
- Accepted Papers
ACM SIGKDD is pleased to announce the winners of the best paper awards for 2017.
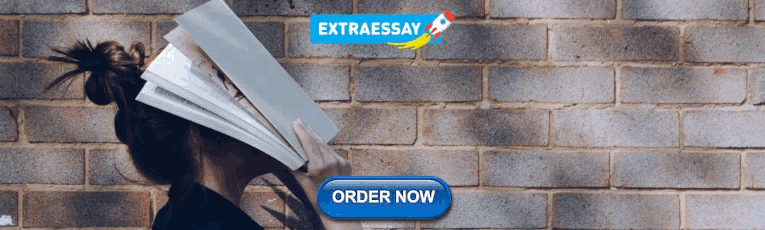
Research Track
BEST PAPER AWARD
Accelerating Innovation Through Analogy Mining Tom Hope (Hebrew University of Jerusalem);Joel Chan (Carnegie Mellon University);Aniket Kittur (Carnegie Mellon University);Dafna Shahaf (Hebrew University of Jerusalem)
Toeplitz Inverse Covariance-Based Clustering of Multivariate Time Series Data David Hallac (Stanford University);Sagar Vare (Stanford University);Stephen Boyd (Stanford University);Jure Leskovec (Stanford University)
Best Reviewers
- Karthik Raman, Google
- Kevin Small, Amazon
- Zoran Obradovic, Temple University
- Pauli Miettinen, Max Planck Institute
Applied Data Science Track
HinDroid: An Intelligent Android Malware Detection System Based on Structured Heterogeneous Information Network Yanfang Ye (West Virginia University);Shifu Hou (West Virginia University);Yangqiu Song (West Virginia University)
DeepSD: Generating High Resolution Climate Change Projections through Single Image Super-Resolution Thomas Vandal (Northeastern University);Evan Kodra (risQ Inc.);Sangram Ganguly (Bay Area Environmental Research Institute / NASA Ames Research Center);Andrew Michaelis (University Corporation, Monterey Bay);Ramakrishna Nemani (NASA Ames Research Center);Auroop Ganguly (Northeastern University)
- Charalampos Mavroforakis, Boston University
- Noam Koenigstein, Microsoft
- Alexandros Ntoulas, LinkedIn
Audience Appreciation Award
An efficient bandit algorithm for realtime multivariate optimization Daniel Hill (Amazon.com);Houssam Nassif (Amazon.com);Yi Liu (Amazon.com);Anand Iyer (Amazon.com);S. V. N. Vishwanathan (Amazon.com)
RESEARCH TRACK PAPERS - ORAL
Title & Authors |
---|
|
|
|
|
|
|
|
|
|
|
|
|
|
|
|
|
|
|
|
|
|
|
|
|
|
|
|
|
|
|
|
|
|
|
|
|
|
|
|
|
|
|
|
|
|
|
|
|
|
|
|
|
|
|
|
|
|
|
|
|
|
|
|
|
RESEARCH TRACK PAPERS - POSTER
Title & Authors |
---|
|
|
|
|
|
|
|
|
|
|
|
|
|
|
|
|
|
|
|
|
|
|
|
|
|
|
|
|
|
|
|
|
|
|
|
|
|
|
|
|
|
|
|
|
|
|
|
|
|
|
|
|
|
|
|
|
|
|
|
|
|
|
|
|
|
|
APPLIED DATA SCIENCE TRACK PAPERS - ORAL
Title & Authors |
---|
|
|
|
|
|
|
|
|
|
|
|
|
|
|
|
|
|
|
|
|
|
|
|
|
|
|
|
|
|
|
|
|
|
|
|
|
APPLIED DATA SCIENCE TRACK PAPERS - POSTER
Title & Authors |
---|
|
|
|
|
|
|
|
|
|
|
|
|
|
|
|
|
|
|
|
|
|
|
|
|
|
|
|
|
|
|
|
|
|
|
|
|
|
|
|
|
|
|
|
|
|
|
|
|
|
|
Poster Session Maps
Important dates.
- Camera Ready Deadline June 9, 2017
- Startup Grant Deadline June 16, 2017
- Student Grants Deadline June 17, 2017
- Promotional Video Deadline June 18, 2017
- Tutorials August 13, 2017
- Workshops August 14, 2017
- Main Conference August 15 - 17, 2017
- Keynote Speakers
- Applied Data Science Invited Panels
- Applied Data Science Invited Talks
- Plenary Panel
- Conventional Tutorials
- Hands-On Tutorials
- Meet the Editors Panel
- Networking With Experts
For any questions regarding registration, please email the Registration Manager, Brooke Daley ( [email protected] )
Save the Date
Data Mining of Project Management Data: An Analysis of Applied Research Studies
New citation alert added.
This alert has been successfully added and will be sent to:
You will be notified whenever a record that you have chosen has been cited.
To manage your alert preferences, click on the button below.
New Citation Alert!
Please log in to your account
Information & Contributors
Bibliometrics & citations, index terms.
Applied computing
Operations research
Industry and manufacturing
Information systems
Information systems applications
Data mining
Association rules
Enterprise information systems
Enterprise resource planning
Recommendations
Mining fuzzy specific rare itemsets for education data.
Association rule mining is an important data analysis method for the discovery of associations within data. There have been many studies focused on finding fuzzy association rules from transaction databases. Unfortunately, in the real world, one may ...
Mining uncertain data for constrained frequent sets
Data mining aims to search for implicit, previously unknown, and potentially useful pieces of information---such as sets of items that are frequently co-occurring together---that are embedded in data. The mined frequent sets can be used in the discovery ...
TCOM, an innovative data structure for mining association rules among infrequent items
Association rule mining is one of the most important areas in data mining, which has received a great deal of attention. The purpose of association rule mining is the discovery of association relationships or correlations among a set of items. In this ...
Information
Published in.

In-Cooperation
- Kyoto University: Kyoto University
- Nanyang Technological University
Association for Computing Machinery
New York, NY, United States
Publication History
Permissions, check for updates, author tags.
- Business Analytics
- Data Mining
- Industrial Applications
- Project Management
- Research-article
- Refereed limited
Contributors
Other metrics, bibliometrics, article metrics.
- 0 Total Citations
- 169 Total Downloads
- Downloads (Last 12 months) 8
- Downloads (Last 6 weeks) 0
View Options
Login options.
Check if you have access through your login credentials or your institution to get full access on this article.
Full Access
View options.
View or Download as a PDF file.
View online with eReader .
Share this Publication link
Copying failed.
Share on social media
Affiliations, export citations.
- Please download or close your previous search result export first before starting a new bulk export. Preview is not available. By clicking download, a status dialog will open to start the export process. The process may take a few minutes but once it finishes a file will be downloadable from your browser. You may continue to browse the DL while the export process is in progress. Download
- Download citation
- Copy citation
We are preparing your search results for download ...
We will inform you here when the file is ready.
Your file of search results citations is now ready.
Your search export query has expired. Please try again.
Grab your spot at the free arXiv Accessibility Forum
Help | Advanced Search
Statistics > Other Statistics
Title: data-mining research in education.
Abstract: As an interdisciplinary discipline, data mining (DM) is popular in education area especially when examining students' learning performances. It focuses on analyzing educational related data to develop models for improving learners' learning experiences and enhancing institutional effectiveness. Therefore, DM does help education institutions provide high-quality education for its learners. Applying data mining in education also known as educational data mining (EDM), which enables to better understand how students learn and identify how improve educational outcomes. Present paper is designed to justify the capabilities of data mining approaches in the filed of education. The latest trends on EDM research are introduced in this review. Several specific algorithms, methods, applications and gaps in the current literature and future insights are discussed here.
Comments: | 5 pages |
Subjects: | Other Statistics (stat.OT); Computers and Society (cs.CY) |
Cite as: | [stat.OT] |
(or [stat.OT] for this version) | |
Focus to learn more arXiv-issued DOI via DataCite |
Submission history
Access paper:.
- Other Formats
References & Citations
- Google Scholar
- Semantic Scholar
BibTeX formatted citation

Bibliographic and Citation Tools
Code, data and media associated with this article, recommenders and search tools.
- Institution
arXivLabs: experimental projects with community collaborators
arXivLabs is a framework that allows collaborators to develop and share new arXiv features directly on our website.
Both individuals and organizations that work with arXivLabs have embraced and accepted our values of openness, community, excellence, and user data privacy. arXiv is committed to these values and only works with partners that adhere to them.
Have an idea for a project that will add value for arXiv's community? Learn more about arXivLabs .
Recent advances in domain-driven data mining
- Published: 27 December 2022
- Volume 15 , pages 1–7, ( 2023 )
Cite this article
- Chuanren Liu 1 ,
- Ehsan Fakharizadi 2 ,
- Tong Xu 3 &
- Philip S. Yu 4
3070 Accesses
2 Citations
1 Altmetric
Explore all metrics
Data mining research has been significantly motivated by and benefited from real-world applications in novel domains. This special issue was proposed and edited to draw attention to domain-driven data mining and disseminate research in foundations, frameworks, and applications for data-driven and actionable knowledge discovery. Along with this special issue, we also organized a related workshop to continue the previous efforts on promoting advances in domain-driven data mining. This editorial report will first summarize the selected papers in the special issue, then discuss various industrial trends in the context of the selected papers, and finally document the keynote talks presented by the workshop. Although many scholars have made prominent contributions with the theme of domain-driven data mining, there are still various new research problems and challenges calling for more research investigations in the future. We hope this special issue is helpful for scholars working along this critically important line of research.
Explore related subjects
- Artificial Intelligence
Avoid common mistakes on your manuscript.
1 Summary of research contributions
Data mining has been a trending research area with contributions from diverse communities including computer scientists, statisticians, mathematicians, as well as other researchers and engineers working on data-intensive problems. While many researchers focus on general data mining methodologies for standardized problem settings, such as unsupervised learning and supervised learning, applying general solutions to specific problems may still be a nontrivial challenge. This is mainly due to the need to incorporate domain knowledge in implementing data mining solutions for novel real-world applications. Oftentimes standardized solutions must be significantly revised to accommodate unique characteristics of input data and deliver actionable results in novel application domains. Essentially, data mining research is highly applied. Many classic research problems are motivated by real-world applications and results of data mining research are expected to provide practical implications to business managers, government agencies, and all members of our society.
1.1 Overview of domain-driven data mining
Domain-driven data mining aims to bridge the gaps between theoretical research and practical applications in data mining and transform data intelligence to business value and impact [ 11 , 12 ]. Domain-driven data mining has been proposed as a research framework for discovering actionable knowledge and intelligence in a complex environment to directly transform data to decisions or enable decision-making actions [ 3 , 16 ].
Domain-driven data mining handles ubiquitous X-complexities and X-intelligences surrounding domain-driven actionable intelligence discovery. Examples of X-complexities and X-intelligences are related to domain complexity and intelligence, data complexity and intelligence, behavior complexity and intelligence, network complexity and intelligence, social complexity and intelligence, organizational complexity and intelligence, human complexity and intelligence, and their integration and meta-synthesis [ 8 , 16 ]. Analyzing and learning X-complexities and X-intelligences result in X-analytics [ 8 ] in various domains and on specific purposes. Examples are business analytics, behavior analytics, social analytics, operational analytics, risk analytics, customer analytics, insurance analytics, learning analytics, cybersecurity analytics, and financial analytics [ 15 , 21 , 24 , 26 , 28 , 29 , 31 , 38 , 40 , 41 , 42 , 43 , 51 ]. One prominent example of learning data complexities for in-depth data intelligence is the research on non-IID learning, which learns interactions and couplings (including correlation and dependency) involved in heterogeneous data, behaviors, and systems. Non-IID learning is applicable to many real-world applications such as non-IID outlier detection, non-IID recommendation, non-IID multimedia and multimodal analytics, and non-IID federated learning [ 5 , 6 , 17 ].
