, Carlyle KE, et al. COVID-19-related misinformation among parents of patients with pediatric cancer. Emerg Infect Dis. Mar 2021;27(2):650-652. [ FREE Full text ] [ CrossRef ] [ Medline ]
- Ali S, Khalid A, Zahid E. Is COVID-19 immune to misinformation? A brief overview. Asian Bioeth Rev. Jun 2021;13(2):255-277. [ FREE Full text ] [ CrossRef ] [ Medline ]
- Jafari A, Mohammadpour M, Ghanbarzadegan A, Rossi-Fedele G, Bastani P. Oral health practitioners' knowledge, attitude, and awareness about coronavirus: A systematic review and meta-analysis. J Educ Health Promot. 2021;10:39. [ FREE Full text ] [ CrossRef ] [ Medline ]
- Sanyam SD, Sah SK, Chaudhary P, Burton MJ, Hoffman JJ. Knowledge and awareness-based survey of COVID-19 within the eye care profession in Nepal: Misinformation is hiding the truth. PLoS One. 2021;16(7):e0254761. [ FREE Full text ] [ CrossRef ] [ Medline ]
- Nxumalo CT, Mchunu GG. A qualitative study to explore primary health care practitioners' perceptions and understanding regarding the COVID-19 pandemic in KwaZulu-Natal, South Africa. Afr J Prim Health Care Fam Med. Nov 26, 2021;13(1):e1-e11. [ FREE Full text ] [ CrossRef ] [ Medline ]
- Abbasi K. Where now in the danse macabre of COVID-19 and misinformation? BMJ. Aug 17, 2023;382:1884. [ CrossRef ]
- Pesko MF, Cummings KM, Douglas CE, Foulds J, Miller T, Rigotti NA, et al. United States public health officials need to correct e-cigarette health misinformation. Addiction. May 2023;118(5):785-788. [ CrossRef ] [ Medline ]
Abbreviations
content similarity measure |
elaboration likelihood model |
health information management |
National Health Service |
Edited by G Eysenbach, T Leung; submitted 15.04.22; peer-reviewed by G Nneji, S-F Tsao; comments to author 07.06.22; revised version received 29.09.22; accepted 12.07.24; published 19.08.24.
©Dhouha Kbaier, Annemarie Kane, Mark McJury, Ian Kenny. Originally published in the Journal of Medical Internet Research (https://www.jmir.org), 19.08.2024.
This is an open-access article distributed under the terms of the Creative Commons Attribution License (https://creativecommons.org/licenses/by/4.0/), which permits unrestricted use, distribution, and reproduction in any medium, provided the original work, first published in the Journal of Medical Internet Research (ISSN 1438-8871), is properly cited. The complete bibliographic information, a link to the original publication on https://www.jmir.org/, as well as this copyright and license information must be included.
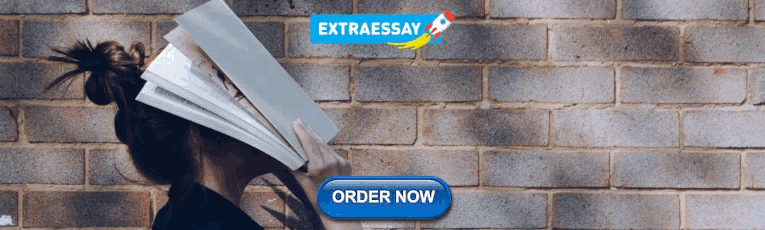
IMAGES
COMMENTS
Kaplan and Haenlein (2010) de ne social media as "a group of Internet-based. applications that build on the ideological and technological foundations of Web. 2.0, and that allow the creation and ...
In business world social media became popular after 2012 and academic literature also indicates social media evolved after 2000 ( Boyd & Ellison, 2007 ). Therefore, the document published in 2000 and after had been considered for the review only. Firstly the keyword "social media" was searched in Scopus database.
Introduction. The term "social media" (SM) was first used in 1994 on a Tokyo online media environment, called Matisse. 1 It was in these early days of the commercial Internet that the first SM platforms were developed and launched. Over time, both the number of SM platforms and the number of active SM users have increased significantly, making it one of the most important applications of ...
The review reveals that social media platforms facilitate global connectivity and foster community building, yet they also pose significant challenges such as superficial connections, social ...
Accordingly, although the systematic review of literature identified a large array of social media platforms investigated for their role in innovation, Facebook (23 articles), Twitter (18 articles) and YouTube (9 articles) were the most popular, followed by LinkedIn (7 articles), online blogs (6 articles) and Chinese Public SM platforms WeChat ...
Social media platforms incorporate a wide range of online media, including word-of-mouth forums such as Social Networking (SNS) (like Myspace and Facebook), ... This systematic literature review has revealed that the second-most used theory in social media for knowledge sharing research, is the Technology Acceptance Model (TAM).
A collection of 229 qualitative studies were identified through a systematic literature review process. A subset of 55 of these articles report studies involving a combination of qualitative and quantitative methods. ... Some authors have focused on categorization of trends in academic literature related to specific social media platforms such ...
