We’re fighting to restore access to 500,000+ books in court this week. Join us!
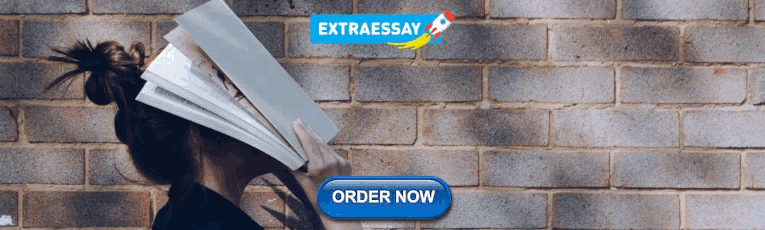
Internet Archive Audio
- This Just In
- Grateful Dead
- Old Time Radio
- 78 RPMs and Cylinder Recordings
- Audio Books & Poetry
- Computers, Technology and Science
- Music, Arts & Culture
- News & Public Affairs
- Spirituality & Religion
- Radio News Archive
- Flickr Commons
- Occupy Wall Street Flickr
- NASA Images
- Solar System Collection
- Ames Research Center
- All Software
- Old School Emulation
- MS-DOS Games
- Historical Software
- Classic PC Games
- Software Library
- Kodi Archive and Support File
- Vintage Software
- CD-ROM Software
- CD-ROM Software Library
- Software Sites
- Tucows Software Library
- Shareware CD-ROMs
- Software Capsules Compilation
- CD-ROM Images
- ZX Spectrum
- DOOM Level CD

- Smithsonian Libraries
- FEDLINK (US)
- Lincoln Collection
- American Libraries
- Canadian Libraries
- Universal Library
- Project Gutenberg
- Children's Library
- Biodiversity Heritage Library
- Books by Language
- Additional Collections
- Prelinger Archives
- Democracy Now!
- Occupy Wall Street
- TV NSA Clip Library
- Animation & Cartoons
- Arts & Music
- Computers & Technology
- Cultural & Academic Films
- Ephemeral Films
- Sports Videos
- Videogame Videos
- Youth Media
Search the history of over 866 billion web pages on the Internet.
Mobile Apps
- Wayback Machine (iOS)
- Wayback Machine (Android)
Browser Extensions
Archive-it subscription.
- Explore the Collections
- Build Collections
Save Page Now
Capture a web page as it appears now for use as a trusted citation in the future.
Please enter a valid web address
- Donate Donate icon An illustration of a heart shape
Case study research : design and methods
Bookreader item preview, share or embed this item, flag this item for.
- Graphic Violence
- Explicit Sexual Content
- Hate Speech
- Misinformation/Disinformation
- Marketing/Phishing/Advertising
- Misleading/Inaccurate/Missing Metadata
obscured text on back cover
![[WorldCat (this item)] [WorldCat (this item)]](https://archive.org/images/worldcat-small.png)
plus-circle Add Review comment Reviews
1,859 Previews
31 Favorites
Better World Books
DOWNLOAD OPTIONS
No suitable files to display here.
PDF access not available for this item.
IN COLLECTIONS
Uploaded by station16.cebu on December 23, 2021
SIMILAR ITEMS (based on metadata)
Have a language expert improve your writing
Run a free plagiarism check in 10 minutes, generate accurate citations for free.
- Knowledge Base
Methodology
- What Is a Case Study? | Definition, Examples & Methods
What Is a Case Study? | Definition, Examples & Methods
Published on May 8, 2019 by Shona McCombes . Revised on November 20, 2023.
A case study is a detailed study of a specific subject, such as a person, group, place, event, organization, or phenomenon. Case studies are commonly used in social, educational, clinical, and business research.
A case study research design usually involves qualitative methods , but quantitative methods are sometimes also used. Case studies are good for describing , comparing, evaluating and understanding different aspects of a research problem .
Table of contents
When to do a case study, step 1: select a case, step 2: build a theoretical framework, step 3: collect your data, step 4: describe and analyze the case, other interesting articles.
A case study is an appropriate research design when you want to gain concrete, contextual, in-depth knowledge about a specific real-world subject. It allows you to explore the key characteristics, meanings, and implications of the case.
Case studies are often a good choice in a thesis or dissertation . They keep your project focused and manageable when you don’t have the time or resources to do large-scale research.
You might use just one complex case study where you explore a single subject in depth, or conduct multiple case studies to compare and illuminate different aspects of your research problem.
Research question | Case study |
---|---|
What are the ecological effects of wolf reintroduction? | Case study of wolf reintroduction in Yellowstone National Park |
How do populist politicians use narratives about history to gain support? | Case studies of Hungarian prime minister Viktor Orbán and US president Donald Trump |
How can teachers implement active learning strategies in mixed-level classrooms? | Case study of a local school that promotes active learning |
What are the main advantages and disadvantages of wind farms for rural communities? | Case studies of three rural wind farm development projects in different parts of the country |
How are viral marketing strategies changing the relationship between companies and consumers? | Case study of the iPhone X marketing campaign |
How do experiences of work in the gig economy differ by gender, race and age? | Case studies of Deliveroo and Uber drivers in London |
Here's why students love Scribbr's proofreading services
Discover proofreading & editing
Once you have developed your problem statement and research questions , you should be ready to choose the specific case that you want to focus on. A good case study should have the potential to:
- Provide new or unexpected insights into the subject
- Challenge or complicate existing assumptions and theories
- Propose practical courses of action to resolve a problem
- Open up new directions for future research
TipIf your research is more practical in nature and aims to simultaneously investigate an issue as you solve it, consider conducting action research instead.
Unlike quantitative or experimental research , a strong case study does not require a random or representative sample. In fact, case studies often deliberately focus on unusual, neglected, or outlying cases which may shed new light on the research problem.
Example of an outlying case studyIn the 1960s the town of Roseto, Pennsylvania was discovered to have extremely low rates of heart disease compared to the US average. It became an important case study for understanding previously neglected causes of heart disease.
However, you can also choose a more common or representative case to exemplify a particular category, experience or phenomenon.
Example of a representative case studyIn the 1920s, two sociologists used Muncie, Indiana as a case study of a typical American city that supposedly exemplified the changing culture of the US at the time.
While case studies focus more on concrete details than general theories, they should usually have some connection with theory in the field. This way the case study is not just an isolated description, but is integrated into existing knowledge about the topic. It might aim to:
- Exemplify a theory by showing how it explains the case under investigation
- Expand on a theory by uncovering new concepts and ideas that need to be incorporated
- Challenge a theory by exploring an outlier case that doesn’t fit with established assumptions
To ensure that your analysis of the case has a solid academic grounding, you should conduct a literature review of sources related to the topic and develop a theoretical framework . This means identifying key concepts and theories to guide your analysis and interpretation.
There are many different research methods you can use to collect data on your subject. Case studies tend to focus on qualitative data using methods such as interviews , observations , and analysis of primary and secondary sources (e.g., newspaper articles, photographs, official records). Sometimes a case study will also collect quantitative data.
Example of a mixed methods case studyFor a case study of a wind farm development in a rural area, you could collect quantitative data on employment rates and business revenue, collect qualitative data on local people’s perceptions and experiences, and analyze local and national media coverage of the development.
The aim is to gain as thorough an understanding as possible of the case and its context.
In writing up the case study, you need to bring together all the relevant aspects to give as complete a picture as possible of the subject.
How you report your findings depends on the type of research you are doing. Some case studies are structured like a standard scientific paper or thesis , with separate sections or chapters for the methods , results and discussion .
Others are written in a more narrative style, aiming to explore the case from various angles and analyze its meanings and implications (for example, by using textual analysis or discourse analysis ).
In all cases, though, make sure to give contextual details about the case, connect it back to the literature and theory, and discuss how it fits into wider patterns or debates.
If you want to know more about statistics , methodology , or research bias , make sure to check out some of our other articles with explanations and examples.
- Normal distribution
- Degrees of freedom
- Null hypothesis
- Discourse analysis
- Control groups
- Mixed methods research
- Non-probability sampling
- Quantitative research
- Ecological validity
Research bias
- Rosenthal effect
- Implicit bias
- Cognitive bias
- Selection bias
- Negativity bias
- Status quo bias
Cite this Scribbr article
If you want to cite this source, you can copy and paste the citation or click the “Cite this Scribbr article” button to automatically add the citation to our free Citation Generator.
McCombes, S. (2023, November 20). What Is a Case Study? | Definition, Examples & Methods. Scribbr. Retrieved August 26, 2024, from https://www.scribbr.com/methodology/case-study/
Is this article helpful?
Shona McCombes
Other students also liked, primary vs. secondary sources | difference & examples, what is a theoretical framework | guide to organizing, what is action research | definition & examples, get unlimited documents corrected.
✔ Free APA citation check included ✔ Unlimited document corrections ✔ Specialized in correcting academic texts

Enter the URL below into your favorite RSS reader.
A case study of the assistive technology network in Sierra Leone before and after a targeted systems-level investment
- Citation (BibTeX)

Sorry, something went wrong. Please try again.
If this problem reoccurs, please contact Scholastica Support
Error message:
View more stats
Many people with disabilities in low-income settings, such as Sierra Leone, do not have access to the assistive technology (AT) they need, yet research to measure and address this issue remains limited. This paper presents a case study of the Assistive Technology 2030 (AT2030) funded Country Investment project in Sierra Leone. The research explored the nature and strength of the AT stakeholder network in Sierra Leone over the course of one year, presenting a snapshot of the network before and after a targeted systems level investment.
Mixed-method surveys were distributed via the Qualtrics software twice, in December 2021 and September 2022 to n=20 and n=16 participants (respectively). Qualitative data was analyzed thematically, while quantitative data was analyzed with the NodeXL software and MS Excel to generate descriptive statistics, visualizations, and specific metrics related to indegree, betweenness and closeness centrality of organizations grouped by type.
Findings suggest the one-year intervention did stimulate change within the AT network in Sierra Leone, increasing the number of connections within the AT network and strengthening existing relationships within the network. Findings are also consistent with existing data suggesting cost is a key barrier to AT access for both organizations providing AT and people with disabilities to obtain AT.
While this paper is the first to demonstrate that a targeted investment in AT systems and policies at the national level can have a resulting impact on the nature and strength of the AT, it only measures outcomes at one-year after investment. Further longitudinal impact evaluation would be desirable. Nonetheless, the results support the potential for systemic investments which leverage inter-organizational relationships and prioritize financial accessibility of AT, as one means of contributing towards increased access to AT for all, particularly in low-income settings.
Assistive technology (AT) is an umbrella term which broadly encompasses assistive products (AP) and the related services which improves function and enhances the user’s participation in all areas of life. 1 Assistive products are “any external products (including devices, equipment, instruments and software) […] with the primary purpose to maintain or improve an individual’s functioning and independence and/or well-being, or to prevent impairments and secondary health conditions”. 2
Recently, awareness for the urgent need to improve access to Assistive Technology has expanded, as 2022 global population statistics highlights one in three people, or 2.5 billion people, requires at least one assistive product. 1 The demand for AT is projected to increase to 3.5 billion people by 2050, yet 90% of them lack access to the products and services they need. 1 , 3 A systemic approach which adequately measures outcomes and impact is urgently required to stimulate evidence-based policies and systems which support universal access to AT. 1 , 4 , 5 However, a systemic approach first necessitates baseline understandings of the existing system, inclusive of sociopolitical context and the key stakeholders working within that context.
Assistive technology is necessary for people with disabilities to engage in activities of daily living, such as personal care or employment, and social engagement. 6 Moreover, people with disabilities also require AT to enact their basic human rights, as outlined in the United Nations Convention on the Rights of Persons with Disabilities (UNCRPD). 7 Unfortunately, many people do not have access to the AT they require, an inequity which is perpetuated within low-income settings. 8 Despite this growing disparity and a well-documented association between poverty and disability, 9 research gaps remain related to AT within low-income settings in the global South. 10
In Sierra Leone, the national prevalence of disability is estimated to be 1.3%, according to the most recent population and housing census data. 11 , 12 This is unusually low, as compared to the 16% global prevalence (World Health Organization, 2022). National stakeholders within the AT network argue this statistic does not adequately represent the true scope of disability in Sierra Leone. 10 Their stance is supported by survey data from the Rapid Assistive Technology Assessment (rATA) across a subset of the population in Freetown, which indicated a dramatically different picture: a 24.9% prevalence of self-reported disability on the basis of the Washington Group Questions (20.6% reported as having “some difficulty”, while 4.3% rated “a lot of difficulty” or above), predominantly mobility and vision related disabilities. 13 The rATA also highlighted 62.5% of older people surveyed indicated having a disability, while the incidence of disability among females was nearly 2% higher than in males. 13
Despite the 2011 Sierra Leone Disability Act being implemented, access to AT in Sierra Leone remains poor. 13 The rATA suggests only 14.9% of those with disabilities in Freetown have the assistive products they require, an alarming rate which also fails to consider people with disabilities not surveyed in rural Sierra Leone where access to such services is likely lower. 13 Meanwhile, it is estimated over half of the population of Sierra Leone lives in poverty, with 13% in extreme poverty. 14 As affordability ranks as the top barrier for AT access, poverty further perpetuates the challenges of people with disabilities within this subset of the population to access necessary AT. 13 Within the context of low-resource settings it is therefore imperative that those resources which are allocated to provide assistive products are used in the most optimal manner, and that different stakeholders work together to co-construct a systemic approach which can identify and prioritise those most in need.
This paper presents a dataset collected in tandem with an Assistive Technology 2030 (AT2030) funded Country Investment project in Sierra Leone in collaboration with Clinton Health Access Initiative (CHAI). The study aimed to explore the nature and strength of the assistive technology stakeholder network in Sierra Leone over the course of one year through a mixed methods survey methodology. We provide a systemic snapshot of the AT network in Sierra Leone, highlighting what assistive products are available, who provides and receives them, and how. We also present a relational analysis of the existing AT network, inclusive of the organizations working within areas of AT and their degrees of connectivity and collaboration amongst one another. We hope that such data can strengthen the provision of AT in Sierra Leone through identifying assistive product availability, procurement, and provision, as well as the nature of the relationships between (the relationality ) of the AT network. We also sought to provide an overview of any possible changes to the network over the course of a one-year investment by AT2030.
This study used a mixed methods survey approach, facilitated by Qualtrics online survey software. Surveys were collaboratively developed and distributed at the two time periods in December 2021 and September 2022 (herein respectively described as Baseline=T1 and Follow Up= T2).
Intervention
This paper presents the Sierra Leone country project built within a larger, targeted investment in assistive technology systems development in four African countries,by AT2030, a project led by the Global Disability Innovation Hub and funded by UK Aid. The four in-country projects were administered by Clinton Health Access Initiative (CHAI) in partnership with local government ministries and agencies. As part of this investment, CHAI and its partners convened a Technical Working Group which brought together key stakeholders in the assistive technology field. Over the course of one year, the Technical Working Group had an overarching goal to develop and strengthen key assistive technology related policies in each of the four countries. The data in this study on the AT network in Sierra Leone was collected at the outset and following completion of the AT2030 investment, by researchers who were not part of the investment process, thus allowing for third-party evaluation. To maintain objectivity, neither CHAI nor the funder were responsible for the design, data collection, analysis or reporting of results, but this paper has benefited from a programmatic perspective provided by CHIA.
Participants
Participants included members of relevant ministries involved in assistive technology leadership and/or delivery, and staff representing relevant non-profit organizations (both international and local), service providers and organizations for persons with disabilities. Participants were asked to respond on behalf of their organization. All prospective participants were identified by the researchers and local project partners, including those coordinating the investment identified above, and added to a distribution list on Qualtrics, which only contained pertinent identifying information such as name, organization, and email. Over the course of the study, n=20 (T1) and n=16 (T2) participants consented to and completed surveys. While the relatively small sample size may inherently restrict the generalizability of this study, the sample size is reflective of the size of the assistive technology network in Sierra Leone, which we aimed to explore.
Data collection
The survey was emailed to the distribution list at two time points: December 2021 (T1) and September 2022 (T2). Two reminder emails were sent out via Qualtrics at two-week intervals following each time point, to participants who had not yet completed the surveys as a means to stimulate participant retention. The T1 and T2 surveys were identical, however the T2 survey utilized display logic functionalities such as conditional skipping to prevent retained respondents from completing redundant questions such as demographic information. If a participant completed the survey for the first time during the T2 period, they received the survey in its entirety without conditional skipping.
Survey content
Survey questions aimed to capture what AT is available, how it is being provided, who is receiving it and how. Questions also consisted of demographic information and qualitative prompts to identify participants’ roles within the AT network and critical challenges experienced in enacting their roles, as well as the nature and strength of relationships between stakeholders. Additional data was collected on participatory engagement in policy development, knowledge of assistive technology, and capacity for leadership which will be published separately.
Using the methodology reported by Smith and colleagues, 15 the WHO priority assistive products list was provided for respondents to select the products and associated services their organization provides. Additionally, the survey requested respondents to select from a list of organizations, which ones they were aware of as working within AT areas in Sierra Leone, followed by a subsequent 5-point Likert scale (1-5, 1= no relationship, 5= collaboration) to indicate which organizations they had working relationships with and to what extent. In attempts to maximize response rates and maintain participant retention, two reminder emails were sent to participants for T1 and T2; however, challenges encountered were participant drop-out from T1 to T2.
Data analysis
Data was reviewed across the two time periods and descriptive statistics (counts and means) were calculated for all variables using MS Excel software. Qualitative data employed content analysis of the text responses from each open-ended survey question, with a particular emphasis on themes which represented commonalities or a lack of representation across all stakeholders. Network data was analyzed using the NodeXL software and MS Excel to generate visualizations, and specific metrics related to indegree, betweenness and closeness centrality of organizations grouped by organization type. Indegree represents the total number of incoming connections per organization, while weighted indegree represents the sum of weights (strength) of each connection. Closeness centrality represents the relationship of the organization to the centre of the network (lower scores indicate greater centrality). To accommodate for different response rates at baseline and follow up, indegree was calculated as a proportion of incoming connections out of the total respondents (n) for that time point. Weighted indegree was calculated as a proportion of the sum of weights of incoming connections divided by the total possible weighting for the respondents for that time point (i.e. n*5). Statistical comparisons for overall network metrics across T1 and T2 were calculated using a paired t-test in SPSS v.28. While means are also reported by organization type as a subsample of the overall data, no statistical tests were carried out due to small subsample sizes.
The study received ethical approval from Maynooth University and the Sierra Leone Ethics and Scientific Review Committee. Each survey contained a mandatory informed consent section which required completion prior to respondents accessing the survey questions. Respondents were not required to answer any specific questions and were not coerced to participate. All respondents received a unique identification code to preserve anonymity, and any identifying information was removed prior to data analysis.
A total of 27 participants from 24 organizations participated in the surveys across both baseline and follow-up time points (T1 n=20 and T2 n= 16). Nine individuals and 11 organizations were retained across both T1 and T2 surveys. The majority of participants represented International non-governmental organizations (n=9), followed by Organizations of Persons with Disabilities (n=8), Government Ministry (n=4), Service Delivery organisations (n=4) and Academic Institutions (n=2).
Additionally, the respondents were requested to identify multiple areas of AT that their organizations were aligned with. Advocacy ranked as the top selection (24.5%), followed by direct service provision (14.9%), human resources and capacity building (14.9%), policy or systems development (13.8%), product selection and/or procurement (13.8%), data and information systems (11.7%), and financing (6.4%).
Assistive Products in Sierra Leone
Participants were asked to select from the APL which products and/or product services they provide. Manual wheelchairs, crutches, canes, lower limb prosthetics and orthopaedic footwear were the most selected across both time points. Table 1 lists summarises the types of assistive products and services provided in Sierra Leone, and the number of organisations providing each product and/or service across all 50 APL products.
No products or services provided | Alarm signallers, audio players, closed captioning displays, fall detectors, global positioning locators, hearing loops/FM systems, magnifiers (digital hand-held and optical), personal emergency alarm, pill organizers, watches |
1 organization providing product or service | Braille displays/note takers, communication software, gesture to voice technology, incontinence products, keyboard and mouse emulation software, pressure relief mattresses, screen readers, simplified mobile phones, tablets*, upright supportive chair and table for children*, rubber tips*, pencil grips*, adapted cups*, sponges*, weighted spoons*, weighted vests*, rollators**, time management products**, travel aids** |
2-3 organizations providing product or service | Communication boards, deafblind communicators, hearing aids, orthoses (lower limb, spinal and upper limb), personal digital assistant, pressure relief cushions, prostheses (lower limb), recorders, spectacles, therapeutic footwear, video communication devices, walking frames, wheelchairs (power), |
4-5 organizations providing product or service | Braille writing equipment, canes/sticks, clubfoot braces, handrails/grab bars, standing frames, tricycles, white canes, |
6-9 organizations product or service | Chairs for shower/bath/toilet, ramps |
10 or more organizations | Crutches/axillary, wheelchairs (manual) |
*Other assistive product offered but not on the Assistive Product List **Assistive product not provided, only service related to the prescription, servicing and maintenance, and customization of that Assistive product
Respondents indicated that the products they provide were most commonly procured by their organizations through purchase (38.7%), followed by donation (29%), building products themselves (22.6%) or other (9.7%), which was explicated as recycling used products.
Providers of Assistive Products in Sierra Leone
Participants were asked to indicate whether their organization provided assistive products and/or related services. The findings highlighted 38.3% of stakeholders directly provided AT and 40.4% directly provided AT related services to beneficiaries, while only 21.3% indicated they do not provide AT or AT related services at all.
More specifically, respondents who did indicate providing products and/or services indicated they provided the following services: provision of locally made assistive products, repairs and maintenance of assistive products, education and training of users on the utility of assistive products, referrals of people with disabilities to service providers, prosthetic and orthotics, accessibility assessments, and rehabilitation service provision. Participants whom do not directly provide AT or AT related services indicated their work falls within AT advocacy, fundraising, procurement, policy, and research.
When asked about the challenges they experienced procuring and distributing these products to beneficiaries, qualitative data indicated difficulty sourcing materials, challenges obtaining products due to poor infrastructure, poor quality standards and/or customizability of products, and low technical and managerial support as common barriers. High product and material costs and inadequate funds from both the organizations and beneficiaries was the most commonly cited challenge.
Beneficiaries of Assistive Products in Sierra Leone
When probed on the number of clients they served each month, respondents indicated the range of beneficiaries spanned from as little as 10 per month to upwards of 1000, while one respondent noted there was no fixed number as they serve at the national level. Respondents noted that their beneficiaries were predominantly people with mobility related disabilities or functional limitations (21.4%), closely followed by people with vision disabilities (17.9%), communication disabilities (15.4%) and hearing disabilities (13.1%).
Participants emphasized children and adolescents were the highest populations served, with an equal representation among the ages of 5-12 (23.7%) and 13-18 (23.7%). Adults aged 20-50 years (21%) closely followed, while children under 4 (15.8%) and adults over 50 years of age (15.8%) are equally less represented as beneficiaries of assistive products and services in Sierra Leone.
Respondents whose organizations provide assistive products indicated that their beneficiaries most commonly received APs free of cost (63.2%), followed by client payment (26.3%) and a fixed cost structure (10.5%).
Network Analysis
Respondents were asked to indicate which organizations in the AT network they were aware of, and subsequently to rate the strength of their relationship with the organizations they indicated an awareness of. The degree of relationality among these stakeholders involved in the assistive technology network was then analyzed across the two time points and organizational relationships were visualized using the NodeXL software, presented in Figure 1 and Figure 2 . The colored nodes in the figures depict the various sub-types of organizations, while the lines between the nodes represent their relationships, with thicker lines indicating stronger relationships. The red nodes represent government ministries and agencies, the green represent service delivery organizations, blue represents organization of persons with disabilities, black represents NGOs and yellow represents academic institutions.