Domain-driven data mining also handles typical research issues and gaps in existing body of knowledge for domain-driven and actionable intelligence delivery. The research on domain-driven actionable intelligence discovery includes but is not limited to: quantifying knowledge actionability (rather than just interestingness) of data mining results [ 14 ], domain knowledge representation and domain generalization [ 30 ], domain-driven actionable knowledge discovery process [ 3 , 16 ], context-aware analytics and learning [ 46 ], discovering actionable patterns by combined mining [ 4 , 54 ] and high-utility mining [ 27 ], pattern relation analysis [ 4 ], cross-domain and transfer learning [ 24 , 36 , 45 , 51 ], data-to-decision transformation [ 8 ], personalized learning and recommendation [ 49 ], next-best action learning and recommendation [ 13 , 23 ], reflective learning with explicit and implicit feedback [ 32 , 50 ], explainable and interpretable analytics and learning [ 18 ], unbiased and fair analytics and learning [ 1 , 25 , 32 ], privacy and security-preserved analytics [ 52 ], and ethical analytics [ 34 ].
To better understand the challenges, recent advances, and new opportunities in domain-driven data mining, this special issue, along with other related activities, was proposed to call for the latest theoretical and practical developments, expert opinions on the open challenges, lessons learned, and best practices in domain-driven data mining. The special issue received submissions from researchers with different backgrounds, but all focusing on data-intensive research topics with novel applications. The papers accepted in this special issue explored novel factors and challenges such as socioeconomic, organizational, human-centered, and cultural aspects in different data mining tasks. In the following, we first provide a summary of the selected papers in the special issue.
1.2 Applied and flexible deep learning
Deep representation learning has attracted much attention in recent years. For chronic disease diagnosis, Zhang et al. [ 48 ] designed an unsupervised representation learning method to obtain informative correlation-aware signals from multivariate time series data. The key idea was a contrastive learning framework with a graph neural network (GNN) encoder to capture inter- and intra-correlation of multiple longitudinal variables. The work also considered modeling uncertainty quantification with evidential theory to assist the decision-making process in detecting chronic diseases. Also based on deep learning models, Sun et al. [ 37 ] adopted the sequential long short-term memory (LSTM) models in the domain of sports analytics for the baseball industry. With the numbers of home runs as the predictive target, the authors applied their models on the data from Major league Baseball (MLB) to support important decisions in managing players and teams. The results showed that deep learning model could perform better and bring valuable information to meet users’ needs. Focusing on more fundamental deep learning techniques, Zhao et al. [ 53 ] developed a flexible approach to compact architecture search for deep multitask learning (MTL) problems. Though sharing model architectures is a popular method for MTL problems, identifying the appropriate components to be shared by multiple tasks is still a challenge. Based on the expressive reinforcement learning framework, this paper proposed to discover flexible and compact MTL architectures with efficient search space and cost.
1.3 Interpretable and actionable predictions
A critical challenge facing data mining research is to discover actionable knowledge that can directly support decision-making tasks. In the domain of agricultural business and ecosystem management, Basak et al. [ 2 ] applied machine learning methods for a novel problem of soil moisture forecasting. The two modeling challenges were accurate long-term prediction and interpretable hydrological parameters. The proposed domain-driven solution was rooted in deterministic and physically based hydrological redistribution processes of gravity and suction.
As another example of actionable knowledge discovery, Dey et al. [ 19 ] proposed a systematic approach for fire station location planning. As urban fires could adversely affect the socioeconomic growth and ecosystem health of our communities, the authors applied various data mining and machine learning models in working with the Victoria Fire Department to make important decisions for selecting location of a new fire station. The key idea in their approach was to develop effective models for demand prediction and utilize the models to define a generalized index to measure quality of fire service in urban settings. The paper integrated multiple data sources and important domain knowledge/requirements in the modeling process. The final decision task was formulated as an integer programming problem to select the optimal location with maximum service coverage.
For sequential e-commerce product recommendation, Nasir and Ezeife [ 33 ] proposed the Semantic Enabled Markov Model Recommendation system to address long-standing challenges such as model complexity, data sparsity, and ambiguous predictions. Their system was proposed to extract and integrate sequential and semantic knowledge as well as contextual features. The new system showed improved recommendation performance for multiple e-commerce recommendation tasks.
1.4 Unsupervised learning with domain knowledge
Incorporating domain knowledge for unsupervised learning is particularly challenging due to the lack of clearly defined learning target. In the domain of health care, Jasinska-Piadlo et al. [ 22 ] explored the advantages and the challenges of a “domain-led” approach versus a data-driven approach to K -means clustering analysis. The authors compared expert opinions and principal component analysis for selecting the most useful variables to be used for the K -means clustering. The paper discussed comparative advantages of each approach and illustrated that domain knowledge played an important role at the interpretation stage of the clustering results. The authors developed a practical checklist guiding how to enable the integration of domain knowledge into a data mining project.
Similarly, text mining and natural language process are important research tools in many areas. However, many state-of-the-art text and language models are developed for general context, and careful adaption is often needed in applying such techniques on domain-specific data. In this special issue, Villanes and Healey [ 39 ] investigated the use of sentiment dictionaries to estimate sentiment for large document collections. The authors presented a semiautomatic method for extending general sentiment dictionary for a specific target domain. To minimize manual effort, the authors combined statistical term identification and term evaluation using Amazon Mechanical Turk in a study on dengue fever. The same approach could be potentially applied for constructing similar term-based sentiment dictionary in other target domains.
2 New trends from the industry perspective
A continuing trend in the data mining field has been the proliferation of its applications to new domains. This is partly due to the advancements in machine learning technologies evidenced by and promoted through frequent reports of new performance records on benchmark tasks. Another contributor to this proliferation is the increase in the quantity of data collected, stored, and appropriately documented for mining since the benefits of leveraging this data has become more apparent. Some of the works in this special issue demonstrated how data mining techniques can be applied in agriculture [ 2 ], health care and medicine [ 22 , 48 ], and city planning [ 19 ].
One aspect of data quality at the core of this expansion is the growing use of rich data formats. Image, audio, video, and raw text can now be almost directly fed into models that process them to extract meaningful features, patterns, and insights. These formats now often supplement the tabular data structures of the past as shown by Nasir and Ezeife [ 33 ]. To accommodate using these new formats, data mining and machine learning models have adapted to support multi-channel, multimodal, and sequential inputs [ 33 , 37 ].
As more domains employ novel data mining techniques, there have been more opportunities for cross-domain spillovers. We now see more examples of transfer learning, where models trained on one (source) domain are applied in another (target) domain suffering from data scarcity. However, learning generalized models that perform well on multiple tasks could be a challenging process [ 53 ]. These models are often trained with self-supervision on large data and contain millions or billions of learned parameters, such as models for language processing (e.g., BERT, GPT-3, XLNet) and image classification (ResNet, EfficientNet, Inception). A fundamental property of many generalized models is their ability to encode the input data into a vectorized representation, as evidenced by Zhang et al. [ 48 ].
Another recent challenge in data mining, one that is especially amplified in the case of transfer learning involving large models, is the issue of compactness. In many domains, where there is a need for scalable low-latency inferences and when the cost of training new models and deploying them could get high, it becomes necessary to restrict the model size. There are several techniques to accomplish these objectives including pruning, distilling, and training with constraints as Zhao et al. [ 53 ] demonstrated in this special issue.
Along with these trends, there have been several key developments in the structures used for data mining. One that has drastically improved the ability to digest sequential data is the invention of transformer structures. Transformers have effectively revolutionized the deep learning field by enabling models to understand the internal relationship between interdependent data points. These structures are the primary building blocks of some of the large generalized models mentioned above. Another recent progress is the improved ability of the generative models that learn not to score or classify but to create rich outputs such as images, texts, or audio. We also continue seeing more expansion in the field of graph neural network, where models learn and reproduce attributes of a graph data structure [ 48 ].
The sophistication of data mining methods has resulted in improved performance but comes at a cost. Models that use larger and richer input data, capture complex interaction between data points, and map the inputs to abstract representation spaces are very hard if not impossible to interpret. In many domains, it is important for the model outputs to be explainable to decision makers. Explainability matters for three reasons. First, explainable results are more powerful at both convincing decision makers and educating them with insights from the data [ 2 ]. Explainability is also a safeguard against models learning human biases and learning to discriminate. Finally, in some applications, it is necessary to understand not just the predicted value, but also the uncertainty of the predictions. Uncertainty modeling and quantification may be necessary in order to know when to rely on the machine and when to rely on the human. A recently popularized concept in this area is the human-in-the-loop approach, where models continuously receive and learn input from human experts and human decision makers, and meanwhile, experts use model predictions in their decision making on regular basis. Our authors in this special issue have demonstrated great potential of domain-driven data mining in addressing the aforementioned challenges, and more work is needed in the future with the collaboration between academia and industry.
3 Domain-driven data mining workshop
To facilitate the exchange of recent advances in domain-driven data mining, the Domain-Driven Data Mining Workshop was organized as a part of the 2021 SIAM International Conference on Data Mining. The workshop invited three keynote speakers and received paper submissions from multiple institutions. The papers accepted by the workshop were later invited for potential publication in this special issue. In the following, we review the invited keynote talks at the Domain-Driven Data Mining Workshop.
3.1 Actionable intelligence discovery
We first invited Dr. Longbing Cao for his keynote talk, “Domain-Driven and Actionable Intelligence Discovery.” In 2004, Dr. Cao proposed the concept “domain-driven data mining” and has led to implement many large enterprise data science projects for actionable knowledge discovery for governments and businesses, involving over 10 domains including capital markets, banking, insurance, telecommunication, transport, education, smart cities, online business, and public sectors (e.g., financial service, taxation, social welfare, IP, regulation, immigration).
Dr. Cao led a series of activities and proposed “domain-driven data mining” for “actionable knowledge discovery” in complex domains and problems, when discovering “actionable intelligence” was not a trivial task. The significant developments of data science, new-generation AI, and deep neural learning make domain-driven actionable intelligent discovery possible with progress made such as in representing and learning various complexities and intelligences in complex systems, data, and behaviors. In his talk, Dr. Cao first reviewed the aims, progresses, and gaps of conventional data mining/knowledge discovery and machine learning, domain-driven actionable knowledge discovery, and challenges and opportunities in domain-driven actionable intelligence discovery. Then, Dr. Cao discussed related strategic issues in data science thinking [ 8 ], new-generation AI [ 9 ], and actionable deep learning. Dr. Cao shared many thought-provoking illustrations, case studies, and theoretical and practical challenges in industry and government data sciences.
Particularly, Dr. Cao has made broad and in-depth contribution in understanding data complexities and data intelligence. One of his recent foci is learning from non-IID data, forming the research on non-IID learning [ 10 , 17 ]. Non-IID learning goes beyond the classic analytical and learning systems based on the common independent and identically distributed (IID) assumption widely taken in existing science, technology, and engineering. It studies the comprehensive non-IIDnesses [ 5 ], i.e., coupling relationships and interactions (including but beyond correlation and dependency) [ 6 ], and heterogeneities (including but beyond nonidentical distribution) in data, behaviors, and systems. The research on non-IID learning has evolved to almost all areas in data mining, analytics, and learning [ 17 ], such as non-IID data preparation, non-IID feature engineering, non-IID representation learning, non-IID similarity and metric learning, non-IID statistical learning, non-IID learning architecture, non-IID ensemble learning, non-IID federated learning, non-IID transfer learning, non-IID evaluation and validation, and various non-IID learning applications, such as non-IID recommender systems, non-IID outlier detection, non-IID information retrieval, and non-IID image and vision learning [ 5 , 20 , 35 , 47 , 55 ].
For instance, Cao [ 7 ] emphasized the critical issues of the intrinsic assumption that IID users and items in existing recommender systems, leading to false, misleading or incorrect recommendation, and poor performance in cold-start, sparse, and dynamic recommendations. Therefore, a non-IID theoretical framework is needed in order to build a deep and comprehensive understanding of the intrinsic nature of recommendation problems, from the perspective of both couplings and heterogeneities. Such research investigations led by Dr. Cao have triggered the paradigm shift from IID to non-IID recommendation research and can hopefully deliver informed, relevant, personalized, and actionable recommendations. All together, these contributions led to exciting new directions and fundamental solutions to address various challenges including cold-start, sparse data-based, cross-domain, group-based, and shilling attack-related issues in recommender systems.
