Academia.edu no longer supports Internet Explorer.
To browse Academia.edu and the wider internet faster and more securely, please take a few seconds to upgrade your browser .
Enter the email address you signed up with and we'll email you a reset link.
- We're Hiring!
- Help Center
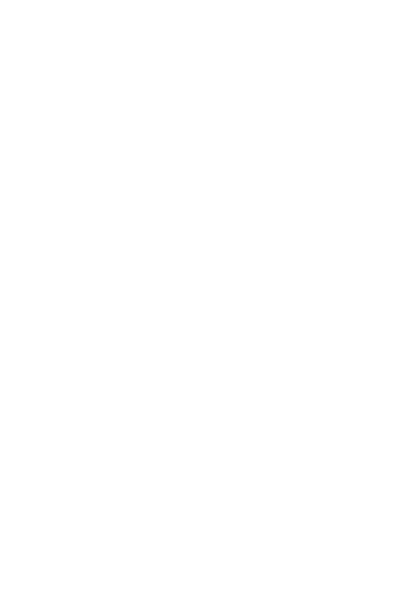
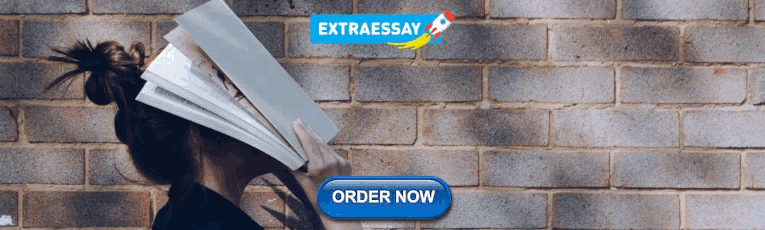
The Sustainability of Tourism Supply Chain: A Case Study Research

2011, Tourismos: an International …
The dynamic environment and the globalization of the tourism sector accelerate the necessity to improve sustainable supply chain management. In tourism sector the supply chain is composed by a diversity of firms with higher heterogeneity and in general without ...
Related Papers
Pema Wangdra
The main objective of this study was to propose the comprehensive sustainable supply chain model for tourism, tourism business mapping and tourism model canvas which can help practitioners in decision making and implementation of policy to sustain tourism industry. Many tourist destinations had extinct due to excessive tourism and general neglect from government, sectors and communities. The lack of sustainable supply chain resulted in disorganization in tourism industry. Therefore, supply chain players need to maintain strong relationship to sustain its resources in generation. The proposed models were created with throughout analysis of past research papers and case studies from Bhutan.Model was implemented to Phu Tub Burg, a popular tourist destination in Phetchaboon province in Thailand. The lack of policy was the reason for the excessive tourist in that place. The model helped to sustain supply chain management in tourism to create and maintain green business in tourism. To sus...
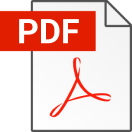
Supply Chain Management of Tourism Towards Sustainability
Kullapa Soratana
Tourism management
Kuwait Chapter of Arabian Journal of Business and Management Review
Shahram Gilaninia
International Journal of Business and Management
Guven Sevil
Journal ijmr.net.in(UGC Approved)
Journal of Sustainable Tourism
wirman syafri
Marianna Sigala
ABSTRACT As more and more travelers wish to create their personalised trip itineraries, the provision of geographical information and services on travel websites is an unavoidable necessity. The evolution of geoportals, geocollaborative portals and web 2.0 present numerous opportunities and services for making the trip planning process less complex and time consuming, more efficient and more social and collaborative for travelers and their travel companions.
Lita Sudiono
The development of tourism is inextricably linked to the operation of tourism enterprises and tourism products offered by them. A competitive advantage for enterprises, in the market of tourist services, can be provided by innovative products comprising a response to the ever-increasing customer expectations. The specificity of product development in the tourism industry requires the commitment and cooperation of many partners – operators in the tourist supply chain. The purpose of this article is to identify the areas of research analysed in the international literature in the field of tourism supply chain, published in the Web of Science. The results of the analysis are presented in the form of a map of themes (research areas) most commonly occurring in configuration with the term of the tourism supply chain, as well as in terms of their coexistence in the analysed pool of articles. The process of preparing the article involved the use of the methods of bibliometric analysis and network analysis.
Anna Farmaki , Patrizia Modica
This study investigates the impacts of economic, social and environmental sustainability practices of companies in the hospitality supply chain on consumers’ satisfaction, loyalty and willingness to pay higher prices. Utilizing data collected from 288 tourists visiting south Sardinia, the study indicates that while economic sustainability practices have positive impacts on consumers’ satisfaction, loyalty and willingness to pay a premium, sustainability practices related to environmental and social dimensions have a direct positive impact on satisfaction and an indirect positive impact on consumer loyalty and willingness to pay a premium. Additionally, findings reveal that satisfaction is likely to mediate the impact of environmental and social sustainability practices on the loyalty of consumers. The theoretical and managerial implications of the study are provided.
Loading Preview
Sorry, preview is currently unavailable. You can download the paper by clicking the button above.
RELATED PAPERS
daria jaremen
Mohamed AbdelAziiz
Mirko Perano
I Putu Astawa
Veronica Popovici
2011 IEEE International Conference on Industrial Engineering and Engineering Management
Suhaiza Zailani
EUROPEAN JOURNAL OF ENVIRONMENTAL SCIENCES
ELENI FELEKI
South Asian Journal of Marketing & Management Research (SAJMMR)
tigist berhanu
Dejan Dragan, Prof., PhD.
International Journal of Supply Chain Management
Mary Ismowati
jalayer khalilzadeh , Pezhman Hatamifar
Sustainability
Victoria Labajo
Gürkan AKDAĞ
Uncertain Supply Chain Management
Ponirin Ponirin
Clarisse Alemão
Anna Farmaki
RELATED TOPICS
- We're Hiring!
- Help Center
- Find new research papers in:
- Health Sciences
- Earth Sciences
- Cognitive Science
- Mathematics
- Computer Science
- Academia ©2024
- Corpus ID: 167653097
The sustainability of tourism supply chain: a case study research.
- M. Costa , L. Carvalho
- Published 2011
- Environmental Science, Business
Figures and Tables from this paper
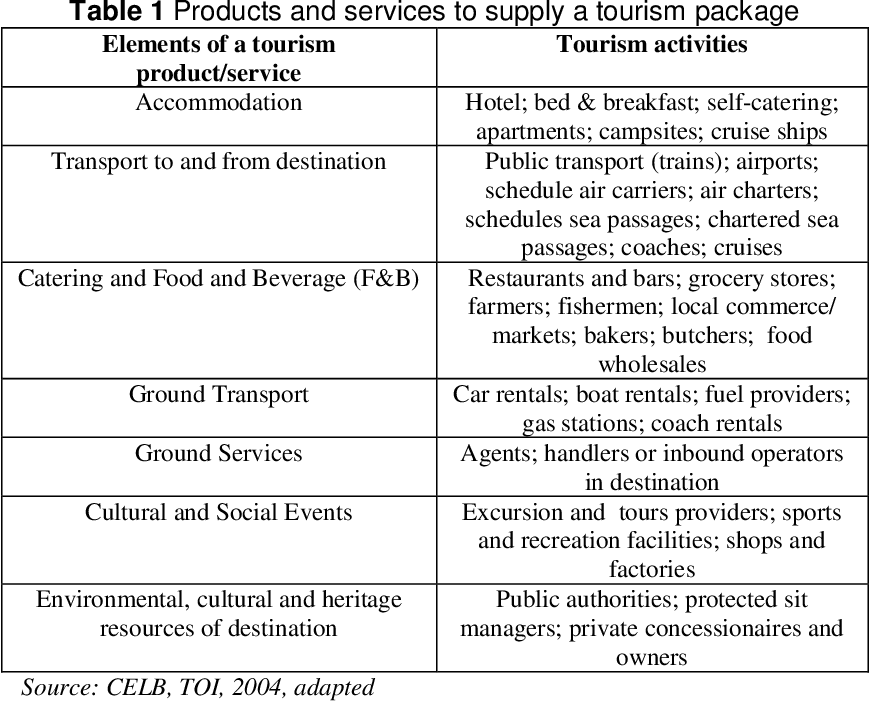
7 Citations
Sustainable supply chain management in tourism: a systematic literature review.
- Highly Influenced
Key Success Factors for improving Community-Based Tourism Supply Chain
From tourism supply chains to tourism value ecology, an evaluation of tourism development as diversification strategy in the united arab emirates, the role of car rental business in travel and tourism industry: a case study of rent a car companies in lefkada island, transport infrastructures, environment impacts and tourists' welfare: a choice experiment to elicit tourist preferences in siena–italy, a multi-national satisfaction analysis: an application on tourists in antalya, 22 references, rhe life-cycle of agro tourist enterprises, product improvement or innovation: what is the key to success in tourism, barriers to implementing sustainable tourism policy in mass tourism destinations, ecotourism and its impact on the regional economy - a study of north bengal (india)., tourism: a community approach, tourism - principles and practice, case study research: design and methods, case study research, design and methods, turismo cidade e cultura: planeamento e gestão sustentável, related papers.
Showing 1 through 3 of 0 Related Papers
Advertisement
Knowledge Dynamics in Rural Tourism Supply Chains: Challenges, Innovations, and Cross-Sector Applications
- Published: 06 March 2024
Cite this article
- Wenming Liu 1 &
- Jingjing Li 2
189 Accesses
Explore all metrics
This study explores the concept and practical application of green supply chains within ecotourism and rural tourism, drawing valuable insights from X Town, China. Here, we redefine ecotourism as encompassing natural and human ecological systems, recognizing its increasing popularity. Notably, we present a green supply chain model for rural tourism, functioning as a comprehensive management system centered on ecological protection and the interconnected optimization of five key chains: information, logistics, capital, knowledge, and service. By analyzing the X Town model, we dissect its five crucial elements. The information chain prioritizes streamlined data flow for optimal decision-making. The logistic chain focuses on robust infrastructure and green product channels for enhanced delivery. The capital chain ensures strategic financial support through a combination of government allocations, social capital, and dedicated credit cards. The knowledge chain fosters innovation and best practices through dynamic knowledge-sharing initiatives. Finally, the service chain prioritizes high-quality tourism experiences alongside robust environmental protection efforts. Using advanced evaluation methods, we assess the performance of X Town’s green supply chain. While the overall score is impressive (89.052), highlighting its success, room for improvement remains, particularly in the service chain (86.582). This indicates the need for further investment in enhancing tourism service quality and environmental protection measures. This analysis provides valuable insights for X Town’s future development, including service chain improvement recommendations, and contributes to the broader understanding of green supply chain management in rural tourism. This data-driven analysis helps policymakers and researchers, and the X Town model may inspire sustainable rural tourism development in other regions.
This is a preview of subscription content, log in via an institution to check access.
Access this article
Subscribe and save.
- Get 10 units per month
- Download Article/Chapter or eBook
- 1 Unit = 1 Article or 1 Chapter
- Cancel anytime
Price includes VAT (Russian Federation)
Instant access to the full article PDF.
Rent this article via DeepDyve
Institutional subscriptions
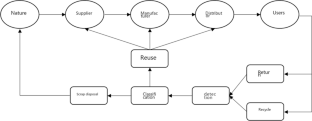
Similar content being viewed by others
Designing a Sustainable Tourism Supply Chain: A Case Study from Asia
Tourism and Sustainability: Transforming Global Value Chains to Networks
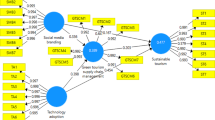
Rebuilding sustainable green tourism supply chain through technology adoption and social media branding in Zimbabwe post-COVID-19.
Explore related subjects.
- Artificial Intelligence
Data Availability
The datasets used and/or analyzed during the current study are available from the corresponding author on reasonable request.
Ahmad, A. F., & Karadas, G. (2021). Managers’ perceptions regarding the effect of leadership on organizational performance: Mediating role of green supply chain management practices. SAGE Open, 11 (2), 21582440211018690.
Article Google Scholar
Almeida, A., & Machado, L. P. (2021). Rural development and rural tourism: The impact of infrastructure investments. In R. A. Castanho, G. Couto, & R. Santos (Eds.), Peripheral territories, tourism, and regional development (p. 1). IntechOpen.
Google Scholar
Amores-Salvadó, J., Martin-de Castro, G., & Albertini, E. (2023). Walking the talk, but above all, talking the walk: Looking green for market stakeholder engagement. Corporate Social Responsibility and Environmental Management, 30 (1), 431–442.
An, W., & Alarcon, S. (2020). How can rural tourism be sustainable? A Systematic Review. Sustainability, 12 (18), 7758.
Bag, S., Pretorius, J. H. C., Gupta, S., & Dwivedi, Y. K. (2021). Role of institutional pressures and resources in the adoption of big data analytics powered artificial intelligence, sustainable manufacturing practices and circular economy capabilities. Technological Forecasting and Social Change, 163 , 120420.
Bag, S., Dhamija, P., Bryde, D. J., & Singh, R. K. (2022). Effect of eco-innovation on green supply chain management, circular economy capability, and performance of small and medium enterprises. Journal of Business Research, 141 , 60–72.
Baloch, Q. B., Shah, S. N., Iqbal, N., Sheeraz, M., Asadullah, M., Mahar, S., & Khan, A. U. (2023). Impact of tourism development upon environmental sustainability: A suggested framework for sustainable ecotourism. Environmental Science and Pollution Research, 30 (3), 5917–5930.
Article PubMed Google Scholar
Prosper Bangwayo-Skeete, Barnes, D. C., Schuhmann, P., Skeete, R. W., & Torres, E. N. (2023). A multisector analysis of tourist delight and positive visitor outcomes. Journal of Quality Assurance in Hospitality & Tourism , 1–32. https://doi.org/10.1080/1528008x.2023.2240015
Ben Hassen, T. (2022). The GCC economies in the wake of COVID-19: Toward post-oil sustainable knowledge-based economies? Sustainability, 14 (18), 11251.
Caffyn, A. (2022). Contested rural landscapes: Contemporary entanglements of tourism and farming. Journal of Sustainable Tourism , 1–23. https://doi.org/10.1080/09669582.2022.2134399
Carlsson, F., Gravert, C., Johansson-Stenman, O., & Kurz, V. (2021). The use of green nudges as an environmental policy instrument. Review of Environmental Economics and Policy, 15 (2), 216–237.
Chao, P. E. N. G., Biao, M. A., & Zhang, C. (2021). Poverty alleviation through e-commerce: Village involvement and demonstration policies in rural China. Journal of Integrative Agriculture, 20 (4), 998–1011.
Chen, J., Guan, J., Xu, J., & Clergeau, C. (2018). Constructing the green supply chain for rural tourism in China: Perspective of front–back stage decoupling. Sustainability, 10 (11), 4276.
Cheng, E. C. (2018). Successful transposition of lesson study: A knowledge management perspective . Springer.
Croce, V. (2018). With growth comes accountability: Could a leisure activity turn into a driver for sustainable growth? Journal of Tourism Futures, 4 (3), 218–232.
Cunha, C., Kastenholz, E., & Carneiro, M. J. (2020). Entrepreneurs in rural tourism: Do lifestyle motivations contribute to management practices that enhance sustainable entrepreneurial ecosystems? Journal of Hospitality and Tourism Management, 44 , 215–226.
Dai, J., Xie, L., & Chu, Z. (2021). Developing sustainable supply chain management: The interplay of institutional pressures and sustainability capabilities. Sustainable Production and Consumption, 28 , 254–268.
de Oliveira Santos, H., Alves, J. L. S., de Melo, F. J. C., & de Medeiros, D. D. (2020). An approach to implement cleaner production in services: Integrating quality management process. Journal of Cleaner Production, 246 , 118985.
de Oliveira, R. T., Ghobakhloo, M., & Figueira, S. (2023). Industry 4.0 towards social and environmental sustainability in multinationals: Enabling circular economy, organizational social practices, and corporate purpose. Journal of Cleaner Production , 139712. https://doi.org/10.1016/j.jclepro.2023.139712
De Silva, M., Howells, J., & Meyer, M. (2018). Innovation intermediaries and collaboration: Knowledge–based practices and internal value creation. Research Policy, 47 (1), 70–87.
Debnath, B., Siraj, M. T., Rashid, K. H. O., Bari, A. M., Karmaker, C. L., & Al Aziz, R. (2023). Analyzing the critical success factors to implement green supply chain management in the apparel manufacturing industry: Implications for sustainable development goals in the emerging economies. Sustainable Manufacturing and Service Economics, 2 , 100013.