Overall, this representation depicts a relatively centralized network with a higher degree of connections between organizations. Furthermore, ministries and government agencies appear towards the centre of the network, indicating a relatively greater role in connecting organizations to one another, however it is noteworthy that these are not the most central organizations in the network.
Table 2 provides quantitative data which demonstrates the overall number and strength of interconnections among the organizations within the assistive technology network in Sierra Leone. Indegree is the number of identified inward connections, or the number of other organizations who identified a connection with that organization. Indegree data are presented as a mean value per organization type to preserve anonymity. The data visualized in Table 2 significantly increased over one year from baseline to follow up, while the relative centrality of organizations did not change, at least over the one-year time period of this study.
Organization Type | Indegree Mean (SD) | Weighted Indegree Mean (SD) | Closeness Centrality Mean (SD) | |||
Baseline | Follow Up | Baseline | Follow Up | Baseline | Follow Up | |
Ministry or Government Agency | 0.46 (0.11) | 0.50 (0.16) | 9.07 (3.49) | 10.36 (4.34) | 0.53 (0.01) | 0.64 (0.14) |
Organization of Persons with Disabilities | 0.23 (0.07) | 0.38 (0.11) | 3.73 (0.91) | 6.73 (2.19) | 0.54 (0.09) | 0.58 (0.13) |
Service Delivery Organization | 0.34 (0.06) | 0.48 (0.09) | 6.00 (1.52) | 7.84 (2.76) | 0.57 (0.13) | 0.53 (0.01) |
Local NGO | 0.24 (0.07) | 0.40 (0.17) | 4.48 (2.05) | 6.96 (3.87) | 0.52 (0.01) | 0.52 (0.02) |
International NGO | 0.27 (0.07) | 0.40 (0.16) | 4.90 (1.64) | 7.29 (3.77) | 0.55 (0.07) | 0.56 (0.07) |
Overall | 0.29 (0.11) | 0.42* (0.14) | 5.12 (2.34) | 7.51* (3.31) | 0.54 (0.08) | 0.56 (0.10) |
SD – standard deviation, NGO – non-governmental organization *Differs significantly from baseline at p<0.01 (two-tailed)
Overall, there was a statistically significant increase in indegree scores between the two timepoints suggesting a higher level of connection among AT organizations in Sierra Leone following the 1-year investment. This suggests those organizations built more relationships and expanded their reach within the AT network. As relationship strength was measured on a 5-point scale (no awareness, awareness, communication, cooperation, collaboration), we can interpret increases in weighted indegree to suggest greater inter-organizational working between members of the network (please refer to Table 2 ).
These findings suggest the one-year intervention did indeed stimulate change within the AT network in Sierra Leone, increasing the number connections within the AT network, and strengthening existing relationships within the network.
The most common assistive products available in Sierra Leone were indicated to be manual wheelchairs, crutches, canes, lower limb prosthetics and orthopaedic footwear. This aligns with our participants ranking mobility related disabilities or functional limitations as the most common reason for beneficiary referral, as well as the rATA data 13 ). The global report on AT notes “the type, complexity, magnitude and duration of a humanitarian crisis impacts the need for and supply of assistive technology”. 1 When we factor in the sociopolitical context of Sierra Leone and its history of civil war, and the population requiring these products due to political violence, such as lower limb amputations, it is also not surprising that mobility related products are so widely available due to population need. Moreover, as many low-income settings procure their products through donations, often from abroad, these items are probable to be in high circulation in relation to the high global prevalence of mobility related disabilities, likely shaping what products donors perceive as being most relevant. 1
Interestingly, data from the rATA shows the people with disabilities who did have AP, most often obtained their product(s) through purchase, despite cost being the most significant barrier to access. 13 As such, these APs were often purchased through informal and unregulated providers who offer lower costs, such as market vendors. 16 In comparison, our findings demonstrated AT stakeholders providing AP did so predominantly at no-cost. This discrepancy could suggest those who need AT most are not aware of the regulated providers who offer free AP and/or AP services in Sierra Leone, or they simply cannot access them due to infrastructural barriers, or not having AT needed to navigate their environment in the first place. For example, our data highlighted only two organizations offering spectacles, yet the rATA indicated spectacles as being the most common AP obtained by people with disabilities sampled in Sierra Leone. This further supports our interpretation that access to free APs is limited if only a small subset of regulated providers are offering them, leading to an increased reliance on people with disabilities procuring APs from informal and unregulated providers in Sierra Leone. An interconnected and coherent national AT network could offer a way forward, should collaborative relationships among AT stakeholders continue to forge and their collective resources, contacts and beneficiaries were to be cross-pollinated for the advancement of beneficiary access.
As technology and what constitutes as AT continues to advance, juxtaposed with the prevalence of disability increasing, there is a risk that the gap in access to AT will continue to rise. 17 It is therefore paramount that the goal of improving AT related outcomes, such as improved access to AT for all, is first warranted by the measurement of such outcomes. 4 This paper has attempted to provide a systemic snapshot of the AT network in Sierra Leone, highlighting key information such as what assistive products are presently available, who provides them, who receives them (and how), and the relational cohesion of the network itself.
This paper is the first to demonstrate that a targeted investment in assistive technology systems and policies at the national level can have a resulting impact on the nature and strength of the assistive technology ecosystem relationships. It is therefore recommended as an intervention to engage stakeholders within the assistive technology space, in particular policy makers who have power to formulate AP related policy and access. However, this work is limited in scope as it only provides a reassessment of outcomes following the one-year investment, and does provide a more longitudinal evaluation of the impact of that investment in the longer term.
Future research is recommended to replicate the work done to date to evaluate whether there is an improvement in access to assistive technologies over a longer period of time as a result of targeted policy and systems change, as well as larger impacts on policy formulation for AP access. For example, attention to data collection of which types and categories of AP are being manufactured locally can inform policy formation to encourage continuity of local manufacturing, while improving access to AP. Moreover, further studies to investigate factors influencing limited uptake of free AP by persons with disabilities, as explicated above and discovered in this study, are recommended.
CONCLUSIONS
Cohesive AT networks are particularly important in low-income settings such as Sierra Leone, where the intersection of poverty and disability disproportionately reduces people with disabilities’ access to the AT they need. Power and colleagues 18 have proposed the Assistive Technology Embedded Systems Thinking (ATEST) Model as a way of conceptualising the embedded relationships between individual-community- system-country-world influences on assistive technology provision. This paper suggests that even where resources are scarce and systemic relationships are uneven, an internationally-funded investment, which embraces the participation of country-level stakeholders and service providing organisations can result in enhanced inter-organisational working, which in turn has the potential to use existing resources more optimally, allowing greater access to services for individuals most in need.
The findings of this paper demonstrate an increase in organizational collaboration can strengthen assistive technology networks, however key barriers to access remain cost for both organizations providing AT and people with disabilities to obtain AT. Future work should use systemic approaches to leverage organizational relationality and prioritize financial accessibility of AT within systemic approaches to AT policy and practice, to leverage existing resources (particularly no-cost AT) and advance towards the ultimate goal of increased access to AT for all.
Ethics Statement
Ethical approval for the study was granted by Maynooth University and the Sierra Leone Ethics and Scientific Review Committee. The study involved human participants but was not a clinical trial. All participants provided informed consent freely and were aware they could withdraw from the study at any time.
Data Availability
All data generated or analysed during this study are included in this article.
This work was funded by the Assistive Technology 2030 project, funded by the United Kingdom Foreign Commonwealth Development Office (FCDO; UK Aid) and administered by the Global Disability Innovation Hub.
Authorship Contributions
Stephanie Huff led the manuscript preparation and contributed to data analysis. Emma M. Smith led the research design, data collection, analysis and contributed to manuscript preparation. Finally, Malcolm MacLachlan contributed to research design, analysis, manuscript review, and supervision. All authors read and approved the final manuscript.
Disclosure of interest
The authors completed the ICMJE Disclosure of Interest Form (available upon request from the corresponding author) and disclose no relevant interests.
Correspondence to:
Emma M. Smith Maynooth University Maynooth, Co. Kildare Ireland [email protected]
Submitted : February 27, 2024 BST
Accepted : June 26, 2024 BST

An official website of the United States government
Here’s how you know
Official websites use .gov A .gov website belongs to an official government organization in the United States.
Secure .gov websites use HTTPS A lock ( Lock A locked padlock ) or https:// means you’ve safely connected to the .gov website. Share sensitive information only on official, secure websites.
Guidance Regarding Methods for De-identification of Protected Health Information in Accordance with the Health Insurance Portability and Accountability Act (HIPAA) Privacy Rule
This page provides guidance about methods and approaches to achieve de-identification in accordance with the Health Insurance Portability and Accountability Act of 1996 (HIPAA) Privacy Rule. The guidance explains and answers questions regarding the two methods that can be used to satisfy the Privacy Rule’s de-identification standard: Expert Determination and Safe Harbor 1 . This guidance is intended to assist covered entities to understand what is de-identification, the general process by which de-identified information is created, and the options available for performing de-identification.
In developing this guidance, the Office for Civil Rights (OCR) solicited input from stakeholders with practical, technical and policy experience in de-identification. OCR convened stakeholders at a workshop consisting of multiple panel sessions held March 8-9, 2010, in Washington, DC. Each panel addressed a specific topic related to the Privacy Rule’s de-identification methodologies and policies. The workshop was open to the public and each panel was followed by a question and answer period. Read more on the Workshop on the HIPAA Privacy Rule's De-Identification Standard. Read the Full Guidance .
1.1 Protected Health Information 1.2 Covered Entities, Business Associates, and PHI 1.3 De-identification and its Rationale 1.4 The De-identification Standard 1.5 Preparation for De-identification
Guidance on Satisfying the Expert Determination Method
2.1 Have expert determinations been applied outside of the health field? 2.2 Who is an “expert?” 2.3 What is an acceptable level of identification risk for an expert determination? 2.4 How long is an expert determination valid for a given data set? 2.5 Can an expert derive multiple solutions from the same data set for a recipient? 2.6 How do experts assess the risk of identification of information? 2.7 What are the approaches by which an expert assesses the risk that health information can be identified? 2.8 What are the approaches by which an expert mitigates the risk of identification of an individual in health information? 2.9 Can an Expert determine a code derived from PHI is de-identified? 2.10 Must a covered entity use a data use agreement when sharing de-identified data to satisfy the Expert Determination Method?
Guidance on Satisfying the Safe Harbor Method
3.1 When can ZIP codes be included in de-identified information? 3.2 May parts or derivatives of any of the listed identifiers be disclosed consistent with the Safe Harbor Method? 3.3 What are examples of dates that are not permitted according to the Safe Harbor Method? 3.4 Can dates associated with test measures for a patient be reported in accordance with Safe Harbor? 3.5 What constitutes “any other unique identifying number, characteristic, or code” with respect to the Safe Harbor method of the Privacy Rule? 3.6 What is “actual knowledge” that the remaining information could be used either alone or in combination with other information to identify an individual who is a subject of the information? 3.7 If a covered entity knows of specific studies about methods to re-identify health information or use de-identified health information alone or in combination with other information to identify an individual, does this necessarily mean a covered entity has actual knowledge under the Safe Harbor method? 3.8 Must a covered entity suppress all personal names, such as physician names, from health information for it to be designated as de-identified? 3.9 Must a covered entity use a data use agreement when sharing de-identified data to satisfy the Safe Harbor Method? 3.10 Must a covered entity remove protected health information from free text fields to satisfy the Safe Harbor Method?
Glossary of Terms
Protected health information.
The HIPAA Privacy Rule protects most “individually identifiable health information” held or transmitted by a covered entity or its business associate, in any form or medium, whether electronic, on paper, or oral. The Privacy Rule calls this information protected health information (PHI) 2 . Protected health information is information, including demographic information, which relates to:
- the individual’s past, present, or future physical or mental health or condition,
- the provision of health care to the individual, or
- the past, present, or future payment for the provision of health care to the individual, and that identifies the individual or for which there is a reasonable basis to believe can be used to identify the individual. Protected health information includes many common identifiers (e.g., name, address, birth date, Social Security Number) when they can be associated with the health information listed above.
For example, a medical record, laboratory report, or hospital bill would be PHI because each document would contain a patient’s name and/or other identifying information associated with the health data content.
By contrast, a health plan report that only noted the average age of health plan members was 45 years would not be PHI because that information, although developed by aggregating information from individual plan member records, does not identify any individual plan members and there is no reasonable basis to believe that it could be used to identify an individual.
The relationship with health information is fundamental. Identifying information alone, such as personal names, residential addresses, or phone numbers, would not necessarily be designated as PHI. For instance, if such information was reported as part of a publicly accessible data source, such as a phone book, then this information would not be PHI because it is not related to heath data (see above). If such information was listed with health condition, health care provision or payment data, such as an indication that the individual was treated at a certain clinic, then this information would be PHI.
Back to top
Covered Entities, Business Associates, and PHI
In general, the protections of the Privacy Rule apply to information held by covered entities and their business associates. HIPAA defines a covered entity as 1) a health care provider that conducts certain standard administrative and financial transactions in electronic form; 2) a health care clearinghouse; or 3) a health plan. 3 A business associate is a person or entity (other than a member of the covered entity’s workforce) that performs certain functions or activities on behalf of, or provides certain services to, a covered entity that involve the use or disclosure of protected health information. A covered entity may use a business associate to de-identify PHI on its behalf only to the extent such activity is authorized by their business associate agreement.
See the OCR website https://www.hhs.gov/ocr/privacy/ for detailed information about the Privacy Rule and how it protects the privacy of health information.
De-identification and its Rationale
The increasing adoption of health information technologies in the United States accelerates their potential to facilitate beneficial studies that combine large, complex data sets from multiple sources. The process of de-identification, by which identifiers are removed from the health information, mitigates privacy risks to individuals and thereby supports the secondary use of data for comparative effectiveness studies, policy assessment, life sciences research, and other endeavors.
The Privacy Rule was designed to protect individually identifiable health information through permitting only certain uses and disclosures of PHI provided by the Rule, or as authorized by the individual subject of the information. However, in recognition of the potential utility of health information even when it is not individually identifiable, §164.502(d) of the Privacy Rule permits a covered entity or its business associate to create information that is not individually identifiable by following the de-identification standard and implementation specifications in §164.514(a)-(b). These provisions allow the entity to use and disclose information that neither identifies nor provides a reasonable basis to identify an individual. 4 As discussed below, the Privacy Rule provides two de-identification methods: 1) a formal determination by a qualified expert; or 2) the removal of specified individual identifiers as well as absence of actual knowledge by the covered entity that the remaining information could be used alone or in combination with other information to identify the individual.
Both methods, even when properly applied, yield de-identified data that retains some risk of identification. Although the risk is very small, it is not zero, and there is a possibility that de-identified data could be linked back to the identity of the patient to which it corresponds.
Regardless of the method by which de-identification is achieved, the Privacy Rule does not restrict the use or disclosure of de-identified health information, as it is no longer considered protected health information.
The De-identification Standard
Section 164.514(a) of the HIPAA Privacy Rule provides the standard for de-identification of protected health information. Under this standard, health information is not individually identifiable if it does not identify an individual and if the covered entity has no reasonable basis to believe it can be used to identify an individual.
§ 164.514 Other requirements relating to uses and disclosures of protected health information. (a) Standard: de-identification of protected health information. Health information that does not identify an individual and with respect to which there is no reasonable basis to believe that the information can be used to identify an individual is not individually identifiable health information.
Sections 164.514(b) and(c) of the Privacy Rule contain the implementation specifications that a covered entity must follow to meet the de-identification standard. As summarized in Figure 1, the Privacy Rule provides two methods by which health information can be designated as de-identified.
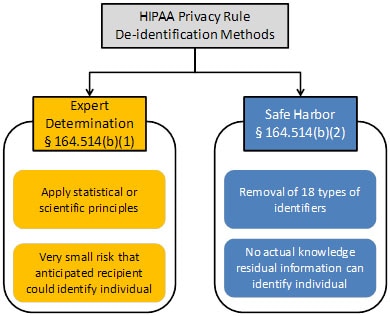
Figure 1. Two methods to achieve de-identification in accordance with the HIPAA Privacy Rule.
The first is the “Expert Determination” method:
(b) Implementation specifications: requirements for de-identification of protected health information. A covered entity may determine that health information is not individually identifiable health information only if: (1) A person with appropriate knowledge of and experience with generally accepted statistical and scientific principles and methods for rendering information not individually identifiable: (i) Applying such principles and methods, determines that the risk is very small that the information could be used, alone or in combination with other reasonably available information, by an anticipated recipient to identify an individual who is a subject of the information; and (ii) Documents the methods and results of the analysis that justify such determination; or
The second is the “Safe Harbor” method:
(2)(i) The following identifiers of the individual or of relatives, employers, or household members of the individual, are removed:
(B) All geographic subdivisions smaller than a state, including street address, city, county, precinct, ZIP code, and their equivalent geocodes, except for the initial three digits of the ZIP code if, according to the current publicly available data from the Bureau of the Census: (1) The geographic unit formed by combining all ZIP codes with the same three initial digits contains more than 20,000 people; and (2) The initial three digits of a ZIP code for all such geographic units containing 20,000 or fewer people is changed to 000
(C) All elements of dates (except year) for dates that are directly related to an individual, including birth date, admission date, discharge date, death date, and all ages over 89 and all elements of dates (including year) indicative of such age, except that such ages and elements may be aggregated into a single category of age 90 or older
(D) Telephone numbers
(L) Vehicle identifiers and serial numbers, including license plate numbers
(E) Fax numbers
(M) Device identifiers and serial numbers
(F) Email addresses
(N) Web Universal Resource Locators (URLs)
(G) Social security numbers
(O) Internet Protocol (IP) addresses
(H) Medical record numbers
(P) Biometric identifiers, including finger and voice prints
(I) Health plan beneficiary numbers
(Q) Full-face photographs and any comparable images
(J) Account numbers
(R) Any other unique identifying number, characteristic, or code, except as permitted by paragraph (c) of this section [Paragraph (c) is presented below in the section “Re-identification”]; and
(K) Certificate/license numbers
(ii) The covered entity does not have actual knowledge that the information could be used alone or in combination with other information to identify an individual who is a subject of the information.
Satisfying either method would demonstrate that a covered entity has met the standard in §164.514(a) above. De-identified health information created following these methods is no longer protected by the Privacy Rule because it does not fall within the definition of PHI. Of course, de-identification leads to information loss which may limit the usefulness of the resulting health information in certain circumstances. As described in the forthcoming sections, covered entities may wish to select de-identification strategies that minimize such loss.
Re-identification
The implementation specifications further provide direction with respect to re-identification , specifically the assignment of a unique code to the set of de-identified health information to permit re-identification by the covered entity.
If a covered entity or business associate successfully undertook an effort to identify the subject of de-identified information it maintained, the health information now related to a specific individual would again be protected by the Privacy Rule, as it would meet the definition of PHI. Disclosure of a code or other means of record identification designed to enable coded or otherwise de-identified information to be re-identified is also considered a disclosure of PHI.
(c) Implementation specifications: re-identification. A covered entity may assign a code or other means of record identification to allow information de-identified under this section to be re-identified by the covered entity, provided that: (1) Derivation. The code or other means of record identification is not derived from or related to information about the individual and is not otherwise capable of being translated so as to identify the individual; and (2) Security. The covered entity does not use or disclose the code or other means of record identification for any other purpose, and does not disclose the mechanism for re-identification.
Preparation for De-identification
The importance of documentation for which values in health data correspond to PHI, as well as the systems that manage PHI, for the de-identification process cannot be overstated. Esoteric notation, such as acronyms whose meaning are known to only a select few employees of a covered entity, and incomplete description may lead those overseeing a de-identification procedure to unnecessarily redact information or to fail to redact when necessary. When sufficient documentation is provided, it is straightforward to redact the appropriate fields. See section 3.10 for a more complete discussion.
In the following two sections, we address questions regarding the Expert Determination method (Section 2) and the Safe Harbor method (Section 3).
In §164.514(b), the Expert Determination method for de-identification is defined as follows:
(1) A person with appropriate knowledge of and experience with generally accepted statistical and scientific principles and methods for rendering information not individually identifiable: (i) Applying such principles and methods, determines that the risk is very small that the information could be used, alone or in combination with other reasonably available information, by an anticipated recipient to identify an individual who is a subject of the information; and (ii) Documents the methods and results of the analysis that justify such determination
Have expert determinations been applied outside of the health field?
Yes. The notion of expert certification is not unique to the health care field. Professional scientists and statisticians in various fields routinely determine and accordingly mitigate risk prior to sharing data. The field of statistical disclosure limitation, for instance, has been developed within government statistical agencies, such as the Bureau of the Census, and applied to protect numerous types of data. 5
Who is an “expert?”
There is no specific professional degree or certification program for designating who is an expert at rendering health information de-identified. Relevant expertise may be gained through various routes of education and experience. Experts may be found in the statistical, mathematical, or other scientific domains. From an enforcement perspective, OCR would review the relevant professional experience and academic or other training of the expert used by the covered entity, as well as actual experience of the expert using health information de-identification methodologies.
What is an acceptable level of identification risk for an expert determination?
There is no explicit numerical level of identification risk that is deemed to universally meet the “very small” level indicated by the method. The ability of a recipient of information to identify an individual (i.e., subject of the information) is dependent on many factors, which an expert will need to take into account while assessing the risk from a data set. This is because the risk of identification that has been determined for one particular data set in the context of a specific environment may not be appropriate for the same data set in a different environment or a different data set in the same environment. As a result, an expert will define an acceptable “very small” risk based on the ability of an anticipated recipient to identify an individual. This issue is addressed in further depth in Section 2.6.
How long is an expert determination valid for a given data set?
The Privacy Rule does not explicitly require that an expiration date be attached to the determination that a data set, or the method that generated such a data set, is de-identified information. However, experts have recognized that technology, social conditions, and the availability of information changes over time. Consequently, certain de-identification practitioners use the approach of time-limited certifications. In this sense, the expert will assess the expected change of computational capability, as well as access to various data sources, and then determine an appropriate timeframe within which the health information will be considered reasonably protected from identification of an individual.
Information that had previously been de-identified may still be adequately de-identified when the certification limit has been reached. When the certification timeframe reaches its conclusion, it does not imply that the data which has already been disseminated is no longer sufficiently protected in accordance with the de-identification standard. Covered entities will need to have an expert examine whether future releases of the data to the same recipient (e.g., monthly reporting) should be subject to additional or different de-identification processes consistent with current conditions to reach the very low risk requirement.
Can an expert derive multiple solutions from the same data set for a recipient?
Yes. Experts may design multiple solutions, each of which is tailored to the covered entity’s expectations regarding information reasonably available to the anticipated recipient of the data set. In such cases, the expert must take care to ensure that the data sets cannot be combined to compromise the protections set in place through the mitigation strategy. (Of course, the expert must also reduce the risk that the data sets could be combined with prior versions of the de-identified dataset or with other publically available datasets to identify an individual.) For instance, an expert may derive one data set that contains detailed geocodes and generalized aged values (e.g., 5-year age ranges) and another data set that contains generalized geocodes (e.g., only the first two digits) and fine-grained age (e.g., days from birth). The expert may certify a covered entity to share both data sets after determining that the two data sets could not be merged to individually identify a patient. This certification may be based on a technical proof regarding the inability to merge such data sets. Alternatively, the expert also could require additional safeguards through a data use agreement.
How do experts assess the risk of identification of information?
No single universal solution addresses all privacy and identifiability issues. Rather, a combination of technical and policy procedures are often applied to the de-identification task. OCR does not require a particular process for an expert to use to reach a determination that the risk of identification is very small. However, the Rule does require that the methods and results of the analysis that justify the determination be documented and made available to OCR upon request. The following information is meant to provide covered entities with a general understanding of the de-identification process applied by an expert. It does not provide sufficient detail in statistical or scientific methods to serve as a substitute for working with an expert in de-identification.
A general workflow for expert determination is depicted in Figure 2. Stakeholder input suggests that the determination of identification risk can be a process that consists of a series of steps. First, the expert will evaluate the extent to which the health information can (or cannot) be identified by the anticipated recipients. Second, the expert often will provide guidance to the covered entity or business associate on which statistical or scientific methods can be applied to the health information to mitigate the anticipated risk. The expert will then execute such methods as deemed acceptable by the covered entity or business associate data managers, i.e., the officials responsible for the design and operations of the covered entity’s information systems. Finally, the expert will evaluate the identifiability of the resulting health information to confirm that the risk is no more than very small when disclosed to the anticipated recipients. Stakeholder input suggests that a process may require several iterations until the expert and data managers agree upon an acceptable solution. Regardless of the process or methods employed, the information must meet the very small risk specification requirement.
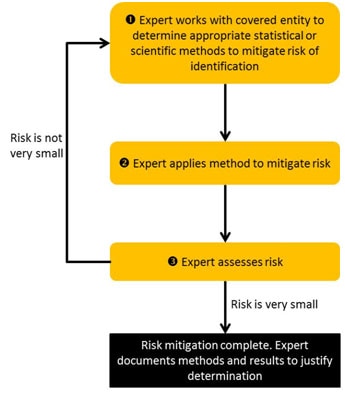
Figure 2. Process for expert determination of de-Identification.
Data managers and administrators working with an expert to consider the risk of identification of a particular set of health information can look to the principles summarized in Table 1 for assistance. 6 These principles build on those defined by the Federal Committee on Statistical Methodology (which was referenced in the original publication of the Privacy Rule). 7 The table describes principles for considering the identification risk of health information. The principles should serve as a starting point for reasoning and are not meant to serve as a definitive list. In the process, experts are advised to consider how data sources that are available to a recipient of health information (e.g., computer systems that contain information about patients) could be utilized for identification of an individual. 8
Table 1. Principles used by experts in the determination of the identifiability of health information.
Prioritize health information features into levels of risk according to the chance it will consistently occur in relation to the individual. | Results of a patient’s blood glucose level test will vary | |
Demographics of a patient (e.g., birth date) are relatively stable | ||
Determine which external data sources contain the patients’ identifiers and the replicable features in the health information, as well as who is permitted access to the data source. | The results of laboratory reports are not often disclosed with identity beyond healthcare environments. | |
Patient name and demographics are often in public data sources, such as vital records -- birth, death, and marriage registries. | ||
Determine the extent to which the subject’s data can be distinguished in the health information. | It has been estimated that the combination of and is unique for approximately 0.04% of residents in the United States . This means that very few residents could be identified through this combination of data alone. | |
It has been estimated that the combination of a patient’s and is unique for over 50% of residents in the United States , . This means that over half of U.S. residents could be uniquely described just with these three data elements. | ||
The greater the replicability, availability, and distinguishability of the health information, the greater the risk for identification. | Laboratory values may be very distinguishing, but they are rarely independently replicable and are rarely disclosed in multiple data sources to which many people have access. | |
Demographics are highly distinguishing, highly replicable, and are available in public data sources. |
When evaluating identification risk, an expert often considers the degree to which a data set can be “linked” to a data source that reveals the identity of the corresponding individuals. Linkage is a process that requires the satisfaction of certain conditions. The first condition is that the de-identified data are unique or “distinguishing.” It should be recognized, however, that the ability to distinguish data is, by itself, insufficient to compromise the corresponding patient’s privacy. This is because of a second condition, which is the need for a naming data source, such as a publicly available voter registration database (see Section 2.6). Without such a data source, there is no way to definitively link the de-identified health information to the corresponding patient. Finally, for the third condition, we need a mechanism to relate the de-identified and identified data sources. Inability to design such a relational mechanism would hamper a third party’s ability to achieve success to no better than random assignment of de-identified data and named individuals. The lack of a readily available naming data source does not imply that data are sufficiently protected from future identification, but it does indicate that it is harder to re-identify an individual, or group of individuals, given the data sources at hand.
Example Scenario Imagine that a covered entity is considering sharing the information in the table to the left in Figure 3. This table is devoid of explicit identifiers, such as personal names and Social Security Numbers. The information in this table is distinguishing, such that each row is unique on the combination of demographics (i.e., Age , ZIP Code , and Gender ). Beyond this data, there exists a voter registration data source, which contains personal names, as well as demographics (i.e., Birthdate , ZIP Code , and Gender ), which are also distinguishing. Linkage between the records in the tables is possible through the demographics. Notice, however, that the first record in the covered entity’s table is not linked because the patient is not yet old enough to vote.

