Click through the PLOS taxonomy to find articles in your field.
For more information about PLOS Subject Areas, click here .
Loading metrics
Open Access
Peer-reviewed
Research Article
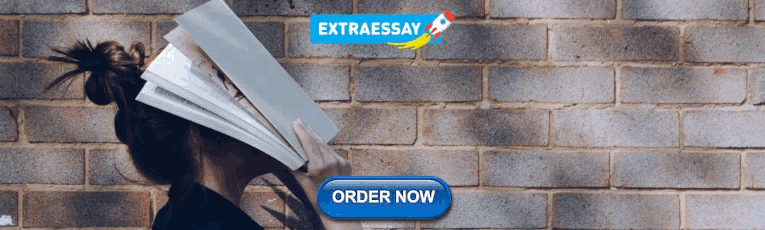
Review of guidance papers on regression modeling in statistical series of medical journals
Roles Data curation, Formal analysis, Investigation, Methodology, Software, Visualization, Writing – original draft, Writing – review & editing
* E-mail: [email protected] (CW); [email protected] (GR)
Affiliations Institute of Biometry and Clinical Epidemiology, Corporate Member of Freie Universität Berlin and Humboldt-Universität zu Berlin, Charité—Universitätsmedizin Berlin, Berlin, Germany, Center for Medical Statistics, Informatics and Intelligent Systems, Section for Clinical Biometrics, Medical University of Vienna, Vienna, Austria

Roles Data curation, Formal analysis, Investigation, Methodology, Writing – review & editing
Affiliations Institute of Biometry and Clinical Epidemiology, Corporate Member of Freie Universität Berlin and Humboldt-Universität zu Berlin, Charité—Universitätsmedizin Berlin, Berlin, Germany, School of Business and Economics, Emmy Noether Group in Statistics and Data Science, Humboldt-Universität zu Berlin, Berlin, Germany
Roles Data curation, Formal analysis, Investigation, Writing – review & editing
Affiliation Institute of Biometry and Clinical Epidemiology, Corporate Member of Freie Universität Berlin and Humboldt-Universität zu Berlin, Charité—Universitätsmedizin Berlin, Berlin, Germany
Roles Validation, Writing – review & editing
Affiliation School of Business and Economics, Emmy Noether Group in Statistics and Data Science, Humboldt-Universität zu Berlin, Berlin, Germany
Roles Conceptualization, Funding acquisition, Project administration, Resources, Supervision, Writing – review & editing
Affiliation Faculty of Medicine and Medical Center, Institute of Medical Biometry and Statistics, University of Freiburg, Freiburg, Germany
Affiliation Department of Biomedical Data Sciences, Leiden University Medical Center, Leiden, The Netherlands
Roles Conceptualization, Funding acquisition, Investigation, Supervision, Validation, Writing – review & editing
Affiliation Center for Medical Statistics, Informatics and Intelligent Systems, Section for Clinical Biometrics, Medical University of Vienna, Vienna, Austria
Roles Conceptualization, Funding acquisition, Investigation, Methodology, Project administration, Resources, Supervision, Validation, Writing – original draft, Writing – review & editing
¶ Membership of the topic group 2 of the STRATOS initiative is listed in the Acknowledgments.
- Christine Wallisch,
- Paul Bach,
- Lorena Hafermann,
- Nadja Klein,
- Willi Sauerbrei,
- Ewout W. Steyerberg,
- Georg Heinze,
- Geraldine Rauch,
- on behalf of topic group 2 of the STRATOS initiative
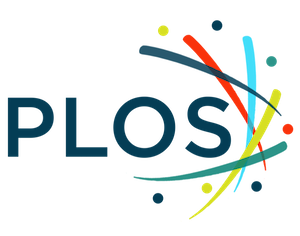
- Published: January 24, 2022
- https://doi.org/10.1371/journal.pone.0262918
- Reader Comments
Although regression models play a central role in the analysis of medical research projects, there still exist many misconceptions on various aspects of modeling leading to faulty analyses. Indeed, the rapidly developing statistical methodology and its recent advances in regression modeling do not seem to be adequately reflected in many medical publications. This problem of knowledge transfer from statistical research to application was identified by some medical journals, which have published series of statistical tutorials and (shorter) papers mainly addressing medical researchers. The aim of this review was to assess the current level of knowledge with regard to regression modeling contained in such statistical papers. We searched for target series by a request to international statistical experts. We identified 23 series including 57 topic-relevant articles. Within each article, two independent raters analyzed the content by investigating 44 predefined aspects on regression modeling. We assessed to what extent the aspects were explained and if examples, software advices, and recommendations for or against specific methods were given. Most series (21/23) included at least one article on multivariable regression. Logistic regression was the most frequently described regression type (19/23), followed by linear regression (18/23), Cox regression and survival models (12/23) and Poisson regression (3/23). Most general aspects on regression modeling, e.g. model assumptions, reporting and interpretation of regression results, were covered. We did not find many misconceptions or misleading recommendations, but we identified relevant gaps, in particular with respect to addressing nonlinear effects of continuous predictors, model specification and variable selection. Specific recommendations on software were rarely given. Statistical guidance should be developed for nonlinear effects, model specification and variable selection to better support medical researchers who perform or interpret regression analyses.
Citation: Wallisch C, Bach P, Hafermann L, Klein N, Sauerbrei W, Steyerberg EW, et al. (2022) Review of guidance papers on regression modeling in statistical series of medical journals. PLoS ONE 17(1): e0262918. https://doi.org/10.1371/journal.pone.0262918
Editor: Tim Mathes, Witten/Herdecke University, GERMANY
Received: June 28, 2021; Accepted: January 8, 2022; Published: January 24, 2022
Copyright: © 2022 Wallisch et al. This is an open access article distributed under the terms of the Creative Commons Attribution License , which permits unrestricted use, distribution, and reproduction in any medium, provided the original author and source are credited.
Data Availability: The data was collected within the review and is available as supporting information S6.
Funding: CW: I-4739-B Austrian Science Fund, https://www.fwf.ac.at/en/ LH: RA 2347/8-1, German Research Foundation, https://www.dfg.de/en/ WS: SA 580/10-1, German Research Foundation, https://www.dfg.de/en/ All funders had no role in study design, data collection and analysis, decision to publish, or preparation of the manuscript.
Competing interests: The authors have declared that no competing interests exist.
Introduction
Knowledge transfer from the rapidly growing body of methodological research in statistics to application in medical research does not always work as it should [ 1 ]. Possible reasons for this problem are the lack of guidance and that not all statistical analyses are conducted by statistical experts but often by medical researchers who may or may not have a solid statistical background. Applied researchers cannot be aware of all statistical pitfalls and the most recent developments in statistical methodology. Keeping up is already challenging for a professional biostatistical researcher, who is often restricted to an area of main interest. Moreover, articles on statistical methodology are often written in a rather technical style making knowledge transfer even more difficult. Therefore, there is a need for statistical guidance documents and tutorials written in more informal language, explaining difficult concepts intuitively and with illustrative educative examples. The international STRengthening Analytical Thinking for Observational Studies (STRATOS) initiative ( http://stratos-initiative.org ) aims to provide accessible and accurate guidance documents for relevant topics in the design and analysis of observational studies [ 1 ]. Guidance is intended for applied statisticians and other medical researchers with varying levels of statistical education, experience and interest. Some medical journals are aware of this situation and regularly publish isolated statistical tutorials and shorter articles or even whole series of articles with the intention to provide some methodological guidance to their readership. Such articles and series can have a high visibility among medical researchers. Although some of the articles are short notes or rather introductory texts, we will use the phrase ‘statistical tutorial’ for all articles in our review.
Regression modeling plays a central role in the analysis of many medical studies, in particular, of observational studies. More specifically, regression model building involves aspects such as selection of a model type that matches the type of outcome variable, selection of explanatory variables to include in a model, choosing an adequate coding of the variables, deciding on how flexibly the association of continuous variables with the outcome should be modeled, planning and performing model diagnostics, model validation and model revision, reporting of a model and describing how well differences in the outcome can be explained by differences in the covariates. Some of the choices made during model building will strongly depend on the aim of modeling. Shmueli (2010) [ 2 ] distinguished between three conceptual modeling approaches: descriptive, predictive and explanatory modeling. In practice these aims are still often not well clarified, leading to confusion about which specific approach is useful in a modeling problem at hand. This confusion, and an ever-growing body of literature in regression modeling may explain why a common state-of-the-art is still difficult to define [ 3 ]. However, not all studies require an analysis with the most advanced techniques and there is the need for guidance for researchers without a strong background in statistical methodology, who might be “medical students or residents, or epidemiologists who completed only a few basic courses in applied statistics” according to the definition of level-1 researchers by the STRATOS initiative [ 1 ].
If suitable guidance for level-1 researchers in peer-reviewed journals was available, many misconceptions about regression model building could be avoided [ 4 – 6 ]. The researchers need to be informed about methods that are easily implemented, and they need to know about strengths and weaknesses of common approaches [ 3 ]. Suitable guidance should also point to possible pitfalls, elaborate on dos and don’ts in regression analyses, and provide software recommendations and understandable code for different methods and aspects. In this review, we focused on low-dimensional regression models where the sample size exceeds the number of candidate predictors. Moreover, we will not specifically address the field of causal inference, which goes beyond classical regression modeling.
So far, it is unclear what aspects of regression modeling have already been well-covered by related tutorials and where gaps still exist. Furthermore, suitable tutorial papers may be published but they are unknown to (nearly all) clinicians and therefore widely ignored in their analyses.
The objective of this review was to provide an evidence-based information basis assessing the extent to which regression modeling has been covered by series of statistical tutorials published in medical journals. Specifically, we sought to define a catalogue of important aspects on regression modeling, to identify series of statistical tutorials in medical journals, and to evaluate which aspects were treated in the identified articles and at which level of sophistication. Thereby, we put an intended focus on the choice of the regression model type, on variable selection and for continuous variables on the functional form. Furthermore, this paper will provide an overview, which helps to inform a broad audience of medical researchers about the availability of suitable papers written in English.
The remainder of this review is organized as follows: In the next section, the review protocol is described. Subsequently, we summarize the results of the review by means of descriptive measures. Finally, we discuss implications of our results suggesting potential topics for future tutorials or entire series.
Material and methods
The protocol of this review describing the detailed design was already published by Bach et al. (2020) [ 7 ]. In here, we summarize its main characteristics.
Eligibility criteria
First, we identified series of statistical tutorials and papers published in medical journals with a target audience mainly or exclusively consisting of medical researchers or practitioners. Second, we searched for topic-relevant articles on regression modeling within these series. Journals with a target audience of pure theoretical, methodological or statistical focus were not considered. We included medical journals if they were available in English language since this implies high international impact and broad visibility. Moreover, the series had to comprise at least five or more articles including at least one topic-relevant article. We focused on statistical series only since we believed that entire series have higher impact and visibility than isolated articles.
Sources of information & search strategy
After conducting a pilot study for a systematic search for series of statistical tutorials, we had to adapt our search strategy since sensitive keywords to identify statistical series could not be found. Therefore, we consulted more than 20 members of the STRATOS initiative via email in spring 2018 for suggestions on statistical series addressing medical researchers. We also asked them to forward this request to colleagues, which resembles snowball sampling [ 8 , 9 ]. This call was repeated at two international STRATOS meetings in summer 2018 and in 2019. The search was closed on June 30 st , 2019. Our approach also included elements of respondent-driven sampling [ 10 ] by offering collaboration and co-authorship in case of relevant contribution to the review. In addition, we included several series that were additionally proposed by a reviewer during the peer-review process of this manuscript, and which were published by the end of June, 2019 to be consistent with the original request.
Data management & selection process
The list of all resulting statistical series suggested is available as S1 File .
Two independent raters selected relevant statistical series from the pool of candidate series by applying the inclusion criteria outlined above.
An article within a series was considered to be topic-relevant if the title included one of the following keywords: regression , linear , logistic , Cox , survival , Poisson , multivariable , multivariate , or if the title suggested that the main topic of the article was statistical regression modeling . Both raters decided on the topic-relevance of an article independently and resolved discrepancies by discussion. To facilitate the selection of relevant statistical series, we designed a report form called inclusion form ( S2 File ).
Data collection process
After the identification of relevant series and topic-relevant articles, a content analysis was performed on all topic-relevant articles using an article content form ( S3 File ). The article content form was filled-in for every identified topic-relevant article by the two raters independently and again discrepancies were resolved by discussion. The results of completed article content forms were copied into a data base for further quantitative analysis.
In total 44 aspects of regression modeling were examined in the article content form ( S3 File ), which were related to four areas: type of regression model , general aspects of regression modeling , functional form of continuous predictors , and selection of variables . The 44 aspects cover topics of different complexity. Some aspects can be considered basic, others are more advanced. This was also commented in the S3 File for orientation. We mainly focused on predictive and descriptive models and did not consider particular aspects attributed to ethological models.
For each aspect, we evaluated whether it was mentioned at all, and if yes, the extent of explanation (short = one sentence only / medium = more than one sentence to one paragraph / long = more than one paragraph) [ 7 ]. We recorded whether examples and software commands were provided, and if recommendations or warnings were given with respect to each aspect. A box for comments provided space to note recommendations, warnings and other issues. In the article content form, it was also possible to add further aspects to each area. A manual for raters was created to support an objective evaluation of the aspects ( S4 File ).
Summary measures & synthesis of results
This review was designed as an explorative study and uses descriptive statistics to summarize results. We calculated absolute and relative frequencies to analyze the 44 statistical aspects. We used stacked bar charts to describe the ordinal variable extent of explanation for each aspect. To structure the analysis, we grouped the aspects into the afore mentioned areas: type of regression model , general aspects of regression modeling , determination of functional form for continuous predictors and selection of variables .
We conducted the above analyses both article-wise and series-wise. In the article-wise analysis, each article was considered individually. For the series-wise analysis, the results from all articles in a series were pooled and each series was considered as the unit of observation. This means, if an aspect was explained in at least one article, this also counted for the entire series.
Risk of bias
The risk of bias by missing a series was addressed extensively in the protocol of this study [ 7 , 11 , 12 ]. Moreover, bias could result from the inclusion criterion of series, which was the requirement of at least five articles in a series. This may have led to a less representative set of series. We set this inclusion criterion to identify highly visible series. Bias could also result from the specific choice of aspects of regression modeling to be screened. We tried to minimize this bias by the possibility for free text entries that could later be combined into additional aspects.
This review has been written according to the PRISMA reporting guideline [ 13 , 14 ], compare S1 Checklist . This review does not include patients or humans. The data that were collected within the review are available in S1 Data .
Selection of series and articles
The initial query revealed 47 series of statistical tutorials ( Fig 1 and S1 File ). Out of these 47 series, two series were not published in a medical journal and five series did not target an audience with low statistical knowledge. Therefore, these series were excluded. Five and ten series were excluded because they were not written in English or they did not comprise at least five articles, respectively. Further, we excluded three series because they did not contain any topic-relevant article. The list of the series and the reason for each excluded series is found in S1 File . Finally, we included 23 series with 57 topic-relevant articles.
- PPT PowerPoint slide
- PNG larger image
- TIFF original image
https://doi.org/10.1371/journal.pone.0262918.g001
Characteristics of the series
Each series contained between one to nine topic-relevant articles (two on average, Table 1 ). The variability of the average number of article pages per series illustrates that the extent of the articles was very different (1 to 10.3 pages). Whereas the series Statistics Notes in the BMJ typically used a single page to discuss a topic, hence pointing only to the most relevant issues, there were longer papers with a length of up to 16 pages [ 15 , 16 ]. The series in the BMJ is also the one spanning over the longest time period (1994–2018). Beside of the series in the BMJ , only the Archives of Disease in Childhood and the Nutrition series started publishing papers already in the last century. Fig 2 shows that most series were published only during a short period, perhaps paralleling terms of office of an Editor.
https://doi.org/10.1371/journal.pone.0262918.g002
We considered 44 aspects, see S3 File .
https://doi.org/10.1371/journal.pone.0262918.t001
The most informative series with respect to our pre-specified list of aspects was published in Revista Española de Cardiologia , which mentioned 35 aspects in two articles on regression modeling ( Table 1 ). Similarly, Circulation and Archives of Disease in Childhood covered 31 and 30 aspects in three article each. The number of articles and the years of publication varied across the series ( Fig 2 ). Some series comprised only five articles whereas Statistics Notes of the BMJ published 68 short articles, which was very successful with some articles that were cited about 2000 times. Almost all series covered multivariable regression in at least one article. The range of regression types varied across series. Most statistical series were published with the intention to improve the knowledge of their readership about how to apply appropriate methodology in data analyses and how to critically appraise published research [ 17 – 19 ].
Characteristics of articles
The top three articles that covered the highest number of aspects (27 to 34 out of 44 aspects) on six to seven pages were published in Revista Española de Cardiologia , Deutsches Ärzteblatt International , and in European Journal of Cardio-Thoracic Surgery [ 20 – 22 ]. The article of Nuñez et al. [ 22 ] published in Revista Española de Cardiologia covered the most popular regression types (linear, logistic and Cox regression) and explained not only general aspect but also gave insights into non-linear modeling and variable selection. Schneider et al. [ 20 ] covered all regression types that we considered in our review in their publication in Deutsches Ärzteblatt International . The top-ranked article in European Journal of Cardio-Thoracic Surgery [ 21 ] particularly focused on the development and validation of prediction models.
Explanation of aspects in the series
Almost all statistical series included at least one article that mentioned or explained multivariable regression ( Table 1 ). Logistic regression was the most frequently described regression type in 19 out of 23 series (83%), followed by linear regression (78%). Cox regression/survival model (including proportional hazards regression) was mentioned in twelve series (52%) and was less extensively described than linear and logistic regression. Poisson regression was covered by three series (13%). Each of the considered general aspects of regression modeling were mentioned in at least four series (17%) ( Fig 3 ) except for random effect models , which were treated in only one series (4%). Interpretation of regression coefficients , model assumptions , and different purposes of regression mode were covered in 19 series (83%). The aspect different purposes of regression models comprised at least one statement in an article concerning purposes of regression models, which could be identified by keywords like prediction, description, explanation, etiology, or confounding. More than one sentence was used for the explanation of different purposes in 15 series (65%). In 18 series (78%), reporting of regression results and regression diagnostics were described, which was done extensively in most series. Aspects like treatment of binary covariates , missing values , measurement error , and adjusted coefficient of determination were rather infrequently mentioned and found in four to seven series each (25–30%).
Extent of explanation of general aspects of regression modeling in statistical series: One sentence only (light grey), more than one sentence to one paragraph (grey) and more than one paragraph (black).
https://doi.org/10.1371/journal.pone.0262918.g003
At least one aspect of functional forms of continuous predictors , was mentioned in 17 series (74%), but details were hardly ever given ( Fig 4 ). The possibility of non-linear relation and non-linear transformations were raised in 16 (70%) and eleven series (48%), respectively. Dichotomization of continuous covariates was found in eight series (35%) and it was extensively discussed in two (9%). More advanced techniques like the use of splines or fractional polynomials were mentioned in some series but detailed information for splines was not provided. Generalized additive models were never mentioned.
Extent of explanation of aspects of functional forms of continuous predictors in statistical series: One sentence only (light grey), more than one sentence to one paragraph (grey) and more than one paragraph (black).
https://doi.org/10.1371/journal.pone.0262918.g004
Selection of variables was mentioned in 15 series (65%) and described extensively in ten series (43%) ( Fig 5 ). However, specific variable selection methods were rarely described in detail. Backward elimination , selection based on background knowledge , forward selection , and stepwise selection were the most frequently described selection methods in seven to eleven series (30–48%). Univariate screening , which is still popular in medical research, was only described in three series (13%) in up to one paragraph. Other aspects of variable selection were hardly ever mentioned. Selection based on AIC/BIC , relating to best subset selection or stepwise selection based on these information criteria, and the choice of the significance level were found in 2 series only (9%). Relative frequencies of aspects mentioned in articles are detailed in Figs 1 – 3 in S5 File .
Extent of explanation of aspects of selection of variables in statistical series: One sentence only (light grey), more than one sentence to one paragraph (grey) and more than one paragraph (black).
https://doi.org/10.1371/journal.pone.0262918.g005
We found general recommendations for software in nine articles of nine different series. Authors mentioned R, Nanostat, GLIM package, SAS and SPSS [ 75 – 78 ]. SAS as well as R were recommended in three articles. In only one article the authors referred to a specific package in R. Detailed code examples were provided in two articles only [ 16 , 58 ]. In the article of Curran-Everett [ 58 ], the R script file was provided as appendix and in the article of Obuchowski [ 16 ], code chunks were included throughout the text directly showing how to derive the reported results. In all, software recommendations were rare and mostly not detailed.
Recommendations and warnings in the series
Recommendations and warnings were given on many aspects of our list. All statements are listed in S5 File : Table 1 and some frequent statements across articles are summarized below.
Statements on general aspects
We found numerous recommendations and warnings on general aspects as described in the following. Concerning data preparation, some authors recommended to impute missing values in multivariable models, e.g. by multiple imputation [ 20 – 22 , 31 ]. Steyerberg et al. [ 31 ] and Grant et al. [ 21 ] discouraged from using a complete case analysis to handle missing values. As an aspect of model development, number of observations/events per variable was a disputed topic in several articles [ 79 – 81 ]. In seven articles, we found explicit recommendations for the number of observations (in linear models) or the events per variable (in logistic and Cox/survival models), varying between at least ten to 20 observations/events per variable [ 16 , 20 , 22 , 25 , 31 , 33 , 55 ]. Several recommendations and warnings were given on model assumptions and model diagnostics . Many series authors recommended to check assumptions graphically [ 24 , 27 , 44 , 58 , 72 ] and they warned that models may be inappropriate if the assumptions are not met [ 20 , 24 , 31 , 33 , 52 , 55 , 56 , 62 ]. In the context of Cox proportional hazards model, authors especially mentioned the proportional hazards assumption [ 24 , 44 , 49 , 56 , 62 ]. Concerning reporting of results, some authors warned to not confuse odds ratios with relative risks or hazard ratios [ 25 , 44 , 59 ]. Several warnings could also be found on reporting performance of a model. Most authors did not recommend to report the coefficient of determination R 2 [ 20 , 27 , 51 , 61 ] and indicated that the pitfall of R 2 is that its value increases with increasing number of covariates in the model [ 15 ]. Schneider et al. [ 20 ] and Richardson et al. [ 61 ] recommended to use the adjusted coefficient of determination instead. We also found many recommendations and statements about model validation for prediction models. Authors of the evaluated articles recommended cross-validation or bootstrap validation instead of split sample validation if internal validation is performed [ 21 , 22 , 31 , 70 , 72 ]. It was also suggested that internal validation is not sufficient for the model to be used in clinical practice and an external validation should be executed as well [ 21 ]. In several articles, we found that authors warned about applying the Hosmer-Lemeshow test because of potential pitfalls [ 31 , 60 , 61 ]. For reporting regression results , in two articles the guideline for Transparent Reporting of multivariable prediction models for Individual Prognosis or Diagnosis (TRIPOD) was mentioned [ 21 , 71 , 82 ].
Statements on functional form of continuous predictors
Dichotomization of continuous predictors is an aspect of functional forms of continuous predictors that was frequently discussed. Many authors argued against categorization of continuous variables because it may lead to loss of power, to increased risk of false positive results, to underestimation of variation, and to concealment of non-linearities [ 21 , 26 , 31 , 69 ]. However, other authors advised to categorize continuous variables if the relation to the outcome is non-linear [ 24 , 25 , 59 ].
Statements on variable selection
We also found recommendations in favor of or against specific variable selection methods. Four articles explicitly recommended to take advantage of background knowledge to select variables [ 15 , 20 , 48 , 59 ]. Univariate screening was advised against by one article [ 19 ]. Comparing stepwise selection methods, Grant et al. [ 21 ] preferred backward elimination over forward selection. Authors warned about consequences of stepwise methods such as unstable selection and overfitting [ 21 , 31 ]. It was also pointed out that selected models must be interpreted with greatest caution and implications should be checked on new data [ 28 , 53 ].
Methodological gaps in the series
This descriptive analysis of contents gives rise to some observations on important gaps and possibly misleading recommendations. First, we found that one general type of regression models, Poisson regression, was not treated in most series. This omission is probably due to the fact that Poisson regression is less frequently applied in medical research because most outcomes are binary or time-to-event and, therefore, logistic and Cox regression are more frequent. Second, several series introduced the possibility of non-linear relations of continuous covariates with the outcome. However, only few statements on how to deal with non-linearities by specifying flexible functional forms in multiple regression were available. Third, we did not find very detailed information on advantages and disadvantages of data-driven variable selection methods in any of the series. Finally, tutorials on statistical software and on specific code examples were hardly found in the reviewed series.