Dessai, A. G. (2023). Sustainable tourism. Environment, resources and sustainable tourism: Goa as a case study (pp. 187–228). Springer Nature Singapore.
Chapter Google Scholar
Dharmayanti, N., Ismail, T., Hanifah, I. A., & Taqi, M. (2023). Exploring sustainability management control system and eco-innovation matter sustainable financial performance: The role of supply chain management and digital adaptability in indonesian context. Journal of Open Innovation: Technology, Market, and Complexity, 9 (3), 100119.
Dias, Á. L., Cunha, I., Pereira, L., Costa, R. L., & Gonçalves, R. (2022). Revisiting small-and medium-sized enterprises’ innovation and resilience during COVID-19: The tourism sector. Journal of Open Innovation: Technology, Market, and Complexity, 8 (1), 11.
Dutta, P., Choi, T. M., Somani, S., & Butala, R. (2020). Blockchain technology in supply chain operations: Applications, challenges and research opportunities. Transportation Research Part e: Logistics and Transportation Review, 142 , 102067.
Ebinger, F., & Omondi, B. (2020). Leveraging digital approaches for transparency in sustainable supply chains: A conceptual paper. Sustainability, 12 (15), 6129.
Engesser, V., Rombaut, E., Vanhaverbeke, L., & Lebeau, P. (2023). Autonomous delivery solutions for last-mile logistics operations: A literature review and research agenda. Sustainability, 15 (3), 2774.
Ersoy, P., Börühan, G., Kumar Mangla, S., Hormazabal, J. H., Kazancoglu, Y., & Lafcı, Ç. (2022). Impact of information technology and knowledge sharing on circular food supply chains for green business growth. Business Strategy and the Environment, 31 (5), 1875–1904.
Feng, S., Zhang, R., & Li, G. (2022). Environmental decentralization, digital finance and green technology innovation. Structural Change and Economic Dynamics, 61 , 70–83.
Ferreira, J., Coelho, A., & Moutinho, L. (2020). Dynamic capabilities, creativity and innovation capability and their impact on competitive advantage and firm performance: The moderating role of entrepreneurial orientation. Technovation, 92 , 102061.
Fontoura, P., & Coelho, A. (2022). How to boost green innovation and performance through collaboration in the supply chain: Insights into a more sustainable economy. Journal of Cleaner Production, 359 , 132005.
Funduk, M., Biondić, I., & Simonić, A. L. (2023). Revitalizing rural tourism: A Croatian case study in sustainable practices. Sustainability, 16 (1), 31.
Ghalehkhondabi, I., & Ardjmand, E. (2020). Sustainable E-waste supply chain management with price/sustainability-sensitive demand and government intervention. Journal of Material Cycles and Waste Management, 22 , 556–577.
Govindan, K., & Hasanagic, M. (2018). A systematic review on drivers, barriers, and practices towards circular economy: A supply chain perspective. International Journal of Production Research, 56 (1–2), 278–311.
Gruchmann, T., Topp, M., & Seeler, S. (2022). Sustainable supply chain management in tourism: A systematic literature review. Supply Chain Forum: An International Journal, 23 (4), 329–346. https://doi.org/10.1080/16258312.2022.2085504
Gualandris, J., Longoni, A., Luzzini, D., & Pagell, M. (2021). The association between supply chain structure and transparency: A large-scale empirical study. Journal of Operations Management, 67 (7), 803–827.
Guo, X., Jaramillo, Y. J. L., Bloemhof-Ruwaard, J., & Claassen, G. D. H. (2019). On integrating crowdsourced delivery in last-mile logistics: A simulation study to quantify its feasibility. Journal of Cleaner Production, 241 , 118365.
Gupta, V., Roy, H., Chhabra, M., Sanchez-Canizares, S. M., & Sahu, G. (2022). How the sanitation dimensions impacts consumer perceptions and emotions in five-star hotels due to COVID-19 pandemic? International Journal of Tourism Cities, 8 (4), 912–930.
Gürlek, M., & Kılıç, İ. (2021). A true friend becomes apparent on a rainy day: Corporate social responsibility practices of top hotels during the COVID-19 pandemic. Current Issues in Tourism, 24 (7), 905–918.
Halimatussadiah, A., Nainggolan, D., Yui, S., Moeis, F. R., & Siregar, A. A. (2021). Progressive biodiesel policy in Indonesia: Does the Government’s economic proposition hold? Renewable and Sustainable Energy Reviews, 150 , 111431.
Hampton, M. P., Jeyacheya, J., & Long, P. H. (2018). Can tourism promote inclusive growth? Supply chains, ownership and employment in Ha Long Bay, Vietnam. The Journal of Development Studies, 54 (2), 359–376.
Hjalager, A. M., Kwiatkowski, G., & Østervig Larsen, M. (2018). Innovation gaps in Scandinavian rural tourism. Scandinavian Journal of Hospitality and Tourism, 18 (1), 1–17.
Hong, J., Zheng, R., Deng, H., & Zhou, Y. (2019). Green supply chain collaborative innovation, absorptive capacity and innovation performance: Evidence from China. Journal of Cleaner Production, 241 , 118377.
Jawaad, M., & Zafar, S. (2020). Improving sustainable development and firm performance in emerging economies by implementing green supply chain activities. Sustainable Development, 28 (1), 25–38.
Jayasinghe, R. S., Chileshe, N., & Rameezdeen, R. (2019). Information-based quality management in reverse logistics supply chain. Benchmarking: An International Journal , 26 (7), 2146–2187. https://doi.org/10.1108/bij-08-2018-0238
Ji, Z., Xu, H., & Cui, Q. (2022). Tourism and poverty alleviation in Tibet, China: The role of government in enhancing local linkages. Asia Pacific Journal of Tourism Research, 27 (2), 173–191.
Kazancoglu, Y., Kazancoglu, I., & Sagnak, M. (2018). A new holistic conceptual framework for green supply chain management performance assessment based on circular economy. Journal of Cleaner Production, 195 , 1282–1299.
Kim, N., & Shim, C. (2018). Social capital, knowledge sharing and innovation of small-and medium-sized enterprises in a tourism cluster. International Journal of Contemporary Hospitality Management, 30 (6), 2417–2437.
Kim, S., & Jamal, T. (2018). The co-evolution of rural tourism and sustainable rural development in Hongdong, Korea: Complexity, conflict and local response. In B. Lane & E. Kastenholz (Eds.), Rural tourism (pp. 231–253). Routledge.
Koster, R. L., & Carson, D. A. (2019). Considerations for differentiating among rural tourism geographies. Perspectives on Rural Tourism Geographies , 253–271. https://doi.org/10.1007/978-3-030-11950-8_14
Kuzey, C., Fritz, M. M., Uyar, A., & Karaman, A. S. (2022). Board gender diversity, CSR strategy, and eco-friendly initiatives in the transportation and logistics sector. International Journal of Production Economics, 247 , 108436.
Lane, B., & Kastenholz, E. (Eds.). (2018). Rural tourism: New concepts, new research, new practice . Routledge.
Lau, K. W., Lee, P. Y., & Chung, Y. Y. (2018). A collective organizational learning model for organizational development. Leadership & Organization Development Journal, 40 (1), 107–123.
Lee, S. M., & Choi, D. (2021). Supply chain governance mechanisms, green supply chain management, and organizational performance. Sustainability, 13 (23), 13146.
Leite, H. D. L. K., Binotto, E., Padilha, A. C. M., & de Oliveira Hoeckel, P. H. (2023). Cooperation in rural tourism routes: Evidence and insights. Journal of Hospitality and Tourism Management, 57 , 84–96.
Li, G., Li, L., Choi, T. M., & Sethi, S. P. (2020). Green supply chain management in Chinese firms: Innovative measures and the moderating role of quick response technology. Journal of Operations Management, 66 (7–8), 958–988.
Li, P., Rao, C., Goh, M., & Yang, Z. (2021a). Pricing strategies and profit coordination under a double echelon green supply chain. Journal of Cleaner Production, 278 , 123694.
Li, Y., Liu, B., Chen, P., & Huan, T. C. (2021b). Tourism service providers’ physical attractiveness and customers’ service quality evaluation: Is warmth or competence more important? Tourism Review, 76 (6), 1260–1278.
Lim, S. F. W., Jin, X., & Srai, J. S. (2018). Consumer-driven e-commerce: A literature review, design framework, and research agenda on last-mile logistics models. International Journal of Physical Distribution & Logistics Management, 48 (3), 308–332.
Liu, C., Dou, X., Li, J., & Cai, L. A. (2020). Analyzing government role in rural tourism development: An empirical investigation from China. Journal of Rural Studies, 79 , 177–188.
Ma, L., Liu, Z., Huang, X., & Li, T. (2019). The impact of local government policy on innovation ecosystem in knowledge resource scarce region: Case study of Changzhou, China. Science, Technology and Society, 24 (1), 29–52.
Mani, V., Jabbour, C. J. C., & Mani, K. T. (2020). Supply chain social sustainability in small and medium manufacturing enterprises and firms’ performance: Empirical evidence from an emerging Asian economy. International Journal of Production Economics, 227 , 107656.
McCord, C., Bernhard, P., Walsh, M., Rosner, C., & Console, K. (2020). A consolidated model for telepsychology practice. Journal of Clinical Psychology, 76 (6), 1060–1082.
Article PubMed PubMed Central Google Scholar
Melkonyan, A., Gruchmann, T., Lohmar, F., Kamath, V., & Spinler, S. (2020). Sustainability assessment of last-mile logistics and distribution strategies: The case of local food networks. International Journal of Production Economics, 228 , 107746.
Moadab, A., Farajzadeh, F., & Fatahi Valilai, O. (2022). Drone routing problem model for last-mile delivery using the public transportation capacity as moving charging stations. Scientific Reports, 12 (1), 6361.
Article ADS CAS PubMed PubMed Central Google Scholar
Muduli, K. K., Luthra, S., Kumar Mangla, S., Jabbour, C. J. C., Aich, S., & de Guimaraes, J. C. F. (2020). Environmental management and the “soft side” of organisations: Discovering the most relevant behavioural factors in green supply chains. Business Strategy and the Environment, 29 (4), 1647–1665.
Muniz, E. C. L., Dandolini, G. A., Biz, A. A., & Ribeiro, A. C. (2021). Customer knowledge management and smart tourism destinations: A framework for the smart management of the tourist experience–SMARTUR. Journal of Knowledge Management, 25 (5), 1336–1361.
Musavengane, R., & Kloppers, R. (2020). Social capital: An investment towards community resilience in the collaborative natural resources management of community-based tourism schemes. Tourism Management Perspectives, 34 , 100654.
Naclerio, A. G., & De Giovanni, P. (2022). Blockchain, logistics and omnichannel for last mile and performance. The International Journal of Logistics Management, 33 (2), 663–686.
Nakamoto, Y., Tokito, S., & Kito, M. (2023). Impact of vehicle electrification on global supply chains and emission transfer. Environmental Research Letters, 18 (5), 054021.
Article ADS Google Scholar
Nolan, J., & Bott, G. (2018). Global supply chains and human rights: Spotlight on forced labour and modern slavery practices. Australian Journal of Human Rights, 24 (1), 44–69.
Pakdeechoho, N., & Sukhotu, V. (2018). Sustainable supply chain collaboration: Incentives in emerging economies. Journal of Manufacturing Technology Management, 29 (2), 273–294.
Pan, S. Y., Gao, M., Kim, H., Shah, K. J., Pei, S. L., & Chiang, P. C. (2018). Advances and challenges in sustainable tourism toward a green economy. Science of the Total Environment, 635 , 452–469.
Article ADS CAS PubMed Google Scholar
Pan, J. S., Song, P. C., Chu, S. C., & Peng, Y. J. (2020). Improved compact cuckoo search algorithm applied to location of drone logistics hub. Mathematics, 8 (3), 333.
Panahifar, F., Byrne, P. J., Salam, M. A., & Heavey, C. (2018). Supply chain collaboration and firm’s performance: The critical role of information sharing and trust. Journal of Enterprise Information Management, 31 (3), 358–379.
Pinto, M. M. A., Kovaleski, J. L., Yoshino, R. T., & Pagani, R. N. (2019). Knowledge and technology transfer influencing the process of innovation in green supply chain management: A multicriteria model based on the DEMATEL method. Sustainability, 11 (12), 3485.
Rahman, T., Ali, S. M., Moktadir, M. A., & Kusi-Sarpong, S. (2020). Evaluating barriers to implementing green supply chain management: An example from an emerging economy. Production Planning & Control, 31 (8), 673–698.
Raub, S. P., & Martin-Rios, C. (2019). “Think sustainable, act local”–A stakeholder-filter-model for translating SDGs into sustainability initiatives with local impact. International Journal of Contemporary Hospitality Management, 31 (6), 2428–2447.
Revell, A., Stokes, D., & Chen, H. (2010). Small businesses and the environment: Turning over a new leaf? Business Strategy and the Environment, 19 (5), 273–288.
Rosalina, P. D., Dupre, K., & Wang, Y. (2021). Rural tourism: A systematic literature review on definitions and challenges. Journal of Hospitality and Tourism Management, 47 , 134–149.
Rosyidah, M., Khoirunnisa, N., Rofiatin, U., Asnah, A., Andiyan, A., & Sari, D. (2022). Measurement of key performance indicator Green Supply Chain Management (GSCM) in palm industry with green SCOR model. Materials Today: Proceedings, 63 , S326–S332.
CAS Google Scholar
Ruiz-Real, J. L., Uribe-Toril, J., de Pablo Valenciano, J., & Gázquez-Abad, J. C. (2022). Rural tourism and development: Evolution in scientific literature and trends. Journal of Hospitality & Tourism Research, 46 (7), 1322–1346.
Saarinen, J. (2019). Communities and sustainable tourism development: Community impacts and local benefit creation in tourism. A Research Agenda for Sustainable Tourism , 206–222. https://doi.org/10.4337/9781788117104.00020
Saarinen, J., Rogerson, C. M., & Hall, C. M. (2019). Geographies of tourism development and planning. Tourism planning and development (pp. 1–11). Routledge.
Saberi, S., Kouhizadeh, M., Sarkis, J., & Shen, L. (2019). Blockchain technology and its relationships to sustainable supply chain management. International Journal of Production Research, 57 (7), 2117–2135.
Saeed, A., Rasheed, F., Waseem, M. and Tabash, M.I. (2022). Green human resource management and environmental performance: The role of green supply chain management practices. Benchmarking: An International Journal , 29 (9), 2881–2899. https://doi.org/10.1108/BIJ-05-2021-0297
Sahoo, S., & Vijayvargy, L. (2021). Green supply chain management practices and its impact on organizational performance: Evidence from Indian manufacturers. Journal of Manufacturing Technology Management, 32 (4), 862–886.
Sarkar M.K., Rao, A. C., & Singh, A. K. (2023). Sustainability and Talent management: a two-pronged approach for the evolution of e-commerce in India. Circular Economy and Sustainability . https://doi.org/10.1007/s43615-023-00282-1
Sauer, P. C., & Seuring, S. (2019). Extending the reach of multi-tier sustainable supply chain management–Insights from mineral supply chains. International Journal of Production Economics, 217 , 31–43.
Shafiee, S., Ghatari, A. R., Hasanzadeh, A., & Jahanyan, S. (2019). Developing a model for sustainable smart tourism destinations: A systematic review. Tourism Management Perspectives, 31 , 287–300.
Shahzad, M., Qu, Y., Zafar, A. U., Rehman, S. U., & Islam, T. (2020). Exploring the influence of knowledge management process on corporate sustainable performance through green innovation. Journal of Knowledge Management, 24 (9), 2079–2106.
Sharma, A., Adhikary, A., & Borah, S. B. (2020). Covid-19′ s impact on supply chain decisions: Strategic insights from NASDAQ 100 firms using Twitter data. Journal of Business Research, 117 , 443–449.
Sharma, D., Sharma, J. K., & Srivastava, B. K. (2021). Green and environment sustainable supply chain practices in leading Indian manufacturing companies. In Sustainable energy systems: innovative perspectives: SES 2020 (pp. 89–102). Springer International Publishing.
Sheng, X., Chen, L., Yuan, X., Tang, Y., Yuan, Q., Chen, R., & Liu, H. (2023). Green supply chain management for a more sustainable manufacturing industry in China: A critical review. Environment, Development and Sustainability, 25 (2), 1151–1183.
Shkabatur, J., Bar-El, R., & Schwartz, D. (2022). Innovation and entrepreneurship for sustainable development: Lessons from Ethiopia. Progress in Planning, 160 , 100599.