3.2 A deep learning framework
We invited Dr. Balaji Padmanabhan for his keynote talk titled “Domain-Driven Data Mining: Examples and a Deep Learning Framework.” Dr. Padmanabhan is the Anderson Professor of Global Management and Professor of Information Systems at the University of South Florida’s Muma College of Business, where he is also the director of the Center for Analytics and Creativity. He has worked in data science, AI/machine learning, and business analytics for over two decades in the areas of research, teaching, business management, mentoring graduate students, and designing academic programs. He has also worked with over twenty firms on machine learning and data science initiatives in a variety of sectors. He has published extensively in data science and related areas at premier journals and conferences in the field and has served on the editorial board of leading journals including Management Science, MIS Quarterly, INFORMS Journal on Computing, Information Systems Research, Big Data, ACM Transactions on MIS, and the Journal of Business Analytics.
Dr. Padmanabhan witnessed and led the development of data mining. “I did my PhD at that time when the term of data mining first came up,” he shared with the audience of the workshop audience and reviewed the history of domain-driven data mining research. Then he presented a series of examples over the last two decades of his work. In generalizing from these examples, he emphasized that there are often different extents to which “domain” matters in different data mining endeavors. Dr. Padmanabhan encouraged the workshop audience to “think domain-driven,” which often motivates novel domain-driven methods that can meanwhile be applied more broadly (or “domain free”). Dr. Padmanabhan also shared a general framework for domain-driven deep learning in business research and used this framework to show how researchers can highlight significant contributions and position their own papers and ideas. Dr. Padmanabhan’s insightful cases and valuable research advice were greatly appreciated by the workshop audience from research communities of both computer science and management information systems.
In his talk, Dr. Padmanabhan also shared that his department has completed 100 projects in 7 years with about 30 companies, and funded postdoctoral research in analytics. His department has several outreach initiatives such as Economic Analytics Initiative and Florida Business Analytics Forum. Dr. Padmanabhan highlighted that such industrial collaborations and initiatives have greatly rewarded research activities particularly in domain-driven data mining projects. Dr. Padmanabhan encouraged researchers to actively reach out to industry not only when finding data but also to ask for new research questions.
3.3 Human resource management
We invited Dr. Hui Xiong for his keynote talk, “Artificial Intelligence in Human Resource Management.” Dr. Hui Xiong is a Distinguished Professor at the Rutgers, the State University of New Jersey. He also served as the Smart City Chief Scientist and the Deputy Dean of Baidu Research Institute in charge of several research laboratories. He is a co-Editor-in-Chief of Encyclopedia of GIS, an Associate Editor of IEEE Transactions on Big Data (TBD), ACM Transactions on Knowledge Discovery from Data (TKDD), and ACM Transactions on Management Information Systems (TMIS). Dr. Xiong has chaired for many international conferences in data mining, including a Program Co-Chair (2013) and a General Co-Chair (2015) for the IEEE International Conference on Data Mining (ICDM), and a Program Co-Chair of the Research Track (2018) and the Industry Track (2012) for the ACM SIGKDD International Conference on Knowledge Discovery and Data Mining (KDD). Dr. Xiong’s research has generated substantive impact beyond academia. He is an ACM distinguished scientist and has been honored by the ICDM-2011 Best Research Paper Award, the 2017 IEEE ICDM Outstanding Service Award, and the 2018 Ram Charan Management Practice Award as the Grand Prix winner from the Harvard Business Review. In 2020, he was named as an AAAS Fellow and an IEEE Fellow.
Dr. Xiong shared a successful story in leveraging big data technology for human resource management. Indeed, the availability of large-scale human resource (HR) data has enabled unparalleled opportunities for business leaders to understand talent behaviors and generate useful talent knowledge, which in turn deliver intelligence for real-time decision making and effective people management at work. In his talk, Dr. Xiong introduced a powerful set of innovative Artificial Intelligence (AI) techniques developed for intelligent human resource management, such as recruiting, performance evaluation, talent retention, talent development, job matching, team management, leadership development, and organization culture analysis. With his rich experiences and close collaborations with the industry, Dr. Xiong demonstrated how the results of talent analytics can be used for other business applications, such as market trend analysis and financial investment.
4 Concluding remarks
This special issue was proposed and edited to draw attention to domain-driven data mining and disseminate research in foundations, frameworks, and applications for data-driven and actionable knowledge discovery. This special issue and related activities on recent advances in domain-driven data mining continued the previous efforts including the workshop series on the same topic during 2007–2014 with the IEEE International Conference on Data Mining and a special issue published by the IEEE Transactions on Knowledge and Data Engineering [ 44 ]. Although many scholars have made significant contributions with the theme of domain-driven data mining, there are still various new research problems and challenges calling for more research investigations in the coming years. We hope this special issue is helpful for scholars working along this critically important line of research.
Alves, G., Amblard, M., Bernier, F., Couceiro, M., Napoli, A.: Reducing unintended bias of ML models on tabular and textual data. In: DSAA, pp. 1–10 (2021)
Basak, A., Schmidt, K.M., Mengshoel, O.J.: From data to interpretable models: machine learning for soil moisture forecasting. Int. J. Data Sci. Anal. (2022). https://doi.org/10.1007/s41060-022-00347-8
Cao, L.: Domain-driven data mining: challenges and prospects. IEEE Trans. Knowl. Data Eng. 22 (6), 755–769 (2010)
Article Google Scholar
Cao, L.: Combined mining: analyzing object and pattern relations for discovering and constructing complex yet actionable patterns. Wiley Interdiscip. Rev. Data Min. Knowl. Discov. 3 (2), 140–155 (2013)
Cao, L.: Non-iidness learning in behavioral and social data. Comput. J. 57 (9), 1358–1370 (2014)
Cao, L.: Coupling learning of complex interactions. Inf. Process. Manag. 51 (2), 167–186 (2015)
Cao, L.: Non-iid recommender systems: a review and framework of recommendation paradigm shifting. Engineering 2 (2), 212–224 (2016)
Cao, L.: Data Science Thinking: The Next Scientific, Technological and Economic Revolution. Data Analytics. Springer, Berlin (2018)
Book Google Scholar
Cao, L.: A new age of AI: features and futures. IEEE Intell. Syst. 37 (1), 25–37 (2022)
Cao, L.: Beyond i.i.d.: non-iid thinking, informatics, and learning. IEEE Intell. Syst. 37 (04), 5–17 (2022)
Cao, L., Zhang, C.: Domain-driven actionable knowledge discovery in the real world. In: PAKDD 2006, pp. 821–830 (2006)
Cao, L., Zhang, C.: The evolution of kdd: towards domain-driven data mining. IJPRAI 21 (4), 677–692 (2007)
Google Scholar
Cao, L., Zhu, C.: Personalized next-best action recommendation with multi-party interaction learning for automated decision-making. PLoS ONE 17 , 1–22 (2022)
Cao, L., Luo, D., Zhang, C.: Knowledge actionability: satisfying technical and business interestingness. IJBIDM 2 (4), 496–514 (2007)
Cao, L., Zhang, C., Yang, Q., Bell, D.A., Vlachos, M., Taneri, B., Keogh, E.J., Yu, P.S., Zhong, N., Ashrafi, M.Z., Taniar, D., Dubossarsky, E., Graco, W.: Domain-driven, actionable knowledge discovery. IEEE Intell. Syst. 22 (4), 78–88 (2007)
Cao, L., Yu, P.S., Zhang, C., Zhao, Y.: Domain Driven Data Mining. Springer, Berlin (2010)
Book MATH Google Scholar
Cao, L., Philip, S.Y., Zhao, Z.: Shallow and deep non-iid learning on complex data. In: Proceedings of the ACM SIGKDD International Conference on Knowledge Discovery and Data Mining, (2022)
Carlevaro, A., Mongelli, M.: A new SVDD approach to reliable and explainable AI. IEEE Intell. Syst. 37 (2), 55–68 (2022)
Dey, A., Heger, A., England, D.: Urban fire station location planning using predicted demand and service quality index. Int. J. Data Sci. Anal. (2022). https://doi.org/10.1007/s41060-022-00328-x
Do, T.D.T., Cao, L.: Gamma-Poisson dynamic matrix factorization embedded with metadata influence. In: NeurIPS 2018, pp. 5829–5840 (2018)
He, F., Li, Y., Xu, T., Yin, L., Zhang, W., Zhang, X.: A data-analytics approach for risk evaluation in peer-to-peer lending platforms. IEEE Intell. Syst. 35 (3), 85–95 (2020)
Jasinska-Piadlo, A., Bond, R., Biglarbeigi, P., Brisk, R., Campbell, P., Browne, F., McEneaneny, D.: Data-driven versus a domain-led approach to k-means clustering on an open heart failure dataset. Int. J. Data Sci. Anal. (2022). https://doi.org/10.1007/s41060-022-00346-9
Jin, B., Yang, H., Sun, L., Liu, C., Qu, Y., Tong, J.: A treatment engine by predicting next-period prescriptions. In: KDD, pp. 1608–1616 (2018)
Kanter, J.M., Gillespie, O., Veeramachaneni, K.: Label, segment, featurize: a cross domain framework for prediction engineering. In: DSAA, pp. 430–439 (2016)
Ke, W., Liu, C., Shi, X., Dai, Y., Yu, P.S., Zhu, X.: Addressing exposure bias in uplift modeling for large-scale online advertising. In: ICDM, pp. 1156–1161 (2021)
Kompan, M., Gaspar, P., Macina, J., Cimerman, M., Bieliková, M.: Exploring customer price preference and product profit role in recommender systems. IEEE Intell. Syst. 37 (1), 89–98 (2022)
Lin, J.C.-W., Gan, W., Fournier-Viger, P., Hong, T.-P., Tseng, V.S.: Mining high-utility itemsets with various discount strategies. In: DSAA, pp. 1–10 (2015)
Liu, C., Zhu, W.: Precision coupon targeting with dynamic customer triage. In: DSAA, pp. 420–428 (2020)
Liu, Q., Zeng, X., Liu, C., Zhu, H., Chen, E., Xiong, H., Xie, X.: Mining indecisiveness in customer behaviors. In: ICDM, pp. 281–290 (2015)
Long, M., Wang, J., Sun, J.-G., Yu, P.S.: Domain invariant transfer kernel learning. IEEE Trans. Knowl. Data Eng. 27 (6), 1519–1532 (2015)
Ma, D., Narayanan, V.K., Liu, C., Fakharizadi, E.: Boundary salience: the interactive effect of organizational status distance and geographical proximity on coauthorship tie formation. Soc. Netw. 63 , 162–173 (2020)
Melucci, M.: Investigating sample selection bias in the relevance feedback algorithm of the vector space model for information retrieval. In: DSAA, pp. 83–89 (2014)
Nasir, M., Ezeife, C.I.: Semantic enhanced Markov model for sequential e-commerce product recommendation. Int. J. Data Sci. Anal., (2022) https://doi.org/10.1007/s41060-022-00343-y
O’Leary, D.E.: Ethics for big data and analytics. IEEE Intell. Syst. 31 (4), 81–84 (2016)
Pang, G., Cao, L., Chen, L.: Homophily outlier detection in non-iid categorical data. Data Min. Knowl. Discov. 35 (4), 1163–1224 (2021)
Article MATH Google Scholar
Ruiz-Dolz, R., Alemany, J., Barberá, S.H., García-Fornes, A.: Transformer-based models for automatic identification of argument relations: a cross-domain evaluation. IEEE Intell. Syst. 36 (6), 62–70 (2021)
Sun, H.-C., Lin, T.-Y., Tsai, Y.-L.: Performance prediction in major league baseball by long short-term memory networks. Int. J. Data Sci. Anal. (2022). https://doi.org/10.1007/s41060-022-00313-4
Teng, M., Zhu, H., Liu, C., Xiong, H.: Exploiting network fusion for organizational turnover prediction. ACM Trans. Manag. Inf. Syst. 12 (2), 16:1-16:18 (2021)
Villanes, A., Healey, C.G.: Domain-specific text dictionaries for text analytics. Int. J. Data Sci. Analy., Special Issue on Domain-Driven Data Mining (2022)
Xiang, H., Lin, J., Chen, C.-H., Kong, Y.: Asymptotic meta learning for cross validation of models for financial data. IEEE Intell. Syst. 35 (2), 16–24 (2020)
Xu, L., Wei, X., Cao, J., Yu, P.S.: Multiple social role embedding. In: DSAA, pp. 581–589. IEEE (2017)
Yang, D., Bingqing, Q., Cudré-Mauroux, P.: Location-centric social media analytics: challenges and opportunities for smart cities. IEEE Intell. Syst. 36 (5), 3–10 (2021)
Yang, J., Liu, C., Teng, M., Xiong, H., Liao, M., Zhu, V.: Exploiting temporal and social factors for B2B marketing campaign recommendations. In: ICDM, pp. 499–508 (2015)
Zhang, C., Yu, P., Bell, D.: Introduction to the domain-drive data mining special section. IEEE Trans. Knowl. Data Eng. 22 (6), 753–754 (2010)
Zhang, J., He, M.: CRTL: context restoration transfer learning for cross-domain recommendations. IEEE Intell. Syst. 36 (4), 65–72 (2021)
Zhang, K., Chen, E., Liu, Q., Liu, C., Lv, G.: A context-enriched neural network method for recognizing lexical entailment. In: AAAI, pp. 3127–3134 (2017)
Zhang, Q., Cao, L., Zhu, C., Li, Z., Sun, J.: Coupledcf: learning explicit and implicit user-item couplings in recommendation for deep collaborative filtering. In: IJCAI 2018, pp. 3662–3668 (2018)
Zhang, X., Wang, Y., Zhang, L., Jin, B., Zhang, H.: Exploring unsupervised multivariate time series representation learning for chronic disease diagnosis. Int. J. Data Sci. Anal. (2022). https://doi.org/10.1007/s41060-021-00290-0
Zhang, Y., Liu, G., Liu, A., Zhang, Y., Li, Z., Zhang, X., Li, Q.: Personalized geographical influence modeling for POI recommendation. IEEE Intell. Syst. 35 (5), 18–27 (2020)
Zhang, Y., Bai, G., Zhong, M., Li, X., Ryan, K.L.K.: Differentially private collaborative coupling learning for recommender systems. IEEE Intell. Syst. 36 (1), 16–24 (2021)
Zhang, Y., Zhang, X., Shen, T., Zhou, Y., Wang, Z.: Feature-option-action: a domain adaption transfer reinforcement learning framework. In: DSAA, pp. 1–12 (2021)
Zhang, Z., Liu, Q., Huang, Z., Wang, H., Lu, C., Liu, C., Chen, E.: Graphmi: extracting private graph data from graph neural networks. In: IJCAI, pp. 3749–3755 (2021)
Zhao, J., Lv, W., Du, B., Ye, J., Sun, L., Xiong, G.: Deep multi-task learning with flexible and compact architecture search. Int. J. Data Sci. Anal., Special Issue on Domain-Driven Data Mining (2022)
Zhao, Y., Zhang, H., Cao, L., Zhang, C., Bohlscheid, H.: Combined pattern mining: from learned rules to actionable knowledge. In: AI 2008, pp. 393–403 (2008)
Zhu, C., Cao, L., Yin, J.: Unsupervised heterogeneous coupling learning for categorical representation. IEEE Trans. Pattern Anal. Mach. Intell. 44 (1), 533–549 (2022)
Download references
Author information
Authors and affiliations.