Misleading recommendations in the series
Quality assessment of recommendations would have been controversial and we did not intend doing it. Nevertheless, here we mention two issues that we consider as severely misleading. Although univariate screening as a method for variable selection was never recommended in any of the series, one article showed an example with the application of this procedure to pre-filter the explanatory variables based on their associations with the outcome variable [ 47 ]. It is known since long that univariate screening should be avoided because it has the potential to wrongly reject important variables [ 83 ]. In another article it was suggested that a model can be considered robust if results from both backward elimination and forward selection agree [ 20 ]. Such agreement does not support robustness of stepwise methods: relying on agreement is a poor strategy [ 84 , 85 ].
Series and articles recommended to read
Depending on the aim of the planned study, as well as the focus and knowledge level of the reader, different series and articles might be recommended. The series in Circulation comprised three papers about multiple linear and logistic regression [ 24 – 26 ], which provide basics and describe many essential aspects of univariable and multivariable regression modeling. For more advanced researchers, we recommend the article of Nuñ ez et al. in Revista Española de Cardiologia [ 22 ], which gives a quick overview of aspects and existing methods including functional forms and variable selection. The Nature Methods series published short articles focusing on few, specific aspects of regression modeling [ 34 – 42 ]. This series might be of interest if one likes to spent more time on learning about regression modeling. If someone is especially interested in prediction models, we recommend a concise publication in the European Heart Journal [ 31 ], which provides details on model development and validation for predictive purposes. For the same topic we can also recommend the paper by Grant et al. [ 21 ]. We consider all series and articles recommended in this paragraph as suitable reading for medical researchers but this does not imply that we agree to all explanations, statements and aspects discussed.
Summary and consequences for future work
This review summarizes the knowledge about regression modeling that is transferred through statistical tutorials published in medical journals. A total of 23 series with 57 topic-relevant articles were identified and evaluated for coverage of 44 aspects of regression modeling. We found that almost all aspects of regression modeling were at least mentioned in any of the series. Several aspects of regression modeling, in particular most general aspects, were covered. However, detailed descriptions and explanations of non-linear relations and variable selection in multivariable models were lacking. Only few papers provided suitable methods and software guidance for analysts with a relatively weak statistical background and limited practical experience as recommended by the STRATOS initiative [ 1 ]. However, we confess that currently there is no agreement on state of the art methodology [ 3 ].
Nevertheless, readers of statistical tutorials should not only be informed about the possibility of non-linear relations of continuous predictors with the outcome but they should also be given a brief overview about which methods are generally available and may be suitable. This could be achieved by tutorials that introduce readers to methods like fractional polynomials or splines, explaining similarities and differences between these approaches, e.g., by comparative, commented analyses of realistic data sets. Such documents could also show how alternative analyses (considering/ignoring potential non-linearities) may result in conflicting results and explain the reasons for such discrepancies.
Detailed tutorials on variable selection could aim at describing the mechanism of different variable selection methods, which can easily be applied with standard statistical software, and should state in what situations variable selection methods are needed and could be used. For example, if sufficient background knowledge is available, prefiltering or even the selection of variables should be based on this information rather than using data-driven methods on the entire data set. Such tutorials should provide comparisons and interpretation of the results of various variable selection methods and suggest adequate methods for different data settings.
Generally, the articles also lacked details on software to perform statistical analysis and usually did not provide code chunks, descriptions of specific functions, an appendix with commented code or references to software packages. Future work should also focus on filling this gap by recommendations of software as well as providing well commented and documented code for different statistical methods in a format that is accessible by non-experts. We recommend that software, packages and functions therein to apply certain methods should be reported in every statistical tutorial article. The respective code to derive analysis results could be provided in an appendix or directly in the manuscript text, if not too lengthy. Any provided code in the appendix should be well-structured and lavishly commented referring to the particular method and describing all defined parameter settings. This will encourage medical researchers to increase the reproducibility of their research by also publishing their statistical code, e.g., in electronic appendices to their publications. For example, worked examples with openly accessible data sets and commented code allowing fully reproducible results have a high potential to guide researchers in their own statistical tasks. On the contrary, we discourage from using point-and-click software programs, which sometimes output far more analysis results than requested. Users may pick inadequate methods or report wrong results inadvertently, which could debilitate their research work.
Generally, our review may stimulate the development of targeted gap-filling guidance and tutorial papers in the field of regression modeling, which should support medical researchers in several ways: 1) by explaining how to interpret published results correctly, 2) by guiding them how to critically appraise the methodology used in a published article, 3) by enabling them to plan, perform basic statistical analyses and report results in a proper way and 4) by helping them to identify situations in which the advice of a statistical expert is required. In S3 File : CRF article screening we commented which aspects should usually be addressed by an expert and which aspects are considered basic.
Strengths and limitations
According to our knowledge this is the first review on series of statistical tutorials in the medical field with the focus on regression modeling. Our review followed a pre-specified and published protocol to which many experienced researchers in the field of applied regression modeling contributed. One aspect of this contribution was the collection of series of statistical tutorials that could not be identified by common keyword searches.
We standardized the selection process by designing an inclusion checklist for series of statistical tutorials and by providing a manual for the content form with which we extracted the actual information of the article and series. Another strength is that the data collection process was performed objectively since each article was analyzed by two out of three independent raters. Discrepancies were discussed among all three of them to find a consent. This procedure avoided that single opinions were transferred to the output of this review. This review is informative for many clinical colleagues who are interested in statistical issues in regression modeling and search for suitable literature.
This review also has limitations. An automated, systematic search was not possible because series could not be identified by common keywords neither on the series’ title level nor on the article’s title level. Thus, not all available series may have been found. To enrich our initial query, we also searched on certain journals’ webpages and requested our expert panel from the STRATOS initiative to complement our list with other series they were aware of. We also included series that were suggested by one reviewer during the peer-review procedure of this manuscript. This selection strategy may impose a bias towards higher-quality journals since series of less prestigious journals might not be known to the experts. However, the higher-quality journals can be considered as the primary source of information for researchers seeking advice on statistical methodology.
We considered only series with at least five articles. This boundary is of course to a certain extend arbitrary. It was motivated by the fact that we intended to do analyses on the series level, which is only reasonable if a series covers an adequate number of articles. We also assumed that larger series are more visible and well-known to researchers.
We also might have missed or excluded some important aspects of regression modeling in our catalogue. The catalogue of aspects was developed and discussed by several experienced researchers of the STRATOS initiative working in the field of regression modeling. After submission of the protocol paper some more aspects were added on request of its reviewers [ 7 ]. However, further important aspects such as meta-regression, diagnostic models, causal inference, reproducibility or open data and open software code were not addressed. We encourage researchers to repeat similar reviews on these related fields.
A third limitation is that we only searched for series whereas there might be other educational papers on regression modeling that were published as single articles. However, we believe that the average visibility of an entire series and thereby its educational impact is much higher than for isolated articles. This does not negate that there could be excellent isolated articles, which can have a high impact for training medical researchers. While working on the final version of this paper we became aware of the series Big-data Clinical Trial Column in the Annals of Translational Medicine . Until 1 January 2019 they had published 36 papers and the series would have been eligible for our review. Obviously, we might have overseen further series, but it is unlikely that it has a larger effect on the results of our review.
Moreover, there are many introductory textbooks, educational workshops and online video tutorials, some of them with excellent quality, which were not considered here. A detailed review of such sources clearly was out of our scope.
Despite many series of statistical tutorials being available to guide medical researchers on various aspects of regression modeling, several methodological gaps still persist, specifically on addressing nonlinear effects, model specification and variable selection. Furthermore, papers are published in a large number of different journals and are therefore likely unknown to many medical researchers. This review fills the latter gap, but many more steps are needed to improve the quality and interpretation of medical research. More detailed statistical guidance and tutorials with a low technical level on regression modeling and other topics are needed to better support medical researchers who perform or interpret regression analyses.
Supporting information
S1 checklist. prisma reporting guideline..
https://doi.org/10.1371/journal.pone.0262918.s001
S1 File. List of candidate series for potential inclusion in the review.
https://doi.org/10.1371/journal.pone.0262918.s002
S2 File. Case report form–series inclusion.
https://doi.org/10.1371/journal.pone.0262918.s003
S3 File. Case report form–article screening.
https://doi.org/10.1371/journal.pone.0262918.s004
S4 File. Manual for the article screening sheet.
https://doi.org/10.1371/journal.pone.0262918.s005
S5 File. Supplementary figures and tables.
https://doi.org/10.1371/journal.pone.0262918.s006
S1 Data. Collected data.
https://doi.org/10.1371/journal.pone.0262918.s007
Acknowledgments
When this article was written, topic group 2 of STRATOS consisted of the following members: Georg Heinze (Co-chair, [email protected] ), Medical University of Vienna, Austria; Willi Sauerbrei (co-chair, [email protected] ), University of Freiburg, Germany; Aris Perperoglou (co-chair, [email protected] ), AstraZeneca, London, Great Britain; Michal Abrahamowicz, Royal Victoria Hospital, Montreal, Canada; Heiko Becher, Medical University Center Hamburg, Eppendorf, Hamburg, Germany; Harald Binder, University of Freiburg, Germany; Daniela Dunkler, Medical University of Vienna, Austria; Rolf Groenwold, Leiden University, Leiden, Netherlands; Frank Harrell, Vanderbilt University School of Medicine, Nashville TN, USA; Nadja Klein, Humboldt Universität, Berlin, Germany; Geraldine Rauch, Charité–Universitätsmedizin Berlin, Germany; Patrick Royston, University College London, Great Britain; Matthias Schmid, University of Bonn, Germany.
We thank Edith Motschall (Freiburg) for her important support in the pilot study where we tried to define keywords for identifying statistical series within medical journals. We thank several members of the STRATOS initiative for proposing a high number of candidate series and we thank Frank Konietschke for English language editing in our protocol.
- View Article
- PubMed/NCBI
- Google Scholar
- 75. R Core Team. R: A Language and Environment for Statistical Computing. Vienna, Austria: R Foundation for Statistical Computing; 2021.
- 77. SAS Institute Inc. The SAS system for Windows. Release 9.4. Cary, NC: SAS Institute Inc.; 2021.
- 78. IBM Corporation. IBM SPSS Statistics for Windows, Version 27.0. Armonk, NY: IBM Corporation; 2020.
- Privacy Policy

Home » Regression Analysis – Methods, Types and Examples
Regression Analysis – Methods, Types and Examples
Table of Contents

Regression Analysis
Regression analysis is a set of statistical processes for estimating the relationships among variables . It includes many techniques for modeling and analyzing several variables when the focus is on the relationship between a dependent variable and one or more independent variables (or ‘predictors’).
Regression Analysis Methodology
Here is a general methodology for performing regression analysis:
- Define the research question: Clearly state the research question or hypothesis you want to investigate. Identify the dependent variable (also called the response variable or outcome variable) and the independent variables (also called predictor variables or explanatory variables) that you believe are related to the dependent variable.
- Collect data: Gather the data for the dependent variable and independent variables. Ensure that the data is relevant, accurate, and representative of the population or phenomenon you are studying.
- Explore the data: Perform exploratory data analysis to understand the characteristics of the data, identify any missing values or outliers, and assess the relationships between variables through scatter plots, histograms, or summary statistics.
- Choose the regression model: Select an appropriate regression model based on the nature of the variables and the research question. Common regression models include linear regression, multiple regression, logistic regression, polynomial regression, and time series regression, among others.
- Assess assumptions: Check the assumptions of the regression model. Some common assumptions include linearity (the relationship between variables is linear), independence of errors, homoscedasticity (constant variance of errors), and normality of errors. Violation of these assumptions may require additional steps or alternative models.
- Estimate the model: Use a suitable method to estimate the parameters of the regression model. The most common method is ordinary least squares (OLS), which minimizes the sum of squared differences between the observed and predicted values of the dependent variable.
- I nterpret the results: Analyze the estimated coefficients, p-values, confidence intervals, and goodness-of-fit measures (e.g., R-squared) to interpret the results. Determine the significance and direction of the relationships between the independent variables and the dependent variable.
- Evaluate model performance: Assess the overall performance of the regression model using appropriate measures, such as R-squared, adjusted R-squared, and root mean squared error (RMSE). These measures indicate how well the model fits the data and how much of the variation in the dependent variable is explained by the independent variables.
- Test assumptions and diagnose problems: Check the residuals (the differences between observed and predicted values) for any patterns or deviations from assumptions. Conduct diagnostic tests, such as examining residual plots, testing for multicollinearity among independent variables, and assessing heteroscedasticity or autocorrelation, if applicable.
- Make predictions and draw conclusions: Once you have a satisfactory model, use it to make predictions on new or unseen data. Draw conclusions based on the results of the analysis, considering the limitations and potential implications of the findings.
Types of Regression Analysis
Types of Regression Analysis are as follows:
Linear Regression
Linear regression is the most basic and widely used form of regression analysis. It models the linear relationship between a dependent variable and one or more independent variables. The goal is to find the best-fitting line that minimizes the sum of squared differences between observed and predicted values.
Multiple Regression
Multiple regression extends linear regression by incorporating two or more independent variables to predict the dependent variable. It allows for examining the simultaneous effects of multiple predictors on the outcome variable.
Polynomial Regression
Polynomial regression models non-linear relationships between variables by adding polynomial terms (e.g., squared or cubic terms) to the regression equation. It can capture curved or nonlinear patterns in the data.
Logistic Regression
Logistic regression is used when the dependent variable is binary or categorical. It models the probability of the occurrence of a certain event or outcome based on the independent variables. Logistic regression estimates the coefficients using the logistic function, which transforms the linear combination of predictors into a probability.
Ridge Regression and Lasso Regression
Ridge regression and Lasso regression are techniques used for addressing multicollinearity (high correlation between independent variables) and variable selection. Both methods introduce a penalty term to the regression equation to shrink or eliminate less important variables. Ridge regression uses L2 regularization, while Lasso regression uses L1 regularization.
Time Series Regression
Time series regression analyzes the relationship between a dependent variable and independent variables when the data is collected over time. It accounts for autocorrelation and trends in the data and is used in forecasting and studying temporal relationships.
Nonlinear Regression
Nonlinear regression models are used when the relationship between the dependent variable and independent variables is not linear. These models can take various functional forms and require estimation techniques different from those used in linear regression.
Poisson Regression
Poisson regression is employed when the dependent variable represents count data. It models the relationship between the independent variables and the expected count, assuming a Poisson distribution for the dependent variable.
Generalized Linear Models (GLM)
GLMs are a flexible class of regression models that extend the linear regression framework to handle different types of dependent variables, including binary, count, and continuous variables. GLMs incorporate various probability distributions and link functions.
Regression Analysis Formulas
Regression analysis involves estimating the parameters of a regression model to describe the relationship between the dependent variable (Y) and one or more independent variables (X). Here are the basic formulas for linear regression, multiple regression, and logistic regression:
Linear Regression:
Simple Linear Regression Model: Y = β0 + β1X + ε
Multiple Linear Regression Model: Y = β0 + β1X1 + β2X2 + … + βnXn + ε
In both formulas:
- Y represents the dependent variable (response variable).
- X represents the independent variable(s) (predictor variable(s)).
- β0, β1, β2, …, βn are the regression coefficients or parameters that need to be estimated.
- ε represents the error term or residual (the difference between the observed and predicted values).
Multiple Regression:
Multiple regression extends the concept of simple linear regression by including multiple independent variables.
Multiple Regression Model: Y = β0 + β1X1 + β2X2 + … + βnXn + ε
The formulas are similar to those in linear regression, with the addition of more independent variables.
Logistic Regression:
Logistic regression is used when the dependent variable is binary or categorical. The logistic regression model applies a logistic or sigmoid function to the linear combination of the independent variables.
Logistic Regression Model: p = 1 / (1 + e^-(β0 + β1X1 + β2X2 + … + βnXn))
In the formula:
- p represents the probability of the event occurring (e.g., the probability of success or belonging to a certain category).
- X1, X2, …, Xn represent the independent variables.
- e is the base of the natural logarithm.
The logistic function ensures that the predicted probabilities lie between 0 and 1, allowing for binary classification.
Regression Analysis Examples
Regression Analysis Examples are as follows:
- Stock Market Prediction: Regression analysis can be used to predict stock prices based on various factors such as historical prices, trading volume, news sentiment, and economic indicators. Traders and investors can use this analysis to make informed decisions about buying or selling stocks.
- Demand Forecasting: In retail and e-commerce, real-time It can help forecast demand for products. By analyzing historical sales data along with real-time data such as website traffic, promotional activities, and market trends, businesses can adjust their inventory levels and production schedules to meet customer demand more effectively.
- Energy Load Forecasting: Utility companies often use real-time regression analysis to forecast electricity demand. By analyzing historical energy consumption data, weather conditions, and other relevant factors, they can predict future energy loads. This information helps them optimize power generation and distribution, ensuring a stable and efficient energy supply.
- Online Advertising Performance: It can be used to assess the performance of online advertising campaigns. By analyzing real-time data on ad impressions, click-through rates, conversion rates, and other metrics, advertisers can adjust their targeting, messaging, and ad placement strategies to maximize their return on investment.
- Predictive Maintenance: Regression analysis can be applied to predict equipment failures or maintenance needs. By continuously monitoring sensor data from machines or vehicles, regression models can identify patterns or anomalies that indicate potential failures. This enables proactive maintenance, reducing downtime and optimizing maintenance schedules.
- Financial Risk Assessment: Real-time regression analysis can help financial institutions assess the risk associated with lending or investment decisions. By analyzing real-time data on factors such as borrower financials, market conditions, and macroeconomic indicators, regression models can estimate the likelihood of default or assess the risk-return tradeoff for investment portfolios.
Importance of Regression Analysis
Importance of Regression Analysis is as follows:
- Relationship Identification: Regression analysis helps in identifying and quantifying the relationship between a dependent variable and one or more independent variables. It allows us to determine how changes in independent variables impact the dependent variable. This information is crucial for decision-making, planning, and forecasting.
- Prediction and Forecasting: Regression analysis enables us to make predictions and forecasts based on the relationships identified. By estimating the values of the dependent variable using known values of independent variables, regression models can provide valuable insights into future outcomes. This is particularly useful in business, economics, finance, and other fields where forecasting is vital for planning and strategy development.
- Causality Assessment: While correlation does not imply causation, regression analysis provides a framework for assessing causality by considering the direction and strength of the relationship between variables. It allows researchers to control for other factors and assess the impact of a specific independent variable on the dependent variable. This helps in determining the causal effect and identifying significant factors that influence outcomes.
- Model Building and Variable Selection: Regression analysis aids in model building by determining the most appropriate functional form of the relationship between variables. It helps researchers select relevant independent variables and eliminate irrelevant ones, reducing complexity and improving model accuracy. This process is crucial for creating robust and interpretable models.
- Hypothesis Testing: Regression analysis provides a statistical framework for hypothesis testing. Researchers can test the significance of individual coefficients, assess the overall model fit, and determine if the relationship between variables is statistically significant. This allows for rigorous analysis and validation of research hypotheses.
- Policy Evaluation and Decision-Making: Regression analysis plays a vital role in policy evaluation and decision-making processes. By analyzing historical data, researchers can evaluate the effectiveness of policy interventions and identify the key factors contributing to certain outcomes. This information helps policymakers make informed decisions, allocate resources effectively, and optimize policy implementation.
- Risk Assessment and Control: Regression analysis can be used for risk assessment and control purposes. By analyzing historical data, organizations can identify risk factors and develop models that predict the likelihood of certain outcomes, such as defaults, accidents, or failures. This enables proactive risk management, allowing organizations to take preventive measures and mitigate potential risks.
When to Use Regression Analysis
- Prediction : Regression analysis is often employed to predict the value of the dependent variable based on the values of independent variables. For example, you might use regression to predict sales based on advertising expenditure, or to predict a student’s academic performance based on variables like study time, attendance, and previous grades.
- Relationship analysis: Regression can help determine the strength and direction of the relationship between variables. It can be used to examine whether there is a linear association between variables, identify which independent variables have a significant impact on the dependent variable, and quantify the magnitude of those effects.
- Causal inference: Regression analysis can be used to explore cause-and-effect relationships by controlling for other variables. For example, in a medical study, you might use regression to determine the impact of a specific treatment while accounting for other factors like age, gender, and lifestyle.
- Forecasting : Regression models can be utilized to forecast future trends or outcomes. By fitting a regression model to historical data, you can make predictions about future values of the dependent variable based on changes in the independent variables.
- Model evaluation: Regression analysis can be used to evaluate the performance of a model or test the significance of variables. You can assess how well the model fits the data, determine if additional variables improve the model’s predictive power, or test the statistical significance of coefficients.
- Data exploration : Regression analysis can help uncover patterns and insights in the data. By examining the relationships between variables, you can gain a deeper understanding of the data set and identify potential patterns, outliers, or influential observations.
Applications of Regression Analysis
Here are some common applications of regression analysis:
- Economic Forecasting: Regression analysis is frequently employed in economics to forecast variables such as GDP growth, inflation rates, or stock market performance. By analyzing historical data and identifying the underlying relationships, economists can make predictions about future economic conditions.
- Financial Analysis: Regression analysis plays a crucial role in financial analysis, such as predicting stock prices or evaluating the impact of financial factors on company performance. It helps analysts understand how variables like interest rates, company earnings, or market indices influence financial outcomes.
- Marketing Research: Regression analysis helps marketers understand consumer behavior and make data-driven decisions. It can be used to predict sales based on advertising expenditures, pricing strategies, or demographic variables. Regression models provide insights into which marketing efforts are most effective and help optimize marketing campaigns.
- Health Sciences: Regression analysis is extensively used in medical research and public health studies. It helps examine the relationship between risk factors and health outcomes, such as the impact of smoking on lung cancer or the relationship between diet and heart disease. Regression analysis also helps in predicting health outcomes based on various factors like age, genetic markers, or lifestyle choices.
- Social Sciences: Regression analysis is widely used in social sciences like sociology, psychology, and education research. Researchers can investigate the impact of variables like income, education level, or social factors on various outcomes such as crime rates, academic performance, or job satisfaction.
- Operations Research: Regression analysis is applied in operations research to optimize processes and improve efficiency. For example, it can be used to predict demand based on historical sales data, determine the factors influencing production output, or optimize supply chain logistics.
- Environmental Studies: Regression analysis helps in understanding and predicting environmental phenomena. It can be used to analyze the impact of factors like temperature, pollution levels, or land use patterns on phenomena such as species diversity, water quality, or climate change.
- Sports Analytics: Regression analysis is increasingly used in sports analytics to gain insights into player performance, team strategies, and game outcomes. It helps analyze the relationship between various factors like player statistics, coaching strategies, or environmental conditions and their impact on game outcomes.
Advantages and Disadvantages of Regression Analysis
Advantages of Regression Analysis | Disadvantages of Regression Analysis |
---|---|
Provides a quantitative measure of the relationship between variables | Assumes a linear relationship between variables, which may not always hold true |
Helps in predicting and forecasting outcomes based on historical data | Requires a large sample size to produce reliable results |
Identifies and measures the significance of independent variables on the dependent variable | Assumes no multicollinearity, meaning that independent variables should not be highly correlated with each other |
Provides estimates of the coefficients that represent the strength and direction of the relationship between variables | Assumes the absence of outliers or influential data points |
Allows for hypothesis testing to determine the statistical significance of the relationship | Can be sensitive to the inclusion or exclusion of certain variables, leading to different results |
Can handle both continuous and categorical variables | Assumes the independence of observations, which may not hold true in some cases |
Offers a visual representation of the relationship through the use of scatter plots and regression lines | May not capture complex non-linear relationships between variables without appropriate transformations |
Provides insights into the marginal effects of independent variables on the dependent variable | Requires the assumption of homoscedasticity, meaning that the variance of errors is constant across all levels of the independent variables |
About the author
Muhammad Hassan
Researcher, Academic Writer, Web developer
You may also like

Narrative Analysis – Types, Methods and Examples

Phenomenology – Methods, Examples and Guide

ANOVA (Analysis of variance) – Formulas, Types...

Histogram – Types, Examples and Making Guide

Framework Analysis – Method, Types and Examples

Symmetric Histogram – Examples and Making Guide
IEEE Account
- Change Username/Password
- Update Address
Purchase Details
- Payment Options
- Order History
- View Purchased Documents
Profile Information
- Communications Preferences
- Profession and Education
- Technical Interests
- US & Canada: +1 800 678 4333
- Worldwide: +1 732 981 0060
- Contact & Support
- About IEEE Xplore
- Accessibility
- Terms of Use
- Nondiscrimination Policy
- Privacy & Opting Out of Cookies
A not-for-profit organization, IEEE is the world's largest technical professional organization dedicated to advancing technology for the benefit of humanity. © Copyright 2024 IEEE - All rights reserved. Use of this web site signifies your agreement to the terms and conditions.