Figure 3. Linking two data sources to identity diagnoses.
Thus, an important aspect of identification risk assessment is the route by which health information can be linked to naming sources or sensitive knowledge can be inferred. A higher risk “feature” is one that is found in many places and is publicly available. These are features that could be exploited by anyone who receives the information. For instance, patient demographics could be classified as high-risk features. In contrast, lower risk features are those that do not appear in public records or are less readily available. For instance, clinical features, such as blood pressure, or temporal dependencies between events within a hospital (e.g., minutes between dispensation of pharmaceuticals) may uniquely characterize a patient in a hospital population, but the data sources to which such information could be linked to identify a patient are accessible to a much smaller set of people.
Example Scenario An expert is asked to assess the identifiability of a patient’s demographics. First, the expert will determine if the demographics are independently replicable . Features such as birth date and gender are strongly independently replicable—the individual will always have the same birth date -- whereas ZIP code of residence is less so because an individual may relocate. Second, the expert will determine which data sources that contain the individual’s identification also contain the demographics in question. In this case, the expert may determine that public records, such as birth, death, and marriage registries, are the most likely data sources to be leveraged for identification. Third, the expert will determine if the specific information to be disclosed is distinguishable . At this point, the expert may determine that certain combinations of values (e.g., Asian males born in January of 1915 and living in a particular 5-digit ZIP code) are unique, whereas others (e.g., white females born in March of 1972 and living in a different 5-digit ZIP code) are never unique. Finally, the expert will determine if the data sources that could be used in the identification process are readily accessible , which may differ by region. For instance, voter registration registries are free in the state of North Carolina, but cost over $15,000 in the state of Wisconsin. Thus, data shared in the former state may be deemed more risky than data shared in the latter. 12
What are the approaches by which an expert assesses the risk that health information can be identified?
The de-identification standard does not mandate a particular method for assessing risk.
A qualified expert may apply generally accepted statistical or scientific principles to compute the likelihood that a record in a data set is expected to be unique, or linkable to only one person, within the population to which it is being compared. Figure 4 provides a visualization of this concept. 13 This figure illustrates a situation in which the records in a data set are not a proper subset of the population for whom identified information is known. This could occur, for instance, if the data set includes patients over one year-old but the population to which it is compared includes data on people over 18 years old (e.g., registered voters).
The computation of population uniques can be achieved in numerous ways, such as through the approaches outlined in published literature. 14 , 15 For instance, if an expert is attempting to assess if the combination of a patient’s race, age, and geographic region of residence is unique, the expert may use population statistics published by the U.S. Census Bureau to assist in this estimation. In instances when population statistics are unavailable or unknown, the expert may calculate and rely on the statistics derived from the data set. This is because a record can only be linked between the data set and the population to which it is being compared if it is unique in both. Thus, by relying on the statistics derived from the data set, the expert will make a conservative estimate regarding the uniqueness of records.
Example Scenario Imagine a covered entity has a data set in which there is one 25 year old male from a certain geographic region in the United States. In truth, there are five 25 year old males in the geographic region in question (i.e., the population). Unfortunately, there is no readily available data source to inform an expert about the number of 25 year old males in this geographic region.
By inspecting the data set, it is clear to the expert that there is at least one 25 year old male in the population, but the expert does not know if there are more. So, without any additional knowledge, the expert assumes there are no more, such that the record in the data set is unique. Based on this observation, the expert recommends removing this record from the data set. In doing so, the expert has made a conservative decision with respect to the uniqueness of the record.
In the previous example, the expert provided a solution (i.e., removing a record from a dataset) to achieve de-identification, but this is one of many possible solutions that an expert could offer. In practice, an expert may provide the covered entity with multiple alternative strategies, based on scientific or statistical principles, to mitigate risk.
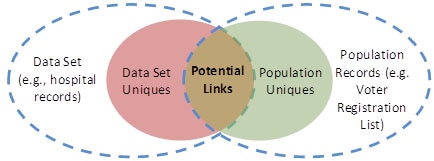
Figure 4. Relationship between uniques in the data set and the broader population, as well as the degree to which linkage can be achieved.
The expert may consider different measures of “risk,” depending on the concern of the organization looking to disclose information. The expert will attempt to determine which record in the data set is the most vulnerable to identification. However, in certain instances, the expert may not know which particular record to be disclosed will be most vulnerable for identification purposes. In this case, the expert may attempt to compute risk from several different perspectives.
What are the approaches by which an expert mitigates the risk of identification of an individual in health information?
The Privacy Rule does not require a particular approach to mitigate, or reduce to very small, identification risk. The following provides a survey of potential approaches. An expert may find all or only one appropriate for a particular project, or may use another method entirely.
If an expert determines that the risk of identification is greater than very small, the expert may modify the information to mitigate the identification risk to that level, as required by the de-identification standard. In general, the expert will adjust certain features or values in the data to ensure that unique, identifiable elements no longer, or are not expected to, exist. Some of the methods described below have been reviewed by the Federal Committee on Statistical Methodology 16 , which was referenced in the original preamble guidance to the Privacy Rule de-identification standard and recently revised.
Several broad classes of methods can be applied to protect data. An overarching common goal of such approaches is to balance disclosure risk against data utility. 17 If one approach results in very small identity disclosure risk but also a set of data with little utility, another approach can be considered. However, data utility does not determine when the de-identification standard of the Privacy Rule has been met.
Table 2 illustrates the application of such methods. In this example, we refer to columns as “features” about patients (e.g., Age and Gender) and rows as “records” of patients (e.g., the first and second rows correspond to records on two different patients).
Table 2. An example of protected health information.
15 | Male | 00000 | Diabetes |
21 | Female | 00001 | Influenza |
36 | Male | 10000 | Broken Arm |
91 | Female | 10001 | Acid Reflux |
A first class of identification risk mitigation methods corresponds to suppression techniques. These methods remove or eliminate certain features about the data prior to dissemination. Suppression of an entire feature may be performed if a substantial quantity of records is considered as too risky (e.g., removal of the ZIP Code feature). Suppression may also be performed on individual records, deleting records entirely if they are deemed too risky to share. This can occur when a record is clearly very distinguishing (e.g., the only individual within a county that makes over $500,000 per year). Alternatively, suppression of specific values within a record may be performed, such as when a particular value is deemed too risky (e.g., “President of the local university”, or ages or ZIP codes that may be unique). Table 3 illustrates this last type of suppression by showing how specific values of features in Table 2 might be suppressed (i.e., black shaded cells).
Table 3. A version of Table 2 with suppressed patient values.
Male | 00000 | Diabetes | |
21 | Female | 00001 | Influenza |
36 | Male | Broken Arm | |
Female | Acid Reflux |
A second class of methods that can be applied for risk mitigation are based on generalization (sometimes referred to as abbreviation) of the information. These methods transform data into more abstract representations. For instance, a five-digit ZIP Code may be generalized to a four-digit ZIP Code, which in turn may be generalized to a three-digit ZIP Code, and onward so as to disclose data with lesser degrees of granularity. Similarly, the age of a patient may be generalized from one- to five-year age groups. Table 4 illustrates how generalization (i.e., gray shaded cells) might be applied to the information in Table 2.
Table 4. A version of Table 2 with generalized patient values.
Under 21 | Male | 0000* | Diabetes |
Between 21 and 34 | Female | 0000* | Influenza |
Between 35 and 44 | Male | 1000* | Broken Arm |
45 and over | Female | 1000* | Acid Reflux |
A third class of methods that can be applied for risk mitigation corresponds to perturbation . In this case, specific values are replaced with equally specific, but different, values. For instance, a patient’s age may be reported as a random value within a 5-year window of the actual age. Table 5 illustrates how perturbation (i.e., gray shaded cells) might be applied to Table 2. Notice that every age is within +/- 2 years of the original age. Similarly, the final digit in each ZIP Code is within +/- 3 of the original ZIP Code.
Table 5. A version of Table 2 with randomized patient values.
16 | Male | 00002 | Diabetes |
20 | Female | 00000 | Influenza |
34 | Male | 10000 | Broken Arm |
93 | Female | 10003 | Acid Reflux |
In practice, perturbation is performed to maintain statistical properties about the original data, such as mean or variance.
The application of a method from one class does not necessarily preclude the application of a method from another class. For instance, it is common to apply generalization and suppression to the same data set.
Using such methods, the expert will prove that the likelihood an undesirable event (e.g., future identification of an individual) will occur is very small. For instance, one example of a data protection model that has been applied to health information is the k -anonymity principle. 18 , 19 In this model, “ k ” refers to the number of people to which each disclosed record must correspond. In practice, this correspondence is assessed using the features that could be reasonably applied by a recipient to identify a patient. Table 6 illustrates an application of generalization and suppression methods to achieve 2-anonymity with respect to the Age, Gender, and ZIP Code columns in Table 2. The first two rows (i.e., shaded light gray) and last two rows (i.e., shaded dark gray) correspond to patient records with the same combination of generalized and suppressed values for Age, Gender, and ZIP Code. Notice that Gender has been suppressed completely (i.e., black shaded cell).
Table 6, as well as a value of k equal to 2, is meant to serve as a simple example for illustrative purposes only. Various state and federal agencies define policies regarding small cell counts (i.e., the number of people corresponding to the same combination of features) when sharing tabular, or summary, data. 20 , 21 , 22 , 23 , 24 , 25 , 26 , 27 However, OCR does not designate a universal value for k that covered entities should apply to protect health information in accordance with the de-identification standard. The value for k should be set at a level that is appropriate to mitigate risk of identification by the anticipated recipient of the data set. 28
Table 6. A version of Table 2 that is 2-anonymized.
Under 30 | 0000* | Diabetes | |
Under 30 | 0000* | Influenza | |
Over 30 | 1000* | Broken Arm | |
Over 30 | 1000* | Acid Reflux |
As can be seen, there are many different disclosure risk reduction techniques that can be applied to health information. However, it should be noted that there is no particular method that is universally the best option for every covered entity and health information set. Each method has benefits and drawbacks with respect to expected applications of the health information, which will be distinct for each covered entity and each intended recipient. The determination of which method is most appropriate for the information will be assessed by the expert on a case-by-case basis and will be guided by input of the covered entity.
Finally, as noted in the preamble to the Privacy Rule, the expert may also consider the technique of limiting distribution of records through a data use agreement or restricted access agreement in which the recipient agrees to limits on who can use or receive the data, or agrees not to attempt identification of the subjects. Of course, the specific details of such an agreement are left to the discretion of the expert and covered entity.
Can an Expert determine a code derived from PHI is de-identified?
There has been confusion about what constitutes a code and how it relates to PHI. For clarification, our guidance is similar to that provided by the National Institutes of Standards and Technology (NIST) 29 , which states:
“ De-identified information can be re-identified (rendered distinguishable) by using a code, algorithm, or pseudonym that is assigned to individual records. The code, algorithm, or pseudonym should not be derived from other related information* about the individual, and the means of re-identification should only be known by authorized parties and not disclosed to anyone without the authority to re-identify records. A common de-identification technique for obscuring PII [Personally Identifiable Information] is to use a one-way cryptographic function, also known as a hash function, on the PII.
*This is not intended to exclude the application of cryptographic hash functions to the information.”
In line with this guidance from NIST, a covered entity may disclose codes derived from PHI as part of a de-identified data set if an expert determines that the data meets the de-identification requirements at §164.514(b)(1). The re-identification provision in §164.514(c) does not preclude the transformation of PHI into values derived by cryptographic hash functions using the expert determination method, provided the keys associated with such functions are not disclosed, including to the recipients of the de-identified information.
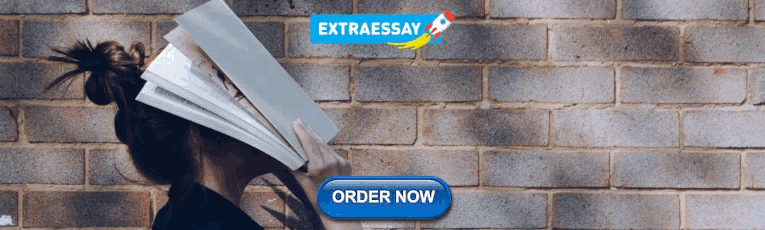
Must a covered entity use a data use agreement when sharing de-identified data to satisfy the Expert Determination Method?
No. The Privacy Rule does not limit how a covered entity may disclose information that has been de-identified. However, a covered entity may require the recipient of de-identified information to enter into a data use agreement to access files with known disclosure risk, such as is required for release of a limited data set under the Privacy Rule. This agreement may contain a number of clauses designed to protect the data, such as prohibiting re-identification. 30 Of course, the use of a data use agreement does not substitute for any of the specific requirements of the Expert Determination Method. Further information about data use agreements can be found on the OCR website. 31 Covered entities may make their own assessments whether such additional oversight is appropriate.
In §164.514(b), the Safe Harbor method for de-identification is defined as follows:
(R) Any other unique identifying number, characteristic, or code, except as permitted by paragraph (c) of this section; and
When can ZIP codes be included in de-identified information?
Covered entities may include the first three digits of the ZIP code if, according to the current publicly available data from the Bureau of the Census: (1) The geographic unit formed by combining all ZIP codes with the same three initial digits contains more than 20,000 people; or (2) the initial three digits of a ZIP code for all such geographic units containing 20,000 or fewer people is changed to 000. This means that the initial three digits of ZIP codes may be included in de-identified information except when the ZIP codes contain the initial three digits listed in the Table below. In those cases, the first three digits must be listed as 000.
OCR published a final rule on August 14, 2002, that modified certain standards in the Privacy Rule. The preamble to this final rule identified the initial three digits of ZIP codes, or ZIP code tabulation areas (ZCTAs), that must change to 000 for release. 67 FR 53182, 53233-53234 (Aug. 14, 2002)).
Utilizing 2000 Census data, the following three-digit ZCTAs have a population of 20,000 or fewer persons. To produce a de-identified data set utilizing the safe harbor method, all records with three-digit ZIP codes corresponding to these three-digit ZCTAs must have the ZIP code changed to 000. Covered entities should not, however, rely upon this listing or the one found in the August 14, 2002 regulation if more current data has been published .
The 17 restricted ZIP codes are:
The Department notes that these three-digit ZIP codes are based on the five-digit ZIP Code Tabulation Areas created by the Census Bureau for the 2000 Census. This new methodology also is briefly described below, as it will likely be of interest to all users of data tabulated by ZIP code. The Census Bureau will not be producing data files containing U.S. Postal Service ZIP codes either as part of the Census 2000 product series or as a post Census 2000 product. However, due to the public’s interest in having statistics tabulated by ZIP code, the Census Bureau has created a new statistical area called the Zip Code Tabulation Area (ZCTA) for Census 2000. The ZCTAs were designed to overcome the operational difficulties of creating a well-defined ZIP code area by using Census blocks (and the addresses found in them) as the basis for the ZCTAs. In the past, there has been no correlation between ZIP codes and Census Bureau geography. Zip codes can cross State, place, county, census tract, block group, and census block boundaries. The geographic designations the Census Bureau uses to tabulate data are relatively stable over time. For instance, census tracts are only defined every ten years. In contrast, ZIP codes can change more frequently. Because of the ill-defined nature of ZIP code boundaries, the Census Bureau has no file (crosswalk) showing the relationship between US Census Bureau geography and U.S. Postal Service ZIP codes.
ZCTAs are generalized area representations of U.S. Postal Service (USPS) ZIP code service areas. Simply put, each one is built by aggregating the Census 2000 blocks, whose addresses use a given ZIP code, into a ZCTA which gets that ZIP code assigned as its ZCTA code. They represent the majority USPS five-digit ZIP code found in a given area. For those areas where it is difficult to determine the prevailing five-digit ZIP code, the higher-level three-digit ZIP code is used for the ZCTA code. For further information, go to: https://www.census.gov/programs-surveys/geography/guidance/geo-areas/zctas.html
The Bureau of the Census provides information regarding population density in the United States. Covered entities are expected to rely on the most current publicly available Bureau of Census data regarding ZIP codes. This information can be downloaded from, or queried at, the American Fact Finder website (http://factfinder.census.gov). As of the publication of this guidance, the information can be extracted from the detailed tables of the “Census 2000 Summary File 1 (SF 1) 100-Percent Data” files under the “Decennial Census” section of the website. The information is derived from the Decennial Census and was last updated in 2000. It is expected that the Census Bureau will make data available from the 2010 Decennial Census in the near future. This guidance will be updated when the Census makes new information available.
May parts or derivatives of any of the listed identifiers be disclosed consistent with the Safe Harbor Method?
No. For example, a data set that contained patient initials, or the last four digits of a Social Security number, would not meet the requirement of the Safe Harbor method for de-identification.
What are examples of dates that are not permitted according to the Safe Harbor Method?
Elements of dates that are not permitted for disclosure include the day, month, and any other information that is more specific than the year of an event. For instance, the date “January 1, 2009” could not be reported at this level of detail. However, it could be reported in a de-identified data set as “2009”.
Many records contain dates of service or other events that imply age. Ages that are explicitly stated, or implied, as over 89 years old must be recoded as 90 or above. For example, if the patient’s year of birth is 1910 and the year of healthcare service is reported as 2010, then in the de-identified data set the year of birth should be reported as “on or before 1920.” Otherwise, a recipient of the data set would learn that the age of the patient is approximately 100.
Can dates associated with test measures for a patient be reported in accordance with Safe Harbor?
No. Dates associated with test measures, such as those derived from a laboratory report, are directly related to a specific individual and relate to the provision of health care. Such dates are protected health information. As a result, no element of a date (except as described in 3.3. above) may be reported to adhere to Safe Harbor.
What constitutes “any other unique identifying number, characteristic, or code” with respect to the Safe Harbor method of the Privacy Rule?
This category corresponds to any unique features that are not explicitly enumerated in the Safe Harbor list (A-Q), but could be used to identify a particular individual. Thus, a covered entity must ensure that a data set stripped of the explicitly enumerated identifiers also does not contain any of these unique features. The following are examples of such features:
Identifying Number There are many potential identifying numbers. For example, the preamble to the Privacy Rule at 65 FR 82462, 82712 (Dec. 28, 2000) noted that “Clinical trial record numbers are included in the general category of ‘any other unique identifying number, characteristic, or code.’
Identifying Code A code corresponds to a value that is derived from a non-secure encoding mechanism. For instance, a code derived from a secure hash function without a secret key (e.g., “salt”) would be considered an identifying element. This is because the resulting value would be susceptible to compromise by the recipient of such data. As another example, an increasing quantity of electronic medical record and electronic prescribing systems assign and embed barcodes into patient records and their medications. These barcodes are often designed to be unique for each patient, or event in a patient’s record, and thus can be easily applied for tracking purposes. See the discussion of re-identification.
Identifying Characteristic A characteristic may be anything that distinguishes an individual and allows for identification. For example, a unique identifying characteristic could be the occupation of a patient, if it was listed in a record as “current President of State University.”
Many questions have been received regarding what constitutes “any other unique identifying number, characteristic or code” in the Safe Harbor approach, §164.514(b)(2)(i)(R), above. Generally, a code or other means of record identification that is derived from PHI would have to be removed from data de-identified following the safe harbor method. To clarify what must be removed under (R), the implementation specifications at §164.514(c) provide an exception with respect to “re-identification” by the covered entity. The objective of the paragraph is to permit covered entities to assign certain types of codes or other record identification to the de-identified information so that it may be re-identified by the covered entity at some later date. Such codes or other means of record identification assigned by the covered entity are not considered direct identifiers that must be removed under (R) if the covered entity follows the directions provided in §164.514(c).
What is “actual knowledge” that the remaining information could be used either alone or in combination with other information to identify an individual who is a subject of the information?
In the context of the Safe Harbor method, actual knowledge means clear and direct knowledge that the remaining information could be used, either alone or in combination with other information, to identify an individual who is a subject of the information. This means that a covered entity has actual knowledge if it concludes that the remaining information could be used to identify the individual. The covered entity, in other words, is aware that the information is not actually de-identified information.
The following examples illustrate when a covered entity would fail to meet the “actual knowledge” provision.
Example 1: Revealing Occupation Imagine a covered entity was aware that the occupation of a patient was listed in a record as “former president of the State University.” This information in combination with almost any additional data – like age or state of residence – would clearly lead to an identification of the patient. In this example, a covered entity would not satisfy the de-identification standard by simply removing the enumerated identifiers in §164.514(b)(2)(i) because the risk of identification is of a nature and degree that a covered entity must have concluded that the information could identify the patient. Therefore, the data would not have satisfied the de-identification standard’s Safe Harbor method unless the covered entity made a sufficient good faith effort to remove the ‘‘occupation’’ field from the patient record.
Example 2: Clear Familial Relation Imagine a covered entity was aware that the anticipated recipient, a researcher who is an employee of the covered entity, had a family member in the data (e.g., spouse, parent, child, or sibling). In addition, the covered entity was aware that the data would provide sufficient context for the employee to recognize the relative. For instance, the details of a complicated series of procedures, such as a primary surgery followed by a set of follow-up surgeries and examinations, for a person of a certain age and gender, might permit the recipient to comprehend that the data pertains to his or her relative’s case. In this situation, the risk of identification is of a nature and degree that the covered entity must have concluded that the recipient could clearly and directly identify the individual in the data. Therefore, the data would not have satisfied the de-identification standard’s Safe Harbor method.
Example 3: Publicized Clinical Event Rare clinical events may facilitate identification in a clear and direct manner. For instance, imagine the information in a patient record revealed that a patient gave birth to an unusually large number of children at the same time. During the year of this event, it is highly possible that this occurred for only one individual in the hospital (and perhaps the country). As a result, the event was reported in the popular media, and the covered entity was aware of this media exposure. In this case, the risk of identification is of a nature and degree that the covered entity must have concluded that the individual subject of the information could be identified by a recipient of the data. Therefore, the data would not have satisfied the de-identification standard’s Safe Harbor method.
Example 4: Knowledge of a Recipient’s Ability Imagine a covered entity was told that the anticipated recipient of the data has a table or algorithm that can be used to identify the information, or a readily available mechanism to determine a patient’s identity. In this situation, the covered entity has actual knowledge because it was informed outright that the recipient can identify a patient, unless it subsequently received information confirming that the recipient does not in fact have a means to identify a patient. Therefore, the data would not have satisfied the de-identification standard’s Safe Harbor method.
If a covered entity knows of specific studies about methods to re-identify health information or use de-identified health information alone or in combination with other information to identify an individual, does this necessarily mean a covered entity has actual knowledge under the Safe Harbor method?
No. Much has been written about the capabilities of researchers with certain analytic and quantitative capacities to combine information in particular ways to identify health information. 32 , 33 , 34 , 35 A covered entity may be aware of studies about methods to identify remaining information or using de-identified information alone or in combination with other information to identify an individual. However, a covered entity’s mere knowledge of these studies and methods, by itself, does not mean it has “actual knowledge” that these methods would be used with the data it is disclosing. OCR does not expect a covered entity to presume such capacities of all potential recipients of de-identified data. This would not be consistent with the intent of the Safe Harbor method, which was to provide covered entities with a simple method to determine if the information is adequately de-identified.
Must a covered entity suppress all personal names, such as physician names, from health information for it to be designated as de-identified?
No. Only names of the individuals associated with the corresponding health information (i.e., the subjects of the records) and of their relatives, employers, and household members must be suppressed. There is no explicit requirement to remove the names of providers or workforce members of the covered entity or business associate. At the same time, there is also no requirement to retain such information in a de-identified data set.
Beyond the removal of names related to the patient, the covered entity would need to consider whether additional personal names contained in the data should be suppressed to meet the actual knowledge specification. Additionally, other laws or confidentiality concerns may support the suppression of this information.
Must a covered entity use a data use agreement when sharing de-identified data to satisfy the Safe Harbor Method?
No. The Privacy Rule does not limit how a covered entity may disclose information that has been de-identified. However, nothing prevents a covered entity from asking a recipient of de-identified information to enter into a data use agreement, such as is required for release of a limited data set under the Privacy Rule. This agreement may prohibit re-identification. Of course, the use of a data use agreement does not substitute for any of the specific requirements of the Safe Harbor method. Further information about data use agreements can be found on the OCR website. 36 Covered entities may make their own assessments whether such additional oversight is appropriate.
Must a covered entity remove protected health information from free text fields to satisfy the Safe Harbor Method?
PHI may exist in different types of data in a multitude of forms and formats in a covered entity. This data may reside in highly structured database tables, such as billing records. Yet, it may also be stored in a wide range of documents with less structure and written in natural language, such as discharge summaries, progress notes, and laboratory test interpretations. These documents may vary with respect to the consistency and the format employed by the covered entity.
The de-identification standard makes no distinction between data entered into standardized fields and information entered as free text (i.e., structured and unstructured text) -- an identifier listed in the Safe Harbor standard must be removed regardless of its location in a record if it is recognizable as an identifier.
Whether additional information must be removed falls under the actual knowledge provision; the extent to which the covered entity has actual knowledge that residual information could be used to individually identify a patient. Clinical narratives in which a physician documents the history and/or lifestyle of a patient are information rich and may provide context that readily allows for patient identification.
Medical records are comprised of a wide range of structured and unstructured (also known as “free text”) documents. In structured documents, it is relatively clear which fields contain the identifiers that must be removed following the Safe Harbor method. For instance, it is simple to discern when a feature is a name or a Social Security Number, provided that the fields are appropriately labeled. However, many researchers have observed that identifiers in medical information are not always clearly labeled. 37 . 38 As such, in some electronic health record systems it may be difficult to discern what a particular term or phrase corresponds to (e.g., is 5/97 a date or a ratio?). It also is important to document when fields are derived from the Safe Harbor listed identifiers. For instance, if a field corresponds to the first initials of names, then this derivation should be noted. De-identification is more efficient and effective when data managers explicitly document when a feature or value pertains to identifiers. Health Level 7 (HL7) and the International Standards Organization (ISO) publish best practices in documentation and standards that covered entities may consult in this process.
Example Scenario 1 The free text field of a patient’s medical record notes that the patient is the Executive Vice President of the state university. The covered entity must remove this information.
Example Scenario 2 The intake notes for a new patient include the stand-alone notation, “Newark, NJ.” It is not clear whether this relates to the patient’s address, the location of the patient’s previous health care provider, the location of the patient’s recent auto collision, or some other point. The phrase may be retained in the data.
Glossary of terms used in Guidance Regarding Methods for De-identification of Protected Health Information in Accordance with the Health Insurance Portability and Accountability Act (HIPAA) Privacy Rule. Note: some of these terms are paraphrased from the regulatory text; please see the HIPAA Rules for actual definitions.
A person or entity that performs certain functions or activities that involve the use or disclosure of protected health information on behalf of, or provides services to, a covered entity. A member of the covered entity’s workforce is not a business associate. A covered health care provider, health plan, or health care clearinghouse can be a business associate of another covered entity. | |
---|---|
Any entity that is | |
A hash function that is designed to achieve certain security properties. Further details can be found at http://csrc.nist.gov/groups/ST/hash/ | |
A “disclosure” of Protected Health Information (PHI) is the sharing of that PHI outside of a covered entity. The sharing of PHI outside of the health care component of a covered entity is a disclosure. | |
A mathematical function which takes binary data, called the message, and produces a condensed representation, called the message digest. Further details can be found at http://csrc.nist.gov/groups/ST/hash/ | |
Any information, whether oral or recorded in any form or medium, that: | |
Information that is a subset of health information, including demographic information collected from an individual, and: (1) Is created or received by a health care provider, health plan, employer, or health care clearinghouse; and (2) Relates to the past, present, or future physical or mental health or condition of an individual; the provision of health care to an individual; or the past, present, or future payment for the provision of health care to the individual; and (i) That identifies the individual; or (ii) With respect to which there is a reasonable basis to believe the information can be used to identify the individual. | |
Individually identifiable health information: (1) Except as provided in paragraph (2) of this definition, that is: (i) Transmitted by electronic media; (ii) Maintained in electronic media; or (iii) Transmitted or maintained in any other form or medium. (2) Protected health information excludes individually identifiable health information in: (i) Education records covered by the Family Educational Rights and Privacy Act, as amended, 20 U.S.C. 1232g; (ii) Records described at 20 U.S.C. 1232g(a)(4)(B)(iv); and (iii) Employment records held by a covered entity in its role as employer. | |
Withholding information in selected records from release. |
Read the Full Guidance
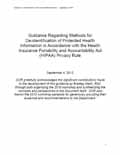
Comments & Suggestions
In an effort to make this guidance a useful tool for HIPAA covered entities and business associates, we welcome and appreciate your sending us any feedback or suggestions to improve this guidance. You may submit a comment by sending an e-mail to [email protected] .
Read more on the Workshop on the HIPAA Privacy Rule's De-Identification Standard
Acknowledgements
OCR gratefully acknowledges the significant contributions made by Bradley Malin, PhD, to the development of this guidance, through both organizing the 2010 workshop and synthesizing the concepts and perspectives in the document itself. OCR also thanks the 2010 workshop panelists for generously providing their expertise and recommendations to the Department.
Disclaimer Policy: Links with this icon ( ) mean that you are leaving the HHS website.
- The Department of Health and Human Services (HHS) cannot guarantee the accuracy of a non-federal website.
- Linking to a non-federal website does not mean that HHS or its employees endorse the sponsors, information, or products presented on the website. HHS links outside of itself to provide you with further information.
- You will be bound by the destination website's privacy policy and/or terms of service when you follow the link.
- HHS is not responsible for Section 508 compliance (accessibility) on private websites.
For more information on HHS's web notification policies, see Website Disclaimers .
Academia.edu no longer supports Internet Explorer.
To browse Academia.edu and the wider internet faster and more securely, please take a few seconds to upgrade your browser .
Enter the email address you signed up with and we'll email you a reset link.
- We're Hiring!
- Help Center
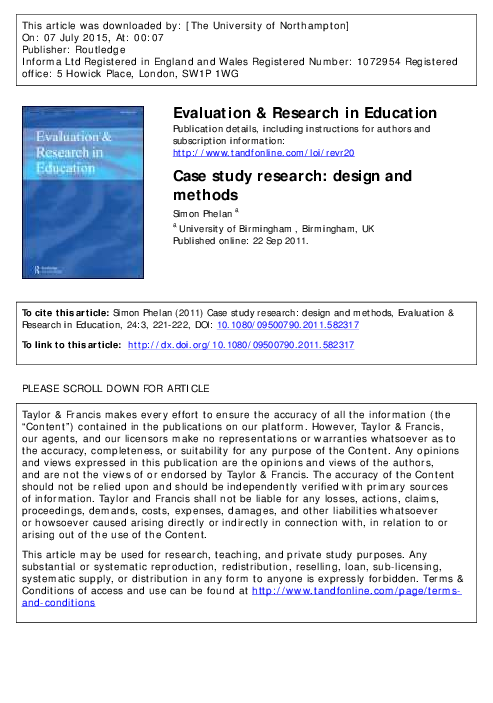
Case study research: design and methods, 4th ed, Robert Yin