The University of Tennessee, Knoxville, USA
Chuanren Liu
Snap Inc., Seattle, WA, USA
Ehsan Fakharizadi
University of Science and Technology of China, Hefei, China
University of Illinois Chicago, Chicago, USA
Philip S. Yu
You can also search for this author in PubMed Google Scholar
Corresponding author
Correspondence to Chuanren Liu .
Additional information
Publisher's note.
Springer Nature remains neutral with regard to jurisdictional claims in published maps and institutional affiliations.
Rights and permissions
Reprints and permissions
About this article
Liu, C., Fakharizadi, E., Xu, T. et al. Recent advances in domain-driven data mining. Int J Data Sci Anal 15 , 1–7 (2023). https://doi.org/10.1007/s41060-022-00378-1
Download citation
Published : 27 December 2022
Issue Date : January 2023
DOI : https://doi.org/10.1007/s41060-022-00378-1
Share this article
Anyone you share the following link with will be able to read this content:
Sorry, a shareable link is not currently available for this article.
Provided by the Springer Nature SharedIt content-sharing initiative
- Find a journal
- Publish with us
- Track your research

An official website of the United States government
The .gov means it’s official. Federal government websites often end in .gov or .mil. Before sharing sensitive information, make sure you’re on a federal government site.
The site is secure. The https:// ensures that you are connecting to the official website and that any information you provide is encrypted and transmitted securely.
- Publications
- Account settings
Preview improvements coming to the PMC website in October 2024. Learn More or Try it out now .
- Advanced Search
- Journal List
- Int J Environ Res Public Health

Data Mining in Healthcare: Applying Strategic Intelligence Techniques to Depict 25 Years of Research Development
Maikel luis kolling.
1 Graduate Program of Industrial Systems and Processes, University of Santa Cruz do Sul, Santa Cruz do Sul 96816-501, Brazil; [email protected] (M.L.K.); [email protected] (M.K.S.)
Leonardo B. Furstenau
2 Department of Industrial Engineering, Federal University of Rio Grande do Sul, Porto Alegre 90035-190, Brazil; rb.csinu.2xm@uanetsrufodranoel
Michele Kremer Sott
Bruna rabaioli.
3 Department of Medicine, University of Santa Cruz do Sul, Santa Cruz do Sul 96816-501, Brazil; moc.liamg@iloiabbaranurb
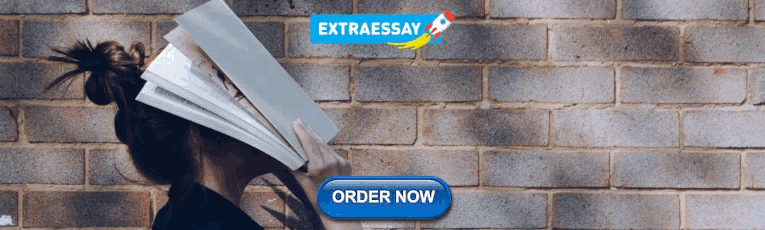
Pedro Henrique Ulmi
4 Department of Computer Science, University of Santa Cruz do Sul, Santa Cruz do Sul 96816-501, Brazil; [email protected]
Nicola Luigi Bragazzi
5 Laboratory for Industrial and Applied Mathematics (LIAM), Department of Mathematics and Statistics, York University, Toronto, ON M3J 1P3, Canada
Leonel Pablo Carvalho Tedesco
Associated data.
Not applicable.
In order to identify the strategic topics and the thematic evolution structure of data mining applied to healthcare, in this paper, a bibliometric performance and network analysis (BPNA) was conducted. For this purpose, 6138 articles were sourced from the Web of Science covering the period from 1995 to July 2020 and the SciMAT software was used. Our results present a strategic diagram composed of 19 themes, of which the 8 motor themes (‘NEURAL-NETWORKS’, ‘CANCER’, ‘ELETRONIC-HEALTH-RECORDS’, ‘DIABETES-MELLITUS’, ‘ALZHEIMER’S-DISEASE’, ‘BREAST-CANCER’, ‘DEPRESSION’, and ‘RANDOM-FOREST’) are depicted in a thematic network. An in-depth analysis was carried out in order to find hidden patterns and to provide a general perspective of the field. The thematic network structure is arranged thusly that its subjects are organized into two different areas, (i) practices and techniques related to data mining in healthcare, and (ii) health concepts and disease supported by data mining, embodying, respectively, the hotspots related to the data mining and medical scopes, hence demonstrating the field’s evolution over time. Such results make it possible to form the basis for future research and facilitate decision-making by researchers and practitioners, institutions, and governments interested in data mining in healthcare.
1. Introduction
Deriving from Industry 4.0 that pursues the expansion of its autonomy and efficiency through data-driven automatization and artificial intelligence employing cyber-physical spaces, the Healthcare 4.0 portrays the overhaul of medical business models towards a data-driven management [ 1 ]. In akin environments, substantial amounts of information associated to organizational processes and patient care are generated. Furthermore, the maturation of state-of-the-art technologies, namely, wearable devices, which are likely to transform the whole industry through more personalized and proactive treatments, will lead to a noteworthy increase in user patient data. Moreover, the forecast for the annual global growth in healthcare data should exceed soon 1.2 exabytes a year [ 1 ]. Despite the massive and growing volume of health and patient care information [ 2 ], it is still, to a great extent, underused [ 3 ].
Data mining, a subfield of artificial intelligence that makes use of vast amounts of data in order to allow significant information to be extracted through previously unknown patterns, has been progressively applied in healthcare to assist clinical diagnoses and disease predictions [ 2 ]. This information has been known to be rather complex and difficult to analyze. Furthermore, data mining concepts can also perform the analysis and classification of colossal bulks of information, grouping variables with similar behaviors, foreseeing future events, amid other advantages for monitoring and managing health systems ceaselessly seeking to look after the patients’ privacy [ 4 ]. The knowledge resulting from the application of the aforesaid methods may potentially improve resource management and patient care systems, assist in infection control and risk stratification [ 5 ]. Several studies in healthcare have explored data mining techniques to predict incidence [ 6 ] and characteristics of patients in pandemic scenarios [ 7 ], identification of depressive symptoms [ 8 ], prediction of diabetes [ 9 ], cancer [ 10 ], scenarios in emergency departments [ 11 ], amidst others. Thus, the utilization of data mining in health organizations ameliorates the efficiency of service provision [ 12 ], quality of decision making, and reduces human subjectivity and errors [ 13 ].
The understanding of data mining in the healthcare sector is, in this context, vital and some researchers have executed bibliometric analyses in the field with the intention of investigating the challenges, limitations, novel opportunities, and trends [ 14 , 15 , 16 , 17 ]. However, at the time of this study, there were no published works that provided a complete analysis of the field using a bibliometric performance and network analysis (BPNA) (see Table 1 . In the light of this, we have defined three research questions:
- RQ1: What are the strategic themes of data mining in healthcare?
- RQ2: How is the thematic evolution structure of data mining in healthcare?
- RQ3: What are the trends and opportunities of data mining in healthcare for academics and practitioners?
Existing bibliometric analysis of data mining in healthcare in Web of Science (WoS).
Study | Coverage | Focus |
---|---|---|
[ ] | 2000–2017 | Analysis of the evolution of emerging technologies (e.g., data mining, machine learning, among others) in cancer using CiteSpace software. |
[ ] | 2009–2018 | Exploration of data mining and machine learning in public health sector. |
[ ] | 2011–2019 | Investigation of medical data mining using VOSviewer and CiteSpace software. |
This paper | 1995–2020 | A BPNA of data mining in healthcare: performance analysis, strategic themes, thematic evolution structure, trends and future opportunities using SciMAT software. |
Thus, with the objective to lay out a superior understanding of the data mining usage in the healthcare sector and to answer the defined research questions, we have performed a bibliometric performance and network analysis (BPNA) to set fourth an overview of the area. We used the Science Mapping Analysis Software Tool (SciMAT), a software developed by Cobo et al. [ 18 ] with the purpose of identifying strategic themes and the thematic evolution structure of a given field, which can be used as a strategic intelligence tool. The strategic intelligence, an approach that can enhance decision-making in terms of science and technology trends [ 19 , 20 , 21 , 22 , 23 , 24 , 25 , 26 , 27 ], can help researchers and practitioners to understand the area and devise new ideas for future works as well as to identify the trends and opportunities of data mining in healthcare.
This research is structured as follows: Section 2 highlights the methodology and the dataset. Section 3 presents the bibliometric performance of data mining in healthcare. In Section 4 , the strategic diagram presents the most relevant themes according to our bibliometric indicators as well as the thematic network structure of the motor themes and the thematic evolution structure, which provide a complete overview of data mining over time. Section 5 presents the conclusions, limitations, and suggestions for future works.
2. Methodology and Dataset
Attracting attention from companies, universities, and scientific journals, bibliometric analysis enhances decision-making by providing a reliable method to collect information from databases, to transform the aforementioned data into knowledge, and to stimulate wisdom development. Furthermore, the techniques of bibliometric analysis can provide higher and different perspectives of scientific production by using advanced measurement tools and methods to depict how authors, works, journals and institutions are advancing in a specific field of research through the hidden patterns that are embedded in large datasets.
The existing works on bibliometric analysis of data mining in health care in the Web of Science are shown in Table 1 , where it is depicted that only three studies have been performed and the differences between these approaches and this work are explained.