The state of AI in early 2024: Gen AI adoption spikes and starts to generate value
If 2023 was the year the world discovered generative AI (gen AI) , 2024 is the year organizations truly began using—and deriving business value from—this new technology. In the latest McKinsey Global Survey on AI, 65 percent of respondents report that their organizations are regularly using gen AI, nearly double the percentage from our previous survey just ten months ago. Respondents’ expectations for gen AI’s impact remain as high as they were last year , with three-quarters predicting that gen AI will lead to significant or disruptive change in their industries in the years ahead.
About the authors
This article is a collaborative effort by Alex Singla , Alexander Sukharevsky , Lareina Yee , and Michael Chui , with Bryce Hall , representing views from QuantumBlack, AI by McKinsey, and McKinsey Digital.
Organizations are already seeing material benefits from gen AI use, reporting both cost decreases and revenue jumps in the business units deploying the technology. The survey also provides insights into the kinds of risks presented by gen AI—most notably, inaccuracy—as well as the emerging practices of top performers to mitigate those challenges and capture value.
AI adoption surges
Interest in generative AI has also brightened the spotlight on a broader set of AI capabilities. For the past six years, AI adoption by respondents’ organizations has hovered at about 50 percent. This year, the survey finds that adoption has jumped to 72 percent (Exhibit 1). And the interest is truly global in scope. Our 2023 survey found that AI adoption did not reach 66 percent in any region; however, this year more than two-thirds of respondents in nearly every region say their organizations are using AI. 1 Organizations based in Central and South America are the exception, with 58 percent of respondents working for organizations based in Central and South America reporting AI adoption. Looking by industry, the biggest increase in adoption can be found in professional services. 2 Includes respondents working for organizations focused on human resources, legal services, management consulting, market research, R&D, tax preparation, and training.
Also, responses suggest that companies are now using AI in more parts of the business. Half of respondents say their organizations have adopted AI in two or more business functions, up from less than a third of respondents in 2023 (Exhibit 2).

Future frontiers: Navigating the next wave of tech innovations
Join Lareina Yee and Roger Roberts on Tuesday, July 30, at 12:30 p.m. EDT/6:30 p.m. CET as they discuss the future of these technological trends, the factors that will fuel their growth, and strategies for investing in them through 2024 and beyond.
Gen AI adoption is most common in the functions where it can create the most value
Most respondents now report that their organizations—and they as individuals—are using gen AI. Sixty-five percent of respondents say their organizations are regularly using gen AI in at least one business function, up from one-third last year. The average organization using gen AI is doing so in two functions, most often in marketing and sales and in product and service development—two functions in which previous research determined that gen AI adoption could generate the most value 3 “ The economic potential of generative AI: The next productivity frontier ,” McKinsey, June 14, 2023. —as well as in IT (Exhibit 3). The biggest increase from 2023 is found in marketing and sales, where reported adoption has more than doubled. Yet across functions, only two use cases, both within marketing and sales, are reported by 15 percent or more of respondents.
Gen AI also is weaving its way into respondents’ personal lives. Compared with 2023, respondents are much more likely to be using gen AI at work and even more likely to be using gen AI both at work and in their personal lives (Exhibit 4). The survey finds upticks in gen AI use across all regions, with the largest increases in Asia–Pacific and Greater China. Respondents at the highest seniority levels, meanwhile, show larger jumps in the use of gen Al tools for work and outside of work compared with their midlevel-management peers. Looking at specific industries, respondents working in energy and materials and in professional services report the largest increase in gen AI use.
Investments in gen AI and analytical AI are beginning to create value
The latest survey also shows how different industries are budgeting for gen AI. Responses suggest that, in many industries, organizations are about equally as likely to be investing more than 5 percent of their digital budgets in gen AI as they are in nongenerative, analytical-AI solutions (Exhibit 5). Yet in most industries, larger shares of respondents report that their organizations spend more than 20 percent on analytical AI than on gen AI. Looking ahead, most respondents—67 percent—expect their organizations to invest more in AI over the next three years.
Where are those investments paying off? For the first time, our latest survey explored the value created by gen AI use by business function. The function in which the largest share of respondents report seeing cost decreases is human resources. Respondents most commonly report meaningful revenue increases (of more than 5 percent) in supply chain and inventory management (Exhibit 6). For analytical AI, respondents most often report seeing cost benefits in service operations—in line with what we found last year —as well as meaningful revenue increases from AI use in marketing and sales.
Inaccuracy: The most recognized and experienced risk of gen AI use
As businesses begin to see the benefits of gen AI, they’re also recognizing the diverse risks associated with the technology. These can range from data management risks such as data privacy, bias, or intellectual property (IP) infringement to model management risks, which tend to focus on inaccurate output or lack of explainability. A third big risk category is security and incorrect use.
Respondents to the latest survey are more likely than they were last year to say their organizations consider inaccuracy and IP infringement to be relevant to their use of gen AI, and about half continue to view cybersecurity as a risk (Exhibit 7).
Conversely, respondents are less likely than they were last year to say their organizations consider workforce and labor displacement to be relevant risks and are not increasing efforts to mitigate them.
In fact, inaccuracy— which can affect use cases across the gen AI value chain , ranging from customer journeys and summarization to coding and creative content—is the only risk that respondents are significantly more likely than last year to say their organizations are actively working to mitigate.
Some organizations have already experienced negative consequences from the use of gen AI, with 44 percent of respondents saying their organizations have experienced at least one consequence (Exhibit 8). Respondents most often report inaccuracy as a risk that has affected their organizations, followed by cybersecurity and explainability.
Our previous research has found that there are several elements of governance that can help in scaling gen AI use responsibly, yet few respondents report having these risk-related practices in place. 4 “ Implementing generative AI with speed and safety ,” McKinsey Quarterly , March 13, 2024. For example, just 18 percent say their organizations have an enterprise-wide council or board with the authority to make decisions involving responsible AI governance, and only one-third say gen AI risk awareness and risk mitigation controls are required skill sets for technical talent.
Bringing gen AI capabilities to bear
The latest survey also sought to understand how, and how quickly, organizations are deploying these new gen AI tools. We have found three archetypes for implementing gen AI solutions : takers use off-the-shelf, publicly available solutions; shapers customize those tools with proprietary data and systems; and makers develop their own foundation models from scratch. 5 “ Technology’s generational moment with generative AI: A CIO and CTO guide ,” McKinsey, July 11, 2023. Across most industries, the survey results suggest that organizations are finding off-the-shelf offerings applicable to their business needs—though many are pursuing opportunities to customize models or even develop their own (Exhibit 9). About half of reported gen AI uses within respondents’ business functions are utilizing off-the-shelf, publicly available models or tools, with little or no customization. Respondents in energy and materials, technology, and media and telecommunications are more likely to report significant customization or tuning of publicly available models or developing their own proprietary models to address specific business needs.
Respondents most often report that their organizations required one to four months from the start of a project to put gen AI into production, though the time it takes varies by business function (Exhibit 10). It also depends upon the approach for acquiring those capabilities. Not surprisingly, reported uses of highly customized or proprietary models are 1.5 times more likely than off-the-shelf, publicly available models to take five months or more to implement.
Gen AI high performers are excelling despite facing challenges
Gen AI is a new technology, and organizations are still early in the journey of pursuing its opportunities and scaling it across functions. So it’s little surprise that only a small subset of respondents (46 out of 876) report that a meaningful share of their organizations’ EBIT can be attributed to their deployment of gen AI. Still, these gen AI leaders are worth examining closely. These, after all, are the early movers, who already attribute more than 10 percent of their organizations’ EBIT to their use of gen AI. Forty-two percent of these high performers say more than 20 percent of their EBIT is attributable to their use of nongenerative, analytical AI, and they span industries and regions—though most are at organizations with less than $1 billion in annual revenue. The AI-related practices at these organizations can offer guidance to those looking to create value from gen AI adoption at their own organizations.
To start, gen AI high performers are using gen AI in more business functions—an average of three functions, while others average two. They, like other organizations, are most likely to use gen AI in marketing and sales and product or service development, but they’re much more likely than others to use gen AI solutions in risk, legal, and compliance; in strategy and corporate finance; and in supply chain and inventory management. They’re more than three times as likely as others to be using gen AI in activities ranging from processing of accounting documents and risk assessment to R&D testing and pricing and promotions. While, overall, about half of reported gen AI applications within business functions are utilizing publicly available models or tools, gen AI high performers are less likely to use those off-the-shelf options than to either implement significantly customized versions of those tools or to develop their own proprietary foundation models.
What else are these high performers doing differently? For one thing, they are paying more attention to gen-AI-related risks. Perhaps because they are further along on their journeys, they are more likely than others to say their organizations have experienced every negative consequence from gen AI we asked about, from cybersecurity and personal privacy to explainability and IP infringement. Given that, they are more likely than others to report that their organizations consider those risks, as well as regulatory compliance, environmental impacts, and political stability, to be relevant to their gen AI use, and they say they take steps to mitigate more risks than others do.
Gen AI high performers are also much more likely to say their organizations follow a set of risk-related best practices (Exhibit 11). For example, they are nearly twice as likely as others to involve the legal function and embed risk reviews early on in the development of gen AI solutions—that is, to “ shift left .” They’re also much more likely than others to employ a wide range of other best practices, from strategy-related practices to those related to scaling.
In addition to experiencing the risks of gen AI adoption, high performers have encountered other challenges that can serve as warnings to others (Exhibit 12). Seventy percent say they have experienced difficulties with data, including defining processes for data governance, developing the ability to quickly integrate data into AI models, and an insufficient amount of training data, highlighting the essential role that data play in capturing value. High performers are also more likely than others to report experiencing challenges with their operating models, such as implementing agile ways of working and effective sprint performance management.
About the research
The online survey was in the field from February 22 to March 5, 2024, and garnered responses from 1,363 participants representing the full range of regions, industries, company sizes, functional specialties, and tenures. Of those respondents, 981 said their organizations had adopted AI in at least one business function, and 878 said their organizations were regularly using gen AI in at least one function. To adjust for differences in response rates, the data are weighted by the contribution of each respondent’s nation to global GDP.
Alex Singla and Alexander Sukharevsky are global coleaders of QuantumBlack, AI by McKinsey, and senior partners in McKinsey’s Chicago and London offices, respectively; Lareina Yee is a senior partner in the Bay Area office, where Michael Chui , a McKinsey Global Institute partner, is a partner; and Bryce Hall is an associate partner in the Washington, DC, office.
They wish to thank Kaitlin Noe, Larry Kanter, Mallika Jhamb, and Shinjini Srivastava for their contributions to this work.
This article was edited by Heather Hanselman, a senior editor in McKinsey’s Atlanta office.
Explore a career with us
Related articles.

Moving past gen AI’s honeymoon phase: Seven hard truths for CIOs to get from pilot to scale
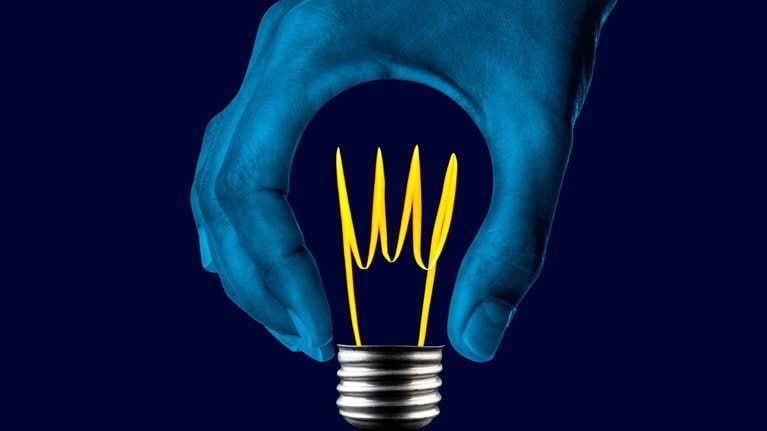
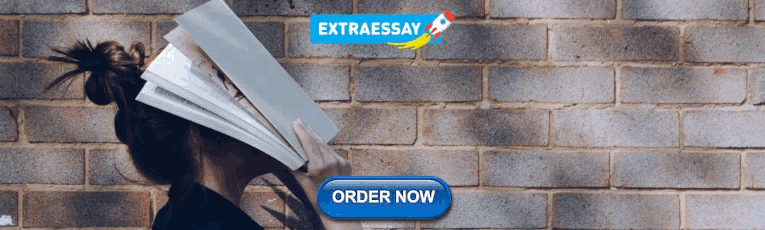
A generative AI reset: Rewiring to turn potential into value in 2024

Implementing generative AI with speed and safety
Executive Compensation Structure, Economic Cycle, and R&D Investment
- Published: 24 July 2024
Cite this article
- Xuelian Zuo 1 ,
- Shiwen Luo ORCID: orcid.org/0000-0002-4016-9914 2 &
- David Yoon Kin Tong 3
The purposes of the paper are as follows: First, explore the impact of executive compensation structure on enterprise research and development (R&D) investment. Second, examining the impact of economic cycle on R&D investment and its effect on the relationship between executive compensation structure and R&D investment in China. Empirical verification is carried out utilizing multiple regression analysis and based on the panel data collected from A-share listed companies in China from 2007 to 2014. The study delivers findings as follows: The higher the proportion of equity return in total executive compensation, the more executives are motivated to consider the enterprise’s long-term value and thus are motivated to increase enterprise R&D investment. Such a relationship is more significantly observed in state-owned enterprises. R&D investment is countercyclical, and the economic cycle weakens the incentive effect of the executive compensation structure on enterprise R&D investment. This study provides a reference to guide China’s listed companies in preparing executive compensation contracts and improving the corporate governance system and a reference to guide the government in supporting R&D investment projects under the current circumstances.
This is a preview of subscription content, log in via an institution to check access.
Access this article
Subscribe and save.
- Get 10 units per month
- Download Article/Chapter or Ebook
- 1 Unit = 1 Article or 1 Chapter
- Cancel anytime
Price includes VAT (Russian Federation)
Instant access to the full article PDF.
Rent this article via DeepDyve
Institutional subscriptions

Data Availability
The datasets used and/or analyzed during the current study are available from the corresponding author on reasonable request.
Abbass, K., Begum, H., Alam, A. F., Awang, A. H., Abdelsalam, M. K., Egdair, I. M. M., & Wahid, R. (2022). Fresh insight through a Keynesian theory approach to investigate the economic impact of the COVID-19 pandemic in Pakistan. Sustainability, 14 (3), 1054. https://doi.org/10.3390/su14031054
Article Google Scholar
Abbass, K., Song, H., Khan, F., Begum, H., & Asif, M. (2022). Fresh insight through the VAR approach to investigate the effects of fiscal policy on environmental pollution in Pakistan. Environmental Science and Pollution Research, 4 , 1–14. https://doi.org/10.1007/s11356-021-17438-x
Aghion, P., Askenazy, P., Berman, N., Cette, G., & Eymard, L. (2012). Credit constraints and the cyclicality of RandD investment: Evidence from France. Journal of the European Economic Association, 10 (5), 1001–1024. https://doi.org/10.1111/j.1542-4774.2012.01093.x
Aghion, P., & Saint-Paul, G. (1998). Virtues of bad times: Interaction between productivity growth and economic fluctuations. Macroeconomic Dynamics, 2 (3), 322–344. https://doi.org/10.1017/S1365100598008025
Aramonte, S. (2015). Innovation, investor sentiment, and firm-level experimentation. Finance and Economics Discussion Series, 67 , 1–46. https://doi.org/10.17016/FEDS.2015.067
Balkin, D. B., Markman, G. D., & Gomez-Mejia, L. R. (2000). Is CEO pay in high technology firms related to innovation? Academy of Management Journal, 43 (6), 1118–1129. https://doi.org/10.5465/1556340
Barker, V. L., & Mueller, G. C. (2002). CEO characteristics and firm R&D spending. Management Science, 48 (6), 782–801. https://doi.org/10.1287/mnsc.48.6.782.187
Bone, S., & Saxon, T. (2000). Developing effective technology strategies. Research Technology Management, 43 (4), 50–58. https://doi.org/10.1080/08956308.2000.11671368
Brown, J. R., Martinsson, G., & Petersen, B. C. (2012). Do financing constraints matter for R&D? European Economic Review, 56 (8), 1512–1529. https://doi.org/10.1016/j.euroecorev.2012.07.007
Bulan, L., & Sanyal, P. (2011). Incentivizing managers to build innovative firms. Annals of Finance, 7 (2), 267–283. https://doi.org/10.1007/s10436-010-0174-2
Choe, C., Tian, G. Y., & Yin, X. (2014). CEO power and the structure of CEO pay. International Review of Financial Analysis, 35 , 237–248. https://doi.org/10.1016/j.irfa.2014.10.004
Daoguang, Y., Hanwen, C., & Qiliang, L. (2017). Media pressure and enterprise innovation. Economic Research, 52 (8), 125–139.
Google Scholar
Dong, J., & Gou, Y. (2010). Corporate governance structure, managerial discretion, and the R&D investment in China. International Review of Economics & Finance, 19 (2), 180–188. https://doi.org/10.1016/j.iref.2009.10.001
Edmans, A., Gabaix, X., Sadzik, T., & Sannikov, Y. (2012). Dynamic CEO compensation. The Journal of Finance, 67 (5), 1603–1647. https://doi.org/10.1111/j.1540-6261.2012.01768.x
Feng, G. F., & Wen, J. (2008). An empirical study on relationship between corporate governance and technical innovation of chinese listed companies. China Industrial Economics, 7 , 91–101.
Fisman, R., & Svensson, J. (2007). Are corruption and taxation really harmful to growth? Firm level evidence. Journal of Development Economics, 83 (1), 63–75. https://doi.org/10.1016/j.jdeveco.2005.09.009
Fu, X. (2012). How does openness affect the importance of incentives for innovation? Research Policy, 41 (3), 512–523. https://doi.org/10.1016/j.respol.2011.12.011
Hao, Q. M., & Zhang, X. Y. (2023). Executive incentive, risk-taking and R&D investment. Commercial Research, 65 (2), 109–117.
Hashmi, H. B. A., Voinea, C. L., Caniëls, M. C., Ooms, W., & Abbass, K. (2023). Do top management team diversity and chief sustainability officer make firms greener? Moderating role of top management team behavioral integration. Sustainable Development, 31 (4), 2536–2547. https://doi.org/10.1002/sd.2529
Hellmann, T., & Thiele, V. (2011). Incentives and innovation: A multitasking approach. American Economic Journal: Microeconomics, 3 (1), 78–128. https://doi.org/10.1257/mic.3.1.78
Honore, F., Munari, F., & Potterie, B. V. P. L. (2015). Corporate governance practices and companies’ R&D intensity: Evidence from European countries. Research Policy, 44 (2), 533–543. https://doi.org/10.1016/j.respol.2014.10.016
Hu, Y. R., Chen, D. D., & Liu, Z. (2018). Financing constraints, cyclicality and smooth mechanism of firms’ R&D investment: Based on the perspective of ownership. Industrial Economics Research, 2 , 78–90. CNKI:SUN:CYJJ.0.2018-02-007
Huang, J., & Kisgen, D. J. (2013). Gender and corporate finance: Are male executives overconfident relative to female executives? Journal of Financial Economics, 108 (3), 822–839. https://doi.org/10.1016/j.jfineco.2012.12.005
Jensen, M. C., & Meckling, W. H. (1976). Theory of the firm: Managerial behavior, agency costs and ownership structure. Journal of Financial Economics, 3 (4), 305–360. https://doi.org/10.1016/0304-405X(76)90026-X
Jensen, M. C., & Murphy, K. J. (1990). CEO incentives: It’s not how much you pay, but how. Harvard Business Review, 68 (3), 138–149. https://doi.org/10.2139/ssrn.146148
Jensen, M. C., & Murphy, K. J. (1990). Performance pay and top manager incentives. Journal of Political Economy, 98 (2), 225–264. https://doi.org/10.1086/261677
Jiang, N., & Huang, W. (2010). An empirical study of the impact of government subsidies on enterprises’ RandD investment in China’s high-tech industry. Science of Science and Management of S.andT, 31 (7), 28–33.
Johnson, T. C. (2007). Optimal learning and new technology bubbles. Journal of Monetary Economics, 54 (8), 2486–2511. https://doi.org/10.1016/j.jmoneco.2007.03.004
Khan, F., Abbass, K., Qun, W., & Asif, M. (2024). Investigating capital flight in South Asian countries: The dual influence of terrorism and corruption. PLoS ONE, 19 (3), e0295695. https://doi.org/10.1371/journal.pone.0295695
Kleer, R. (2010). Government R&D subsidies as a signal for private investors. Research Policy, 39 (10), 1361–1374. https://doi.org/10.1016/j.respol.2010.08.001
Laux, V. (2012). Stock option vesting conditions, CEO turnover, and myopic investment. Journal of Financial Economics, 106 (3), 513–526. https://doi.org/10.1016/j.jfineco.2012.06.003
Lee, D. (2016). Role of R&D in the productivity growth of Korean industries: Technology gap and business cycle. Journal of Asian Economics, 45 , 31–45. https://doi.org/10.1016/j.asieco.2016.06.002
Li, F. Y., Yang, M., & Zh. (2015). Does economic policy uncertainty inhibit corporate investment?—An empirical study based on China’s economic policy uncertainty index. Financial Research, 4 , 115–129. CNKI:SUN:JRYJ.0.2015-04-008
Li, Y., Ye, K., & Wu, P. (2022). R&D investment, marketization degree and specialized operation of enterprises. Scientific Research Management, 43 (4), 158–163.
Lin, C., Lin, P., Song, F. M., et al. (2011). Managerial incentives, CEO characteristics and corporate innovation in China’s private sector. Journal of Comparative Economics, 39 (2), 176–190. https://doi.org/10.1016/j.jce.2009.12.001
Liu, Y., & Jiraporn, P. (2010). The effect of CEO power on bond ratings and yields. Journal of Empirical Finance, 17 (4), 744–762. https://doi.org/10.1016/j.jempfin.2010.03.003
Lu, T., & Dang, Y. (2014). Corporate governance and technological innovation: A comparison by industry. Economic Research, 49 (6), 115–128. CNKI:SUN:JJYJ.0.2014-06-009
Mackey, A. (2008). The effect of CEOs on firm performance. Strategic Management Journal, 29 (12), 1357–1367. https://doi.org/10.1002/smj.708
Manso, G. (2011). Motivating innovation. The Journal of Finance, 66 (5), 1823–1860. https://doi.org/10.1111/j.1540-6261.2011.01688.x
Matsuyama, K. (2001). Growing through cycles in an infinitely lived agent economy. Journal of economic theory, 100 (2), 220–234. https://doi.org/10.1006/jeth.2000.2770
Mehran, H. (1995). Executive compensation structure, ownership, and firm performance. Journal of Financial Economics, 38 (2), 163–184. https://doi.org/10.1016/0304-405X(94)00809-F
Nickell, S., Nicolitsas, D., & Patterson, M. (2001). Does doing badly encourage management innovation? Oxford Bulletin of Economics & Statistics, 63 (1), 5–28. https://doi.org/10.1111/1468-0084.00207
Niu, Y., et al. (2016). Research on the impact of executive compensation incentives on corporate independent innovation—Based on empirical data of high-tech listed companies. Economic and Management Review, 4 , 67–78. https://doi.org/10.13962/j.cnki.37-1486/f.2016.04.009
Parisi, M. L., & Sembenelli, A. (2003). Is private R&D spending sensitive to its price? Empirical evidence on panel data for Italy. Empirica, 30 (4), 357–377.
Pi, Y. H., & Bao, G. M. (2005). An empirical study of the relationship between diversification strategy and R&D intensity of Chinese firms. Science Research Management, 2 , 76–82.
Qian, C., Cao, Q., & Takeuchi, R. (2013). Top management team functional diversity and organizational innovation in China: The moderating effects of environment. Strategic Management Journal, 34 (1), 110–120. https://doi.org/10.1002/smj.1993
Qin, T. C., & Zhang, T. G. (2015). Research on the periodicity of R & D investment of listed companies. Journal of Mathematical Statistics and Management, 3 , 529–539.
Qin, Y., & Jin, Y. (2015). Economic fluctuation, external equity of compensation and corporate performance. Journal of Zhongnan University of Economics and Law, 3 , 94–102.
Song, Z., Storesletten, K., & Zilibotti, F. (2011). Growing like China. American Economic Review, 101 (1), 196–233. https://doi.org/10.1257/aer.101.1.196
Tribo, J. A., Berrone, P., & Surroca, J. (2007). Do the type and number of blockholders influence RandD investments? New evidence from Spain. Corporate Governance: An International Review, 15 (5), 828–842. https://doi.org/10.1111/j.1467-8683.2007.00622.x
Vroom, V. H. (1964). Work and motivation . Wiley.