Related Papers
The Canadian Journal of Program Evaluation
Trista Hollweck
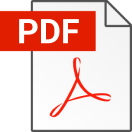
Qualitative Inquiry, vol. 12, no. 2, pp. 219-245
Bent Flyvbjerg
This article examines five common misunderstandings about case-study research: (a) theoretical knowledge is more valuable than practical knowledge; (b) one cannot generalize from a single case, therefore, the single-case study cannot contribute to scientific development; (c) the case study is most useful for generating hypotheses, whereas other methods are more suitable for hypotheses testing and theory building; (d) the case study contains a bias toward verification; and (e) it is often difficult to summarize specific case studies. This article explains and corrects these misunderstandings one by one and concludes with the Kuhnian insight that a scientific discipline without a large number of thoroughly executed case studies is a discipline without systematic production of exemplars, and a discipline without exemplars is an ineffective one. Social science may be strengthened by the execution of a greater number of good case studies.
Yhonier Gonzalez
Evaluation and Program Planning
ashok kumar
The Canadian Journal of Action Research
Trudie Aberdeen
crystal daughtery
In his fourth edition of Case Study Research Design and Methods, Robert K. Yin continues to encourage the formation of better case study research. The text provides a technical yet practical guide to aid the committed researcher. It is an effort to promote rigor and to encourage the recognition of the limitations and awareness of the strengths of case study research. Throughout the text, Yin forthrightly addressed criticisms of the method and provided a solid defense of case study research and its breadth as a research method. An unexpected bonus of the text is found in the cross reference table that provides access to a wealth of classic and contemporary case study research.
Annals of Tourism Research
Asli D.A. Tasci
Adrian Carr
rizwan gujjar
Veritas: The Academic Journal of St Clements Education Group
Mohamed A Eno , abderrazak dammak
Case studies have been subjected to both positive attributes and negative criticisms. Accordingly, there has been a growing academic discussion and debate about the usability of the case study with regard to its reliability. It has been accused of being a less rigorous, undependable, and ungeneralizable research method. The condemnation has led scholars and professionals among the researcher community to raise viewpoints that represent different schools of thought. Each school demonstrated its perception regarding the debate, of course with some concern. Whereas a section of researchers or scholars encourages the method as a useful approach, the other emphasizes its argument based on, among other things, what they call ‘lack of reliability’ of the case study, particularly external validity – whether a study carried out in the approach could indeed be generalized.
Loading Preview
Sorry, preview is currently unavailable. You can download the paper by clicking the button above.
RELATED PAPERS
David E Gray
Teachers College Record
Lesley Bartlett
Fahad Alnaim
Khullar Junior
Arch Woodside
A. Biba Rebolj
Aaron Walter
Ali Haj Aghapour
adilson reis
special issue: the first-year experience
Fabian Frenzel
Raymond Obeng
Journal of Interdisciplinary Studies in Education
Fernando Almeida
Steve Allen
johnpatrick gonzales
EmmsMt Ntuli
The Journal of Agricultural Sciences - Sri Lanka
rohitha rosairo
Dr. BABOUCARR NJIE
Melina Ocampo González
Journal of Service Management
Katrien Verleye
Ricardo D'Ávila
Pragmatic Case Studies in Psychotherapy
Daniel B Fishman
Yana Spasova
RELATED TOPICS
- We're Hiring!
- Help Center
- Find new research papers in:
- Health Sciences
- Earth Sciences
- Cognitive Science
- Mathematics
- Computer Science
- Academia ©2024
Constructing a Novel Network Structure Weighting Technique into the ANP Decision Support System for Optimal Alternative Evaluation: A Case Study on Crowdfunding Tokenization for Startup Financing
- Research Article
- Open access
- Published: 26 August 2024
- Volume 17 , article number 222 , ( 2024 )
Cite this article
You have full access to this open access article
- Chun-Yueh Lin 1
This study constructed a novel decision-making framework for startup companies to evaluate token financing options. A Network structure weighting (NSW) technique was developed and integrated with the analytic network process (ANP) to create a comprehensive assessment model. This innovative approach addressed the limitations of traditional multi-criteria decision-making methods by effectively capturing the complex interdependencies between factors influencing token financing decisions. The proposed model comprises three main steps: (1) utilizing a modified Delphi method to identify key factors affecting token financing, (2) developing the NSW technique to determine the network structure of these factors, and (3) integrating the NSW results into the ANP model to evaluate and rank the critical factors and alternatives. This study applied this framework to assess three token financing alternatives: Initial Coin Offerings (ICO), Initial Exchange Offerings (IEO), and Security Token Offerings (STO). The results indicate that STO is the optimal financing alternative for the analyzed startup scenario in token financing, followed by Initial Exchange Offerings and Initial Coin Offerings. The model identified platform fees, issuance costs, and financing success rate as the three most critical factors influencing the decision. This study contributes to both methodology and practice in FinTech decision-making. The NSW-ANP framework offers a more robust approach to modeling complex financial decisions, while the application to token financing provides valuable insights for startup companies navigating this emerging funding landscape. The proposed framework lays the groundwork for more informed and structured decision-making in the rapidly evolving field of cryptocurrency-based financing.
Explore related subjects
- Artificial Intelligence
Avoid common mistakes on your manuscript.
1 Introduction
Due to the rise and development of Financial Technology (FinTech), as well as the enactment of the Jumpstart Our Business Startups (JOBS) in the U.S. [ 1 ], crowdfunding has become the newest financing means for enterprises in need of external funds [ 2 , 3 ]. In 2014, the total amount of funds raised through crowdfunding reached USD 16.2 billion, which was 167% higher than that of 2013 [ 4 ]. In addition, according to the statistical results of Statista Inc. (2020) [ 5 ], the total amount of alternative financing in 2020 was USD 6.1 billion, among which crowdfunding accounted for the largest market share. For this reason, it could be said that the development scale of crowdfunding in the global financial market has been rocketing.
Crowdfunding involves a number of different forms. The first form is donation-based crowdfunding, which mainly means to raise charity funds for the implementation of programs and projects. The second form is rewards-based crowdfunding, in which the investor can receive non-monetary rewards because of capital contributions. The third form is debt-based crowdfunding, in which the relevant interest arrangements between the investor and the fundraiser are determined in line with credit contracts. The fourth form is equity-based crowdfunding, in which the fundraiser uses the equities of the target company to exchange funds from the investor, while the investor receives such equities and therefore is entitled to that company’s revenues or dividends [ 6 , 7 , 8 ]. Estrin et al. [ 9 ] pointed out that equity-based crowdfunding depends mainly on the Internet or social network platforms. This fund-raising method not only reduces the transaction cost but also stands for a new business pattern under which startup companies can establish their own goodwill and provide investors with opportunities for investment. Although crowdfunding has many advantages for startup companies, risks do exist, including uncertainty of equity ownership, lack of liquidity, and damage to stockholder equity [ 10 , 11 , 12 ]. For this reason, past studies suggested that startup companies might obtain funds by offering tokens on the basis of distributed ledger technology and the immutability of blockchains. This not only could reduce the potential risks of traditional fundraising platforms but also could promote the transparency level of the relevant transactions [ 12 , 13 , 14 ]. Howell et al. [ 15 ] indicated that token financing has become one of the important sources for enterprises to raise funds through digital platforms. Presently, the development of crowdfunding tokenization mainly involves three patterns: (1) initial coin offerings (ICO), (2) initial exchange offerings (IEO), and (3) security token offerings (STO). ICO has the advantages of low cost and high speed. However, the risks of theft and fraud exist [ 15 , 16 , 17 ]. The advantages of IEO include having the business reputation of a third-party platform as a guarantee and handling the relevant transactions directly on the transaction platform. However, the possibility of the token price being manipulated cannot be ruled out [ 17 , 18 ]. The last pattern, STO, has the advantages of the highest level of safety and of being protected by the rules and regulations of regional governments. However, the high complexity of examination and verification as well as excessively low liquidity are problems that cannot be avoided [ 17 , 19 ]. The research results of past literature also show that for startup companies, the efficiency of token financing is higher than that of equity financing [ 20 ]. Furthermore, Chod et al. [ 14 ] pointed out that enterprises may take advantage of the decentralization features of token financing to make it more convenient for token investors in their project investments and reduce the cost of encouraging token investors to join the investment platforms. In this way, it is easier for entrepreneurs in raising funds.
For this reason, the utilization of token financing for the purpose of raising operation efficiency has become an important business strategy. The aforesaid three patterns of crowdfunding tokenization have their respective advantages and disadvantages, as well as potential risks. If startup companies intend to raise funds through virtual currencies, the alternatives of financing in cryptocurrency will affect the financing efficiency and lead to the capital turnover issue. Previous studies on token financing focused more on risk-return analysis [ 21 , 22 , 23 , 24 ], token rules and regulations [ 25 , 26 , 27 ], hedging of tokens [ 28 , 29 , 30 , 31 ], and prediction of price in tokens [ 32 , 33 , 34 , 35 ]. However, there is scarce evidence and a lack of applicable measurement tools in regard to assessing the optimal solution for the token financing of startup companies. Hence, algorithms for multiple criteria decision-making can be utilized for the construction of assessment models, so that the optimal solution for assessment can be reached [ 36 , 37 , 38 ]. Past studies also suggested that the optimal solution can be solved using the analytic hierarchy process (AHP) [ 38 , 39 , 40 , 41 , 42 ]. Although AHP can be used to assess the optimal solutions in different fields, it is unsuitable to use traditional AHP methods for decision-making problems in real situations. AHP is characterized by a hierarchical structure and based upon the presumption that the variables or criteria are independent from each other. Numerous problems relating to the assessment of optimal solutions and the relevant variables are correlated to or dependent on each other; as a result, complicated internal relationships cannot be solved through hierarchical or independent methods [ 43 , 44 ]. To solve this problem, Saaty [ 45 ] proposed the analytic network process (ANP), which added a feedback mechanism and interdependency to the AHP method to solve the problems of a lack of correlation and interdependency. ANP does not require the linear relationship of traditional AHP methods, which is top-down, and can establish an assessment pattern of networked relationships. Past literature has applied ANP models in the assessment of different industries, such as traffic problems [ 46 , 47 ], environment and energy assessment [ 48 , 49 , 50 ], filtration and selection of suppliers [ 51 , 52 , 53 ], and assessment of risk factors [ 54 , 55 , 56 ]. Thus, it can be seen that the problem of correlation or interdependency between criteria or variables cannot be solved effectively through AHP during decision-making, while ANP can effectively solve this problem. Although ANP can overcome the difficulties related to the presumption of independence in AHP, the ANP algorithm cannot ascertain the strength of the dependence and relationships between variables needed to generate a network structure. Previous studies addressing the network structure issue have applied deep machine learning concepts, as demonstrated by Moghaddasi et al., Gharehchopogh et al., and subsequent works by Moghaddasi et al. [ 57 , 58 , 59 , 60 , 61 ]. However, these studies primarily focused on the relationship in the Internet of Things, implicitly highlighting the challenges in applying such approaches to multi-criteria decision-making (MCDM) problems. Additionally, several studies employed the Decision-Making Trial and Evaluation Laboratory (DEMATEL) method to resolve network structures among criteria [ 62 , 63 , 64 , 65 , 66 ]. This approach offers an alternative perspective on capturing complex interrelationships within decision-making frameworks. However, the DEMATEL method has several limitations. First, the relationships derived through DEMATEL may be biased or misleading [ 67 , 68 ]. Additionally, the method faces convergence issues, as it cannot determine relationships between criteria when the data fail to converge [ 69 ]. As evident from Table 1 , there are two primary gaps in the existing literature. First, in terms of network structure methodology, while ANP, DEMATEL, and other decision-making frameworks have been proposed, they each have limitations. Second, regarding the research problem, while many studies have examined different aspects of token financing, there is a notable absence of comprehensive, quantitative decision-making frameworks specifically designed for startup companies evaluating token financing alternatives. In view of the above, this study developed a new network structure weighting (NSW) model, and then integrated NSW into ANP to remedy ANP’s shortcoming of being unable to determine the network structure. Finally, case studies were carried out to assess the optimal solution for startup companies engaging in token financing.
For the proposed NSW-ANP model, the modified Delphi method was utilized to determine the clusters and factors influencing startup companies engaging in token financing. Then, the network structure of these clusters and factors was determined based on the NSW method. Finally, the ANP model was utilized to calculate the weights of various factors and financing schemes for startup companies engaging in token financing and then sequence them to determine the optimal token financing schemes and their key factors. While ANP has been applied in various fields, this study proposed the first application of an enhanced ANP approach (integrated with NSW) to evaluate the token financing options for startups. This novel application demonstrates the versatility and effectiveness of our integrated approach in addressing complex FinTech decision-making scenarios.
This study makes significant contributions to the existing literature in both methodological innovations and novel applications. In terms of methodological advancements, we introduce a novel NSW technique that quantifies the strength of relationships between decision factors in a network structure. Furthermore, we develop an integrated NSW-ANP framework that enhances the capabilities of the traditional ANP by incorporating a more robust method for determining network relationships. With regard to novel applications, this study breaks new ground in two key areas. Firstly, we apply this integrated NSW-ANP framework to evaluate token financing options for startup companies, an area that has not been addressed using such a comprehensive decision-making approach. Secondly, this study provides the first systematic evaluation of ICO, IEO, and STO using a multi-criteria decision-making framework. This framework resolves the complex interdependencies between various factors, offering a more nuanced understanding of these emerging financing mechanisms. By combining methodological innovation with practical application in an emerging field, this study not only advances the theoretical understanding of multi-criteria decision-making processes but also provides valuable insights for practitioners in cryptocurrency-based startup financing. Academically, the new NSW-ANP model put forward in this study could be used for determining the network relationship of a research structure, and be integrated into the ANP to remedy the ANP’s shortcomings. The new integrated decision-making pattern put forward in this study also could provide valuable references for the measurement of the interdependency and correlation among variables in the assessment of the optimal solution of token financing for startup companies. Practically, the proposed framework could provide startup companies with a measurement tool containing a network structure and is valuable, so as to determine the optimal solution of token financing for startup companies introducing token financing to their businesses.
The remainder of this paper is organized as follows: Sect. 1 is the introduction, Sect. 2 describes the research method, Sect. 3 presents the case study, and Sect. 4 offers the conclusions.
2 Methodology
In this study, the clusters and factors were acquired through collecting experts’ opinions and literature reviews via modified Delphi method (MDM) as a first step. Next, the network structure of the clusters and factors was determined on the basis of the network structure weighting (NSW) method. Finally, the analytic network process (ANP) model was utilized to calculate and sequence the weightings of the various factors and financing schemes of startup companies engaging in token financing so that the most suitable token financing scheme and the key factors could be determined. The research method is presented in the following sections.
The Delphi method is an anonymous technique of decision-making by a group of experts. To solve a certain problem or find a solution for a particular future event, these experts are treated as the appraisal targets. For the final goal of reaching a stable group consensus among the experts, the group members are anonymous to each other, and particular procedures and repetitive steps are employed. The Delphi method attempts to combine the knowledge, opinions, and speculative abilities of experts in the field in an interruption-free environment. The Delphi method can be used to deduce what will happen in the future, effectively predict future trends, or reach a consensus over a certain issue [ 70 , 71 ]. This method is based upon the judgment of experts, and multiple rounds of opinion feedback are utilized to solve complicated decision-making problems. The traditional Delphi method emphasizes the following five basic principles [ 72 , 73 ]:
The principle of anonymity: All experts voice their opinions as individuals, and they remain anonymous when doing so.
Iteration: The questionnaire issuer gathers up the experts’ opinions and sends them to other experts. This step is carried out repeatedly.
Controlled feedback: In each round, the experts are required to answer pre-designed questionnaires, and the results are served as references for the next appraisal.
Statistical group responses: Comprehensive judgments are made only after the statistics of all the experts’ opinions are conducted.
Expert consensus: The ultimate goal is to reach a consensus after the experts’ opinions are consolidated.
The procedures of the Delphi method are as follows [ 74 ]:
Select the anonymous experts.
Carry out the first round of the questionnaire survey.
Carry out the second round of the questionnaire survey.
Carry out the third round of the questionnaire survey.
Consolidate the experts’ opinions and reach a consensus.
According to the modified Delphi method, Steps C and D are carried out repeatedly until a consensus is reached among the experts, and the number of experts should be between five and nine [ 75 , 76 ].
In this study, the experts’ opinions were gathered through the Delphi method and the relevant literature was discussed, so that the clusters and factors influencing startup companies engaging in token financing could be obtained.
2.2 NSW Model
This study utilized the Delphi method to collect the clusters and factors that could influence startup companies engaging in token financing schemes. In order to effectively carry out the calculation and assessment of ANP, the network structure of these clusters and factors need to be determined as a prerequisite for the subsequent filtration and selection of the optimal token financing scheme. Therefore, this study put forward the NSW method in order to acquire the relationships and the structure chart between clusters and factors. The NSW procedure is as follows:
Step 1: Collect and confirm the decision factors
The collection and confirmation of the decision factors can be realized through common tools such as literature reviews, the Delphi method, focus group interviews, and brainstorming. When decision-makers or experts need to determine n assessment factors that are consistent with the decision-making issues, the n assessment factors may be defined as \(\{ C_{1} ,C_{2} , \ldots ,C_{n} \}\) .
Step 2: Design the questionnaire
As far as the n factors determined by the decision makers or experts in Step 1 are concerned, a nine-point Likert scale can be utilized to ascertain the correlation and correlation strength between the factors. In the event of n factors, n ( n − 1) comparisons in line with the scale need to be carried out.
Step 3: Calculate the weight of the network structure
Each expert compares and scores the decision factors. After that, all the comparison scores of the experts are used in the matrix construction and weighted calculation. The procedure is as follows:
2.2.1 Establish the Matrix of the Network Correlation and the Correlation Diagram
The correlation matrix is established as M , while \(\{ C_{1} ,C_{2} , \ldots ,C_{n} \}\) are the decision factors. If C i is influenced by C j , \(m_{ij}\) will be the scores of a quantitative judgment given by experts. On the contrary, if \(m_{ij} = 0\) , C i is not influenced by C j . The results can be shown in matrix M ( n × n ) as follows:
The column aggregation and row aggregation of matrix M are:
\({\text{Column}}_{j}\) and \({\text{row}}_{i}\) , respectively, give the scores of factor j , which affects other factors, or factor j , which is influenced by other factors.
2.2.2 Define the Transition Probability Matrix
If transition matrix A is defined by the features of the Markov chain, A = ( a ij ), as shown in Eq. ( 2 ). A is a regular Markov matrix, and the existence of stationary distribution \(x = \left( {x_{1} ,x_{2} , \ldots ,x_{N} } \right)^{T}\) satisfies Ax = x and \(\sum\nolimits_{i} {x_{i} = 1}\) . The characteristic value of 1 can be acquired through the characteristic vector corresponding to the characteristic value of Matrix A , or through the iteration method \(x^{0}\) , where \(x^{k + 1} = Ax^{k}\) , to obtain the characteristic value. x stands for the distribution of probabilities of the various factors being influenced when the transition number approaches infinity, and \(x_{i}\) stands for the network node score of the i th factor.
2.2.3 Calculate the Weightings of the Network Structure
According to the results described in II above, the network node score of each factor is distributed to the correlation diagram of each expert ( n experts have n correlation diagrams). Afterwards, based on the node score of factor i , the strength score of each expert’s factor i influencing other factor j goes through a standardized distribution using the correlation diagram to obtain each expert’s weighted value of the network structure, R, as shown in Eq. ( 3 ). In the end, the \(R(C_{i} ,C_{j} )\) of n experts is averaged and standardized, as shown in Eq. ( 4 ) and Eq. ( 5 ). The standardized results can then be integrated into the ANP model to assess the optimal token financing scheme for startup companies.