In the past, there have been several insightful attempts to review the social media marketing literature. For example, Lamberton and Stephen reviewed and synthesized 160 articles on digital, social ... This was followed by data obtained from social media platforms (23.4%), experimental data (11.5%), and in-depth interviews (8.9%). Notably, 19.9 ...
The spread of social media platforms enhanced academic and professional debate on. social media engagement that attempted to better understand its theoretical founda-. tions and measurements. This ...
The spread of social media platforms enhanced academic and professional debate on social media engagement that attempted to better understand its theoretical foundations and measurements. This paper aims to systematically contribute to this academic debate by analysing, discussing, and synthesising social media engagement literature in the perspective of social media metrics. Adopting a ...
Bucher T, Helmond A (2017) The affordances of social media platforms. In: Burgess J, Poell T, Marwick A (eds) The SAGE Handbook of Social Media. London: SAGE, pp. 233-253. ... Stewart OG (2015) A critical review of the literature of social media's affordances in the classroom. E-Learning and Digital Media 12(5-6): 481-501.
It is the objective of this study to provide a systematic literature review using bibliometric analysis techniques and content analysis to provide a map of research produced between 2009 and 2021. ... Some studies have focused on social media platforms such as Facebook, Twitter, and YouTube (Everson et al., 2013) or Instagram, Pinterest ...
In the age of widespread connectivity, social media has emerged as a dynamic platform shaping public opinion and activism globally. This chapter aims to systematically review literature spanning 2013-2022, aiming to uncover prevalent social media issues influencing public opinion and activism.
The systematic literature review protocol (Fig. 1) has been conducted on the 26 th of March 2020. The study considers an open starting time to trace back to the origin of social media engagement metrics research up to late March 2020. The initial search attempts identified 259 documents.
Social media is a relatively new term that has evolved as a way to describe various platforms for online communication. Overall, the term social media refers to "any technology that facilitates the dissemination and sharing of information over the Internet" (Robbins and Singer, 2014: 387).
Empowerment involves individuals engaging in social media to exert influence on other people or companies. Tsai and Men (Citation 2013) used this six-dimension set in their research on consumer engagement in social media, as did Buzeta et al. (Citation 2020) in their study on consumer engagement with U.S.-based social media platforms.
In this article, the authors present the results from a structured review of the literature, identifying and analyzing the most quoted and dominant definitions of social media (SM) and alternative terms that were used between 1994 and 2019 to identify their major applications. Similarities and differences in the definitions are highlighted to provide guidelines for researchers and managers who ...
In this study, a systematic literature review was carried out to obtain a better and more comprehensive understanding of the research condition on sentiment analysis in social media. The review process is divided into five stages ( Fig. 1 ) which consist of the identification of research questions, search strategy, study selection, data ...
In a review and critique of research on race and racism in the digital realm, Jessie Daniels (2013) identified social media platforms—specifically social network sites (SNSs)—as spaces "where race and racism play out in interesting, sometimes disturbing, ways" (Daniels 2013, 702).Since then, social media research has become a salient academic (sub-)field with its own journal (Social ...
The current literature review aims to present a synthesis of conditions and outcomes relevant for a well-considered, evidence-based use of social media, and teacher professional development. ... Social media platforms and technology education: Facebook on the way to graduate school. International Journal of Technology Management, 66, 358-370 ...
Social media platforms and sleep problems: a systematic literature review, synthesis and framework for future research - Author: Puneet Kaur, Amandeep Dhir, Amal Khalifa Alkhalifa, Anushree Tandon. This study is a systematic literature review (SLR) on prior research examining the impact of the nocturnal use of social media platforms on a user's ...
Method: This study reviews 64 relevant studies on the governance of social media platforms over the last decade. This paper adopts a thematic analysis approach in analyzing the relevant papers and ...
The purpose of this study is to understand the role of social media content on users' engagement behavior. More specifically, we investigate: (i)the direct effects of format and platform on users' passive and active engagement behavior, and (ii) we assess the moderating effect of content context on the link between each content type (rational, emotional, and transactional content) and ...
Purpose: This study aims to identify and prioritize the reasons for social media use among medical practitioners to facilitate the selection of the most appropriate social media platforms for healthcare providers. The findings of this study will help address the challenges posed by the wide variety of available social media platforms and assist healthcare providers in choosing platforms that ...
The social media platforms used in each selected study were identified (Fig. 3). Twitter, Weibo, and Facebook were the main social media platforms used by scholars in public opinion analysis. ... Social Media Analytics: Literature Review and Directions for Future Research. Decision Analysis (2017) deca.2017.0355. Google Scholar [38] C.C. Flores ...
In this world of growing internet users, social media has caught the attention of consumers as well as business enterprises. With the global social media population at a whopping 3.5 billion (), social media marketing (SMM) has surfaced as an immensely powerful tool in the business world.The rise of social media has benefitted both the consumers and business organisations.
Methods: This study used a 5-step scoping review methodology following Arksey and O'Malley's methodology to map relevant literature published in English between January 2012 and March 2024, focusing on health misinformation on social media platforms.
Siyu Zhou is an Assistant Professor in International Communication at the Cheung Kong School of Journalism and Communication, Shantou University (China). He holds a PhD in Communication from Universitat Pompeu Fabra, Spain. Zhou's research interests encompass social media study, intercultural communication, and international education.