Wang, Y. N. (2011). The influence of top management incentives on R&D investment—Evidence from listed manufacturing companies in China. Studies in Science of Science, 7 , 1071–1078.
Wen, W., Cheng, H. F., & Tang, L. J. (2015). The asymmetric impact of business cycle to R&D intensity of China. Studies in Science of Science, 9 , 1357–1364.
Wright, M., Hoskisson, R. E., & Busenitz, L. W. (2001). Firm rebirth: Buyouts as facilitators of strategic growth and entrepreneurship. Academy of Management Perspectives, 15 (1), 111–125. https://doi.org/10.5465/ame.2001.4251486
Wu, J., & Tu, R. (2007). CEO stock option pay and R&D spending: A behavioral agency explanation. Journal of Business Research, 60 (5), 482–492. https://doi.org/10.1016/j.jbusres.2006.12.006
Xie, J., Abbass, K., & Li, D. (2024). Advancing eco-excellence: Integrating stakeholders’ pressures, environmental awareness, and ethics for green innovation and performance. Journal of Environmental Management, 352 , 120027. https://doi.org/10.1016/j.jenvman.2024.120027
Xu, F., & Cheng, K. Q. (2021). The effect of differences in executive incentive styles on corporate innovation persistence-based on the mediating role of R&D investment. Journal of Changchun University of Science and Technology (social Science Edition), 34 (1), 87–93.
Xu, Z.Y., Wang, J., Zhang, N., Han, B. (2023). “Asset structure mismatch, incentive mechanism and corporate dual innovation”. Nankai Business Review, pp. 1–32. link.cnki.net/urlid/12.1288.F.20231213.1708.002.
Yang, X., Zhang, L., & Chen, X. (2014). ketization and cash dividend policy: Governance effect or relieving constraints? Economic and Management Research, 5 , 76–84.
Yu, M. G., Fan, R., & Zhong, H. J. (2016). Chinese industrial policy and corporate technological innovation. China Industrial Economics, 12 , 5–22.
Yu, Y. H., Zhao, Q. F., & Ju, X. S. (2018). Inventor executives and corporate innovation. China Industrial Economy, 3 , 137–155. https://doi.org/10.19581/j.cnki.ciejournal.2018.03.008
Zhai, S. P., & Bi, X. F. (2016). Executive shareholding, government funding and R&D investments in high-tech firms-also discussing the governance effect of equity structure. Science Research, 34 (9), 1371–1380. https://doi.org/10.3969/j.issn.1003-2053.2016.09.011
Zheng, M. B. (2019). Influence of economic fluctuation on corporate R&D input: Evidences from China’s listed enterprises. Journal of Jiangxi University of Finance and Economics, 5 , 104–117.
Zheng, X. T., & Liu, J. P. (2008). The effective combination of tax and subsidy policy to promote R&D activities. Industrial Economics Research, 1 , 26–36.
Zhong, Y. J., Zhang, C. Y., & Chen, D. Q. (2016). Privatization and innovation efficiency: Promotion or suppression? Journal of Finance and Economics, 42 (7), 4–15. https://doi.org/10.16538/j.cnki.jfe.2016.07.001
Download references
Acknowledgements
The helps of data processing of Kejie Chen are appreciated.
Declarations
Author information
Authors and affiliations.
Zhejiang Yuexiu University, Shaoxing, China
Xuelian Zuo
Zhejiang Financial College, Hangzhou, China
International University of Malaya-Wales, Kuala Lumpur, Malaysia
David Yoon Kin Tong
You can also search for this author in PubMed Google Scholar
Corresponding author
Correspondence to Shiwen Luo .
Additional information
Publisher's note.
Springer Nature remains neutral with regard to jurisdictional claims in published maps and institutional affiliations.
Rights and permissions
Springer Nature or its licensor (e.g. a society or other partner) holds exclusive rights to this article under a publishing agreement with the author(s) or other rightsholder(s); author self-archiving of the accepted manuscript version of this article is solely governed by the terms of such publishing agreement and applicable law.
Reprints and permissions
About this article
Zuo, X., Luo, S. & Tong, D.Y.K. Executive Compensation Structure, Economic Cycle, and R&D Investment. J Knowl Econ (2024). https://doi.org/10.1007/s13132-024-02189-0
Download citation
Received : 16 February 2024
Accepted : 06 June 2024
Published : 24 July 2024
DOI : https://doi.org/10.1007/s13132-024-02189-0
Share this article
Anyone you share the following link with will be able to read this content:
Sorry, a shareable link is not currently available for this article.
Provided by the Springer Nature SharedIt content-sharing initiative
- Compensation structure
- Economic cycle
- R&D investment
- Find a journal
- Publish with us
- Track your research
Thank you for visiting nature.com. You are using a browser version with limited support for CSS. To obtain the best experience, we recommend you use a more up to date browser (or turn off compatibility mode in Internet Explorer). In the meantime, to ensure continued support, we are displaying the site without styles and JavaScript.
- View all journals
- Explore content
- About the journal
- Publish with us
- Sign up for alerts
- Open access
- Published: 12 July 2024
The nature of the last universal common ancestor and its impact on the early Earth system
- Edmund R. R. Moody ORCID: orcid.org/0000-0002-8785-5006 1 ,
- Sandra Álvarez-Carretero ORCID: orcid.org/0000-0002-9508-6286 1 ,
- Tara A. Mahendrarajah ORCID: orcid.org/0000-0001-7032-6581 2 ,
- James W. Clark 3 ,
- Holly C. Betts 1 ,
- Nina Dombrowski ORCID: orcid.org/0000-0003-1917-2577 2 ,
- Lénárd L. Szánthó ORCID: orcid.org/0000-0003-3363-2488 4 , 5 , 6 ,
- Richard A. Boyle 7 ,
- Stuart Daines 7 ,
- Xi Chen ORCID: orcid.org/0000-0001-7098-607X 8 ,
- Nick Lane ORCID: orcid.org/0000-0002-5433-3973 9 ,
- Ziheng Yang ORCID: orcid.org/0000-0003-3351-7981 9 ,
- Graham A. Shields ORCID: orcid.org/0000-0002-7828-3966 8 ,
- Gergely J. Szöllősi 5 , 6 , 10 ,
- Anja Spang ORCID: orcid.org/0000-0002-6518-8556 2 , 11 ,
- Davide Pisani ORCID: orcid.org/0000-0003-0949-6682 1 , 12 ,
- Tom A. Williams ORCID: orcid.org/0000-0003-1072-0223 12 ,
- Timothy M. Lenton ORCID: orcid.org/0000-0002-6725-7498 7 &
- Philip C. J. Donoghue ORCID: orcid.org/0000-0003-3116-7463 1
Nature Ecology & Evolution ( 2024 ) Cite this article
58k Accesses
925 Altmetric
Metrics details
- Microbial genetics
- Molecular evolution
- Phylogenetics
The nature of the last universal common ancestor (LUCA), its age and its impact on the Earth system have been the subject of vigorous debate across diverse disciplines, often based on disparate data and methods. Age estimates for LUCA are usually based on the fossil record, varying with every reinterpretation. The nature of LUCA’s metabolism has proven equally contentious, with some attributing all core metabolisms to LUCA, whereas others reconstruct a simpler life form dependent on geochemistry. Here we infer that LUCA lived ~4.2 Ga (4.09–4.33 Ga) through divergence time analysis of pre-LUCA gene duplicates, calibrated using microbial fossils and isotope records under a new cross-bracing implementation. Phylogenetic reconciliation suggests that LUCA had a genome of at least 2.5 Mb (2.49–2.99 Mb), encoding around 2,600 proteins, comparable to modern prokaryotes. Our results suggest LUCA was a prokaryote-grade anaerobic acetogen that possessed an early immune system. Although LUCA is sometimes perceived as living in isolation, we infer LUCA to have been part of an established ecological system. The metabolism of LUCA would have provided a niche for other microbial community members and hydrogen recycling by atmospheric photochemistry could have supported a modestly productive early ecosystem.
Similar content being viewed by others
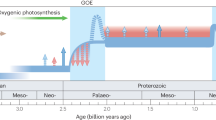
Co‐evolution of early Earth environments and microbial life
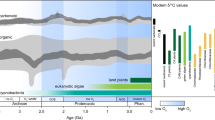
The curious consistency of carbon biosignatures over billions of years of Earth-life coevolution
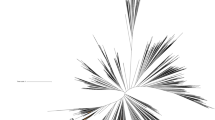
Phanerozoic radiation of ammonia oxidizing bacteria
The common ancestry of all extant cellular life is evidenced by the universal genetic code, machinery for protein synthesis, shared chirality of the almost-universal set of 20 amino acids and use of ATP as a common energy currency 1 . The last universal common ancestor (LUCA) is the node on the tree of life from which the fundamental prokaryotic domains (Archaea and Bacteria) diverge. As such, our understanding of LUCA impacts our understanding of the early evolution of life on Earth. Was LUCA a simple or complex organism? What kind of environment did it inhabit and when? Previous estimates of LUCA are in conflict either due to conceptual disagreement about what LUCA is 2 or as a result of different methodological approaches and data 3 , 4 , 5 , 6 , 7 , 8 , 9 . Published analyses differ in their inferences of LUCA’s genome, from conservative estimates of 80 orthologous proteins 10 up to 1,529 different potential gene families 4 . Interpretations range from little beyond an information-processing and metabolic core 6 through to a prokaryote-grade organism with much of the gene repertoire of modern Archaea and Bacteria 8 , recently reviewed in ref. 7 . Here we use molecular clock methodology, horizontal gene-transfer-aware phylogenetic reconciliation and existing biogeochemical models to address questions about LUCA’s age, gene content, metabolism and impact on the early Earth system.
Estimating the age of LUCA
Life’s evolutionary timescale is typically calibrated to the oldest fossil occurrences. However, the veracity of fossil discoveries from the early Archaean period has been contested 11 , 12 . Relaxed Bayesian node-calibrated molecular clock approaches provide a means of integrating the sparse fossil and geochemical record of early life with the information provided by molecular data; however, constraining LUCA’s age is challenging due to limited prokaryote fossil calibrations and the uncertainty in their placement on the phylogeny. Molecular clock estimates of LUCA 13 , 14 , 15 have relied on conserved universal single-copy marker genes within phylogenies for which LUCA represented the root. Dating the root of a tree is difficult because errors propagate from the tips to the root of the dated phylogeny and information is not available to estimate the rate of evolution for the branch incident on the root node. Therefore, we analysed genes that duplicated before LUCA with two (or more) copies in LUCA’s genome 16 . The root in these gene trees represents this duplication preceding LUCA, whereas LUCA is represented by two descendant nodes. Use of these universal paralogues also has the advantage that the same calibrations can be applied at least twice. After duplication, the same species divergences are represented on both sides of the gene tree 17 , 18 and thus can be assumed to have the same age. This considerably reduces the uncertainty when genetic distance (branch length) is resolved into absolute time and rate. When a shared node is assigned a fossil calibration, such cross-bracing also serves to double the number of calibrations on the phylogeny, improving divergence time estimates. We calibrated our molecular clock analyses using 13 calibrations (see ‘Fossil calibrations’ in Supplementary Information ). The calibration on the root of the tree of life is of particular importance. Some previous studies have placed a younger maximum constraint on the age of LUCA based on the assumption that life could not have survived Late Heavy Bombardment (LHB) (~3.7–3.9 billion years ago (Ga)) 19 . However, the LHB hypothesis is extrapolated and scaled from the Moon’s impact record, the interpretation of which has been questioned in terms of the intensity, duration and even the veracity of an LHB episode 20 , 21 , 22 , 23 . Thus, the LHB hypothesis should not be considered a credible maximum constraint on the age of LUCA. We used soft-uniform bounds, with the maximum-age bound based on the time of the Moon-forming impact (4,510 million years ago (Ma) ± 10 Myr), which would have effectively sterilized Earth’s precursors, Tellus and Theia 13 . Our minimum bound on the age of LUCA is based on low δ 98 Mo isotope values indicative of Mn oxidation compatible with oxygenic photosynthesis and, therefore, total-group Oxyphotobacteria in the Mozaan Group, Pongola Supergroup, South Africa 24 , 25 , dated minimally to 2,954 Ma ± 9 Myr (ref. 26 ).
Our estimates for the age of LUCA are inferred with a concatenated and a partitioned dataset, both consisting of five pre-LUCA paralogues: catalytic and non-catalytic subunits from ATP synthases, elongation factor Tu and G, signal recognition protein and signal recognition particle receptor, tyrosyl-tRNA and tryptophanyl-tRNA synthetases, and leucyl- and valyl-tRNA synthetases 27 . Marginal densities (commonly referred to as effective priors) fall within calibration densities (that is, user-specified priors) when topologically adjacent calibrations do not overlap temporally, but may differ when they overlap, to ensure the relative age relationships between ancestor-descendant nodes. We consider the marginal densities a reasonable interpretation of the calibration evidence given the phylogeny; we are not attempting to test the hypothesis that the fossil record is an accurate temporal archive of evolutionary history because it is not 28 . The duplicated LUCA node age estimates we obtained under the autocorrelated rates (geometric Brownian motion (GBM)) 29 , 30 and independent-rates log-normal (ILN) 31 , 32 relaxed-clock models with our partitioned dataset (GBM, 4.18–4.33 Ga; ILN, 4.09–4.32 Ga; Fig. 1 ) fall within our composite age estimate for LUCA ranging from 3.94 Ga to 4.52 Ga, comparable to previous studies 13 , 18 , 33 . Dating analyses based on single genes, or concatenations that excluded each gene in turn, returned compatible timescales (Extended Data Figs. 1 and 2 and ‘Additional methods’ in Methods ).
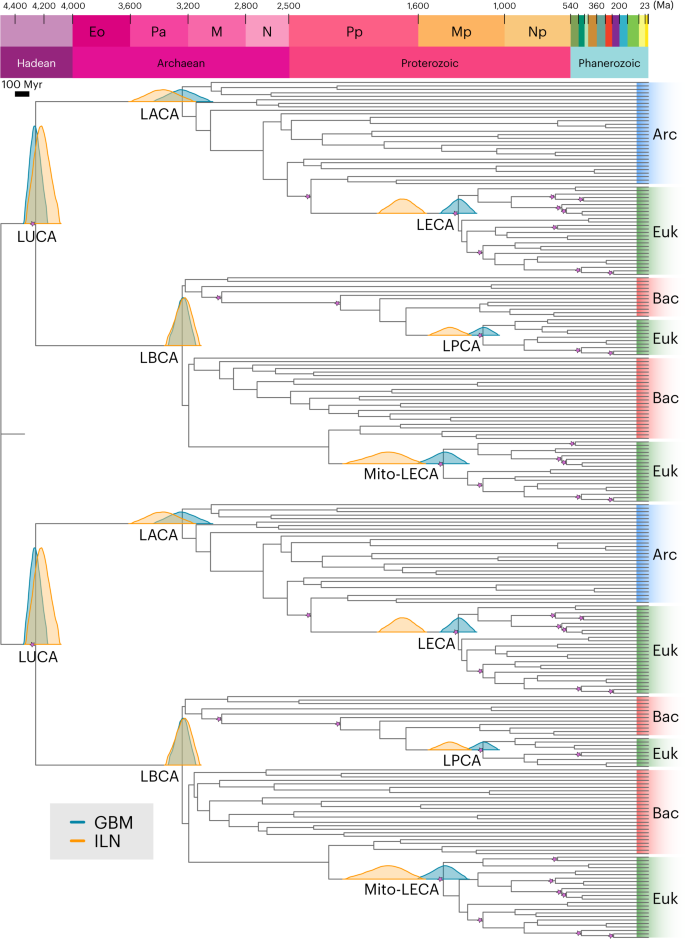
Our results suggest that LUCA lived around 4.2 Ga, with a 95% confidence interval spanning 4.09–4.33 Ga under the ILN relaxed-clock model (orange) and 4.18–4.33 Ga under the GBM relaxed-clock model (teal). Under a cross-bracing approach, nodes corresponding to the same species divergences (that is, mirrored nodes) have the same posterior time densities. This figure shows the corresponding posterior time densities of the mirrored nodes for the last universal, archaeal, bacterial and eukaryotic common ancestors (LUCA, LACA, LBCA and LECA, respectively); the last common ancestor of the mitochondrial lineage (Mito-LECA); and the last plastid-bearing common ancestor (LPCA). Purple stars indicate nodes calibrated with fossils. Arc, Archaea; Bac, Bacteria; Euk, Eukarya.
LUCA’s physiology
To estimate the physiology of LUCA, we first inferred an updated microbial phylogeny from 57 phylogenetic marker genes (see ‘Universal marker genes’ in Methods ) on 700 genomes, comprising 350 Archaea and 350 Bacteria 15 . This tree was in good agreement with recent phylogenies of the archaeal and bacterial domains of life 34 , 35 . For example, the TACK 36 and Asgard clades of Archaea 37 , 38 , 39 and Gracilicutes within Bacteria 40 , 41 were recovered as monophyletic. However, the analysis was equivocal as to the phylogenetic placement of the Patescibacteria (CPR) 42 and DPANN 43 , which are two small-genome lineages that have been difficult to place in trees. Approximately unbiased 44 tests could not distinguish the placement of these clades, neither at the root of their respective domains nor in derived positions, with CPR sister to Chloroflexota (as reported recently in refs. 35 , 41 , 45 ) and DPANN sister to Euryarchaeota. To account for this phylogenetic uncertainty, we performed LUCA reconstructions on two trees: our maximum likelihood (ML) tree (topology 1; Extended Data Fig. 3 ) and a tree in which CPR were placed as the sister of Chloroflexota, with DPANN sister to all other Archaea (topology 2; Extended Data Fig. 4 ). In both cases, the gene families mapped to LUCA were very similar (correlation of LUCA presence probabilities (PP), r = 0.6720275, P < 2.2 × 10 − 16 ). We discuss the results on the tree with topology 2 and discuss the residual differences in Supplementary Information , ‘Topology 1’ (Supplementary Data 1 ).
We used the probabilistic gene- and species-tree reconciliation algorithm ALE 46 to infer the evolution of gene family trees for each sampled entry in the KEGG Orthology (KO) database 47 on our species tree. ALE infers the history of gene duplications, transfers and losses based on a comparison between a distribution of bootstrapped gene trees and the reference species tree, allowing us to estimate the probability that the gene family was present at a node in the tree 35 , 48 , 49 . This reconciliation approach has several advantages for drawing inferences about LUCA. Most gene families have experienced gene transfer since the time of LUCA 50 , 51 and so explicitly modelling transfers enables us to include many more gene families in the analysis than has been possible using previous approaches. As the analysis is probabilistic, we can also account for uncertainty in gene family origins and evolutionary history by averaging over different scenarios using the reconciliation model. Using this approach, we estimated the probability that each KEGG gene family (KO) was present in LUCA and then used the resulting probabilities to construct a hypothetical model of LUCA’s gene content, metabolic potential (Fig. 2 ) and environmental context (Fig. 3 ). Using the KEGG annotation is beneficial because it allows us to connect our inferences to curated functional annotations; however, it has the drawback that some widespread gene families that were likely present in LUCA are divided into multiple KO families that individually appear to be restricted to particular taxonomic groups and inferred to have arisen later. To account for this limitation, we also performed an analysis of COG (Clusters of Orthologous Genes) 52 gene families, which correspond to more coarse-grained functional annotations (Supplementary Data 2 ).
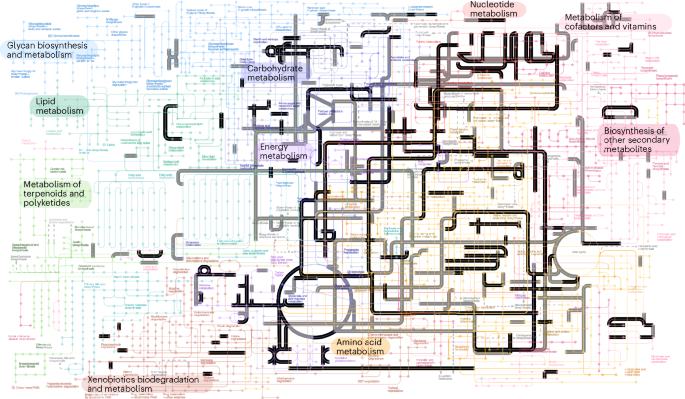
In black: enzymes and metabolic pathways inferred to be present in LUCA with at least PP = 0.75, with sampling in both prokaryotic domains. In grey: those inferred in our least-stringent threshold of PP = 0.50. The analysis supports the presence of a complete WLP and an almost complete TCA cycle across multiple confidence thresholds. Metabolic maps derived from KEGG 47 database through iPath 109 . GPI, glycosylphosphatidylinositol; DDT, 1,1,1-trichloro-2,2-bis(p-chlorophenyl)ethane.
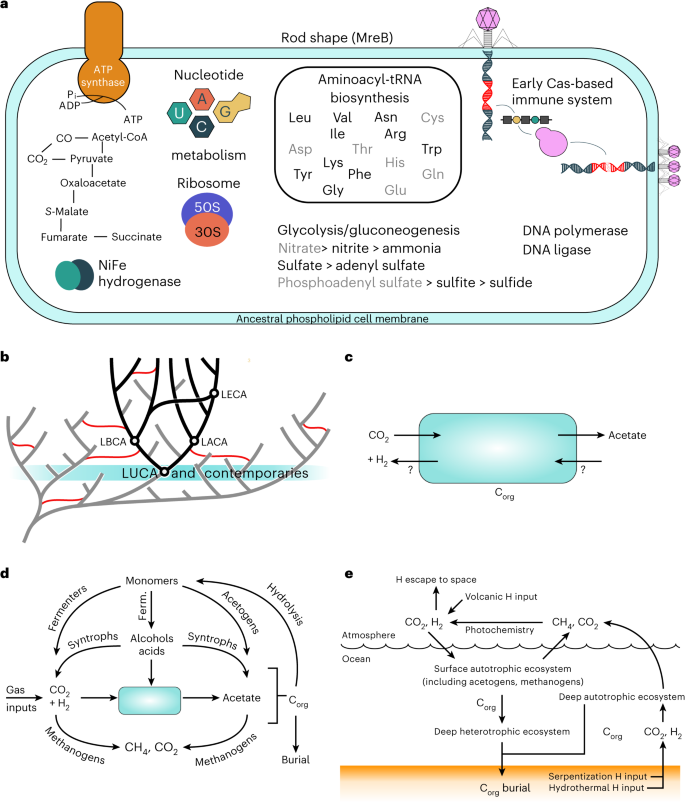
a , A representation of LUCA based on our ancestral gene content reconstruction. Gene names in black have been inferred to be present in LUCA under the most-stringent threshold (PP = 0.75, sampled in both domains); those in grey are present at the least-stringent threshold (PP = 0.50, without a requirement for presence in both domains). b , LUCA in the context of the tree of life. Branches on the tree of life that have left sampled descendants today are coloured black, those that have left no sampled descendants are in grey. As the common ancestor of extant cellular life, LUCA is the oldest node that can be reconstructed using phylogenetic methods. It would have shared the early Earth with other lineages (highlighted in teal) that have left no descendants among sampled cellular life today. However, these lineages may have left a trace in modern organisms by transferring genes into the sampled tree of life (red lines) before their extinction. c , LUCA’s chemoautotrophic metabolism probably relied on gas exchange with the immediate environment to achieve organic carbon (C org ) fixation via acetogenesis and it may also have run the metabolism in reverse. d , LUCA within the context of an early ecosystem. The CO 2 and H 2 that fuelled LUCA’s plausibly acetogenic metabolism could have come from both geochemical and biotic inputs. The organic matter and acetate that LUCA produced could have created a niche for other metabolisms, including ones that recycled CO 2 and H 2 (as in modern sediments). e , LUCA in an Earth system context. Acetogenic LUCA could have been a key part of both surface and deep (chemo)autotrophic ecosystems, powered by H 2 . If methanogens were also present, hydrogen would be released as CH 4 to the atmosphere, converted to H 2 by photochemistry and thus recycled back to the surface ecosystem, boosting its productivity. Ferm., fermentation.