Singh, J., Singh, H., & Kumar, A. (2020). Impact of lean practices on organizational sustainability through green supply chain management–An empirical investigation. International Journal of Lean Six Sigma, 11 (6), 1035–1068.
Soong, K. K., Ahmed, E. M., & Tan, K. S. (2020). Factors influencing Malaysian small and medium enterprises adoption of electronic government procurement. Journal of Public Procurement, 20 (1), 38–61.
Stoddart, M. C., Catano, G., Ramos, H., Vodden, K., Lowery, B., & Butters, L. (2020). Collaboration gaps and regional tourism networks in rural coastal communities. Journal of Sustainable Tourism, 28 (4), 625–645.
Sung, H., & Kim, S. (2019). The effect of organizational culture on supply chain management in uncertain environments. Asia Pacific Journal of Marketing and Logistics, 31 (4), 1003–1026.
Tran, M., Phan, T., Ha, H., & Hoang, T. (2020). The impact of supply chain quality integration on green supply chain management and environmental performance in Vietnam’s tourism industries. Uncertain Supply Chain Management, 8 (4), 693–704.
Tseng, M. L., Chang, C. H., Wu, K. J., Lin, C. W. R., Kalnaovkul, B., & Tan, R. R. (2019). Sustainable agritourism in Thailand: Modeling business performance and environmental sustainability under uncertainty. Sustainability, 11 (15), 4087.
Tumpa, T. J., Ali, S. M., Rahman, M. H., Paul, S. K., Chowdhury, P., & Khan, S. A. R. (2019). Barriers to green supply chain management: An emerging economy context. Journal of Cleaner Production, 236 , 117617.
Van Tran, N., Van Quyet, T., & Kingsbury, A. J. (2023). Banking for tourism in the digital age: Access to credit and cashless payments for community-based tourism household-owned businesses in Vietnam. Tourism Management Perspectives, 48 , 101144.
Vieira, A. C., Oliveira, M. D., & e Costa, C. A. B. (2020). Enhancing knowledge construction processes within multicriteria decision analysis: The collaborative value modelling framework. Omega, 94 , 102047.
Wan, C., Shen, G. Q., & Choi, S. (2019). Waste management strategies for sustainable development. Encyclopedia of Sustainability in Higher Education , 2020–2028. https://doi.org/10.1007/978-3-030-11352-0_194
Wang, C., Zhang, Q., & Zhang, W. (2020). Corporate social responsibility, green supply chain management and firm performance: The moderating role of big-data analytics capability. Research in Transportation Business & Management, 37 , 100557.
Wondirad, A., Bogale, D., & Li, Y. (2022). Practices and challenges of developing handicrafts as a core tourism product in Chencha and Konso, southern Ethiopia. International Journal of Cultural Policy, 28 (3), 306–326.
Wu, J., & Haasis, H. D. (2018). The freight village as a pathway to sustainable agricultural products logistics in China. Journal of Cleaner Production, 196 , 1227–1238.
Yang, J., Yang, R., Chen, M. H., Su, C. H. J., Zhi, Y., & Xi, J. (2021). Effects of rural revitalization on rural tourism. Journal of Hospitality and Tourism Management, 47 , 35–45.
Zhang, Y., Long, H., Ma, L., Tu, S., Li, Y., & Ge, D. (2022). Analysis of rural economic restructuring driven by e-commerce based on the space of flows: The case of Xiaying village in central China. Journal of Rural Studies, 93 , 196–209.
Zhang, Z., Yu, J., & Tian, J. (2023). Community participation, social capital cultivation and sustainable community renewal: A Case study from Xi’an’s Southern Suburbs, China. Journal of the Knowledge Economy . https://doi.org/10.1007/s13132-023-01536-x
Zhou, Z. (2021). Southern Africa’s morphing into a MICE tourism space: Critical insights for institutional investors. In New frontiers in hospitality and tourism management in Africa (pp. 55–72). Springer International Publishing.
Zhou, Z. (2022). Critical shifts in the global tourism industry: Perspectives from Africa. GeoJournal, 87 (2), 1245–1264.
Zhou, T., Koomen, E., & Ke, X. (2020). Determinants of farmland abandonment on the urban–rural fringe. Environmental Management, 65 , 369–384.
Zhu, H., & Zhang, W. (2023). Infrastructure of inclusive finance in China. Financial inclusion in China: Policy, experience, and outlook (pp. 83–104). Springer Nature Singapore.
Download references
Author information
Authors and affiliations.
Institute of Agriculture Economics and Information, Jilin Academy of Agricultural Sciences, Changchun, 130033, Jilin, China
Wenming Liu
Wu Jinglian School of Economics, Changzhou University, Changzhou, 213164, Jiangsu Province, China
Jingjing Li
You can also search for this author in PubMed Google Scholar
Corresponding author
Correspondence to Jingjing Li .
Ethics declarations
Competing interests.
The authors declare no competing interests.
Additional information
Publisher's note.
Springer Nature remains neutral with regard to jurisdictional claims in published maps and institutional affiliations.
Rights and permissions
Springer Nature or its licensor (e.g. a society or other partner) holds exclusive rights to this article under a publishing agreement with the author(s) or other rightsholder(s); author self-archiving of the accepted manuscript version of this article is solely governed by the terms of such publishing agreement and applicable law.
Reprints and permissions
About this article
Liu, W., Li, J. Knowledge Dynamics in Rural Tourism Supply Chains: Challenges, Innovations, and Cross-Sector Applications. J Knowl Econ (2024). https://doi.org/10.1007/s13132-024-01862-8
Download citation
Received : 14 September 2023
Accepted : 15 February 2024
Published : 06 March 2024
DOI : https://doi.org/10.1007/s13132-024-01862-8
Share this article
Anyone you share the following link with will be able to read this content:
Sorry, a shareable link is not currently available for this article.
Provided by the Springer Nature SharedIt content-sharing initiative
- Supply chain management
- Rural development
- Knowledge dynamics
- Environmental sustainability
- Green practices
- Community engagement
- Find a journal
- Publish with us
- Track your research
To read this content please select one of the options below:
Please note you do not have access to teaching notes, sustainability practices in tourism supply chain: importance performance analysis.
Benchmarking: An International Journal
ISSN : 1463-5771
Article publication date: 8 May 2018
The purpose of this paper is to provide strategic recommendations for Indian hotel administrators for improving sustainability practices: environment, economic and social with respect to the supply chain members by analyzing performance dimensions and the importance attached to them.
Design/methodology/approach
Importance performance analysis is a tool to analyze the perception of top-level, middle-level and first-level managers in hotels. Questionnaire is developed to collect the hotel manager’s perceptions. The snowball sampling method is used for data collection.
The paper introduces specific sustainability practices, namely, environment, economic and social factors, at the interface of the tourism supply chain (TSC). This will allow the hotels to identify the importance and performance of various sustainability practices to achieve a long-term competitive advantage. The present work finds that the responding hotel managers have given highest importance to the sustainability practices within the organization and the hotel manager’s perception of sustainability practices in the TSC will vary with respect to the supply chain members.
Research limitations/implications
The effort has been made to capture specific sustainability practices across the supply chain. The paper reinstates the fact that sustainability practices are not firm specific and should be practiced at the supply chain interface. The data for the study were taken from focal organizations perspective which is the hotels.
Practical implications
Results provide the hotel administrators to develop appropriate strategies to improve their practices and functions by analyzing their strengths and weakness regarding their tangible and intangible assets. The identified sustainability practice attributes can act as a benchmark and drive the hotel industry toward possible cost-saving conditions by prioritizing the allocation of the resources while taking care of overall performance.
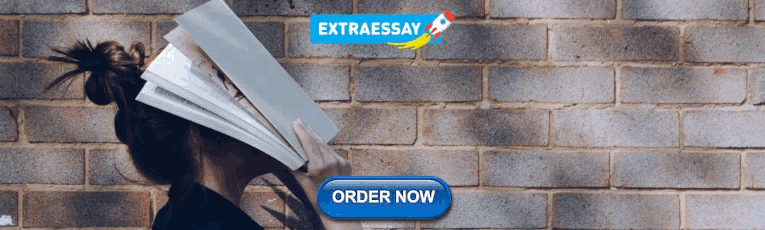
Social implications
Results will help the hotel administrators to identify the better sustainability practices which will reduce the negative effects and protect the Mother Nature.
Originality/value
The study included hotels/resorts from tourism locations: hill station, backwaters and coastal areas, specifically in the Indian context.
- Importance performance analysis
- Sustainability practice
- Action grids
- Tourism supply chain
Acknowledgements
The authors acknowledge the valuable comments given by the reviewers and editor to improve the earlier versions of this paper.
Babu, D.E. , Kaur, A. and Rajendran, C. (2018), "Sustainability practices in tourism supply chain: Importance performance analysis", Benchmarking: An International Journal , Vol. 25 No. 4, pp. 1148-1170. https://doi.org/10.1108/BIJ-06-2016-0084
Emerald Publishing Limited
Copyright © 2018, Emerald Publishing Limited
Related articles
All feedback is valuable.
Please share your general feedback
Report an issue or find answers to frequently asked questions
Contact Customer Support
Pardon Our Interruption
As you were browsing something about your browser made us think you were a bot. There are a few reasons this might happen:
- You've disabled JavaScript in your web browser.
- You're a power user moving through this website with super-human speed.
- You've disabled cookies in your web browser.
- A third-party browser plugin, such as Ghostery or NoScript, is preventing JavaScript from running. Additional information is available in this support article .
To regain access, please make sure that cookies and JavaScript are enabled before reloading the page.
Information
- Author Services
Initiatives
You are accessing a machine-readable page. In order to be human-readable, please install an RSS reader.
All articles published by MDPI are made immediately available worldwide under an open access license. No special permission is required to reuse all or part of the article published by MDPI, including figures and tables. For articles published under an open access Creative Common CC BY license, any part of the article may be reused without permission provided that the original article is clearly cited. For more information, please refer to https://www.mdpi.com/openaccess .
Feature papers represent the most advanced research with significant potential for high impact in the field. A Feature Paper should be a substantial original Article that involves several techniques or approaches, provides an outlook for future research directions and describes possible research applications.
Feature papers are submitted upon individual invitation or recommendation by the scientific editors and must receive positive feedback from the reviewers.
Editor’s Choice articles are based on recommendations by the scientific editors of MDPI journals from around the world. Editors select a small number of articles recently published in the journal that they believe will be particularly interesting to readers, or important in the respective research area. The aim is to provide a snapshot of some of the most exciting work published in the various research areas of the journal.
Original Submission Date Received: .
- Active Journals
- Find a Journal
- Proceedings Series
- For Authors
- For Reviewers
- For Editors
- For Librarians
- For Publishers
- For Societies
- For Conference Organizers
- Open Access Policy
- Institutional Open Access Program
- Special Issues Guidelines
- Editorial Process
- Research and Publication Ethics
- Article Processing Charges
- Testimonials
- Preprints.org
- SciProfiles
- Encyclopedia
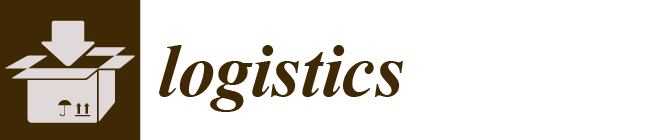
Article Menu
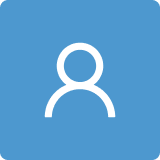
- Subscribe SciFeed
- Recommended Articles
- Google Scholar
- on Google Scholar
- Table of Contents
Find support for a specific problem in the support section of our website.
Please let us know what you think of our products and services.
Visit our dedicated information section to learn more about MDPI.
JSmol Viewer
Simulation model for a sustainable food supply chain in a developing country: a case study of the banana supply chain in malawi.
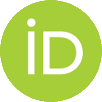
1. Introduction
Problem definition, 2. literature review, 2.1. food sustainable supply chain practices in developing countries, 2.1.1. awareness, 2.1.2. collaboration, 2.1.3. efficiency, 2.1.4. knowledge and information-sharing, 2.1.5. resilience, 2.1.6. governance, 2.2. modelling in sustainable supply chains, 2.2.1. simulation techniques, 2.2.2. design science research, 2.2.3. des and dsr in combination, 2.2.4. gap in the literature, 3. materials and methods, 3.1. dsr methodological approach, 3.2. model input parameters, 3.3. base model assumptions.
- Harvest is always available; therefore, the input is not starved at any point.
- Disruptions caused by resource breakdowns are not modelled (due to a lack of the required statistical data).
- The model operates 24 h, but all operations, up to truck loading, are completed within seven hours, a typical daily shift for the case study.
- A week has five working days, but operations can occur on an additional sixth day.
- Randomness simulation in operations is not performed (due to a lack of statistical data).
- Storage capacity is unlimited at any stage in the SC for the quantities typically harvested.
- Period randomness is evened out.
- There is stable market for the products
3.4. Base Model Validation
3.5. evaluation of alternative model designs, 4.1. standalone model, 4.2. integrated model, 5. discussion, 5.1. theoretical implications, 5.2. managerial implications, 5.3. practical and policy recommendations, 6. conclusions, 6.1. findings, 6.2. research limitations, 6.3. recommendations for future work, supplementary materials, author contributions, data availability statement, conflicts of interest.