Saaty put forward ANP in 1996. This method is rendered through a network structure and derived from an ANP. Practically, there are many questions about decision-making assessment that are not limited to expressing their complex interrelated properties in a hierarchical and independent manner, and they are not of purely linear relationships either. Rather, these questions have a network-like structure [ 45 , 77 , 78 , 79 ]. Based on the original presumption and prerequisite of the analytic hierarchy process (AHP), Saaty [ 45 ] integrated relationship and feedback mechanisms into the AHP model to solve the problem of correlation between different principles.
Saaty pointed out that the relationships of interactive influence between clusters and elements can be analyzed in a graphic manner. Such relationships and interactive influence can be demonstrated through arrow lines [ 45 , 80 ], as shown in Fig. 1 . This network structure is crucial for understanding the fundamental difference between hierarchical and network-based decision-making models. Unlike traditional hierarchical structures, this network allows for complex interdependencies between different elements of the decision-making process. In Fig. 1 , the bidirectional arrows indicate that influence can flow both ways between clusters, reflecting real-world complexities where factors can mutually affect each other.
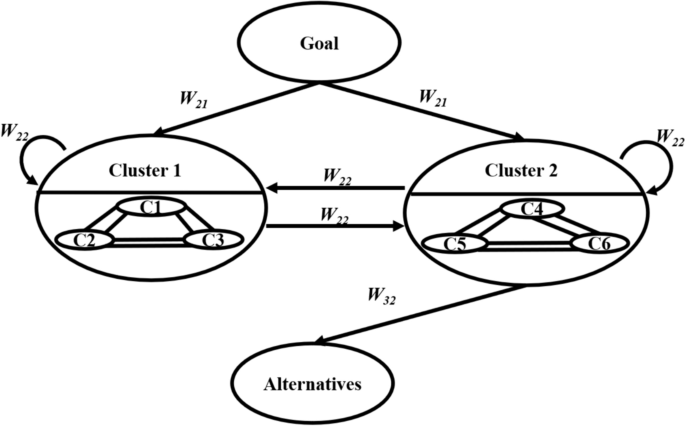
Source : Ref. [ 45 ]
The network structure.
According to the relationships and strengths of different factors in the aforesaid models and structure charts of ANP, a supermatrix is utilized for demonstration, as shown in Fig. 2 . This matrix is a critical component of the ANP, allowing for the quantification of relationships between all elements in the network. It is formed when the various clusters and respective factors contained in such clusters are listed on the left side and upper part of the matrix in an orderly manner. The supermatrix consists of a number of sub-matrices, which are formulated based on the eigenvectors after the comparison of different factors. In Fig. 2 , \(W_{11} ,W_{kk} , \ldots ,W_{nn}\) are the values of the eigenvectors after the comparisons and calculations.
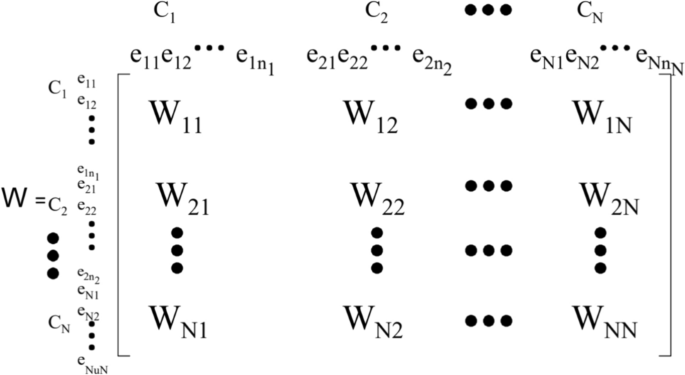
Source : Refs. [ 45 ] [ 80 ]
The supermatrix of a network.
ANP is an algorithm based on AHP and can be divided into four steps. In Step 1, the structures are formed step by step. In Step 2, the questions are raised. In Step 3, comparisons of interdependent clusters are made in pairs and a supermatrix is formed. In Step 4, the ultimate choice and optimal scheme are selected [ 45 , 79 ].
This study apples the ANP as the foundation of our approach due to several key advantages it offers in the context of complex decision-making scenarios. First, it is well-suited for this application because it allows for the consideration of interdependencies and feedback relationships between decision factors, which is crucial in the dynamic and interconnected world of FinTech and token financing. Furthermore, it provides a structured approach to incorporating both qualitative and quantitative factors into the decision-making process. This is particularly beneficial when evaluating token financing options, as it allows us to consider both qualitative and quantitative data. Finally, it is able to prioritize alternatives based on a comprehensive set of criteria and sub-criteria. This is especially valuable when comparing different alternatives, each of which has its own unique set of characteristics and implications. ANP allows for a more comprehensive comparison than simpler decision-making tools. Among various MCDM techniques, the ANP has a superior capacity to model complex systems with intricate interdependencies. While other MCDM techniques, such as the Technique for Order of Preference by Similarity to Ideal Solution (TOPSIS) and the VIseKriterijumska Optimizacija I Kompromisno Resenje (VIKOR) method, offer effective means for ranking alternatives, they exhibit limitations in accounting for the multifaceted interrelationships among criteria.
Consequently, this study employs the ANP method as the foundation for constructing an integrated decision-making model. A brief introduction of the construction program of the network process pattern is as follows:
Step 1: Confirm the research problems and network structure
Determine the targets according to features of the problems and search for decision-making clusters, as well as the factors contained in the various clusters by employing the proposed NSW method to acquire the influencing strength of the various factors; finally, draw the network structure models of the decision-making problems according to the results of NSW.
Step 2: Create pair-wise comparison matrices and priority vectors
Compare the factors in pairs. This step has two parts: the comparison of clusters (in pairs) and the comparison of factors within clusters (in pairs). The comparison of factors within clusters (in pairs) can be divided into the comparison within a particular group and comparisons among different clusters. The assessment scale of the comparison is similar to that of AHP. In addition, the eigenvectors, which are reached through the various comparison matrices, serve as the values of the supermatrix, which can be used to illustrate the interdependency and relative significance among the clusters. Equation ( 6 ) can be utilized to calculate the scores of relative significance in regard to the various clusters and factors. As for the strength of the interdependency among the clusters and among the factors, NSW can be utilized to determine the network structure (as described in Sect. 2.2 .)
Step 3: Construct the supermatrix
The supermatrix can effectively solve problems related to the interdependency among the various clusters and factors within the system (as shown in Fig. 2 ). The values of the supermatrix consist of small matrices, which include the comparison of different factors (in pairs) and the comparison of interdependent factors (in pairs). The numerical values of clusters or factors without the influence of feedback are 0, as shown in Eq. ( 7 ). In this study, it was suggested that the overall network structure could be confirmed by NSW. For this reason, the NSW results were integrated into the supermatrix for subsequent assessment and to determine the strength of the interdependency in the supermatrix, as shown in Eq. ( 8 ).
The ANP calculation process includes three matrices: the unweighted supermatrix, the weighted supermatrix, and the limit supermatrix. The unweighted supermatrix stands for the weightings of the original results of the comparison in pairs. In the weighted supermatrix, the weighted values of a particular element within an unweighted matrix are multiplied by the weighted values of the relevant clusters. In the limit supermatrix, the weighted matrix multiplies itself repeatedly until a stable state is attained. According to ANP, if supermatrix W is in an irreducible state of stability, all columns in the supermatrix will have similar vectors, indicating convergence can be attained. The ultimate weighted values of each cluster, factor, and scheme can be calculated through Eq. ( 9 ) during the convergence process.
Step 4: Evaluate the optimal alternative
Through the ANP framework and the calculations of the unweighted supermatrix, weighted supermatrix, and limit supermatrix, all the alternative schemes, as well as the ultimate values of the groups and factors, can be attained in the limit supermatrix. The ultimate results of the weighted values are then ranked to determine the optimal scheme.
3 Case Study
This study aimed to establish the network structure weighting (NSW) model by integrating NSW into the analytic network process (ANP) and establishing an assessment pattern to analyze the optimal scheme of token financing for startup companies, as well as the weighted values of clusters and factors. The consolidation-type diagram of the analytical process is shown in Fig. 3 . This integrated framework is a key innovation, that employs the Modified Delphi Method to identify relevant factors, and applies the NSW technique to determine the network structure. The results are then integrated into the ANP model for final calculations and analysis. This integrated approach addresses the limitations of traditional ANP by providing a more robust and objective method for determining network relationships. It combines the strengths of expert knowledge (through the Delphi method), systematic relationship quantification (via NSW), and comprehensive decision analysis (through ANP), resulting in a more reliable and nuanced decision-making tool for token financing. First, the modified Delphi method was utilized to calculate the clusters and factors influencing startup companies engaging in token financing. Second, the network structure of the clusters and factors was determined on the basis of the NSW method put forward in this study. Finally, the weighted values of the network structure of NSW were integrated into the ANP model to calculate the weighted values for the various factors and various financing schemes of startup companies engaging in token financing. These weighted values were then sequenced to obtain the optimal scheme and key factors of token financing. Figure 4 presents the integrated framework for evaluating token financing options. This model incorporates five main clusters: Finance, Laws and Regulations, Risk, Investor, and Online Community, each containing several specific factors. The model also includes three token financing alternatives: ICO, IEO, and STO. This structure allows for a comprehensive evaluation of token financing alternatives, considering a wide range of relevant factors. By inclusion of diverse clusters including financial considerations, as well as legal, risk-related, investor-focused, and community aspects, the proposed framework allows startup companies to make well-informed decisions based on a thorough analysis of all relevant factors.
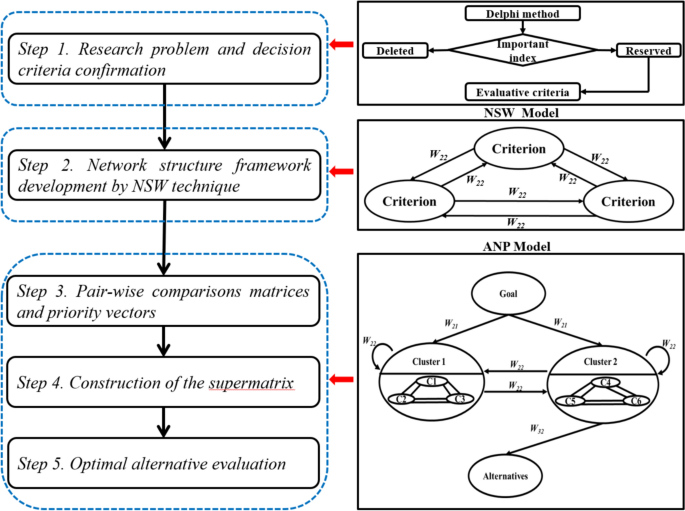
The integration processes
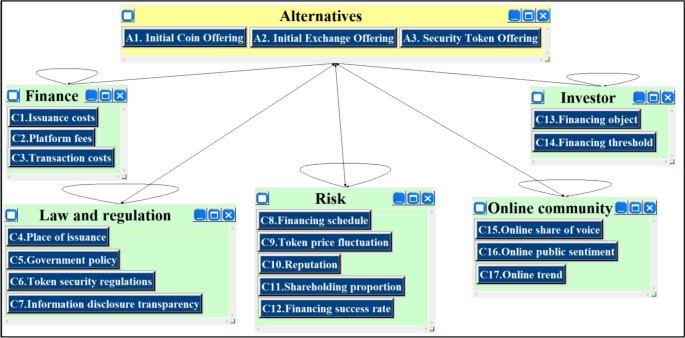
The research model
Step 1: Research the problem and confirm the decision factors
Past literature has pointed out that a research framework can be established only after experts reach a consensus on the factors [ 81 , 82 ]. Regarding the assessment of multiple principals, the number of selected experts should be between five and nine [ 76 ]. Therefore, this study included three scholars and four business starters, totaling seven experts. The goal of this study was to construct a consolidation-type pattern for the optimal scheme of token financing. Taking startup companies as examples, through a literature review and utilization of the Delphi method, a total of 17 factors, five clusters, and three token financing schemes were obtained, as shown in Fig. 4 . Relevant materials of each cluster and factors are shown as follows:
The definitions and illustrations of the clusters, factors, and token financing schemes in this study are as follows:
Finance: This includes issuance costs, platform fees, and transaction costs.
Issuance costs (C1) [ 83 , 84 ]: The costs of issuing tokens in different token financing schemes (for instance, Mint), which can vary.
Platform fees (C2) [ 83 ]: The costs for different token financing schemes to be launched on platforms (for instance, the costs for the schemes to be launched in Finance).
Transaction costs (C3) [ 83 ]: The transaction costs of different token financing schemes, which can vary (for instance, service charges).
Laws and regulations: This includes the place of issuance, government policy, token security regulations, and information disclosure transparency.
Place of issuance (C4): The laws, regulations, and rules of different countries and regions, as far as the issuance of tokens is concerned.
Policies (C5): The degree of support from government authorities on token financing.
Token security regulations (C6) [ 84 ]: The relevant policies on token security.
Information disclosure transparency (C7) [ 85 ]: Policies regarding the information disclosure of enterprises that issue tokens.
Risk: This includes financing schedules, token price fluctuations, reputation, shareholding proportion, and financing success rates.
Financing schedule (C8): The length of the financing scheme. For instance, Initial Coin Offerings (ICO) take a relatively long time, while Security Token Offerings (STO) take a relatively short time.
Token price fluctuations (C9) [ 83 ]: The price fluctuations of token transactions are obvious and influence relevant financing efficiency.
Reputation (C10) [ 86 ]: The degree of the token financing scheme’s requirements for the business reputation of the enterprises. For instance, ICO requires relatively less on the business reputation of the enterprises.
Shareholding proportion (C11): The proportion of shares corresponding to the tokens, which are held by the investors.
Financing success rates (C12) [ 87 ]: The success rates of different token financing schemes for enterprises.
The investor aspect: This includes the financing objects and financing thresholds.
Financing objects (C13): The investors being sought out by enterprises engaging in token financing. For instance, ICO and Initial Exchange Offerings (IEO) focus more on private investors, while STO focuses more on professional investors.
Financing thresholds (C14): The thresholds for enterprises to engage in token financing. For instance, the threshold of STO is relatively high.
The online community aspect: This includes the online sharing of voice, online public sentiment, and online trends.
Online sharing of voice (C15) [ 88 ]: The degree of influence of investors’ preferences of network volume in different financing platforms.
Online public sentiment (C16): The degree of influence of investor sentiment in the social network platforms of different financing platforms.
Online trends (C17): The degree of influence of the tendencies on the investors in the overall environment of token financing.
Token financing schemes: These include ICO, IEO, and STO.
ICO: The development, maintenance, and exchange for the purpose of financing, using blockchain technologies and virtual tokens.
IEO: The issuance and sales of tokens through the endorsement of exchanges. It also refers to the rules under which the exchanges are responsible for knowing your customer (KYC) compliance and anti-money laundering (AML).
STO: ICO is supervised by the government. It refers to the practice of linking the assets of enterprises to tokens through securitization, as well as the sales of such assets.
Step 2: Develop the network structure models through NSW
The results acquired in Step 1 were integrated into the NSW models suggested by this study, so as to determine the network structure. The relevant procedures are as follows:
Step 2.1: Design the questionnaire
In regards to the five clusters and 17 factors obtained by the experts in Step 1, a nine-point Likert scale was utilized to determine the strength of correlation between different factors. In the event of n factors, n ( n − 1) comparisons of the scale were carried out. Because this study referred to seven experts for the development of the network structure model, the data involved were quite complicated. The NSW procedures were illustrated in accordance with the finance clusters, as well as the three factors of issuance costs, platform fees, and transaction costs. The questionnaire design for the finance clusters is shown in Table 2 , in which 0 indicates no influence was observed, while 9 indicates the influence was of the highest level. The strength of correlation among the three factors of finance obtained through the questionnaires of the seven experts is shown in Fig. 5 . Each expert’s assessment is represented in a separate diagram, allowing for a comparison of individual perspectives. The differences in experts’ opinions highlight the subjective nature of these assessments and underscore the importance of aggregating their opinions. The generally strong correlations between factors, particularly between issuance costs and platform fees, suggest that these financial aspects are closely interrelated in token financing decisions. This visualization is crucial for understanding the foundation of our network structure, as it forms the basis for our NSW calculations.
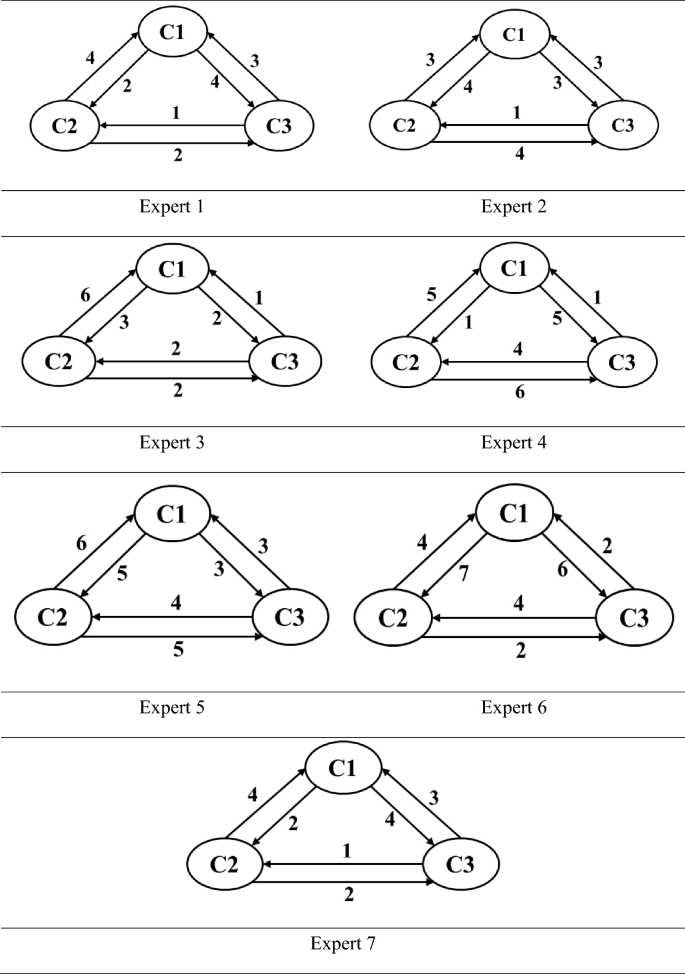
The strength of correlation among the three factors of finance obtained through the questionnaires of the seven experts
Step 2.2: Calculate the weight of the network structure
Each expert compared the factors and scored them in terms of strength. After that, the comparison scores provided by the experts were used in the construction of the matrices and weighted calculations. First, the correlation matrices of the finance clusters, M 1 to M 7 , were established on the basis of Eq. ( 1 ) and the scores of the strength given by the seven experts, as shown below. Second, correlation matrix M was transformed into probability matrices A 1 to A 7 through Eq. ( 2 ), as shown below, and the iteration method was used n times to obtain the characteristic values (eigenvalues) of each questionnaire and factor. Third, this study calculated the weighted values of the correlation among C 1 , C 2 , and C 3 , as well as R ( C i , C j ) 1 to R ( C i , C j ) 7 , through Eq. ( 3 ), as shown in Fig. 6 . This visualization is crucial for understanding how individual expert opinions contribute to the overall network structure. The variation in weights across experts highlights the subjective nature of these assessments and the necessity to aggregate multiple expert opinions. Notably, most experts consistently assign higher weights to the relationships between issuance costs ( C 1 ) and platform fees ( C 2 ), indicating a strong perceived connection between these two factors. In the end, the ultimate weighted values of the network structure (the scores of the correlation degree) were calculated using Eq. ( 4 ) and Eq. ( 5 ). The weighted values of the network structure of the various clusters and factors are shown in Fig. 7 . Figure 7 illustrates the final network structure weights for all five clusters and their respective factors, which is the foundation for our subsequent ANP analysis. These network structure weights provide a comprehensive understanding of the relative importance and interconnectedness of various factors in token financing decisions. They serve as a crucial input for our ANP model, ensuring that the final decision-making process accurately reflects the complex realities of token financing.
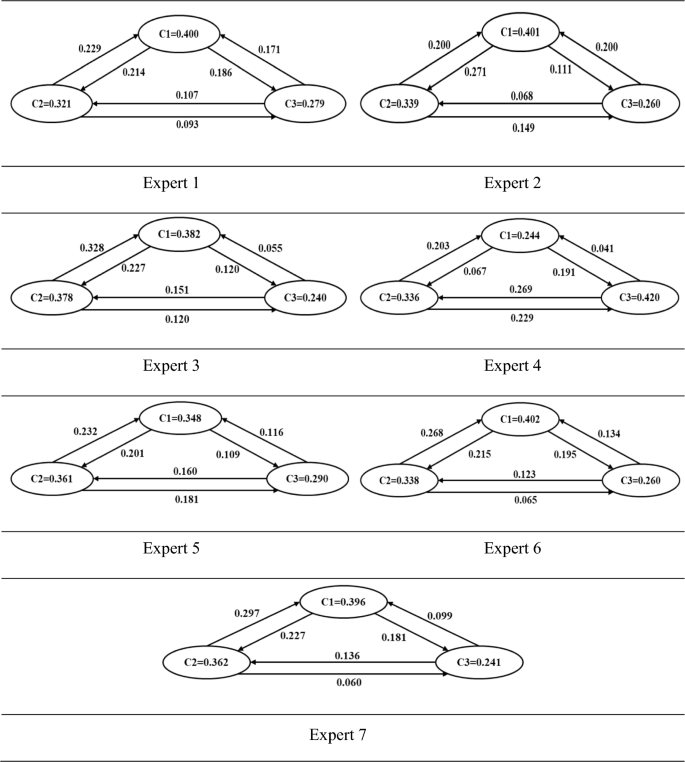
The network structure weights of finance cluster’s factors by 7 experts
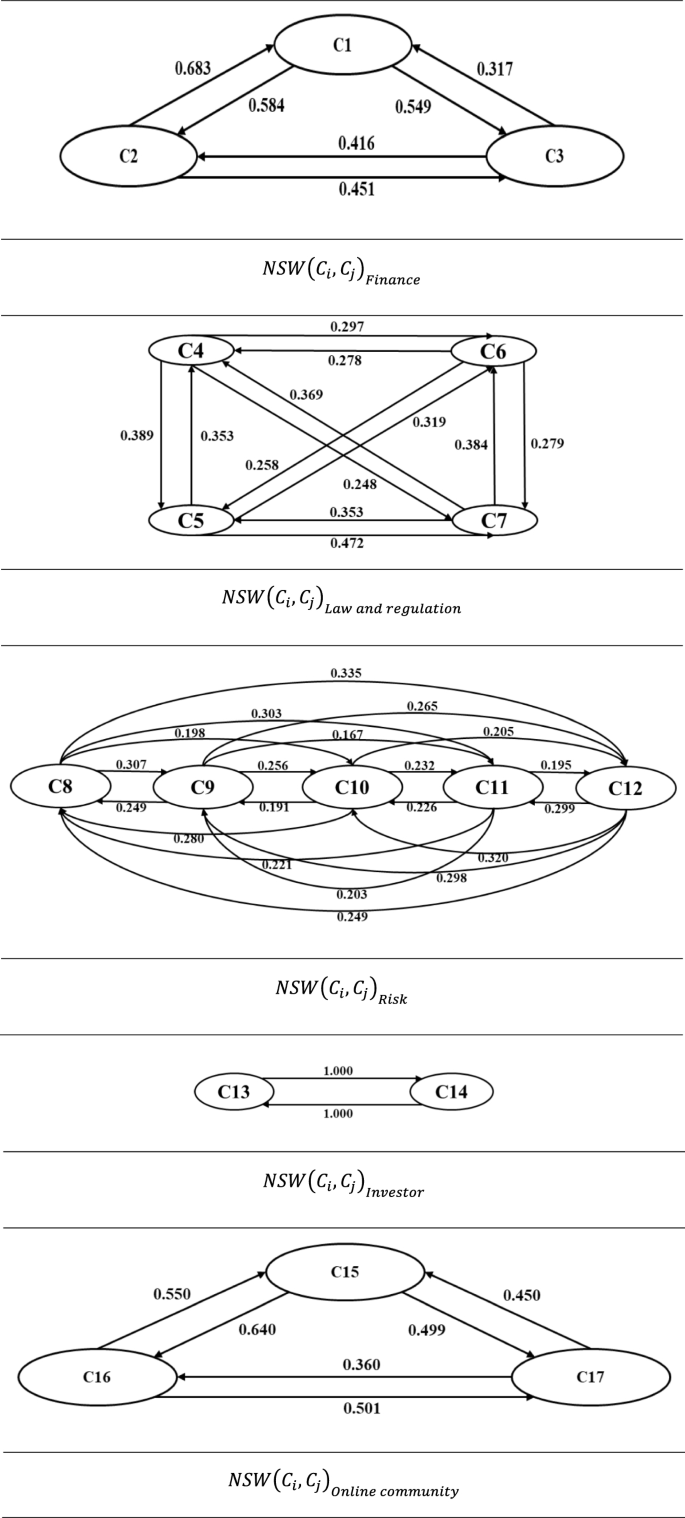
The network structure weights of five cluster’s factors
Upon completing the calculations, the results of the weighted values for the network structure were integrated into the ANP models to establish the comparison matrices and calculate the eigenvectors.
Step 3: Perform pair-wise comparisons of the matrices and priority vectors
The eigenvectors of the clusters and factors were calculated through the AHP processes and pairwise comparison of features of matrices. The eigenvectors of the degree of correlation between different clusters and factors were calculated through NSW. The cases in this study involved five clusters (finance, laws and regulations, risk, investor, and online community), 17 factors (issuance costs, platform fees, transaction costs, place of issuance, government policy, token security regulations, information disclosure transparency, financing schedules, token price fluctuations, reputation, shareholding proportion, financing success rates, financing objects, financing thresholds, online share of voice, online public sentiment, and online trends), as well as three schemes.
The comparison matrices (in pairs) and the geometric method were utilized to calculate the eigenvectors, while the eigenvectors for the network structure of the correlation strength scores were obtained on the basis of NSW. The eigenvectors obtained for the various comparison matrices, as well as the eigenvectors related to the correlation strength of the factors, served as the values of the supermatrix, which was used to illustrate the correlation strength and the relative importance of different clusters. The clusters might confirm the eigenvectors of the network structure through NSW, and the scores of the relative importance were calculated using Eq. ( 6 ). The results of the eigenvectors for the network structure of the various factors are shown in Step 2.2, and the comparison matrices (in pairs) and the weighted values of the five clusters are shown in Table 3 . Table 4 contains the scores for the relative importance of the various factors against the alternative schemes. In this study, Super Decision V2.0 (software) was utilized for the subsequent assessment of the ANP models. The eigenvectors of the network structure obtained through the NSW were inputted into Super Decision V2.0 to integrate NSW and ANP and assess the optimal scheme and the key factors.
Step 4: Construct the supermatrix
The eigenvectors of the relationships among the factors, as well as the eigenvectors regarding the weights of the factors to the schemes, were determined according to the results of Step 3. In Step 4, a supermatrix is established on the basis of the eigenvectors obtained in Step 3, so that the optimal scheme for startup companies engaging in token financing could be measured. During the ANP process, the ultimate weighted values of the various factors and schemes were calculated through the unweighted supermatrix, the weighted supermatrix, and the limit supermatrix. First, the calculated eigenvectors of the NSW model for the factors and pair-wise comparison matrices were utilized to establish the unweighted supermatrix. Second, the unweighted supermatrix was multiplied by the reciprocals of the weighted values of the relevant clusters to generate the weighted supermatrix. Finally, the results of the weighted supermatrix were multiplied by themselves repeatedly until a stable probability distribution was realized. This probability distribution reflected the ultimate weighted values to be reached. The various supermatrices are shown in Tables 5 , 6 , and 7 .
Step 5: Evaluate the optimal alternative
Through the supermatrix mentioned in Step 4, as well as the operation of Super Decision, the ultimate weighted values of the various factors and schemes under the consolidated NSW network structure could be obtained, as shown in Table 8 .
This study suggested the establishment of a set of network assessment procedures integrating the new NSW technique with the ANP model, in order to analyze the optimal scheme for startup companies engaging in token financing. The findings indicated a number of results. The sequence of the weighted values for the five clusters was as follows: finance (0.307) > risk (0.294) > laws and regulations (0.211) > investors (0.106) > online community (0.082). In addition, the sequence of the weighted values for the factors was as follows: platform fees (0.083) > issuance costs (0.078) > financing success rate (0.053) > government policy (0.0049) = financing schedule (0.049) > transaction costs (0.044) > financing threshold (0.040) > information disclosure transparency (0.039) > token price fluctuations (0.032) = shareholding proportion (0.032) > financing object (0.031) > reputation (0.030) > place of issuance (0.027) > token security regulations (0.026) > online share of voice (0.022) > online public sentiment (0.019) > online trend (0.014). Finally, the sequence of the optimal scheme for startup companies engaging in token financing is as follows: ICO (0.057) > IEO (0.101) > STO (0.175). STO is the optimal scheme for startup companies to engage in token financing.
4 Conclusion and Future Work
4.1 conclusion.
The rapid development of FinTech has become one of the goals of inclusive financing. Fintech, which depends on information technology to find solutions in the financial field, is becoming the mainstream future trend in the financial industry, especially in the development of new business patterns. Startup companies might find it difficult to borrow money from traditional financial institutions due to their business operation features and financial structures. For this reason, alternative financing has gradually become an important channel for startup companies to acquire financing. Token financing is a relatively new business pattern in the field of alternative financing, and it can avoid the shortcomings and problems of crowdfunding.