Genome size and cellular features
By using modern prokaryotic genomes as training data, we used a predictive model to estimate the genome size and the number of protein families encoded by LUCA based on the relationship between the number of KEGG gene families and the total number of proteins encoded by modern prokaryote genomes (Extended Data Figs. 5 and 6 ). On the basis of the PPs for KEGG KO gene families, we identified a conservative subset of 399 KOs that were likely to be present in LUCA, with PPs ≥0.75, and found in both Archaea and Bacteria (Supplementary Data 1 ); these families form the basis of our metabolic reconstruction. However, by integrating over the inferred PPs of all KO gene families, including those with low probabilities, we also estimate LUCA’s genome size. Our predictive model estimates a genome size of 2.75 Mb (2.49–2.99 Mb) encoding 2,657 (2,451–2,855) proteins ( Methods ). Although we can estimate the number of genes in LUCA’s genome, it is more difficult to identify the specific gene families that might have already been present in LUCA based on the genomes of modern Archaea and Bacteria. It is likely that the modern version of the pathways would be considered incomplete based on LUCA’s gene content through subsequent evolutionary changes. We should therefore expect reconstructions of metabolic pathways to be incomplete due to this phylogenetic noise and other limitations of the analysis pipeline. For example, when looking at genes and pathways that can uncontroversially be mapped to LUCA, such as the ribosome and aminoacyl-tRNA synthetases for implementing the genetic code, we find that we map many (but not all) of the key components to LUCA (see ‘Notes’ in Supplementary Information ). We interpret this to mean that our reconstruction is probably incomplete but our interpretation of LUCA’s metabolism relies on our inference of pathways, not individual genes.
The inferred gene content of LUCA suggests it was an anaerobe as we do not find support for the presence of terminal oxidases (Supplementary Data 1 ). Instead we identified almost all genes encoding proteins of the archaeal (and most of the bacterial) versions of the Wood–Ljungdahl pathway (WLP) (PP > 0.7), indicating that LUCA had the potential for acetogenic growth and/or carbon fixation 53 , 54 , 55 (Supplementary Data 3 ). LUCA encoded some NiFe hydrogenase subunits ( K06281 , PP = 0.90; K14126 , PP = 0.92), which may have enabled growth on hydrogen (see ‘Notes’ in Supplementary Information ). Complexes involved in methanogenesis such as methyl-coenzyme M reductase and tetrahydromethanopterin S-methyltransferase were inferred to be absent, suggesting that LUCA was unlikely to function as a modern methanogen. We found strong support for some components of the TCA cycle (including subunits of oxoglutarate/2-oxoacid ferredoxin oxidoreductase ( K00175 and K00176 ), succinate dehydrogenase ( K00239 ) and homocitrate synthase ( K02594 )), although some steps are missing. LUCA was probably capable of gluconeogenesis/glycolysis in that we find support for most subunits of enzymes involved in these pathways (Supplementary Data 1 and 3 ). Considering the presence of the WLP, this may indicate that LUCA had the ability to grow organoheterotrophically and potentially also autotrophically. Gluconeogenesis would have been important in linking carbon fixation to nucleotide biosynthesis via the pentose phosphate pathway, most enzymes of which seem to be present in LUCA (see ‘Notes’ in Supplementary Information ). We found no evidence that LUCA was photosynthetic, with low PPs for almost all components of oxygenic and anoxygenic photosystems (Supplementary Data 3 ).
We find strong support for the presence of ATP synthase, specifically, the A ( K02117 , PP = 0.98) and B ( K02118 , PP = 0.94) subunit components of the hydrophilic V/A1 subunit, and the I (subunit a, K02123 , PP = 0.99) and K (subunit c, K02124 , PP = 0.82) subunits of the transmembrane V/A0 subunit. In addition, if we relax the sampling threshold, we also infer the presence of the F1-type β-subunit ( K02112 , PP = 0.94). This is consistent with many previous studies that have mapped ATP synthase subunits to LUCA 6 , 17 , 18 , 56 , 57 .
We obtain moderate support for the presence of pathways for assimilatory nitrate (ferredoxin-nitrate reductase, K00367 , PP = 0.69; ferredoxin-nitrite reductase, K00367 , PP = 0.53) and sulfate reduction (sulfate adenylyltransferase, K00957 , PP = 0.80, and K00958 , PP = 0.73; sulfite reductase, K00392 , PP = 0.82; phosphoadenosine phosphosulfate reductase, K00390 , PP = 0.56), probably to fuel amino acid biosynthesis, for which we inferred the presence of 37 partially complete pathways.
We found support for the presence of 19 class 1 CRISPR–Cas effector protein families in the genome of LUCA, including types I and III (cas3, K07012 , PP = 0.80, and K07475 , PP = 0.74; cas10, K07016 , PP = 0.96, and K19076 , PP = 0.67; and cas7, K07061 , PP = 0.90, K09002 , PP = 0.84, K19075 , PP = 0.97, K19115 , PP = 0.98, and K19140 , PP = 0.80). The absence of Cas1 and Cas2 may suggest LUCA encoded an early Cas system with the means to deliver an RNA-based immune response by cutting (Cas6/Cas3) and binding (CSM/Cas10) RNA, but lacking the full immune-system-site CRISPR. This supports the idea that the effector stage of CRISPR–Cas immunity evolved from RNA sensing for signal transduction, based on the similarities in RNA binding modules of the proteins 58 . This is consistent with the idea that cellular life was already involved in an arms race with viruses at the time of LUCA 59 , 60 . Our results indicate that an early Cas system was an ancestral immune system of extant cellular life.
Altogether, our metabolic reconstructions suggest that LUCA was a relatively complex organism, similar to extant Archaea and Bacteria 6 , 7 . On the basis of ancient duplications of the Sec and ATP synthase genes before LUCA, along with high PPs for key components of those systems, membrane-bound ATP synthase subunits, genes involved in peptidoglycan synthesis ( mraY , K01000 ; murC , K01924 ) and the cytoskeletal actin-like protein, MreB ( K03569 ) (Supplementary Data 3 ), it is highly likely that LUCA possessed the core cellular apparatus of modern prokaryotic life. This might include the basic constituents of a phospholipid membrane, although our analysis did not conclusively establish its composition. In particular, we recovered the following enzymes involved in the synthesis of ether and ester lipids, (alkyldihydroxyacetonephosphate synthase, glycerol 3-phosphate and glycerol 1-phosphate) and components of the mevalonate pathway (mevalonate 5-phosphate dehydratase (PP = 0.84), hydroxymethylglutaryl-CoA reductase (PP = 0.52), mevalonate kinase (PP = 0.51) and hydroxymethylglutaryl-CoA synthase (PP = 0.51)).
Compared with previous estimates of LUCA’s gene content, we find 81 overlapping COG gene families with the consensus dataset of ref. 7 and 69 overlapping KOs with the dataset of ref. 6 . Key points of agreement between previous studies include the presence of signal recognition particle protein, ffh (COG0541, K03106 ) 7 used in the targeting and delivery of proteins for the plasma membrane, a high number of aminoacyl-tRNA synthetases for amino acid synthesis and glycolysis/gluconeogenesis enzymes.
Ref. 6 inferred LUCA to be a thermophilic anaerobic autotroph using the WLP for carbon fixation based on the presence of a single enzyme (CODH), and similarly suggested that LUCA was capable of nitrogen fixation using a nitrogenase. Our reconstruction agrees with ref. 6 that LUCA was an anaerobic autotroph using the WLP for carbon fixation, but we infer the presence of a much more complete WLP than that previously obtained. We did not find strong evidence for nitrogenase or nitrogen fixation, and the reconstruction was not definitive with respect to the optimal growth environment of LUCA.
We used a probabilistic approach to reconstruct LUCA—that is, we estimated the probability with which each gene family was present in LUCA based on a model of how gene families evolve along an overarching species tree. This approach differs from analyses of phylogenetic presence–absence profiles 3 , 4 , 9 or those that used filtering criteria (such as broadly distributed or highly vertically evolving families) to define a high-confidence subset of modern genes that might have been present in LUCA. Our reconstruction maps many more genes to LUCA—albeit each with lower probability—than previous analyses 8 and yields an estimate of LUCA’s genome size that is within the range of modern prokaryotes. The result is an incomplete picture of a cellular organism that was prokaryote grade rather than progenotic 2 and that, similarly to prokaryotes today, probably existed as part of an ecosystem. As the common ancestor of sampled, extant prokaryotic life, LUCA is the oldest node on the species tree that we can reconstruct via phylogenomics but, as Fig. 3 illustrates, it was already the product of a highly innovative period in evolutionary history during which most of the core components of cells were established. By definition, we cannot reconstruct LUCA’s contemporaries using phylogenomics but we can propose hypotheses about their physiologies based on the reconstructed LUCA whose features immediately suggest the potential for interactions with other prokaryotic metabolisms.
LUCA’s environment, ecosystem and Earth system context
The inference that LUCA used the WLP helps constrain the environment and ecology in which it could have lived. Modern acetogens can grow autotrophically on H 2 (and CO 2 ) or heterotrophically on a wide range of alternative electron donors including alcohols, sugars and carboxylic acids 55 . This metabolic flexibility is key to their modern ecological success. Acetogenesis, whether autotrophic or heterotrophic, has a low energy yield and growth efficiency (although use of the reductive acetyl-CoA pathway for both energy production and biosynthesis reduces the energy cost of biosynthesis). This would be consistent with an energy-limited early biosphere 61 .
If LUCA functioned as an organoheterotrophic acetogen, it was necessarily part of an ecosystem containing autotrophs providing a source of organic compounds (because the abiotic source flux of organic molecules was minimal on the early Earth). Alternatively, if LUCA functioned as a chemoautotrophic acetogen it could (in principle) have lived independently off an abiotic source of H 2 (and CO 2 ). However, it is implausible that LUCA would have existed in isolation as the by-products of its chemoautotrophic metabolism would have created a niche for a consortium of other metabolisms (as in modern sediments) (Fig. 3d ). This would include the potential for LUCA itself to grow as an organoheterotroph.
A chemoautotrophic acetogenic LUCA could have occupied two major potential habitats (Fig. 3e ): the first is the deep ocean where hydrothermal vents and serpentinization of sea-floor provided a source of H 2 (ref. 62 ). Consistent with this, we find support for the presence of reverse gyrase (PP = 0.97), a hallmark enzyme of hyperthermophilic prokaryotes 6 , 63 , 64 , 65 , which would not be expected if early life existed at the ocean surface (although the evolution of reverse gyrase is complex 63 ; see ‘Reverse gyrase’ in Supplementary Information ). The second habitat is the ocean surface where the atmosphere would have provided a source of H 2 derived from volcanoes and metamorphism. Indeed, we detected the presence of spore photoproduct lyase (COG1533, K03716 , PP = 0.88) that in extant organisms repairs methylene-bridged thymine dimers occurring in spore DNA as a result of damage induced through ultraviolet (UV) radiation 66 , 67 . However, this gene family also occurs in modern taxa that neither form endospores nor dwell in environments where they are likely to accrue UV damage to their DNA and so is not an exclusive hallmark of environments exposed to UV. Previous studies often favoured a deep-ocean environment for LUCA as early life would have been better protected there from an episode of LHB. However, if the LHB was less intense than initially proposed 20 , 22 , or just a sampling artefact 21 , these arguments weaken. Another possibility may be that LUCA inhabited a shallow hydrothermal vent or a hot spring.
Hydrogen fluxes in these ecosystems could have been several times higher on the early Earth (with its greater internal heat source) than today. Volcanism today produces ~1 × 10 12 mol H 2 yr −1 and serpentinization produces ~0.4 × 10 12 mol H 2 yr − 1 . With the present H 2 flux and the known scaling of the H 2 escape rate to space, an abiotic atmospheric concentration of H 2 of ~150 ppmv is predicted 68 . Chemoautotrophic acetogens would have locally drawn down the concentration of H 2 (in either surface or deep niche) but their low growth efficiency would ensure H 2 (and CO 2 ) remained available. This and the organic matter and acetate produced would have created niches for other metabolisms, including methanogenesis (Fig. 3d ).
On the basis of thermodynamic considerations, CH 4 and CO 2 are expected to be the eventual metabolic end products of the resulting ecosystem, with a small fraction of the initial hydrogen consumption buried as organic matter. The resulting flux of CH 4 to the atmosphere would fuel photochemical H 2 regeneration and associated productivity in the surface ocean (Fig. 3e ). Existing models suggest the resulting global H 2 recycling system is highly effective, such that the supply flux of H 2 to the surface could have exceeded the volcanic input of H 2 to the atmosphere by at least an order of magnitude, in turn implying that the productivity of such a biosphere was boosted by a comparable factor 69 . Photochemical recycling to CO would also have supported a surface niche for organisms consuming CO (ref. 69 ).
In deep-ocean habitats, there could be some localized recycling of electrons (Fig. 3d ) but a quantitative loss of highly insoluble H 2 and CH 4 to the atmosphere and minimal return after photochemical conversion of CH 4 to H 2 means global recycling to depth would be minimal (Fig. 3e ). Hence the surface environment for LUCA could have become dominant (albeit recycling of the resulting organic matter could be spread through ocean depth; ‘Deep heterotrophic ecosystem’ in Fig. 3e ). The global net primary productivity of an early chemoautotrophic biosphere including acetogenic LUCA and methanogens could have been of order ~1 × 10 12 to 7 × 10 12 mol C yr − 1 (~3 orders of magnitude less than today) 69 .
The nutrient supply (for example, N) required to support such a biosphere would need to balance that lost in the burial flux of organic matter. Earth surface redox balance dictates that hydrogen loss to space and burial of electrons/hydrogen must together balance input of electrons/hydrogen. Considering contemporary H 2 inputs, and the above estimate of net primary productivity, this suggests a maximum burial flux in the order of ~10 12 mol C yr − 1 , which, with contemporary stoichiometry (C:N ratio of ~7) could demand >10 11 mol N yr − 1 . Lightning would have provided a source of nitrite and nitrate 70 , consistent with LUCA’s inferred pathways of nitrite and (possibly) nitrate reduction. However, it would only have been of the order 3 × 10 9 mol N yr − 1 (ref. 71 ). Instead, in a global hydrogen-recycling system, HCN from photochemistry higher in the atmosphere, deposited and hydrolysed to ammonia in water, would have increased available nitrogen supply by orders of magnitude toward ~3 × 10 12 mol N yr − 1 (refs. 71 , 72 ). This HCN pathway is consistent with the anomalously light nitrogen isotopic composition of the earliest plausible biogenic matter of 3.8–3.7 Ga (ref. 73 ), although that considerably postdates our inferred age of LUCA. These considerations suggest that the proposed LUCA biosphere (Fig. 3e ) would have been energy or hydrogen limited not nitrogen limited.
Conclusions
By treating gene presence probabilistically, our reconstruction maps many more genes (2,657) to LUCA than previous analyses and results in an estimate of LUCA’s genome size (2.75 Mb) that is within the range of modern prokaryotes. The result is a picture of a cellular organism that was prokaryote grade rather than progenotic 2 and that probably existed as a component of an ecosystem, using the WLP for acetogenic growth and carbon fixation. We cannot use phylogenetics to reconstruct other members of this early ecosystem but we can infer their physiologies based on the metabolic inputs and outputs of LUCA. How evolution proceeded from the origin of life to early communities at the time of LUCA remains an open question, but the inferred age of LUCA (~4.2 Ga) compared with the origin of the Earth and Moon suggests that the process required a surprisingly short interval of geologic time.
Universal marker genes
A list of 298 markers were identified by creating a non-redundant list of markers used in previous studies on archaeal and bacterial phylogenies 10 , 35 , 38 , 74 , 75 , 76 , 77 , 78 , 79 . These markers were mapped to the corresponding COG, arCOG and TIGRFAM profile to identify which profile is best suited to extract proteins from taxa of interest. To evaluate whether the markers cover all archaeal and bacterial diversity, proteins from a set of 574 archaeal and 3,020 bacterial genomes were searched against the COG, arCOG and TIGRFAM databases using hmmsearch (v.3.1b2; settings, hmmsearch–tblout output–domtblout–notextw) 52 , 80 , 81 , 82 . Only hits with an e-value less than or equal to 1 × 10 −5 were investigated further and for each protein the best hit was determined based on the e-value (expect value) and bit-score. Results from all database searches were merged based on the protein identifiers and the table was subsetted to only include hits against the 298 markers of interest. On the basis of this table we calculated whether the markers occurred in Archaea, Bacteria or both Archaea and Bacteria. Markers were only included if they were present in at least 50% of taxa and contained less than 10% of duplications, leaving a set of 265 markers. Sequences for each marker were aligned using MAFFT L-INS-i v.7.407 (ref. 83 ) for markers with less than 1,000 sequences or MAFFT 84 for those with more than 1,000 sequences (setting, –reorder) 84 and sequences were trimmed using BMGE 85 , set for amino acids, a BLOcks SUbstitution Matrix 30 similarity matrix, with a entropy score of 0.5 (v.1.12; settings, -t AA -m BLOSUM30 -h 0.5). Single gene trees were generated with IQ-TREE 2 (ref. 86 ), using the LG substitution matrix, with ten-profile mixture models, four CPUs, with 1,000 ultrafast bootstraps optimized by nearest neighbour interchange written to a file retaining branch lengths (v.2.1.2; settings, -m LG + C10 + F + R -nt 4 -wbtl -bb 1,000 -bnni). These single gene trees were investigated for archaeal and bacterial monophyly and the presence of paralogues. Markers that failed these tests were not included in further analyses, leaving a set of 59 markers (3 arCOGs, 46 COGs and 10 TIGRFAMs) suited for phylogenies containing both Archaea and Bacteria (Supplementary Data 4 ).
Marker gene sequence selection
To limit selecting distant paralogues and false positives, we used a bidirectional or reciprocal approach to identify the sequences corresponding to the 59 single-copy markers. In the first inspection (query 1), the 350 archaeal and 350 bacterial reference genomes were queried against all arCOG HMM (hidden Markov model) profiles (All_Arcogs_2018.hmm), all COG HMM profiles (NCBI_COGs_Oct2020.hmm) and all TIGRFAM HMM profiles (TIGRFAMs_15.0_HMM.LIB) using a custom script built on hmmsearch: hmmsearchTable <genomes.faa> <database.hmm> -E 1 × 10 −5 >HMMscan_Output_e5 (HMMER v.3.3.2) 87 . HMM profiles corresponding to the 59 single-copy marker genes (Supplementary Data 4 ) were extracted from each query and the best-hit sequences were identified based on the e-value and bit-score. We used the same custom hmmsearchTable script and conditions (see above) in the second inspection (query 2) to query the best-hit sequences identified above against the full COG HMM database (NCBI_COGs_Oct2020.hmm). Results were parsed and the COG family assigned in query 2 was compared with the COG family assigned to sequences based on the marker gene identity (Supplementary Data 4 ). Sequence hits were validated using the matching COG identifier, resulting in 353 mismatches (that is, COG family in query 1 does not match COG family in query 2) that were removed from the working set of marker gene sequences. These sequences were aligned using MAFFT L-INS-i 83 and then trimmed using BMGE 85 with a BLOSUM30 matrix. Individual gene trees were inferred under ML using IQ-TREE 2 (ref. 86 ) with model fitting, including both the default homologous substitution models and the following complex heterogeneous substitution models (LG substitution matrices with 10–60-profile mixture models, with empirical base frequencies and a discrete gamma model with four categories accounting for rate heterogeneity across sites): LG + C60 + F + G, LG + C50 + F + G, LG + C40 + F + G, LG + C30 + F + G, LG + C20 + F + G and LG + C10 + F + G, with 10,000 ultrafast bootstraps and 10 independent runs to avoid local optima. These 59 gene trees were manually inspected and curated over multiple rounds. Any horizontal gene transfer events, paralogous genes or sequences that violated domain monophyly were removed and two genes (arCOG01561, tuf ; COG0442, ProS ) were dropped at this stage due to the high number of transfer events, resulting in 57 single-copy orthologues for further tree inference.
Species-tree inference
These 57 orthologous sequences were concatenated and ML trees were inferred after three independent runs with IQ-TREE 2 (ref. 86 ) using the same model fitting and bootstrap settings as described above. The tree with the highest log-likelihood of the three runs was chosen as the ML species tree (topology 1). To test the effect of removing the CPR bacteria, we removed all CPR bacteria from the alignment before inferring a species tree (same parameters as above). We also performed approximately unbiased 44 tree topology tests (with IQ-TREE 2 (ref. 86 ), using LG + C20 + F + G) when testing the significance of constraining the species-tree topology (ML tree; Supplementary Fig. 1 ) to have a DPANN clade as sister to all other Archaea (same parameters as above but with a minimally constrained topology with monophyletic Archaea and DPANN sister to other Archaea present in a polytomy (Supplementary Fig. 2 )) and testing a constraint of CPR to be sister to Chloroflexi (Supplementary Fig. 3 ), and a combination of both the DPANN and CPR constraints (topology 2); these were tested against the ML topology, both using the normal 20 amino acid alignments and also with Susko–Roger recoding 88 .
Gene families
For the 700 representative species 15 , gene family clustering was performed using EGGNOGMAPPER v.2 (ref. 89 ), with the following parameters: using the DIAMOND 90 search, a query cover of 50% and an e-value threshold of 0.0000001. Gene families were collated using their KEGG 47 identifier, resulting in 9,365 gene families. These gene families were then aligned using MAFFT 84 v.7.5 with default settings and trimmed using BMGE 85 (with the same settings as above). Five independent sets of ML trees were then inferred using IQ-TREE 2 (ref. 86 ), using LG + F + G, with 1,000 ultrafast bootstrap replicates. We also performed a COG-based clustering analysis in which COGs were assigned based on the modal COG identifier annotated for each KEGG gene family based on the results from EGGNOGMAPPER v.2 (ref. 89 ). These gene families were aligned, trimmed and one set of gene trees (with 1,000 ultrafast bootstrap replicates) was inferred using the same parameters as described above for the KEGG gene families.
Reconciliations
The five sets of bootstrap distributions were converted into ALE files, using ALEobserve, and reconciled against topology 1 and topology 2 using ALEml_undated 91 with the fraction missing for each genome included (where available). Gene family root origination rates were optimized for each COG functional category as previously described 35 and families were categorized into four different groups based on the probability of being present in the LUCA node in the tree. The most-stringent category was that with sampling above 1% in both domains and a PP ≥ 0.75, another category was with PP ≥ 0.75 with no sampling requirement, another with PP ≥ 0.5 with the sampling requirement; the least stringent was PP ≥ 0.5 with no sampling requirement. We used the median probability at the root from across the five runs to avoid potential biases from failed runs in the mean and to account for variation across bootstrap distributions (see Supplementary Fig. 4 for distributions of the inferred ratio of duplications, transfers and losses for all gene families across all tips in the species tree; see Supplementary Data 5 for the inferred duplications, transfers and losses ratios for LUCA, the last bacterial common ancestor and the last archaeal common ancestor).
Metabolic pathway analysis
Metabolic pathways for gene families mapped to the LUCA node were inferred using the KEGG 47 website GUI and metabolic completeness for individual modules was estimated with Anvi’o 92 (anvi-estimate-metabolism), with pathwise completeness.
Additional testing
We tested for the effects of model complexity on reconciliation by using posterior mean site frequency LG + C20 + F + G across three independent runs in comparison with 3 LG + F + G independent runs. We also performed a 10% subsampling of the species trees and gene family alignments across two independent runs for two different subsamples, one with and one without the presence of Asgard archaea. We also tested the likelihood of the gene families under a bacterial root (between Terrabacteria and Gracilicutes) using reconciliations of the gene families under a species-tree topology rooted as such.
Fossil calibrations
On the basis of well-established geological events and the fossil record, we modelled 13 uniform densities to constrain the maximum and minimum ages of various nodes in our phylogeny. We constrained the bounds of the uniform densities to be either hard (no tail probability is allowed after the age constraint) or soft (a 2.5% tail probability is allowed after the age constraint) depending on the interpretation of the fossil record ( Supplementary Information ). Nodes that refer to the same duplication event are identified by MCMCtree as cross-braced (that is, one is chosen as the ‘driver’ node, the rest are ‘mirrored’ nodes). In other words, the sampling during the Markov chain Monte Carlo (MCMC) for cross-braced nodes is not independent: the same posterior time density is inferred for matching mirror–driver nodes (see ‘Additional methods’ for details on our cross-bracing approach).