- Kiers, J.; Seinhorst, J.; Zwanenburg, M.; Stek, K. Which strategies and corresponding competences are needed to improve supply chain resilience: A COVID-19 based review. Logistics 2022 , 6 , 12. [ Google Scholar ] [ CrossRef ]
- Shakur, M.S.; Lubaba, M.; Debnath, B.; Bari, A.B.M.M.; Rahman, M.A. Exploring the challenges of industry 4.0 adoption in the FMCG sector: Implications for resilient supply chain in emerging economy. Logistics 2024 , 8 , 27. [ Google Scholar ] [ CrossRef ]
- Gardas, B.; Raut, R.; Jagtap, A.H.; Narkhede, B. Exploring the key performance indicators of green supply chain management in agro-industry. J. Model. Manag. 2019 , 14 , 260–283. [ Google Scholar ] [ CrossRef ]
- Gurrala, K.R.; Hariga, M. Key food supply chain challenges: A review of the literature and research gap. Oper. Supply Chain. Manag. 2022 , 15 , 441–460. [ Google Scholar ] [ CrossRef ]
- FAO; IFAD; UNICEF; WFP; WHO. The State of Food Security and Nutrition in the World 2024—Financing to End Hunger, Food Insecurity and Malnutrition in All Its Forms ; FAO: Rome, Italy, 2024. [ Google Scholar ] [ CrossRef ]
- United Nations Environment Programme. Food Waste Index Report 2024 ; United Nations Environment Programme: Nairobi, Kenya, 2024. [ Google Scholar ]
- Guo, J.; Chen, L.; Tang, B. A multi-period multi-objective closed-loop blood supply chain configuration and optimisation under disruption. Int. J. Syst. Sci. Oper. Logist. 2024 , 11 , 2304287. [ Google Scholar ] [ CrossRef ]
- Sharma, S.; Gahlawat, V.K.; Rahul, K.; Mor, R.S.; Malik, M. Sustainable innovations in the food industry through artificial intelligence and big data analytics. Logistics 2021 , 5 , 66. [ Google Scholar ] [ CrossRef ]
- Fan, Y.; de Kleuver, C.; de Leeuw, S.; Behdani, B. Trading off cost, emission, and quality in cold chain design: A simulation approach. Comput. Ind. Eng. 2021 , 158 , 107442. [ Google Scholar ] [ CrossRef ]
- Haji, M.; Kerbache, L.; Muhammad, M.; Al-Ansari, T. Roles of technology in improving perishable food supply chains. Logistics 2020 , 4 , 33. [ Google Scholar ] [ CrossRef ]
- Ahmed, H.F.; Hosseinian-Far, A.; Khandan, R.; Sarwar, D.; E-Fatima, K. Knowledge sharing in the supply chain networks: A perspective of supply chain complexity drivers. Logistics 2022 , 6 , 66. [ Google Scholar ] [ CrossRef ]
- Malik, M.; Gahlawat, V.K.; Mor, R.S.; Dahiya, V.; Yadav, M. Application of optimisation techniques in the dairy supply chain: A systematic review. Logistics 2022 , 6 , 74. [ Google Scholar ] [ CrossRef ]
- Chandrasiri, C.; Dharmapriya, S.; Jayawardana, J.; Kulatunga, A.K.; Weerasinghe, A.N.; Aluwihare, C.P.; Hettiarachchi, D. Mitigating environmental impact of perishable food supply chain by a novel configuration: Simulating banana supply chain in Sri Lanka. Sustainability 2022 , 14 , 12060. [ Google Scholar ] [ CrossRef ]
- Gardas, B.B.; Raut, R.D.; Cheikhrouhou, N.; Narkhede, B.E. A hybrid decision support system for analysing challenges of the agricultural supply chain. Sustain. Prod. Consum. 2019 , 18 , 19–32. [ Google Scholar ] [ CrossRef ]
- Sonar, H.; Sharma, I.; Ghag, N.; Singh, R.K. Barriers for achieving sustainable agri supply chain: Study in context to Indian MSMEs. Int. J. Logist. Res. Appl. 2024 , 27 , 1–20. [ Google Scholar ] [ CrossRef ]
- Marano, V.; Wilhelm, M.; Kostova, T.; Doh, J.; Beugelsdijk, S. Multinational firms and sustainability in global supply chains: Scope and boundaries of responsibility. J. Int. Bus. Stud. 2024 , 55 , 413–428. [ Google Scholar ] [ CrossRef ]
- Lee, C.H. The food crisis in the new cold war era and Korea’s response. In Food in the Making of Modern Korea ; Springer: Singapore, 2024; pp. 265–312. [ Google Scholar ]
- Heydari, M. Cultivating sustainable global food supply chains: A multifaceted approach to mitigating food loss and waste for climate resilience. J. Clean. Prod. 2024 , 442 , 141037. [ Google Scholar ] [ CrossRef ]
- Sukanya, R. Global trade and food security. In Food Security in a Developing World ; Singh, P., Ao, B., Deka, N., Mohan, C., Chhoidub, C., Eds.; Springer: Cham, Switzerland, 2024; pp. 229–258. [ Google Scholar ]
- Yan, B.; Chen, X.; Yuan, Q.; Zhou, X. Sustainability in fresh agricultural product supply chain based on radio frequency identification under an emergency. Cent. Eur. J. Oper. Res. 2020 , 28 , 1343–1361. [ Google Scholar ] [ CrossRef ]
- Gebre, G.G.; Rik, E.; Kijne, A. Analysis of banana value chain in Ethiopia: Approaches to sustainable value chain development. Cogent Food Agric. 2020 , 6 , 1742516. [ Google Scholar ] [ CrossRef ]
- Ikpe, V.; Shamsuddoha, M. Functional model of supply chain waste reduction and control strategies for retailers-the USA retail industry. Logistics 2024 , 8 , 22. [ Google Scholar ] [ CrossRef ]
- Nyalugwe, E.P.; Malidadi, C.; Kabuli, H. An assessment of tomato production practices among rural farmers in major tomato growing districts in Malawi. Afr. J. Agric. Res. 2022 , 18 , 194–206. [ Google Scholar ]
- Nyirenda, Z.; Nankhuni, F.; Brett, M. Has Banana Bunchy Top Disease Turned Malawi into a Banana Importing Country, Forever?—An Analysis of the Malawi Banana Value Chain ; Department of Agricultural, Food, and Resource Economics, Michigan State University: East Lansing, MI, USA, 2019. [ Google Scholar ]
- Mikwamba, K.; Dessein, J.; Kambewa, D. Fighting banana bunchy top disease in Southern Malawi. The interface of knowledge systems and dynamics in a development arena. J. Agric. Educ. Ext. 2019 , 26 , 163–182. [ Google Scholar ] [ CrossRef ]
- FAOSTAT 2023. Available online: https://www.fao.org/faostat/en/#data/QCL (accessed on 17 January 2024).
- Hailu, M.; Workneh, T.S.; Belew, D. Review on postharvest technology of banana fruit. Afr. J. Biotechnol. 2013 , 12 , 635–647. [ Google Scholar ]
- Busogoro, J.-P.; Suleman, J.T.; Ameny, T.; Ndamugoba, D.; Najjemba, A.; Kirichu, S.; Adusabire, M.A.; Munyenyembe, Z.B.; Maulana, T.H.; Okoth, J.R. Reviving the banana industry in Malawi through integrated crop management of local banana germplasm. Trop. Agric. Dev. 2023 , 67 , 1–14. [ Google Scholar ]
- Mbewe, W.; Mtonga, A.; Pankomera, P.; Mwamlima, L.; Katondo, H.; Nyirenda, A.; Phukaphuka, F.; Mataka, L.; Mbalame, H.; Thole, R.; et al. Banana bunchy top virus (Babuvirus; Nanoviridae) detected in all banana growing districts of Malawi. Adv. Sci. Arts 2023 , 1 , 1–13. [ Google Scholar ]
- World Population Review. Banana Consumption by Country 2024. Available online: https://worldpopulationreview.com/country-rankings/banana-consumption-by-country (accessed on 20 May 2024).
- Qasem, A.G.; Aqlan, F.; Shamsan, A.; Alhendi, M. A simulation-optimisation approach for production control strategies in perishable food supply chains. J. Simul. 2021 , 17 , 211–227. [ Google Scholar ] [ CrossRef ]
- Sánchez-Flores, R.B.; Cruz-Sotelo, S.E.; Ojeda-Benitez, S.; Ramírez-Barreto, M.E. Sustainable supply chain management—A literature review on emerging economies. Sustainability 2020 , 12 , 6972. [ Google Scholar ] [ CrossRef ]
- Crippa, M.; Guizzardi, D.; Solazzo, E.; Muntean, M.; Schaaf, E.; Monforti-Ferrario, F.; Banja, M.; Olivier, J.G.J.; Grassi, G.; Rossi, S.; et al. GHG Emissions of All World Countries ; Publications Office of the European Union: Luxembourg, 2021; pp. 1–258. [ Google Scholar ]
- FAO. The State of Food and Agriculture 2019-Moving forward on Food Loss and Waste Reduction ; FAO: Rome, Italy, 2019. [ Google Scholar ]
- Gavurova, B.; Rigelsky, M.; Ivankova, V. Greenhouse Gas Emissions and Health in the Countries of the European Union. Front. Public Health 2021 , 9 , 756652. [ Google Scholar ] [ CrossRef ]
- Li, D.; Wang, X.; Chan, H.K.; Manzini, R. Sustainable food supply chain management. Int. J. Prod. Econ. 2014 , 152 , 1–8. [ Google Scholar ] [ CrossRef ]
- Baba, A.A.M.; Ma’aram, A.; Ishak, F.; Sirat, R.M.; Kadir, A.Z.A. Key performance indicator of sustainability in the Malaysian food supply chain. In Proceedings of the 4th International Conference on Ergonomics & 2nd International Conference on Industrial Engineering, Kuala Terengganu, Malaysia, 27–28 August 2019; pp. 1–7. [ Google Scholar ]
- Mata, M.A.E.; Oguis, G.F.R.; Ligue, K.D.B.; Gamot, R.M.T.; Abaro, K.R.G.; Fordan, Y.C.; Digal, L.N. Model Simulation Approach for Exploring Profitability of Small-scale Cavendish Banana Farmers in Davao Region from Harvest Allocation to Enterprises. Philipp. J. Sci. 2020 , 149 , 283–298. [ Google Scholar ] [ CrossRef ]
- Moussavi, S.-E.; Sahin, E.; Riane, F. A discrete event simulation model assessing the impact of using new packaging in an agri-food supply chain. Int. J. Syst. Sci. Oper. Logist. 2024 , 11 , 2305816. [ Google Scholar ] [ CrossRef ]
- Negi, S. Supply chain efficiency framework to improve business performance in a competitive era. Manag. Res. Rev. 2021 , 44 , 477–508. [ Google Scholar ] [ CrossRef ]
- Raut, R.D.; Gardas, B.B.; Kharat, M.; Narkhede, B. Modeling the drivers of post-harvest losses–MCDM approach. Comput. Electron. Agric. 2018 , 154 , 426–433. [ Google Scholar ] [ CrossRef ]
- Mittal, A.; Krejci, C.C. A hybrid simulation modeling framework for regional food hubs. J. Simul. 2019 , 13 , 28–43. [ Google Scholar ] [ CrossRef ]
- Kumar, A.; Mangla, S.K.; Kumar, P.; Karamperidis, S. Challenges in perishable food supply chains for sustainability management: A developing economy perspective. Bus. Strategy Environ. 2020 , 29 , 1809–1831. [ Google Scholar ] [ CrossRef ]
- Quinlan, C.; Babin, B.; Carr, J.; Griffin, M.; Zikmund, W.G. Business Research Methods , 1st ed.; Cengage Learning EMEA: Hampshire, UK, 2015. [ Google Scholar ]
- Guarnieri, P.; De Aguiar, R.C.C.; Thomé, K.M.; Watanabe, E.A.d.M. The role of logistics in food waste reduction in wholesalers and small retailers of fruits and vegetables: A multiple case study. Logistics 2021 , 5 , 77. [ Google Scholar ] [ CrossRef ]
- Silva, W.H.; Guarnieri, P.; Carvalho, J.M.; Farias, J.S.; Dos Reis, S.A. Sustainable supply chain management: Analysing the past to determine a research agenda. Logistics 2019 , 3 , 14. [ Google Scholar ] [ CrossRef ]
- Tsapi, V.; Assene, M.-N.; Haasis, H.-D. The complexity of the meat supply chain in Cameroon: Multiplicity of actors, interactions and challenges. Logistics 2022 , 6 , 86. [ Google Scholar ] [ CrossRef ]
- Hudnurkar, M.; Jakhar, S.; Rathod, U. Factors affecting collaboration in supply chain: A literature Review. Soc. Behav. Sci. 2014 , 133 , 189–202. [ Google Scholar ] [ CrossRef ]
- Nesadurai, H.E.S. Transnational private governance as a developmental driver in Southeast Asia: The case of sustainable palm oil standards in Indonesia and Malaysia. J. Dev. Stud. 2019 , 55 , 1892–1908. [ Google Scholar ] [ CrossRef ]
- Sharma, R.; Kannan, D.; Darbari, J.D.; Jha, P.C. Group decision making model for selection of performance indicators for sustainable supplier evaluation in agro-food supply chain. Int. J. Prod. Econ. 2024 . [ Google Scholar ] [ CrossRef ]
- Obonyo, E.; Formentini, M.; Ndiritu, W.S.; Naslund, D. Information sharing in African perishable agri-food supply chains: A systematic literature review and research agenda. J. Agribus. Dev. Emerg. Econ. 2023 . [ Google Scholar ] [ CrossRef ]
- Biza, A.; Montastruc, L.; Negny, S.; Admassu, S. Strategic and tactical planning model for the design of perishable product supply chain network in Ethiopia. Comput. Chem. Eng. 2024 , 190 , 108814. [ Google Scholar ] [ CrossRef ]
- Mangla, S.K.; Luthra, S.; Rich, N.; Kumar, D.; Rana, N. Enablers to implement sustainable initiatives in agri-food supply chains. Int. J. Prod. Econ. 2018 , 203 , 379–393. [ Google Scholar ] [ CrossRef ]
- Zaman, S.I.; Kusi-Sarpong, S. Identifying and exploring the relationship among the critical success factors of sustainability toward consumer behavior. J. Model. Manag. 2024 , 19 , 492–522. [ Google Scholar ] [ CrossRef ]
- Rojas-Reyes, J.J.; Rivera-Cadavid, L.; Peña-Orozco, D.L. Disruptions in the food supply chain: A literature review. Heliyon 2024 , 10 . [ Google Scholar ] [ CrossRef ] [ PubMed ]
- Huong, P.T.T.; Everaarts, A.P.; Neeteson, J.J.; Struik, P.C. Vegetable production in the Red River Delta of Vietnam. Wagening. J. Life Sci. 2013 , 67 , 27–36. [ Google Scholar ] [ CrossRef ]
- Asian, S.; Hafezalkotob, A.; John, J.J. Sharing economy in organic food supply chains: A pathway to sustainable development. Int. J. Prod. Econ. 2019 , 218 , 322–338. [ Google Scholar ] [ CrossRef ]
- Namany, S.; Govindan, R.; Alfagih, L.; McKay, G.; Al-Ansari, T. Sustainable food security decision-making: An agent-based modelling approach. J. Clean. Prod. 2020 , 255 , 120296. [ Google Scholar ] [ CrossRef ]
- Han, J.-W.; Zuo, M.; Zhu, W.-Y.; Zuo, J.-H.; Yang, X.-T. A comprehensive review of cold chain logistics for fresh agricultural products: Current status, challenges, and future trends. Trends Food Sci. Technol. 2021 , 109 , 536–551. [ Google Scholar ] [ CrossRef ]
- Tchonkouang, R.D.; Onyeaka, H.; Nkoutchou, H. Assessing the vulnerability of food supply chains to climate change-induced disruptions. Sci. Total Environ. 2024 , 920 , 171047. [ Google Scholar ] [ CrossRef ]
- Tako, A.A.; Robinson, S. Model development in discrete-event simulation and system dynamics: An empirical study of expert modellers. Eur. J. Oper. Res. 2010 , 207 , 784–794. [ Google Scholar ]
- Koulouris, A.; Misailidis, N.; Petrides, D. Applications of process and digital twin models for production simulation and scheduling in the manufacturing of food ingredients and products. Food Bioprod. Process. 2021 , 126 , 317–333. [ Google Scholar ] [ CrossRef ]
- Turnitsa, C. Conceptual Modeling. In Modeling and Simulation in the Systems Engineering Life Cycle-Core Concepts and Accompanying Lectures ; Loper, M.L., Ed.; Springer: London, UK, 2015; pp. 39–49. [ Google Scholar ]
- Kelton, D.; Sadowski, R.P.; Zupick, N.B. Simulation with Arena , 6th ed.; McGraw-Hill Education: New York, NY, USA, 2015. [ Google Scholar ]
- Gupta, E.; Kanu, N.J.; Munot, A.; Sutar, V.; Vates, U.K.; Singh, G.K. Stochastic and Deterministic Mathematical Modeling and Simulation to Evaluate the Novel COVID-19 Pandemic Control Measures. Am. J. Infect. Dis. 2020 , 16 , 134–170. [ Google Scholar ] [ CrossRef ]
- Rossetti, M.D. Simulation Modeling and Arena ; Open Educational Resources: Fayetteville, NC, USA, 2021. [ Google Scholar ]
- McGarraghy, S.; Olafsdottir, G.; Kazakov, R.; Huber, É.; Loveluck, W.; Gudbrandsdottir, I.Y.; Čechura, L.; Esposito, G.; Samoggia, A.; Aubert, P.-M.; et al. Conceptual system dynamics and agent-based modelling simulation of interorganisational fairness in food value chains: Research agenda and case studies. Agriculture 2022 , 12 , 280. [ Google Scholar ] [ CrossRef ]
- Vaishnavi, V.K.; Kuechler, W.J. Design Science Research Methods and Patterns: Innovating Information and Communication Technology , 2nd ed.; Taylor & Francis Group: Boca Raton, FL, USA, 2015. [ Google Scholar ]
- Venable, J.; Baskerville, R. Eating our own cooking: Toward a more rigorous design science of research methods. Electron. J. Bus. Res. Methods 2012 , 10 , 141–153. [ Google Scholar ]
- Peffers, K.; Tuunanen, T.; Niehaves, B. Design science research genres: Introduction to the special issue on exemplars and criteria for applicable design science research. Eur. J. Inf. Syst. 2018 , 27 , 129–139. [ Google Scholar ] [ CrossRef ]
- Budde, L.; Liao, S.; Haenggi, R.; Friedli, T. Use of DES to develop a decision support system for lot size decision-making in manufacturing companies. Prod. Manuf. Res. 2022 , 10 , 494–518. [ Google Scholar ] [ CrossRef ]
- Maïzi, Y.; Bendavid, Y. Hybrid RFID-IoT simulation modeling approach for analysing scrubs’ distribution solutions in operating rooms. Bus. Process Manag. J. 2023 , 29 , 1734–1761. [ Google Scholar ] [ CrossRef ]
- Feng, P.; Feenberg, A. Thinking About Design: Critical Theory of Technology and the Design Process. In Philosophy and Design: From Engineering to Architecture ; Vermaas, P.E., Kroes, P., Light, A., Moore, S.A., Eds.; Springer: Berlin/Heidelberg, Germany, 2008; pp. 105–118. [ Google Scholar ]