However, the development history of token financing is diversified and complicated. Previous studies in this field focused more on the analysis of the values of virtual currencies. Generally speaking, when startup companies are faced with the option of token financing, which is a new business pattern, they have relatively little information available for business assessments and decision making. When startup companies assess the optimal scheme for token financing, they often use multi-principle decision-making models, which can solve the problems of filtration and selection in token financing. However, multi-principle decision-making models depend heavily on the presumption that the variables (or criteria) are independent from each other. Therefore, such models might not be suitable for the assessment of decision-making problems in the real world.
ANP can be used to solve the problem of independence assumption in traditional multi-principle decision-making models. Although ANP can overcome the problem of independence assumption, it is still unable to ascertain the strength of the dependence and relationships between variables before producing a network structure. In this study, a new model, NSW, was put forward. This new model could be used to calculate the correlation between variables and generate the network structure. In addition, NSW could be integrated into ANP to generate the network structure. In the end, the assessment of the optimal scheme for startup companies engaging in token financing served as the case study. The results of this study show that finance is the most critical cluster in the assessment aspect. In other words, when startup companies intend to engage in token financing, financial issue is the first aspect to be considered. Token financing is the most up-to-date financing method in the era of FinTech, and capital turnover and financial structure are key issues during the development of startup companies. The sequence of key factors are platform fees, issuance costs, and financing success rate. Moreover, this sequence suggests that when startup companies intend to engage in token financing, the key factors are the aspect of costs and the success rate of financing. Finally, the optimal scheme for startup companies engaging in token financing is STO. After considering financial issues, costs, and relevant risks, startup companies should, based on the cost assessment and the success rate of financing, adopt STO for token financing to promote the financial efficiency of such companies.
This study proposed the NSW technique as a novel tool for validating network structures in decision-making processes and integrated NSW into the ANP model to develop a comprehensive framework for evaluating optimal token financing strategies. The contributions of this study in token-based financing include both methodological advancement and practical application. In terms of methodology, this study integrated the NSW technique with the ANP to enhance the robustness of existing frameworks in capturing complex interrelationships within decision-making processes. This innovative approach addresses limitations in traditional methods by providing a more comprehensive quantification of the strength and directionality of relationships between decision factors. As for practical application, this study presents the first comprehensive evaluation of token financing options for startup companies utilizing this advanced decision-making approach. The integrated NSW-ANP framework can be applied to ICO, IEO, and STO, thus offering valuable options for cryptocurrency-based startup financing. This systematic evaluation considers the intricate interdependencies among various factors influencing the selection of optimal financing strategies. By bridging the gap between theoretical innovation and practical implementation, this study not only advances the field of multi-criteria decision-making but also provides startup entrepreneurs and investors with a sophisticated tool for token-based financing options. Academically, this study provided a new NSW technique, as well as the application procedures to integrate NSW into ANP. This study also presented a case study of the assessment of the optimal scheme for startup companies engaging in token financing. Practically, this new framework could provide entrepreneurs of startup companies with valuable measurement tools for promoting their company’s capital turnover rate through token financing under the rapid development of FinTech.
4.2 Limitation and Future Research
While acknowledging the substantial advantages offered by our integrated framework, it is imperative to recognize its inherent limitations. The following constraints warrant further investigation and potential mitigation in future research:
The potential complexity and mathematical technique of the proposed model, which might make it challenging to implement for organizations.
The static nature of the model, which may not fully capture the decision risks of uncertainty in the cryptocurrency and token financing landscape.
At the current stage of development, the model may not comprehensively capture the effects of factor weight variations on the rankings of alternatives.
After discussing these limitations, we will outline potential directions for future research. This section will propose several avenues for extending and refining our work:
Expanding the application of the NSW-ANP method to other areas of FinTech decision-making beyond token financing.
Integration of fuzzy set theory into the NSW-ANP model to address decision uncertainty risks.
A sensitivity analysis was conducted to ascertain the effects of factor weight variations on the rankings of alternatives.
Data Availability
Not applicable.
Ivanov, V., Knyazeva, A.: US securities-based crowdfunding under Title III of the JOBS Act. In: DERA White Paper (2017). Accessed 15 Jan 2022
Rossi, M.: The new ways to raise capital: an exploratory study of crowdfunding. Int. J. Financ. Res. 5 (2), 8–18 (2014)
Article Google Scholar
Walthoff-Borm, X., Schwienbacher, A., Vanacker, T.: Equity crowdfunding: first resort or last resort? J. Bus. Ventur. 33 (4), 513–533 (2018)
Massolution. (2015). 2015CF: The Crowdfunding Industry Report. Massolution. https://www.smv.gob.pe/Biblioteca/temp/catalogacion/C8789.pdf . Accessed 20 Jan 2022
Statista Inc.: Alternative Financing Report 2021 (2021). https://www.statista.com/study/47352/fintech-report-alternative-financing/ . Accessed 10 Jan 2022
Lu, Y., Chang, R., Lim, S.: Crowdfunding for solar photovoltaics development: a review and forecast. Renew. Sustain. Energy Rev. 93 , 439–450 (2018)
Bagheri, A., Chitsazan, H., Ebrahimi, A.: Crowdfunding motivations: a focus on donors’ perspectives. Technol. Forecast. Soc. Chang. 146 , 218–232 (2019)
Petruzzelli, A.M., Natalicchio, A., Panniello, U., Roma, P.: Understanding the crowdfunding phenomenon and its implications for sustainability. Technol. Forecast. Soc. Chang. 141 , 138–148 (2019)
Estrin, S., Gozman, D., Khavul, S.: Case study of the equity crowdfunding landscape in London: an entrepreneurial and regulatory perspective. FIRES Case Study 5 (2), 1–62 (2016)
Google Scholar
Kuti, M., Madarász, G.: Crowdfunding. Public Financ. Q. 59 (3), 355 (2014)
Agrawal, A., Catalini, C., Goldfarb, A.: Some simple economics of crowdfunding. Innov. Policy Econ. 14 (1), 63–97 (2014)
Zhu, H., Zhou, Z.Z.: Analysis and outlook of applications of blockchain technology to equity crowdfunding in China. Financ. Innov. 2 (1), 29 (2016)
Baber, H.: Blockchain-based crowdfunding. In: Blockchain Technology for Industry 4.0, pp. 117–130. Springer, Singapore (2020)
Chapter Google Scholar
Chod, J., Trichakis, N., Yang, S.A.: Platform tokenization: financing, governance, and moral hazard. Manage. Sci. 68 (9), 6411–6433 (2021)
Howell, S.T., Niessner, M., Yermack, D.: Initial coin offerings: financing growth with cryptocurrency token sales. Rev. Financ. Stud. 33 (9), 3925–3974 (2020)
Amsden, R., Schweizer, D.: Are blockchain crowdsales the new ‘gold rush’? Success determinants of initial coin offerings (April 16, 2018) (2018)
Ante, L., Fiedler, I.: Cheap signals in security token offerings. Quant. Financ. Econ. 4 (4), 608–639 (2020)
Miglo, A.: Choice between IEO and ICO: speed vs. liquidity vs. risk. FinTech 1 (3), 276–293 (2022)
Kondova, G., Simonella, G.: Blockchain in startup financing: ICOs and STOs in Switzerland. J. Strateg. Innov. Sustain. 14 (6), 43–48 (2019)
Gryglewicz, S., Mayer, S., Morellec, E.: Optimal financing with tokens. J. Financ. Econ. 142 (3), 1038–1067 (2021)
Canh, N.P., Wongchoti, U., Thanh, S.D., Thong, N.T.: Systematic risk in cryptocurrency market: evidence from DCC-MGARCH model. Financ. Res. Lett. 29 , 90–100 (2019)
Borri, N.: Conditional tail-risk in cryptocurrency markets. J. Empir. Financ. 50 , 1–19 (2019)
Liu, Y., Tsyvinski, A.: Risks and returns of cryptocurrency. Rev. Financ. Stud. 34 (6), 2689–2727 (2021)
Zhang, W., Li, Y., Xiong, X., Wang, P.: Downside risk and the cross-section of cryptocurrency returns. J. Bank. Finance 133 , 106246 (2021)
Borri, N., Shakhnov, K.: Regulation spillovers across cryptocurrency markets. Financ. Res. Lett. 36 , 101333 (2020)
Feinstein, B.D., Werbach, K.: The impact of cryptocurrency regulation on trading markets. J. Financ. Regul. 7 (1), 48–99 (2021)
Chokor, A., Alfieri, E.: Long and short-term impacts of regulation in the cryptocurrency market. Q. Rev. Econ. Finance 81 , 157–173 (2021)
Beneki, C., Koulis, A., Kyriazis, N.A., Papadamou, S.: Investigating volatility transmission and hedging properties between Bitcoin and Ethereum. Res. Int. Bus. Financ. 48 , 219–227 (2019)
Okorie, D.I., Lin, B.: Crude oil price and cryptocurrencies: evidence of volatility connectedness and hedging strategy. Energy Econ. 87 , 104703 (2020)
Thampanya, N., Nasir, M.A., Huynh, T.L.D.: Asymmetric correlation and hedging effectiveness of gold & cryptocurrencies: from pre-industrial to the 4th industrial revolution✰. Technol. Forecast. Soc. Chang. 159 , 120195 (2020)
Sebastião, H., Godinho, P.: Bitcoin futures: an effective tool for hedging cryptocurrencies. Financ. Res. Lett. 33 , 101230 (2020)
Walther, T., Klein, T., Bouri, E.: Exogenous drivers of bitcoin and cryptocurrency volatility—a mixed data sampling approach to forecasting. J. Int. Finan. Markets. Inst. Money 63 , 101133 (2019)
Ma, F., Liang, C., Ma, Y., Wahab, M.I.M.: Cryptocurrency volatility forecasting: a Markov regime-switching MIDAS approach. J. Forecast. 39 (8), 1277–1290 (2020)
Article MathSciNet Google Scholar
Köchling, G., Schmidtke, P., Posch, P.N.: Volatility forecasting accuracy for Bitcoin. Econ. Lett. 191 , 108836 (2020)
Yen, K.C., Cheng, H.P.: Economic policy uncertainty and cryptocurrency volatility. Financ. Res. Lett. 38 , 101428 (2021)
Hamdan, S., Cheaitou, A.: Supplier selection and order allocation with green criteria: an MCDM and multi-objective optimization approach. Comput. Oper. Res. 81 , 282–304 (2017)
Lin, S.W.: Identifying the critical success factors and an optimal solution for mobile technology adoption in travel agencies. Int. J. Tour. Res. 19 (2), 127–144 (2017)
Lin, C.Y.: Optimal core operation in supply chain finance ecosystem by integrating the fuzzy algorithm and hierarchical framework. Int. J. Comput. Intell. Syst. 13 (1), 259–274 (2020)
Kilic, B., Ucler, C.: Stress among ab-initio pilots: a model of contributing factors by AHP. J. Air Transp. Manag. 80 , 101706 (2019)
Achu, A.L., Thomas, J., Reghunath, R.: Multi-criteria decision analysis for delineation of groundwater potential zones in a tropical river basin using remote sensing, GIS and analytical hierarchy process (AHP). Groundw. Sustain. Dev. 10 , 100365 (2020)
Gündoğdu, F.K., Duleba, S., Moslem, S., Aydın, S.: Evaluating public transport service quality using picture fuzzy analytic hierarchy process and linear assignment model. Appl. Soft Comput. 100 , 106920 (2021)
Awad, J., Jung, C.: Extracting the planning elements for sustainable urban regeneration in Dubai with AHP (analytic hierarchy process). Sustain. Cities Soc. 76 , 103496 (2022)
Jorge-García, D., Estruch-Guitart, V.: Comparative analysis between AHP and ANP in prioritization of ecosystem services—a case study in a rice field area raised in the Guadalquivir marshes (Spain). Eco. Inform. 70 , 101739 (2022)
Tsai, W.H., Chou, W.C.: Selecting management systems for sustainable development in SMEs: a novel hybrid model based on DEMATEL, ANP, and ZOGP. Expert Syst. Appl. 36 (2), 1444–1458 (2009)
Saaty, T.L.: Decision Making with Dependence and Feedback: The Analytic Network Process, vol. 4922. RWS publications, Pittsburgh (1996)
Munim, Z.H., Duru, O., Ng, A.K.: Transhipment port’s competitiveness forecasting using analytic network process modelling. Transp. Policy 124 , 70–82 (2022)
Eshtiaghi, K., Aliyannezhadi, M., Najafian, A.: Identification and prioritization of factors affecting the adoption of electric vehicles using analytic network process. Int. J. Hum. Cap. Urban Manag 6 , 323–336 (2021)
Pang, N., Nan, M., Meng, Q., Zhao, S.: Selection of wind turbine based on fuzzy analytic network process: a case study in China. Sustainability 13 (4), 1792 (2021)
Asadi, A., Moghaddam Nia, A., Bakhtiari Enayat, B., Alilou, H., Ahmadisharaf, E., Kimutai Kanda, E., Chessum Kipkorir, E.: An integrated approach for prioritization of river water quality sampling points using modified Sanders, analytic network process, and hydrodynamic modeling. Environ. Monit. Assess. 193 (8), 1–15 (2021)
Toth, W., Vacik, H., Pülzl, H., Carlsen, H.: Deepening our understanding of which policy advice to expect from prioritizing SDG targets: introducing the analytic network process in a multi-method setting. Sustain. Sci. 17 (4), 1473–1488 (2022)
Fallahpour, A., Nayeri, S., Sheikhalishahi, M., Wong, K.Y., Tian, G., Fathollahi-Fard, A.M.: A hyper-hybrid fuzzy decision-making framework for the sustainable-resilient supplier selection problem: a case study of Malaysian palm oil industry. Environ. Sci. Pollut. Res. (2021). https://doi.org/10.1007/s11356-021-12491-y
Mabrouk, N.: Green supplier selection using fuzzy Delphi method for developing sustainable supply chain. Decis. Sci. Lett. 10 (1), 63–70 (2021)
Thanh, N.V., Lan, N.T.K.: A new hybrid triple bottom line metrics and fuzzy MCDM model: sustainable supplier selection in the food-processing industry. Axioms 11 (2), 57 (2022)
Erol, H., Dikmen, I., Atasoy, G., Birgonul, M.T.: An analytic network process model for risk quantification of mega construction projects. Expert Syst. Appl. 191 , 116215 (2022)
Tabatabaee, S., Mahdiyar, A., Mohandes, S.R., Ismail, S.: Towards the development of a comprehensive lifecycle risk assessment model for green roof implementation. Sustain. Cities Soc. 76 , 103404 (2022)
Rehman, O., Ali, Y., Sabir, M.: Risk assessment and mitigation for electric power sectors: a developing country’s perspective. Int. J. Crit. Infrastruct. Prot. 36 , 100507 (2022)
Moghaddasi, K., Rajabi, S., Soleimanian Gharehchopogh, F., Hosseinzadeh, M.: An energy-efficient data offloading strategy for 5G-enabled vehicular edge computing networks using double deep Q-network. Wireless Pers. Commun. 133 (3), 2019–2064 (2023)
Gharehchopogh, F.S., Abdollahzadeh, B., Barshandeh, S., Arasteh, B.: A multi-objective mutation-based dynamic Harris Hawks optimization for botnet detection in IoT. Internet of Things 24 , 100952 (2023)
Moghaddasi, K., Rajabi, S., Gharehchopogh, F.S.: Multi-objective secure task offloading strategy for blockchain-enabled IoV-MEC systems: a double deep Q-network approach. IEEE Access 12 , 3437–3463 (2024)
Moghaddasi, K., Rajabi, S., Gharehchopogh, F.S.: An enhanced asynchronous advantage actor-critic-based algorithm for performance optimization in mobile edge computing-enabled internet of vehicles networks. Peer-to-Peer Netw. Appl. 17 , 1169–1189 (2024)
Moghaddasi, K., Rajabi, S., Gharehchopogh, F.S., Ghaffari, A.: An advanced deep reinforcement learning algorithm for three-layer D2D-edge-cloud computing architecture for efficient task offloading in the internet of things. Sustain. Comput.: Inf. Syst. 43 , 100992 (2024)
Kashyap, A., Kumar, C., Shukla, O.J.: A DEMATEL model for identifying the impediments to the implementation of circularity in the aluminum industry. Decis. Anal. J. 5 , 100134 (2022)
Kamranfar, S., Azimi, Y., Gheibi, M., Fathollahi-Fard, A.M., Hajiaghaei-Keshteli, M.: Analyzing green construction development barriers by a hybrid decision-making method based on DEMATEL and the ANP. Buildings 12 (10), 1641 (2022)
Karasan, A., Ilbahar, E., Cebi, S., Kahraman, C.: Customer-oriented product design using an integrated neutrosophic AHP & DEMATEL & QFD methodology. Appl. Soft Comput. 118 , 108445 (2022)
Nezhad, M.Z., Nazarian-Jashnabadi, J., Rezazadeh, J., Mehraeen, M., Bagheri, R.: Assessing dimensions influencing IoT implementation readiness in industries: a fuzzy DEMATEL and fuzzy AHP analysis. J. Soft Comput. Decis. Anal. 1 (1), 102–123 (2023)
Du, Y.W., Shen, X.L.: Group hierarchical DEMATEL method for reaching consensus. Comput. Ind. Eng. 175 , 108842 (2023)
Si, S.L., You, X.Y., Liu, H.C., Zhang, P.: DEMATEL technique: a systematic review of the state-of-the-art literature on methodologies and applications. Math. Probl. Eng. 2018 , 1–33 (2018)
Sun, Y., Zhang, S., Huang, Z., Miao, B.: Probabilistic linguistic-based group DEMATEL method with both positive and negative influences. Complexity 2021 , 1–20 (2021)
Šmidovnik, T., Grošelj, P.: Solution for convergence problem in DEMATEL method: DEMATEL of finite sum of influences. Symmetry 15 (7), 1357 (2023)
Linstone, H.A., Turoff, M. (eds.): The Delphi method, pp. 3–12. Addison-Wesley, Reading, MA (1975)
Murry, J.W., Jr., Hammons, J.O.: Delphi: a versatile methodology for conducting qualitative research. Rev. High. Educ. 18 (4), 423–436 (1995)
Skulmoski, G.J., Hartman, F.T., Krahn, J.: The Delphi method for graduate research. J. Inf. Technol. Educ.: Res. 6 (1), 1–21 (2007)
Okoli, C., Pawlowski, S.D.: The Delphi method as a research tool: an example, design considerations and applications. Information and Management 42 (1), 15–29 (2004)
Wu, C.R., Lin, C.T., Chen, H.C.: Evaluating competitive advantage of the location for Taiwanese hospitals. J. Inf. Optim. Sci. 28 (5), 841–868 (2007)
Sung, W.C.: Application of Delphi method, a qualitative and quantitative analysis, to the healthcare management. J. Healthc. Manag. 2 (2), 11–19 (2001)
Hasson, F., Keeney, S.: Enhancing rigour in the Delphi technique research. Technol. Forecast. Soc. Chang. 78 (9), 1695–1704 (2011)
Atmaca, E., Basar, H.B.: Evaluation of power plants in Turkey using analytic network process (ANP). Energy 44 (1), 555–563 (2012)
Keramati, A., Salehi, M.: Website success comparison in the context of e-recruitment: an analytic network process (ANP) approach. Appl. Soft Comput. 13 (1), 173–180 (2013)
Meade, L., Sarkis, J.: Strategic analysis of logistics and supply chain management systems using the analytical network process. Transp. Res. E: Logist. Transp. Rev. 34 (3), 201–215 (1998)
Saaty, T.L., Vargas, L.G.: Decision making with the analytic network process, vol. 282. Springer, Berlin (2006)
Ali-Yrkko, J., Rouvinen, P., Seppala, T., Yla-Anttila, P.: Who captures value in global supply chains? Case Nokia N95 smartphone. J. Ind. Compet. Trade 11 (3), 63–278 (2011)
Linden, G., Kraemer, K.L., Dedrick, J.: Who captures value in a global innovation network? The case of Apple’s iPod. Commun. ACM 52 (3), 140–144 (2009)
Cong, L.W., Li, Y., Wang, N.: Tokenomics: dynamic adoption and valuation. Rev. Financ. Stud. 34 (3), 1105–1155 (2021)
Myalo, A.S.: Comparative analysis of ICO, DAOICO, IEO and STO. Case study. Financ. Theory Pract. 23 (6), 6–25 (2019)
Chod, J., Lyandres, E.: A theory of ICOS: diversification, agency, and information asymmetry. Manage. Sci. 67 (10), 5969–5989 (2021)
Momtaz, P.P.: Entrepreneurial finance and moral hazard: evidence from token offerings. J. Bus. Ventur. 36 (5), 106001 (2021)
Giudici, G., Adhami, S.: The impact of governance signals on ICO fundraising success. J. Ind. Bus. Econ. 46 (2), 283–312 (2019)
Fisch, C., Masiak, C., Vismara, S., Block, J.: Motives and profiles of ICO investors. J. Bus. Res. 125 , 564–576 (2021)
Download references
This research received no external funding.
Author information
Authors and affiliations.
Department of Public Finance and Tax Administration, National Taipei University of Business, 321, Sec. 1, Jinan Rd., Zhongzheng District, Taipei, 100, Taiwan
Chun-Yueh Lin
You can also search for this author in PubMed Google Scholar
Contributions
Research design, literature review, data collection, data analysis, and manuscript writing are all conducted by Chun Yueh Lin.
Corresponding author
Correspondence to Chun-Yueh Lin .
Ethics declarations
Conflict of interest.
The authors declare no conflict of interest.
Additional information
Publisher's note.
Springer Nature remains neutral with regard to jurisdictional claims in published maps and institutional affiliations.
Rights and permissions
Open Access This article is licensed under a Creative Commons Attribution-NonCommercial-NoDerivatives 4.0 International License, which permits any non-commercial use, sharing, distribution and reproduction in any medium or format, as long as you give appropriate credit to the original author(s) and the source, provide a link to the Creative Commons licence, and indicate if you modified the licensed material. You do not have permission under this licence to share adapted material derived from this article or parts of it. The images or other third party material in this article are included in the article’s Creative Commons licence, unless indicated otherwise in a credit line to the material. If material is not included in the article’s Creative Commons licence and your intended use is not permitted by statutory regulation or exceeds the permitted use, you will need to obtain permission directly from the copyright holder. To view a copy of this licence, visit http://creativecommons.org/licenses/by-nc-nd/4.0/ .
Reprints and permissions
About this article
Lin, CY. Constructing a Novel Network Structure Weighting Technique into the ANP Decision Support System for Optimal Alternative Evaluation: A Case Study on Crowdfunding Tokenization for Startup Financing. Int J Comput Intell Syst 17 , 222 (2024). https://doi.org/10.1007/s44196-024-00643-0
Download citation
Received : 19 March 2024
Accepted : 19 August 2024
Published : 26 August 2024
DOI : https://doi.org/10.1007/s44196-024-00643-0
Share this article
Anyone you share the following link with will be able to read this content:
Sorry, a shareable link is not currently available for this article.
Provided by the Springer Nature SharedIt content-sharing initiative
- Crowdfunding
- Token financing
- Network structure weighting (NSW)
- Analytic network process (ANP)
- Find a journal
- Publish with us
- Track your research
Information
- Author Services
Initiatives
You are accessing a machine-readable page. In order to be human-readable, please install an RSS reader.
All articles published by MDPI are made immediately available worldwide under an open access license. No special permission is required to reuse all or part of the article published by MDPI, including figures and tables. For articles published under an open access Creative Common CC BY license, any part of the article may be reused without permission provided that the original article is clearly cited. For more information, please refer to https://www.mdpi.com/openaccess .
Feature papers represent the most advanced research with significant potential for high impact in the field. A Feature Paper should be a substantial original Article that involves several techniques or approaches, provides an outlook for future research directions and describes possible research applications.
Feature papers are submitted upon individual invitation or recommendation by the scientific editors and must receive positive feedback from the reviewers.
Editor’s Choice articles are based on recommendations by the scientific editors of MDPI journals from around the world. Editors select a small number of articles recently published in the journal that they believe will be particularly interesting to readers, or important in the respective research area. The aim is to provide a snapshot of some of the most exciting work published in the various research areas of the journal.
Original Submission Date Received: .
- Active Journals
- Find a Journal
- Proceedings Series
- For Authors
- For Reviewers
- For Editors
- For Librarians
- For Publishers
- For Societies
- For Conference Organizers
- Open Access Policy
- Institutional Open Access Program
- Special Issues Guidelines
- Editorial Process
- Research and Publication Ethics
- Article Processing Charges
- Testimonials
- Preprints.org
- SciProfiles
- Encyclopedia
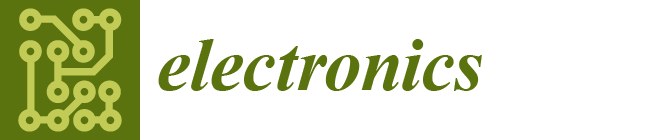
Article Menu