Timetree inference analyses
Timetree inference with the program MCMCtree (PAML v.4.10.7 (ref. 93 )) proceeded under both the GBM and ILN relaxed-clock models. We specified a vague rate prior with the shape parameter equal to 2 and the scale parameter equal to 2.5: Γ(2, 2.5). This gamma distribution is meant to account for the uncertainty on our estimate for the mean evolutionary rate, ~0.81 substitutions per site per time unit, which we calculated by dividing the tree height of our best-scoring ML tree ( Supplementary Information ) into the estimated mean root age of our phylogeny (that is, 4.520 Ga, time unit = 10 9 years; see ‘Fossil calibrations’ in Supplementary Information for justifications on used calibrations). Given that we are estimating very deep divergences, the molecular clock may be seriously violated. Therefore, we applied a very diffuse gamma prior on the rate variation parameter ( σ 2 ), Γ(1, 10), so that it is centred around σ 2 = 0.1. To incorporate our uncertainty regarding the tree shape, we specified a uniform kernel density for the birth–death sampling process by setting the birth and death processes to 1, λ (per-lineage birth rate) = μ (per-lineage death rate) = 1, and the sampling frequency to ρ (sampling fraction) = 0.1. Our main analysis consisted of inferring the timetree for the partitioned dataset under both the GBM and the ILN relaxed-clock models in which nodes that correspond to the same divergences are cross-braced (that is, hereby referred to as cross-bracing A). In addition, we ran 10 additional inference analyses to benchmark the effect that partitioning, cross-bracing and relaxed-clock models can have on species divergence time estimation: (1) GBM + concatenated alignment + cross-bracing A, (2) GBM + concatenated alignment + cross-bracing B (only nodes that correspond to the same divergences for which there are fossil constraints are cross-braced), (3) GBM + concatenated alignment + without cross-bracing, (4) GBM + partitioned alignment + cross-bracing B, (5) GBM + partitioned alignment + without cross-bracing, (6) ILN + concatenated alignment + cross-bracing A, (7) ILN + concatenated alignment + cross-bracing B, (8) ILN + concatenated alignment + without cross-bracing, (9) ILN + partitioned alignment + cross-bracing B, and (10) ILN + partitioned alignment + without cross-bracing. Lastly, we used (1) individual gene alignments, (2) a leave-one-out strategy (rate prior changed for alignments without ATP and Leu , Γ(2, 2.2), and without Tyr , Γ(2, 2.3), but was Γ(2, 2.5) for the rest; see ‘Additional methods’), and (3) a more complex substitution model 94 to assess their impact on timetree inference. Refer to ‘Additional methods’ for details on how we parsed the dataset we used for timetree inference analyses, ran PAML programs CODEML and MCMCtree to approximate the likelihood calculation 95 , and carried out the MCMC diagnostics for the results obtained under each of the previously mentioned scenarios.
We simulated 100 samples of ‘KEGG genomes’ based on the probabilities of each of the (7,467) gene families being present in LUCA using the random.rand function in numpy 96 . The mean number of KEGG gene families was 1,298.25, the 95% HPD (highest posterior density) minimum was 1,255 and the maximum was 1,340. To infer the relationship between the number of KEGG KO gene families encoded by a genome, the number of proteins and the genome size, we used LOESS (locally estimated scatter-plot smoothing) regression to estimate the relationship between the number of KOs and (1) the number of protein-coding genes and (2) the genome size for the 700 prokaryotic genomes used in the LUCA reconstruction. To ensure that our inference of genome size is robust to uncertainty in the number of paralogues that can be expected to have been present in LUCA, we used the presence of probability for each of these KEGG KO gene families rather than the estimated copy number. We used the predict function to estimate the protein-coding genes and genome size of LUCA using these models and the simulated gene contents encoded with 95% confidence intervals.
Additional methods
Cross-bracing approach implemented in mcmctree.
The PAML program MCMCtree was implemented to allow for the analysis of duplicated genes or proteins so that some nodes in the tree corresponding to the same speciation events in different paralogues share the same age. We used the tree topology depicted in Supplementary Fig. 5 to explain how users can label driver or mirror nodes (more on these terms below) so that the program identifies them as sharing the same speciation events. The tree topology shown in Supplementary Fig. 5 can be written in Newick format as:
(((A1,A2),A3),((B1,B2),B3));
In this example, A and B are paralogues and the corresponding tips labelled as A1–A3 and B1–B3 represent different species. Node r represents a duplication event, whereas other nodes are speciation events. If we want to constrain the same speciation events to have the same age (that is, Supplementary Fig. 5 , see labels a and b (that is, A1–A2 ancestor and B1–B2 ancestor, respectively) and labels v and b (that is, A1–A2–A3 ancestor and B1–B2–B3 ancestor, respectively), we use node labels in the format #1, #2, and so on to identify such nodes:
(((A1, A2) #1, A3) #2, ((B1, B2) [#1 B{0.2, 0.4}], B3) #2) 'B(0.9,1.1)';
Node a and node b are assigned the same label (#1) and so they share the same age ( t ): t a = t b . Similarly, node u and node v have the same age: t u = t v . The former nodes are further constrained by a soft-bound calibration based on the fossil record or geological evidence: 0.2 < t a = t b < 0.4. The latter, however, does not have fossil constraints and thus the only restriction imposed is that both t u and t v are equal. Finally, there is another soft-bound calibration on the root age: 0.9 < t r < 1.1.
Among the nodes on the tree with the same label (for example, those nodes labelled with #1 and those with #2 in our example), one is chosen as the driver node, whereas the others are mirror nodes. If calibration information is provided on one of the shared nodes (for example, nodes a and b in Supplementary Fig. 5 ), the same information therefore applies to all shared nodes. If calibration information is provided on multiple shared nodes, that information has to be the same (for example, you could not constrain node a with a different calibration used to constrain node b in Supplementary Fig. 5 ). The time prior (or the prior on all node ages on the tree) is constructed by using a density at the root of the tree, which is specified by the user (for example, 'B(0.9,1.1)' in our example, which has a minimum of 0.9 and a maximum of 1.1). The ages of all non-calibrated nodes are given by the uniform density. This time prior is similar to that used by ref. 29 . The parameters in the birth–death sampling process ( λ , μ , ρ ; specified using the option BDparas in the control file that executes MCMCtree) are ignored. It is noteworthy that more than two nodes can have the same label but one node cannot have two or more labels. In addition, the prior on rates does not distinguish between speciation and duplication events. The implemented cross-bracing approach can only be enabled if option duplication = 1 is included in the control file. By default, this option is set to 0 and users are not required to include it in the control file (that is, the default option is duplication = 0 ).
Timetree inference
Data parsing.
Eight paralogues were initially selected based on previous work showing a likely duplication event before LUCA: the amino- and carboxy-terminal regions from carbamoyl phosphate synthetase, aspartate and ornithine transcarbamoylases, histidine biosynthesis genes A and F , catalytic and non-catalytic subunits from ATP synthase ( ATP ), elongation factor Tu and G ( EF ), signal recognition protein and signal recognition particle receptor ( SRP ), tyrosyl-tRNA and tryptophanyl-tRNA synthetases ( Tyr ), and leucyl- and valyl-tRNA synthetases ( Leu ) 27 . Gene families were identified using BLASTp 97 . Sequences were downloaded from NCBI 98 , aligned with MUSCLE 99 and trimmed with TrimAl 100 (-strict). Individual gene trees were inferred under the LG + C20 + F + G substitution model implemented in IQ-TREE 2 (ref. 86 ). These trees were manually inspected and curated to remove non-homologous sequences, horizontal gene transfers, exceptionally short or long sequences and extremely long branches. Recent paralogues or taxa of inconsistent and/or uncertain placement inferred with RogueNaRok 101 were also removed. Independent verification of an archaeal or bacterial deep split was achieved using minimal ancestor deviation 102 . This filtering process resulted in the five pairs of paralogous gene families 27 ( ATP , EF , SRP , Tyr and Leu ) that we used to estimate the origination time of LUCA. The alignment used for timetree inference consisted of 246 species, with the majority of taxa having at least two copies (for some eukaryotes, they may be represented by plastid, mitochondrial and nuclear sequences).
To assess the impact that partitioning can have on divergence time estimates, we ran our inference analyses with both a concatenated and a partitioned alignment (that is, gene partitioning scheme). We used PAML v.4.10.7 (programs CODEML and MCMCtree) for all divergence time estimation analyses. Given that a fixed tree topology is required for timetree inference with MCMCtree, we inferred the best-scoring ML tree with IQ-TREE 2 under the LG + C20 + F + G4 (ref. 103 ) model following our previous phylogenetic analyses. We then modified the resulting inferred tree topology following consensus views of species-level relationships 34 , 35 , 104 , which we calibrated with the available fossil calibrations (see below). In addition, we ran three sensitivity tests: timetree inference (1) with each gene alignment separately, (2) under a leave-one-out strategy in which each gene alignment was iteratively removed from the concatenated dataset (for example, remove gene ATP but keep genes EF , Leu , SRP and Tyr concatenated in a unique alignment block; apply the same procedure for each gene family), and (3) using the vector of branch lengths, the gradient vector and the Hessian matrix estimated under a complex substitution model (bsinBV method described in ref. 94 ) with the concatenated dataset used for our core analyses. Four of the gene alignments generated for the leave-one-out strategy had gap-only sequences, these were removed when re-inferring the branch lengths under the LG + C20 + F + G4 model (that is, without ATP , 241 species; without EF , 236 species; without Leu , 243 species; without Tyr , 244 species). We used these trees to set the rate prior used for timetree inference for those alignments not including ATP , EF , Leu or Tyr , respectively. The β value (scale parameter) for the rate prior used when analysing alignments without ATP , Leu and Tyr changed minimally but we updated the corresponding rate priors accordingly (see above). When not including SRP , the alignment did not have any sequences removed (that is, 246 species). All alignments were analysed with the same rate prior, Γ(2, 2.5), except for the three previously mentioned alignments.
Approximating the likelihood calculation during timetree inference using PAML programs
Before timetree inference, we ran the CODEML program to infer the branch lengths of the fixed tree topology, the gradient (first derivative of the likelihood function) and the Hessian matrix (second derivative of the likelihood function); the vectors and matrix are required to approximate the likelihood function in the dating program MCMCtree 95 , an approach that substantially reduces computational time 105 . Given that CODEML does not implement the CAT (Bayesian mixture model for across-site heterogeneity) model, we ran our analyses under the closest available substitution model: LG + F + G4 (model = 3). We calculated the aforementioned vectors and matrix for each of the five gene alignments (that is, required for the partitioned alignment), for the concatenated alignment and for the concatenated alignments used for the leave-one-out strategy; the resulting values are written out in an output file called rst2. We appended the rst2 files generated for each of the five individual alignments in the same order the alignment blocks appear in the partitioned alignment file (for example, the first alignment block corresponds to the ATP gene alignment, and thus the first rst2 block will be the one generated when analysing the ATP gene alignment with CODEML). We named this file in_5parts.BV. There is only one rst2 output file for the concatenated alignments, which we renamed in.BV (main concatenated alignment and concatenated alignments under leave-one-out strategy). When analysing each gene alignment separately, we renamed the rst2 files generated for each gene alignment as in.BV.
MCMC diagnostics
All the chains that we ran with MCMCtree for each type of analysis underwent a protocol of MCMC diagnostics consisting of the following steps: (1) flagging and removal of problematic chains; (2) generating convergence plots before and after chain filtering; (3) using the samples collected by those chains that passed the filters (that is, assumed to have converged to the same target distribution) to summarize the results; (4) assessing chain efficiency and convergence by calculating statistics such as R-hat, tail-ESS and bulk-ESS (in-house wrapper function calling Rstan functions, Rstan v.2.21.7; https://mc-stan.org/rstan/ ); and (5) generating the timetrees for each type of analysis with confidence intervals and high-posterior densities to show the uncertainty surrounding the estimated divergence times. Tail-ESS is a diagnostic tool that we used to assess the sampling efficiency in the tails of the posterior distributions of all estimated divergence times, which corresponds to the minimum of the effective sample sizes for quantiles 2.5% and 97.5%. To assess the sampling efficiency in the bulk of the posterior distributions of all estimated divergence, we used bulk-ESS, which uses rank-normalized draws. Note that if tail-ESS and bulk-ESS values are larger than 100, the chains are assumed to have been efficient and reliable parameter estimates (that is, divergence times in our case). R-hat is a convergence diagnostic measure that we used to compare between- and within-chain divergence time estimates to assess chain mixing. If R-hat values are larger than 1.05, between- and within-chain estimates do not agree and thus mixing has been poor. Lastly, we assessed the impact that truncation may have on the estimated divergence times by running MCMCtree when sampling from the prior (that is, the same settings specified above but without using sequence data, which set the prior distribution to be the target distribution during the MCMC). To summarize the samples collected during this analysis, we carried out the same MCMC diagnostics procedure previously mentioned. Supplementary Fig. 6 shows our calibration densities (commonly referred to as user-specified priors, see justifications for used calibrations above) versus the marginal densities (also known as effective priors) that MCMCtree infers when building the joint prior (that is, a prior built without sequence data that considers age constraints specified by the user, the birth–death with sampling process to infer the time densities for the uncalibrated nodes, the rate priors, and so on). We provide all our results for these quality-control checks in our GitHub repository ( https://github.com/sabifo4/LUCA-divtimes ) and in Extended Data Fig. 1 , Supplementary Figs. 7 – 10 and Supplementary Data 6 . Data, figures and tables used and/or generated following a step-by-step tutorial are detailed in the GitHub repository for each inference analysis.
Additional sensitivity analyses
We compared the divergence times we estimated with the concatenated dataset under the calibration strategy cross-bracing A with those inferred (1) for each gene, (2) for gene alignments analysed under a leave-one-out strategy, and (3) for the main concatenated dataset but when using the vector of branch lengths, the gradient vector and the Hessian matrix estimated under a more complex substitution model 94 . The results are summarized in Extended Data Fig. 2 and Supplementary Data 7 and 8 . The same pattern regarding the calibration densities and marginal densities when the tree topology was pruned (that is, see above for details on the leave-one-out strategy) was observed, and thus no additional figures have been generated. As for our main analyses, the results for these additional sensitivity analyses can be found on our GitHub repository ( https://github.com/sabifo4/LUCA-divtimes ).
Reporting summary
Further information on research design is available in the Nature Portfolio Reporting Summary linked to this article.
Data availability
All data required to interpret, verify and extend the research in this article can be found at our figshare repository at https://doi.org/10.6084/m9.figshare.24428659 (ref. 106 ) for the reconciliation and phylogenomic analyses and GitHub at https://github.com/sabifo4/LUCA-divtimes (ref. 107 ) for the molecular clock analyses. Additional data are available at the University of Bristol data repository, data.bris, at https://doi.org/10.5523/bris.405xnm7ei36d2cj65nrirg3ip (ref. 108 ).
Code availability
All code relating to the dating analysis can be found on GitHub at https://github.com/sabifo4/LUCA-divtimes (ref. 107 ), and other custom scripts can be found in our figshare repository at https://doi.org/10.6084/m9.figshare.24428659 (ref. 106 ).
Theobald, D. L. A formal test of the theory of universal common ancestry. Nature 465 , 219–222 (2010).
Article CAS PubMed Google Scholar
Woese, C. R. & Fox, G. E. The concept of cellular evolution. J. Mol. Evol. 10 , 1–6 (1977).
Mirkin, B. G., Fenner, T. I., Galperin, M. Y. & Koonin, E. V. Algorithms for computing parsimonious evolutionary scenarios for genome evolution, the last universal common ancestor and dominance of horizontal gene transfer in the evolution of prokaryotes. BMC Evol. Biol. 3 , 2 (2003).
Article PubMed PubMed Central Google Scholar
Ouzounis, C. A., Kunin, V., Darzentas, N. & Goldovsky, L. A minimal estimate for the gene content of the last universal common ancestor—exobiology from a terrestrial perspective. Res. Microbiol. 157 , 57–68 (2006).
Gogarten, J. P. & Deamer, D. Is LUCA a thermophilic progenote? Nat. Microbiol 1 , 16229 (2016).
Weiss, M. C. et al. The physiology and habitat of the last universal common ancestor. Nat. Microbiol 1 , 16116 (2016).
Crapitto, A. J., Campbell, A., Harris, A. J. & Goldman, A. D. A consensus view of the proteome of the last universal common ancestor. Ecol. Evol. 12 , e8930 (2022).
Kyrpides, N., Overbeek, R. & Ouzounis, C. Universal protein families and the functional content of the last universal common ancestor. J. Mol. Evol. 49 , 413–423 (1999).
Koonin, E. V. Comparative genomics, minimal gene-sets and the last universal common ancestor. Nat. Rev. Microbiol. 1 , 127–136 (2003).
Harris, J. K., Kelley, S. T., Spiegelman, G. B. & Pace, N. R. The genetic core of the universal ancestor. Genome Res. 13 , 407–412 (2003).
Article CAS PubMed PubMed Central Google Scholar
Javaux, E. J. Challenges in evidencing the earliest traces of life. Nature 572 , 451–460 (2019).
Lepot, K. Signatures of early microbial life from the Archean (4 to 2.5 Ga) eon. Earth Sci. Rev. 209 , 103296 (2020).
Article CAS Google Scholar
Betts, H. C. et al. Integrated genomic and fossil evidence illuminates life’s early evolution and eukaryote origin. Nat. Ecol. Evol. 2 , 1556–1562 (2018).
Zhu, Q. et al. Phylogenomics of 10,575 genomes reveals evolutionary proximity between domains Bacteria and Archaea. Nat. Commun. 10 , 5477 (2019).
Moody, E. R. R. et al. An estimate of the deepest branches of the tree of life from ancient vertically evolving genes. eLife 11 , e66695 (2022).
Schwartz, R. M. & Dayhoff, M. O. Origins of prokaryotes, eukaryotes, mitochondria, and chloroplasts. Science 199 , 395–403 (1978).
Shih, P. M. & Matzke, N. J. Primary endosymbiosis events date to the later Proterozoic 994 with cross-calibrated phylogenetic dating of duplicated ATPase proteins. Proc. Natl Acad. Sci. USA 110 , 996 (2013).
Article Google Scholar
Mahendrarajah, T. A. et al. ATP synthase evolution on a cross-braced dated tree of life. Nat. Commun. 14 , 7456 (2023).
Bottke, W. F. & Norman, M. D. The Late Heavy Bombardment. Annu. Rev. Earth Planet. Sci. 45 , 619–647 (2017).
Reimink, J. et al. Quantifying the effect of late bombardment on terrestrial zircons. Earth Planet. Sci. Lett. 604 , 118007 (2023).
Boehnke, P. & Harrison, T. M. Illusory Late Heavy Bombardments. Proc. Natl Acad. Sci. USA 113 , 10802–10806 (2016).
Ryder, G. Mass flux in the ancient Earth–Moon system and benign implications for the origin of life on Earth. J. Geophys. Res. 107 , 6-1–6-13 (2002).
Google Scholar
Hartmann, W. K. History of the terminal cataclysm paradigm: epistemology of a planetary bombardment that never (?) happened. Geosciences 9 , 285 (2019).
Planavsky, N. J. et al. Evidence for oxygenic photosynthesis half a billion years before the great oxidation event. Nat. Geosci. 7 , 283–286 (2014).
Ossa, F. O. et al. Limited oxygen production in the Mesoarchean ocean. Proc. Natl Acad. Sci. USA 116 , 6647–6652 (2019).
Mukasa, S. B., Wilson, A. H. & Young, K. R. Geochronological constraints on the magmatic and tectonic development of the Pongola Supergroup (Central Region), South Africa. Precambrian Res. 224 , 268–286 (2013).
Zhaxybayeva, O., Lapierre, P. & Gogarten, J. P. Ancient gene duplications and the root(s) of the tree of life. Protoplasma 227 , 53–64 (2005).
Article PubMed Google Scholar
Donoghue, P. C. J. & Yang, Z. The evolution of methods for establishing evolutionary timescales. Philos. Trans. R. Soc. Lond. B Biol. Sci. 371 , 3006–3010 (2016).
Thorne, J. L., Kishino, H. & Painter, I. S. Estimating the rate of evolution of the rate of molecular evolution. Mol. Biol. Evol. 15 , 1647–1657 (1998).
Yang, Z. & Rannala, B. Bayesian estimation of species divergence times under a molecular clock using multiple fossil calibrations with soft bounds. Mol. Biol. Evol. 23 , 212–226 (2006).
Rannala, B. & Yang, Z. Inferring speciation times under an episodic molecular clock. Syst. Biol. 56 , 453–466 (2007).
Lemey, P., Rambaut, A., Welch, J. J. & Suchard, M. A. Phylogeography takes a relaxed random walk in continuous space and time. Mol. Biol. Evol. 27 , 1877–1885 (2010).
Craig, J. M., Kumar, S. & Hedges, S. B. The origin of eukaryotes and rise in complexity were synchronous with the rise in oxygen. Front. Bioinform. 3 , 1233281 (2023).
Aouad, M. et al. A divide-and-conquer phylogenomic approach based on character supermatrices resolves early steps in the evolution of the Archaea. BMC Ecol. Evol. 22 , 1 (2022).
Coleman, G. A. et al. A rooted phylogeny resolves early bacterial evolution. Science 372 , eabe0511 (2021).
Guy, L. & Ettema, T. J. G. The archaeal ‘TACK’ superphylum and the origin of eukaryotes. Trends Microbiol. 19 , 580–587 (2011).
Spang, A. et al. Complex Archaea that bridge the gap between prokaryotes and eukaryotes. Nature 521 , 173–179 (2015).
Zaremba-Niedzwiedzka, K. et al. Asgard Archaea illuminate the origin of eukaryotic cellular complexity. Nature 541 , 353–358 (2017).
Eme, L. et al. Inference and reconstruction of the heimdallarchaeial ancestry of eukaryotes. Nature 618 , 992–999 (2023).
Raymann, K., Brochier-Armanet, C. & Gribaldo, S. The two-domain tree of life is linked to a new root for the Archaea. Proc. Natl Acad. Sci. USA 112 , 6670–6675 (2015).
Megrian, D., Taib, N., Jaffe, A. L., Banfield, J. F. & Gribaldo, S. Ancient origin and constrained evolution of the division and cell wall gene cluster in Bacteria. Nat. Microbiol. 7 , 2114–2127 (2022).
Brown, C. T. et al. Unusual biology across a group comprising more than 15% of domain Bacteria. Nature 523 , 208–211 (2015).
Rinke, C. et al. Insights into the phylogeny and coding potential of microbial dark matter. Nature 499 , 431–437 (2013).
Shimodaira, H. An approximately unbiased test of phylogenetic tree selection. Syst. Biol. 51 , 492–508 (2002).
Taib, N. et al. Genome-wide analysis of the Firmicutes illuminates the diderm/monoderm transition. Nat. Ecol. Evol. 4 , 1661–1672 (2020).
Szöllõsi, G. J., Rosikiewicz, W., Boussau, B., Tannier, E. & Daubin, V. Efficient exploration of the space of reconciled gene trees. Syst. Biol. 62 , 901–912 (2013).
Kanehisa, M. & Goto, S. KEGG: kyoto encyclopedia of genes and genomes. Nucleic Acids Res. 28 , 27–30 (2000).
Williams, T. A. et al. Integrative modeling of gene and genome evolution roots the archaeal tree of life. Proc. Natl Acad. Sci. USA 114 , E4602–E4611 (2017).
Dharamshi, J. E. et al. Gene gain facilitated endosymbiotic evolution of Chlamydiae. Nat. Microbiol. 8 , 40–54 (2023).
Doolittle, W. F. Phylogenetic classification and the universal tree. Science 284 , 2124–2128 (1999).
Dagan, T. & Martin, W. The tree of one percent. Genome Biol. 7 , 118 (2006).
Tatusov, R. L. et al. The COG database: an updated version includes eukaryotes. BMC Bioinf. 4 , 41 (2003).
Ragsdale, S. W. & Pierce, E. Acetogenesis and the Wood–Ljungdahl pathway of CO 2 fixation. Biochim. Biophys. Acta 1784 , 1873–1898 (2008).
Schuchmann, K. & Müller, V. Autotrophy at the thermodynamic limit of life: a model for energy conservation in acetogenic bacteria. Nat. Rev. Microbiol. 12 , 809–821 (2014).
Schuchmann, K. & Müller, V. Energetics and application of heterotrophy in acetogenic bacteria. Appl. Environ. Microbiol. 82 , 4056–4069 (2016).
Iwabe, N., Kuma, K., Hasegawa, M., Osawa, S. & Miyata, T. Evolutionary relationship of archaebacteria, eubacteria, and eukaryotes inferred from phylogenetic trees of duplicated genes. Proc. Natl Acad. Sci. USA 86 , 9355–9359 (1989).
Gogarten, J. P. et al. Evolution of the vacuolar H + -ATPase: implications for the origin of eukaryotes. Proc. Natl Acad. Sci. USA 86 , 6661–6665 (1989).
Koonin, E. V. & Makarova, K. S. Origins and evolution of CRISPR–Cas systems. Phil. Trans. R. Soc. Lond. B Biol. Sci. 374 , 20180087 (2019).
Krupovic, M., Dolja, V. V. & Koonin, E. V. The LUCA and its complex virome. Nat. Rev. Microbiol. 18 , 661–670 (2020).