- Bardzell, S.; Bardzell, J.; Forlizzi, J.; Zimmerman, J.; Antanitis, J. Critical Design and Critical Theory: The Challenge of Designing for Provocation. In Proceedings of the DIS ‘12: Proceedings of the Designing Interactive Systems Conference, Newcastle, UK, 11 June 2012; pp. 288–297. [ Google Scholar ]
- Brat, P.; Bugaud, C.; Guillermet, C.; Salmon, F. Review of banana green life throughout the food chain: From auto-catalytic induction to the optimisation of shipping and storage conditions. Sci. Hortic. 2020 , 262 , 109054. [ Google Scholar ] [ CrossRef ]
Click here to enlarge figure
Reference | Awareness | Collaboration | Efficiency | KIS | Resilience | Governance | Modelling Approach |
---|---|---|---|---|---|---|---|
[ ] | ✓ | ✓ | ✓ | ||||
[ ] | ✓ | Mathematical | |||||
[ ] | ✓ | Mathematical | |||||
[ ] | ✓ | ✓ | |||||
[ ] | ✓ | ✓ | Mathematical | ||||
[ ] | ✓ | ✓ | |||||
[ ] | ✓ | ||||||
[ ] | ✓ | ||||||
[ ] | ✓ | ||||||
[ ] | ✓ | Simulation | |||||
[ ] | ✓ | ✓ | |||||
[ ] | ✓ | ✓ | ✓ | Mathematical | |||
[ ] | ✓ | ✓ | ✓ | Mathematical | |||
[ ] | ✓ | ✓ | |||||
[ ] | ✓ | ✓ | |||||
[ ] | ✓ | ✓ | ✓ | ✓ | |||
[ ] | ✓ | ✓ | ✓ | ||||
[ ] | ✓ | ✓ | ✓ | ✓ | |||
[ ] | ✓ | ✓ | ✓ | ||||
[ ] | ✓ | ✓ | ✓ | ✓ | ✓ | Mathematical | |
[ ] | ✓ | ✓ | Mathematical | ||||
[ ] | ✓ | ✓ | |||||
[ ] | ✓ | ✓ | ✓ | ||||
[ ] | ✓ | ✓ | Simulation | ||||
[ ] | ✓ | ✓ | ✓ | ||||
This paper | ✓ | ✓ | ✓ | ✓ | ✓ | ✓ | Simulation and DSR |
Activity/Observation | Distribution Type | Data Points | Mean (Seconds) | Expression | Mean Square Error | df | Chi-Square p-Value |
---|---|---|---|---|---|---|---|
Big trailer reaping | Beta | 100 | 140 | 39 + 240 × BETA (1.18, 1.58) | 0.007812 | 4 | 0.236 |
Big trailer loading | Beta | 100 | 25 | 15.5 + 18 × BETA (1.23, 1.11) | 0.018668 | 5 | 0.345 |
Big trailer transfer | Lognormal | 8 | 4.4 | 3 + LOGN (1.35, 1.16) | 0.057789 | - | - |
Big trailer unloading | Gamma | 100 | 10.2 | 3.5 + GAMM (3.36, 1.99) | 0.007416 | 5 | 0.349 |
Small trailer reaping | Beta | 100 | 360 | 88 + 558 × BETA (2.3, 2.42) | 0.007506 | 3 | 0.203 |
Small trailer loading | Beta | 100 | 15.1 | 10.5 + 9 × BETA (1.01, 0.959) | 0.004127 | 6 | 0.703 |
Small trailer transfer | Beta | 30 | 14.7 | 9.5 + 11 × BETA (0.851, 0.949) | 0.048836 | 2 | 0.116 |
Small trailer unloading | Beta | 80 | 8.3 | 5.5 + 5 × BETA (2.04, 1.6) | 0.012422 | 1 | 0.228 |
Weighing and packing in the grading shed | Beta | 120 | 25.2 | 14.5 + 21 × BETA (0.836, 0.811) | 0.006946 | 7 | 0.132 |
Truck loading | Beta | 250 | 45.1 | 29.5 + 31 × BETA (1.09, 1.08) | 0.004698 | 12 | 0.239 |
Bunch weight | Normal | 300 | 19.456 | NORM (19.5, 4.37) | 0.001133 | 11 | 0.75 |
Indicator | Definition Used | Base Unit | Base Value | Calculation Method |
---|---|---|---|---|
Total production cost | The costs associated with processing services, specifically banana transport from a farm to the customer’s location. | Kwacha | 60,000 | Addition of all operating costs during a system run |
Labour availability | Labour resources to run a process. | Percentage | 74.1 | Available labour divided by required labour |
Lead-time | The time taken from harvesting to completion of sales at the case study company, including waiting time | Hours | 4.8 | Exit time subtract entry time |
Food quality | The ratio of total demand to shortages or wastage of supplied quantity, assuming demand equals harvested amounts. | Percentage | 94.3 | Harvest—waste |
demand | ||||
Shelf-life | The shelf-life is determined by subtracting processing and transport time from the difference between the harvest day and the last day of marketable quality. | Days | 7 | Last usable time subtract harvest time |
Throughput No. of bunches) | The total number of products that exited the system to be available for customers. | Number (bunches) | 128 | |
Throughput (Bunch weight) | The total weight of products that left the system and were available for customers. | Kg | 2510 | Bunch number multiplied by bunch weight mean |
Wastage | The proportion of unconsumed products in a system is determined by subtracting the total harvested from the throughput. | Percentage | 5.7 | Products in, subtract products out |
Indicator | Base Unit | Actual System | Base Model Mean | t-Statistic | p-Value (Two-Sided) |
---|---|---|---|---|---|
Total production cost | Kwacha | 60,000 | 60,012 | −0.022 | 0.982 |
Labour availability | Percentage | 74.1 | 74.10 | ||
Lead-time | Hours | 4.8 | 4.75 | 0.157 | 0.876 |
Food quality | Percentage | 94.3 | 93.47 | 0.426 | 0.671 |
Shelf-life | Days | 7 | 7.39 | −0.155 | 0.877 |
Throughput (Number) | No. of bunches | 128 | 127.75 | −0.017 | 0.986 |
Throughput (Weight) | kg | 2510 | 2527 | −0.17 | 0.095 |
Wastage | Percentage | 5.7 | 6.53 | −0.419 | 0.676 |
Indicator | Base Unit | Base Model Mean | Standalone Simulation Model Mean | Mean Difference | % Difference | t-Statistic | p-Value (Two-Sided) |
---|---|---|---|---|---|---|---|
Total production cost | Kwacha | 60,012 | 58,579 | −1432.96 | 2 | 7.379 | <0.001 |
Labour availability | Percentage | 74.1 | 74.10 | 0.00 | 0 | 0 | 0 |
Lead-time | Hours | 4.75 | 3.46 | 1.29 | 27 | 8.327 × 10 | <0.001 |
Food quality | Percentage | 93.47 | 97.54 | −4.07 | 4 | −3.521 | <0.001 |
Shelf-life | Days | 7.39 | 13.89 | −6.41 | 87 | −8.558 | <0.001 |
Throughput (Number) | No. of bunches | 127.75 | 128.25 | 0.25 | 0 | 0.011 | 0.992 |
Throughput (Weight) | kg | 2527 | 2623.49 | 0.18 | 0 | 0 | 1 |
Wastage | Percentage | 6.53 | 2.46 | 4.07 | 62 | 3.521 | <0.001 |
Indicator * | Base Unit | Base Model Mean | Integrated Model Mean | Mean Difference | % Difference | t-Statistic | p-Value (Two-Sided) |
---|---|---|---|---|---|---|---|
Total production cost | Kwacha | 60,012 | 63,724.8 | −3713 | 6 | −43.389 | <0.001 |
Labour availability ** | Percentage | 74.1 | |||||
Lead-time | Hours | 4.7 | 2.5 | 2.2 | 48 | 135.748 | <0.001 |
Food quality | Percentage | 93.5 | 97.47 | −3.97 | 4 | −17.339 | <0.001 |
Shelf-life | Days | 6.9 | 14.0 | −7.1 | 93 | −25.072 | <0.001 |
Throughput (Number) | No. of Bunches | 128 | 194 | −65.26 | 51 | −52.22 | <0.001 |
Throughput (Weight) | kg | 2527 | 3853 | −1326 | 52 | −12.553 | <0.001 |
Wastage | Percentage | 6.5 | 2.5 | 4 | 61 | 17.339 | <0.001 |
Indicator | Base Unit | Base Value | Base Model Output | Simulated Model Output | Difference | Percentage Difference |
---|---|---|---|---|---|---|
Total production costs | Kwacha | 45,120,000 | 45,302,028 | 48,175,949 | 2,873,921 | 6 |
Labour availability | Percentage | 74.1 | 74.1 | 100 | 26 | 35 |
Lead-time | Hours (mean) | 4.8 | 4.7 | 2 | −2 | 47 |
Food quality | Percentage (mean) | 94.3 | 93.5 | 97 | 4 | 4 |
Shelf-life | Days (mean) | 7 | 7.6 | 14 | 6 | 85 |
Throughput (Number) | No. of bunches | 96,256 | 96,928 | 146,266 | 49,338 | 51 |
Throughput (Weight) | kg | 1,897,560 | 1,910,275 | 2,912,643 | 1,002,368 | 52 |
Wastage | Percentage (mean) | 5.7 | 6.5 | 3 | −4 | 61 |
The statements, opinions and data contained in all publications are solely those of the individual author(s) and contributor(s) and not of MDPI and/or the editor(s). MDPI and/or the editor(s) disclaim responsibility for any injury to people or property resulting from any ideas, methods, instructions or products referred to in the content. |
Share and Cite
Moyo, E.H.; Carstens, S.; Walters, J. Simulation Model for a Sustainable Food Supply Chain in a Developing Country: A Case Study of the Banana Supply Chain in Malawi. Logistics 2024 , 8 , 85. https://doi.org/10.3390/logistics8030085
Moyo EH, Carstens S, Walters J. Simulation Model for a Sustainable Food Supply Chain in a Developing Country: A Case Study of the Banana Supply Chain in Malawi. Logistics . 2024; 8(3):85. https://doi.org/10.3390/logistics8030085
Moyo, Evance Hlekwayo, Stephen Carstens, and Jackie Walters. 2024. "Simulation Model for a Sustainable Food Supply Chain in a Developing Country: A Case Study of the Banana Supply Chain in Malawi" Logistics 8, no. 3: 85. https://doi.org/10.3390/logistics8030085
Article Metrics
Article access statistics, further information, mdpi initiatives, follow mdpi.
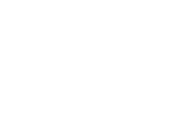
Subscribe to receive issue release notifications and newsletters from MDPI journals
references.bib
What if? Causal Machine Learning in Supply Chain Risk Management
The penultimate goal for developing machine learning models in supply chain management is to make optimal interventions. However, most machine learning models identify correlations in data rather than inferring causation, making it difficult to systematically plan for better outcomes. In this article, we propose and evaluate the use of causal machine learning for developing supply chain risk intervention models, and demonstrate its use with a case study in supply chain risk management in the maritime engineering sector. Our findings highlight that causal machine learning enhances decision-making processes by identifying changes that can be achieved under different supply chain interventions, allowing ”what-if” scenario planning. We therefore propose different machine learning developmental pathways for for predicting risk, and planning for interventions to minimise risk and outline key steps for supply chain researchers to explore causal machine learning.
Keywords: Supply Chain Risk Management, Supply Chain, Machine Learning, Causal Inference, Causal Machine Learning, Delay Prediction
1 Introduction
Advances in artificial intelligence (AI) and machine learning (ML) have opened novel paths to inferring new knowledge from supply chain data, yielding advances in classical problems such as demand forecasting \parencite Soori2023, and entirely new areas such as delay prediction and uncovering hidden supply chain dependencies \parencite Kosasih_2021, Wyrembek_2023.
While these advances have opened up new opportunities, managers often highlight the ”black-box” problem when using AI to make decisions as they are ultimately held responsible if anything goes wrong. The lack of transparency and interpretability in ML models, as well as the provision of comprehensive explanations of AI outputs can lead to a lack of trust and reluctance to fully integrate these technologies into decision-making processes. For instance, consider a model built for predicting a supplier delivery delay. A deep learning model, although providing insights into patterns and trends, would fall short in identifying the underlying causal mechanisms that drive a delay, leading to potential oversights in risk mitigation strategies. For a planner, the ultimate goal of developing an ML model might be to make an intervention such that the foreseen delay is minimised. There might be a number of alternative interventions. For example, one might order from an alternative supplier, order a different quantity or order at a different time period. The planner thus would need to estimate the effect of one of these interventions on the outcome. Doing so, would require a shift from identifying correlations in data, to identifying the underlying causal mechanisms that drive an outcome.
Our aim in this article is not to criticise the use of AI and ML for the development of supply chain risk prediction models, but to highlight that prediction and intervention models have different developmental paths and intended uses. In domains such as healthcare, this observation has motivated research in causal machine learning (CML), which combines machine learning with causal inference to estimate intervention effects and optimise the allocation of interventions amongst groups of intervention recipients. In tandem, there have been calls for more explainable AI in supply chain management \parencite kosasih2024review, and inquiries on how best to use ML models for making optimal interventions. These calls emphasise the growing need for methodologies that not only predict outcomes but also provide actionable insights that can inform strategic decisions. However, to date there have been no studies on CML in supply chain management - a methodology which might help address both issues.
CML is used to estimate a causal quantity of change in a given outcome due to the result of an intervention \parencite kaddour2022causal. A key benefit of CML is that it allows for the estimation of individualised interventions on outcome variables, so that decision-making can be tailored to a given context. CML can handle high dimensional unstructured data and establish which sub groups of data are best recipients of a given intervention. As such, one can perform ”what if” reasoning to evaluate how outcomes will change due to an intervention.
In this article we outline the key steps in developing CML for supply chain intervention models, using a case study in supply chain risk management (SCRM) in the maritime engineering sector. Our hope is that doing so presents an illustrative case that can motivate the use of CML in SCRM tasks where optimal interventions are required. We then discuss the limitations of CML, propose directions for future research and provide recommendations for the reliable use of CML.
2 Causal Machine Learning
Causal machine learning is different from traditional predictive ML, in that traditional ML aims at predicting outcomes, while CML quantifies changes in outcomes due to a given intervention (termed as ”treatment” in CML).
Although methods for estimating intervention effects have a long tradition in the statistical literature, CML offers distinct benefits by combining ML with causal inference. For example, methods from classical statistics often assume prior knowledge about the association between input and output variables, such as linear dependencies. Such knowledge is often not available or assumptions are unrealistic, especially for high-dimensional datasets.
Estimating intervention effects from data is non trivial due to a number of challenges. The first of these is the causal inference problem, where we aim to infer causality from data. Consider the following case of developing an ML model to predict quality defects in a product. In the developed model, it appears that the use of a new supplier is correlated to an increase in defects. If we look closely, we could see that there has been a change in the design of a component of the product, leading to defects. The design change, here, is a confounding variable , which is related to both the input variable (supplier) and the output variable (defects) in a model and can distort the causal relationship between them, giving rise to an apparent causal relationship that is actually spurious. A further complication occurs when intervention effects for individual problem cases may not be observable because one can only observe the factual outcome under a given intervention (e.g. a defect after a supplier has been chosen), but one cannot observe the counterfactual outcome under a different intervention scenario (what the outcome would be, had a different supplier been chosen).
CML seeks to address these limitations by a double/debiased machine learning (DML) framework. DML was first introduced by \textcite chernozhukov2018double and is based on the principles of the Frisch-Waugh-Lovell Theorem \parencite frisch1933partial, lovell1963seasonal. This theorem states that, given the linear model Y= β 0 subscript 𝛽 0 \beta_{0} italic_β start_POSTSUBSCRIPT 0 end_POSTSUBSCRIPT + β 1 subscript 𝛽 1 \beta_{1} italic_β start_POSTSUBSCRIPT 1 end_POSTSUBSCRIPT D+ β 2 subscript 𝛽 2 \beta_{2} italic_β start_POSTSUBSCRIPT 2 end_POSTSUBSCRIPT Z+U, the two following approaches for estimating β 1 subscript 𝛽 1 \beta_{1} italic_β start_POSTSUBSCRIPT 1 end_POSTSUBSCRIPT yield the same result:
Linear regression of Y on D and Z, using Ordinalry Least Squares (OLS).
Three-step procedure: 1) regress D on Z; 2) regress Y on Z; 3) regress the residuals from step 2 on the residuals from step 1 for getting an estimate β 1 subscript 𝛽 1 \beta_{1} italic_β start_POSTSUBSCRIPT 1 end_POSTSUBSCRIPT (all regressions using OLS).
In a similar manner, in DML we can proceed as follows:
Predict D based on Z using a suitable ML algorithm;
Predict Y based on Z using a suitable ML algorithm;
Linear regression of the residuals from step 2 on the residuals from step 1, for getting an estimate of θ 0 subscript 𝜃 0 \theta_{0} italic_θ start_POSTSUBSCRIPT 0 end_POSTSUBSCRIPT .