- Subscribe SciFeed
- Recommended Articles
- Google Scholar
- on Google Scholar
- Table of Contents
Find support for a specific problem in the support section of our website.
Please let us know what you think of our products and services.
Visit our dedicated information section to learn more about MDPI.
JSmol Viewer
Research on energy management in hydrogen–electric coupled microgrids based on deep reinforcement learning.

1. Introduction
- Intelligent hydrogen–electric coupled microgrid energy management strategy: This paper proposes an energy management strategy based on the DDPG. A deep neural network is used to simulate and optimize the energy management strategy of the microgrid by combining the forecast data of PV generation and load demand. The strategy can effectively cope with the influence of uncertain factors, such as PV generation, EV charging loads, and hydrogen charging loads on the optimization results, and ensure that the system supply and demand are balanced throughout the dispatch cycle.
- Optimization of system operation economics and the reduction in light shedding: The DDPG algorithm operates hydrogen production from excess power during peak PV generation hours, which achieves full utilization of PV power and reduces light shedding. In addition, the method achieves a reduction in the system power purchase cost and improves the overall economic efficiency through the operation of charging and hydrogen production during low-price hours and discharging and selling power during high-price hours.
- Load smoothing and grid stability enhancement: Through the optimal scheduling of EV charging loads, the time and magnitude of peak loads are reduced, and the optimized charging load curves are smoother, which significantly reduces the gap between the peaks and valleys of the grid loads and thus enhances the stability and operational efficiency of the grid.
- The effectiveness and superiority of the DDPG algorithm are verified: The accuracy and effectiveness of the DDPG algorithm over the traditional DQN in dealing with continuous action decision-making problems are verified through case studies. The DDPG algorithm is more capable of optimizing the energy management of the microgrid under complex constraints, which significantly reduces the operating cost of the microgrid.
2. Hydrogen–Electric Coupled Microgrid Structure
3. distributed energy system models, 3.1. photovoltaic power generation model, 3.2. battery energy storage system model, 3.3. electrolytic hydrogen production model, 3.4. hydrogen fuel cell model, 3.5. model of hydrogen storage facilities, 4. decision-making model for microgrid energy management, 4.1. objective function, 4.2. constraints, 4.2.1. power and energy balance constraints, 4.2.2. constraints on the operation of photovoltaic power generation systems, 4.2.3. electrolytic hydrogen production system operational constraints.
- Operational Constraints of Electrolytic Cells
4.2.4. Electrochemical Energy Storage Operational Constraints
4.2.5. constraints on the operation of charging/hydrogen cells.
- Charging Load Constraints
5. Optimization Algorithms for Deep Reinforcement Learning
5.1. the principles of the ddpg algorithm, 5.2. implementation of the ddpg algorithm.
- Definition of the State Space
Energy Management Method for PV-Storage-Charging Integrated System Based on DDPG. | |
1: | |
2: | Initialize target networks and , |
3: | Initialize replay buffer |
4: | Set soft update coefficient and learning rate |
5: | episode =1 to max_episodes |
6: | Initialize random process for action exploration |
7: | |
8: | to max_steps |
9: | based on the current policy and exploration |
noise | |
10: | |
11: | in replay buffer |
12: | from |
13: | |
14: | |
15: | Update Actor network using the sampled policy gradient: |
16: | Soft update target networks: |
17: | |
18: | |
19: |
6. Case Study Analysis
6.1. case description, 6.2. simulation analysis, 7. conclusions.
- In hydrogen–electric coupled microgrids, the energy management system can intelligently adjust charging and discharging strategies based on electricity price signals and photovoltaic generation through the DDPG algorithm, achieving “buy low, sell high” operations.
- The DDPG algorithm takes into account the volatility of photovoltaic generation, the uncertainties of charging/hydrogen loads, and other uncertain factors, ensuring supply–demand balance between photovoltaic generation, electric vehicle charging/hydrogen loads, and the energy storage system during the scheduling period, thus enhancing the reliability and stability of system operation.
- Through the DDPG algorithm, hydrogen–electric coupled microgrids can participate in flexible grid regulation based on electricity price incentive signals by adjusting charging loads and energy storage systems, reducing peak loads, and improving grid stability and economic efficiency.
- The accuracy of the DDPG algorithm in continuous action problems has been validated through comparisons with the DQN algorithm.
Author Contributions
Data availability statement, conflicts of interest.
- Shi, T.; Sheng, J.; Chen, Z.; Zhou, H. Simulation Experiment Design and Control Strategy Analysis in Teaching of Hydrogen-Electric Coupling System. Processes 2024 , 12 , 138. [ Google Scholar ] [ CrossRef ]
- Cai, G.; Chen, C.; Kong, L.; Peng, L.; Zhang, H. Modeling and Control of grid-connected system of wind power/photovoltaic/Hydrogen production/Supercapacitor. Power Syst. Technol. 2016 , 40 , 2982–2990. [ Google Scholar ] [ CrossRef ]
- Zhang, R.; Li, X.; Wang, X.; Wang, Q.; Qi, Z. Optimal scheduling for hydrogen-electric hybrid microgrid with vehicle to grid technology. In 2021 China Automation Congress (CAC) ; IEEE: Piscataway, NJ, USA, 2021; pp. 6296–6300. [ Google Scholar ]
- Guanghui, L. Research on Modeling and Optimal Control of Wind-Wind Hydrogen Storage Microgrid System. Master’s Thesis, North China University of Technology, Beijing, China, 2024. [ Google Scholar ]
- Huo, Y.; Wu, Z.; Dai, J.; Huo, Y.; Wu, Z.; Dai, J.; Duan, W.; Zhao, H.; Jiang, J.; Yao, R. An Optimal Dispatch Method for the Hydrogen-Electric Coupled Energy Microgrid. In World Hydrogen Technology Convention ; Springer Nature Singapore: Singapore, 2023; pp. 69–75. [ Google Scholar ]
- Hou, L.; Dong, J.; Herrera, O.E.; Mérida, W. Energy management for solar-hydrogen microgrids with vehicle-to-grid and power-to-gas transactions. Int. J. Hydrogen Energy 2023 , 48 , 2013–2029. [ Google Scholar ] [ CrossRef ]
- Yu, L.; Qin, S.; Zhang, M.; Shen, C.; Jiang, T.; Guan, X. Deep reinforcement learning for smart building energy management: A survey. arXiv 2020 , arXiv:2008.05074. [ Google Scholar ]
- Zheng, J.; Song, Q.; Wu, G.; Chen, H.; Hu, Z.; Chen, Z.; Weng, C.; Chen, J. Low-carbon operation strategy of regional integrated energy system based on the Q learning algorithm. J. Electr. Power Sci. Technol. 2022 , 37 , 106–115. [ Google Scholar ]
- Xu, H.; Lu, J.; Yang, Z.; Li, Y.; Lu, J.; Huang, H. Decision optimization model of incentive demand response based on deep reinforcement learning. Autom. Electr. Power Syst. 2021 , 45 , 97–103. [ Google Scholar ]
- Shuai, C. Microgrid Energy Management and Scheduling Based on Reinforcement Learning. Ph.D. Thesis, University of Science and Technology Beijing, Beijing, China, 2023. [ Google Scholar ]
- Kim, B.; Zhang, Y.; Van Der Schaar, M.; Lee, J.W. Dynamic pricing and energy consumption scheduling with reinforcement learning. IEEE Trans. Smart Grid 2016 , 7 , 2187–2198. [ Google Scholar ] [ CrossRef ]
- Shi, T.; Xu, C.; Dong, W.; Zhou, H.; Bokhari, A.; Klemeš, J.J.; Han, N. Research on energy management of hydrogen electric coupling system based on deep reinforcement learning. Energy 2023 , 282 , 128174. [ Google Scholar ] [ CrossRef ]
- Liu, J.; Chen, J.; Wang, X.; Zeng, J.; Huang, Q. Research on Energy Management and Optimization Strategy of micro-energy networks based on Deep Reinforcement Learning. Power Syst. Technol. 2020 , 44 , 3794–3803. [ Google Scholar ] [ CrossRef ]
- Ji, Y.; Wang, J.; Xu, J.; Fang, X.; Zhang, H. Real-Time Energy Management of a Microgrid Using Deep Reinforcement Learning. Energies 2019 , 12 , 2291. [ Google Scholar ] [ CrossRef ]
- Darshi, R.; Shamaghdari, S.; Jalali, A.; Arasteh, H. Decentralized Reinforcement Learning Approach for Microgrid Energy Management in Stochastic Environment. Int. Trans. Electr. Energy Syst. 2023 , 2023 , 1190103. [ Google Scholar ] [ CrossRef ]
- Kolodziejczyk, W.; Zoltowska, I.; Cichosz, P. Real-Time Energy Purchase Optimization for a Storage-Integrated Photovoltaic System by Deep Reinforcement Learning. Control Eng. Pract. 2021 , 106 , 104598. [ Google Scholar ] [ CrossRef ]
- Nicola, M.; Nicola, C.I.; Selișteanu, D. Improvement of the Control of a Grid Connected Photovoltaic System Based on Synergetic and Sliding Mode Controllers Using a Reinforcement Learning Deep Deterministic Policy Gradient Agent. Energies 2022 , 15 , 2392. [ Google Scholar ] [ CrossRef ]
- Wang, C.; Zhang, J.; Wang, A.; Wang, Z.; Yang, N.; Zhao, Z.; Lai, C.S.; Lai, L.L. Prioritized sum-tree experience replay TD3 DRL-based online energy management of a residential microgrid. Appl. Energy 2024 , 368 , 123471. [ Google Scholar ] [ CrossRef ]
- Guo, C.; Wang, X.; Zheng, Y.; Zhang, F. Real-time optimal energy management of microgrid with uncertainties based on deep reinforcement learning. Energy 2022 , 238 , 121873. [ Google Scholar ] [ CrossRef ]
- Benhmidouch, Z.; Moufid, S.; Ait-Omar, A.; Abbou, A.; Laabassi, H.; Kang, M.; Chatri, C.; Ali, I.H.O.; Bouzekri, H.; Baek, J. A novel reinforcement learning policy optimization based adaptive VSG control technique for improved frequency stabilization in AC microgrids. Electr. Power Syst. Res. 2024 , 230 , 110269. [ Google Scholar ] [ CrossRef ]
Click here to enlarge figure
Parameter | Values |
---|---|
Photovoltaic array | 600 kW |
Capacity of electrical energy storage system | 72–288 kW·h |
Electrical energy storage power rating | 100 kW |
Electrolyzer rated power | 750 kW |
Capacity of hydrogen storage tank | 1000 Nm |
Capacity of charging | 30 × 30 kW |
Total refueling rate | 30 × 5 Nm /h |
Batch size | 64 |
Hydrogen refueling service price | 5.8 ¥/Nm |
Carbon trading price | 0.07 ¥/kg |
Parameter | Values |
---|---|
Hidden layer | [400, 300, 256, 128] |
Actor network learning rate | 0.001 |
Critic network learning rate | 0.001 |
Target network learning rate | 0.001 |
Discount factor | 0.99 |
Episodes | 1000 |
Step size | 100 |
Batch size | 64 |
Experience playback pool capacity | 20,000 |
Hidden layer | [400, 300, 256, 128] |
Actor network learning rate | 0.001 |
Cost (CNY) | Before Optimization | DQN | DDPG |
---|---|---|---|
Power purchase cost | 9625.27 | 9038.19 | 8677.2 |
Charging income | 8056.33 | 7783.61 | 7838.3 |
Hydrogen charge yield | 11,314.79 | 11,201.6421 | 11,314.79 |
Carbon revenue | 147.89 | 147.89 | 147.89 |
Net revenue | 9893.74 | 10,094.9521 | 10,623.78 |
The statements, opinions and data contained in all publications are solely those of the individual author(s) and contributor(s) and not of MDPI and/or the editor(s). MDPI and/or the editor(s) disclaim responsibility for any injury to people or property resulting from any ideas, methods, instructions or products referred to in the content. |
Share and Cite
Shi, T.; Zhou, H.; Shi, T.; Zhang, M. Research on Energy Management in Hydrogen–Electric Coupled Microgrids Based on Deep Reinforcement Learning. Electronics 2024 , 13 , 3389. https://doi.org/10.3390/electronics13173389
Shi T, Zhou H, Shi T, Zhang M. Research on Energy Management in Hydrogen–Electric Coupled Microgrids Based on Deep Reinforcement Learning. Electronics . 2024; 13(17):3389. https://doi.org/10.3390/electronics13173389
Shi, Tao, Hangyu Zhou, Tianyu Shi, and Minghui Zhang. 2024. "Research on Energy Management in Hydrogen–Electric Coupled Microgrids Based on Deep Reinforcement Learning" Electronics 13, no. 17: 3389. https://doi.org/10.3390/electronics13173389
Article Metrics
Further information, mdpi initiatives, follow mdpi.
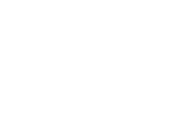
Subscribe to receive issue release notifications and newsletters from MDPI journals
Case study as a research method