Koonin, E. V., Dolja, V. V. & Krupovic, M. The logic of virus evolution. Cell Host Microbe 30 , 917–929 (2022).
Lever, M. A. Acetogenesis in the energy-starved deep biosphere—a paradox? Front. Microbiol. 2 , 284 (2011).
PubMed Google Scholar
Martin, W. & Russell, M. J. On the origin of biochemistry at an alkaline hydrothermal vent. Phil. Trans. R. Soc. Lond. B Biol. Sci. 362 , 1887–1925 (2007).
Catchpole, R. J. & Forterre, P. The evolution of reverse gyrase suggests a nonhyperthermophilic last universal common ancestor. Mol. Biol. Evol. 36 , 2737–2747 (2019).
Groussin, M., Boussau, B., Charles, S., Blanquart, S. & Gouy, M. The molecular signal for the adaptation to cold temperature during early life on Earth. Biol. Lett. 9 , 20130608 (2013).
Boussau, B., Blanquart, S., Necsulea, A., Lartillot, N. & Gouy, M. Parallel adaptations to high temperatures in the Archaean eon. Nature 456 , 942–945 (2008).
Chandor, A. et al. Dinucleotide spore photoproduct, a minimal substrate of the DNA repair spore photoproduct lyase enzyme from Bacillus subtilis. J. Biol. Chem. 281 , 26922–26931 (2006).
Chandra, T. et al. Spore photoproduct lyase catalyzes specific repair of the 5R but not the 5S spore photoproduct. J. Am. Chem. Soc. 131 , 2420–2421 (2009).
Kasting, J. F. The evolution of the prebiotic atmosphere. Orig. Life 14 , 75–82 (1984).
Kharecha, P. A. A Coupled Atmosphere–Ecosystem Model of the Early Archean Biosphere . PhD thesis, Pennsylvania State Univ. (2005).
Barth, P. et al. Isotopic constraints on lightning as a source of fixed nitrogen in Earth’s early biosphere. Nat. Geosci. 16 , 478–484 (2023).
Tian, F., Kasting, J. F. & Zahnle, K. Revisiting HCN formation in Earth’s early atmosphere. Earth Planet. Sci. Lett. 308 , 417–423 (2011).
Zahnle, K. J. Photochemistry of methane and the formation of hydrocyanic acid (HCN) in the Earth’s early atmosphere. J. Geophys. Res. 91 , 2819–2834 (1986).
Stüeken, E. E., Boocock, T., Szilas, K., Mikhail, S. & Gardiner, N. J. Reconstructing nitrogen sources to Earth’s earliest biosphere at 3.7 Ga. Front. Earth Sci. 9 , 675726 (2021).
Ciccarelli, F. D. et al. Toward automatic reconstruction of a highly resolved tree of life. Science 311 , 1283–1287 (2006).
Yutin, N., Makarova, K. S., Mekhedov, S. L., Wolf, Y. I. & Koonin, E. V. The deep archaeal roots of eukaryotes. Mol. Biol. Evol. 25 , 1619–1630 (2008).
Petitjean, C., Deschamps, P., López-García, P. & Moreira, D. Rooting the domain Archaea by phylogenomic analysis supports the foundation of the new kingdom Proteoarchaeota. Genome Biol. Evol. 7 , 191–204 (2014).
Williams, T. A., Cox, C. J., Foster, P. G., Szöllősi, G. J. & Embley, T. M. Phylogenomics provides robust support for a two-domains tree of life. Nat. Ecol. Evol. 4 , 138–147 (2020).
Rinke, C. et al. A standardized archaeal taxonomy for the Genome Taxonomy Database. Nat. Microbiol. 6 , 946–959 (2021).
Parks, D. H. et al. Selection of representative genomes for 24,706 bacterial and archaeal species clusters provide a complete genome-based taxonomy. Preprint at bioRxiv https://doi.org/10.1101/771964 (2019).
Finn, R. D., Clements, J. & Eddy, S. R. HMMER web server: interactive sequence similarity searching. Nucleic Acids Res. 39 , W29–W37 (2011).
Makarova, K. S., Wolf, Y. I. & Koonin, E. V. Archaeal Clusters of Orthologous Genes (arCOGs): an update and application for analysis of shared features between Thermococcales, Methanococcales, and Methanobacteriales. Life 5 , 818–840 (2015).
Haft, D. H., Selengut, J. D. & White, O. The TIGRFAMs database of protein families. Nucleic Acids Res. 31 , 371–373 (2003).
Katoh, K., Kuma, K.-I., Toh, H. & Miyata, T. MAFFT version 5: improvement in accuracy of multiple sequence alignment. Nucleic Acids Res. 33 , 511–518 (2005).
Katoh, K., Misawa, K., Kuma, K.-I. & Miyata, T. MAFFT: a novel method for rapid multiple sequence alignment based on fast Fourier transform. Nucleic Acids Res. 30 , 3059–3066 (2002).
Criscuolo, A. & Gribaldo, S. BMGE (Block Mapping and Gathering with Entropy): a new software for selection of phylogenetic informative regions from multiple sequence alignments. BMC Evol. Biol. 10 , 210 (2010).
Minh, B. Q. et al. IQ-TREE 2: new models and efficient methods for phylogenetic inference in the genomic era. Mol. Biol. Evol. 37 , 1530–1534 (2020).
Eddy, S. R. Accelerated profile HMM searches. PLoS Comput. Biol. 7 , e1002195 (2011).
Susko, E. & Roger, A. J. On reduced amino acid alphabets for phylogenetic inference. Mol. Biol. Evol. 24 , 2139–2150 (2007).
Cantalapiedra, C. P., Hernández-Plaza, A., Letunic, I., Bork, P. & Huerta-Cepas, J. eggNOG-mapper v2: functional annotation, orthology assignments, and domain prediction at the metagenomic scale. Mol. Biol. Evol. 38 , 5825–5829 (2021).
Buchfink, B., Reuter, K. & Drost, H.-G. Sensitive protein alignments at tree-of-life scale using DIAMOND. Nat. Methods 18 , 366–368 (2021).
Szöllősi, G. J., Davín, A. A., Tannier, E., Daubin, V. & Boussau, B. Genome-scale phylogenetic analysis finds extensive gene transfer among fungi. Phil. Trans. R. Soc. Lond. B Biol. Sci. 370 , 20140335 (2015).
Eren, A. M. et al. Community-led, integrated, reproducible multi-omics with anvi’o. Nat. Microbiol. 6 , 3–6 (2021).
Yang, Z. PAML 4: phylogenetic analysis by maximum likelihood. Mol. Biol. Evol. 24 , 1586–1591 (2007).
Wang, S. & Luo, H. Dating the bacterial tree of life based on ancient symbiosis. Preprint at bioRxiv https://doi.org/10.1101/2023.06.18.545440 (2023).
dos Reis, M. & Yang, Z. Approximate likelihood calculation on a phylogeny for Bayesian estimation of divergence times. Mol. Biol. Evol. 28 , 2161–2172 (2011).
Harris et al. Array programming with NumPy. Nature 585 , 357–362 (2020).
Altschul, S. F., Gish, W., Miller, W., Myers, E. W. & Lipman, D. J. Basic local alignment search tool. J. Mol. Biol. 215 , 403–410 (1990).
Sayers, E. W. et al. Database resources of the National Center for Biotechnology Information. Nucleic Acids Res. 39 , D38–D51 (2011).
Edgar, R. C. MUSCLE: multiple sequence alignment with high accuracy and high throughput. Nucleic Acids Res. 32 , 1792–1797 (2004).
Capella-Gutiérrez, S., Silla-Martínez, J. M. & Gabaldón, T. trimAl: a tool for automated alignment trimming in large-scale phylogenetic analyses. Bioinformatics 25 , 1972–1973 (2009).
Aberer, A. J., Krompaß, D. & Stamatakis, A. RogueNaRok: An efficient and exact algorithm for rogue taxon identification. Exelixis-RRDR-2011–10 (Heidelberg Institute for Theoretical Studies, 2011).
Tria, F. D. K., Landan, G. & Dagan, T. Phylogenetic rooting using minimal ancestor deviation. Nat. Ecol. Evol. 1 , 193 (2017).
Hoang, D. T., Chernomor, O., von Haeseler, A., Minh, B. Q. & Vinh, L. S. UFBoot2: improving the ultrafast bootstrap approximation. Mol. Biol. Evol. 35 , 518–522 (2018).
Burki, F., Roger, A. J., Brown, M. W. & Simpson, A. G. B. The new tree of eukaryotes. Trends Ecol. Evol. 35 , 43–55 (2020).
Battistuzzi, F. U., Billing-Ross, P., Paliwal, A. & Kumar, S. Fast and slow implementations of relaxed-clock methods show similar patterns of accuracy in estimating divergence times. Mol. Biol. Evol. 28 , 2439–2442 (2011).
Moody, E. R. R. The nature of the last universal common ancestor and its impact on the early Earth system. figshare https://doi.org/10.6084/m9.figshare.24428659 (2024).
Álvarez-Carretero, S. The nature of the last universal common ancestor and its impact on the early Earth system—timetree inference analyses. Zenodo https://doi.org/10.5281/zenodo.11260523 (2024).
Moody, E. R. R. et al. The nature of the Last Universal Common Ancestor and its impact on the early Earth system. Nat. Ecol. Evol. https://doi.org/10.5523/bris.405xnm7ei36d2cj65nrirg3ip (2024).
Darzi, Y., Letunic, I., Bork, P. & Yamada, T. iPath3.0: interactive pathways explorer v3. Nucleic Acids Res. 46 , W510–W513 (2018).
Download references
Acknowledgements
Our research is funded by the John Templeton Foundation (62220 to P.C.J.D., N.L., T.M.L., D.P., G.A.S., T.A.W. and Z.Y.; the opinions expressed in this publication are those of the authors and do not necessarily reflect the views of the John Templeton Foundation), Biotechnology and Biological Sciences Research Council (BB/T012773/1 to P.C.J.D. and Z.Y.; BB/T012951/1 to Z.Y.), by the European Research Council under the European Union’s Horizon 2020 research and innovation programme (947317 ASymbEL to A.S.; 714774, GENECLOCKS to G.J.S.), Leverhulme Trust (RF-2022-167 to P.C.J.D.), Gordon and Betty Moore Foundation (GBMF9741 to T.A.W., D.P., P.C.J.D., A.S. and G.J.S.; GBMF9346 to A.S.), Royal Society (University Research Fellowship (URF) to T.A.W.), the Simons Foundation (735929LPI to A.S.) and the University of Bristol (University Research Fellowship (URF) to D.P.).
Author information
Authors and affiliations.
Bristol Palaeobiology Group, School of Earth Sciences, University of Bristol, Bristol, UK
Edmund R. R. Moody, Sandra Álvarez-Carretero, Holly C. Betts, Davide Pisani & Philip C. J. Donoghue
Department of Marine Microbiology and Biogeochemistry, NIOZ, Royal Netherlands Institute for Sea Research, Den Burg, The Netherlands
Tara A. Mahendrarajah, Nina Dombrowski & Anja Spang
Milner Centre for Evolution, Department of Life Sciences, University of Bath, Bath, UK
James W. Clark
Department of Biological Physics, Eötvös University, Budapest, Hungary
Lénárd L. Szánthó
MTA-ELTE ‘Lendulet’ Evolutionary Genomics Research Group, Budapest, Hungary
Lénárd L. Szánthó & Gergely J. Szöllősi
Institute of Evolution, HUN-REN Center for Ecological Research, Budapest, Hungary
Global Systems Institute, University of Exeter, Exeter, UK
Richard A. Boyle, Stuart Daines & Timothy M. Lenton
Department of Earth Sciences, University College London, London, UK
Xi Chen & Graham A. Shields
Department of Genetics, Evolution and Environment, University College London, London, UK
Nick Lane & Ziheng Yang
Model-Based Evolutionary Genomics Unit, Okinawa Institute of Science and Technology Graduate University, Okinawa, Japan
Gergely J. Szöllősi
Department of Evolutionary & Population Biology, Institute for Biodiversity and Ecosystem Dynamics (IBED), University of Amsterdam, Amsterdam, The Netherlands
Bristol Palaeobiology Group, School of Biological Sciences, University of Bristol, Bristol, UK
Davide Pisani & Tom A. Williams
You can also search for this author in PubMed Google Scholar
Contributions
The project was conceived and designed by P.C.J.D., T.M.L., D.P., G.J.S., A.S. and T.A.W. Dating analyses were performed by H.C.B., J.W.C., S.Á.-C., P.J.C.D. and E.R.R.M. T.A.M., N.D. and E.R.R.M. performed single-copy orthologue analysis for species-tree inference. L.L.S., G.J.S., T.A.W. and E.R.R.M. performed reconciliation analysis. E.R.R.M. performed homologous gene family annotation, sequence, alignment, gene tree inference and sensitivity tests. E.R.R.M., A.S. and T.A.W. performed metabolic analysis and interpretation. T.M.L., S.D. and R.A.B. provided biogeochemical interpretation. E.R.R.M., T.M.L., A.S., T.A.W., D.P. and P.J.C.D. drafted the article to which all authors (including X.C., N.L., Z.Y. and G.A.S.) contributed.
Corresponding authors
Correspondence to Edmund R. R. Moody , Davide Pisani , Tom A. Williams , Timothy M. Lenton or Philip C. J. Donoghue .
Ethics declarations
Competing interests.
The authors declare no competing interests.
Peer review
Peer review information.
Nature Ecology & Evolution thanks Aaron Goldman and the other, anonymous, reviewer(s) for their contribution to the peer review of this work. Peer reviewer reports are available.
Additional information
Publisher’s note Springer Nature remains neutral with regard to jurisdictional claims in published maps and institutional affiliations.
Extended data
Extended data fig. 1 comparison of the mean divergence times and confidence intervals estimated for the two duplicates of luca under each timetree inference analysis..
Black dots refer to estimated mean divergence times for analyses without cross-bracing, stars are used to identify those under cross-bracing and triangles for estimated upper and lower confidence intervals. Straight lines are used to link mean divergence time estimates across the various inference analyses we carried out, while dashed lines are used to link the estimated confidence intervals. The node label for the driver node is “248”, while it is “368” for the mirror node, as shown in the title of each graph. Coloured stars and triangles are used to identify which LUCA time estimates were inferred under the same cross-braced analysis for the driver-mirror nodes (that is, equal time and CI estimates). Black dots and triangles are used to identify those inferred when cross-bracing was not enabled (that is, different time and CI estimates). -Abbreviations. “GBM”: Geometric Brownian motion relaxed-clock model; “ILN”: Independent-rate log-normal relaxed-clock model; “conc, cb” dots/triangles: results under cross-bracing A when the concatenated dataset was analysed under GBM (red) and ILN (blue); “conc, fosscb”: results under cross-bracing B when the concatenated dataset was analysed under GBM (orange) and ILN (cyan); “part, cb” dots/triangles: results under cross-bracing A when the partitioned dataset was analysed under GBM (pink) and ILN (purple); “part, fosscb”: results under cross-bracing B when the concatenated dataset was analysed under GBM (light green) and ILN (grey); black dots and triangles: results when cross-bracing was not enabled for both concatenated and partitioned datasets.
Extended Data Fig. 2 Comparison of the posterior time estimates and confidence intervals for the two duplicates of LUCA inferred under the main calibration strategy cross-bracing A with the concatenated dataset and with the datasets for the three additional sensitivity analyses.
Dots refer to estimated mean divergence times and triangles to estimated 2.5% and 97.5% quantiles. Straight lines are used to link the mean divergence times estimated in the same analysis under the two different relaxed-clock models (GBM and ILN). Labels in the x axis are informative about the clock model under which the analysis ran and the type of analysis we carried (see abbreviations below). Coloured dots are used to identify which time estimates were inferred when using the same dataset and strategy under GBM and ILN, while triangles refer to the corresponding upper and lower quantiles for the 95% confidence interval. -Abbreviations. “GBM”: Geometric Brownian motion relaxed-clock model; “ILN”: Independent-rate log-normal relaxed-clock model; “main-conc”: results obtained with the concatenated dataset analysed in our main analyses under cross-bracing A; “ATP/EF/Leu/SRP/Tyr”: results obtained when using each gene alignment separately; “noATP/noEF/noLeu/noSRP/noTyr”: results obtained when using concatenated alignments without the gene alignment mentioned in the label as per the “leave-one-out” strategy; “main-bsinbv”: results obtained with the concatenated dataset analysed in our main analyses when using branch lengths, Hessian, and gradient calculated under a more complex substitution model to infer divergence times.
Extended Data Fig. 3 Maximum Likelihood species tree.
The Maximum Likelihood tree inferred across three independent runs, under the best fitting model (according to BIC: LG + F + G + C60) from a concatenation of 57 orthologous proteins, support values are from 10,000 ultrafast bootstraps. Referred to as topology I in the main text. Tips coloured according to taxonomy: Euryarchaeota (teal), DPANN (purple), Asgardarchaeota (cyan), TACK (blue), Gracilicutes (orange), Terrabacteria (red), DST (brown), CPR (green).
Extended Data Fig. 4 Maximum Likelihood tree for focal reconciliation analysis.
Maximum Likelihood tree (topology II in the main text), where DPANN is constrained to be sister to all other Archaea, and CPR is sister to Chloroflexi. Tips coloured according to taxonomy: Euryarchaeota (teal), DPANN (purple), Asgardarchaeota (cyan), TACK (blue), Gracilicutes (orange), Terrabacteria (red), DST (brown), CPR (green). AU topology test, P = 0.517, this is a one-sided statistical test.
Extended Data Fig. 5 The relationship between the number of KO gene families encoded on a genome and its size.
LOESS regression of the number of KOs per sampled genome against the genome size in megabases. We used the inferred relationship for modern prokaryotes to estimate LUCA’s genome size based on reconstructed KO gene family content, as described in the main text. Shaded area represents the 95% confidence interval.
Extended Data Fig. 6 The relationship between the number of KO gene families encoded on a genome and the total number of protein-coding genes.
LOESS regression of the number of KOs per sampled genome against the number of proteins encoded for per sampled genome. We used the inferred relationship for modern prokaryotes to estimate the total number of protein-coding genes encoded by LUCA based on reconstructed KO gene family content, as described in the main text. Shaded area represents the 95% confidence interval.
Supplementary information
Supplementary information.
Supplementary Notes and Figs. 1–10.
Reporting Summary
Peer review file, supplementary data 1.
This table contains the results of the reconciliations for each gene family. KEGG_ko is the KEGG orthology ID; arc_domain_prop is the proportion of the sampled Archaea; bac_domain_prop is the proportion of the sampled bacteria; gene refers to gene name, description and enzyme code; map refers to the different KEGG maps of which this KEGG gene family is a component; pathway is a text description of the metabolic pathways of which these genes are a component; alignment_length refers to the length of the alignment in amino acids; highest_COG_cat refers to the number of sequences placed in the most frequent COG category; difference_1st_and_2nd is the difference between the most frequent COG category and the second most frequent COG category; categories is the number of different COG categories assigned to this KEGG gene family; COG_freq is the proportion of the sequences placed in the most frequent COG category; COG_cat is the most frequent COG functional category; Archaea is the number of archaeal sequences sampled in the gene family; Bacteria is the number of bacterial sequences sampled in the gene family; alternative_COGs is the number of alternative COG gene families assigned across this KEGG orthologous gene family; COG_perc is the proportion of the most frequent COG ID assigned to this KEGG gene family; COG is the COG ID of the most frequenty COG assigned to this gene family; COG_NAME is the description of the most frequent COG ID assigned to this gene family; COG_TAG is the symbol associated with the most frequent COG gene familiy; sequences is the total number of sequences assigned to this gene family; Arc_prop is the proportion of Archaea that make up this gene family; Bac_prop is the proportion of Bacteria that make up this gene family; constrained_median is the median probability (PP) that this gene was present in LUCA from our reconciliation under the focal constrained tree search across the 5 independent bootstrap distribution reconciliations; ML_median is the median PP of the gene family being present in LUCA with gene tree bootstrap distributions against the ML species-tree topology across the 15 independent bootstrap distribution reconciliations; MEAN_OF_MEDIANS is the mean value across the constrained and ML PP results; RANGE_OF_MEDIANS is the range of the PPs for the constrained and ML topology PPs for LUCA; Probable_and_sampling_threshold_met is our most stringent category of gene families inferred in LUCA with 0.75 + PP and a sampling requirement of 1% met in both Archaea and Bacteria; Possible_and_sampling_threshold_met is a threshold of 0.50 + PP and sampling both domains; probable is simply 0.75 + PP; and possible is 0.50 + PP.
Supplementary Data 2
PP for COGs. This table contains the results for the reconciliations of COG-based gene family clustering against the constrained focal species-tree topology. Columns are named similarly to Supplementary Data 1 but each row is a different COG family. The column Modal_KEGG_ko refers to the most frequent KEGG gene family in which a given COG is found; sequences_in_modal_KEGG refers to the number of sequences in the most frequent KEGG gene family.
Supplementary Data 3
Module completeness. Estimated pathway completeness for KEGG metabolic modules (with a completeness greater than zero in at least one confidence threshold) using Anvi’o’s stepwise pathway completeness 48 . Module_name is the name of the module; module_category is the broader category into which the module falls; module_subcategory is a more specific category; possible_anvio includes the gene families with a median PP ≥ 0.50; probable_anvio related to gene families PP ≥ 0.75; and _ws refers to the sampling requirement being met (presence in at least 1% of the sampled Archaea and Bacteria).
Supplementary Data 4
Marker gene metadata for all markers checked during marker gene curation, including the initial 59 single-copy marker genes used in species-tree inference (see Methods ). Data include marker gene set provenance, marker gene name, marker gene description, presence in different marker gene sets 49 , 50 , 51 , 52 , 53 , 54 , 55 , 56 , 57 , 58 , and presence in Archaea and Bacteria. When available, marker genes are matched with their arCOG, TIGR, and COG ID and their respective occurrence across different taxonomic sets is quantified.
Supplementary Data 5
The ratio of duplications, transfers and losses in relation to the total number of copies for the deep ancestral nodes: the LUCA, archaeal (LACA) and bacterial (LBCA) common ancestors, and the average (mean) and 95th percentile.
Supplementary Data 6
Spreadsheet containing a list of the estimated divergence times for all timetree inferences carried out and the corresponding results of the MCMC diagnostics. Tabs Divtimes_GBM-allnodes and Divtimes_ILN-allnodes represent a list of the estimated divergence times (Ma) for all nodes under the 12 inference analyses we ran under GBM and ILN, respectively. Tabs Divtimes_GBM-highlighted and Divtimes_ILN-highlighted represent a list of the estimated divergence times (Ma) for selected nodes ordered according to their mirrored nodes under the 12 inference analyses we ran under GBM and ILN, respectively. Each of the tabs MCMCdiagn_prior, MCMCdiagn_postGBM and MCMCdiagn_postILN contains the statistical results of the MCMC diagnostics we ran for each inference analysis. Note that, despite the analyses carried out when sampling from the prior could have only been done three times (that is, data are not used, and thus only once under each calibration strategy was enough), we repeated them with each dataset regardless. In other words, results for (1) ‘concatenated + cross-bracing A’ and ‘partitioned + cross-bracing A’; (2) ‘concatenated + without cross-bracing’ and ‘partitioned + without cross-bracing’; and (3) ‘concatenated + cross-bracing B’ and ‘partitioned + cross-bracing B’ would be equivalent, respectively. For tabs 1–4, part represents partitioned dataset; conc, concatenated dataset; cb, cross-bracing A; notcb, without cross-bracing; fosscb, cross-bracing B; mean_t, mean posterior time estimate; 2.5%q, 2.5% quantile of the posterior time density for a given node; and 97.5%q, 97.5% quantile of the posterior time density for a given node. For tabs 5–7, med. num. samples collected per chain represents median of the total amount of samples collected per chain; min. num. samples collected per chain, minimum number of samples collected per chain; max. num. samples collected per chain, minimum number of samples collected per chain; num. samples used to calculate stats, number of samples collected by all chains that passed the filters that were used to calculate the tail-ESS, bulk-ESS and R-hat values. For tail-ESS, we report the median, minimum, and maximum tail-ESS values; all larger than 100 as required for assuming reliable parameter estimates. For bulk-ESS, we report the median, minimum and maximum bulk-ESS values; all larger than 100 as required for assuming reliable parameter estimates. For R-hat, minimum and maximum values reported, all smaller than 1.05 as required to assume good mixing.