A wide range of ML approaches, such as random forests, boosting or neural networks, can be utilised for the prediction tasks above \parencite Bach_2022_JMLR.
𝜂 \partial_{\eta} ∂ start_POSTSUBSCRIPT italic_η end_POSTSUBSCRIPT indicating the pathwise Gateaux derivative operator.
As discussed earlier, the use of ML estimators to approximate the nuisance functions η 0 subscript 𝜂 0 \eta_{0} italic_η start_POSTSUBSCRIPT 0 end_POSTSUBSCRIPT in steps 2 and 3 introduces a regularisation bias. Imposing the Neyman orthogonality condition to our score function (and thus to our estimators of θ 0 subscript 𝜃 0 \theta_{0} italic_θ start_POSTSUBSCRIPT 0 end_POSTSUBSCRIPT as well as η 0 subscript 𝜂 0 \eta_{0} italic_η start_POSTSUBSCRIPT 0 end_POSTSUBSCRIPT makes our estimator of θ 0 subscript 𝜃 0 \theta_{0} italic_θ start_POSTSUBSCRIPT 0 end_POSTSUBSCRIPT free of the regularisation bias.
A final ingredient of DML is the strategy of sample splitting. Estimating the nuisance functions on the same dataset used for the parameter θ 0 subscript 𝜃 0 \theta_{0} italic_θ start_POSTSUBSCRIPT 0 end_POSTSUBSCRIPT can lead to overfitting bias. However, this issue can be mitigated through sample splitting, meaning that the nuisance functions η 0 subscript 𝜂 0 \eta_{0} italic_η start_POSTSUBSCRIPT 0 end_POSTSUBSCRIPT are estimated using one part of the dataset, while the score function for the desired parameter θ 0 subscript 𝜃 0 \theta_{0} italic_θ start_POSTSUBSCRIPT 0 end_POSTSUBSCRIPT is computed with the remaining data. Implementing sample splitting across K 𝐾 K italic_K folds and adopting repeated cross-fitting enhances the robustness of the estimates \parencite bach2024doubleml. For this, we follow the procedure below:
randomly partition our data into two subsets
fit our ML models for D and Y on the first subset.
estimate θ 0 , 1 \theta_{0},_{1} italic_θ start_POSTSUBSCRIPT 0 end_POSTSUBSCRIPT , start_POSTSUBSCRIPT 1 end_POSTSUBSCRIPT in the second subset using the models obtained in step 2.
fit our ML models in the second subset.
estimate θ 0 , 2 \theta_{0},_{2} italic_θ start_POSTSUBSCRIPT 0 end_POSTSUBSCRIPT , start_POSTSUBSCRIPT 2 end_POSTSUBSCRIPT in the first subset, using the models obtained in step 4.
obtain our final estimator θ 0 subscript 𝜃 0 \theta_{0} italic_θ start_POSTSUBSCRIPT 0 end_POSTSUBSCRIPT as an average of θ 0 , 1 \theta_{0},_{1} italic_θ start_POSTSUBSCRIPT 0 end_POSTSUBSCRIPT , start_POSTSUBSCRIPT 1 end_POSTSUBSCRIPT and θ 0 , 2 \theta_{0},_{2} italic_θ start_POSTSUBSCRIPT 0 end_POSTSUBSCRIPT , start_POSTSUBSCRIPT 2 end_POSTSUBSCRIPT
The choice of the ML model itself is case dependent. In the case study presented in the next section, we choose an interactive regression model (IRM). We do so because our treatment (intervention variable) is binary which an IRM can handle in the DML framework. We will discuss the steps involved in applying DML, including IRM, within the context of the case study next.
3 Causal Machine Learning applied to Supply Chain Risk Management: An illustrative case study
3.1 related research.
Leaning on the observation that most of extant SCRM research focuses on post-delay mitigation strategies, recent advances in ML research have presented a promising avenue for predicting risk. Based on this, one of the earliest applications of ML in supply chain management was on supplier delay prediction \parencite brintrup2020supply, where Material Requirements Planning (MRP) data was used to predict order delays. Several follow on studies investigated classification models (e.g. \textcite LOLA, Sarbas2023, WANI2022731, douaioui2024enhancing, BASSIOUNI2024), and regression based approaches (e.g. \textcite STEINBERG2023100003, Gabellini_2024, balster2020eta, Shah_23) to predict delay severity and lead times. Other research focused on the role of information sharing for delay prediction among multiple buyers \parencite Zheng2023, efficacy of ML to predict delays during Covid-19 (e.g. \textcite Zaghdoudi_2022, mariappan2022large, mariappan2023using). Delay prediction since then has been applied to aerospace, maritime, eCommerce, and pharmaceutical case studies.
Whilst delay prediction has been a popular area of research, authors raised the lack of interpretability on the use of ML in evaluating risk, which prevent these models to be used in practical contexts \parencite baryannis2019predicting, brintrup2023trustworthy. Authors proposed the use of interpretable ML models such as decision trees \parencite baryannis2019predicting, multi-criteria decision-making \parencite WyrembekBaryannis2024,ABDULLA2023100342,ABDULLA2024100074 and neurosymbolic methods \parencite kosasih2024review. However, they also pointed out the trade off between performance and interpretability - black box ML models were often more accurate but their findings were not easily interpretable.
In this study we take an alternative view on this conundrum, highlighting the different developmental paths for the use of ML in SCRM. The first of these is prediction, which is to use past data to make estimations on the future, where the goal of the predictive process is to make as accurate estimations as possible. The second developmental path, which is less well studied, is causal inference. In domains such as healthcare analytics causal inference has been used for interpreting causes of change in an outcome variable of interest, and using this interpretation for planning case-specific interventions, which researchers in SCRM have not yet made full use of.
Although ML models hitherto proposed for SCRM can accurately predict whether a supply chain delay will occur and how long it might last, they are not effective in determining causes of delays, which is a prerequisite in mitigation planning. Current models that predict risk assume the onus of finding causality on the practitioner. As causality is unknown or at least subjectively attributed, once a delay is predicted, the practitioner can only plan for reactive steps that occur after the delay, rather than focus on preventing it through mitigation.
To address this issue, we propose complementing ML based delay prediction with causal inference, such that effective control can be implemented prior to the risk being manifested, when possible. We use the following problem setting for exploring this complementary avenue.
3.2 Problem setting
Our case study originates from the maritime engineering sector, where complex engineering assets are produced. The problem setting involves three warehouses, each with a historical dataset that includes previous transactions with their suppliers. 26% of suppliers are shared across the warehouses. The data encompasses orders delivered from 2015 to 2022, with all buyers located in the United Kingdom. Fig. 1 visualises the network of relationships between suppliers and buyers.

Note. Red suppliers are connected to all buyers (B1, B2, B3), blue suppliers to B1 and B2, cyan suppliers to B1 and B3, purple suppliers to B2 and B3, cyan suppliers only to B1, green suppliers only to B2, and gold suppliers only to B3.
A total of 77,526 orders have been given to 268 suppliers over this time period, with approximately 62% of the orders delayed (Table LABEL:tab1 ). Our aim is to create a causal model for creating effective interventions to prevent delivery delays at the order stage. We use the terms intervention and treatment interchangeably as the former is familiar to supply chain practitioners, whereas the latter is a standard term used in CML research.
Statistics | Buyer B1 | Buyer B2 | Buyer B3 |
---|---|---|---|
Delayed rate (%) | 56% | 51% | 68% |
On-Time rate (%) | 44% | 49% | 32% |
Maximum of delays (days) | 1669 | 2227 | 1070 |
Mean of delays (days) | 121.18 | 68.93 | 64.56 |
Standard deviation of delays (days) | 160.16 | 95.50 | 87.28 |
During the data preprocessing step, missing and duplicated values were removed. Outliers were kept to preserve information. Categorical variables were encoded using the one-hot encoding method.
To estimate the effectiveness of interventions, information about the following variables is necessary: the intervention of interest, the observed supply chain outcome and problem characteristics (covariates). For example, in delay prediction, one could use MRP records with information about order properties such as supplier selection, ordered products and quantities, requested delivery time (any of which could become the treatment), the deviation from expected arrival times (the observed outcome), and other variables relating to the order (the covariates).
In our case study, the outcome of interest is “Delay”, and treatment variables of interest that could be reasonably controlled by the buyers, are the season the order was given in and whether the supplier supplied to multiple warehouses. The latter decision was made due to a hypothesised effect that if a supplier had competing interests products were more likely to be delayed due to prioritisation. This was linked to the company’s interest to explore whether to pursue a unique sourcing policy for other orders.
Intervention and outcome variables, and covariates are detailed in Table LABEL:tab2 .
Feature Name | Data type | Description |
---|---|---|
Supplier | Categorical | Legal name of supplier. |
Project | Categorical | Name of the project that the ordered product is used for. |
Part Description | Categorical | Characteristic description of the ordered product. |
Quantity | Numerical | Quantity of the ordered product. |
Price | Numerical | Price for ordered product. |
Delay | Numerical | Duration of delay in days. |
Season | Categorical | Quarter of the year |
Multi | Binary | Depicts whether a supplier supplies to another buyer in the network (1 - supplier supplies to more than one buyer, 0 - supplier supply to only one buyer). |
3.3 Causal Graph Generation
To make causal quantities identifiable, we need to assume knowledge about the causal relationships, which can be depicted as directed acyclical graphs (DAGs). Based on the causal graph, causal effects, represented by directed edges, can be specified by a set of equations called structural equations. These equations can therefore be seen as a causal interpretation of DAGs, which additionally allow statements about the distribution of variables of interest under what-if scenarios.
Graphs in CML help the practitioner analyse cause and effect relationships \parencite Pearl2009Causality. DAGs are used to illustrate causal relations \parencite pearl1995causal, where nodes i and j represent two variables. Then node i causes j if a directed edge E[i,j] from i to j exists. In a DAG no loops are permitted. Nodes may be connected through a path showing steps of causality involved in a given outcome. Thus every edge in a causal graph indicates a direct or indirect causal effect or correlation through other nodes of the graph.
A DAG can provide an initial starting point for the CML process by ensuring that selected features accurately reflect the underlying causal structure, thereby facilitating a more accurate estimation of causal effects. Note that the resulting variables identified by the DAG help in selecting relevant features that are then input into the CML process.
DAGs in CML are usually built using domain knowledge to lay initial assumptions. Following this process in our case study with practitioners from the company resulted in Fig. 2 .

Variable “Multi” suggests that a supplier’s network relationships can significantly influence their operational priorities and capacities, which in turn affects other downstream variables, including “Delay”. The “Supplier” node not only affects “Part Description”, “Project”, and “Quantity”, but also has a direct impact on “Price” and “Delay”. This indicates that changes in the supplier’s situation can directly alter the cost of parts and the delivery schedule.
Furthermore, the graph illustrates that “Part Description” and “Project” also feed into “Delay”, underscoring how specific parts and their intended use in projects can complicate or expedite delivery timelines. “Season” emerges as another critical factor that indirectly impacts “Delay” through its influence on “Quantity” and “Price”. This reflects the seasonal variability in supply chain operations, where certain times of the year can affect availability, pricing, and ultimately, delivery schedules.
Even though the DAG in Fig. 2 illustrates some of the appropriate causal channels among the variables for use in CML, one might argue that it is subjective. To address this, we experiment with automatic DAG (autoDAG) generation to provide a more objective perspective. Fig. 3 shows automatically generated DAGs created by three algorithms: Hill Climbing, Tabu Search, and the Peter Clark (PC) algorithm. We detail their application next.
3.3.1 Hill Climbing
Let autoDAG be written as G = ( V , E ) 𝐺 𝑉 𝐸 G=(V,E) italic_G = ( italic_V , italic_E ) , where E 𝐸 E italic_E is a set of edges and V 𝑉 V italic_V is a set of variables defined as V = { X 1 , X 2 , … , X n } 𝑉 subscript 𝑋 1 subscript 𝑋 2 … subscript 𝑋 𝑛 V=\{X_{1},X_{2},\ldots,X_{n}\} italic_V = { italic_X start_POSTSUBSCRIPT 1 end_POSTSUBSCRIPT , italic_X start_POSTSUBSCRIPT 2 end_POSTSUBSCRIPT , … , italic_X start_POSTSUBSCRIPT italic_n end_POSTSUBSCRIPT } .
The Hill Climbing algorithm is a heuristic search method that can be used to determine the optimal structure of a Bayesian Network from data \parencite adhitama2022hill. Note that a Bayesian Network is a type of DAG, designed to visualise Bayesian probability theory. The algorithm’s objective function is the K2 score, which measures the compatibility of a graph structure G 𝐺 G italic_G with the observed data. The aim is to maximise the K2 score, thereby evaluating how well the network structure represents the dependencies in the data. Through a series of local modifications and evaluations, the algorithm converges on a graph that best fits the observed data \parencite cooper1992bayesian.
The Hill Climbing algorithm follows these steps:
Start with an initial graph G 0 = ( V , E 0 ) subscript 𝐺 0 𝑉 subscript 𝐸 0 G_{0}=(V,E_{0}) italic_G start_POSTSUBSCRIPT 0 end_POSTSUBSCRIPT = ( italic_V , italic_E start_POSTSUBSCRIPT 0 end_POSTSUBSCRIPT ) , where E 0 subscript 𝐸 0 E_{0} italic_E start_POSTSUBSCRIPT 0 end_POSTSUBSCRIPT can be a random set of edges or an empty set of edges.
Generate candidate graphs in each iteration, by making local changes to the current graph G t subscript 𝐺 𝑡 G_{t} italic_G start_POSTSUBSCRIPT italic_t end_POSTSUBSCRIPT . The possible changes include:
Adding an edge: G ′ = ( V , E t ∪ { ( X i → X j ) } ) superscript 𝐺 ′ 𝑉 subscript 𝐸 𝑡 → subscript 𝑋 𝑖 subscript 𝑋 𝑗 G^{\prime}=(V,E_{t}\cup\{(X_{i}\rightarrow X_{j})\}) italic_G start_POSTSUPERSCRIPT ′ end_POSTSUPERSCRIPT = ( italic_V , italic_E start_POSTSUBSCRIPT italic_t end_POSTSUBSCRIPT ∪ { ( italic_X start_POSTSUBSCRIPT italic_i end_POSTSUBSCRIPT → italic_X start_POSTSUBSCRIPT italic_j end_POSTSUBSCRIPT ) } )
Removing an edge: G ′ = ( V , E t ∖ { ( X i → X j ) } ) superscript 𝐺 ′ 𝑉 subscript 𝐸 𝑡 → subscript 𝑋 𝑖 subscript 𝑋 𝑗 G^{\prime}=(V,E_{t}\setminus\{(X_{i}\rightarrow X_{j})\}) italic_G start_POSTSUPERSCRIPT ′ end_POSTSUPERSCRIPT = ( italic_V , italic_E start_POSTSUBSCRIPT italic_t end_POSTSUBSCRIPT ∖ { ( italic_X start_POSTSUBSCRIPT italic_i end_POSTSUBSCRIPT → italic_X start_POSTSUBSCRIPT italic_j end_POSTSUBSCRIPT ) } )
Reversing an edge: G ′ = ( V , ( E t ∖ { ( X i → X j ) } ) ∪ { ( X j → X i ) } ) superscript 𝐺 ′ 𝑉 subscript 𝐸 𝑡 → subscript 𝑋 𝑖 subscript 𝑋 𝑗 → subscript 𝑋 𝑗 subscript 𝑋 𝑖 G^{\prime}=(V,(E_{t}\setminus\{(X_{i}\rightarrow X_{j})\})\cup\{(X_{j}% \rightarrow X_{i})\}) italic_G start_POSTSUPERSCRIPT ′ end_POSTSUPERSCRIPT = ( italic_V , ( italic_E start_POSTSUBSCRIPT italic_t end_POSTSUBSCRIPT ∖ { ( italic_X start_POSTSUBSCRIPT italic_i end_POSTSUBSCRIPT → italic_X start_POSTSUBSCRIPT italic_j end_POSTSUBSCRIPT ) } ) ∪ { ( italic_X start_POSTSUBSCRIPT italic_j end_POSTSUBSCRIPT → italic_X start_POSTSUBSCRIPT italic_i end_POSTSUBSCRIPT ) } )
For each candidate graph G ′ superscript 𝐺 ′ G^{\prime} italic_G start_POSTSUPERSCRIPT ′ end_POSTSUPERSCRIPT , calculate the K2 score.