2.1. Methodology
For this study we have applied BPNA, a method that combines science mapping with performance analysis, to the field of data mining in healthcare with the support of the SciMAT software. This methodology has been chosen in view of the fact that such a combination, in addition to assisting decision-making for academics and practitioners, allows us to perform a deep investigation into the field of research by giving a new perspective of its intricacies. The BPNA conducted in this paper was composed of four steps outlined below.
2.1.1. Discovery of Research Themes
The themes were identified using a frequency and network reduction of keywords. In this process, the keywords were firstly normalized using the Salton’s Cosine, a correlation coefficient, and then clustered through the simple center algorithm. Finally, the thematic evolution structure co-word network was normalized using the equivalence index.
2.1.2. Depicting Research Themes
The previously identified themes were then plotted on a bi-dimensional diagram composed of four quadrants, in which the “vertical axis” characterizes the density (D) and the “horizontal axis” characterizes the centrality (C) of the theme [ 28 , 29 ] ( Figure 1 a) [ 18 , 20 , 25 , 30 , 31 , 32 , 33 ].

Strategic diagram ( a ). Thematic network structure ( b ). Thematic evolution structure ( c ).
- (a) First quadrant—motor themes: trending themes for the field of research with high development.
- (b) Second quadrant—basic and transversal themes: themes that are inclined to become motor themes in the future due to their high centrality.
- (c) Third quadrant—emerging or declining themes: themes that require a qualitative analysis to define whether they are emerging or declining.
- (d) Fourth quadrant—highly developed and isolated themes: themes that are no longer trending due to a new concept or technology.
2.1.3. Thematic Network Structure and Detection of Thematic Areas
The results were organized and structured in (a) a strategic diagram (b) a thematic network structure of motor themes, and (c) a thematic evolution structure. The thematic network structure ( Figure 1 b) represents the co-occurrence between the research themes and underlines the number of relationships (C) and internal strength among them (D). The thematic evolution structure ( Figure 1 c) provides a proper picture of how the themes preserve a conceptual nexus throughout the following sub-periods [ 23 , 34 ]. The size of the clusters is proportional to the number of core documents and the links indicate co-occurrence among the clusters. Solid lines indicate that clusters share the main theme, and dashed lines represent the shared cluster elements that are not the name of the themes [ 35 ]. The thickness of the lines is proportional to the inclusion index, which indicates that the themes have elements in common [ 35 ]. Furthermore, in the thematic network structure the themes were then manually classified between data mining techniques and medical research concepts.
2.1.4. Performance Analysis
The scientific contribution was measured by analyzing the most important research themes and thematic areas using the h-index, sum of citations, core documents centrality, density, and nexus among themes. The results can be used as a strategic intelligence approach to identify the most relevant topics in the research field.
2.2. Dataset
Composed of 6138 non-duplicated articles and reviews in English language, the dataset used in this work was sourced from the Web of Science (WoS) database utilizing the following query string (“data mining” and (“health*” OR “clinic*” OR “medic* OR “disease”)). The documents were then processed and had their keywords, both the author’s and the index controlled and uncontrolled terms, extracted and grouped in accordance with their meaning. In order to remove duplicates and terms which had less than two occurrences in the documents, a preprocessing step was applied to the authors, years, publication dates, and keywords. For instance, the preprocessing has reduced the total number of keywords from 21,838 to 5310, thus improving the bibliometric analysis clarity. With the exception of the strategic diagram that was plotted utilizing a single period (1995–July 2020), in this study, the timeline was divided into three sub-periods: 1995–2003, 2004–2012, and 2013–July 2020.
Subsequently, a network reduction was applied in order to exclude irrelevant words and co-occurrences. For the network extraction we wanted to identify co-occurrence among words. For the mapping process, we used a simple center algorithm. Finally, a core mapper was used, and the h-index and sum citations were selected. Figure 2 shows a good representation of the steps of the BPNA.

Workflow of the bibliometric performance and network analysis (BPNA).
3. Bibliometric Performance of Data Mining in Healthcare
In this section, we measured the performance of the field of data mining in healthcare in terms of publications and citations over time, the most productive and cited researchers, as well as productivity of scientific journals, institutions, countries, and most important research areas in the WoS. To do this, we used indicators such as: number of publications, sum of citations by year, journal impact factor (JIF), geographic distribution of publications, and research field. For this, we examined the complete period (1995 to July 2020).
3.1. Publications and Citations Overtime
Figure 3 shows the performance analysis of publications and citations of data mining in healthcare over time from 1995 to July 2020 in the WoS. The first sub-period (1995–2003) shows the beginning of the research field with 316 documents and a total of 13,483 citations. Besides, the first article in the WoS was published by Szolovits (1995) [ 36 ] who presented a tutorial for handling uncertainty in healthcare and highlighted the importance to develop data mining techniques in order to assist the healthcare sector. This sub-period shows a slightly increasing number of citations until 2003 and the year with the highest number of citations was 2002.

Number of publications over time (1995–July 2020).
The slightly increasing number continues from the first sub-period to the second subperiod (2004–2013) with a total of 1572 publications and 55,734 citations. The year 2006 presents the highest number of citations mainly due to the study of Fawcett [ 37 ] which attracted 7762 citations. The author introduced the concept of Receiver Operating Characteristics (ROC). This technique is widely used in data mining to assist medical decision-making.
From the second to the third sub-period, it is possible to observe a huge increase in the number of publications (4250 publications) and 41,821 citations. This elevated increase may have occurred due to the creation of strategies to implement emerging technologies in the healthcare sector in order to move forward with the third digital revolution in healthcare, the so-called Healthcare 4.0 [ 1 , 38 ]. Furthermore, although the citations are showing a positive trend, it is still possible to observe a downward trend from 2014 to 2020. This may happen, as Wang [ 39 ] highlights, due to the fact that a scientific document needs three to seven years to reach its peak point of citation [ 34 ]. Therefore, this is not a real trend.
3.2. Most Productive and Cited Authors
Table 2 displays the most productive and cited authors from 1995 to July 2020 of data mining in healthcare in the WoS. Leading as the most productive researcher in the field of data mining in healthcare is Li, Chien-Feng, a pathologist at Chi Mei Hospital which is sixth-ranked in publication numbers. He dedicates his studies to the molecular diagnosis of cancer with innovative technologies. In the sequence, Acharya, U. Rajendra, ranked in the top 1% of highly cited researchers in five consecutive years (2016, 2017, 2018, 2019, and 2020) in computer science according to Thomson’s essential science indicators, shares second place with Chung, Kyungyong from the Division of Engineering and Computer Science at the Kyonggi University in Su-won-si, South Korea. On the other hand, Bate, Andrew C., a member of the Food and Drug Administration (FDA) Science Council of Pharmacovigilance Subcommittee, which is the fourth-ranked institution in publication count as the most cited researcher with 945 citations. Subsequently, Lindquist, Marie, who monitors global pharmacovigilance and data management development at the World Health Organization (WHO), is ranked second with 943 citations. Last but not least, Edwards, E.R., an orthopedic surgeon at the Royal Australasian College of Surgeons is ranked third with 888 citations. Notably, this study does not demonstrate a direct correlation between the number of publications and the number of citations.
Most Cited/Productive authors from 1995 to July 2020.
Author Citation | Citations | Author Productivity | Documents |
---|---|---|---|
Bate, Andrew C. | 945 | Li, Chien-Feng | 36 |
Lindquist, Marie | 943 | Acharya, U. Rajendra | 21 |
Edwards, E.R. | 888 | Chung, Kyungyong | 21 |
Moore, Jason H. | 711 | Chen, Gang | 19 |
Cook, Diane, J. | 599 | Lee, Sung-Wei | 18 |
Eppig, Janan, T. | 577 | Moore, Jason H. | 17 |
White, Bill, C. | 541 | Cano, Maria | 17 |
Bellazi, Riccardo | 527 | Chang, I-Wei | 16 |
Szarfman, A. | 511 | He, Hong-Lin | 16 |
Lambin, Philippe | 489 | Moro, Pedro L. | 16 |
3.3. Productivity of Scientific Journals, Universities, Countries and Most Important Research Fields
Table 3 shows the journals that publish studies related to data mining in healthcare. PLOS One is the first ranked with 124 publications, followed by Expert Systems with Applications with 105, and Artificial Intelligence in Medicine with 75. On the other hand, the journal Expert Systems with Applications is the journal that had the highest Journal Impact Factor (JIF) from 2019–2020.
Journals that publish studies to data mining in healthcare.
Journal | Doc. | JIF |
---|---|---|
PLOS One | 124 | 2.74 |
Expert Systems with Applications | 105 | 5.89 |
Artificial Intelligence in Medicine | 75 | 4.47 |
Journal of Biomedical Informatics | 75 | 3.57 |
BMC Bioinformatics | 66 | 2.13 |
Journal of Medical Systems | 65 | 2.83 |
IEEE Access | 65 | 3.74 |
Computer Methods and Programs in Biomedicine | 59 | 3.63 |
International Journal of Advanced Computer Science and Applications | 54 | 1.32 |
Journal of The American Medical Informatics Association | 53 | 4.11 |
Table 4 shows the most productive institutions and the most productive countries. The first ranked is Columbia University followed by U.S. FDA Registration and Harvard University. In terms of country productivity, United States is the first in the rank, followed by China and England. In comparison with Table 2 , it is possible to notice that the most productive author is not related to the most productive institutions (Columbia University and U.S. FDA Registration). Besides, the institution with the highest number of publications is in the United States, which is found to be the most productive country.
Institutions and countries that publish studies to data mining in healthcare.
University | Documents | Country | Documents |
---|---|---|---|
Columbia University | 62 | United States | 1973 |
U.S. FDA Registration | 62 | China | 923 |
Harvard University | 60 | England | 370 |
Stanford University | 55 | India | 354 |
Chinese Academy of Sciences | 53 | Germany | 312 |
Chi Mei Medical Center | 47 | Italy | 297 |
University of Pennsylvania | 45 | Taiwan | 294 |
Kaohsiung Medical University | 44 | Australia | 282 |
University of Toronto | 44 | Canada | 252 |
University of Pittsburgh | 44 | Netherlands | 117 |
Regarding Columbia University, it is possible to verify its prominence in data mining in healthcare through its advanced data science programs, which are one of the best evaluated and advanced in the world. We highlight the Columbia Data Science Society, an interdisciplinary society that promotes data science at Columbia University and the New York City community.
The U.S. FDA Registration has a data mining council to promote the prioritization and governance of data mining initiatives within the Center for Biological Research and Evaluation to assess spontaneous reports of adverse events after the administration of regulated medical products. In addition, they created an Advanced and Standards-Based Network Analyzer for Clinical Assessment and Evaluation (PANACEA), which supports the application of standards recognition and network analysis for reporting these adverse events. It is noteworthy that the FDA Adverse Events Reporting System (FAERS) database is the main resource that identifies adverse reactions in medications marketed in the United States. A text mining system based on EHR that retrieves important clinical and temporal information is also highlighted along with support for the Cancer Prevention and Control Division at the Centers for Disease Control and Prevention in a big data project.
The Harvard University offers online data mining courses and has a Center for Healthcare Data Analytics created by the need to analyze data in large public or private data sets. Harvard research includes funding and providing healthcare, quality of care, studies on special and disadvantaged populations, and access to care.
Table 5 presents the most important WoS subject research fields of data mining in healthcare from 1995 to July 2020. Computer Science Artificial Intelligence is the first ranked with 768 documents, followed by Medical Informatics with 744 documents, and Computer Science Information Systems with 722 documents.
Most relevant WoS subject categories and research fields.
WoS Subject Categories | Doc. |
---|---|
Computer Science Artificial Intelligence | 768 |
Medical Informatics | 744 |
Computer Science Information Systems | 722 |
Computer Science Interdisciplinary Applications | 603 |
Mathematical Computational Biology | 505 |
Health Care Sciences Services | 419 |
Pharmacology Pharmacy | 370 |
Engineering Electrical Electronic | 364 |
Computer Science Theory Methods | 357 |
Biochemical Research Methods | 304 |
4. Science Mapping Analysis of Data Mining in Healthcare
In this section the science mapping analysis of data mining in healthcare is depicted. The strategic diagram shows the most relevant themes in terms of centrality and density. The thematic network structure uncovers the relationship (co-occurrence) between themes and hidden patterns. Lastly, the thematic evolution structure underlines the most important themes of each sub-period and shows how the field of study is evolving over time.