Supplementary Data 7
Spreadsheet containing a list of the posterior time estimates for LUCA obtained under the main calibration strategy cross-bracing A with the concatenated dataset and with the datasets for the three additional sensitivity analyses. The first column ‘label’ contains the node number for both the driver and mirror nodes for LUCA (the latter includes the term -dup in the label). Columns mean_t, 2.5%q, and 97.5%q refer to the estimated mean divergence times, and the 2.5%/97.5% quantiles of the posterior time density for the corresponding node. Main-conc, refers to results obtained with the concatenated dataset analysed in our main analyses under cross-bracing A; ATP/EF/Leu/SRP/Tyr, results obtained when using each gene alignment separately; noATP/noEF/noLeu/noSRP/noTyr, results obtained when using concatenated alignments without the gene alignment mentioned in the label as per the leave-one-out strategy; main-bsinbv, results obtained with the concatenated dataset analysed in our main analyses when using branch lengths, Hessian and gradient calculated under a more complex substitution model to infer divergence times.
Supplementary Data 8
Spreadsheet containing a list of the estimated divergence times for all timetree inferences carried out for the sensitivity analyses and the corresponding results for the MCMC diagnostics. Tabs Divtimes_GBM-allnodes and Divtimes_ILN-allnodes represent a list of the estimated divergence times (Ma) for all nodes under the 11 inference analyses we ran under GBM and ILN when testing the impact on divergence times estimation when (1) analysing each gene alignment individually, (2) following a leave-one-out strategy, and (3) using the branch lengths, Hessian and gradient estimated under a more complex model for timetree inference (bsinBV approach). Tabs Divtimes_GBM-highlighted and Divtimes_ILN-highlighted represent a list of the estimated divergence times (Ma) for selected nodes ordered according to their mirrored nodes we ran under GBM and ILN for the sensitivity analyses (we also included the results with the main concatenated dataset for reference). Each of tabs MCMCdiagn_prior, MCMCdiagn_postGBM and MCMCdiagn_postILN contains the statistical results of the MCMC diagnostics we ran for the sensitivity analyses. Note that, despite the analyses carried out when sampling from the prior could have only been done once for each different tree topology (that is, data are not used, only topological changes may affect the resulting marginal densities), we ran them with each dataset regardless as part of our pipeline. For tabs 1–4, main-conc represents results obtained with the concatenated dataset analysed in our main analyses under cross-bracing A; ATP/EF/Leu/SRP/Tyr, results obtained when using each gene alignment separately; noATP/noEF/noLeu/noSRP/noTyr, results obtained when using concatenated alignments without the gene alignment mentioned in the label as per the leave-one-out strategy; main-bsinbv, results obtained with the concatenated dataset analysed in our main analyses when using branch lengths, Hessian and gradient calculated under a more complex substitution model to infer divergence times; mean_t, mean posterior time estimate; 2.5%q, 2.5% quantile of the posterior time density for a given node; and 97.5%q, 97.5% quantile of the posterior time density for a given node. For tabs 5–7, med. num. samples collected per chain represents the median of the total amount of samples collected per chain; min. num. samples collected per chain, minimum number of samples collected per chain; max. num. samples collected per chain, minimum number of samples collected per chain; num. samples used to calculate stats, number of samples collected by all chains that passed the filters that were used to calculate the tail-ESS, bulk-ESS and R-hat values. For tail-ESS, we report the median, minimum and maximum tail-ESS values; all larger than 100 as required for assuming reliable parameter estimates. For bulk-ESS, we report the median, minimum and maximum bulk-ESS values; all larger than 100 as required for assuming reliable parameter estimates. For R-hat, minimum and maximum values are reported, all smaller than 1.05 as required to assume good mixing.
Rights and permissions
Open Access This article is licensed under a Creative Commons Attribution 4.0 International License, which permits use, sharing, adaptation, distribution and reproduction in any medium or format, as long as you give appropriate credit to the original author(s) and the source, provide a link to the Creative Commons licence, and indicate if changes were made. The images or other third party material in this article are included in the article’s Creative Commons licence, unless indicated otherwise in a credit line to the material. If material is not included in the article’s Creative Commons licence and your intended use is not permitted by statutory regulation or exceeds the permitted use, you will need to obtain permission directly from the copyright holder. To view a copy of this licence, visit http://creativecommons.org/licenses/by/4.0/ .
Reprints and permissions
About this article
Cite this article.
Moody, E.R.R., Álvarez-Carretero, S., Mahendrarajah, T.A. et al. The nature of the last universal common ancestor and its impact on the early Earth system. Nat Ecol Evol (2024). https://doi.org/10.1038/s41559-024-02461-1
Download citation
Received : 19 January 2024
Accepted : 04 June 2024
Published : 12 July 2024
DOI : https://doi.org/10.1038/s41559-024-02461-1
Share this article
Anyone you share the following link with will be able to read this content:
Sorry, a shareable link is not currently available for this article.
Provided by the Springer Nature SharedIt content-sharing initiative
Quick links
- Explore articles by subject
- Guide to authors
- Editorial policies
Sign up for the Nature Briefing: Microbiology newsletter — what matters in microbiology research, free to your inbox weekly.

- Search for: Toggle Search
Mile-High AI: NVIDIA Research to Present Advancements in Simulation and Gen AI at SIGGRAPH
NVIDIA is taking an array of advancements in rendering, simulation and generative AI to SIGGRAPH 2024 , the premier computer graphics conference, which will take place July 28 – Aug. 1 in Denver.
More than 20 papers from NVIDIA Research introduce innovations advancing synthetic data generators and inverse rendering tools that can help train next-generation models. NVIDIA’s AI research is making simulation better by boosting image quality and unlocking new ways to create 3D representations of real or imagined worlds.
The papers focus on diffusion models for visual generative AI, physics-based simulation and increasingly realistic AI-powered rendering. They include two technical Best Paper Award winners and collaborations with universities across the U.S., Canada, China, Israel and Japan as well as researchers at companies including Adobe and Roblox.
These initiatives will help create tools that developers and businesses can use to generate complex virtual objects, characters and environments. Synthetic data generation can then be harnessed to tell powerful visual stories, aid scientists’ understanding of natural phenomena or assist in simulation-based training of robots and autonomous vehicles.
Diffusion Models Improve Texture Painting, Text-to-Image Generation
Diffusion models, a popular tool for transforming text prompts into images, can help artists, designers and other creators rapidly generate visuals for storyboards or production, reducing the time it takes to bring ideas to life.
Two NVIDIA-authored papers are advancing the capabilities of these generative AI models.
ConsiStory , a collaboration between researchers at NVIDIA and Tel Aviv University, makes it easier to generate multiple images with a consistent main character — an essential capability for storytelling use cases such as illustrating a comic strip or developing a storyboard. The researchers’ approach introduces a technique called subject-driven shared attention, which reduces the time it takes to generate consistent imagery from 13 minutes to around 30 seconds.
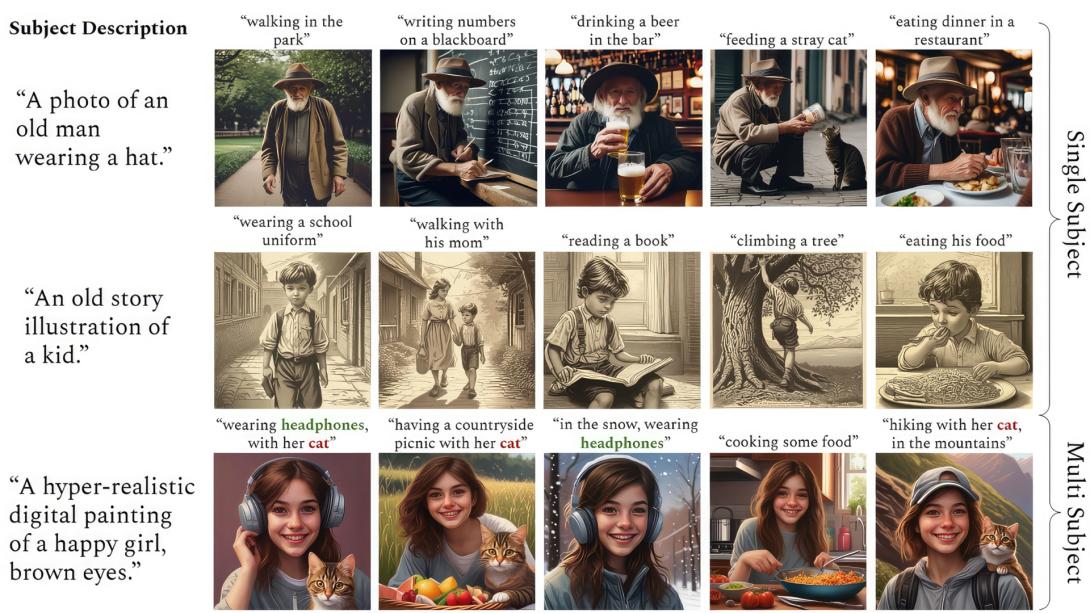
NVIDIA researchers last year won the Best in Show award at SIGGRAPH’s Real-Time Live event for AI models that turn text or image prompts into custom textured materials. This year, they’re presenting a paper that applies 2D generative diffusion models to interactive texture painting on 3D meshes, enabling artists to paint in real time with complex textures based on any reference image.
Kick-Starting Developments in Physics-Based Simulation
Graphics researchers are narrowing the gap between physical objects and their virtual representations with physics-based simulation — a range of techniques to make digital objects and characters move the same way they would in the real world.
Several NVIDIA Research papers feature breakthroughs in the field, including SuperPADL, a project that tackles the challenge of simulating complex human motions based on text prompts (see video at top).
Using a combination of reinforcement learning and supervised learning, the researchers demonstrated how the SuperPADL framework can be trained to reproduce the motion of more than 5,000 skills — and can run in real time on a consumer-grade NVIDIA GPU.
Another NVIDIA paper features a neural physics method that applies AI to learn how objects — whether represented as a 3D mesh, a NeRF or a solid object generated by a text-to-3D model — would behave as they are moved in an environment.
A paper written in collaboration with Carnegie Mellon University researchers develops a new kind of renderer — one that, instead of modeling physical light, can perform thermal analysis, electrostatics and fluid mechanics . Named one of five best papers at SIGGRAPH, the method is easy to parallelize and doesn’t require cumbersome model cleanup, offering new opportunities for speeding up engineering design cycles.
In the example above, the renderer performs a thermal analysis of the Mars Curiosity rover, where keeping temperatures within a specific range is critical to mission success.
Additional simulation papers introduce a more efficient technique for modeling hair strands and a pipeline that accelerates fluid simulation by 10x.
Raising the Bar for Rendering Realism, Diffraction Simulation
Another set of NVIDIA-authored papers present new techniques to model visible light up to 25x faster and simulate diffraction effects — such as those used in radar simulation for training self-driving cars — up to 1,000x faster.
A paper by NVIDIA and University of Waterloo researchers tackles free-space diffraction , an optical phenomenon where light spreads out or bends around the edges of objects. The team’s method can integrate with path-tracing workflows to increase the efficiency of simulating diffraction in complex scenes, offering up to 1,000x acceleration. Beyond rendering visible light, the model could also be used to simulate the longer wavelengths of radar, sound or radio waves.
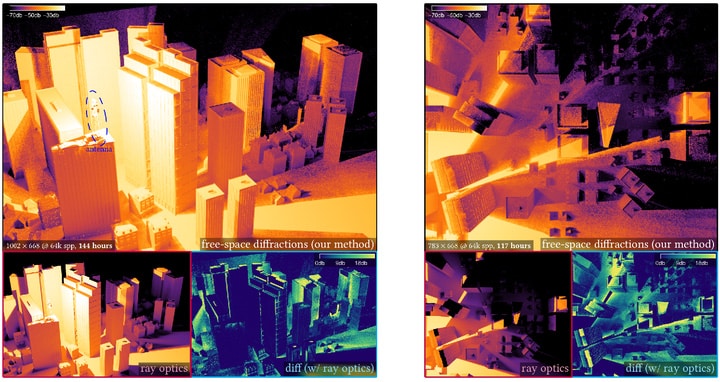
Path tracing samples numerous paths — multi-bounce light rays traveling through a scene — to create a photorealistic picture. Two SIGGRAPH papers improve sampling quality for ReSTIR, a path-tracing algorithm first introduced by NVIDIA and Dartmouth College researchers at SIGGRAPH 2020 that has been key to bringing path tracing to games and other real-time rendering products.
One of these papers, a collaboration with the University of Utah, shares a new way to reuse calculated paths that increases effective sample count by up to 25x , significantly boosting image quality. The other improves sample quality by randomly mutating a subset of the light’s path. This helps denoising algorithms perform better, producing fewer visual artifacts in the final render.
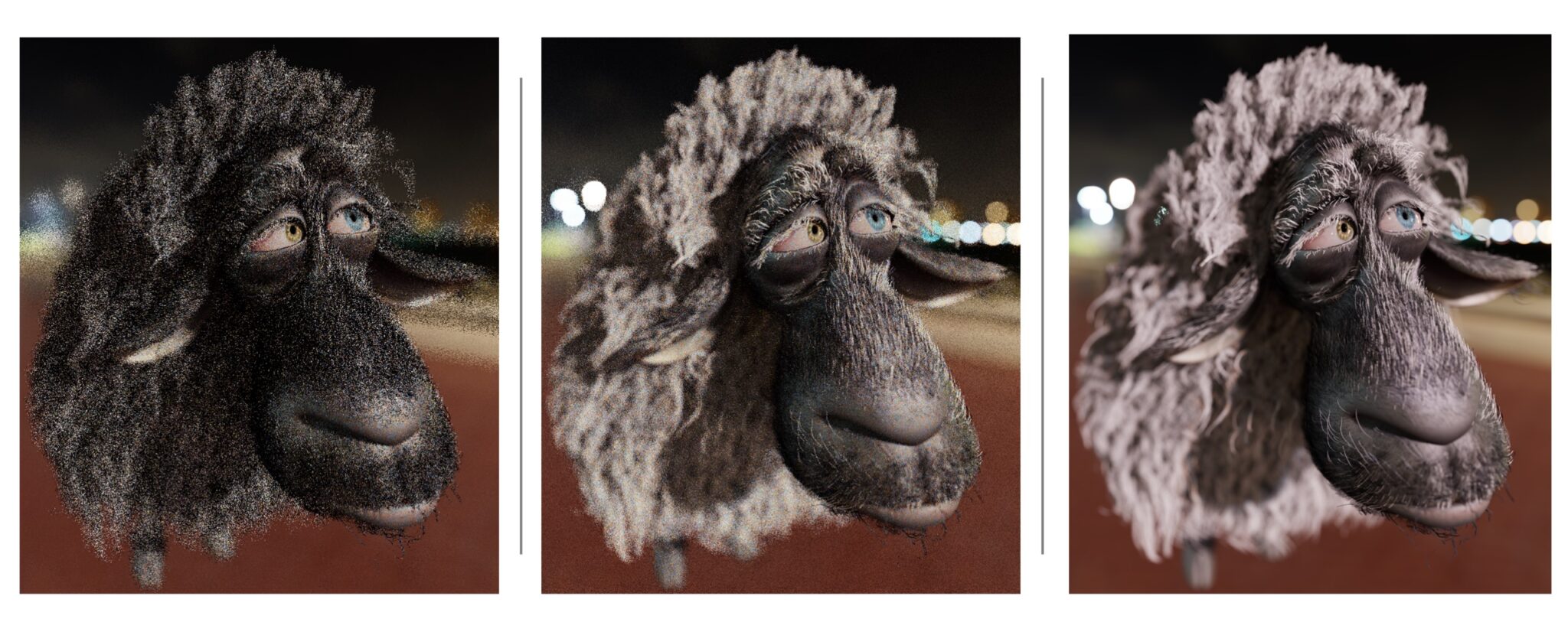
Teaching AI to Think in 3D
NVIDIA researchers are also showcasing multipurpose AI tools for 3D representations and design at SIGGRAPH.
One paper introduces fVDB, a GPU-optimized framework for 3D deep learning that matches the scale of the real world. The fVDB framework provides AI infrastructure for the large spatial scale and high resolution of city-scale 3D models and NeRFs , and segmentation and reconstruction of large-scale point clouds.
A Best Technical Paper award winner written in collaboration with Dartmouth College researchers introduces a theory for representing how 3D objects interact with light . The theory unifies a diverse spectrum of appearances into a single model.
And a collaboration with University of Tokyo, University of Toronto and Adobe Research introduces an algorithm that generates smooth, space-filling curves on 3D meshes in real time. While previous methods took hours, this framework runs in seconds and offers users a high degree of control over the output to enable interactive design.
NVIDIA at SIGGRAPH
Learn more about NVIDIA at SIGGRAPH . Special events include a fireside chat between NVIDIA founder and CEO Jensen Huang and Meta founder and CEO Mark Zuckerberg , as well as a fireside chat with Huang and Lauren Goode , senior writer at WIRED, on the impact of robotics and AI in industrial digitalization.
NVIDIA researchers will also present OpenUSD Day by NVIDIA , a full-day event showcasing how developers and industry leaders are adopting and evolving OpenUSD to build AI-enabled 3D pipelines.
NVIDIA Research has hundreds of scientists and engineers worldwide, with teams focused on topics including AI, computer graphics, computer vision, self-driving cars and robotics. See more of their latest work .
Share on Mastodon
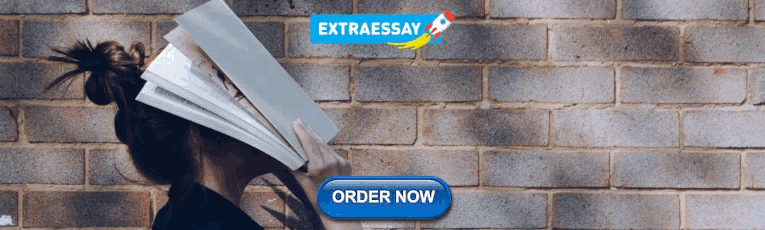
IMAGES
VIDEO
COMMENTS
7.1 Introduction. Regression analysis is one of the most fr equently used tools in market resear ch. In its. simplest form, regression analys is allows market researchers to analyze rela tionships ...
Abstract. Multiple regression is one of the most significant forms of regression and has a wide range. of applications. The study of the implementation of multiple regression analysis in different ...
Linear regression is a statistical procedure for calculating the value of a dependent variable from an independent variable. Linear regression measures the association between two variables. It is ...
Regression analysis is a statistical technique for estimating the relationship among variables which have reason and result relation. Main focus of univariate regression is analyse the relationship between a dependent variable and one independent variable and formulates the linear relation equation between dependent and independent variable.
The aim of linear regression analysis is to estimate the coefficients of the regression equation b 0 and b k (k∈K) so that the sum of the squared residuals (i.e., the sum over all squared differences between the observed values of the i th observation of y i and the corresponding predicted values \( {\hat{y}}_i \)) is minimized.The lower part of Fig. 1 illustrates this approach, which is ...
Regression analysis is an important statistical method that is commonly used to determine the relationship between ... Schober P, Vetter TR. Linear regression in medical research. Anesth Analg ...
These questions can in principle be answered by multiple linear regression analysis. In the multiple linear regression model, Y has normal distribution with mean. The model parameters β 0 + β 1 + +β ρ and σ must be estimated from data. β 0 = intercept. β 1 β ρ = regression coefficients.
Linear regression is used to study the linear relationship between a dependent variable Y (blood pressure) and one or more independent variables X (age, weight, sex). The dependent variable Y must be continuous, while the independent variables may be either continuous (age), binary (sex), or categorical (social status).
A regression analysis is used for one (or more) of three purposes: modeling the relationship between x and y; prediction of the target variable (forecasting); and testing of hypotheses. The chapter introduces the basic multiple linear regression model, and discusses how this model can be used for these three purposes.
SUBMIT PAPER. Advances in Developing Human Resources. Impact Factor: 3.1 / 5-Year Impact ... Testing the principle assumptions of regression analysis is a process. As such, the presentation of this process in a systems framework provides a comprehensive plan with step-by-step guidelines to help determine the optimal statistical model for a ...
The value of t .025 is found in a t-table, using the usual df of t for assessing statistical significance of a regression coefficient (N - the num-ber of X's - 1), and is the value that leaves a tail of the t-curve with 2.5% of the total probability. For instance, if df = 30, then t.025 = 2.042.
Although regression models play a central role in the analysis of medical research projects, there still exist many misconceptions on various aspects of modeling leading to faulty analyses. Indeed, the rapidly developing statistical methodology and its recent advances in regression modeling do not seem to be adequately reflected in many medical publications. This problem of knowledge transfer ...
158 PART II: BAsIc And AdvAnced RegRessIon AnAlysIs 5A.2 Statistical Regression Methods The regression procedures that we cover in this chapter are known as statistical regression methods.The most popular of these statistical methods include the standard, forward, backward, and stepwise meth- ods, although others (not covered here), such as the Mallows Cp method (e.g., Mallows, 1973) and the
Linear regression refers to the mathematical technique of fitting given data to a function of a certain type. It is best known for fitting straight lines. In this paper, we explain the theory behind linear regression and illustrate this technique with a real world data set. This data relates the earnings of a food truck and the population size of the city where the food truck sells its food.
Alan O. Sykes, "An Introduction to Regression Analysis" (Coase-Sandor Institute for Law & Economics Working Paper No. 20, 1993). This Working Paper is brought to you for free and open access by the Coase-Sandor Institute for Law and Economics at Chicago Unbound. It has been accepted for inclusion in Coase-Sandor Working Paper Series in Law and ...
Logistic Regression: Logistic regression is used when the dependent variable is binary or categorical. The logistic regression model applies a logistic or sigmoid function to the linear combination of the independent variables. Logistic Regression Model: p = 1 / (1 + e^- (β0 + β1X1 + β2X2 + … + βnXn)) In the formula: p represents the ...
Abstract. Although regression models play a central role in the analysis of medical research projects, there still exist many misconceptions on various aspects of modeling leading to faulty analyses. Indeed, the rapidly developing statistical methodology and its recent advances in regression modeling do not seem to be adequately reflected in ...
Home environment and reading achievement research has been largely dominated by a focus on early reading acquisition, while research on the relationship between home environments and reading success with preadolescents (Grades 4-6) has been largely overlooked. There are other limitations as well. Clarke and Kurtz-Costes (1997) argued that prior ...
The research methodology is based on statistical analysis, which in this paper includes the multiple regression analysis. This type of analysis is used for modeling and analyzing several variables. The multiple regression analysis extends regression analysis Titan et al., by describing the relationship between a dependent
Regression analysis is a statistical method to analyze financial data, commonly using the least square regression technique. The regression analysis has significance for all the fields of study, and almost all the fields apply least square regression methods for data analysis. However, the ordinary least square regression technique can give misleading and wrong results in the presence of ...
Abstract: In many different sciences, including medicine, engineering, and observational studies, the investigation of the relationship between variables, i.e., dependents, and independents, is defined as research objectives. Employing statistical methods to achieve the relationship between variables is very time-consuming or costly in many scenarios and does not provide practical application.
Principal component analysis is used in the ordinary least squares (OLS) regression model to demonstrate its robustness and reliability in predicting cotton crop yield. The model successfully captures the intrinsic patterns in the information and produces accurate predictions that demonstrate robust predictive capabilities when applied to new data.
Today, we are releasing the team's first paper, which introduces a new research direction for empirically aligning superhuman models. Current alignment methods, such as reinforcement learning from human feedback (RLHF), rely on human supervision. However, future AI systems will be capable of extremely complex and creative behaviors that will ...
According to Ali and Younas (2021), researchers use regression analysis in order to describe or present the relationship between one or more independent variables and a dependent variable ...
About the research. The online survey was in the field from February 22 to March 5, 2024, and garnered responses from 1,363 participants representing the full range of regions, industries, company sizes, functional specialties, and tenures. Of those respondents, 981 said their organizations had adopted AI in at least one business function, and ...
This paper presents a new set of foundation models, called Llama 3. It is a herd of language models that natively support multilinguality, coding, reasoning, and tool usage. Our largest model is a dense Transformer with 405B parameters and a context window of up to 128K tokens. This paper presents an extensive empirical evaluation of Llama 3.
The regression analysis was performed by adding the state ownership and its interaction term in model 4. The regression results show that the interaction term has a significant positive relationship with corporate R&D investment. ... This paper conducts the in-depth research on the essential process of executive compensation contract affecting ...
Here we infer that LUCA lived ~4.2 Ga (4.09-4.33 Ga) through divergence time analysis of pre-LUCA gene duplicates, calibrated using microbial fossils and isotope records under a new cross ...
MLR, an extension of linear regression, is a statistical approach employed in data analysis and modeling to investigate the correlation between a dependent variable and two or more independent ...
NVIDIA is taking an array of advancements in rendering, simulation and generative AI to SIGGRAPH 2024, the premier computer graphics conference, which will take place July 28 - Aug. 1 in Denver.. More than 20 papers from NVIDIA Research introduce innovations advancing synthetic data generators and inverse rendering tools that can help train next-generation models.