- Universiti Teknologi Malaysia
Discover the world's research
- 25+ million members
- 160+ million publication pages
- 2.3+ billion citations

- CLEOPHAS OWINO
- Luis Eduardo Cañón-Ramirez

- Asma Nasser Alzahrani

- Muhbib Abdul Wahab
- Putri Amalia Farhati
- TRANSPORT POLICY
- Julien Baltazar

- Hernán Espinoza-Acero
- Tito Galarza-Minaya
- Elisabete Vidal
- Monika Belhaj

- Jacqueline Scheepers

- EDUC TECHNOL SOC

- Steven Mcdonough
- TESOL QUART
- ELLEN BLOCK
- Donald T. Campbell
- LAW SOC REV
- George I. Lovell
- Jacques Hamel
- Stéphane Dufour
- Dominic Fortin

- Recruit researchers
- Join for free
- Login Email Tip: Most researchers use their institutional email address as their ResearchGate login Password Forgot password? Keep me logged in Log in or Continue with Google Welcome back! Please log in. Email · Hint Tip: Most researchers use their institutional email address as their ResearchGate login Password Forgot password? Keep me logged in Log in or Continue with Google No account? Sign up
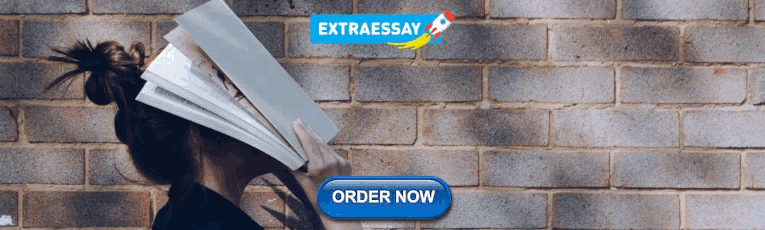
IMAGES
COMMENTS
Learn how to conduct and analyze a case study as a qualitative research method. Download the PDF article from ResearchGate and explore related topics.
The case study method is a research strategy that aims to gain an in-depth understanding of a specific phenomenon by collecting and analyzing specific data within its true context (Rebolj, 2013 ...
A case study is one of the most commonly used methodologies of social research. This article attempts to look into the various dimensions of a case study research strategy, the different epistemological strands which determine the particular case study type and approach adopted in the field, discusses the factors which can enhance the effectiveness of a case study research, and the debate ...
McMaster University, West Hamilton, Ontario, Canada. Qualitative case study methodology prov ides tools for researchers to study. complex phenomena within their contexts. When the approach is ...
Case study method is the most widely used method in academia for researchers interested in qualitative research (Baskarada, 2014).Research students select the case study as a method without understanding array of factors that can affect the outcome of their research.
ve as a brief refresher to the case study method. As a refresher, the chapter does not fully cover all the options or nuances that you might encounter when customizing your own case study (refer to Yin, 2009a, to obtain a full rendition of the entire method).Besides discussing case study design, data collection, and analysis, the refresher addr.
nfinished business that goes beyond this sixth edition. Three topics especially deserve your attention: (1) the role of plausible rival explanations, (2) case-based compared with variable-based approaches to designing and conducting case study research, and (3) the relationsh. p between case study research.
6 Understanding Case stUdy researCh The term 'case study' is, or should be, reserved for a particular design of research, where the focus is on an in-depth study of one or a limited number of cases. In practice, however, its use is rather messier and more complex: To refer to a work as a 'case study' might mean: (a) that its method is ...
How to do Better Case Studies: (With Illustrations from 20 Exemplary Case Studies) In: The SAGE Handbook of Applied Social Research Methods. By: Robert K. Yin. Edited by: Leonard Bickman & Debra J. Rog Pub. Date: 2013 Access Date: May 18, 2018 Publishing Company: SAGE Publications, Inc. City: Thousand Oaks Print ISBN: 9781412950312 Online ISBN ...
CASE STUDY RESEARCH. urt SchochInthis chapter, I provide an introduction to case. study design. The chapter begins with a definition of case study research and a description of its origins and philosophical. nderpinnings. I share dis-cipline-specific applications of case study methods and describe the appropriate research questions addressed by.
First is to provide a step-by-step guideline to research students for conducting case study. Second, an analysis of authors' multiple case studies is presented in order to provide an application of step-by-step guideline. This article has been divided into two sections. First section discusses a checklist with four phases that are vital for ...
Definition of case study. Case study method enables a researcher to closely examine the data within a specific context. In most cases, a case study method selects a small geographical area or a very limited number of individuals as the subjects of study. Case studies, in their true essence, explore and investigate contemporary real-life ...
Case study research : design and methods by Yin, Robert K. Publication date 2014 Topics Case method, Social sciences -- Research -- Methodology Publisher Los Angeles : SAGE ... Pdf_module_version 0.0.17 Ppi 360 Rcs_key 24143 Republisher_date 20220107134201 Republisher_operator [email protected] ...
Case Study Research: Principles and Practices provides a general understanding of the case study method as well as speci c tools for its successful implemen-tation. These tools are applicable in a variety of elds, including anthropology, business and management, communications, economics, education, medicine,
Thousand Oaks, CA: Sage. 282 pages. (ISBN 978-1-4522-4256-9). Reviewed by Trista Hollweck, University of Ottawa Robert K. Yin's Case Study Research Design and Methods (2014) is currently in its fifth edition and continues to be a seminal text for researchers and students engaged in case study research.
Key Words: Case Study and Qualitative Methods Introduction To graduate students and researchers unfamiliar with case study methodology, there is often misunderstanding about what a case study is and how it, as a form of qualitative research, can inform professional practice or evidence-informed decision making in both clinical and policy realms.
contemporary issue or phenomenon in a. bounded system. Case study research. requires in-depth investigation. conducted into an individual, group, or event to gain an understanding of. a real-life ...
Components of Research Designs For case studies, fIve components of a research design are especially important: 1. a study's questions, 2. its propositions, if any, 3. its unites) of analysis, 4. the logic linking the data to the propositions, and 5. the criteria for interpreting the findings. study questions.
Revised on November 20, 2023. A case study is a detailed study of a specific subject, such as a person, group, place, event, organization, or phenomenon. Case studies are commonly used in social, educational, clinical, and business research. A case study research design usually involves qualitative methods, but quantitative methods are ...
This paper presents a case study of the Assistive Technology 2030 (AT2030) funded Country Investment project in Sierra Leone. The research explored the nature and strength of the AT stakeholder network in Sierra Leone over the course of one year, presenting a snapshot of the network before and after a targeted systems level investment. Methods
1. Abstract. Qualitative case study methodology enables researchers to conduct an in-depth exploration of intricate phenomena within some. specific context. By keeping in mind research students ...
In this case, the expert may determine that public records, such as birth, death, and marriage registries, are the most likely data sources to be leveraged for identification. ... However, a covered entity's mere knowledge of these studies and methods, by itself, does not mean it has "actual knowledge" that these methods would be used ...
See Full PDFDownload PDF. Robert K. Yin. (2014). Case Study Research Design and Methods (5th ed.). Thousand Oaks, CA: Sage. 282 pages. This article examines five common misunderstandings about case-study research: (a) theoretical knowledge is more valuable than practical knowledge; (b) one cannot generalize from a single case, therefore, the ...
Methods and assessment tools varied significantly among studies, limiting comparisons between studies. Marginalized or Underrepresented Students The post-secondary population in the United States is predominately White, female, and from a higher socioeconomic status, as compared to non-college emerging adults ( Arnett, 2016 ).
This study constructed a novel decision-making framework for startup companies to evaluate token financing options. A Network structure weighting (NSW) technique was developed and integrated with the analytic network process (ANP) to create a comprehensive assessment model. This innovative approach addressed the limitations of traditional multi-criteria decision-making methods by effectively ...
describe case study as "a methodology, a type of design in qualitative research, an object of study and a product of the inquiry" (p. 245). They conclude with a
Therefore, further research involving a large-scale multi-center study is needed. In conclusion, TAC is rarely used for the treatment of CI in Japan. However, based on previous reports and our data, TAC appears to be a safe and effective method for preventing second-trimester loss and preterm delivery in high-risk patients.
Hydrogen energy represents an ideal medium for energy storage. By integrating hydrogen power conversion, utilization, and storage technologies with distributed wind and photovoltaic power generation techniques, it is possible to achieve complementary utilization and synergistic operation of multiple energy sources in the form of microgrids. However, the diverse operational mechanisms, varying ...
Case study method enables a researcher to closely examine the data within a specific context. In most cases, a case study method selects a small geograph ical area or a very li mited number. of ...