4.1. Strategic Diagram Analysis
Figure 4 presents 19 clusters, 8 of which are categorized as motor themes (‘NEURAL-NETWORKS’, ‘CANCER’, ‘ELETRONIC-HEALTH-RECORDS’, ‘DIABETES-MELLITUS’, ‘ADVERSE-DRUG-EVENTS’, ‘BREAST-CANCER’, ‘DEPRESSION’ and ‘RANDOM-FOREST’), 2 as basic and transversal themes (‘CORONARY-ARTERY-DISEASE’ and ‘PHOSPHORYLATION’), 7 as emerging or declining themes (‘PERSONALIZED-MEDICINE’, ‘DATA-INTEGRATION’, ‘INTENSIVE-CARE-UNIT’, ‘CLUSTER-ANALYSIS’, ‘INFORMATION-EXTRACTION’, ‘CLOUD-COMPUTING’ and ‘SENSORS’), and 2 as highly developed and isolated themes (‘ALZHEIMERS-DISEASE’, and ‘METABOLOMICS’).

Strategic diagram of data mining in healthcare (1995–July 2020).
Each cluster of themes was measured in terms of core documents, h-index, citations, centrality, and density. The cluster ‘NEURAL-NETWORKS’ has the highest number of core documents (336) and is ranked first in terms of centrality and density. On the other hand, the cluster ‘CANCER’ is the most widely cited with 5810 citations.
4.2. Thematic Network Structure Analysis of Motor Themes
The motor themes have an important role regarding the shape and future of the research field because they correspond to the key topics to everyone interested in the subject. Therefore, they can be considered as strategic themes in order to develop the field of data mining in healthcare. The eight motor themes are discussed below, and they are displayed below in Figure 5 together with the network structure of each theme.

Thematic network structure of mining in healthcare (1995–July 2020). ( a ) The cluster ‘NEURAL-NETWORKS’. ( b ) The cluster ‘CANCER’. ( c ) The cluster ‘ELECTRONIC-HEALTH-RECORDS’. ( d ) The cluster ‘DIABETES-MELLITUS’. ( e ) The cluster ‘BREAST-CANCER’. ( f ) The cluster ‘ALZHEIMER’S DISEASE’. ( g ) The cluster ‘DEPRESSION’. ( h ) The cluster ‘RANDOM-FOREST’.
4.2.1. Neural Network (a)
The cluster ‘NEURAL-NETWORKS’ ( Figure 5 a) is the first ranked in terms of core documents, h-index, centrality, and density. The ‘NEURAL-NETWORKS’ cluster is strongly influenced by subthemes related to data science algorithms, such as ‘SUPPORT-VECTOR-MACHINE’, ‘DECISION-TREE’, among others. This network represents the use of data mining techniques to detect patterns and find important information correlated to patient health and medical diagnosis. A reasonable explanation for this network might be related to the high number of studies which conducted benchmarking of neural networks with other techniques to evaluate performance (e.g., resource usage, efficiency, accuracy, scalability, etc.) [ 40 , 41 , 42 ]. Besides, the significant size of the cluster ‘MACHINE-LEARNING’ is expected since neural networks is a type of machine learning. On the other hand, the subtheme ‘HEART-DISEASE’ stands out as the single disease in this network, which can be justified by the high number of researches with the goal to apply data mining to support decision-making in heart disease treatment and diagnosis.
4.2.2. Cancer (b)
The cluster ‘CANCER’ ( Figure 5 b) is the second ranked in terms of core documents, h-index, and density. On the other hand, it is the first in terms of citations (5810). This cluster is highly influenced by the subthemes related to the studies of cancer genes mutations, such as ‘BIOMAKERS’, ‘GENE-EXPRESSION’, among others. The use of data mining techniques has been attracting attention and efforts from academics in order to help solve problems in the field of oncology. Cancer is known as the disease that kills the most people in the 21st century due to various environmental pollutions, food pesticides and additives [ 14 ], eating habits, mental health, among others. Thus, controlling any form of cancer is a global strategy and can be enhanced by applying data mining techniques. Furthermore, the subtheme ‘PROSTATE-CANCER’ highlights that the most efforts of data mining applications focused on prostate cancer’s studies. Prostate cancer is the most common cancer in men. Although the benefits of traditional clinical exams for screening (digital rectal examination, the prostate-specific antigen and blood test and transrectal ultrasound), there is still a lack in terms of efficacy to reduce mortality with the use of such tests [ 43 ]. In this sense, data mining may be a suitable solution since it has been used in bioinformatics analyses to understand prostate cancer mutation [ 44 , 45 ] and uncover useful information that can be used for diagnoses and future prognostic tests which enhance both patients and clinical decision-making [ 46 ].
4.2.3. Electronic Health Records (HER—c)
The cluster ‘ELECTRONIC-HEALTH-RECORDS’ ( Figure 5 c) represents the concept in which patient’s health data are stored. Such data are continuously increasing over time, thereby creating a large amount of data (big data) which has been used as input (EHR) for healthcare decision support systems to enhance clinical decision-making. The clusters ‘NATURAL-LANGUAGE-PROCESSING’ and ‘TEXT MINING’ highlight that these mining techniques are the most frequently used with data mining in healthcare. Another pattern that must be highlighted is the considerable density among the clusters ‘SIGNAL-DETECTION’ and ‘PHARMACOVIGILANCE’ which represents the use of data mining to depict a broad range of adverse drug effects and to identify signals almost in real-time by using EHR [ 47 , 48 ]. Besides, the cluster ‘MISSING-DATA’ is related to studies focused on the challenge regarding to incomplete EHR and missing data in healthcare centers, which compromise the performance of several prediction models [ 49 ]. In this sense, techniques to handle missing data have been under improvement in order to move forward with the accurate prediction based on medical data mining applications [ 50 ].
4.2.4. Diabetes Mellitus (DM—d)
Nowadays, DM is one of the most frequent endocrine disorders [ 51 ] and affected more than 450 million people worldwide in 2017 and is expected to grow to 693 million by the year 2045. The same applies for the 850 billion dollars spent just in 2017 by the health sector [ 52 ]. The cluster ‘DIABETES-MELLITUS’ ( Figure 5 d) has a strong association with the risk factor subtheme group (e.g., ‘INSULIN-RESISTENCE’, ‘OBESITY’, ‘BODY-MASS-INDEX’, ‘CARDIOVASCULAR-DISEASE’, and ‘HYPERTENSION’). However, the obesity (cluster ‘OBESITY’) is the major risk factor related to DM, particularly in Type 2 Diabetes (T2D) [ 51 ]. T2D shows a prevalence of 90% of worldwide diabetic patients when compared with T1D and T3D, mainly characterized by insulin resistance [ 51 ]. Thus, this might justify the presence of the clusters ‘TYPE-2-DIABETES’ and ‘INSULIN-RESISTANCE’ which seems to be highly developed by data mining academics and practitioners. The massive number of researches into all facets of DM has led to the formation of huge volumes of EHR, in which the mostly applied data mining technique is the association rules technique. It is used to identify associations among risk factors [ 51 ], thusly justifying the appearance of the cluster ‘ASSOCIATION-RULES’.
4.2.5. Breast Cancer (e)
The cluster ‘BREAST-CANCER’ ( Figure 5 e) presents the most prevalent type of cancer affecting approximately 12.5% of women worldwide [ 53 , 54 ]. The cluster ‘OVEREXPRESSION’ and ‘METASTASIS’ highlights the high number of studies using data mining to understand the association of overexpression of molecules (e.g., MUC1 [ 54 ], TRIM29 [ 55 ], FKBP4 [ 56 ], etc.) with breast cancer metastasis. Such overexpression of molecules also appears in other forms of cancers, justifying the group of subthemes: ‘LUNG CANCER’, ‘GASTRIC-CANCER’, ‘OVARIAN-CANCER’, and ‘COLORECTALCANCER’. Moreover, the cluster ‘IMPUTATION’ highlight efforts to develop imputation techniques (data missingness) for breast cancer record analysis [ 57 , 58 ]. Besides, the application of data mining to depict breast cancer characteristics and their causes and effects has been highly supported by ‘MICROARRAY-DATA’ [ 59 , 60 ], ‘PATHWAY’ [ 61 ], and ‘COMPUTER-AIDED-DIAGNOSIS’ [ 62 ].
4.2.6. Alzheimer’s Disease (AD—f)
The cluster ‘ALZHEIMER’S DISEASE’ ( Figure 5 f) is highly influenced by subthemes related to diseases, such as ‘DEMENTIA’ and ‘PARKINSON’S-DISEASE’. This co-occurrence happens because the AD is a neurodegenerative illness which leads to dementia and Parkinson’s disease. Studies show that the money spent on AD in 2015 was about $828 billion [ 63 ]. In this sense, data mining has been widely used with ‘GENOME-WIDE-ASSOCIATION’ techniques in order to identify genes related to the AD [ 64 , 65 ] and prediction of AD by using data mining in ‘MRI’ Brain images [ 66 , 67 ]. The cluster ‘NF-KAPPA-B’ highlights the efforts to identify associations of NF-κB (factor nuclear kappa B) with AD by using data mining techniques which can be used to advance anti-drug developments [ 68 ].
4.2.7. Depression (g)
The cluster ‘DEPRESSION’ ( Figure 5 g) presents a common disease which affects over 260 million people. In the worst case, it can lead to suicide which is the second leading cause of death in young adults. The cluster ‘DEPRESSION’ is a highly associated cluster. Its connections mostly represent the subthemes that have been the research focus of data mining applications [ 69 ]. The connection between both the sub theme ‘SOCIAL-MEDIA’ and ‘ADOLESCENTS’, especially in times of social isolation, are extremely relevant to help identify early symptoms and tendencies among the population [ 70 ]. Furthermore, the presence of the ‘COMORBIDITY’ and ‘SYMPTONS’ is not surprising given knowledge discovery properties of the data mining field could provide significant insights into the etiology of depression [ 71 ].
4.2.8. Random Forest (h)
An ensemble learning method that is used in this study is the last cluster approach, which, among other things, is used for classification. The presence of the ‘BAYESIAN-NETWORK’ subtheme, supported by the connection between both and the ‘INFERENCE’, might represent another alternative to which the applications in data mining using random forest are benchmarked against [ 72 ]. Since the ‘RANDOM-FOREST’ ( Figure 5 h) cluster has barely passed the threshold from a basic and transversal theme to a motor theme, the works developed under this cluster are not yet as interconnected as the previous one. Thus, the theme with the most representativeness is the ‘AIR-POLLUTION’ in conjunction with ‘POLLUTION’, where studies have been performed in order to obtain ‘RISK-ASSESSMENT’ through the exploration of the knowledge hidden in large databases [ 73 ].
4.3. Thematic Evolution Structure Analysis
The Computer Science’s themes related to data mining and the medical research concepts, depicted, respectively, in the grey and blue areas of the thematic evolution diagram ( Figure 6 ), demonstrates the evolution of the research field over the different sub-periods addressed in this study. In this way, each individual theme relevance is illustrated through its cluster size as well as with its relationships throughout the different sub-periods. Thus, in this section, an analysis of the different trends on themes will be presented to give a brief insight into the factors that might have influenced its evolution. Furthermore, the proceeding analysis will be split into two thematic areas where, firstly, the grey area (practices and techniques related to data mining in healthcare) will be discussed followed by the blue one (health concepts and disease supported by data mining).

Thematic evolution structure of mining in healthcare (1995–July 2020).
4.3.1. Practices and Techniques Related to Data Mining in Healthcare
The cluster ‘KNOWLEDGE-DISCOVERY’ ( Figure 6 , 1995–2012), often known as a synonym for data mining, provides a broader view of the field differing in this way from the algorithm focused theme, that is data mining, where its appearance and, later in the third period, its fading could provide a first insight into the overall evolution of the data mining papers applied to healthcare. The occurrence of the cluster knowledge discovery in the first two periods could demonstrate the focus of the application of the data mining techniques in order to classify and predict conditions in the medical field. This gives rise to a competition with early machine learning techniques that could be potentially evidenced through the presence of the cluster ‘NEURAL-NETWORK’, which the data mining techniques could probably be benchmarked against. The introduction of the ‘FEATURE-SELECTION’, ‘ARTIFICIAL-INTELLIGENCE’, and ‘MACHINE-LEARNING’ clusters together with the fading of ‘KNOWLEDGE-DISCOVERY’ could imply the occurrence of a disruption of the field in the third sub-period that has led to a change in the perspective on the studies.