Among the candidate graphs, select the one that maximises the K2 score. If the highest score for G ′ superscript 𝐺 ′ G^{\prime} italic_G start_POSTSUPERSCRIPT ′ end_POSTSUPERSCRIPT is greater than the current score for G t subscript 𝐺 𝑡 G_{t} italic_G start_POSTSUBSCRIPT italic_t end_POSTSUBSCRIPT , update G t subscript 𝐺 𝑡 G_{t} italic_G start_POSTSUBSCRIPT italic_t end_POSTSUBSCRIPT to G ′ superscript 𝐺 ′ G^{\prime} italic_G start_POSTSUPERSCRIPT ′ end_POSTSUPERSCRIPT .
Continue generating candidates, calculating K2 scores, and updating the graph until no further improvements can be made, indicating that a local maximum in the K2 score has been reached.
This approach optimises the search for new structures while exploiting promising ones to avoid overfitting DAGs. From Figure 3 a, it can be seen that our algorithm has performed quite well in constructing the domain knowledge DAG. However, it is important to note that the treatment variable “Multi” has no direct or indirect effect on any other variables affecting the outcome variable. This is monitored in subsequent DAG generations to decide on keeping the variable in the model.

3.3.2 Tabu Search
The Tabu Search algorithm is a metaheuristic optimisation technique aimed at solving combinatorial optimisation problems \parencite airoldi2006markov. Unlike other local search methods, Tabu Search employs a memory structure to avoid revisiting recently explored solutions, thus escaping local optima and enhancing the search process \parencite kobayashi2021selecting. In constructing a DAG from observational data, the primary goal is to identify a graph structure that maximises the Bayesian Information Criterion (BIC). BIC balances model fit and complexity by penalising models with excessive parameters to avoid overfitting \parencite zhou2023data.
The Tabu Search algorithm follows these steps:
Start with an initial graph G 0 = ( V , E 0 ) subscript 𝐺 0 𝑉 subscript 𝐸 0 G_{0}=(V,E_{0}) italic_G start_POSTSUBSCRIPT 0 end_POSTSUBSCRIPT = ( italic_V , italic_E start_POSTSUBSCRIPT 0 end_POSTSUBSCRIPT ) , where E 0 subscript 𝐸 0 E_{0} italic_E start_POSTSUBSCRIPT 0 end_POSTSUBSCRIPT is an empty set of edges (in our case, the edges from treatment variables to outcome variable were defined). Initialise a Tabu list to track recently explored solutions.
Create a neighbourhood of the current DAG by making local changes to generate candidate DAGs:
Calculate the BIC score for each candidate DAG G ′ superscript 𝐺 ′ G^{\prime} italic_G start_POSTSUPERSCRIPT ′ end_POSTSUPERSCRIPT .
Among the candidate DAGs G ′ superscript 𝐺 ′ G^{\prime} italic_G start_POSTSUPERSCRIPT ′ end_POSTSUPERSCRIPT , select the one that maximises BIC score as the new current DAG. Record the move that led to this DAG in the Tabu list to prevent immediate reversal.
Add the recent move to the Tabu list. Remove the oldest entries if necessary to keep the list manageable.
Allow exceptional moves that may otherwise be in the Tabu list if a candidate solution has a BIC score significantly better than the current best solution.
Repeat the process of neighbourhood search, BIC evaluation, candidate selection, and Tabu list updating until a stopping criterion is met. In our case, the stopping criterion is convergence to a solution where no significant improvement is observed.
After the stopping criterion is met, output the DAG with the highest BIC score encountered during the search.
This method, the results of which are shown in Fig. 3 b, is known to escape local optimal solutions and to thoroughly traverse through the solution space for capturing major conditional independencies and causal relationships in the data.
3.3.3 Peter Clark Algorithm
The PC algorithm was developed by Peter Spirtes and Clark Glymour \parencite spirtes1991. It is a general algorithm to generate DAGs based on observational data. The primary objective of the algorithm is to optimise the DAG structure to accurately represent the conditional interdependencies among variables in the data. This involves minimising false positives (incorrectly adding edges) and false negatives (incorrectly removing necessary edges). This process consists of two main stages:
Constructing an undirected graph;
Orienting the edges to form a DAG.
In the first step, the algorithm initialises a complete undirected graph G 𝐺 G italic_G on the set of variables V 𝑉 V italic_V \parencite spirtes1993. A complete graph means each pair of variables in V 𝑉 V italic_V is initially connected by an edge. The next step involves testing for conditional independence between pairs of variables. For each pair of variables ( X i , X j ) subscript 𝑋 𝑖 subscript 𝑋 𝑗 (X_{i},X_{j}) ( italic_X start_POSTSUBSCRIPT italic_i end_POSTSUBSCRIPT , italic_X start_POSTSUBSCRIPT italic_j end_POSTSUBSCRIPT ) , the algorithm checks if there exists a subset of variables S i j ⊆ V ∖ { X i , X j } subscript 𝑆 𝑖 𝑗 𝑉 subscript 𝑋 𝑖 subscript 𝑋 𝑗 S_{ij}\subseteq V\setminus\{X_{i},X_{j}\} italic_S start_POSTSUBSCRIPT italic_i italic_j end_POSTSUBSCRIPT ⊆ italic_V ∖ { italic_X start_POSTSUBSCRIPT italic_i end_POSTSUBSCRIPT , italic_X start_POSTSUBSCRIPT italic_j end_POSTSUBSCRIPT } such that X i subscript 𝑋 𝑖 X_{i} italic_X start_POSTSUBSCRIPT italic_i end_POSTSUBSCRIPT and X j subscript 𝑋 𝑗 X_{j} italic_X start_POSTSUBSCRIPT italic_j end_POSTSUBSCRIPT are conditionally independent given S i j subscript 𝑆 𝑖 𝑗 S_{ij} italic_S start_POSTSUBSCRIPT italic_i italic_j end_POSTSUBSCRIPT . If such a set S i j subscript 𝑆 𝑖 𝑗 S_{ij} italic_S start_POSTSUBSCRIPT italic_i italic_j end_POSTSUBSCRIPT is found, the edge ( X i , X j ) subscript 𝑋 𝑖 subscript 𝑋 𝑗 (X_{i},X_{j}) ( italic_X start_POSTSUBSCRIPT italic_i end_POSTSUBSCRIPT , italic_X start_POSTSUBSCRIPT italic_j end_POSTSUBSCRIPT ) is removed from the graph G 𝐺 G italic_G .
To test conditional independence, the algorithm starts with a graph where each variable is connected to every other variable. Using the Fisher z -test, it assesses whether the correlation between X i subscript 𝑋 𝑖 X_{i} italic_X start_POSTSUBSCRIPT italic_i end_POSTSUBSCRIPT and X j subscript 𝑋 𝑗 X_{j} italic_X start_POSTSUBSCRIPT italic_j end_POSTSUBSCRIPT , controlling for S i j subscript 𝑆 𝑖 𝑗 S_{ij} italic_S start_POSTSUBSCRIPT italic_i italic_j end_POSTSUBSCRIPT , is statistically different from zero. If the test indicates conditional independence, the edge ( X i , X j ) subscript 𝑋 𝑖 subscript 𝑋 𝑗 (X_{i},X_{j}) ( italic_X start_POSTSUBSCRIPT italic_i end_POSTSUBSCRIPT , italic_X start_POSTSUBSCRIPT italic_j end_POSTSUBSCRIPT ) is removed. This step is necessary in order to remove edges that represent indirect dependencies and simplify the graph.
Once the undirected graph G 𝐺 G italic_G is constructed, the next stage is to orient the edges to convert it into a DAG. This involves:
detecting v -structures: A v -structure occurs if there are two edges ( X i , X k ) subscript 𝑋 𝑖 subscript 𝑋 𝑘 (X_{i},X_{k}) ( italic_X start_POSTSUBSCRIPT italic_i end_POSTSUBSCRIPT , italic_X start_POSTSUBSCRIPT italic_k end_POSTSUBSCRIPT ) and ( X j , X k ) subscript 𝑋 𝑗 subscript 𝑋 𝑘 (X_{j},X_{k}) ( italic_X start_POSTSUBSCRIPT italic_j end_POSTSUBSCRIPT , italic_X start_POSTSUBSCRIPT italic_k end_POSTSUBSCRIPT ) such that X i subscript 𝑋 𝑖 X_{i} italic_X start_POSTSUBSCRIPT italic_i end_POSTSUBSCRIPT and X j subscript 𝑋 𝑗 X_{j} italic_X start_POSTSUBSCRIPT italic_j end_POSTSUBSCRIPT are not conditionally independent given any subset of the other variables. These edges are oriented towards the common node X k subscript 𝑋 𝑘 X_{k} italic_X start_POSTSUBSCRIPT italic_k end_POSTSUBSCRIPT , forming the structure X i → X k ← X j → subscript 𝑋 𝑖 subscript 𝑋 𝑘 ← subscript 𝑋 𝑗 X_{i}\rightarrow X_{k}\leftarrow X_{j} italic_X start_POSTSUBSCRIPT italic_i end_POSTSUBSCRIPT → italic_X start_POSTSUBSCRIPT italic_k end_POSTSUBSCRIPT ← italic_X start_POSTSUBSCRIPT italic_j end_POSTSUBSCRIPT .
propagating edge directions: The algorithm then applies a series of orientation rules to ensure no cycles are formed, maintaining the graph as acyclic. For example:
If X i → X j → subscript 𝑋 𝑖 subscript 𝑋 𝑗 X_{i}\rightarrow X_{j} italic_X start_POSTSUBSCRIPT italic_i end_POSTSUBSCRIPT → italic_X start_POSTSUBSCRIPT italic_j end_POSTSUBSCRIPT and X j − X k subscript 𝑋 𝑗 subscript 𝑋 𝑘 X_{j}-X_{k} italic_X start_POSTSUBSCRIPT italic_j end_POSTSUBSCRIPT - italic_X start_POSTSUBSCRIPT italic_k end_POSTSUBSCRIPT , then X j → X k → subscript 𝑋 𝑗 subscript 𝑋 𝑘 X_{j}\rightarrow X_{k} italic_X start_POSTSUBSCRIPT italic_j end_POSTSUBSCRIPT → italic_X start_POSTSUBSCRIPT italic_k end_POSTSUBSCRIPT to prevent the cycle X i → X j → X k → subscript 𝑋 𝑖 subscript 𝑋 𝑗 → subscript 𝑋 𝑘 X_{i}\rightarrow X_{j}\rightarrow X_{k} italic_X start_POSTSUBSCRIPT italic_i end_POSTSUBSCRIPT → italic_X start_POSTSUBSCRIPT italic_j end_POSTSUBSCRIPT → italic_X start_POSTSUBSCRIPT italic_k end_POSTSUBSCRIPT .
If X i − X j subscript 𝑋 𝑖 subscript 𝑋 𝑗 X_{i}-X_{j} italic_X start_POSTSUBSCRIPT italic_i end_POSTSUBSCRIPT - italic_X start_POSTSUBSCRIPT italic_j end_POSTSUBSCRIPT and there is a path X i → X k → X j → subscript 𝑋 𝑖 subscript 𝑋 𝑘 → subscript 𝑋 𝑗 X_{i}\rightarrow X_{k}\rightarrow X_{j} italic_X start_POSTSUBSCRIPT italic_i end_POSTSUBSCRIPT → italic_X start_POSTSUBSCRIPT italic_k end_POSTSUBSCRIPT → italic_X start_POSTSUBSCRIPT italic_j end_POSTSUBSCRIPT , then X i → X j → subscript 𝑋 𝑖 subscript 𝑋 𝑗 X_{i}\rightarrow X_{j} italic_X start_POSTSUBSCRIPT italic_i end_POSTSUBSCRIPT → italic_X start_POSTSUBSCRIPT italic_j end_POSTSUBSCRIPT .
These rules are applied iteratively until all edges are correctly oriented without forming any cycles. By following these steps, the PC algorithm constructs a DAG that captures the causal structure underpinning the observed data, providing valuable insights into the relationships between variables.
Observing autoDAGs created by the PC algorithm and Tabu Search we see a reflection of the cause and effect relationships between variables in our case study. Despite some differences between the graphs, they can still be rationally explained. For instance, the Tabu Search graph shows a direct influence of “Season” on “Quantity”. This is logical because seasonality can significantly affect the quantity of products ordered (e.g, product availability). On the other hand, the PC algorithm graph does not show this direct influence. Instead, we see that “Quantity” affects “Project”. In real world scenarios, this is plausible because the quantity of parts needed for a project is often predefined. Therefore, seasonality may not directly impact the quantity ordered for a project, as the requirements are already established based on project specifications and timelines.
This example of differences shows that despite the different algorithmic approaches, both graphs can be rationally explained based on domain knowledge. The autoDAGs confirm the appropriate causal channels among the variables for use in CML.
3.4 Development of the Interactive Regression Model
As mentioned earlier, CML involves the application of a suitable ML model through a DML framework to estimate the relationship between the outcome variable and treatment as well as covariates. In our case study, decisions about mitigating delivery risk are based on binary characteristics X 𝑋 X italic_X , such as supplier selection. We thus choose an Interactive Regression Model (IRM) that can handle such a form of treatment. IRM takes the following form \parencite chernozhukov2018double:
(1) | ||||
where Y 𝑌 Y italic_Y is the outcome variable and D 𝐷 D italic_D is the binary and heterogeneous treatment. X 𝑋 X italic_X represents a high-dimensional vector of confounding covariates ( X 1 , … , X p ) subscript 𝑋 1 … subscript 𝑋 𝑝 (X_{1},\ldots,X_{p}) ( italic_X start_POSTSUBSCRIPT 1 end_POSTSUBSCRIPT , … , italic_X start_POSTSUBSCRIPT italic_p end_POSTSUBSCRIPT ) . U 𝑈 U italic_U and V 𝑉 V italic_V signify stochastic errors \parencite Bach_2022_JMLR. Conditional expectations \parencite chernozhukov2018double are as follows:
(2) | ||||
These are unknown and might be complex functions of X 𝑋 X italic_X . In this model, the target parameter of interest are the average treatment effect, known as ATE \parencite schacht2023causally:
(3) |
ATE conceptualises potential outcomes, which are the outcomes that would hypothetically be observed if a certain intervention was applied. ATE measures effects at the level of the study sample. By comparing the average outcome for cases receiving the intervention versus those which do not (control group), ATE helps in understanding how effective a treatment is, on average, across a specific sample grouping. In other words, ATE measures the mean difference in outcomes between units assigned to the treatment and those assigned to the control across the entire population \parencite pirracchio2016propensity.
In the case of IRM, the score for an ATE estimator is given by the linear formula \parencite schacht2023causally:
(4) | ||||
For a more granular view, we can estimate the conditional average treatment effect (CATE), which is the effect of an intervention for a particular subgroup of samples defined by the covariates. Understanding the heterogeneity in intervention effects can inform about subgroups of cases where interventions are not effective, and is relevant for individualizing interventions for specific cases. CATEs are the average effect of a treatment on an outcome for a specific subgroup within the population \parencite jacob2021cate. The IRM model also offers the ability to calculate CATE as:
(5) |
where X ~ ~ 𝑋 \tilde{X} over~ start_ARG italic_X end_ARG represents a set of covariates that are not necessarily included in X 𝑋 X italic_X \parencite semenova2021.
Finally, the IRM model can be used to estimate deterministic binary treatment policies using classification trees \parencite bach2024doubleml. Note that a deterministic binary treatment policy refers to a decision-making rule that assigns one of the two possible treatments based on observable characteristics. The policy estimation process is informed by the components ψ b ( W i , η ) subscript 𝜓 𝑏 subscript 𝑊 𝑖 𝜂 \psi_{b}\left(W_{i},\eta\right) italic_ψ start_POSTSUBSCRIPT italic_b end_POSTSUBSCRIPT ( italic_W start_POSTSUBSCRIPT italic_i end_POSTSUBSCRIPT , italic_η ) in equation 4 \parencite athey2021policy. \textcite athey2021policy proposed to estimate the treatment assignment rule as:
(6) |
where the weights λ i subscript 𝜆 𝑖 \lambda_{i} italic_λ start_POSTSUBSCRIPT italic_i end_POSTSUBSCRIPT are defined as | ψ b ( W i , η ^ ) | subscript 𝜓 𝑏 subscript 𝑊 𝑖 ^ 𝜂 |\psi_{b}(W_{i},\hat{\eta})| | italic_ψ start_POSTSUBSCRIPT italic_b end_POSTSUBSCRIPT ( italic_W start_POSTSUBSCRIPT italic_i end_POSTSUBSCRIPT , over^ start_ARG italic_η end_ARG ) | and the target H i subscript 𝐻 𝑖 H_{i} italic_H start_POSTSUBSCRIPT italic_i end_POSTSUBSCRIPT is the sign of ψ b ( W i , η ^ ) subscript 𝜓 𝑏 subscript 𝑊 𝑖 ^ 𝜂 \psi_{b}(W_{i},\hat{\eta}) italic_ψ start_POSTSUBSCRIPT italic_b end_POSTSUBSCRIPT ( italic_W start_POSTSUBSCRIPT italic_i end_POSTSUBSCRIPT , over^ start_ARG italic_η end_ARG ) . This method aligns with creating a decision framework that emphasises the weight and direction indicated by the treatment effect estimations ψ b subscript 𝜓 𝑏 \psi_{b} italic_ψ start_POSTSUBSCRIPT italic_b end_POSTSUBSCRIPT \parencite athey2021policy.