One instance that could represent such a disruption could have been a well-known paper published by Alex Krizhevsky, Ilya Sutskever, and Geoffrey Hinton [ 74 ], where a novel technique in neural networks was firstly applied to a major image recognition competition. A vast advantage over the other algorithms that have been used was obtained. The connection between the work previously mentioned and its impact on the data mining on healthcare research could be majorly supported by the disappearance of the cluster ‘IMAGE- MINING’ of the second sub-period which has no connections further on. Furthermore, the presence of the clusters ‘MACHINE-LEARNING’, ‘ARTIFICIAL-INTELLIGENCE’, ‘SUPPORT-VECTOR-MACHINES’, and ‘LOGISTIC-REGRESSION’ may be the evidence of a shift of focus on the data mining community for health care where, besides attempting to compete with machine learning algorithms, they are now striving to further improve the results previously obtained with machine learning through data mining. Moreover, given the presence of the colossal feature selection cluster, which circumscribes algorithms that enhance classification accuracy through a better selection of parameters, this trend could be given credence in consequence of its presence since it may be encompassing publications from the formerly stated clusters.
Although still small, the presence of the cluster ‘SECURITY’ in the last sub-period ( Figure 6 , 2013–2020) is, at the very least, relevant given the sensitive data that is handled in the medical space, such as patient’s history and diseases. Above all, the recent leaks of personal information have devised an ever-increasing attention to this topic focusing on, among other things, the de-identification of the personal information [ 75 , 76 , 77 ]. These kind of security processes allow, among others, data mining researchers to make use of the vast sensitive information that is stored in hospitals without any linkage that could associate a person to the data. For instance, the MIMIC Critical Care Database [ 78 ], an example of a de-identified database, has been allowing further research into many diseases and conditions in a secure way that would otherwise have been extremely impaired due to data limitations.
4.3.2. Health Concepts and Disease Supported by Data Mining
The cluster ‘GENE-EXPRESSION’ stands out in the first period and second period ( Figure 6 , 1995–2012) of medical research concepts and establishes strong co-occurrence with the cluster ‘CANCER’ in the third sub-period. This link can be explained by research involving the microarray technology, which makes it possible to detect deletions and duplications in the human genome by analyzing the expression of thousands of genes in different tissues. It is also possible to confirm the importance of genetic screening not only for cancer, but for several diseases, such as ‘ALZHEIMER’ and other brain disorders, thereby assisting in preventive medicine and enabling more efficient treatment plans [ 79 ]. For example, a research was carried out to analyze complex brain disorders such as schizophrenia from expression gene microarrays [ 80 ].
Sequencing technologies have undergone major improvements in recent decades to determine evolutionary changes in genetic, epigenetic mechanisms, and in the ‘MOLECULAR-CLASSIFICATION’, a topic that gained prominence as a cluster in the first period. An example of this can be found in a study published in 2010 which combined a global optimization algorithm called Dongguang Li (DGL) with cancer diagnostic methods based on gene selection and microarray analysis. It performed the molecular classification of colon cancers and leukemia and demonstrated the importance of machine learning, data mining, and good optimization algorithms for analyzing microarray data in the presence of subsets of thousands of genes [ 81 ].
The cluster ‘PROSTATE-CANCER’ in the second period ( Figure 6 , 2004–2012) presents a higher conceptual nexus to ‘MOLECULAR-CLASSIFICATION’ in the first sub-period and the same happens with clusters, such as ‘METASTASIS’, ‘BREAST-CANCER’, and ‘ALZHEIMER’, which appear more recently in the third sub-period. The significant increase in the incidence of prostate cancer in recent years results in the need for greater understanding of the disease in order to increase patient survival, since prostate cancer with metastasis was not well explored, despite having a survival rate much smaller compared to the early stages. In this sense, the understanding of age-specific survival of patients with prostate cancer in a hospital in using machine learning started to gain attention by academics and highlighted the importance of knowing survival after diagnosis for decision making and better genetic counseling [ 82 ]. In addition, the relationship between prostate cancer and Alzheimer’s disease is explained by the fact that the use of androgen deprivation therapy, used to treat prostate cancer, is associated with an increased risk of Alzheimer’s disease and dementia [ 81 ]. Therefore, the risks and benefits of long-term exposure to this therapy must be weighed. Finally, the relationship between prostate cancer and breast cancer in the thematic evolution can be explained due to the fact that studies are showing that men with a family history of breast cancer have a 21% higher risk of developing prostate cancer, including lethal disease [ 83 ].
The cluster ‘PHARMACOVIGILANCE’ appears in the second sub-period ( Figure 6 , 2004–2012) showing a strong co-occurrence with clusters of the third sub-period: ‘ADVERSE-DRUGS-REACTIONS’ and ‘ELECTRONIC-HEALTH-RECORDS’. In recent years, data-mining algorithms have stood out for their usefulness in detecting and screening patients with potential adverse drug reactions and, consequently, they have become a central component of pharmacovigilance, important for reducing the morbidity and mortality associated with the use of medications [ 48 ]. The importance of electronic medical records for pharmacovigilance is evident, which act as a health database and enable drug safety assessors to collect information. In addition, such medical records are also essential to optimize processes within health institutions, ensure more safety of patient data, integrate information, and facilitate the promotion of science and research in the health field [ 84 ]. These characteristics explain the large number of studies of ‘ELECTRONIC-HEALTH-RECORDS’ in the third sub-period and the growth of this theme in recent years, since the world has started to introduce electronic medical records, although currently there are few institutions that still use physical medical records.
The ‘DEPRESSION’ appears in the second sub-period ( Figure 6 , 2004–2012) and remains as a trend in the third sub-period with a significant increase in publications on the topic. It is known that this disease is numerous and is increasing worldwide, but that it still has many stigmas in its treatment and diagnosis. Globalization and the contemporary work environment [ 85 ] can be explanatory factors for the increase in the theme from the 2000s onwards and the COVID-19 pandemic certainly contributed to the large number of articles on mental health published in 2020. In this context, improving the detection of mental disorders is essential for global health, which can be enhanced by applying data mining to quantitative electroencephalogram signals to classify between depressed and healthy people and can act as an adjuvant clinical decision support to identify depression [ 69 ].
5. Conclusions
In this research, we have performed a BPNA to depict the strategic themes, the thematic network structure, and the thematic evolution structure of the data mining applied in healthcare. Our results highlighted several significant pieces of information that can be used by decision-makers to advance the field of data mining in healthcare systems. For instance, our results could be used by editors from scientific journals to enhance decision-making regarding special issues and manuscript review. From the same perspective, healthcare institutions could use this research in the recruiting process to better align the position needs to the candidate’s qualifications based on the expanded clusters. Furthermore, Table 2 presents a series of authors whose collaboration network may be used as a reference to identify emerging talents in a specific research field and might become persons of interest to greatly expand a healthcare institution’s research division. Additionally, Table 3 and Table 4 could also be used by researchers to enhance the alignment of their research intentions and partner institutions to, for instance, encourage the development of data mining applications in healthcare and advance the field’s knowledge.
The strategic diagram ( Figure 4 ) depicted the most important themes in terms of centrality and density. Such results could be used by researchers to provide insights for a better comprehension of how diseases like ‘CANCER’, ‘DIABETES-MELLITUS’, ‘ALZHEIMER’S-DISEASE’, ‘BREAST-CANCER’, ‘DEPRESSION’, and ‘CORONARY-ARTERY-DISEASE’ have made use of the innovations in the data mining field. Interestingly, none of the clusters have highlighted studies related to infectious diseases, and, therefore, it is reasonable to suggest the exploration of data mining techniques in this domain, especially given the global impact that the coronavirus pandemic has had on the world.
The thematic network structure ( Figure 5 ) demonstrates the co-occurrences among clusters and may be used to identify hidden patterns in the field of research to expand the knowledge and promote the development of scientific insights. Even though exhaustive research of the motor themes and their subthemes has been performed in this article, future research must be conducted in order to depict themes from the other quadrants (Q2, Q3, and Q4), especially emerging and declining themes, to bring to light relations between the rise and decay of themes that might be hidden inside the clusters.
The thematic evolution structure showed how the field is evolving over time and presented future trends of data mining in healthcare. It is reasonable to predict that clusters such as ‘NEURAL-NETWORKS’, ‘FEATURE-SELECTION’, ‘EHR’ will not decay in the near future due to their prevalence in the field and, most likely, due to the exponential increase in the amount of patient health that is being generated and stored daily in large data lakes. This unprecedented increase in data volume, which is often of dubious quality, leads to great challenges in the search for hidden information through data mining. Moreover, as a consequence of the ever-increasing data sensitivity, the cluster ‘SECURITY’, which is related to the confidentiality of the patient’s information, is likely to remain growing during the next years as government and institutions further develop structures, algorithms, and laws that aim to assure the data’s security. In this context, blockchain technologies specifically designed to ensure integrity and publicity of de-identified, similarly as it is done by the MIMIC-III (Medical Information Mart for Intensive Care III) [ 78 ], may be crucial to accelerate the advancement of the field by providing reliable information for health researchers across the world. Furthermore, future researches should be conducted in order to understand how these themes will behave and evolve during the next years, and interpret the cluster changes to properly assess the trends here presented. These results could also be used as teaching material for classes, as it provides strategic intelligence applications and the field’s historical data.
In terms of limitations, we used the WoS database since it has index journals with high JIF. Therefore, we suggest to analyze other databases, such as Scopus, PubMed, among others in future works. Besides, we used the SciMAT to perform the analysis and other bibliometric software, such as VOS viewer, Cite Space, Sci2tool, etc., could be used to explore different points of view. Such information will support this study and future works to advance the field of data mining in healthcare.
Author Contributions
Conceptualization, M.L.K., L.B.F., L.P.C.T. and N.L.B.; Data curation, L.B.F.; Formal analysis, L.B.F., B.R., and P.H.U.; Funding acquisition, N.L.B.; Investigation, M.L.K., L.B.F., L.P.C.T. and M.K.S.; Methodology, L.B.F.; Project administration, L.B.F., N.L.B. and L.P.C.T.; Resources, N.L.B.; Supervision, L.B.F., N.L.B. and L.P.C.T.; Validation, N.L.B. and L.P.C.T.; Visualization, N.L.B.; Writing—original draft, L.B.F. and N.L.B.; Writing—review & editing, N.L.B. All authors have read and agreed to the published version of the manuscript.
This study was financed in part by the Coordenação de Aperfeiçoamento de Pessoal de Nível Superior—Brazil (CAPES)—Finance Code 001, and in part by the Brazilian Ministry of Health. N.L.B. is partially supported by the CIHR 2019 Novel Coronavirus (COVID-19) rapid research program.
Institutional Review Board Statement
Informed consent statement, data availability statement, conflicts of interest.
The authors declare no conflict of interest.
Publisher’s Note: MDPI stays neutral with regard to jurisdictional claims in published maps and institutional affiliations.
Thank you for visiting nature.com. You are using a browser version with limited support for CSS. To obtain the best experience, we recommend you use a more up to date browser (or turn off compatibility mode in Internet Explorer). In the meantime, to ensure continued support, we are displaying the site without styles and JavaScript.
- View all journals
Data mining articles from across Nature Portfolio
Data mining is the process of extracting potentially useful information from data sets. It uses a suite of methods to organise, examine and combine large data sets, including machine learning, visualisation methods and statistical analyses. Data mining is used in computational biology and bioinformatics to detect trends or patterns without knowledge of the meaning of the data.
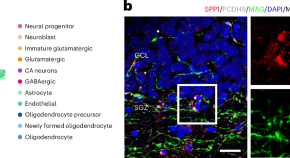
A cellular reference atlas across human brain regions
We present the Brain Cell Atlas, integrating single-cell transcriptomes of 26 million cells from 70 human and 103 mouse studies, covering 14 major brain regions. This atlas takes advantage of the integration of big data, enabling the discovery of putative neural progenitors in adults and microglial regional variations.
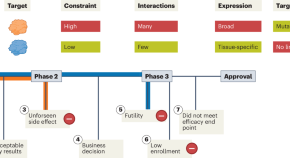
Stopped clinical trials give evidence for the value of genetics
A new analysis of clinical trials that were stopped early generates insights on the role of genetics in drug development and provides a new resource for researchers aiming to improve success rates of clinical trials.
Latest Research and Reviews
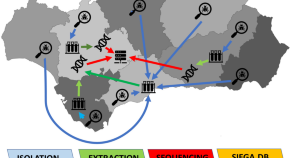
The integrated genomic surveillance system of Andalusia (SIEGA) provides a One Health regional resource connected with the clinic
- Carlos S. Casimiro-Soriguer
- Javier Pérez-Florido
- Joaquin Dopazo
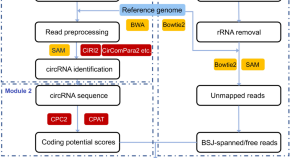
An updated resource for the detection of protein-coding circRNA with CircProPlus
- Yunchang Liu
- Yundai Chen
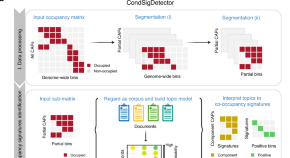
Decoding the genomic landscape of chromatin-associated biomolecular condensates
The authors develop CondSigDetector, a computational framework designed to detect condensate-like chromatin-associated protein co-occupancy signatures, to predict genomic loci and component proteins of distinct chromatin-associated biomolecular condensates.
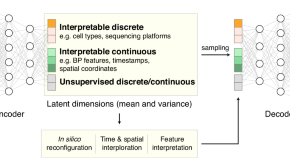
Building a learnable universal coordinate system for single-cell atlas with a joint-VAE model
UniCoord is a joint-VAE model designed to create a universal coordinate system for singlecell transcriptomic data, capturing major heterogeneities in a lower-dimensional latent space to enhance cell annotation and data augmentation.
- Haoxiang Gao
- Xuegong Zhang
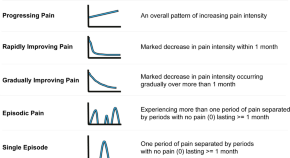
Development, validation and use of custom software for the analysis of pain trajectories
- M. R. van Ittersum
- A. de Zoete
- P. McCarthy
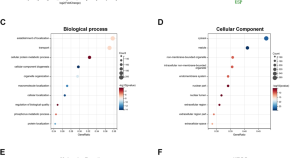
Comprehensive analysis identifies ubiquitin ligase FBXO42 as a tumor-promoting factor in neuroblastoma
- Jianwu Zhou
News and Comment
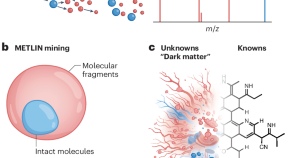
The hidden impact of in-source fragmentation in metabolic and chemical mass spectrometry data interpretation
- Martin Giera
- Aries Aisporna
- Gary Siuzdak
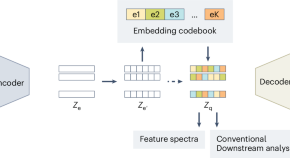
Discrete latent embeddings illuminate cellular diversity in single-cell epigenomics
CASTLE, a deep learning approach, extracts interpretable discrete representations from single-cell chromatin accessibility data, enabling accurate cell type identification, effective data integration, and quantitative insights into gene regulatory mechanisms.
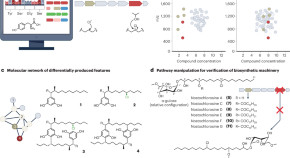
Discovering cryptic natural products by substrate manipulation
Cryptic halogenation reactions result in natural products with diverse structural motifs and bioactivities. However, these halogenated species are difficult to detect with current analytical methods because the final products are often not halogenated. An approach to identify products of cryptic halogenation using halide depletion has now been discovered, opening up space for more effective natural product discovery.
- Ludek Sehnal
- Libera Lo Presti
- Nadine Ziemert
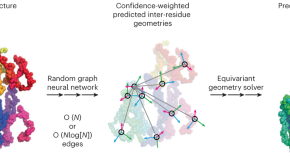
Chroma is a generative model for protein design
- Arunima Singh
Quick links
- Explore articles by subject
- Guide to authors
- Editorial policies

- Corpus ID: 16027966
Data-Mining Research in Education
- Jiechao Cheng
- Published in arXiv.org 28 March 2017
- Computer Science, Education
7 Citations
Analyzing student's learning interests in the implementation of blended learning using data mining, educational fuzzy data-sets and data mining in a linear fuzzy real environment, intelligent academic specialties selection in higher education for ukrainian entrants: a recommendation system, survey on predicting performance of an employee using data mining techniques, the significance of investigating the relationship between mathematical thinking and computational thinking using linguistic aspects, classification of imbalanced banking dataset using dimensionality reduction, a study of feature selection by fuzzy curves and fuzzy surface, 38 references, data mining in education, introduction to the special section on educational data mining, enhancing teaching and learning through educational data mining and learning analytics: an issue brief, mining the student assessment data: lessons drawn from a small scale case study, the state of educational data mining in 2009: a review and future visions., educational data mining: a survey from 1995 to 2005, a collaborative educational association rule mining tool, a web-based intelligent report e-learning system using data mining techniques, data mining for student retention management, examining students' online interaction in a live video streaming environment using data mining and text mining, related papers.
Showing 1 through 3 of 0 Related Papers
IEEE Account
- Change Username/Password
- Update Address
Purchase Details
- Payment Options
- Order History
- View Purchased Documents
Profile Information
- Communications Preferences
- Profession and Education
- Technical Interests
- US & Canada: +1 800 678 4333
- Worldwide: +1 732 981 0060
- Contact & Support
- About IEEE Xplore
- Accessibility
- Terms of Use
- Nondiscrimination Policy
- Privacy & Opting Out of Cookies
A not-for-profit organization, IEEE is the world's largest technical professional organization dedicated to advancing technology for the benefit of humanity. © Copyright 2024 IEEE - All rights reserved. Use of this web site signifies your agreement to the terms and conditions.
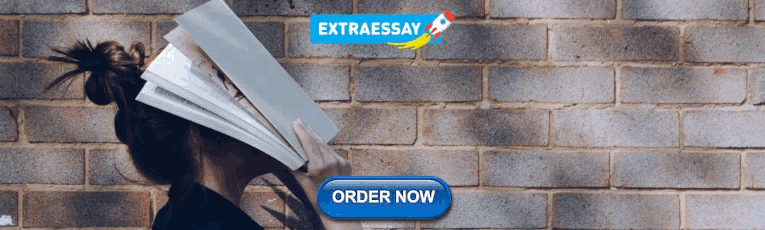
IMAGES
COMMENTS
Data mining is also known as Knowledge Discovery in Database (KDD). It is also defined as the process which includes extracting the interesting, interpretable and useful information from the raw data. There are different sources that generate raw data in very large amount. This is the main reason the applications of data mining are increasing rapidly. This paper reviews data mining techniques ...
Address: 20, Myasnitskaya Street, Moscow 101000, Russia. Abstract. This work analyzes the intellectual structure of data mining as a scientific discipline. T o do this, we use. topic analysis ...
DMT. Data mining techniques are applied with respect to different. aspects of data mining as data obtained from diff erent sources. can be different and asyn chronous. Data mining is a v ast field ...
PDF | Abstract: An in-depth analysis of the challenges and developments in the data mining industry is the aim of the study. ... the work prese nted in this research paper: ... CSUR), 2017. 50(3 ...
Secondly, we note that research on data mining methodologies has grown substantially since 2007, an observation supported by the 3-year and 10-year constructed mean trendlines. In particular, the number of publications have roughly tripled over past decade hitting all time high with 24 texts released in 2017. ... An interesting paper was ...
Data Mining and Knowledge Discovery is a leading technical journal focusing on the extraction of information from vast databases. Publishes original research papers and practice in data mining and knowledge discovery. Provides surveys and tutorials of important areas and techniques. Offers detailed descriptions of significant applications.
Kai Shu, Amy Sliva, Suhang Wang, Jiliang Tang, Huan Liu. View a PDF of the paper titled Fake News Detection on Social Media: A Data Mining Perspective, by Kai Shu and 4 other authors. Social media for news consumption is a double-edged sword. On the one hand, its low cost, easy access, and rapid dissemination of information lead people to seek ...
Educational Data Science (EDS) is defined as the use of data gathered from educational environments/settings for solving educational problems (Romero & Ventura, 2017). Data science is a concept to unify statistics, data analysis, machine learning and their related methods. This survey is an updated and improved version of the previous one ...
ACM SIGKDD is pleased to announce the winners of the best paper awards for 2017.. Research Track. BEST PAPER AWARD. Winner. Accelerating Innovation Through Analogy Mining Tom Hope (Hebrew University of Jerusalem);Joel Chan (Carnegie Mellon University);Aniket Kittur (Carnegie Mellon University);Dafna Shahaf (Hebrew University of Jerusalem)
This paper presents a rigorous methodological analysis of the applied research published in academic literature, on the application of data mining (DM) for project management (PM). ... Haksoz, C., Pakter, S., Ulun, S., 2017. Wind Turbine Accidents: A Data Mining Study. IEEE Systems Journal, 11(3), 1567--1578. Crossref. Google Scholar [9 ...
Applying data mining in education also known as educational data mining (EDM), which enables to better understand how students learn and identify how improve educational outcomes. Present paper is designed to justify the capabilities of data mining approaches in the filed of education. The latest trends on EDM research are introduced in this ...
Data mining research has been significantly motivated by and benefited from real-world applications in novel domains. This special issue was proposed and e ... He is an ACM distinguished scientist and has been honored by the ICDM-2011 Best Research Paper Award, the 2017 IEEE ICDM Outstanding Service Award, and the 2018 Ram Charan Management ...
Payment Options. View Purchased Documents. Profile Information. Communications Preferences. Profession and Education. Technical interests. Need Help? US & Canada: +1 800 678 4333. Worldwide: +1 732 981 0060.
Explore the latest full-text research PDFs, articles, conference papers, preprints and more on DATA MINING. Find methods information, sources, references or conduct a literature review on DATA MINING
The SPC generated their recommendations on the paper based on the PC members' review and ensuing discussion. Finally, we deliberated on the recommendations, reviews and discussions, and accepted 93 excellent papers that together cover a wide range of areas in the frontier of Data Mining research and are arranged into 18 technical sessions.
2000-2017: Analysis of the evolution of emerging technologies (e.g., data mining, machine learning, among others) in cancer using CiteSpace software. ... Table 5 presents the most important WoS subject research fields of data mining in healthcare from 1995 to July 2020. Computer Science Artificial Intelligence is the first ranked with 768 ...
Data mining is the process of extracting potentially useful information from data sets. It uses a suite of methods to organise, examine and combine large data sets, including machine learning ...
Volume 15, 2017, Pages 104-116. ... DM Through Machine Learning and Data Mining. This section presents key papers of the study. ... In the present study, the recent literature was reviewed with respect to applications of machine learning and data mining methods in Diabetes research. The first sections describe briefly the two main research ...
Data-Mining Research in Education. Jiechao Cheng. Published in arXiv.org 28 March 2017. Computer Science, Education. TLDR. Several specific algorithms, methods, applications and gaps in the current literature and future insights are discussed here, which enables to better understand how students learn and identify how improve educational outcomes.
One such preprocessing algorithm in EDM is clustering. Many studies on EDM have focused on the application of various data mining algorithms to educational attributes. Therefore, this paper provides over three decades long (1983-2016) systematic literature review on clustering algorithm and its applicability and usability in the context of EDM.
A variety of researches focusing on knowledge view, technique view, and application view can be found in the literature. However, no previous effort has been made to review the different views of data mining in a systematic way, especially in nowadays big data [5-7]; mobile internet and Internet of Things [8-10] grow rapidly and some data mining researchers shift their attention from data ...
Diabetes is a chronic and non -communicable disease that. destabilizes the normal control of blood glucose. concentration in the body. The blood glucose concentration is. usually regulated by two ...
Data mining applications are utilized in many banking sectors for client segmentation and productivity, credit scores and authorization, predicting payment default, advertising, detecting fake transactions, etc. This paper presents a general idea about the model of Data Mining techniques and diverse cyber crimes in banking applications. It also provides an inclusive survey of competent and ...