4 Experimental evaluation
4.1 experimental setup.
Experiments were conducted using Python on an Apple Macbook M1 with 8GB RAM. XGBoost was employed as the main classifier and regressor for training the IRM model. By employing XGBRegressor and XGBClassifier, the relationships between variables can be estimated in a highly flexible manner, avoiding the imposition of rigid assumptions on the functional forms of g 𝑔 g italic_g and m 𝑚 m italic_m . This flexibility is crucial for capturing complex patterns and interactions within the data, significantly enhancing the reliability and accuracy of the estimation process.
To identify the optimal hyperparameters, we utilised GridSearchCV within scikit-learn version 1.4.2 \parencite scikit-learn , which systematically iterates through multiple combinations of parameter settings, performing cross-validation to determine the best performance configuration. The default 5-fold cross-validation was used.
We used the package DoubleML which is built on top scikit-learn and implements DML and provides estimation of causal effects in different models e.g., the partially linear regression model (PRL), the partially linear instrumental variable regression model (PLIV), the interactive regression model (IRM), and the interactive instrumental variable regression model (IIVM).
4.2 Results
ATE estimates are presented in Table LABEL:tab3 . As ATE provides population level patterns, we can interpret the result as follows. When a buyer is purchasing from a supplier that is connected to another warehouse, the duration of the delay will increase on average by approximately 17 days. This result is statistically significant based on p-values and t-statistics. Hence, the original hypothesis on multiple warehouses being served by the supplier contributing to a delay cause can be considered correct. Note that even if we have actually noted this as causality, the reasons behind this still need to be determined. Interpreting results through causal links does not always equate to fully explaining the reasoning and causal path behind an outcome, since interpretability and explainability do not necessarily overlap in the context of AI \parencite Antoniou2022A. As appreciated by the practitioner, the effect might indeed be due to an inherent prioritisation on the side of the supplier, but it may also be due to the supplier batching production, e.g. due to set up and delivery costs involved, or even as supplier has exceeded capacity due to multiple requests. The actual root causes of supplier behaviour and potential solutions need to be explained through discussions with the supplier.
Treatment | Coef | t-statistics | P-value | Std error |
---|---|---|---|---|
Multi | 16.74 | 17.59 | 2.96 10 | 0.534 |
First quarter | -5.90 | -9.72 | 2.38 10 | 0.662 |
Second quarter | 8.22 | 13.13 | 2.14 10 | 0.607 |
Third quarter | 10.12 | 16.20 | 4.71 10 | 0.624 |
Fourth quarter | -18.70 | -27.97 | 3.49 10 | 0.669 |
Another observation is that buying parts in the second and third quarter will increase the delay by 8 and 10 days, respectively. In contrast, buying products during the first and fourth quarters will decrease the delay by approximately 6 and 19 days, respectively. These seasonal variations are quite significant. Seasonal order placement therefore needs to play an important role in scheduling deliveries from the supplier which may in turn impact the buyers’ own production and delivery schedules.
These results based on ATE and supported by the DAG analysis, provide a clear understanding of the causal factors affecting delivery delays. They underscore the importance of considering both the supplier’s network and seasonal timing when planning orders, thus enabling more strategic decision-making to mitigate risks in the supply chain.
An analysis using CATE provides a more granular interpretation based on order specifics. Fig. 4 represents one-dimensional CATEs that depend on the covariate “Quantity”. To estimate the effect, we used a B-splines basis with 5 degrees of freedom. Fig. 4 a shows that for small quantities, the effect is uncertain and highly variable. For quantities up to 100-200, the effect increases. For quantities greater than 200, the effect becomes constant, though there is more uncertainty for higher quantities. In the first, second, and third quarters, we can see that the effect decreases and then becomes constant, with increased uncertainty. In contrast, the effect in the fourth quarter increases and then also becomes constant, but there is less uncertainty compared to other quarters.
By estimating the CATEs, we gain insights into how specific covariates, such as the order quantity, influence the effect of interventions across different scenarios. This complements the ATE analysis by revealing heterogeneity in treatment effects and highlighting the importance of tailoring interventions to specific contexts. Through this approach, supply chain practitioners can better understand the nuances of how different factors interact and affect outcomes, thereby improving the precision and effectiveness of their decision-making processes.
Next, we estimated deterministic binary treatment policies. Fig. 5 represents policy trees for treatment variables. Starting with the “Multi” variables, we observe in Fig. 5 a that the first split is based on the status of Project 15. Then, if a practitioner is ordering for Project 15 and the price of the order is less than or equal to 0.015 (normalised), we should use suppliers connected to only one warehouse for this part. In contrast, when the price is greater than 0.015, we could consider suppliers that serve multiple warehouses. However, if supplier S176 is used, deliveries should be supplied by suppliers connected to only one warehouse.
Fig. 5 b shows the policy tree for the treatment “First quarter”, with splits based on the usage of suppliers S103 and S69. In the case of the second, third, and fourth quarters, there is only one split based on the status of Project 6 and Supplier S103. Note that in the case of the “Fourth quarter”, the policy tree suggests that Supplier S103 should not be used during this time, whereas, during the second quarter of the year, this particular supplier should be used exclusively.

5 Conclusions & managerial implications
Causal machine learning in supply chain management represents a significant advancement in tackling the complexities and challenges of modern businesses. Unlike traditional ML models that primarily focus on correlations with the primary aim of maximising predictive accuracy, this approach delves into the causal relationship between control variables and a change in an outcome. Through this process, CML allows policies to be drawn from data, in order to influence a predicted outcome variable.
In this paper we illustrated the application of CML in the context of supply chain risk management through an empirical case study in the maritime engineering sector, to predict and mitigate supply chain risk, in relation to delivery delays.
Our results indicate the significant potential of using CML to generate effective interventions for minimising delays. For example, we found that buyers who utilise suppliers that serve multiple warehouses experience longer delivery delays compared to those who use suppliers dedicated to a single buyer and that the season in which an order is placed strongly impacts upon its potential delay. Such insights then led to designing specific policies for suppliers, using control variables to mitigate risks.
The use of DAGs played a crucial role in our study as a means to explicitly formulate causal problems. DAGs define causal relationships between variables and warranty that our approach is correctly structured in understanding how different factors influence supply chain delays. The graphical representation through DAGs also assists in identifying and focusing on the most relevant causal pathways for maximal intervention impact. To complement the subjective insights gained from practitioner knowledge, we also employed automated DAG generation methods such as Hill Climbing, Tabu Search and PC algorithm. These balance out the subjective perspective of expert knowledge by removing bias and acting as a means to validate initial assumptions. This dual approach enhances the strength and robustness of causal inferences and interventions.
Implementing CML for understanding delivery delays has important managerial implications as it can lead to improved decision-making, enhanced efficiency, and reduced costs. By identifying and leveraging causal relationships, managers can develop more targeted and effective strategies to address specific issues within the supply chain. CML should be used in tandem with traditional ML whereby traditional ML allows for accurate forecasting whereas CML allows for effective interventions by changing causal parameters. This complementary approach ensures that businesses can not only predict potential disruptions but also also apply targeted interventions to mitigate their impact, thereby enhancing the overall performance of supply chain, and thus its competitive advantage.
Our study has limitations that open up avenues for further research. Primarily, the case-study nature of our research limits the applicability of our findings to other sectors, suggesting a need for additional research to validate the effectiveness of CML across different industrial contexts. Applying CML to other SCRM-related issues, such as inventory management, predictive maintenance and quality control would be particularly noteworthy as these areas provide ample space for interventions given the availability of rich datasets.
Causal Federated Learning could provide another promising avenue for future research, where actions of multiple self-interested but interdependent agents (firms) in a supply chain can be considered within a CML framework to help identify supply chain failures in a privacy preserving manner. This method could facilitate collaborative yet secure data analysis across different entities, potentially uncovering systemic inefficiencies and opportunities for interventions.
Concluding, the adoption of causal machine learning in supply chain risk management holds the potential to revolutionise the field by providing deeper insights and more precise interventions. As businesses continue to face increasingly complex issues affecting supply chains, the integration of CML can drive significant advancements in efficiency, resilience, durability, and overall performance. Further exploration and validation of CML across diverse industrial contexts will be critical to unlocking its full potential and fostering innovation in supply chain risk management.
Acknowledgments
This research has been supported by funds granted by the Minister of Science of the Republic of Poland under the “Regional Initiative for Excellence” Programme for the implementation of the project “The Poznań University of Economics and Business for Economy 5.0: Regional Initiative – Global Effects (RIGE)”
[title=References, heading=bibintoc]
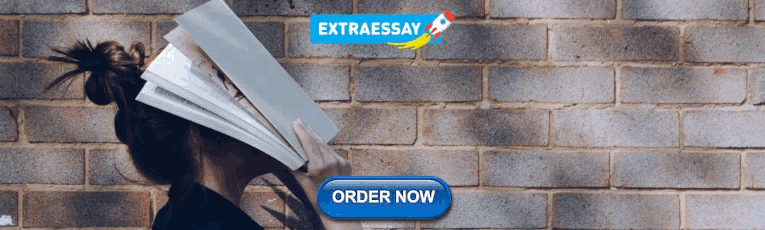
IMAGES
COMMENTS
2.1 Tourism Supply Chain. A tourism supply chain is a network of organizations, both public and private, engaged in activities ranging from flights and accommodation to the distribution and marketing of the final tourism product at a specific destination (Zhang et al. 2009 ). A typical tourism supply chain involves suppliers, tour operators ...
The uncertainty environment brought about by COVID-19 has broken the original stable hierarchical network structure and the whole supply and demand system of the tourism supply chain (TSC), which has brought heavy losses to the tourism industry. Using the case research and consensual qualitative research (CQR) method, taking the real situation and perception of individual supply chain members ...
ABSTRACT. The tourism industry was seen as one of the main beneficiaries of globalisation prior to the outbreak of the Covid-19 pandemic. However, while tourism is a major driver of economic growth and social well-being, a transformation of the industry towards more sustainable practices along the tourism supply chain (TSC) is needed to ensure that sustainable development goals can be reached.
Tourism is an important driver for global development. Ensure the sustainability and the performance of regional tourism industry is vital to the sustainable development. The aim of this research is to study the sustainability of tourism supply chain of Palmela village using a case study research. The paper has two parts, the first part ...
However, since a full supply chain is composed of horizontal, vertical and diagonal suppliers, there is a gap in literature regarding the dynamics of how these triadic relationships operate within a tourism supply chain. Using a multiple case study design involving four tour operators in Macau, this paper explores the interplay of relationships ...
This chapter aims to analyze. the process in which a successful startup redesigned the tourism supply chain in. multiple emerging Asian economies to redistrib ute greater bene ts to disadvantaged ...
aintaining a small team and ensuring the local people were offered a good deal.Earlier in the chapter, we reviewed seven critical issues in tourism supply chain management identified in Zhang et ...
The dynamic environment and the globalization of the tourism sector accelerate the necessity to improve sustainable supply chain management. In tourism sector the supply chain is composed by a diversity of firms with higher heterogeneity and in
Findings. The results show that existing empirical studies have adopted four types of logic (supply logic, destination logic, global value chain and tourism global value chain), which complement each other in explaining the entire concept of TVC.
The dynamic environment and the globalization of the tourism sector accelerate the necessity to improve sustainable supply chain management. In tourism sector the supply chain is composed by a diversity of firms with higher heterogeneity and in general without trade alliances. These circumstances improve the complexity of this chain and difficult the study of the sector.
This study will evaluate rural tourism supply chain with Data Envelopment Analysis (DEA) in Mesr village, Iran, in 2015 and will offer solutions in way of improvement and enrichment of its ...
Using case study research methodology, this study interviewed 13 hotel managers in Akaroa, a famous tourist destination ... key management issues in tourism supply chains: supply chain coordination, supply management, two party relationships, information technology, demand management, inventory management, and product
Tourism supply chains involve many components: accommodation, transport, excursions, bars and restaurants, handicrafts, food production, waste disposal, and the infrastructure that supports tourism within chosen destinations. The importance of one such component, transport, for the efficiency of the tourism supply chain is precisely the topic ...
lay a central role (Spasić & Pavlović 2015). In light of these issues, the integration of sustainability into supply chain management (SCM) of DMCs is imperative to the sustainable growth of tou. m at the destination (Swarbrooke 2005, 235). However, there has not been much research effort, especially in the last decade, devoted to extensively ...
This study explores the concept and practical application of green supply chains within ecotourism and rural tourism, drawing valuable insights from X Town, China. Here, we redefine ecotourism as encompassing natural and human ecological systems, recognizing its increasing popularity. Notably, we present a green supply chain model for rural tourism, functioning as a comprehensive management ...
In the post-COVID-19 era, the tourism industry needs to focus on green transformation and new technologies to transform TSC, supply chain management and B2B relationships. It also needs to ensure employee retention and policies to attract new talent.,This study provides a comprehensive, objective and integrative overview of the evolution of TSC.
Tourism supply chain. The major SC activity of tour operators is the bulk purchase of products (e.g. accommodation, transportation, excursions), their bundling in tour packages and the later's distribution-sale in a single package price that is lower than the prices' sum of the package's products. ... A case study of green supply-chain ...
Page (2003) points out that the provision of tourism products and services involves a wide range of interrelated tourism suppliers, and plots a structure of a tourism supply chain. Descriptive studies of tourism supply chains include those of Alford, 2005, Scavarda et al., 2001, Tapper and Font, 2004, and Yilmaz and Bititci (2006).
"Environmental Supply Chain Management in Tourism: The Case of Large Tour Operators." Journal of Cleaner Production, 17 (16): 1385-92. Crossref. Web of Science ... Racherla P. (2011). "Assessing the Value of Collaborations in Tourism Networks: A Case Study of Elkhart County, Indiana." Journal of Travel and Tourism Marketing, 28 (1): 97 ...
The purpose of the present paper is to identify the processes for assessing tourism supply chain performance and study the performance of the supply chain of rural tourism resorts in central Mazandaran. Performance assessment components were categorized according to the six SCOR model processes, and a decision tree was designed.
This exploratory study examines the importance of firm-supply chain relationships for tourism development. Based upon prior observations, two firms seeking to boost their tourism offerings and operating in a popular Vietnamese tourist destination were selected.
future studies of TSCM, and Section 6 concludes the study. 2 Supply chain management in tourism 2.1 Overview of supply chain management The fierce global competition in the 21st century is focused on supply chains rather than on individual companies. From a macro perspective, a supply chain is a network of
The effort has been made to capture specific sustainability practices across the supply chain. The paper reinstates the fact that sustainability practices are not firm specific and should be practiced at the supply chain interface. The data for the study were taken from focal organizations perspective which is the hotels.
2 Nissan Supply Chain Operations Recovery Case Study In March 2011, Japan was hit by one of the most severe catastrophes in its nation when a 9.0 magnitude earthquake, a tsunami, and a nuclear crisis hit the nation. This disaster came with tremendous losses for the country, adversely affecting all levels of society and industrial sectors. The automobile sector was among the sectors that were ...
Globalisation has significantly impacted the food supply chain (FSC) landscape by presenting both challenges and opportunities for sustainable management [1,2].The FSC faces increasing pressure to reduce waste, enhance security, and ensure health and safety for practitioners and consumers [3,4].The 2024 edition of The State of Food Security and Nutrition in the World report presents crucial ...
In this article we outline the key steps in developing CML for supply chain intervention models, using a case study in supply chain risk management (SCRM) in the maritime engineering sector. Our hope is that doing so presents an illustrative case that can motivate the use of CML in SCRM tasks where optimal interventions are required. We then ...